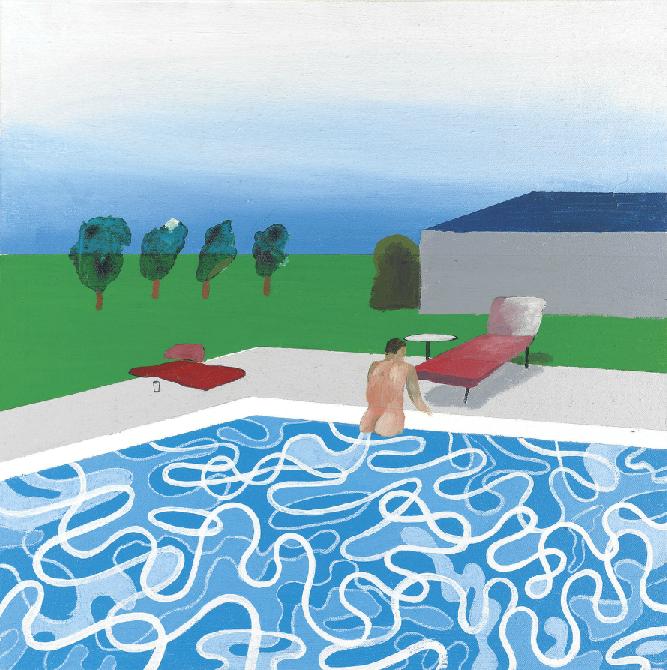
22 minute read
Feature: A deep dive into deep mindLily Pfaffenzeller
A deep dive into
DeepMind
Advertisement
Lily Pfaffenzeller delves into the development of DeepMind, the company that seems to be solving everything using artificial intelligence.
Who is the most intelligent person you know? A classmate? Curie? Einstein? Whoever you thought of, could there be someone even more intelligent? Someone so smart that they could take the problems plaguing humanity for millennia, and solve them in mere seconds? Someone so genius, that they could face the most complex enigmas of science and technology, regardless of a complete lack of training or knowledge? At first glance, this is a seemingly impossible concept: to concentrate this level of intelligence into a single locale, we would have to replicate and perfect the human mind. And yet in 2010, the company DeepMind succeeded. Well, almost. Although DeepMind’s AI systems are not 100% perfect (for now), the intelligence they have built is already redefining the world as we know it. From defeating chess grandmasters to unravelling the intricacies of protein folding, this is only the beginning of what DeepMind has to offer. Let’s dive a bit deeper into DeepMind’s past, present and future, uncovering the potential of their artificial intelligence.
In the beginning...
Around 14 years ago, an idea drifted into the mind of Demis Hassabis. It's worth noting that his mind had already borne several successes: the former child chess prodigy completed his A levels two years early, and received a Double First from Cambridge University in Computer Science. On top of that, his PhD from UCL was followed by two postdocs from Harvard and MIT (you know, the usual). Amid his education, Hassabis had also become a videogame designer, with his first simulation game “Theme Park” that he had coded at 17 becoming a multi-million sensation. But this idea would be his greatest yet: “What if you could solve intelligence, and use that to solve everything else?” he wondered. Two years later, Hassabis embarked on a mission to do exactly that. Together with fellow researcher Shane Legg and childhood friend Mustafa Sullyman, the trio began building an army of scientists, researchers and ethicists, all working with Artificial Intelligence. Thus, the company DeepMind was born.
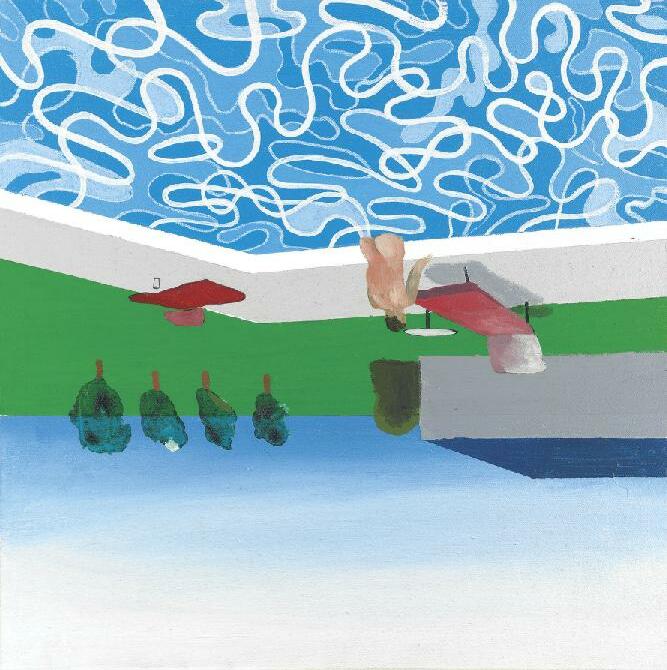
2010
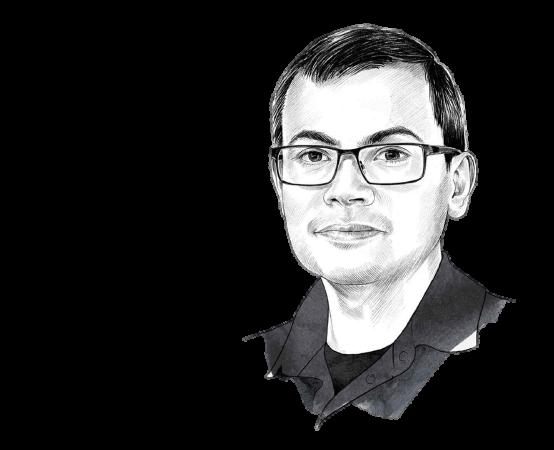
DeepMind is cofounded by Demis Hassabis, Shane Legg and Mustafa Suleyman
2014
Google buys DeepMind for around $500 million
2013
DeepMind publishes research on their AI systems that can beat Atari games
Artificial Intelligence (AI) refers to intelligence shown by machines which can be utilised, manipulated and developed to automate intellectual tasks usually performed by humans.
An illustration of Demis Hassabis by the artist Lauren Crow
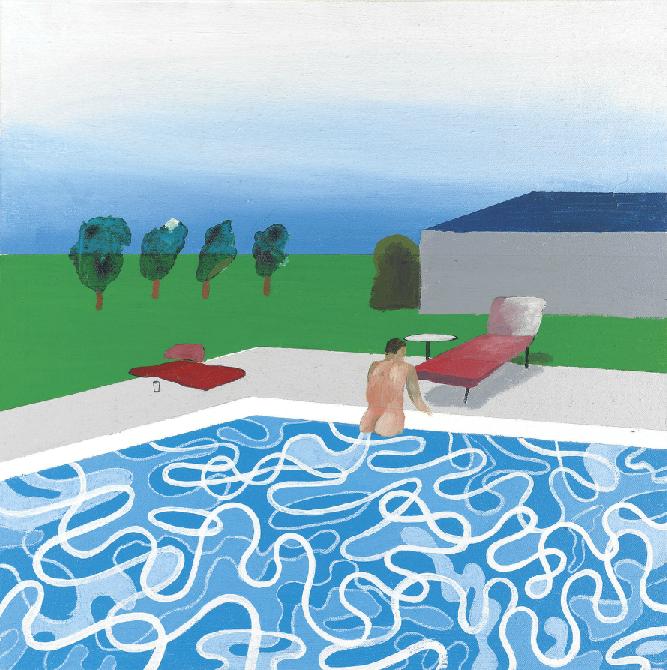
Me, myself and AGI
If you go onto DeepMind’s website today, it won’t take you long to encounter three letters: AGI, otherwise known as Artificial General Intelligence. DeepMind has directed its aim of solving intelligence towards creating an AGI - a risky move, considering its controversial past. For one, muttering “AGI” two decades ago would most likely evoke an eye roll, and even today you may simply garner a dismissive response. Julian Togelius, an AI researcher at New York University for one, likened a belief in AGI to “ a belief in magic” , though this statement seems to be ageing as well as J.K Rowling’s Twitter reputation.
As it has gathered wider acceptance, AGI has come to signify a type of Artificial Intelligence (AI) with an intelligence similar to that of a human. But if we want this system to take humanity ’s enigmas and solve them, surely the AI’s intelligence should exceed that of a human’s? To aim for such an AI could be detrimental, however, as this similarity to human cognition is crucial in allowing humans to be able to interact with it. By developing artificial intelligence that understands the world the same way we do, DeepMind ensures that we can trust the explanations that an AI may offer us. This doesn’t exactly mean that it has to “think” precisely like a human either, since there are drawbacks in attempting to exactly replicate human or animal brains. Instead, the best components are considered and given to a system. In other words, the human mind is simply an inspiration, not a replica, for the AI has to take things further in its own way. This concept first emerged in the 1950s, where scientists programmed a first generation computer to learn with the human brain as a template. Similar to the brain's neurones forming a complex network, the programme also consisted of many basic units that received inputs from connected units - just like neurones receive signals from other neurones.
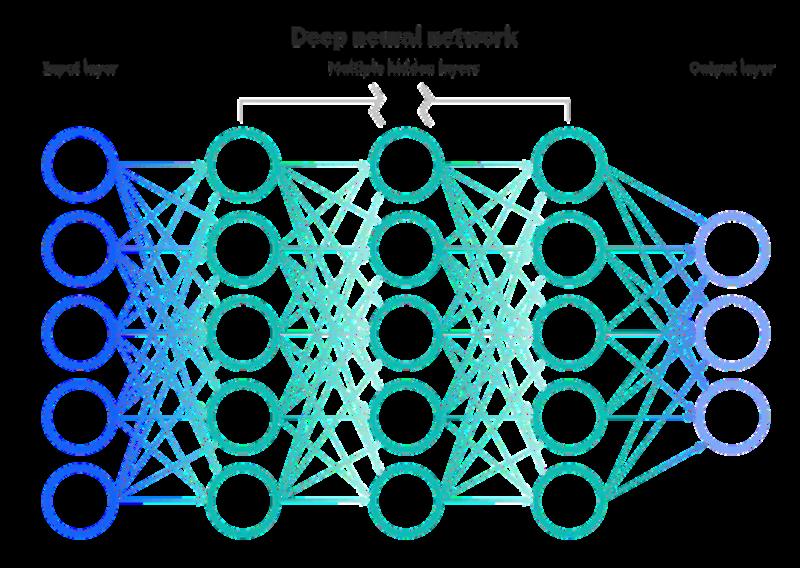
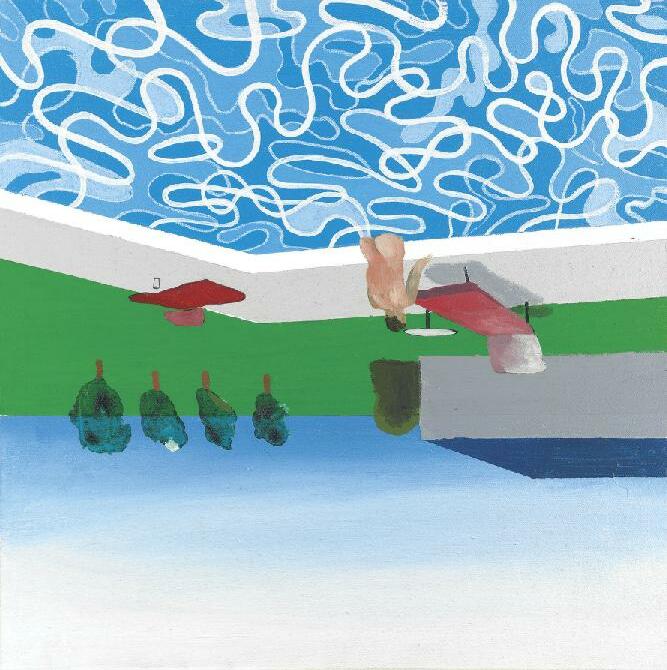
While neurones pass these signals on as electrical impulses, these units would pass the inputs on as simple calculations within hidden layers. Finally, answers would be recorded via designated output units. When many different inputs result in the same output, pathways strengthen, and the computer model “learns” .
DeepMind’s director of Neuroscience research Matt Botvinick was at the forefront of utilising and enhancing these neural networks, where he aimed to identify the aspects of our own intelligence that we could use for inspiration in building AI. It seemed that memory was a good place to start…
A neural network diagram, with the left most dark blue units representing the input layer, the middle three columns representing the hidden layers, and the light blue units on the right being the output layer.
Memory
Botvinick was particularly drawn to an aspect of memory known as replay. Replay is a phenomenon that was discovered in the medial temporal lobe within the hippocampus, where a specific pattern of neural activity suggests that past experiences are being replayed. In the Nobel winning prize work of John O’Keefe and others, it was discovered that as a rat scampered down a track, a particular pattern of neural activity would arise as it returned to travel down the same route. You can verify this by sticking some electrodes in an unfortunate rat’s hippocampus to conjure these same connections, showing a memory is being replayed.
2015
AlphaGo beats European champion Fan Hui
2020
AlphaFold's predictions achieve an accuracy score comparable to lab techniques
2019
AlphaFold “ solves” protein folding
2021
DeepMind open sources the code for AlphaFold
Afterwards, during a period of rest (such as sleep), electrical impulses are spontaneously and rapidly refired down the same neural pathway, known as a replay sequence. Scientists found that by disrupting the sequences, they ’d significantly impaired the rodent’s ability to perform a new task. Hence, O'Keefe and his team had not only realised replay's cruciality to memory, but more importantly its fundamental role in learning.
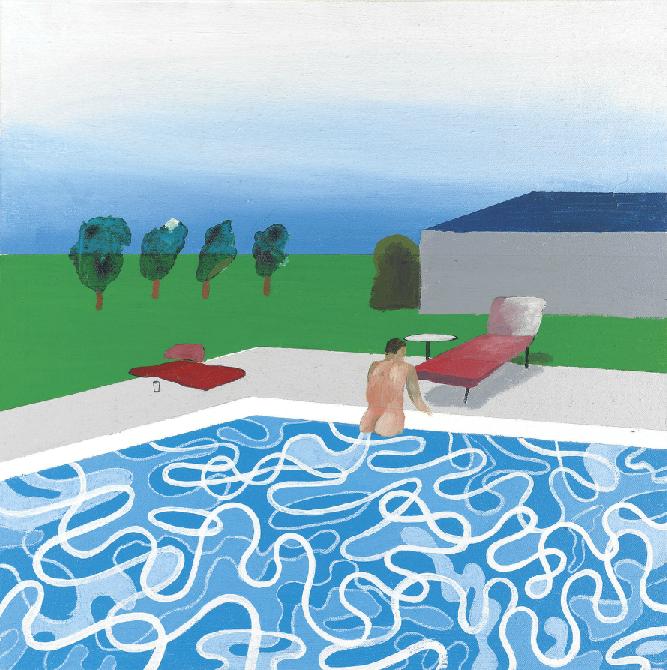
Awake
Neural Network Replay Buffer
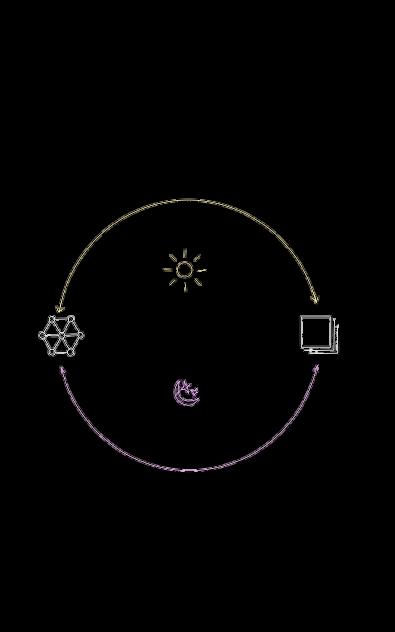
Asleep
Artificial neural networks collect experience by interacting with the environment, save that experience to a replay buffer, and later play it back to continue learning from it.
This factor wriggled its way into DeepMind’s development of AI when replay was put to the test in 2015. An Artificial Intelligence system termed “DQN” (deep Q-network) was thrown into a series of arcade games. By replaying memories of past moves, the AI could learn from its experiences and work out what sequences of moves worked well, which ones were mistakes, and find strategies that otherwise wouldn’t have been obvious. Such was the case when DQN was confronted with the game Breakout. You've probably played it before, but if it doesn't ring a bell, here's what the game entails: just break through a wall by controlling a bat to hit and direct a ball. But there was a catch: unlike the average human player, the AI had no clue what the objective was or what it was controlling. All DQN had to work with was the raw, rainbow coloured pixels of the game interface. And so, the agent began learning the game entirely by itself. When you watch the first 100 games, it's pretty obvious DQN is a rookie player: you can see from the left-hand image that the agent is pretty appalling, having missed the ball most of the time. After 300 games, DQN reaches human capabilities, grasping the bat-to-ball concept as it no longer misses the ball. 500 games later, the agent had identified an optimal strategy and began playing at the superhuman level shown on the right. The contrast between before and after couldn’t be starker. By replaying memories of past moves, the AI could learn from its experiences and deduce what sequences of moves worked well, which ones were mistakes and find strategies that otherwise wouldn’t have been obvious.
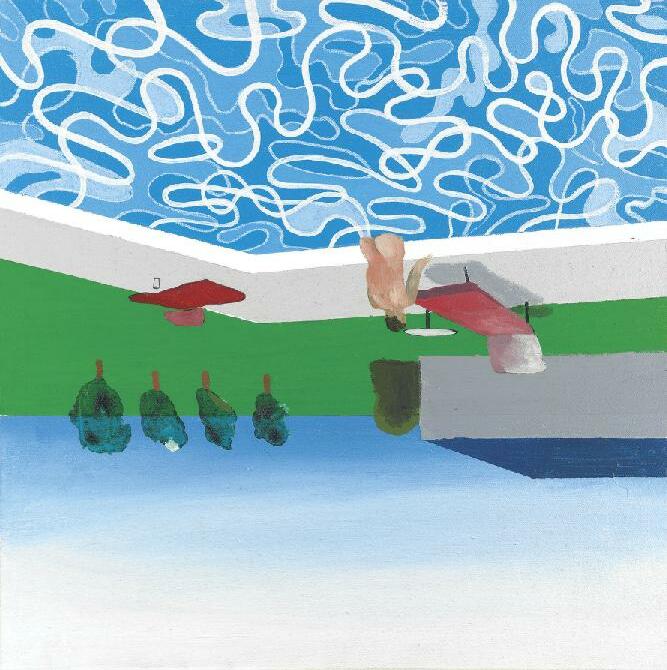
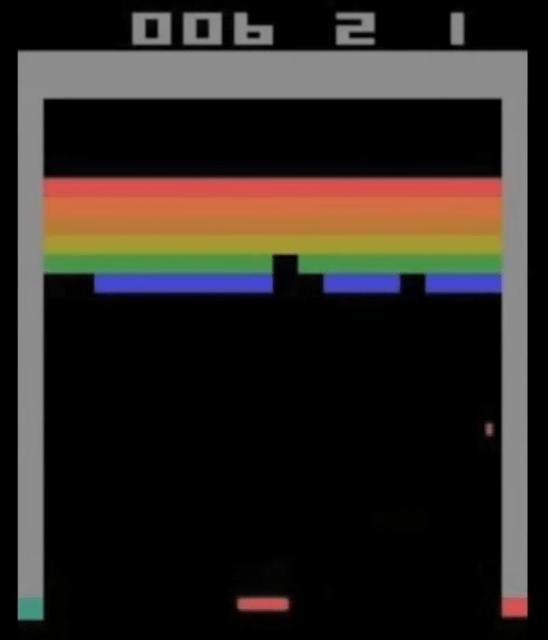
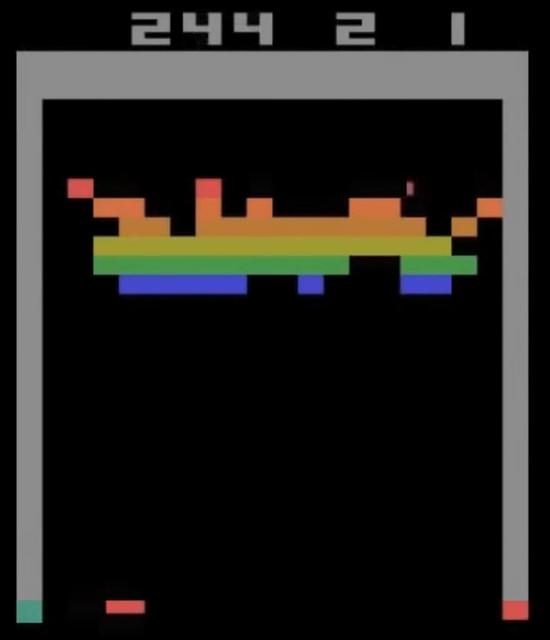
Footage taken from a video screen recording of DQN playing breakthrough. Credit: WIRED UK on youtube
Replay wasn’t only the force at work here, however. Deep reinforcement learning was also pivotal in shaping DQN.
Deep Reinforcement Learning
If you couldn’t get enough of Vanessa’s article on the Big Bang theory, then you ’ll like this next bit. Season 3, Episode 3 “The Gothowitz Deviation” sees Sheldon trying to surreptitiously alter the habits of his roommate Leonard's irritating girlfriend, Penny. After dinner, she offers to take Sheldon’s plate to the kitchen. “How thoughtful!” Sheldon exclaims. “Would you like a chocolate?” he offers. Moments later, Penny apologises for talking too loudly, proceeding to shut up; Sheldon offers her chocolate. When taking a phone call from a friend she decides to leave the room for once; Sheldon offers her chocolate. Penny agrees when Sheldon asks her and Leonard to “keep the decibel level to a minimum” at night when - well, you get the point. Leonard picks up on this too, outrageously accusing Sheldon of “ using chocolates on my girlfriend as positive reinforcement for what you consider correct behaviour!” . Indeed, this is exactly what Sheldon is doing: following each behaviour favourable to him with a reward makes it more likely that the behaviour will reoccur in the future. Similarly, DeepMind uses a method called Deep Reinforcement Learning to train its AI systems. Instead of being told directly what to do, an AI system, or agent, must find it out for itself by trial and error, just like DQN in hundreds of games of Breakout. Unlike Penny though, machines tend to lack interest in chocolate, so their reward takes the form of 1s or 0s. Thus, every time DQN hit the ball (the favourable action), it was rewarded with a signal of +1. And so, if a programme as small as DQN could learn through such methods, what was stopping DeepMind from thinking broader and bigger?
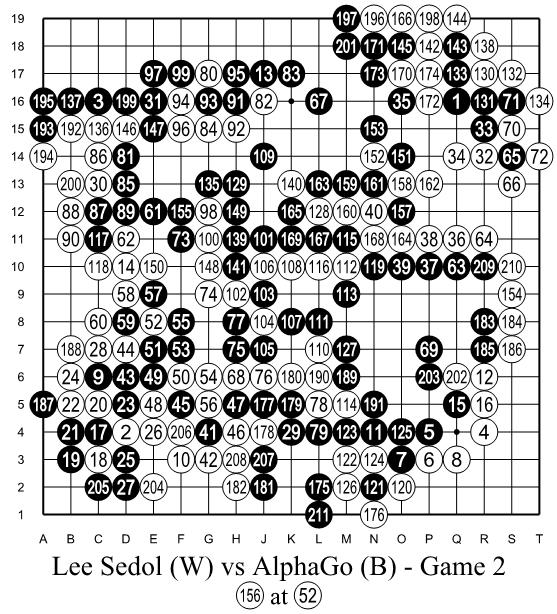
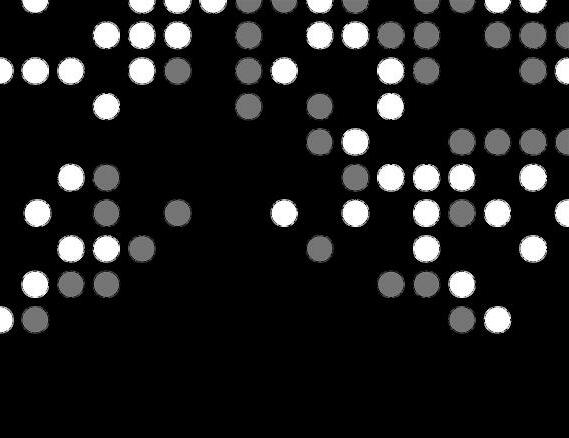
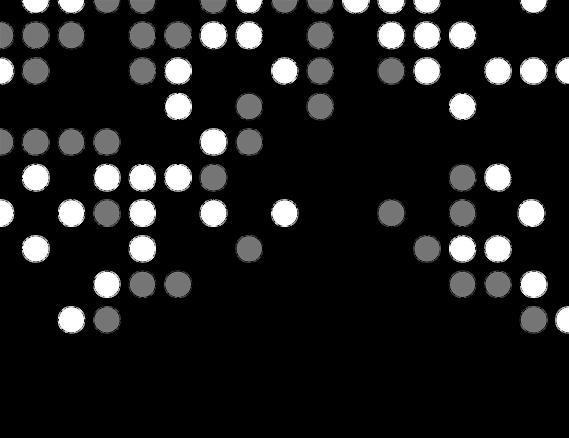
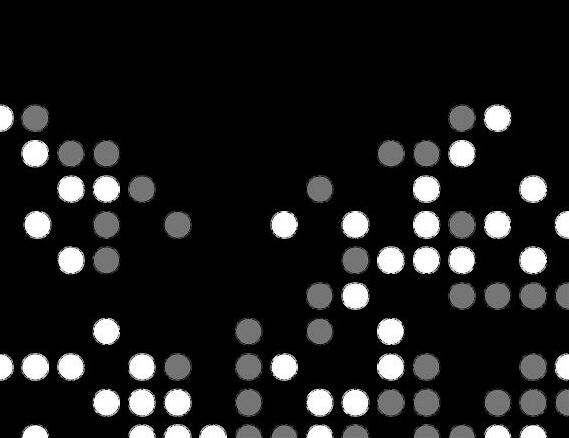
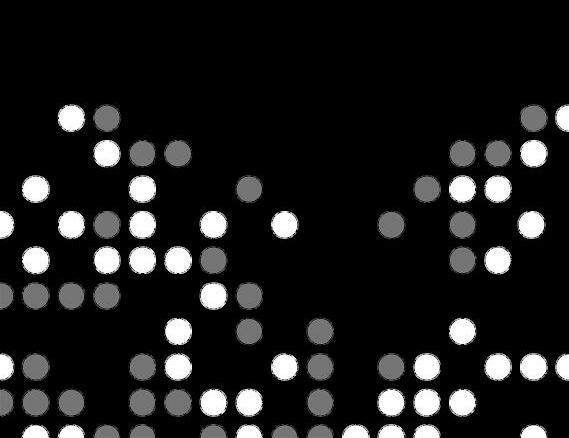
Ready, set…
In the beginning, DeepMind began training AI with the simple Atari arcade games. Within one year they jumped to training AI with the hardest: Go. Go, or Weiqi, sounds rather similar to Chess at first, but in reality is much more complex. Two players compete on a board: one takes black, the other white. Each piece is a counter, which they alternate in placing on the board. The aim? To surround the largest total area on the board by capturing their opponent’s stones.
Black's move to "a" captures the surrounded five white stones
Although these rules are massively simplified, the game is relatively easy to pick up regardless. Don’t let this deceive you, though: behind the invitingly easy façade lies a profoundly complex network of strategy, tactic and possibilities. In fact, there are more than 10170 possible board configurations - that exceeds the number of atoms in the known universe. This complexity is what cements Go’s title as the most challenging game for AI. Experts were convinced that an artificial victor of Go was yet to stroll onto the scene for another decade, if ever. All one had to do was take one look at the facts, which didn’t point towards a bright future: winning this board game required multiple layers of strategic thinking, a factor standard AI in the past fell short of. Standard AI systems, which test all possible moves and positions using a search tree, would collapse under the sheer number of possible moves while evaluating the strength of each possible board position. Knowing this, DeepMind attacked the dilemma from a new perspective. Drawing from deep reinforcement methods, a computer programme was thrown into countless games of Go.
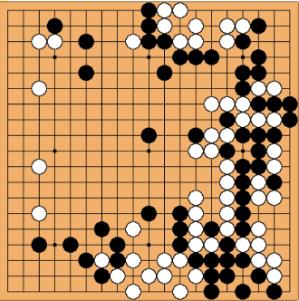
A professional game of Go in progress The AI that would soon become known far and wide as AlphaGo started small by playing games at an amateur level. Once it had gained a reasonable understanding of human play, AlphaGo went a bit Dr Jekyll and Mr Hyde, playing against different
versions of itself thousands of times. As time went on, Deep reinforcement learning methods began to take hold: as AlphaGo learned from its mistakes, the agent became increasingly stronger in both learning and decision-making. And so, AlphaGo was finally ready… “Dear Mr Fan,... as the strongest Go player in Europe, we [DeepMind] would like to invite you to our offices in London… to share with you an exciting Go project that we are working on”
In the next step of the project, Hassabis addressed the above email to professional Go player and 2013-2015 European Champion, Fan Hui. Hassabis wanted AlphaGo to play against Hui in a game of 5 rounds. “It’s just a programme, ” Hui dismissively stated when confronted with the concept. He soon realised that this was perhaps more than a mere programme when he lost the first round. In the second game, despite a valiant effort to alter his style, Hui still lost. AlphaGo triumphed once again in the third, with such consistency being maintained throughout the fourth and fifth. In its first ever game of go against a renowned champion, AlphaGo won 5-0. Hui left London that day defeated, but AlphaGo wasn’t finished just yet.
…go!
200 million people worldwide watched in anticipation as AlphaGo and legendary Go player Mr Lee Sedol came face to face in March 2016 in Seoul, South Korea. Sedol, who held the titles of 18 world championships, seemed just as unphased as the pre-game Hui in his preceding interview to the game:
Logically speaking, Sedol wasn’t thinking erroneously: he held a 9p ranking (the highest possible) in the Go professional ranking system, which massively outweighed Fan Hui’s meagre 2p. Lee Sedol even predicted a score of 5-0 or 4-1. With the whole world watching, Sedol turned out to be correct, though perhaps not in the way many predicted: the ultimate score totalled 4-1, to AlphaGo. During the games, AlphaGo played several inventive winning moves, many of which - including move 37 in game two - were so surprising that they upended hundreds of years of wisdom. As a result, AlphaGo became the first ever machine to earn a professional ranking at the 9p level. More than this though, AlphaGo’s victory Lee Sedol (W) vs AlphaGo (B) in game 2: black wins by demonstrates that the resignation. Wesalius, Lee Sedol (W) vs AlphaGo (B) capacity of AI and deep Game 2- BW, CC BY-SA 4.0 learning doesn’t just reach into the realms of games, but has implications for wider society too…
Crash course: proteins
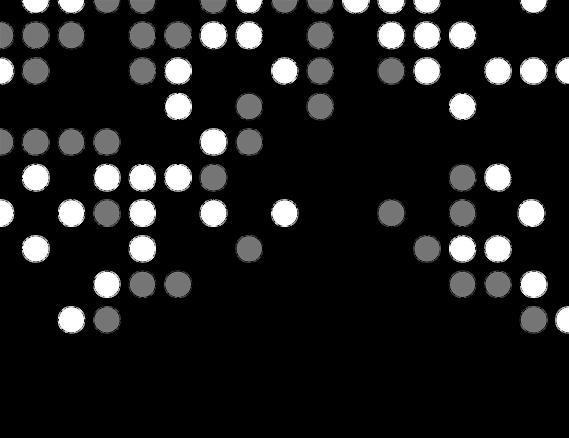
If a "universally most important molecule" competition existed, there’s no doubt that proteins would emerge victorious. Many of the world’s greatest challenges boil down to proteins and their roles, from developing treatments for diseases to finding enzymes that break down industrial waste. The field of biochemistry is dedicated to scrutinising the vast world of protein structure, and genetics similarly centres around the proteins that may arise from a genetic sequence, such as my personal favourite, ‘AAAAAAAAAAAAAAAAAAAAAAAAAA… ’ - a bunch of Adenine bases, translating into the amino acid Phenylalanine. Amino acids are the fundamental building blocks of all proteins, linked together in a linear chain known as the primary structure. Teachers love using the analogy of a necklace: if you take a string of beads, a different shape would represent a different amino acid, with the string equating to the peptide bonds holding the beads together.
This necklace then coils and ravels up in a process known as protein folding: maybe some beads are magnetic and attract or repel each other, perhaps others are sticky - either way, these beads, or amino acids, interact very specifically with each other to give rise to a very specific shape. Even a change of one amino acid can produce an entirely different protein. Christian Anfisen, the 1972 Nobel Laureate in Chemistry, postulated this in stating that a protein's amino acid sequence should fully determine its structure. If you have the amino acid’s primary structure, then you can figure out its 3D shape, right? If only nature was so simple. It would take longer than the age of the known universe to enumerate all possible configurations of a typical protein by brute force calculation, with a chap called Cyrus Levinthal estimating 10 to the 300 possible conformations a typical protein could fold into. Out of the 200 million proteins we know of (and counting), only a small fraction of the shapes have been deciphered. If one were to embark on discovering a protein’s structure, much more painstaking and expensive methods - such as X-ray crystallography - would have to be called into play. An alternative possibility of determining a protein’s larger 3D shape from just its primary structure could revolutionise structural biology. But as Levinthal pointed out, this is not so simple. Thus, the 50-year-old protein folding problem was born.
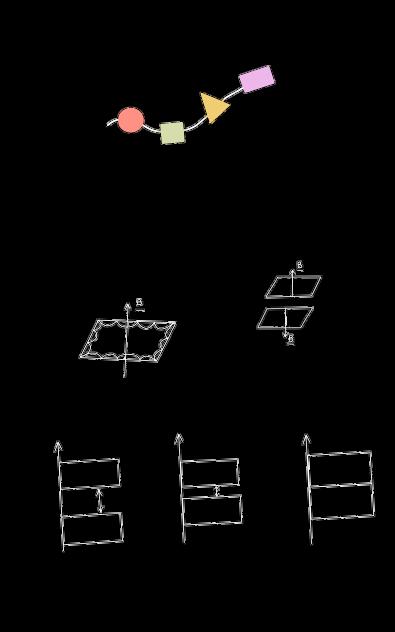
A solution unfolds
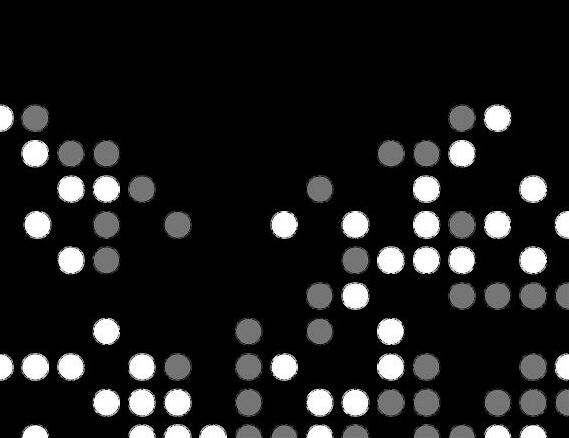
Enter DeepMind. Unsurprisingly, the company was drawn to the biological enigma because of a game called "Foldit" . The aim is in the name: gamers would fold proteins the best they could, competing against other players in an attempt to score the highest. Although players typically weren’t biochemists, some were able to find breakthrough protein structures that would go on to be published in Nature.
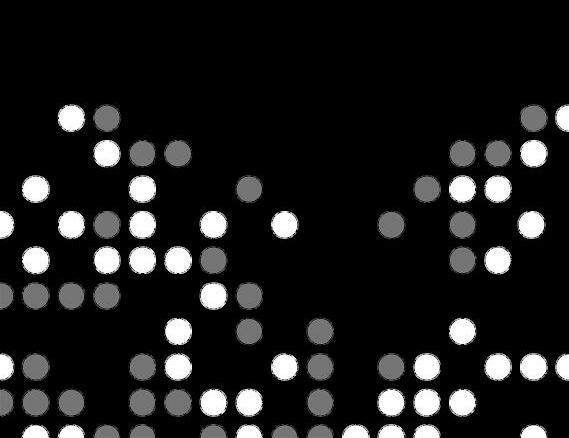
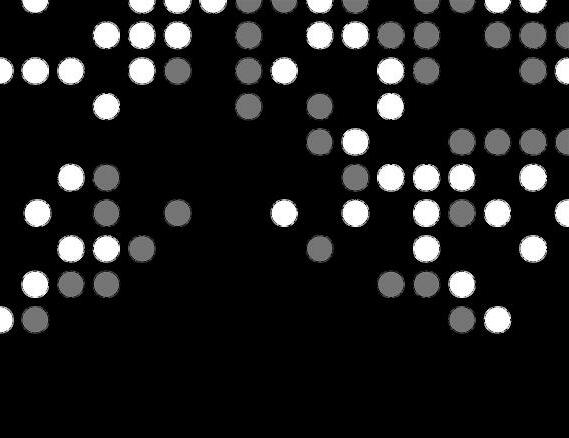
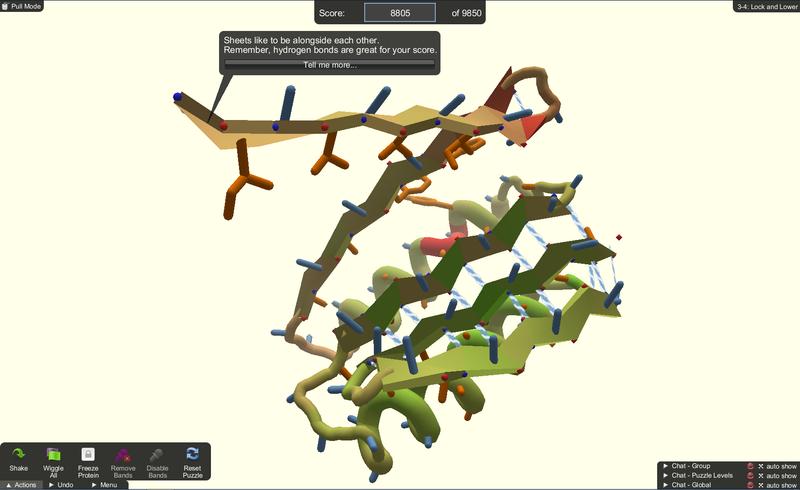
An example of a Foldit puzzle that a human can see the obvious answer to - fix the sheet that is sticking out! Credi: Foldit website
In mimicking a human Go player to create the successful AlphaGo, could DeepMind develop a similar AI to mimic human Foldit players that were folding proteins? Riding the high of AlphaGo’s successes, Demis proposed this idea to his team before they had even gotten off the flight returning from AlphaGo’s match in 2016. It wasn’t a bad idea - the protein folding problem already had ideal foundations laid out for DeepMind to begin working from: previous decades of work by experimental biologists had already determined some structures, and the CASP competition offered the perfect opportunity to put an AI to the test. The Critical Assessment Protein Structure Prediction, or CASP, is a regular global competition amongst biologists who use computers to fold proteins. One is challenged with a set of 100 protein sequences and asked to produce the 3D structure where results are compared afterwards. The accuracy of a CASP protein prediction is measured using the Global Distance Test (GDT): a visual sketch of the true folded protein is taken and the calculated competition entries are superimposed on top of it. The more amino acids that are in the correct position, the higher the score along the scale of 0-100, with 100 being an exact agreement with the experimental structure. If DeepMind could develop an AI that excelled in CASP, then they could ultimately verify that their system held the key to protein folding. And so, AlphaFold began its development. Their initial AlphaFold model involved repurposing an AI technique typically used in image analysis where the AI would work out which amino acids should be positioned close together in the final protein. As AlphaFold crunched through the different structures that could fit in a slow and highly computationally-power intensive process, it seemed to work. Thus in 2018, AlphaFold saw itself enter the CASP competition for the first time, coming out on top. However, its average GDT score of 58.9 was nowhere near close to the target of 90, which was the benchmark score that would officially deem a folded structure true. On this basis, DeepMind decided to redouble its efforts: AlphaFold2 saw the repurposed image recognition system eradicated, replaced by an AI that had been redesigned from the ground up solely to understand protein folding. The AI tool had been trained on thousands of previously solved protein structures to recognise patterns in the 3D structure based on its primary sequence. Once given a sequence, AlphaFold2 proposed a possible structure in a matter of seconds. That year in 2020, AlphaFold2 would follow in the footsteps of its older counterpart in the CASP competition. Up against 100 other groups, AlphaFold2 began folding its way through the 90 challenge sequences. A month
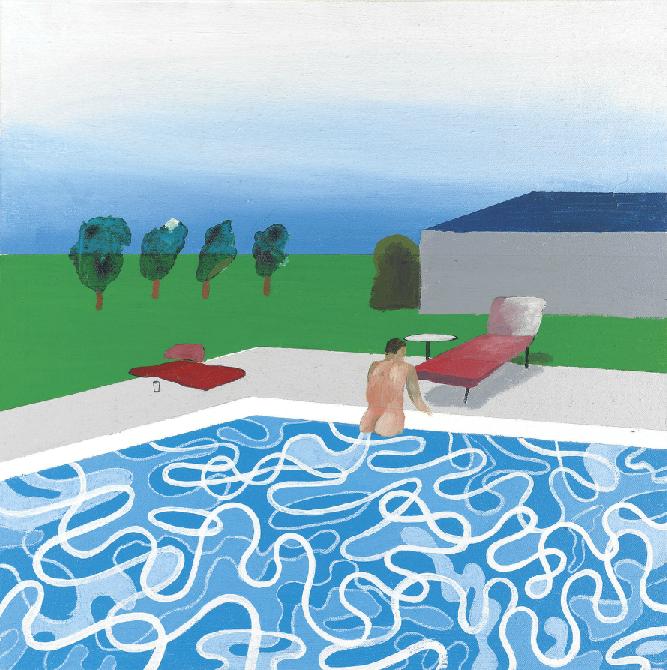
had passed by the time results chimed in: AlphaGo2 scored an average of 92.4, across all targets.
In fact, it was so precise that structures contained atomic details, achieving a level so exact that it enabled results to be used for drug design.
AlphaFold2 scored an average of 92.4 out of 100 in the 2020 CASP competition
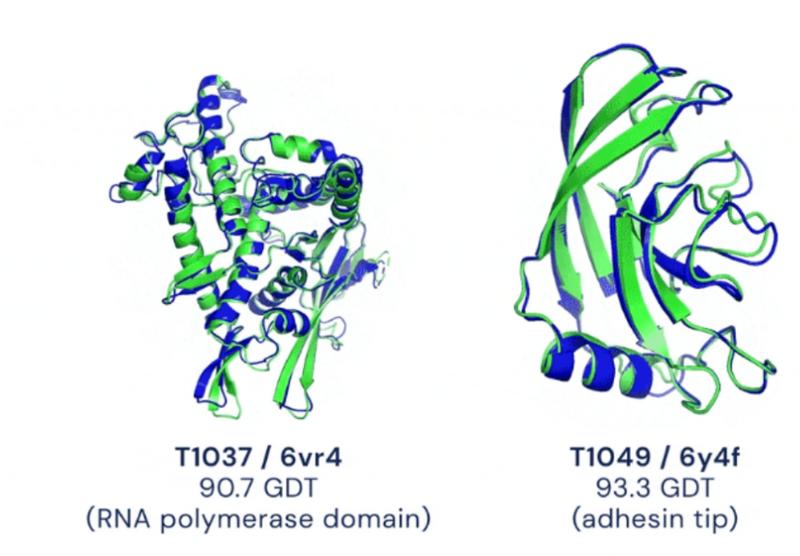
Two examples of protein targets in the free modelling category. AlphaFold predicts highly accurate structures measured against experimental result.
It didn’t take long for the news about AlphaFold’s success to reach the labs of biologists. A collaboration with another research group saw several predictions of the SARS-CoV2 virus, with two of the structures, ORF3a and ORF8 later being confirmed. A follow-up announcement in July 2021 released the source code for the tool, alongside a publicly accessible database containing predicted structures of the human proteome (our entire set of proteins). However, AlphaFold has only made a sizable dent in the world of structural biology. The protein folding problem has yet to be officially solved, with DeepMind’s AI still falling short in several significant areas: predictions falter in accuracy when it comes to intrinsically disordered regions in proteins (areas that lack a defined structure due to their flexibility). AlphaFold also has yet to explore dimeric proteins, which are a type of protein whose structures change depending on their interactions with other proteins and molecules. Who knows… perhaps in a few years a new and improved AlphaFold3 may enter the protein folding scene.
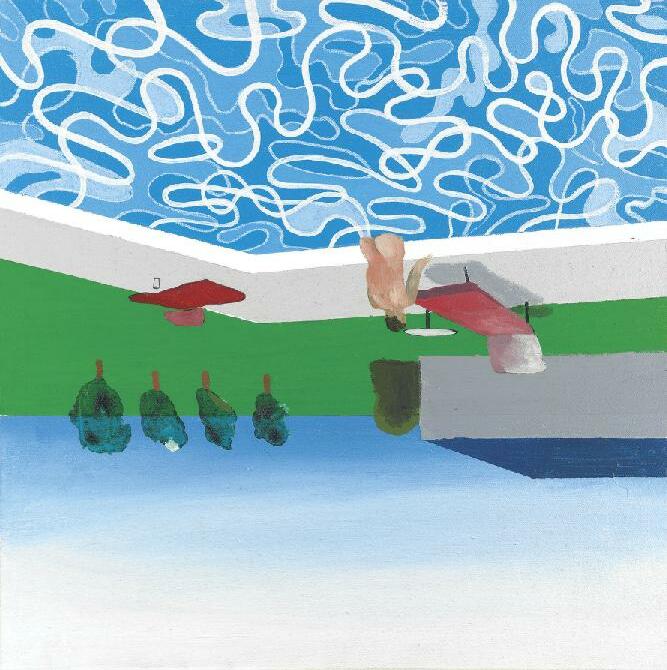
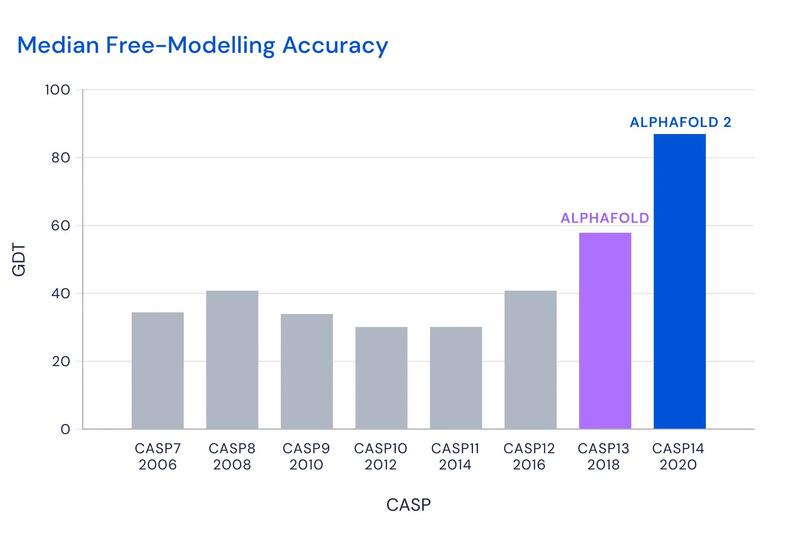
Improvements in the median accuracy of predictions made by AlphaFold2 compared to AlphaFold and previous winning scores of the CASP competition. Credit: DeepMind<https://assets-global.websitefiles.com/621e749a546b7592125f38ed/62277c4c42bcdc0bcf269b11 _
The ethical elephant in the room
Unlike this article, which has conveniently left the rather messy topic of ethics until the last minute, ethical concerns punctuate each of DeepMind’s milestones.
This is scrawled across their website section dedicated solely to ethics. Indeed, with great power comes great responsibility (to quote Spiderman): this new and novel era of Artificial Intelligence is unfolding rapidly, stirring up unease amongst the excitement. Beyond the confines of the DeepMind headquarters, how might AI be implemented, used and abused in society? Technology at heart is relatively neutral: a machine itself can’t be bad or good - it falls upon how others use it. Consider the GPS, for example. It was invented to launch nuclear missiles in the past, but you ’ll now find them being used by Uber drivers. In a similar way, could DeepMind’s systems also have a hidden dark side?
Having already anticipated a handful of risks, the company signed public pledges against them, such as the use of lethal autonomous weapons. Additionally, DeepMind’s designated team of ethicists and policy researchers have become intrinsic to the AI research team, keeping a close eye on how technological advances may impact society, identifying and further reducing risk. With their ethics team on board, hopefully it’ll be some time before we’re launched into an AI-dominated dystopia, if ever.
“We want AI to benefit the world, so we must be thoughtful about how it’s built and used. ''
"I think artificial intelligence is like any powerful new technology...it has to be used responsibly. If it's used irresponsibly it could do harm.
Demis Hassabis
Back to the surface
DeepMind is a world of its own. Honestly speaking, this was barely a “deep dive” into DeepMind, but rather a casual paddle in this vast sea of innovation. I highly recommend exploring their website, or their Spotify podcast (presented by Hannah Fry) to learn about these discussed concepts in more depth. From the potential to combat climate change to cancer, keep a close eye on DeepMind for now: who knows what technologies will emerge from their labs, or when. But when it does, you won’t want to miss it.