ClassificationoftheStateofManufacturingProcess underIndeterminacy
MuhammadAslam* andOsamaHasanArif
DepartmentofStatistics,FacultyofScience,KingAbdulazizUniversity,Jeddah21589,SaudiArabia; oarif@kau.edu.sa
* Correspondence:magmuhammad@kau.edu.saoraslam_ravian@hotmail.com;Tel.: +966-593-329-841
Received:27August2019;Accepted:15September2019;Published:19September2019
Abstract: Inthispaper,thediagnosisofthemanufacturingprocessundertheindeterminate environmentispresented.Thesimilaritymeasureindexwasusedtofindtheprobabilityof thein-controlandtheout-of-controloftheprocess.Theaveragerunlength(ARL)wasalsocomputed forvariousvaluesofspecifiedparameters.AnexamplefromtheJuiceCompanyisconsideredunder theindeterminateenvironment.Fromthisstudy,itisconcludedthattheproposeddiagnosisscheme undertheneutrosophicstatisticsisquitesimpleandeffectiveforthecurrentstateofthemanufacturing processunderuncertainty.Theuseoftheproposedmethodundertheuncertaintyenvironmentin theJuiceCompanymayeliminatethenon-conformingitemsandalternativelyincreasetheprofitof thecompany.
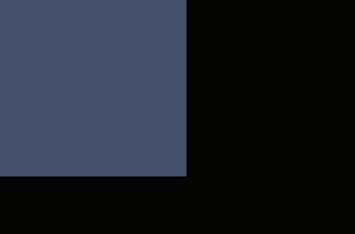
Keywords: similarityindex;diagnosis;process;indeterminacy;neutrosophicstatistics
1.Introduction
Tocontrolthenon-conformingproductsintheindustryisanimportanttaskforindustrial engineers.Theirmissionistominimizethenon-conformingproductwhichcanbeachievedonlyifthe problemsinthemanufacturingprocesscanbetackledimmediately.Thecontrolchartsareessential toolsintheindustrytomonitorthemanufacturingprocess.Thesetoolsareusedtoindicatethestateof theprocess.Atimelyindicationaboutthestateoftheprocessleadstothehighqualityoftheproduct. Epprechtetal.[1]andChiuandKuo[2]proposedachartformonitoringone,andmorethanone, non-conformingproduct,respectively.Hsu[3]designedavariablechartusingtheimprovedsampling schemes.HoandQuinino[4]proposedanattributecharttocontrolthevariationintheprocess.Aslam etal.[5]andAslametal.[6]workedonatime-truncatedchartfortheBirnbaum-Saundersdistribution andtheWeibulldistributionrespectively.Jeyadurgaetal.[7]workedonanattributechartunder truncatedlifetests.
Toanalyzethevagueandfuzzydata,thefuzzylogicisapplied.Thefuzzylogicisapplied toanalyzethedatawhentheexperimentersareunsureabouttheexactvaluesoftheparameters. Therefore,themonitoringoftheprocesshavingfuzzydataisdoneusingthefuzzy-basedcontrolcharts. AfshariandGildeh[8]andErcanTeksenandAnagun[9]workedonfuzzyattributeandvariablecharts, respectively.FadaeiandPooya[10]workedonafuzzyoperatingcharacteristiccurve.Formoredetails, thereadermayrefertoJamkhanehetal.[11]whodiscussedtherectifyingfuzzysinglesamplingplan. SenturkandErginel[12]studiedvariablecontrolchartsusingfuzzyapproach.ErcanTeksenand Anagun[9]workedonthefuzzyX-barandR-charts.MoredetailsonfuzzylogiccanbeseeninLee andKim[13]andGrzegorzewski[14].
Afuzzyandimprecisedatausuallyhaveindeterminatevalues.Fuzzyandvaguedataonly consideredthemembershipofthetruthandfalsevalues.Aneutrosophiclogicdealswithmembership oftruth,falseandindeterminacyvalues.Therefore,theneutrosophiclogicisusefultoanalyzethedata
Mathematics 2019, 7,870;doi:10.3390/math7090870 www.mdpi.com/journal/mathematics
havingindeterminacy.Smarandache[15]introducedtheneutrosophicstatistics,whichanalyzethedata whenindeterminacyispresented.Aslam[16]andAslamandArif[17]introducedtheneutrosophic statisticsintheareaofqualitycontrol.Moredetailsabouttheneutrosophiclogiccanbeseenin references[18–23].
Thesimilaritymeasureindex(SMI)hasbeenwidelyusedinavarietyoffieldsforclassification purposes.Inmedicalsciences,thisindexisusedtoclassifythepatientshavingaparticulardisease ornotunderindeterminacy,seeDeandMishra[24].Byexploringtheliteratureandtothebest oftheauthor’sknowledge,thereisnoworkontheprocessmonitoringusingSMI.Inthispaper,a methodtoclassifythestateoftheprocessusingSMIisintroduced.Theoperationalprocessofthe proposedmethodisalsogiven.Theproposedclassificationmethodissimpleinapplicationcompared totheexistingmethodunderclassicalstatistics.Itisexpectedthattheproposeddiagnosismethod forthemanufacturingprocessundertheindeterminateenvironmentwillbeeffective,adequateand easycomparedtotheexistingcontrolchartsunderclassicalstatistics.InSection 2,theSMIindexis introducedinprocesscontrol.AcomparativestudyandapplicationaregiveninSections 3 and 4, respectively.Someconcludedremarksaregiveninthelastsection.
2.TheProposedChartBasedonSMI
Supposethat ZN = sN + uNI; ZN ∈ [ZL, ZU ] isaneutrosophicnumberhavingadeterminedpart sN andanindeterminatepart uNI, I ∈ [infI,infU] denotestheindeterminacy.Noteherethat ZN ∈ [ZL, ZU ] isreducedtothedeterminednumber ZN = sN whennoindeterminacyisfound.Thepractitioners cannotrecordobservationsofthevariableofinterestinthepreciseanddeterminedforminthe presenceofindeterminacy.Themonitoringofthedatahavingneutrosophicnumbersusingclassical statisticsasdiscussedinreference[25]maymisleaddecision-makersregardingthestateoftheprocess. Forexample,thepractitionersdecidetheprocessisinthecontrolstateusingclassicalstatistics,butin fact,someobservationsareintheindeterminacyinterval.Moredetailsonthisissuecanbeseenin reference[26].Supposethat tU, fU and IU presentstheprobabilitiesofthenon-defective,defective andindeterminate.Fortheclassificationofthestateoftheprocess,let t = 1 and f = 0 showthatthe processisincontrol.Therefore,thevalueofSMIcloseto1indicatesthattheprocessisincontroland thevaluesawayfromtheSMIshowtheprocessisout-of-control.TheSMIfromDeandMishra[24]is givenby: SMI = 1 |(tL tU ) (IL IU ) ( fL fU )| 3 (1 −|(tL tU ) + (IL IU ) + ( fL fU )|) (1) Notehere 0 ≤ tL, IL, fL ≤ 1, 0 ≤ tU, IU, fU ≤ 1, 0 ≤ tL + fL ≤ 1, 0 ≤ tU + fU ≤ 1, tL + IL + fL ≤ 2, tU + IU + fU ≤ 2.
BasedonSMI,thefollowingclassificationprocedureisproposedtodiagnosethestateofthe manufacturingprocess.
Step1: Selectarandomsampleofsize n anddetermine tU, fU and IU Step2: ComputethevaluesofSMI.Classifytheprocessin-controlifSMI ≥ 0.95,otherwise, theout-of-control.
TheoperationalprocessoftheproposedmethodisalsogivenwiththehelpofFigure 1
START
Take a sample of n from the production process
Compute the values of SMI SMI≥095
In-control Out-of-control YES NO Figure1. Theoperationalprocessoftheproposedmethod.
Noteherethatunlikethetraditionalcontrolchartsunderclassicalstatistics,theproposedchart usingSMIisindependentofthecontrollimitsandthecontrollimitscoefficients.Theproposedchart reducestothetraditionalcontrolchartsunderclassicalstatisticsifnoindeterminacyisfound.Suppose thattheprobabilityofin-controloftheprocessisdeterminedfromSMI.Let SMI = Pin,the Pin forthe processisgivenby Pin = 1 |(tL tU ) (IL IU ) ( fL fU )| 3 (1 −|(tL tU ) + (IL IU ) + ( fL fU )|) (2)
Theaveragerunlength(ARL)isusedtoseewhenontheaveragetheprocessisexpectedtobe out-of-control.TheARLunderindeterminacyisgivenby: ARL = 1 1 |(tL tU ) (IL IU ) ( fL fU )| 3 (1 −|(tL tU ) + (IL IU ) + ( fL fU )|)
(3)
Thevaluesof tU, fU and IU forvariousvaluesof n aregiveninTables 1–3.Tables 1 and 2 are givenwhen n = 25and n = 50,respectively.Table 3 ispresentedforavariablesamplesize.InTable 4, thevaluesof Pin andARLaregivenfortheparametersgiveninTables 1–3.Theclassificationofthestate oftheprocessbasedonSMIisalsopresentedinTable 4.Theprocessissaidtobethein-control(IN) stateifSMI ≥ 0.95 andtheout-of-control(OOC)stateifSMI < 0.95.ItisnotednospecifictrendinARL values.Thefollowingalgorithmisusedtoclassifythestateoftheprocessusingtheproposedmethod.
Step1: Specify n anddeterminethevaluesof tU, fU and IU
Step2: UsetheSMItofindtheprobabilityofin-control.
Step3: ClassifytheprocessINifSMI ≥ 0.95andOOCifSMI < 0.95.
Table1. Neutrosophicdatawhen n = 25.
Sample No. Sample Size
Numberof Non-Defective Units ND tU Numberof Indeterminate Units I IU 12530.12210.8410.04 22540.16190.7620.08 32520.08230.9200 42550.2200.840.16 52520.08220.8810.04 62510.04220.8820.08 72500200.850.2 82540.16210.8400 92560.24170.6820.08 102510.04230.9210.04 112520.08200.830.12 122550.2180.7220.08 132540.16190.7620.08 142580.32160.6410.04 152530.12210.8410.04 162520.08210.8420.08 172550.2170.6830.12 182530.12190.7630.12 192570.28170.6810.04 202510.04230.9210.04 212500230.9220.08 222520.08190.7640.16 232550.2170.6830.12 242570.28170.6810.04 252580.32170.6800
Sample No. Sample Size
Numberof Defective Units D fU
Table2. Neutrosophicdatawhen n = 50.
Numberof Defective Units D fU
Numberof Non-Defective Units ND tU
Numberof Indeterminate Units I IU 15010.02480.9610.02 25020.04470.9410.02 35030.06450.920.04 45050.1430.8620.04 55020.04430.8650.1 65060.12410.8230.06 75010.02460.9230.06 85020.04440.8840.08 95070.14370.7460.12 105080.16340.6860.12 115010.02470.9420.04 125060.12430.8610.02 135010.02410.8280.16 145030.06390.7880.16 155060.12410.8230.06 165030.06450.920.04 175090.18400.810.02 185020.04410.8270.14 195040.08460.9200 205060.12430.8610.02 215010.02470.9420.04 225070.14430.8600 235020.04450.930.06 245000480.9620.04 255010.02480.9610.02
Sample No. Sample Size
Table3. Neutrosophicdatawithvariablesamplesize.
Numberof Defective units D fU
Numberof Non-Defective Units ND tU Numberof Indeterminate Units I IU 1100120.120780.780100.10 28080.100670.83850.06 38060.075690.86350.06 410090.090890.89020.02 5110100.091990.90010.01 6110120.109980.89100.00 7100110.110850.85040.04 8100160.160790.79050.05 990100.111660.733140.16 109060.067720.800120.13 11110200.182890.80910.01 12120150.125990.82560.05 1312090.0751080.90030.03 1412080.0671070.89250.04 1511060.055950.86490.08 168080.100720.90000.00 1780100.125690.86310.01 188070.088680.85050.06 199050.056780.86770.08 2010080.080880.88040.04 2110050.050880.88070.07 2210080.080910.91010.01 23100100.100880.88020.02 249060.067800.88940.04 259090.100800.88910.01
Table4. Classificationoftheprocess. n = 25 n = 50VariableSampleSize
Pin ClassificationARL Pin ClassificationARL Pin ClassificationARL
0.9451OOC180.9865IN740.9223OOC13 0.9165OOC120.9797IN490.9438OOC18 0.9729IN370.9660IN290.9526IN21 0.8265OOC60.9521IN210.9626IN27 0.9591IN240.9521IN210.9654IN29 0.9591IN240.9380OOC160.9629IN27 0.9309OOC140.9729IN370.9486OOC19 0.9451OOC180.9591IN240.9273OOC14 0.8869OOC90.9092OOC110.9040OOC10 0.9729IN370.8763OOC80.9300OOC14 0.9309OOC140.9797IN490.9335OOC15 0.9018OOC100.9521IN210.9398OOC17 0.9165OOC120.9380OOC160.9628IN27 0.8717OOC80.9237OOC130.9630IN27 0.9451OOC180.9380OOC160.9532IN21 0.9451OOC180.9660IN290.9660IN29 0.8869OOC90.9309OOC140.9526IN21 0.9165OOC120.9380OOC160.9480OOC19 0.8869OOC90.9729IN370.9526IN21 0.9729IN370.9521IN210.9591IN24 0.9729IN370.9797IN490.9591IN24 0.9165OOC120.9521IN210.9695IN33 0.8869OOC90.9660IN290.9591IN24 0.8869OOC90.9865IN740.9610IN26 0.8869OOC90.9865IN740.9619IN26
Note:IN = in-controlandOOC = out-of-control.
3.ComparativeStudy
Inthissection,acomparisonoftheeffectivenessoftheproposedmethodisgivenoverthecontrol chartsunderclassicalstatisticsreportedinreference[25].AccordingtoAslametal.[26],amethod whichdealswithindeterminacyissaidtobemoreeffectivethanthemethodwhichprovidesthe determinedvalues.Theproposedmethodreducestothetraditionalmethodunderclassicalstatisticsif noindeterminacyisrecorded.Fromreference[25],itisnotedthatthecontrolchartunderclassical statisticsdoesnotconsiderthemeasureofindeterminacywhichmakesitlimitedtobeusedinan uncertaintyenvironment.Theperformanceoftheexistingcontrolchartdependsonthecontrollimit coefficientwhichisdeterminedthroughthecomplicatedsimulationprocess.Ontheotherhand, thecurrentmethodconsideredthemeasureofindeterminacytoevaluatetheperformanceofthecontrol chart.Inaddition,theproposedmethodisindependentofthecontrollimitcoefficient.Theproposed processcanbeappliedeasilytoclassifythestateoftheprocess.Noteherethat,theproposedmethod reducestothemethodunderclassicalstatisticsifnoindeterminacyisfoundintheproductiondata. ThevaluesofARLfromtheproposedmethodandmethodunderclassicalstatisticsdiscussedby Montgomery[25]areshowninTable 5 when n = 25and D = 2.Itiswell-knowntheorythatthesmaller thevaluesofARLmeansmoreefficientthecontrolchartprocess[25].FromTable 5,itcanbeseen thattheproposedmethodprovidesthesmallervaluesofARLthantheexistingmethod.Itmeansthe proposedcontrolcharthastheabilitytodetectashiftintheprocessearlierthanthemethodunder classicalstatistics.Forexample,when n = 25and d = 2,thevalueofARLoftheexistingmethodfrom Table 5 is37.Ontheotherhand,theproposedmethodprovidessmallervaluesofARLwhichare24, 14,18and12.Fromthiscomparison,itisconcludedthattheprocessisclassifiedasIN.Theindustrial engineerscanexpecttheprocesstobeout-of-controlatthe37thsamplebyusingtheexistingmethod andonthe12thsampleforsamplenumber22usingtheproposedmethod.Therefore,theproposed methodisefficientindetectingshiftsearlierthantheexistingmethod.Fromthiscomparison,isthe authorsconcludedthattheproposedmethodismoreeffectivethantheexistingchartsasitconsidered themeasureofindeterminacyandindicatedwhentheprocesswasOCC.
Table5. Thecomparisonoftheproposedmethodwithexistingmethodwhen n = 25and D = 2. SampleNoARLControlChart
4.Application
Inthissection,adiscussionoftheapplicationoftheproposedmethodinanorangejuicecompany isgiven.AccordingtoMontgomery[25],“Frozenorangejuiceconcentrateispackedin6-ozcardboard cans.Thesecansareformedonamachinebyspinningthemfromcardboardstockandattachinga metalbottompanel”.Byinspection,itwasfoundthatasampleof50juicecanswasformed.Some canswerefoundtobeleakingandsomewerelabeledasgood.Forsomecans,theindustrialengineer isindeterminateaboutwhetherthejuiceproductislabeledaseitherconformingandnon-conforming. Therefore,classicalstatisticscannotbeappliedtomonitortheprocessinthepresenceofindeterminacy. Thedatafor n = 50isshowninTable 2.Theclassificationofthestateoftheprocessforthejuicecansis showninTable 4.FromTable 4,itisnotedthatthefirstfivesubgroupsshowthattheprocessistheIN controlstate.The5thsubgroupshowsthattheprocessisOOCandindustrialengineershouldtake actiontobringbacktheprocessintheINstate.ItisnotedthatoveralleightsamplesareinOOCstate. Fromthisstudy,itisconcludedthattheuseoftheproposedmethodtoclassifythestateoftheprocess isquiteeasy,effectiveandadequatetobeappliedunderanuncertaintyenvironment.
5.ConclusionsandRemarks
Inthispaper,thediagnosisofthemanufacturingprocessundertheindeterminateenvironment waspresented.Thesimilaritymeasureindexwasusedtofindtheprobabilityofthein-controlandthe out-of-controloftheprocess.Theaveragerunlength(ARL)wasalsocomputedforvariousvaluesof specifiedparameters.Anindustrialexamplewasgiventoexplainthestateoftheprocess.Anindustrial exampleundertheindeterminateenvironmentwaspresented.Fromthisstudy,itisconcludedthat theproposeddiagnosisschemeundertheneutrosophicstatisticsisquitesimpleandeffectiveforthe currentstateofthemanufacturingprocessunderuncertainty.Thepractitionerscanapplytheproposed methodtosavetimeandeffortsintheindustry.Theproposedmethodusingnon-normalmeasurescan beconsideredasfutureresearch.
AuthorContributions: Conceivedanddesignedtheexperiments,M.A.;Performedtheexperiments,M.A. Analyzedthedata,M.A.andO.H.A.;Contributedreagents/materials/analysistools,M.A.;Wrotethepaper,M.A. andO.H.A.
Funding: ThisarticlewasfundedbytheDeanshipofScientificResearch(DSR)atKingAbdulazizUniversity, Jeddah.Theauthors,therefore,acknowledgewiththanksDSRtechnicalandfinancialsupport.
Acknowledgments: Theauthorsaredeeplythankfultoeditorandreviewersfortheirvaluablesuggestionsto improvethequalityofthismanuscript.
ConflictsofInterest: Theauthorsdeclarenoconflictofinterest.
References
1. Epprecht,E.K.;Costa,A.F.;Mendes,F.C.AdaptiveControlChartsforAttributes. IIETrans. 2003, 35,567–582. [CrossRef]
2. Chiu,J.-E.;Kuo,T.-I.AttributeControlChartforMultivariatePoissonDistribution. Commun.Stat.Theory Methods 2007, 37,146–158.[CrossRef]
3. Hsu,L.-F.Noteon‘Designofdouble-andtriple-samplingX-barcontrolchartsusinggeneticalgorithms’. Int.J.Prod.Res. 2004, 42,1043–1047.[CrossRef]
4. Ho,L.L.;Quinino,R.C.Anattributecontrolchartformonitoringthevariabilityofaprocess. Int.J.Prod. Econ. 2013, 145,263–267.
5. Aslam,M.;Arif,O.H.;Jun,C.-H.AnAttributeControlChartBasedontheBirnbaum-SaundersDistribution UsingRepetitiveSampling. IEEEAccess 2016, 4,9350–9360.[CrossRef]
6. Aslam,M.;Arif,O.H.;Jun,C.-H.AnattributecontrolchartforaWeibulldistributionunderaccelerated hybridcensoring. PLoSONE 2017, 12,e0173406.[CrossRef][PubMed]
7. Jeyadurga,P.;Balamurali,S.;Aslam,M.Designofanattributenpcontrolchartforprocessmonitoringbased onrepetitivegroupsamplingundertruncatedlifetests. Commun.Stat.TheoryMethods 2018, 47,5934–5955. [CrossRef]
8. Afshari,R.;Gildeh,B.S.Constructionoffuzzymultipledeferredstatesamplingplan.InProceedingsofthe 2017Joint17thWorldCongressofInternationalFuzzySystemsAssociationand9thInternationalConference onSoftComputingandIntelligentSystems(IFSA-SCIS),Otsu,Japan,27–30June2017.
9. ErcanTeksen,H.;Anagun,A.S.DifferentmethodstofuzzyX -Rcontrolchartsusedinproduction:Interval type-2fuzzysetexample. J.Enterp.Inf.Manag. 2018, 31,848–866.[CrossRef]
10. Fadaei,S.;Pooya,A.FuzzyUcontrolchartbasedonfuzzyrulesandevaluatingitsperformanceusingfuzzy OCcurve. TQMJ. 2018, 30,232–247.[CrossRef]
11. Jamkhaneh,E.B.;Sadeghpour-Gildeh,B.;Yari,G.Importantcriteriaofrectifyinginspectionforsingle samplingplanwithfuzzyparameter. Int.J.Contemp.Math.Sci. 2009, 4,1791–1801.
12. Senturk,S.;Erginel,N.DevelopmentoffuzzyX ~-R~andX ~-S~controlchartsusing α-cuts. Inf.Sci. 2009, 179,1542–1551.[CrossRef]
13. Lee,H.;Kim,S.Black-boxclassifierinterpretationusingdecisiontreeandfuzzylogic-basedclassifier implementation. Int.J.FuzzyLog.Intell.Syst. 2016, 16,27–35.[CrossRef]
14. Grzegorzewski,P.Onseparabilityoffuzzyrelations. Int.J.FuzzyLog.Intell.Syst. 2017, 17,137–144. [CrossRef]
15. Smarandache,F. IntroductiontoNeutrosophicStatistics;InfiniteStudy:ElSegundo,CA,USA,2014.
Mathematics 2019, 7,870
16.
17.
18.
19.
Aslam,M.DesignofSamplingPlanforExponentialDistributionunderNeutrosophicStatisticalInterval Method. IEEEAccess 2018, 6,64153–64158.[CrossRef]
Aslam,M.;Arif,O.H.TestingofGroupedProductfortheWeibullDistributionUsingNeutrosophicStatistics. Symmetry 2018, 10,403.[CrossRef]
Broumi,S.;Bakali,A.;Talea,M.;Smarandache,F.;ALi,M.ShortestPathProblemunderBipolarNeutrosphic Setting. Appl.Mech.Mater. 2017, 859,59–66.[CrossRef]
Broumi,S.;Bakali,A.;Talea,M.;Smarandache,F.;Ullah,K.BipolarNeutrosophicMinimumSpanningTree. Availableonline: https://books.google.com.sa/books?id=VopuDwAAQBAJ&printsec=frontcover&hl=ar#v= onepage&q&f=false (accessedon25August2019).
20.
21.
Abdel-Basset,M.;Gunasekaran,M.;Mohamed,M.;Chilamkurti,N.Three-waydecisionsbasedon neutrosophicsetsandAHP-QFDframeworkforsupplierselectionproblem. FutureGener.Comput.Syst. 2018, 89,19–30.[CrossRef]
Abdel-Basset,M.;Gunasekaran,M.;Mohamed,M.;Smarandache,F.Anovelmethodforsolvingthefully neutrosophiclinearprogrammingproblems. NeuralComput.Appl. 2018, 31,1595–1605.[CrossRef]
22. Broumi,S.;Bakali,A.;Talea,M.;Smarandache,F.;Kishore,K.K.;¸Sahin,R.Shortestpathproblemunder intervalvaluedneutrosophicsetting. J.Fundam.Appl.Sci. 2018, 10,168–174.
23. Abdel-Basset,M.;Nabeeh,N.A.;El-Ghareeb,H.A.;Aboelfetouh,A.Utilisingneutrosophictheorytosolve transitiondifficultiesofIoT-basedenterprises. Enterp.Inf.Syst. 2019,1–21.[CrossRef]
24. De,S.;Mishra,J.InconsistentDataUsingNeutrosophicLogictoDiseaseDiagnosisforPrevention. InProceedingsofthe13thInternationalConferenceonRecentTrendsinEngineeringScienceandManagement, SchoolofElectronicsandCommunicationsEngineering,REVA,Bangalore,India,23–24April2018.
25. Montgomery,D.C. IntroductiontoStatisticalQualityControl;JohnWiley&Sons:JeffersonCity,MO,USA,2007.
26. Aslam,M.;Khan,N.;Khan,M.Z.MonitoringtheVariabilityintheProcessUsingNeutrosophicStatistical IntervalMethod. Symmetry 2018, 10,562.[CrossRef]
© 2019bytheauthors.LicenseeMDPI,Basel,Switzerland.Thisarticleisanopenaccess articledistributedunderthetermsandconditionsoftheCreativeCommonsAttribution (CCBY)license(http://creativecommons.org/licenses/by/4.0/).
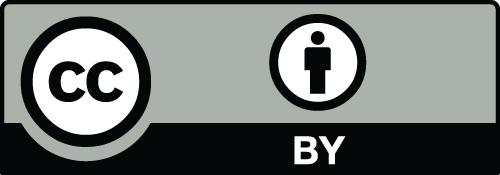