AREA VICE PRESIDENT & GENERAL MANAGER OF DATAIKU META
HOW DATAIKU IS UNLOCKING THE POWER OF EVERYDAY AI ACROSS ORGANIZATIONS
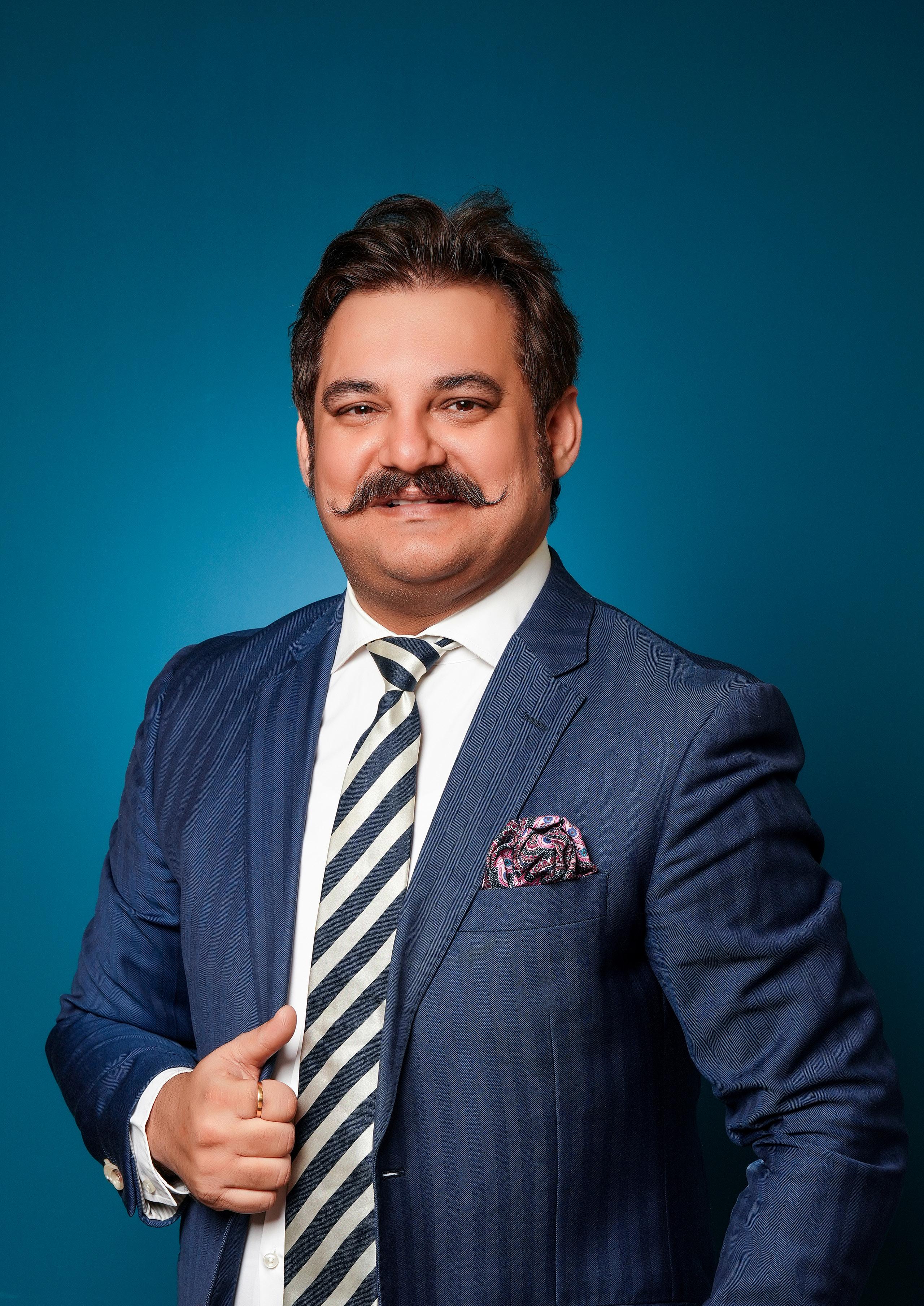
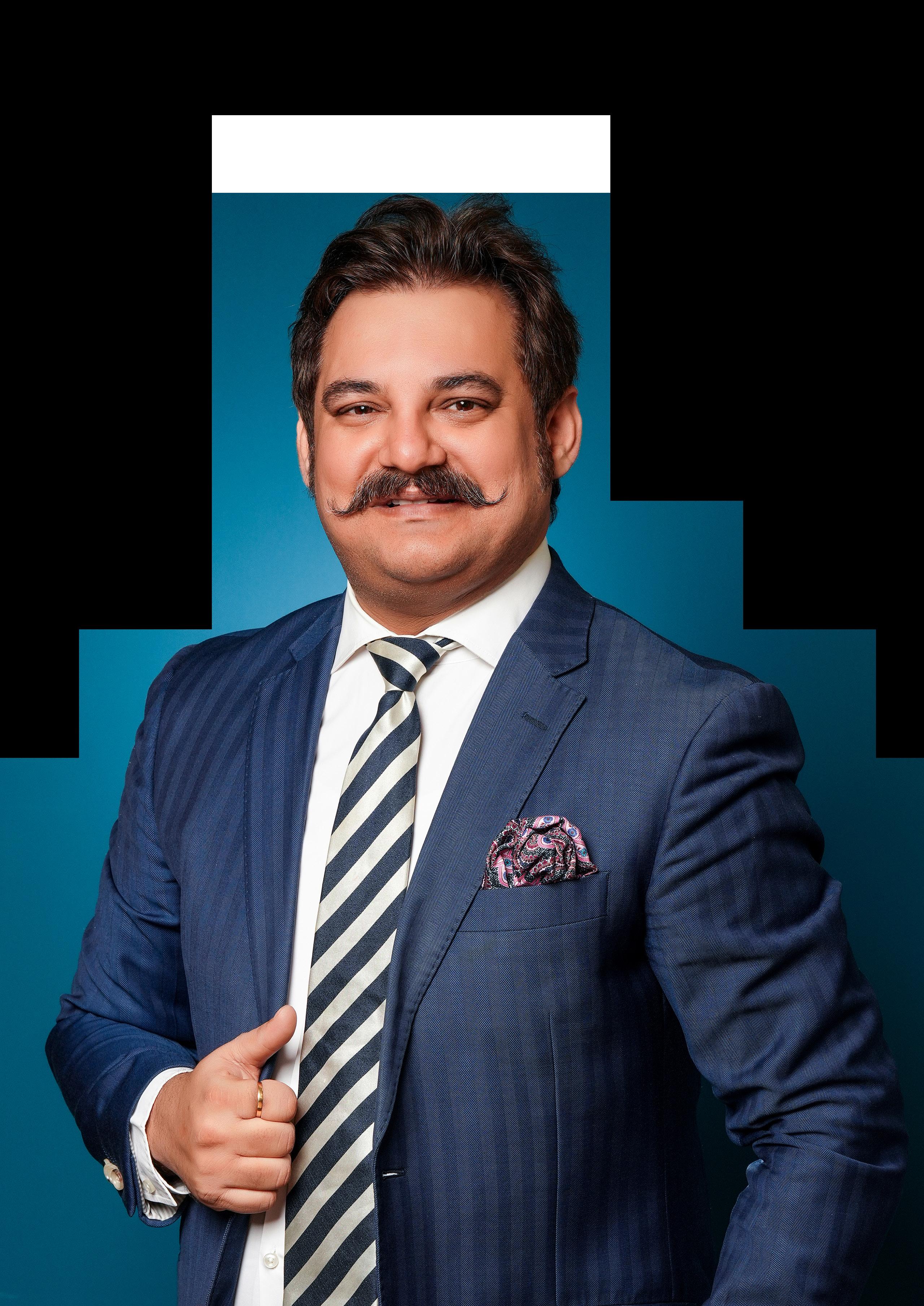
AREA VICE PRESIDENT & GENERAL MANAGER OF DATAIKU META
HOW DATAIKU IS UNLOCKING THE POWER OF EVERYDAY AI ACROSS ORGANIZATIONS
Agentic AI is the latest buzzword in the world of artificial intelligence, and this time, the buzz is justified. By combining the versatility of LLMs with traditional programming, agentic AI promises to revolutionize how tasks are automated and executed. Gartner describes agentic AI as digital agents capable of decision-making and autonomous task execution.
These agents can assist in lead qualification, monitor critical infrastructure, and make real-time decisions. They operate with memory, planning, and sensing capabilities, potentially reducing reliance on traditional websites and applications.
Some signs this trend is gaining momentum include vendors announcing “cognitive behavior” capabilities in their software. For instance, Microsoft and Salesforce have introduced CRM agents that can perform tasks on behalf of users.
What sets agentic AI apart is its dual capability to learn from user behavior and act independently. These systems not only draw from static databases and pre-trained models but also dynamically incorporate real-time interactions and insights. This continuous learning process empowers agentic AI to handle multifaceted, multistep tasks that were previously deemed too complex for automation. For example, an AI agent could autonomously draft a legal document, seek input from a user, refine it based on feedback, and even integrate relevant updates from external databases, all without human intervention at each step.
The implications of agentic AI for modern organizations are profound. However, as AI agents expand, ethical governance becomes crucial. Organizations must ensure transparency, avoid bias, and establish trust in these autonomous systems. This is why, as Gartner highlights, it is imperative for enterprises to invest in AI governance platforms to ensure model transparency, traceability, and ethical compliance. These platforms help organizations mitigate risks like bias, promote fairness, and prevent reputational harm. Given the increasing regulatory landscape, AI governance will soon be as vital as cybersecurity.
Agentic AI is more than just a technological milestone; it is a paradigm shift. For businesses and individuals alike, understanding and leveraging agentic AI will be key to staying ahead in an increasingly automated world. As this technology continues to evolve, one thing is clear: agentic AI is not just the future of AI—it is the future of work itself.
JEEVAN THANKAPPAN
06
Abu Dhabi launches Hub71+ for AI innovation. Hub71 has launched Hub71+ AI, a specialist ecosystem designed to support startups harnessing crosssector AI innovation.
12 AI double agent dilemma. Lakshmi Hanspal, Chief Trust Officer at DigiCert, on balancing Generative AI’s opportunities and risks.
20 Can AI safeguard data privacy?
Nada Khalil, cyber trust advisory consultant at Help AG, discusses AI’s impact on data privacy.
32 The Quantum Leap. Dr. Sana Amairi-Pyka from TII discusses the role of Quantum communication.
34 The foundation of AI. Cesar Cernuda, President of NetApp, on why AI success hinges on intelligent data infrastructure.
36 How long the AI wave will last?
Janus Henderson,Portfolio Manager at Alison Porter, shares investor outlook for 2025.
Our North Star Council serves as the editorial guiding light of the AI Times, providing strategic direction and ensuring our content remains on the cutting edge of AI innovation.
Dr. Jassim Haji President of the International Group of Artificial Intelligence
Venkatesh Mahadevan Founding Board Member CAAS
Jayanth N Kolla Founder & Partner Convergence Catalyst
Idoia Salazar Founder & President OdiseIA
If you would like to be a part of our North Star Council, please reach out to us at jeevan@gecmediagroup.com
PUBLISHER TUSHAR SAHOO tushar@gecmediagroup.com
CO-FOUNDER & CEO RONAK SAMANTARAY ronak@gecmediagroup.com
MANAGING EDITOR Jeevan Thankappan jeevan@gcemediagroup.com
ASSISTANT EDITOR SEHRISH TARIQ sehrish@gcemediagroup.com
GLOBAL HEAD, CONTENT AND STRATEGIC ALLIANCES ANUSHREE DIXIT anushree@gecmediagroup.com
CHIEF COMMERCIAL OFFICER RICHA S richa@gecmediagroup.com
PROJECT LEAD JENNEFER LORRAINE MENDOZA jennefer@gecmediagroup.com
SALES AND ADVERTISING sales@gecmediagroup.com
Content Writer KUMARI AMBIKA
IT MANAGER VIJAY BAKSHI
DESIGN TEAM CREATIVE LEAD AJAY ARYA
SR. DESIGNER SHADAB KHAN DESIGNERS
JITESH KUMAR, SEJAL SHUKLA
PRODUCTION
RITURAJ SAMANTARAY S.M. MUZAMIL
PRODUCTION CIRCULATION, SUBSCRIPTIONS info@gecmediagroup.com
DESIGNED BY
SUBSCRIPTIONS info@gecmediagroup.com
PRINTED BY Al Ghurair Printing & Publishing LLC. Masafi Compound, Satwa, P.O.Box: 5613, Dubai, UAE
(UAE) Office No #115 First Floor , G2 Building Dubai Production City Dubai, United Arab Emirates Phone : +971 4 564 8684
(USA) 31 FOXTAIL LAN, MONMOUTH JUNCTION, NJ - 08852 UNITED STATES OF AMERICA Phone :+ 1 732 794 5918
Hub71 has launched Hub71+ AI, a specialist ecosystem designed to support startups harnessing cross-sector AI innovation. Launched during Abu Dhabi Finance Week (ADFW), the new specialist ecosystem provides AI startups with the necessary infrastructure and resources to thrive in a rapidly evolving global economy. The initiative underscores Abu Dhabi’s commitment to advancing AI across priority sectors, aligning with the UAE capital’s
broader economic vision.
Between 2021 and 2023, the number of AI companies in Abu Dhabi increased at a compound annual growth rate (CAGR) of 67%. Furthermore, studies indicate that, on average, one AI company was established every two days in Abu Dhabi during the first half of 2024. Hub71+ AI is set to accelerate this momentum by supporting startups and developing the infrastructure required to drive AI adoption across various sectors.
AI71 joins as an anchor partner of Hub71+ AI and will offer compute power credits for their API Hub, which provides pay-as-you-go access to the globally ranked Falcon series of Large Language Models and other tools. AI71 will also provide access to their team of AI researchers.
In addition, Core42 joins Hub71+ AI as an anchor partner, empowering regional startups with preferential access to Core42’s advanced products and solutions. Core42 will offer startups cloud credits that can leverage the deployments of next-generation digital infrastructure for AI including current deployments of training and inference capacity in the UAE, USA, and a growing global footprint.
Additionally, Core42’s Sovereign Public Cloud offering which leverages Microsoft Azure, can be utilized for startups that are in need to offer a sovereign implementation for regulated industries and the public sector.
AppliedAI has revealed the first results of its Opus 1 Alpha Large Work Model (LWM) and Work Knowledge Graph (WKG), which has outperformed all evaluated Large Language Models (LLMs) in complex workflow generation and optimization across key benchmarks. The Opus LWM powers AppliedAI’s new automation platform Opus.com, developed in Abu Dhabi, now being deployed to AppliedAI’s foundational customers.
Opus.com is a first-of-its-kind generative AI workflow platform
to automate critical processes in regulated industries such as banking, healthcare, life sciences and insurance to reduce operating costs and eliminate human error. Opus workflows deliver supervised automation outcomes ensuring more consistent, highly auditable and transparent processes in regulated industries.
Opus allows users to create industrial-grade businesstailored workflows in less than 15 minutes for the majority of complex business processes.
Inception, a G42 company specializing in AI-native products, in collaboration with the Mohamed bin Zayed University of Artificial Intelligence (MBZUAI) today announced the launch of AraGen Leaderboard, a framework designed to redefine the evaluation of Arabic Large Language Models (LLMs). Powered by the new internally developed 3C3H metric, this framework delivers a transparent, robust, and holistic evaluation system that balances factual accuracy and usability, setting new standards for Arabic Natural Language Processing (NLP).
Serving over 400 million Arabic speakers worldwide, the AraGen Leaderboard addresses critical gaps in AI evaluation by offering a meticulously constructed evaluation dataset tailored to the unique linguistic and cultural intricacies of the Arabic language and region. The dynamic nature of this leaderboard tackles challenges such as benchmark leakage, reproducibility issues, and the absence of holistic metrics to evaluate both core knowledge and practical utility.
The introduction of generative tasks represents a groundbreaking advancement for Arabic LLMs, offering a new dimension to the evaluation process. Unlike traditional leaderboards that primarily focused on static, likelihood accuracy-based benchmarks, which fail to capture realworld performance, AraGen’s Leaderboard addresses these limitations.
“The AraGen Leaderboard redefines Arabic LLM evaluation, setting a new standard for fairness, inclusivity, and innovation,” said Andrew Jackson, CEO of Inception. “By addressing the gaps in previous benchmarks and introducing generative tasks, the platform empowers researchers, developers, and organizations to create culturally aligned AI technologies.
Tealium, an independent customer data platform (CDP), has entered into a global multi-year Strategic Collaboration Agreement (SCA) with Amazon Web Services (AWS). This partnership highlights Tealium’s investment in the Middle East and Africa (MEA) region, particularly the United Arab Emirates by aligning with regional data residency regulations and supporting the nation’s digital transformation initiatives.
By leveraging AWS’s secure and scalable cloud infrastructure, Tealium empowers enterprises in the MEA region to optimize AI-driven data collection, management, and activation solutions. This collaboration enables businesses to seamlessly access Tealium’s real-time CDP, ensuring compliance with local data privacy and residency requirements.
“As we expand our presence in the MEA region, we are committed to enabling enterprises to harness the power of AI-driven data strategies while maintaining the highest standards of data privacy and security,” said Robert Coyne, Senior Vice President and Managing Director for Tealium EMEA. “This collaboration highlights our dedication to innovation and customer success, supporting businesses in achieving hyper-personalization and enhanced customer engagement.”
AIQ has entered a strategic partnership with World Wide Technology (WWT), a global leader in technology solutions and services, to fast-track the adoption of artificial intelligence (AI)-powered innovations across the global energy industry.
The partnership between AIQ and WWT is designed to unlock new opportunities in the energy sector by advancing the development, scalability, and application of AI solutions at every stage of deployment. By streamlining the transition from concept to large-scale implementation, this collaboration will maximize both reach and impact across energy operations.
Magzhan Kenesbai, Acting Managing Director at AIQ commented, “Partnering with World Wide Technology enables us to accelerate our shared vision of driving impactful AI innovation within the energy sector. This collaboration reaffirms our commitment to empower the industry with practical, scalable AI solutions that
drive operational efficiency and increase productivity across the entire value chain, while aligning with our sustainability objectives.”
Together, AIQ and WWT will also work to expand the infrastructure and resources dedicated to AI innovation in the energy sector aiming to deliver comprehensive endto-end solutions. Their AI Lab as a Service program will democratize access to compute infrastructure and AI talent across the energy sector by delivering sandbox environments for proof of concepts, and piloting advanced AI systems in record time.
Positive Technologies has released an in-depth report examining the potential use of artificial intelligence in cyberattacks. According to the report, AI could eventually be used by attackers across all tactics outlined in the MITRE ATT&CK matrix and in 59% of its techniques.
Experts highlight that within a year of ChatGPT-4’s release, the number of phishing attacks increased by 1,265%, and they predict AI will
continue to enhance the capabilities of cybercriminals.
Analysts believe that, amidst the rapid development of such technologies, developers of language models don’t do enough to protect LLMs from being misused by hackers generating malicious texts, code, or instructions. This oversight could contribute to a surge in cybercrime. And simplify the execution of attacks.
Google has introduced “Willow,” a groundbreaking quantum chip poised to drive innovation and real-world applications in quantum computing. According to the company, Willow addresses common error issues in quantum computing, offering seamless performance. Developed over more than a decade, the chip is a critical step in Google’s long-term quantum roadmap. Traditionally, quantum computing faces higher error rates as the number of qubits increases. However, a recent paper published in Nature reveals that Willow defies this trend, reducing errors by 50%. This breakthrough marks a significant leap forward in making quantum computing more reliable and practical for future technologies.
Ericsson and Mobily in KSA have successfully completed a trial to explore Ericsson’s Artificial Intelligence (AI)powered 5G uplink interference Optimizer. This initiative aims to enhance 5G mobile uplink performance, delivering superior connectivity and improved user experiences across Mobily’s network. Performed with the Uplink Interference Optimizer module, part of the Ericsson Cognitive Software portfolio, the trial demonstrated that the proportion of transmissions conducted under highquality conditions has increased by 80%, which results in spectral efficiency and throughput enhancement.
Amazon Web Services has announced new innovations for Amazon Bedrock, a fully managed service for building and scaling generative artificial intelligence (AI) applications with high-performing foundation models.
Amazon Bedrock provides customers with the broadest selection of fully managed models from leading AI companies, including AI21 Labs, Anthropic, Cohere, Meta, Mistral AI, and Stability AI.
CODE81 has announced the launch of its Riyadh office, marking a key milestone following its strategic spin-off from Gulf Business Solutions (GBS). This move allows CODE81 to sharpen its focus on delivering advanced digital transformation solutions tailored to Saudi Arabia’s needs, aligning closely with the ambitious goals of Vision 2030.
The spin-off empowers CODE81 to hone its focus on delivering advanced technology solutions tailored to the Kingdom’s unique needs. Building on a legacy of expertise cultivated under GBS, the company combines innovative capabilities in data analytics, artificial intelligence (AI), low-code application development and automation with a localized approach to serve Saudi Arabia’s public and private sectors effectively.
The Riyadh office represents not only a physical expansion but also a strategic evolution that enhances CODE81’s ability to meet the growing demand for AI and application development solutions in the Kingdom. By leveraging emerging technologies, CODE81 aims to empower organizations across the Kingdom to achieve their digital transformation objectives and remain competitive in a rapidly evolving global market.
Nader Paslar, General Manager at CODE81, said, “Our spin-off from GBS and the launch of our Riyadh office reflect our confidence in Saudi Arabia’s dynamic growth potential. By focusing on the Kingdom, we aim to not only deliver world-class digital solutions but also create meaningful opportunities for local talent. This expansion reinforces our commitment to Saudi Vision 2030 and to supporting the Kingdom’s transformation into a global hub for technology and innovation.”
Pure Storage has announced the expansion of its AI solutions with the new Pure Storage GenAI Pod, a full-stack solution providing turnkey designs built on the Pure Storage platform. Organizations can use the Pure Storage GenAI Pod to accelerate AI-
powered innovation and reduce the time, cost, and specialty technical skills required to deploy generative AI (GenAI) projects. Pure Storage also announced the certification of FlashBlade//S500 with NVIDIADGX SuperPOD, accelerating enterprise AI deployments with Ethernet compatibility.
Companies today face significant challenges deploying GenAI and retrievalaugmented generation (RAG) in private clouds. This includes navigating the complexity of deploying hardware, software, foundational models, and development tools that power GenAI workloads in a timely and cost-effective manner. At the same time, they need a single, unified storage platform to address all of their storage needs, including the most critical challenges and opportunities posed by AI.
The Pure Storage GenAI Pod, built on the Pure Storage platform, includes new validated designs that enable turnkey solutions for GenAI use cases that help organizations solve many of these challenges. Unlike most other full-stack solutions, the Pure Storage GenAI Pod enables organizations to accelerate AI initiatives with one-click deployments and streamlined Day 2 operations for vector databases and foundation models. With the integration of Portworx, these services provide automated deployments of NVIDIA NeMo and NIM microservices through the NVIDIA AI Enterprise software platform, as well as the Milvus vector database, while further simplifying Day 2 operations.
The Technology Innovation Institute (TII), a leading global applied research center under Abu Dhabi’s Advanced Technology Research Council (ATRC), has unveiled Falcon 3, the latest iteration of its open-source large language model (LLM) series. This
groundbreaking release sets new performance standards for small LLMs and democratizes access to advanced artificial intelligence by enabling the model to operate efficiently on light infrastructures, including laptops. Falcon 3 introduces superior reasoning and
enhanced fine-tuning capabilities, making it a more powerful and usable AI model.
Falcon 3 is designed to democratize access to highperformance AI, offering models that are both powerful and efficient.
Eighty-four percent of enterprise CIOs believe Artificial Intelligence (AI) will be as significant to their businesses as the rise of the internet. However, only 11% say they’ve fully implemented the technology, citing an array of technical and organizational challenges, led by security and data infrastructure, that must be overcome first. The data, which comes from a new Salesforce survey of 150 verified CIOs of companies with 1,000 or more employees, offers a snapshot of the state of enterprise AI, along with the hurdles ahead that must be addressed as companies pursue their AI strategies.
Key findings include:
• CIOs feel pressure to be AI experts. Sixty-one percent of CIOs feel they’re expected to know more about AI than they do, and their peers at other companies are their top sources of information.
• CIOs agree that AI is a game changer, but are cautious. Eighty-four percent of CIOs believe AI will be as significant to businesses as the internet, but 67% are taking a more cautious approach compared to other technologies.
• IT is focusing on data initiatives before leaning into AI. CIOs report spending a median of 20% of their budgets on data infrastructure and management, versus 5% on AI. Security or privacy threats and a lack of trusted data rank as CIOs’ biggest AI fears.
• Business partners must examine their AI timelines. Sixty-six percent of CIOs believe they’ll see return on investment (ROI) from AI investments, but 68% believe their line-of-business stakeholders have unreasonable expectations for when that ROI will occur.
• CIOs see a mismatch between departments when it comes to AI. While functions like customer service are seen as having the most AI use cases, they may be perceived as being the least prepared for the technology.
Amazon has introduced Amazon Nova, a new generation of foundation models (FMs) that have state-of-the-art intelligence across a wide range of tasks, and industryleading price performance. Amazon Nova models will be available in Amazon Bedrock, and include: Amazon Nova Micro (a very fast, text-to-text model); and Amazon Nova Lite, Amazon Nova Pro, and Amazon Nova Premier (multimodal models that can process text, images, and videos to generate text). Amazon also launched two additional models – Amazon Nova Canvas (which generates studioquality images) and Amazon Nova Reel (which generates studio-quality videos).
“Inside Amazon, we have about 1,000 generative AI applications in motion, and we’ve had a bird’s-eye view of what application builders are still grappling with,” said Rohit Prasad, SVP of Amazon Artificial General Intelligence. “Our new Amazon Nova models are intended to help with these challenges for internal and external builders, and provide compelling intelligence and content generation while also delivering meaningful progress on latency, cost-effectiveness, customization, Retrieval Augmented Generation (RAG), and agentic capabilities.”
Amazon Nova includes four state-of-the-art models. The first, Amazon Nova Micro, is a text-only model that delivers the lowest latency responses at very low cost. The next three are: Amazon Nova Lite, a very low-cost multimodal model that is lightning fast for processing image, video, and text inputs; Amazon Nova Pro, a highly capable multimodal model with the best combination of accuracy, speed, and cost for a wide range of tasks; and Amazon Nova Premier, the most capable of Amazon’s multimodal models for complex reasoning tasks and for use as the best teacher for distilling custom models. Amazon Nova Micro, Amazon Nova Lite, and Amazon Nova Pro are generally available today; Amazon Nova Premier will be available in the Q1 2025 timeframe.
Lakshmi Hanspal, Chief Trust Officer at DigiCert, on balancing Generative AI’s opportunities and risks.
I’ve recently joined the organization as the Chief Trust Officer. I’ll describe the role as I see it. My background is as a technology and AI executive with deep expertise in
The Chief Trust Officer role is unique and reflects a major shift in how organizations perceive trust—it has moved from being a compliance checkbox to a foundational business asset. I’ve been fortunate to lead security at scale in companies like Box, SAP, and Amazon, and I’ve seen firsthand how trust has transformed into a critical cornerstone for
As a Chief Trust Officer, my focus is on:
• Securing digital experiences,
• Ensuring ethical AI development,
• Driving data privacy initiatives. These responsibilities are delivered as part of a platform offering, aligning with the growing customer expectations for privacy, AI transparency, and ethical practices.
CHIEF TRUST OFFICER AT DIGICERT, ON BALANCING GENERATIVE AI’S OPPORTUNITIES AND RISKS
What was your keynote, ‘The Gen AI Double Agent Dilemma: Ally or Adversary,’ at Black Hat MEA this year about?
My keynote centered on a key topic that’s on everyone’s mind: the partnership between Generative AI (Gen AI) and cybersecurity. People are debating whether Gen AI is an ally or an adversary. But the truth is—it doesn’t matter. The real focus should be on building synergies through human-AI collaboration.
Here’s what I covered:
1. Defining Generative AI: It’s important to align on the definition: Gen AI creates net-new content based on learned data.
2. Real-World Examples: I connected the discussion to initiatives like Saudi Arabia’s Vision 2030 and looked at its intersection with Gen AI and cybersecurity. For example:
• Smart Cities: AI for energy usage, traffic analysis, and predictive analytics.
• Skill Development: Gen AI upskills the workforce, creating new job opportunities in growing sectors.
• Critical Infrastructure: Robust security mechanisms to protect national defense and e-government services.
The key message I wanted to deliver was: It’s not about whether AI is autonomous; it’s about human oversight and collaboration. Governance, ethical practices, and transparency are essential to ensure AI serves its purpose as a trusted partner.
Let’s talk about adversarial AI—what risks are we seeing, and how can we mitigate them? We are already witnessing adversarial AI being used to amplify cyber threats. Examples include:
• AI-powered ransomware,
• Deepfakes,
• Sophisticated phishing campaigns. The challenge is balancing our approach. This has become a security arms race between defenders and attackers.
Here’s the mindset I advocate:
1. Are we participating in the race?: It’s not about being first; it’s about making sure we’re in the race with the right investments, skillsets, and priorities.
2. Focus on the right areas: AI isn’t a hammer where everything looks like a nail. We must focus on where AI can deliver the most value—incident response, vulnerability management, threat detection, and secure-by-design products.
AI gives us the ability to match the pace and velocity of modern threats. For example, Fortune 100 companies have seen a massive surge in AI-enabled attacks in the last 8 months compared to previous years. This arms race means defenders must adapt with tools that scale effectively alongside AI-driven threats.
What about data privacy—can frameworks like GDPR and CCPA address AI-related risks?
Data privacy is a top concern for anyone implementing or using AI today. I ran surveys ahead of my keynote, and the results were clear: concerns about data misuse and privacy intrusions dominate the conversation.
While foundational frameworks like GDPR and CCPA remain critical, the real challenge lies in scale, complexity, and velocity:
• How do we prove compliance as AI systems evolve rapidly?
• How do we ensure data privacy at scale—from training models to production environments?
Changes to regulations are needed, especially around:
• High-impact AI oversight (e.g., national defense, critical infrastructure),
• AI governance standardization, ensuring we meet the highest ethical standards.
AI must respect data subject rights— including the right to be forgotten— and learn to “unlearn” data when necessary. For organizations, proving adherence to privacy laws at AI scale is a bar-raising expectation.
Do you see opportunities for your organization in the Middle East?
Yes, I see significant opportunities in three key areas:
1. Data Sovereignty and Digital Trust: Delivering solutions at the scale and complexity that this region demands, especially for companies like Aramco that operate globally.
2. AI as a Game-Changer: Ensuring AI is built securely and can deliver transformative outcomes for our customers, with a shared responsibility model.
3. Regulatory Leadership: The Middle East has already set benchmarks for data sovereignty and governance, which have become global standards. This presents opportunities to further innovate and align AI systems with regional frameworks.
The future of AI and cybersecurity isn’t about choosing sides. It’s about:
• Building human-AI synergies,
• Ensuring ethical governance,
• Delivering solutions with trust, transparency, and oversight as foundational principles. By taking a balanced, proactive approach, we can address today’s challenges while shaping a future where AI enhances security, privacy, and trust at scale.
GLOBAL CIO EXPERTISE, DRIVING INNOVATION FOR PEOPLE AND PLANET
CONSULTING | RESEARCH | ON DEMAND
RESEARCH
INSIGHT & BENCHMARKING
EMERGING TECHNOLOGIES
GOVERNANCE
RISK & COMPLIANCE
CYBER SECURITY
DIGITAL TRANSFORMATION
DEOPS & DIGITAL INFRASTRUCTURE
ERP & CRM
Dataiku hosted the inaugural Middle East edition of its flagship Everyday AI Summit in Dubai last month at the iconic Dubai Opera. Featuring presentations from leading executives at the company, including Philip Coady, Chief Revenue Officer; Sid Bhatia, Area VP & General Manager – META; Kurt Muehmel, Head of AI Strategy; and Jean-Guillaume Appert, Senior Director of Product Management, the conference provided attendees with an indepth understanding of GenAI’s role in the enterprise. It also showcased some of the latest innovations from Dataiku and explored trends set to shape the next phases of data science and AI.
In addition to these company presentations, the Summit highlighted real-world success stories from prominent regional companies such as AW Rostamani, Emirates Global Aluminium, and Mashreq Bank. These organizations have successfully leveraged the Dataiku platform to operationalize AI use cases across their enterprises.
On the sidelines of the event, we sat down with Bhatia and Muehmel to discuss the Everyday AI series, a global initiative aimed at spotlighting customer success.
“What we wanted to showcase wasn’t just Dataiku itself, even though we organize this series, but primarily the stories of our customers,” explained Muehmel. “The focus was highlighting their real-world use of AI in their everyday work. Of course, our
customers are also eager to learn what’s new at Dataiku, so we shared our vision as well.”
Bhatia added that the event’s primary purpose was to bring genuine regional success stories to the forefront. “We had customers from diverse sectors and roles, including business analysts, data scientists, data engineers, line-of-business managers, and executives. This diversity created a rich forum for sharing ideas and experiences,” he said.
Dataiku executives believe Generative AI is advancing at a much faster pace- more quickly that we have seen with previous technologies.
“I would say it’s maturing very quickly— more quickly than we’ve seen with previous technologies. Where we are now —actually, almost exactly two years—after the release of ChatGPT, is that we have not only initial POCs but also real production use cases being deployed,” said Muehmel.
He highlighted this is really the focus of Dataiku: providing our customers with the tools they need to build those use cases and deploy them at a production scale. “We don’t build the models ourselves, but we provide the tooling and frameworks so they can use these models in their specific context.This aligns with what I just heard from one of our large customers. They mentioned that they can’t simply provide developers with access to the models—they need a comprehensive framework.”
That’s exactly what Dataiku offers through its platform, specifically the LLM Mesh, which is the solution to connect and enable this capability.
According to Muehmel, this marks a continuation of the company’s platform vision: empowering organizations to seamlessly connect with the technology they require for specific use cases. “The LLM Mesh can be thought of as an architecture for building agentic applications within the enterprise. To accomplish this, it’s essential to combine various components with your enterprise data, retrieval systems, and prompts, all within an environment that supports modular development and incorporates a shared layer of services.”
These shared services include controls
AREA VICE PRESIDENT & GENERAL MANAGER OF DATAIKU META
for cost management, security enforcement, and ensuring the safety of usage—features that are essential for every application. “You don’t want to develop each of these elements independently for every application. Instead, you need a shared backbone of connectivity and value-added services to streamline development,” added Muehmel.
The LLM Mesh provides this backbone by offering connectivity, optionality across various models, a registry of all the components you want to build with, and a framework for combining these components into the applications you need. In essence, it’s an architecture for building agentic applications in the enterprise.
Along with LLM Mesh, Dataiku has recently launched a set of tools designed to enforces enterprise-level policies, called LLM Guard Services.
“I spoke with a customer who had been conducting experiments outside of Dataiku and received a call in the middle of the night from their cloud provider about unexpectedly high expenses. Someone had made an error that was driving up the costs of the LLM service they were using. A feature like Cost Guard would have prevented this by enabling monitoring, enforcing budgets, and providing detailed reporting on who is spending what, for which purposes, and with which services,” explained Muehmel.
But cost management is just one part of the equation. Safety Guard ensures that the LLM doesn’t generate undesirable content and enforces policies for protecting personally identifiable information (PII) and intellectual property (IP). These additional services are critical for enterprises to confidently roll out generative AI at scale. Without them, organizations face risks such as spiraling costs or unacceptable errors, making deploying generative AI too risky to undertake.
When asked about some of the
challenges that Dataiku’s customers— and enterprises in general—face when implementing generative AI, Muehmel said the nature of the challenges is different from previous technologies.
“With previous technologies, like traditional machine learning, you really needed to know Python and have some understanding of statistics to grasp how the models work. You and I know that with generative AI, you simply type instructions in natural language and get a response. So, the challenge now is more about controlling access to ensure it doesn’t become overused and overly costly.”
According to him, sending enterprisescale volumes of data to these models can be costly. It is crucial to manage access and ensure these technologies are seamlessly integrated into the organization’s overall data infrastructure. While the models are effective for specific tasks, their true value emerges when they are integrated into a larger system that facilitates organizational tasks—whether it’s summarization, employing RAG (retrieval-augmented generation) techniques, or even developing intelligent agents.
As many GenAI use cases transition from the inferencing stage to full production, we asked Muehmel about the trends and developments he sees on his radar.
He identified document retrieval as a “sweet spot” for GenAI, allowing organizations to extract, summarize, and interact with large volumes of information efficiently. He also highlighted “agenda use cases,” where AI performs reasoning and tool usage autonomously within defined guidelines—an area poised for growth in enterprise applications.
Bhatia added the most common category involves customer analytics use cases. These include customer segmentation, upsell and cross-sell opportunities, customer churn analysis, customer risk mitigation, and leveraging various types of data to build intelligence that enables better customer marketing.
The second area of focus is risk mitigation. Dataiku works with many financial institutions, large telecom companies in the region, and insurance companies. These
“You and I know that with generative AI, you simply type instructions in natural language and get a response.”
KURT MUEHMEL, HEAD OF AI STRATEGY AT DATAIKU
organizations are looking at ways to contain risk, whether it’s market risk or operational risk, and Dataiku builds models to help manage and mitigate those risks.
“One key pattern we’ve observed is that AI is no longer seen as futuristic—it’s very much here and is now a board-level conversation. Many of these use cases are tied to value drivers discussed at the board level, such as reducing costs, increasing revenue, improving operational efficiency, launching new products and services, and eliminating inefficiencies. Much of this data science work tends to be repetitive in nature, and automating it allows teams to focus on the bigger picture,” said Bhatia.
In an age where the AI moniker is added by every vendor, Bhatia said Dataiku’s approach is unique. “AI is not new to us—Dataiku was founded 11 years ago, and our core mission has always been to make AI accessible, even for the smallest organizations. While we work with very large customers, we’ve also successfully onboarded about 700 customers worldwide, including more than 50 major data-driven enterprises in the region.”
He added that what sets Dataiku apart— and is a key differentiator in the market—is its open platform. “This means we embrace open-source technologies, integrating the latest innovations, including advancements in LLMs, directly into our solution. That’s the first differentiator. The second is that our platform is highly collaborative. It doesn’t cater to just one type of user. As I mentioned earlier, different profiles—business analysts, data scientists, and line-of-business managers—can all work on the same data seamlessly. Even users without coding skills, such as business professionals, can engage with data effectively.”
Third, and equally important, is that Dataiku provides robust governance. “Innovation shouldn’t compromise governance, so when you build a model, you can ensure it meets governance standards. This holistic approach allows us to deliver everything—from data preparation to model monitoring—within a single product and interface. This unified experience is one of our biggest strengths and a critical differentiator,” he concluded.
CYBER TRUST ADVISORY CONSULTANT, HELP AG, DISCUSSES AI’S IMPACT ON DATA PRIVACY.
“Can
safeguard privacy?”
Why is data protection particularly important in the context of AI?
AI is truly transforming industries by automating decision-making, identifying patterns, and making predictions. Let’s consider an example from healthcare. AI systems can analyze patient data to predict illnesses early or optimize treatments. However, imagine if such a system were exposed and patient data were leaked—this could severely impact the healthcare sector financially and damage its reputation. These systems rely heavily on collecting vast amounts of patient data to function effectively. This reliance on data underscores the importance of protection and security. For instance, in Saudi Arabia, several regulations and frameworks have been implemented to ensure the security and ethical use of AI systems.
For example, the Personal Data Protection Law (PDPL) from the National Data Management Office (NDMO) focuses on privacy protection. Additionally, the AI Ethics and Principles Framework from SDAIA ensures that AI systems in Saudi Arabia are secure, reliable, and operate ethically. Such measures highlight the crucial balance between leveraging AI’s transformative potential and safeguarding privacy and trust.
Do you think traditional frameworks, such as GDPR and CCPA, are sufficient to address AI-related privacy risks? Actually, they can. These frameworks are capable of addressing AI-specific risks. However, to be very honest, we also need to include AI-powered security governance in every organization. For instance, there is the ISO standard for artificial systems, which
we need to establish and implement to strengthen global security and privacy while building trust in AI systems.
Do you think AI itself can be used to enhance data privacy?
AI, as a tool, comes into question here because AI systems operate by collecting data. This data collection can happen in two ways: directly and indirectly.
In the direct approach, the user is aware of the data being collected, either by actively providing input or through explicit consent. However, in the indirect approach, data is collected passively, such as through actions like comments, likes, and shares on social media. This information is then used to personalize user experiences or identify user behaviors.
Given the vast amount of data being collected by AI systems, I believe ensuring data privacy is a significant challenge.
How important is human oversight, or the “human-in-the-middle” approach, to ensure that AI systems remain ethical? Human oversight is indeed crucial. One of the most important aspects is ensuring that AI system operators are fully aware of privacy concerns. I would like to emphasize the need for operators to adopt a privacy-bydesign approach, where privacy is prioritized from the outset. They must ask critical questions, such as: Why are we collecting this data? Is it necessary? Are we putting anyone at risk? These considerations are essential for operators to act responsibly when dealing with AI systems.
Transparency is another key element. Operators must be open with users by publishing clear privacy policies, obtaining user consent, and explaining how data is being collected, processed, and stored. Additionally, they should outline the security measures in place to safeguard user data. Such transparency builds trust and ensures ethical practices in AI operations.
What is Help AG offering in the market for data privacy?
Help AG is a cybersecurity-specific consulting company. We provide a range of services, including artificial intelligence security and governance in health. At Help AG, we don’t focus on just one phase of the process; instead, we consider the entire AI system lifecycle. This begins with the collection and
preparation of data, where data classification plays a crucial role. For example, when collecting data in the Saudi market, it’s important to classify it appropriately. We assist in aligning data practices with PDPL (Personal Data Protection Law), ISO standards, and the NCA (National Cybersecurity Authority) framework.
Additionally, we offer data protection tools, such as Data Loss Prevention (DLP) solutions, to ensure that no data is mishandled or leaked. These measures enable organizations to safeguard their data effectively while maintaining compliance with regulatory standards.
What emerging technologies or techniques are most effective for ensuring data protection in AI?
Regarding emerging technologies and techniques in the market, we must highlight the importance of privacy-by-design and security-by-design principles. Among the emerging technologies, one notable technique is differential privacy. This is a fascinating approach because it blurs the data, allowing you to gain insights and understand the full picture without compromising any private information.
Another significant technology is homomorphic encryption, which enables computations to be performed on encrypted data without the need to decrypt it. This ensures that data can be processed securely without exposing any sensitive information.
Human oversight is indeed crucial. One of the most important aspects is ensuring that AI system operators are fully aware of privacy concerns.
We also have a very important technique known as federated learning. With federated learning, data remains on local devices—such as your servers, mobile phones, or other devices—and the learning process occurs without transferring or moving the data elsewhere. This is a crucial advancement in emerging technologies that enhances both privacy and security.
Additionally, I would like to emphasize the importance of consent mechanisms. It is vital for users to know what is happening with their data—who is accessing it, who has connected to it, and where exactly their data is stored at any given moment. Clear and transparent consent processes are fundamental to building trust and ensuring ethical data practices.
GUEST ARTICLE
GALLOWAY
Head of Government Affairs at SolarWinds, writes about how governments can set the standard for AI transparency.
It’s evident that in the Middle East, government initiatives and action set the pace for technological advancement. Cloud-first strategies laid out by the UAE and Saudi Arabia in 2019 and 2020 respectively, have meant that today, this is now the preferred computing paradigm for many of these nations’ private enterprises. And now, the region’s forward-focused leaders have set their sights on AI. The UAE was of course the first country in the world to appoint a Minister of State for Artificial Intelligence, and that was as far back as October 2017. And this year, Saudi Arabia signaled its intentions of setting up a US$40billion AI investment fund.
The ongoing integration of AI into public services is reshaping the way governments interact with their citizens, offering unprecedented efficiencies and capabilities. But it is important to recognize that this technological leap brings with it the critical need to maintain, and even enhance, public trust in the government’s use of these capabilities. The responsible deployment of AI, combined with an unwavering commitment to transparency and security, is essential in fostering this trust.
AI’s integration into public sector functions has been both expansive and impactful. From automating routine tasks to providing sophisticated analytics for decision-making, AI applications are becoming indispensable in areas such as law enforcement or social services. In law enforcement, predictive policing tools can help Middle East nations maintain their pristine records in maintaining social order, while on government portals, AI-driven chatbots such as the UAE’s ‘U-Ask’ can allow users to access information about government services in one place. These applications not only improve efficiencies but also enhance
accuracy and responsiveness in public services.
While AI-driven applications are broadly advantageous to the public sector, AI, by its nature, raises concerns around trust: its complex algorithms can be opaque, its decision-making process impenetrable. When AI systems fail—whether through error, bias, or misuse—the repercussions for public trust can be significant. Conversely, when implemented responsibly, AI has the potential to greatly enhance trust through demonstrated efficacy and reliability. Therefore, a key principle that government entities must build their AI strategies upon is Transparency and Trust.
A foundational way government entities can maintain accountability in their AI initiatives is by adhering to a robust observability strategy. Observability provides in-depth visibility into an IT system, which is an essential resource for overseeing extensive tools and intricate public sector workloads, both on-prem and in the cloud. This capability is vital for ensuring that AI operations function correctly and ethically. By implementing comprehensive observability tools, government agencies can track AI’s decision-making processes, diagnose problems in real time, and ensure that operations remain accountable. This level of oversight is essential not only for internal management but also for demonstrating to the public that AI systems are under constant and careful scrutiny.
Observability also aids in compliance with regulatory standards by providing detailed data points for auditing and
reporting purposes. This piece of the puzzle is essential for government entities that must adhere to strict governance and accountability standards. Overall, observability not only enhances the operational aspects of AI systems but also plays a pivotal role in building public trust by ensuring these systems are transparent, secure, and aligned with user needs and regulatory requirements.
Equally critical in reinforcing public trust are robust security measures. Protecting data privacy and integrity in AI systems is paramount, as it prevents misuse and unauthorized access, but it also creates an environment where the public feels confident about depending on these systems. Essential security practices for AI systems in government entities include robust data encryption, stringent access controls, and comprehensive vulnerability assessments. These protocols
ensure that sensitive information is safeguarded and that the systems themselves are secure against both external attacks and internal leaks.
Even with these efforts, there will continuously be challenges in making sure AI builds, rather than erodes, public trust. The sheer complexity of the technology can make it hard for people to understand how AI works, which can lead to mistrust. Within government departments, resistance to change can also slow down the adoption of important transparency and security measures. Addressing these challenges requires an ongoing commitment to policy development, stakeholder engagement, and public education.
To navigate these challenges effectively, it’s paramount that governments adhere to another key principle in their design of AI systems: Simplicity and Accessibility. All strategies around implementing AI need to be thoughtful and need to make sense to all stakeholders
and users. There needs to be a gradual build-up of trust in the tools rather than a jarring change, which can immediately put users on the defensive. Open communication and educating both the public and public sector personnel about AI’s capabilities and limitations can demystify the technology and aid adoption.
PwC estimates that by 2030, AI will deliver US$320billion in value to the Middle East. With governments in the region focused on growing the contribution of the digital economy to overall GDP, AI will be a fundamental enabler of their ambitions. While AI has immense potential to enhance public services, its impact on the public is complex. Government entities once again have the chance to lead by example in the responsible use of AI. And as has been the precedent, we can then expect the private sector to follow suit.
• Launched: 2014
• Specialization: Azure Machine Learning is a cloud-based service that provides tools for building, deploying, and managing ML models. It supports popular ML frameworks like TensorFlow, PyTorch, and Scikit-learn. It specializes in automated ML (AutoML), enabling faster model creation with minimal coding, and integrates seamlessly with other Azure services for large-scale deployments.
explores and highlights the leading MLOps platforms revolutionizing machine learning operations.
• Launched: 2015 (by Google Brain Team)
• Specialization: TensorFlow is an open-source ML framework designed for numerical computation. It excels in deep learning and is widely used for tasks involving image and speech recognition, natural language processing (NLP), and recommendation systems. TensorFlow is known for its flexibility, supporting research and production-level deployments.
• ML Launched: Early 2010s, with products like AutoML added in 2018
• Specialization: GCP provides a suite of ML services, including AutoML for building models with minimal expertise, BigQuery ML for using SQL in model training, and Vertex AI for unified ML development. GCP specializes in combining AI with data analytics at scale, leveraging its advanced infrastructure and tools like TensorFlow.
• Founded: 2013
• Launched: 2017
• Specialization: Amazon SageMaker simplifies the end-to-end ML pipeline, including data preparation, model training, tuning, and deployment. It offers prebuilt algorithms, AutoML capabilities, and integrations with AWS services like S3 and Lambda, making it highly scalable for diverse applications in commerce, healthcare, and more.
• Specialization: Dataiku is an end-to-end ML and AI platform focusing on democratizing data science. It provides a collaborative environment for data preparation, visualization, and ML model development. It stands out for its no-code/ low-code capabilities, making it accessible for non-experts while still catering to advanced data scientists.
• Founded: 2006
• Specialization: RapidMiner is an integrated data science platform for data preparation, machine learning, and model deployment. It specializes in visual workflows and automated ML, making it ideal for business users who want to leverage ML without extensive coding expertise.
• Launched: 2021 (rebranding of Google AI and ML tools)
• Specialization: Vertex AI provides a unified platform for ML operations, integrating data preparation, training, and deployment. It specializes in MLOps (Machine Learning Operations), simplifying the entire lifecycle of ML projects, and supports AutoML for creating models with minimal coding.
• Launched: 2010
• Specialization: IBM Watson offers a range of AI and ML solutions for industries like healthcare, finance, and customer service. It specializes in NLP, conversational AI, and predictive analytics. Watson is known for its enterprisegrade features, emphasizing explainable AI and ethical AI practices.
• Founded: 1997 (expanded into analytics and ML in 2010s)
• Specialization: Alteryx focuses on data analytics and preparation with ML capabilities. It specializes in automating repetitive tasks and offering intuitive workflows for predictive modeling, enabling business analysts to build models without requiring advanced coding skills.
• Launched: 2016 (by Facebook’s AI Research Lab, FAIR)
• Specialization: PyTorch is an open-source deep learning framework designed for flexibility and ease of use in research and production. It specializes in dynamic computation graphs, which allow for adaptive and interactive model development. PyTorch excels in deep learning applications such as computer vision, natural language processing (NLP), and reinforcement learning. It is widely adopted in academia and the industry due to its extensive community support and native integration with Python.
• Launched: Late 2010s
• Specialization: AWS ML encompasses a variety of services like Amazon SageMaker, Rekognition, and Translate. It specializes in providing scalable ML tools integrated into the AWS ecosystem, making it suitable for developers and enterprises looking to leverage cloudnative AI solutions.
• Founded: 2013
• Specialization: Domino Data Lab offers an enterprise MLOps platform that enables data science teams to build, deploy, and monitor machine learning models at scale. It specializes in collaboration, reproducibility, and model management for large organizations. Domino supports various tools and frameworks like R, Python, and TensorFlow, allowing data scientists to work seamlessly while ensuring compliance and scalability.
The power demands of artificial intelligence (AI), combined with the impacts of reindustrialisation, electric vehicles (EVs), and the transition to renewable energy, sees the technology represent a significant investment opportunity across the entire value chain, including data centre and grid infrastructure, as well as electrification end markets.
However, it is important to always consider and question potential risks, particularly the physical limitations on AI’s growth, where its insatiable thirst for energy will come from, and how its associated emissions may fuel new climate concerns.
Currently, to train OpenAI’s ChatGPT-4 in just ten days, one would need 10,000 Blackwell GPUs costing roughly US$400 million. In contrast, as little as six years ago, training such a large language model (LLM) would have required millions of the older type of GPUs to do the same job. In fact, it would have required over six million Volta GPUs at a cost of US$61.5 billion – making it prohibitively expensive. This differential underscores not only the substantial cost associated with Blackwell’s predecessors but also the enormous energy requirements for training LLMs like ChatGPT-4. Previously, the energy cost alone for
CHAMBERLAYNE, CFA
Head of Global Sustainable Equities, Portfolio Manager, Janus Henderson Investors, discusses how innovation seeks to meet AI’s energy needs but highlights unresolved issues regarding its energy sources and the technology’s potential impact on climate.
training such an LLM could reach as much as US$140 million, rendering the process economically unviable. The significant leap in the computing efficiency of these chips, particularly in terms of power efficiency, however; has now made it economically feasible to train LLMs.
Nvidia’s innovations in the power efficiency of its chips have indeed enabled advancements in AI. However, there is a significant caveat to consider. Although we often assess the cost-effectiveness of these chips in terms of computing power per unit of energy – Floating Point Operations Per Second (FLOPS) per watt – it’s important to note that the newer chips come with a higher power rating (Figure 2). This means that, in absolute terms, these new chips consume more power than their predecessors.
Couple this with Nvidia’s strong sales growth, indicating an incredible demand for computing power from companies like Alphabet (Google), OpenAI, Microsoft and Meta, driven by the ever-increasing size of datasets to develop AI technologies, the power implications of AI’s rapid expansion have begun to raise eyebrows.
Interestingly, thanks to efficiency improvements, global data centre power consumption has remained relatively
constant over the past decade, despite a massive twelvefold increase in internet traffic and an eightfold rise in datacentre workloads.1 An International Energy Agency (IEA) report highlighted how data centres consumed an estimated 460 terawatt-hours (TWh) in 2022, representing roughly 2% of global energy demand,2 which was largely the same level as it was in 2010.
But, with the advent of AI and its thirst for energy, data centre energy consumption is set to surge. In fact, the IEA estimates that data centres’ total electricity consumption could more than double to reach over 1,000 TWh in 2026 – roughly equivalent to the electricity consumption of Japan.3 This highlights how demand for AI is creating a paradigm shift in power demand growth. Since the Global Financial Crisis (GFC), demand for electricity in the US has witnessed a flat 1% bump annually – until recently.4 Driven by AI, increasing manufacturing/industrial production and broader electrification trends, US electricity demand is expected to grow 2.4% annually.5 Further, based on analysis of available disclosures from technology companies, public data centre providers and utilities, and data from the Environmental Investigation Agency, Barclays Research estimates that data centres account for 3.5% of US electricity consumption today, and data
Currently, to train OpenAI’s ChatGPT-4 in just ten days, one would need 10,000 Blackwell GPUs costing roughly US$400 million. In contrast, as little as six years ago, training such a large language model (LLM) would have required millions of the older type of GPUs to do the same job.
centre electricity use could be above 5.5% in 2027 and more than 9% by 2030.6
To illustrate the real-world implications of AI’s increasing power demands, Microsoft recently announced a deal with Constellation Energy concerning the recommissioning of an 835 megawatt (MW) nuclear reactor at the Three Mile Island site in Pennyslyvania.9
This deal highlights the sheer scale of efforts being made to meet the growing power needs of AI. The move is part of Microsoft’s broader commitment to its decarbonisation path, demonstrating how corporate power demands are intersecting with sustainable energy solutions.
The cost of recommissioning the nuclear reactor is estimated at US$1.6 billion, with a projected timeline of three years for the reactor to become operational, with Microsoft targeting a 2028 completion date.
Advancements in AI, when paired with innovations in renewables, may hold the key to sustainably meeting rising energy demand. The IEA reported that power sector investment in solar photovoltaic (PV) technology is projected to exceed US$500 billion in 2024, surpassing all other generation sources combined. By integrating AI into various solar energy applications, such as using technology to analyse meteorological data to produce more accurate weather forecasts, intermittent energy supply can be mitigated.16 Researchers are also relying on AI to accelerate innovation in energy storage systems, given existing conventional lithium batteries are unable to fulfil efficiency and capacity requirements. While AI will create additional demand for energy, it also has the potential to solve challenges related to the net zero transition.
We are witnessing signs of growing demand for AI across various sectors including healthcare, transportation, finance, and industry, and we anticipate this to be a sustained, long-term trend.
What is quantum communication?
I Quantum communication is one of the most advanced quantum technologies. It leverages the unique quantum properties of light to exchange encryption keys for secure communication.
People often discuss post-quantum cryptography and its relationship with quantum computing’s impact on cybersecurity. Let me clarify: post-quantum cryptography is fundamentally different from quantum key distribution (QKD), a core component of quantum communication.
• Post-quantum cryptography involves algorithms designed to withstand the computational power of quantum computers. It prepares us for a future where quantum computers could break classical encryption methods, such as RSA.
• Quantum communication, on the other hand, uses single photons to carry
DR. SANA AMAIRI-PYKA FROM TECHNOLOGY INNOVATION INSTITUTE, DISCUSSES THE ROLE OF QUANTUM COMMUNICATION IN A POSTQUANTUM WORLD.
QUANTUM COMMUNICATIONS EXPERT, TECHNOLOGY INNOVATION INSTITUTE (TII)
encryption keys. This unique approach ensures that any attempt to eavesdrop on or intercept the key will be detected. If someone tries to spy on the communication, the system will alert you in advance, confirming whether the key has been compromised. This makes quantum communication a technology of the future, offering a level of security where you already know— before using it—that your key has not been compromised. Unlike algorithmic systems,
this certainty is a game-changer.
How does quantum communication enhance security over traditional communication technologies?
Quantum communication leverages the principles of quantum mechanics—such as superposition and entanglement—to provide security advantages that traditional systems cannot. A primary example is Quantum Key Distribution (QKD), where any interception of the quantum keys by an unauthorized party immediately alters their state, alerting the communicating parties to potential eavesdropping. This real-time detection capability is intrinsic to quantum mechanics, ensuring a level of security unmatched by classical encryption methods.
How soon will quantum threats become a reality?
There’s ongoing speculation about the timeline for quantum computing to become a significant cybersecurity threat. While some reports claim advancements in quantum computers, such as those in China, have demonstrated hacking capabilities, these claims remain unproven.
Quantum computing is advancing rapidly, driven by significant investments from both industry and governments. Optimistic estimates suggest a 30% likelihood of classical encryption systems like RSA-2048 being compromised within the next 5 to 10 years. Some experts extend this timeline to 15 years.
The critical issue is preparation. For nations and organizations to secure their systems against quantum attacks, they require at least a decade to build quantumsecure infrastructure. This is why action must begin now.
What are some specific applications of high information-sharing ability enabled by quantum communication that could revolutionize current communication systemsl?
Quantum communication’s high-security information-sharing capabilities have the potential to transform several sectors:
- Financial sector: Quantum channels can protect sensitive financial information, ensuring integrity and security in financial transactions. It can also be used for securing backup solutions and blockchains.
- Defence & cybersecurity: Quantumsecured networks can safeguard
command and control data, reducing espionage risks.
- Healthcare Data Security: Patient information can be securely shared between facilities, enhancing confidentiality and privacy.
- Smart grids: Quantum-secured smart grids for data and power distribution.
What recent advancements in quantum technology have accelerated interest in quantum communication and networking? Several recent advancements have propelled interest in quantum communication and networking. From a technological breakthrough point of view, improved single photon detection solutions and advances in error correction techniques have made practical applications of quantum technology more feasible, fueling interest and development. Moreover, Standardization Efforts such as The work of the ETSI ISG (https://www.etsi.org/ technologies/quantum-key-distribution) in QKD which is important to enable the future interoperability of the quantum communication networks being deployed around the world. In addition, International Investments are now more and more focused on QKD solutions.
What are the main challenges in implementing quantum communication protocols in real-world communication systems?
Implementing quantum communication in real-world systems, like any new technologies comes with exciting challenges that are actively being addressed. Scaling up requires efficient qubit generation, long-distance transmission, and precise detection, while the systems’ sensitivity to environmental factors is improving with better control techniques. Although QKD needs dedicated fiber links and comes with costs, ongoing innovationsare making these networks increasingly feasible and affordable, paving the way for secure, largescale quantum communication.
What is TII’s role in quantum research? TII, under the Advanced Technology Research Council (ATRC), leads R&D in emerging technologies. With over 1,300 engineers and scientists across 10 research centers, its Quantum Research Center (QRC) hosts 120+ experts, making it the largest quantum research hub in the Middle East.
“The critical issue is preparation. For nations and organi zations to secure their systems against quantum attacks, they require at least a decade to build quantumsecure infra structure. This is why action must begin now.”
PRESIDENT OF NETAPP, ON WHY AI SUCCESS HINGES ON INTELLIGENT DATA
What bring you to town?
We’ve been in the region for more than 20 years. So, we’ve been investing in the UAE for over 20 years. I’m the president of the company, and I certainly try to come by from time to time to see how things are going and visit some of our customer partners. This was a great opportunity for me to follow up after George, our CEO, was here. There’s no doubt we’re seeing significant momentum in the country, and I want to ensure that we continue to support our customers in their journey.
What sort of opportunities do you see in this part of the world for NetApp?
I think the world is changing, and we’ve all seen this transformation over the past several years. What’s particularly interesting is the speed of the change—faster than we’ve ever experienced before. We now live in a world driven by data and intelligence. It’s no longer just about having data; it’s about having intelligent data and knowing how to leverage it effectively.
As you know, NetApp is a company with over 30 years of experience. We’re a Fortune 500 company with 5,300 employees globally and thousands of partners across the globe. We’ve been helping customers and organizations build intelligent data infrastructures. Today, everyone is talking about AI. In recent years, we’ve seen a focus on digital transformation. Our role is to enable that transformation, supporting customers as they integrate AI into their data by ensuring they have an intelligent data infrastructure.
In this region, we’re seeing significant adoption of new technologies and a lot of momentum around AI. Industries such as oil and gas, telecom, fintech, and even the public sector are heavily investing in AI initiatives. AI begins with data—having the right data, ensuring its security, compliance, and alignment with privacy policies. That’s where we’re committed to supporting our customers.
As you mentioned, this is the age of AI, but there’s a gap between data environments and AI environments. How do you bridge that gap?
That’s a great question, and one I’m often asked. When we talk about AI and data, people frequently ask, “Which is more important?” My response is that AI is part of the data ecosystem. AI relies on algorithms and data. Even if you have the best algorithm,
if your data is flawed, the outcomes will be inaccurate. The most critical element is ensuring data integrity. This means having secure, accessible, well-structured data that is optimized for performance.
Here’s an interesting statistic: on average, only 30% of the data organizations store is ever used. The remaining 70% often remains untapped, despite its potential to provide valuable insights. With AI and large language models, we’re now leveraging unstructured data to create models and perform inferences using structured data. This allows data scientists and decisionmakers to derive actionable insights using the right algorithms.
You’ve had two strong quarters recently. What is driving this growth?
Several factors contribute to this growth, starting with customer trust. As I mentioned, we’ve been present in this region for over 20 years, and globally, we operate in more than 100 countries. Our customers trust our technology and our commitment to long-term partnerships. These results are a reflection of that trust.
Additionally, we’ve been at the forefront of innovation, especially in the hybrid cloud space. Over the past few years, the world has shifted towards hybrid cloud environments. Companies want the flexibility to run workloads on-premises, in public cloud environments, or in private cloud setups. NetApp is the only company with a firstparty service integrated natively across the three major hyperscalers—Microsoft (Azure NetApp Files), AWS (FSx for ONTAP), and Google Cloud (Cloud Volumes ONTAP). This unique capability allows us to provide customers with the interoperability they need to achieve their goals.
Our consistent focus on supporting AI and data needs, coupled with our deep partnerships and innovative technologies, is driving our growth.
Do you see hyperscalers adopting flash storage anytime soon?
Our flash storage business is experiencing rapid growth, with a 19% increase in the last quarter compared to the market’s growth of 9–10%. Hyperscalers appreciate that our technology enables their customers to run workloads seamlessly across environments. Flash is becoming increasingly common in on-premises deployments, and many of our new workloads are flash-based.
“In this region, we’re seeing significant adoption of new tech nologies and a lot of momentum around AI. Industries such as oil and gas, telecom, fintech, and even the public sector are heavily investing in AI initiatives.”
Following two years of back-to-back double-digit returns, investors continue to ask whether investment in generative artificial intelligence (gen AI) is now over; and if we are at the top of the AI hype cycle. While we are no longer in the early stages of the gen AI ‘fourth wave’ of technology, we believe that investors are still underestimating both the length and magnitude of investment required, as well as the long-term disruption and benefits it will bring.
Firstly, it’s important to clarify the difference between a compute wave and a theme (like cybersecurity, electric vehicles, or clean technology). A compute wave requires broad investment across the full stack of technology – from silicon building blocks, to user interface and applications. The PC internet era required an initial shift from the analogue world to digital, driving prices of compute down, which democratised access and connected homes. The mobile cloud wave that internet infrastructure was built upon, was catalysed by the launch of the iPhone in 2007. It’s notable that a key mobile application like Uber did not have its initial public offering (IPO) until 2019.
The parallel here is that infrastructure has to be built and scale achieved before the most exciting and useful new applications are fully developed and adopted. The pace
HENDERSON
Portfolio Manager at Alison Porter, shares invest outlook for 2025.
of capital expenditure investment in AI data centres has been unprecedented and the demand for accelerated compute from the likes of NVIDIA have grown at an unparalleled pace. However, this generative AI wave has yet to witness an interface shift or new edge device requirements. Our experience shows this to be consistent with the pattern of previous tech waves. It spans resource and productivity optimisation; shifts in payments and financial systems; transportation being reimagined by autonomous capabilities; healthcare diagnostics, surgery and drug discovery; humanoid robots, and the more familiar upgraded PC and mobile devices. We know there is much yet to come as AI copilots and autonomous agents become commonplace across the economy. For investors, patience is required to benefit from this pervasive and transformative wave.
And as previous tech waves have also demonstrated, investors should not expect the pace of development and adoption of generative AI to be linear. There are bottlenecks in infrastructure development and the availability of silicon (microchips), such as NVIDIA’s latest Blackwell chip. Indeed, past waves have typically run for more than six years and delivered outsized returns – but all have also involved elevated volatility and frequent drawdowns.
A changing political regime in the US will bring additional volatility as taxes,
tariffs and regulations are reconsidered. Technology and artificial intelligence are a national priority for many countries and central to a broader deglobalisation and reindustrialisation strategy being undertaken. While this is expected to increase volatility, it will also serve as a tailwind to demand in infrastructure and areas like autonomous driving, where we are likely to see accelerated development as new federal laws emerge.
Artificial intelligence as a wave underlies a wide variety of long-term investment themes such as Next Generation Infrastructure (including Cybersecurity), Fintech, Electrification (including power, electric vehicles and Clean Tech) and Internet 3.0, which help guide idea generation. A focus on competitive advantage, responsible management, scalable profitability and new product innovation – at a rational price, and irrespective of market capitalisation or geography, is essential. We believe marrying the bottom-up identification of tech leaders and thematic idea generation with underappreciated earnings power can help us to navigate the ongoing challenge of valuation in this dynamic and innovative
GEN
The build-out of infrastructure and applications for generative AI is expected
to take years to play out. It is important to note that with each wave of technology not only has more investment been required to realise its potential, but more disruption in more sectors across the broader economy has ensued. As the gen AI wave matures, disruption across many other sectors will accelerate – just as it has in the past. The technology sector continues to leverage its balance sheet strength advantage to invest heavily in future research and development, supporting its capability to generate attractive returns for investors.
As previous tech waves have also demon strated, investors should not expect the pace of develo pment and adoption of generative AI to be linear. There are bottlenecks in infras tructure develo pment and the availability of silicon (microchips), such as NVIDIA’s latest Blackwell chip.
We continue to be excited by the outlook for technology equities. Our focus remains on finding the leaders across the sector by navigating the hype cycle, and we believe that a focus on stock fundamentals can help to drive consistent returns. As gen AI matures, it essentially gives the ‘vampire’ (technology) superpowers to use its FAANGs to suck more share from the wider economy. We believe that investors will be well served to remain focused on the companies and sectors that are driving, rather than experiencing, disruption.
• It is important for investors to recognise that compute waves like AI are typically lengthy, given the potential to disrupt multiple sectors and magnitude of investment required. As with previous tech waves, volatility is to be expected.
• As the AI wave matures into 2025, it gives the ‘vampire’ (technology) superpowers to use its FAANGs to suck more share from the wider economy. Hence, the role of active management and stock selection is increasingly essential in the fastevolving tech landscape, particularly as narrow thematic approaches show limitations.
• We believe a focus on the fundamental strengths and potential of companies driving disruption, rather than those at its receiving end, is key to a rewarding investment in the tech sector.
Innovation Officer EMEA, ServiceNow, on how to measure the business impact of GenAI
Generative AI (GenAI) is the A-lister of modern tech. It has turned heads and stoked controversy as much as any emergent technology in living memory. It has potential impact for individuals, businesses, and governments. It is poised to be an ever-greater part of our lives as it finds its way into smartphones, PCs, and other devices. It is here to stay.
PwC recently surveyed Middle East CEOs and found that almost three quarters expected GenAI to “significantly change” their day-to-day operations over the next three years. This finding follows earlier estimates from PwC analysts that predicted a potential economic impact in the United Arab Emirates (UAE) of as much as 14% of 2030 GDP because of general (non-generative) AI. This was expected to be the largest relative impact to an economy anywhere in the region. Additionally, in the latter half of 2023,
PwC’s consultancy unit, Strategy&, projected a US$5.3-billion economic boost for the UAE from GenAI alone.
The UAE’s progress is reflected in a global push towards AI adoption. A recent ServiceNow and Oxford Economics survey of more than 4,400 global executives found 81% were planning to increase their AI spending in the next year. We identified the pacesetters and found that two thirds of these leaders (67%) reported a “good visibility [into] AI deployment and utilization” compared with 33% of non-pacesetters. Some 65% of AI leaders said they were “operating with a clear, shared AI vision toward business transformation across the wider organization”, while only 31% of everyone else said this.
But among all respondents, only 35% had established formal metrics to measure the business impact of AI. This is a significant finding for the UAE given the projected economic impact of AI, and specifically GenAI, that is expected to occur here. If organizations wait for others’ successes and failures before exploring their own options, they will miss out any early-adopter advantages. To move wisely, an organization must be able to measure impact as adoption proceeds. One pro-tip when it comes to metrics is to not confine measurements to one corporate area.
First, look at financial effects. Metrics must be able to capture more than just cost savings and revenue boosts. The business should account for productivity gains and changes in the execution speeds of processes. Also consider the adding or subtraction of risk that can be attributed to GenAI as this has a direct bearing on future costs.
Operational impact can be measured by going beyond saved time and resources and looking to tie those outcomes to improvements in customer and employee experiences. It is also helpful to introduce measurement of the quality of adoption — the frequency and breadth of use of GenAI tools — so business leaders can better determine the technology’s contribution to operational and financial outcomes.
If we do not learn our lessons now, we risk exclusion from a technological revolution that will stamp organizations as winners or losers for years to come. Holistic approaches to value will allow GenAI adopters a measure of control over which stamp they receive.
While the enterprise is measuring impact, it should remember that apparent gains may have hidden, or even obvious, costs. GenAI may allow great efficiency in a call center, for example, by enabling selfservice within the customer support function. This benefit may emerge in the metrics but behind the data may be disgruntled customers and demotivated employees. This would be very bad news for a brand that was previously lauded in the marketplace for its customer-first ethos.
When we look to the AI pacesetters and examine their approaches, the main takeaway must be that while they do use discrete data points like cost reductions and time savings, they do not stop there. If GenAI is to live up to its potential, adopters must approach AI metrics holistically. Finance and operations are entangled with the technologies that enhance them. Metrics must produce consistent, contextual results to be actionable and impactful.
We can think of a GenAI value triangle in which financial and operational impacts are stacked against quality of adoption to reveal an overall business value. If every GenAI project is evaluated in these terms, the enterprise is on the road to AI maturity. A good investment in GenAI is one in which the organization can point to measurably positive operational outcomes accompanied by clear popularity for the solution among users. Adoption tells a story that no amount of on-paper operational benefits can refute. That is why measurement of user engagement is critical to the success of AI in general, and generative AI in particular.
In the digitalization race what we are seeing right now with GenAI is a revving of the engine. When it reaches full speed, we need to be ready to apply the brakes and turn the wheel accordingly. If we do not learn our lessons now, we risk exclusion from a technological revolution that will stamp organizations as winners or losers for years to come. Holistic approaches to value will allow GenAI adopters a measure of control over which stamp they receive.
Artificial intelligence (AI) has become a regular feature of life over the past couple of years. Its applications extend from suggesting automated email responses and helping us create better resumés to optimizing industrial asset performance, supporting smarter decisions and even predicting future scenarios through technologies such as neural networks, deep-learning, and reinforcement learning.
We’ll see an expansion of those use cases in 2025, with AI driving strategic shifts in how industry operates. Expect AI to become a direct partner in your organization’s growth, making complex processes simpler and helping people at every level to access the insights they need, precisely when they need them.
As a science, AI dates to the 1950s. As its exponential adoption over the past couple of years indicates, AI will become a major contributor to future industrial growth.
In 2025, different areas of AI will continue to evolve and will be integrated much more closely – while barriers to entry will drop. Sophisticated analytics will become available at many more points across the industrial value chain, delivering end-to-end insights from shopfloor to top floor in a more humanized, conversational manner. This industrial intelligence will
By adopting AI systems designed with ethical considerations and human-centric features, industries are making strategic shifts that align with evolving technology and sustainability priorities, says Jim Chappell, Global Head of AI at AVEVA. GUEST ARTICLE
accelerate new pathways to efficiency, profitability and sustainability. The trend brings early investors in AI closer to Industry 5.0. In this Collaborative Age, humans and advanced technologies will join forces to provide prosperity while respecting the planet’s production limits.
Business leaders recognize the value of onboarding industrial AI to ensure operational agility, outpace competitors, and seize emerging opportunities. Nearly three-fourths (71%) of C-suite executives say investing in intelligence and insights is a priority for the next 12 months, according to the recent AVEVA Industrial Intelligence Index report.
We see three major AI business trends begin to play out as the science continues to evolve.
Until recently, AI was typically designed for data teams and specialists, but that’s rapidly changing. The trend toward natural language and voice-based interfaces will allow operators with little or no technical training to interact more closely with all types of AI. Expect productivity to increase as more non-expert industrial workers use AI to do their jobs better and solve problems in real
time – without needing specialized training or even an understanding of how the technology works. New recruits, for example, will spend less time training on systems when they can ask a tool such as the Industrial AI Assistant for what they need and get it. Humanized AI will democratize data-driven decisionmaking, unlocking competitive advantages across the board.
AI BECOMES THE BASIS OF A SIMPLIFIED USER EXPERIENCE
Think about how much time we spend learning to navigate different software, and understanding their menus, commands, shortcuts. With human-like interactions, AI will now be integrated as the ‘front end’ for industrial software systems. Instead of opening up dashboards and scrolling through various data inputs, we will simply be able to ask the system to generate a custom report or design an asset-specific console.
Generative AI (GenAI) is already helping us draft emails and create presentations. As the trend spreads to industrial settings, its benefits to business will show up as enhanced productivity, streamlined workflows and shorter time-to-value, without heavy investment in retraining.
APPLIED AI BECOMES OUR INDUSTRIAL WORKHORSE
AI is also becoming a sharper tool for operations and is evolving beyond enhancing efficiency. Predictive maintenance, for example, is now mainstream and is continuing to advance with the infusion of prescriptive and prognostic capabilities. Going forward, we’ll rely on industrial AI tools to do more of the heavy lifting, from crunching numbers to optimizing complex operations in real time.
GenAI will accelerate engineering timelines by further automating routine tasks. For example, in design, Generative Design AI (GenDAI) will automatically create optimized pipe layouts for a new factory, with set objectives to minimize overall tube length, reduce sharp bends for higher flow, and factor in space constraints.
This is a significant step toward Agentic AI which, in turn, will eventually take us closer to Artificial General Intelligence (AGI), or true human-like intelligence.
In addition, Autonomous AI can now handle dynamic processes, responding to complex changes and disruptions nearinstantaneously, with human supervision. Thanks to reinforcement learning, AIenabled systems can optimize even in transient situations such as startups, shutdowns, changes in input levels and other unexpected disruptions.
The value here is threefold. Stabilizing operations within seconds means minimal losses and significantly less downtime. As a result, industrial resilience increases. Finally, time to value rises as AI tools handle the complex, time-consuming tasks.
HOW THE EVOLUTION OF INDUSTRIAL AI SETS THE STAGE FOR INDUSTRY 5.0
As AI improves our workplace experience, expect to hear the term Industry 5.0 more frequently. It’s about elevating human expertise with AI-driven insights to unlock new value more sustainably. We’re seeing the emergence of AI systems as dynamic partners, acting as ‘intelligent agents’ that are capable of autonomous action as they learn continuously from complex data streams. This is a significant step toward Agentic AI which, in turn, will eventually take us closer to Artificial General Intelligence (AGI), or true human-like intelligence.
As currently available technologies evolve towards Industry 5.0, attention will focus on Responsible AI, where AI applications are built according to safe, trustworthy and ethical principles. Expect to see frameworks for Responsible AI to ensure AI decisions are equitable, understandable and accountable.
How has AI adoption within businesses evolved over the last year, and what trends do you foresee for 2025?
Rabea Ataya, CEO and Founder of Bayt.com, discusses the evolving adoption of AI, its impact on businesses and the job market, and the skills and strategies needed to thrive in this AI-driven era.
Over the past year, AI has moved rapidly from the periphery to the core of many business strategies. Initially, organizations focused on small-scale applications—like basic résumé screening or automating a few routine tasks. Today, companies are embedding AI much more deeply, from generating effective job descriptions to accelerating candidate shortlisting. At Bayt.com, for instance, we’ve seen employers shift from simply testing AI tools to relying on them for better hiring outcomes. By 2025, I expect AI to become integral to workforce planning and decisionmaking. Generative and conversational AI will evolve further, allowing employers and job seekers to interact with platforms in more natural, intuitive ways. We’ll see a stronger emphasis on responsible and explainable
CEO AND FOUNDER OF BAYT.COM
AI, ensuring transparency and building trust, and on using AI to complement human judgment rather than replace it.
AI is reshaping the job market—what new opportunities and challenges does it present for job seekers today?
AI offers job seekers the chance to present themselves more authentically and effectively. Tools that we’ve integrated at Bayt.com—like “Tailor My CV”—let candidates customize their résumés to highlight the skills and potential that matter most to employers. Conversational AI (currently in beta) can help job seekers refine their searches more fluidly, focusing on roles that truly align with their interests and expertise. However, the challenge is that as routine tasks become automated, candidates must adapt and hone more creative, strategic, and interpersonal skills. This shift means continuous learning and staying agile are critical. Ultimately, AI can streamline the job hunt and help candidates stand out, but success depends on embracing these tools and developing the kind of problem-solving, communication, and emotional intelligence that machines cannot replicate.
How can businesses balance AI-driven automation with the need to retain and upskill employees?
The goal shouldn’t be to replace people with technology, but to use AI as a lever to elevate human potential. Businesses can deploy AI to handle repetitive tasks, freeing employees to focus on higher-value activities—such as strategizing, innovating, and building stronger relationships with clients or candidates. To make this balance work, companies must invest in upskilling. As we’ve observed at Bayt.com, when organizations integrate AI thoughtfully, they can help their teams acquire new competencies, especially in interpreting AI-driven insights and managing tools effectively. In this way, automation doesn’t mean losing jobs; it means transforming them, enabling employees to grow into roles that are more fulfilling and future-proof. The best outcomes occur when AI and human talent complement each other, driving both productivity and employee engagement.
What industries in the UAE and the region are leading the way in AI adoption, and how is this influencing employment trends?
The UAE’s forward-thinking policies have
enabled sectors like finance, logistics, retail, and healthcare to move quickly in AI adoption. Finance, for example, uses AI for personalized services and fraud detection, while logistics firms optimize supply chains through predictive analytics. Healthcare is exploring AI-assisted diagnostics and patient support tools. At Bayt.com, we’re seeing a ripple effect in the job market: employers across these industries are increasingly seeking talent who can work comfortably with AI-driven insights. This is shifting hiring criteria, encouraging professionals to develop multidisciplinary skills that combine technical know-how with strategic thinking. The result is a more dynamic, agile workforce poised to tackle evolving challenges and opportunities.
For job seekers, what skills are now in demand to stay competitive in an AIaugmented job market?
While understanding basic AI concepts and data analysis is useful, employers are looking for more than just technical credentials. They value critical thinking, creativity, empathy, and the ability to interpret AIderived insights. With tools like Bayt. com’s AI-powered résumé optimizations and conversational search capabilities, job seekers can focus on roles best suited to their unique strengths. The skill set that will stand the test of time isn’t about outsmarting AI, but about working with it—translating complex information into actionable strategies, collaborating effectively, and maintaining a human touch in an increasingly digital world.
“While understanding basic AI concepts and data analysis is useful, employers are looking for more than just technical credentials.”
What are your predictions for the business landscape in 2025, particularly in the UAE?
By 2025, I anticipate the UAE will be a leading global example of AI-driven, human-centric economic development. Companies will rely on AI not only for efficiency and cost-savings but for strategic growth, informed decision-making, and personalized customer experiences. Initiatives like Emiratization will continue to shape a workforce that’s both techsavvy and deeply engaged in the nation’s future. At Bayt.com, we’ll remain dedicated to empowering job seekers and employers by enhancing our AI tools, focusing on authenticity, transparency, and trust.
From the boardroom to your goto news podcast, conversations about the availability of and use cases for AI are everywhere. It’s no surprise why AI innovations and their surrounding excitement are ubiquitous: AI has undoubtedly improved society in many ways, ranging from increasing business efficiencies to generating better outcomes in sectors like healthcare and education.
Cybersecurity practitioners benefit from AI, using this technology to enhance threat detection and response times by automating anomaly and vulnerability detection. Teams also use AI-driven cybersecurity tools to predict and prevent attacks by analyzing patterns and adopting evolving threats.
Conversely, the growing cybercrime market is thriving on cheap and accessible wins. As AI evolves, it’s already lowering the barrier to entry for aspiring cybercriminals, increasing access to the tactics and intelligence needed to execute successful attacks regardless of an adversary’s knowledge. In addition to enhancing accessibility, AI enables malicious actors to create more believable phishing threats, complete with contextaware and regionalized language.
THE AI-ENABLED CYBERCRIME PROJECT
DEREK MANKY
Chief Security Strategist & Global VP Threat Intelligence | Board Advisor, Threat Alliances at FortiGuard Labs, on exploring and mitigating AI-driven cybercrime.
While defenders navigate a changing threat landscape in which attackers continually identify new ways to harness AI for their benefit, collaborating across public sectors, industries, and borders is crucial to developing new strategies and practices to combat AIdriven cybercrime. Fortinet is proud to work with the UC Berkeley Center for Long-Term Cybersecurity (CLTC), the Berkeley Risk and Security Lab (BRSL), and other public and private sector organizations on a new effort: AI-Enabled Cybercrime: Exploring Risks, Building Awareness, and Guiding Policy Responses. CLTC was established in 2015 as a research and collaboration hub at the University of California, Berkeley, and serves as a convening platform and bridge between academic research and the needs of decision-makers in government, industry, and civil society relating to the future of security. BRSL at UC Berkeley’s Goldman School of Public Policy is an academic research institute focused on the intersection of technology and security. The lab conducts analytical research and designs and fields wargames.
This latest effort, AI-Enabled Cybercrime, is a structured set of tabletop exercises (TTXs), surveys, workshops, and interviews that will take place over the next nine months, engaging subject matter experts worldwide and sharing findings in a public-facing report and follow-on presentations. The project will
simulate real-world scenarios to uncover the dynamics of AI-powered cybercrime and develop forward-looking defense strategies. This effort will help decision-makers in policy and industry navigate the changing nature of cybersecurity, support the development of proactive AI-enabled cybercrime prevention strategies, and inform public policy decisions.
The initiative begins December 17 with a scenario-based TTX conducted at UC Berkeley. Cybersecurity professionals, academic experts, local government officials, and law enforcement representatives will explore generative AI tools like those used to create believable phishing scams and how they catalyze cybercrime.
Follow-up workshops are planned in Singapore and Israel in the first half of next year. The cumulative findings from these workshops will be shared in a public report scheduled for release in the summer of 2025.
In addition to the AI-Enabled Cybercrime initiative, Fortinet has worked with UC Berkeley’s CLTC on other projects to help entities worldwide prepare for future cybersecurity challenges. Last year, Fortinet collaborated with the CLTC and other
As AI evolves, it’s already lowering the barrier to entry for aspiring cyber criminals, increasing access to the tactics and intelligence needed to execute successful attacks regardless of an adversary’s knowledge.
organizations on its Cybersecurity Futures 2030 effort to help leaders across the public and private sectors examine future-focused scenarios and consider how digital security will change in the coming years.
The Cybersecurity Futures 2030 inaugural report, Cybersecurity Futures 2030: New Foundations, which was published last December, includes insights from six global workshops with insights on how technological, political, economic, and environmental changes will impact the future of cybersecurity for governments and organizations and how leaders should start to prepare. Fortinet participated in the Washington, D.C. working session, taking part in a hands-on workshop that included analysis across different geographies and scenario planning for 2030.
COLLABORATION IS
As our adversaries take advantage of new technologies and we assess and adjust our strategies, it’s clear that partnerships strengthen our collective ability to navigate the evolving threat landscape proactively. Ongoing cooperation across industries and borders is a vital component of successfully dismantling sophisticated cybercrime operations, and there are many powerful examples of existing collaborations that are already combatting cybercrime in a meaningful way.
Dismantling cybercrime operations and adversaries’ attack infrastructure is everyone’s responsibility; no organization can achieve this alone. By working together and regularly sharing intelligence and response strategies, we can force cybercriminals to start over, rebuild, and shift their tactics, disrupting their activities and making our digital world safer.
In the wake of the recent COP29 in Baku, Azerbaijan, climate change and sustainability remain firmly under the spotlight. AI has been hailed for its potential to help solve some of the challenges of climate change but has also come under scrutiny for its intense energy demands. Mohamed bin Zayed University of Artificial Intelligence (MBZUAI) has identified several ways in which AI could help to improve sustainability and mitigate some of the worst effects of climate change, as well as how AI itself could be made more efficient.
1. REDUCING THE ENERGY CONSUMPTION OF AI
AI is power hungry and driving unprecedented demand for power, data centers struggling to keep up. Goldman Sachs Research estimates that data center power demand will grow 160% by 2030[1].
MBZUAI is working on numerous initiatives and research projects to address this, focusing on areas such as hardware and software design. One area of exploration is to improve the efficiency of traditional computing architectures such as Graphics Processing Units (GPUs) and Tensor
Processing Units (TPUs). A team at the university is also looking at ways to reduce waste and deploy resources more efficiently in the upper layers, building sustainability into the development and application of AI models.
MBZUAI has also developed its own energy-efficient operating system, AIOS, to reduce energy consumption, carbon footprint and the cost of creating and deploying AI models and applications.
The lost 488 million hectares (Mha) of tree cover between 2001 and 2023, a12% decline since 2000[2]. One of the challenges facing authorities, particularly in large countries such as India and Brazil, is monitoring tree cover to detect when illegal logging or land clearance is taking place. AI can help monitor land by using computer vision and recognition to quickly and accurately assess and report on how land is being used.
MBZUAI’s GeoChat+ is a tool to enhance sustainability, development, and planning with generative AI.
In the energy sector, AI is optimizing operations, increasing efficiency, and promoting sustainability. This is particularly important as more diverse sources of energy enter the grid, including roof-top solar and an increasing array of utility-scale renewables. At the same time, governments are keen to encourage consumers and businesses to think about the way they consume energy to reduce peak demand and help stabilize the grid.
Climate change is making farming more precarious than ever, with droughts, heat waves and intense rain damaging crops and reducing harvests. At the same time, AI technologies can be used to increase crop yields, enhance food security and optimize resources used in agriculture. Drones equipped with AI-powered sensors can monitor crops and detect diseases and nutrient deficiencies early, enabling targeted interventions. And machine learning algorithms can analyze weather patterns and soil data to provide insights for precision farming.
UAE - 18 FEB KSA - 24 FEB SINGAPORE - 24 OCT
INDONESIA - 27 OCT MALAYSIA - 29 OCT INDIA (MUMBAI) - 12 NOV
INDIA (BENGALURU) - 14 NOV KENYA - 19 NOV