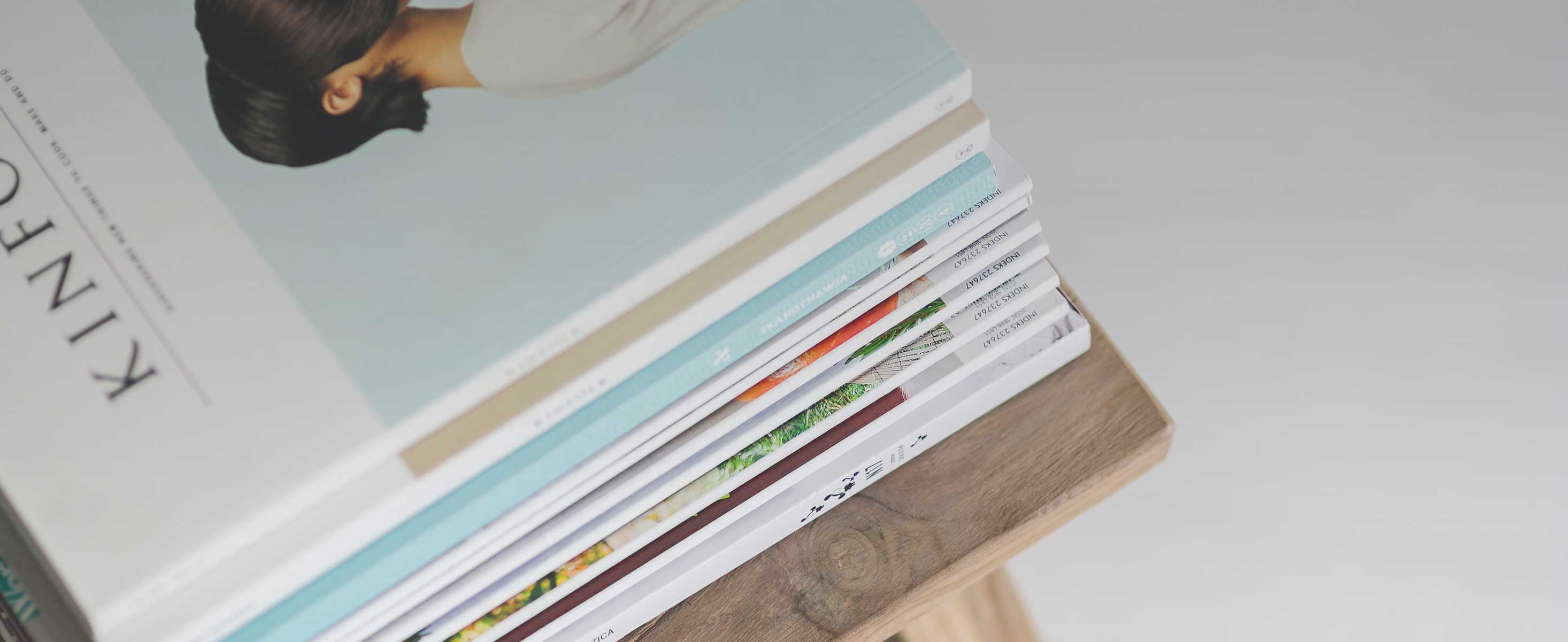
3 minute read
Bass Diffusion Model
Green Cover Planning to Go Big A This reading shows how firms can use Conjoint Analysis for customer insights.
Green Cover Planning to Go Big B This reading shows how firms can use Conjoint Analysis for customer insights.
MODCO Using Customer Complaints and Complements for Strategy Implementation
DISTRICO Executing Strategy This reading shows how firms can use voice of the customer data to obtain customer, strategy, and financial insights. This reading shows how firms can use customer satisfaction surveys to inform a customerbased strategy. Marketing, Strategy, Business, Buying Behavior, Marketing Research, Customer Analytics Marketing, Strategy, Business, Buying Behavior, Marketing Research, Customer Analytics Marketing, Strategy, Business, Buying Behavior, Marketing Research, Customer Analytics
Marketing, Strategy, Marketing Research, Customer Analytics Chapters 4, 5, 12
Chapters 4, 5, 12
Chapters 4, 5, 8, 11, 15
Chapters 4, 5, 8, 11, 15
Besides the Darden Business Publishing and C-CUBES cases, we also envision that instructors will be able to easily adjust the case study examples included in each technical chapter to create additional meaningful assignments for their students. For example, instructors could change some of the data or bring in their own datasets that have a similar format to the case study examples in the chapter. The R code and Tableau packaged workbooks could be used by the students to solve the newly developed assignments. Instructors who adopt this approach could use the cases and code included in the book for teaching purposes (i.e., teaching the respective marketing analytics technique), and then use their adjusted cases as additional (e.g., homework) assignments.
Diverse Examples
Examples are critical to making complex marketing concepts and arguments comprehensible and compelling. This book includes many diverse marketing examples, reflecting different companies and industry segments. The examples reveal how the focal marketing analytics techniques apply to various situations. In addition, the international flavor of the book is consistent with globalization trends in most industries and markets.
Putting It Together: Sample Syllabus for Marketing Analytics Course
The objective of the marketing analytics course is to show students the benefits of using a systematic and analytical approach to marketing decision making. An analytical approach will enable students to:
1. Understand how the “First Principles” of marketing strategy help firms organize the analytics opportunities and challenges in today’s data era in an overarching fashion; 2. Use and execute marketing analytics techniques to understand how to solve marketing analytics problems in a scientific and process-driven manner.
We argue that most analytic challenges facing marketing researchers, consultants, and managers could be integrated under one umbrella that comprises four fundamental marketing problems. We then emphasize how the “First Principles” of marketing strategy help solve the four fundamental marketing problems, and help students develop marketing analytics competencies pertaining to each of the four First Principles. Overall, by completing this course, students will be on their way to being able to make the return on investment case for marketing expenditures that companies are increasingly asking of their executives. Table 3 shows how a fifteen-week, semester-long marketing analytics course based on this book could be structured (not including quizzes and exams). More class syllabi examples are available on the book’s website.
Table 3 Example Outline for Marketing Analytics Course
Week Topic Book Readings/Description
1 The Nature and Scope of Marketing Analytics and Management Chapter 1
2 Data Collection and Measurement Scales & Software Installation
Chapters 2, 6, 10, & 14 R: https://cloud.r-project.org/ Tableau: https://www.tableau.com/ academic/students 3 Customer Segmentation and Targeting Chapter 3 Tool: Cluster Analysis
4 Customer Targeting Chapter 4 Tool: Discriminant Analysis
5 Competitive Positioning and Consumer Preferences Chapter 5 Tool: Perceptual and Preference Maps
6 Constructs, Questionnaire Design, and Data Dimension Reduction
Chapter 11 Tool: Factor Analysis 7 Heuristic Models for Customer Selection Chapter 7 Tool: RFM Analysis
8 Choice Models Chapter 8 Tool: Logistic Regression
9 Customer Lifetime Value for Customer Selection and Optimal Resource Allocation Chapter 9 Tool: Customer Lifetime Value
10 Understanding Consumer Preferences Chapter 12 Tool: Conjoint Analysis