SolvingSolar-WindPowerStationLocationProblem UsinganExtendedWeightedAggregatedSum ProductAssessment(WASPAS)Techniquewith IntervalNeutrosophicSets
Ru-xinNie,Jian-qiangWang*andHong-yuZhang
SchoolofBusiness,CentralSouthUniversity,Changsha410083,China;nrx1690452484@csu.edu.cn(R.N.); Hyzhang@csu.edu.cn(H.Z.)
* Correspondence:jqwang@csu.edu.cn
AcademicEditor:FlorentinSmarandache
Received:28May2017;Accepted:28June2017;Published:4July2017
Abstract: Asoneofthepromisingrenewableenergyresources,solar-windenergyhasincreasingly becomearegionalengineinleadingtheeconomyandraisingcompetitiveness.Selecting asolar-windpowerstationlocationcancontributetoefficientutilizationofresourceandinstruct long-termdevelopmentofsocio-economy.Sincetheselectionprocedureconsistsofseverallocation alternativesandmanyinfluentialcriteriafactors,theselectioncanberecognizedasamultiple criteriadecision-making(MCDM)problem.Tobetterexpressmultipleuncertaintyinformation duringtheselectionprocedure,fuzzysettheoryisintroducedtomanagethatissue.Interval neutrosophicsets(INSs),whicharecharacterizedbytruth-membership,indeterminacy-membership andfalsity-membershipfunctionsintheintervalnumbers(INs)form,arefeasibleinmodelingmore uncertaintyofreality.Inthispaper,anewlyextendedweightedaggregatedsumproductassessment (WASPAS)technique,whichinvolvesnovelthreeprocedures,isutilizedtohandleMCDMissues underINSsenvironment.Somemodificationsareconductedintheextendedmethodcomparingwith theclassicalWASPASmethod.Themostobviousimprovementoftheextendedmethodreliesonthat itcangeneratemorerealisticcriteriaweightinformationbyanobjectiveandsubjectiveintegrated criteriaweightdeterminationmethod.Acasestudyconcerningsolar-windpowerstationlocation selectionisimplementedtodemonstratetheapplicabilityandrationalityoftheproposedmethodin practice.Itsvalidityandfeasibilityarefurtherverifiedbyasensitivityanalysisandacomparative analysis.TheseanalyseseffectivelyrevealthattheextendedWASPAStechniquecanwellmatchthe realityandappropriatelyhandlethesolar-windpowerstationlocationselectionproblem.
Keywords: multiplecriteriadecision-making(MCDM);intervalneutrosophicsets(INSs);weighted aggregatedsumproductassessment(WASPAS);integratedcriteriaweightinformation;solar-wind powerstationlocationselection
1.Introduction
Remarkablegrowthofurbanizationandindustrializationmakeitimperativetoincrease widespreaduseableelectricityforfacilitatingregionaleconomydevelopment[1].Duetotheincreasing awarenessoftheglobalclimatedegradation,conventionalenergyresourcescannotsimultaneously meettheenvironmentalchallengeandenergydemand[2].Thesolar-windenergysystemhasgradually substitutedthestatusoftraditionalenergyforthefriendlyenvironmentconcern[3].Solar-windpower stationdevotestoconvertrenewableresourcessuchassolarandwindenergyintopowerforsupporting socio-economicrequirement[4].Forbettercontributingtoregionalcompetitionanddeterminingfuture energygeneration,itissignificanttoseekagoodsolar-windpowerstationlocation[5].Considerable Symmetry 2017, 9,106;doi:10.3390/sym9070106
www.mdpi.com/journal/symmetry
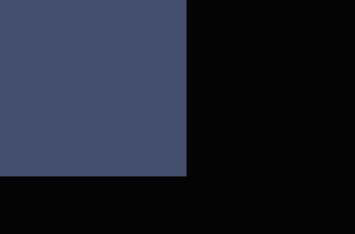
researcheshaveincreasinglyemergedconcerningselectingtherenewableenergylocation[6].Itiswell knownthattheusagestartingpointofmultiplecriteriadecisionanalysis(MCDA)isadefinitionof thesetofdecisionvariants[7].TheaimofMCDAistoselectagoodsolutionaccordingtodecision makers’(DMs’)preferences[8,9].Asnumerousinfluentialfactorsneedtobeconsideredintheselecting procedure,thelocationselectionproblemcanbetreatedasacomplexmultiplecriteriadecision-making (MCDM)problem[6].Inaddition,MCDMmethodshavebeenadoptedaseffectiveinstrumentsin thisfield.Incorporatinganalytichierarchyprocess(AHP)toGISenvironment,themostdesirable nuclearpowerplantlocationwasdeterminedbyMCDManalysistechnique[10].Theframeworkof MCDManalysisbasedonGISwasalsoidentifiedasaneffectivedecisiontoolforwind-farmlocation planningarea[11].Furthermore,greycumulativeprospecttheoryandclouddecisionframework weregraduallyemployedintopowerlocationselection[12,13].Tomanageinevitablefuzzinessand uncertaintyinrealisticapplication,ELECTRE-IIIasoneofthemostcommonlyusedMCDMtechnique wasextendedintointuitionisticfuzzycircumstancesfordeterminingagoodoffshorewindpower stationlocation[14].Theselectionofthesolar-windpowerstationlocationcanberegardedasanother complexMCDMprobleminvolvingmanyinfluentialfactorssuchaseconomicfactors,trafficfactors, naturalresources,environmentalfactorsandsocialfactors[15].ThoughMCDMmethodssuchas ELECTRE-IIhasbeenwidelyemployedintothisfield[15],existingresearchesconcerningsolar-wind powerstationlocationselectionneglecttheimpactofuncertaintyandonlyevaluatethealternatives intherealnumbercontext[16].Effectiveinformationexpressionformshouldbeintroducedintothe selectionproblemforrepresentingmoreabundantandrealisticinformation.
Alongwiththedevelopmentoffuzzysettheory,fuzzyset(FSs)[17]aswellasintervalvaluedfuzzy sets(IVFSs)[18],intuitionisticfuzzysets[19]andinterval-valuedintuitionisticfuzzysets(IVIFSs)[20] wereextensivelyappliedintodealingwithuncertainsituation.Owingtomultipleuncertaintyand informationexistinginpractice,abovetraditionaltypesofFSsareunabletoadequatelyexpress indeterminateinformationandinconsistentinformation[21].Neutrosophicsets(NSs)[22]wereinitially presentedbySmarandachetofeasiblymanagetheseuncertaininformationviaconsideringthetruth membership,indeterminacymembershipandfalsitymembershipfunctionssimultaneously[23,24]. However,NSwaslackingofspecificdescriptiontoadequatelyexpressactualissues.Asaparticular caseofNSs,single-valuedneutrosophicsets(SVNSs)wereintroducedforthefirsttimebySmarandache in1998inhisbook[22],reviewedin[25],whichisalsomentionedbyDenisHowe,fromEngland, inTheFreeOnlineDictionaryofComputing,1999.Sincemoreinformationcanbedescribedby intervalnumbers(INs),intervalneutrosophicset(INS)[26]asafurtherextensionofNSwasutilized invariousfieldssuchastrustworthycloudserviceselection[27],leansupplierselection[28]and medicaldiagnosis[29].RelativebasictheoriesofINSwhichbrieflyfocusonitsaggregationoperators andMCDMmethodshavealreadydevelopedmaturity[30].Particularly,MCDMmethodsincluding outrankingapproach[31],cross-entropymeasure[32],andcorrelationcoefficientmeasure[33]can effectivelydealwithproblemsunderINSenvironment.ThispaperaimstodevelopanovelMCDM techniquewithflexibilityandapplicabilityunderINSscircumstancesformatchingthesolar-wind powerstationlocationselectioncase.
Theweightedaggregatedsumproductassessment(WASPAS)methodwasoriginallypresented in2012regardedasaneffectiveextensionofWeightedProductModel(WPM)andWeightedSum Model(WSM)[34].ItsaccuracyindealingwithMCDMproblemshasbeenprovenbycomparing withthesimpleutilizationoneofWPMorWSM[34].Thismethodhasbeenextensivelyemployed intoMCDMsituationandvariousapplications[35],especiallyinthesiteselectionfield.Thecomplex circuitdesignoflead-zincfrothflotationselectionregardedasaMCDMprocedurewasadequately settledbyWASPASmethodforfullyprocessingcostsandreinforcingtheutilization[36].Selectionof thebestwindfarmlocationwasfeasiblyhandledandassessedbyutilizingtheWASPASmethod[37]. Theselectionoftheconstructionsiteforawasteincinerationplantplaysacriticalroleinpublic healthandcitydevelopment.ItcanbeeffectivelysolvedbymeansoftheWASPASmethod[38]. WithintheMCDMframework,relevantmathematicalmodelandAHPapproachwereincorporated
intotheWASPASmethod,bywhichthelocationselectionproblemofthegaragewasexplicitly formulated[39].ItsrobustnesswasverifiedbycomparingwithotherMCDMmethodswhenfinding agoodsolution[40].Referringtospecificapplicationsetting,WASPASmethodhasbeengeneralized intoavarietyofassessmentfieldssuchasenergysupplysystem[41],solarprojects[42],third-party logisticsproviders[43]andindoorenvironment[35].ThecombinationoftheWASPASmethodwith fuzzysettheoryhasbeenintroducedintodealingwithuncertaintyundergreyvalues[44],interval type-2fuzzysets[43]orSVNSsenvironment[45].IncorporatingIVIFSsintoWASPASmethod,itcan effectivelydealwiththeMCDMproblems[46].RelativeresearchconcerningtheWASPAStechnique withINSsneedstobefurtherinvestigatedtoenrichtheorybasicsandrepresentmoreuncertain information.Consequently,thispapergeneralizestheWASPAStechniqueintoINSscircumstancesfor matchingthesolar-windpowerstationlocationselectionproblem.
Previousresearcheshaverevealedthatincompleteorunknownweightinformationcommonly existswhenapplyingtheMCDMmethodsintoassessment[47].Fromtheperspectiveofthe objectiveweightdeterminationmethods,maximizingderivationmethod[48],themostwidelyutilized method,cangeneratethecriteriaweightsunderweightspartlyknownorcompletelyunknown circumstances.Correspondingmathematicalprogrammingmodelsareconstructedaccordingto differentcircumstancessuchashesitantfuzzysets[49,50],IFSs[51]andmultipletypesoflinguistic circumstances[52,53].Asoneoftherepresentativesubjectiveweightdeterminationmethods,order relationanalysismethod(G1)determinestheweightinformationbyvirtueofDMs’experience judgement[54].G1notonlyreflectsthesubjectivejudgmentofDMs,butalsopossessesconvenience andfeasibilitycomparingtoAHPmethod.Theseadvantagesareduetoitssimpleacquisitionprocess, andthefactthatthereisnoneedtoconstructjudgementmatrix[54].Toadequatelyreflectmorerealistic informationfromboththesubjectiveandobjectiveaspects,thispaperinvestigatesanintegratedcriteria weightutilizingthecombinationstrengthsofabovedeterminationmethods,andfurtheremploysitto theWASPAStechniqueunderINSsenvironment.
Thereminderofthepaperisstructuredasfollows.InSection 2,somebasicconceptsconcerning INsandINSsareroughlyreviewed.InSection 3,theframeworkoftheextendedWASPAStechniqueis constructedbasedontheobjectiveweightandsubjectiveweightintegratedcriteriaweightinformation. Subsequently,toverifyitsapplicabilitywithinINSsenvironment,theextendedWASPAStechniqueis employedintopracticalsolar-windpowerstationlocationcaseinSection 4.InSection 5,asensitivity analysisandacomparativeanalysisareconductedtofurtherdemonstratetherationalityofthe extendedWASPAStechnique.Finally,conclusionsaresummarizedinSection 6
2.Background
Definition2. [30]Let a1 = aL 1 , aU 1 and a2 = aL 2 , aU 2 betwoINs, L(a1) = au 1 aL 1 and L(a2) = au 2 aL 2 , thenthepossibilitydegreeofa1 ≥ a2 isdenotedas:
p(a1 ≥ a2) = max 1 max aU 2 aL 1 L(a1) + L(a2) ,0 ,0 ,(1)
Considerthereexist m INs ai = aL i , aU i (i = 1,2,..., m) ThepossibilitydegreeofEquation(1)canbe denotedas
p ai ≥ aj = max 1 max aU j aL i L(ai ) + L aj ,0 ,0 ,(2)
whencomparingeachIN ai toallINs aj = aL j , aU j (j = 1,2,..., n) Then,acomplementarypossibilitydegree matrixcanbeestablishedas P ai ≥ aj = p11 p12 p1n p21 p22 p2n . pn1 pn2 pnn
n×n
,(3)
whoseelementssatisfytheconditions pij ≥ 0, pij + pji = 1 and pii = 0.5 Itsrankingvectorcanbederived fromtheequationasfollows: pi =
n ∑ j=1 pij + m 2 1 m(m 1) , (i = 1,2,..., m),(4)
2.2.INSs
Duetothefactthatindeterminacyandinconsistencyinformationcommonlyexistinthedaily life,numerousresearchestackledtheNSsastheinstrumenttomanagethatissue[57,58].However, tofullyandadequatelyindicateuncertaintyandfuzzinessinthereality,INisutilizedastheformto depictthetruth-membership,indeterminacy-membershipandfalsity-membershipinformationofNSs ratherthancrispvalues[59].Inthissection,webrieflyreviewsomebasicsofINSs,whichinvolves operationallaws,aggregationoperatorsandscorefunctions.Fortheconvenienceofexpressingthe reality,INSisdefinedmotivatedbythedefinitionofSVNSasfollows:
[60]Let
x Then, anINSAinXis
thereexiststheconditionsthat
(
(3) a1 × a2 = TL 1 TL 2 , TU 1 TU 2 , I L 1 + I L 2 I L 1 I L 2 , IU 1 + IU 2 IU 1 IU 2 , FL 1 + FL 2 FL 1 FL 2 , FU 1 + FU 2 FU 1 FU 2 , (4) ηa1 = 1 1 TL 1 η ,1 1 TU 1 η , I L 1 η , IU 1 η , FL 1 η , FU 1 η , η > 0, (5) a1 η = TL 1 η , TU 1 η , 1 1 I L 1 η ,1 1 IU 1 η , 1 1 FL 1 η ,1 1 FU 1 η , η > 0.
Definition5. [61]Let aj = TL j , TU j , I L j , IU j , FL j , FU j (j = 1,2,..., n) beapermutationoftheINSs. Then,theintervalneutrosophicpowergeneralizedweightedaggregationoperatorisdefinedas INPGWA(a1, a2, , an )
j 1 + T aj aj η n ∑ j=1 ωj 1 + T aj
1/η ,(6) inwhich ωj = (ω1, ω2, ··· , ωn ) istheassociatedweightvectorof aj (j = 1,2,..., n), ωj ∈ [0,1], n ∑ j=1 ωj = 1; T aj = n ∑ i = 1, i = j
Sup aj, ai , Sup aj, ai isthesupportdegreefor aj from ai; η isaparameterbelongingto (0, +∞).
Theorem1. [61]Let aj = TL j , TU j , I L j , IU j , FL j , FU j (j = 1,2,..., n) beapermutationoftheINSs,the aggregatedresultutilizingtheintervalneutrosophicpowergeneralizedweightedaggregationoperatorderived fromDefinition4isshownas
INPGWA(a1, a2, , an) = 1 n ∏ j=1 1 TL j η wj 1/η , 1 n ∏ j=1 1 TU j η wj 1/η , 1 1 n ∏ j=1 1 I L j η wj 1/η ,1 1 n ∏ j=1 1 IU j η wj 1/η , 1 1 n ∏ j=1 1 FL j η wj 1/η ,1 1 n ∏ j=1 1 FU j η wj 1/η ,
(7)
inwhich wj = ωj (1+T(aj )) n ∑ j=1 ωj (1+T(aj )) Particularly,when η → 0 , theintervalneutrosophicpowergeneralizedweighted
aggregationoperatorreducestoanintervalneutrosophicpowergeometricweightedaggregation(INPGWA) operator,whichisshownas
INPGWA(a1, a2, , an) = n ∏ j=1 TL j wj , n ∏ j=1 TU j wj , 1 n ∏ j=1 1 I L j wj ,1 n ∏ j=1 1 IU j wj , 1 n ∏ j=1 1 FL j wj ,1 n ∏ j=1 1 FU j wj ;
(8)
When η → 1 , theintervalneutrosophicpowergeneralizedweightedaggregationoperatorreducesto anintervalneutrosophicpowerweightedaggregation(INPWA)operator,whichisshownas
INPWA(a1, a2, , an ) = 1 n ∏ j=1 1 TL j wj ,1 n ∏ j=1 1 TU j wj , n ∏ j=1 I L j wj , n ∏ j=1 IU j wj , n ∏ j=1 FL j wj , n ∏ j=1 FU j wj .
Definition6. [62]Let a1 = TL 1 , TU 1 , I L 1 , IU 1 , FL 1 , FU 1 and a2 = TL 2 , TU 2 , I L 2 , IU 2 , FL 2 , FU 2 betwo arbitraryINSs.Then,thenormalizedHammingdistancebetweena1 anda2 canbedefinedas d(a1, a2) = 1 6 TL 1 TL 2 + TU 1 TU 2 + I L 1 I L 2 + IU 1 IU 2 + FL 1 FL 2 + FU 1 FU 2 .(10)
Definition7. [63]Let a = TL , TU , I L , IU , FL , FU beanINS,then,itsscorefunctionaswellas accuracyfunctionandcertaintyfunctionaredefinedas
(1) S(a ) = TL + 1 IU + 1 FU , TU + 1 I L + 1 FL , (2) H(a ) = min TL FL , TU FU ,max TL FL , TU FU , (3) B(a ) = TL , TU .
Itscomparisonrulesarespecificallyintroducedin[63].
3.TheFrameworkofanExtendedWASPASTechnique
Inthissection,anextendedWASPAStechniqueisnewlyinvestigatedtomatchsolar-windpower stationlocationselectionissuewithcompletelyunknowncriteriaweightinformation.
3.1.MaximizingDeviationMethodforObjectiveWeightEstimating
ThemaximizingdeviationmethodwasinitiallypresentedbyWang[64]formanagingMCDM problemsinnumericalcontext.Itsmainidealreliesontheperformancevalueofeachalternative differsundercertaincriteria.Thus,itcanbeinferredthatifcertaincriteriamakestheperformance valuesconcerningallthealternativesapparentlydifferent,thecriteriaplaysacriticalroleinseeking agoodalternativeundertheMCDMcontext.Therefore,byvirtueofthisideal,criteriawithsimilar performancevaluewithrespecttoallthealternativesshouldbeallocatedsmallweight;otherwise,the criteriamakeshugedifferencesoveralternativesshouldbeallocatedbiggerweight.Byaboveanalysis, themaximizingdeviationmethodcanbeappliedintospecificMCDMapplicationasaneffectivetool inderivingcompletelyunknowncriteriaweightinformation.Specially,themodel,whichrevealsthe differencesoftheperformancevalueforeachalternative,canbeestablishedinthefollowingwithin theINScontext.
Withrespecttocertaincriteria Cj ∈ C,theperformancederivationvaluesofalternative xi toall theotheralternativescanbeestablishedasfollows:
m
ij
aij
k=1
kj
, i
1,2, , m, j = 1,2, , n (11) inwhich aij and akj representtheperformancevalueofalternative i andalternative k undercriteria j,andcharacterizedby aij = TL ij , TU ij , I L ij , IU ij , FL ij , FU ij and akj = TL kj, TU kj , I L kj, IU kj , FL kj, FU kj ,respectively.
Furthermore,let
∈
(14) ALagrangefunctioncanbeestablishedtosolvethismodel,andshownas
(
ω , γ)
m ∑ i=1
L
j = n ∑ j=
U kj
2 (16)
Consequently,thenormalizedweightvectorofcriteriacanbederivedas ω ∗ j = ωj n ∑ j=1 ωj ,(j = 1,2,..., n).(17)
Basedonaforementioneddiscussion,wecanobtaincriteriaweightvectorviathesemodelsand equationswithincompletelyorcompletelyunknownweightinformationunderINScontext.
3.2.G1forSubjectiveWeightEstimating
G1[54]isoneofthesubjectiveweightestimationmethods,inwhichalltheweightinformation indexderivedfromsubjectiveevaluationofDMaccordingtotheirexperience.Owingtoitspractical applicability,G1wasadoptedtodisposetheweightinformationintheassessmentofelectricvehicle sharingprograms[65].Specificacquisitionprocesswithinthelocationselectioncaseisoutlined asfollows:
Step1 Determinethecriteriarankingorderrelation. LetDMsprovidetheorderrelationoftheset C = C1, ··· , Cj, ··· , Cn accordingtothe importanceofthecriteriajudgingfromtheirexperience.
Step2 Assigntherelativeimportancedegreeindexofadjacentcriteria. Determinetherelativeimportancedegreeindex rj = ωj 1/ωj oftheadjacentcriteria Cj 1 and Cj accordingtoTable 1.
Step3 CalculatethesubjectiveweightsofcriteriabyEquations(18)and(19). ωn = 1 + n ∑ i=2
n ∏ j=i rj
1 ,(18) ωj = n ∏ k=j+1 rk ωn .(19)
Table1. Therelativeimportancedegreeindexamongadjacentcriteria. rj
Description 1.0 Cj 1 isequallyimportantas Cj 1.2 Cj 1 isslightlymoreimportantthan Cj 1.4 Cj 1 isobviouslymoreimportantthan Cj 1.6 Cj 1 isstronglymoreimportantthan Cj 1.8 Cj 1 isextremelymoreimportantthan Cj 3.3.AnExtendedWASPASTechniquewithIntegratedCriteriaWeightInformation
TheWASPASmethod[34]isawell-knowndecision-makingtechniquewhichcaneffectively increasetherankingaccuracybyintegratingWSMandWPM.Ithasbetteraccuracythanonlyusing oneofWSMorWPM,whichhasbeenprovedin[34].Totalimportanceofanalternativeisdetermined bytheaggregatedWASPASmeasure,whichisinessenceajointcriterionderivedfromtheuseof weightedarithmeticandgeometricaveragingoperatorssimultaneously[66].Thefeasibleranking ordercanbeensuredbyalteringtheparameterbetweenthesumtotalrelativeimportanceandthe producttotalrelativeimportanceofalternativecomputedbythesesoperatorswithinthecompletely unknowncriteriainformation.
Inmostpracticalcases,thecriteriaweightinformationtendstobecompletelyunknown,andthere commonlyexistsrelationshipamongalternatives.Tothisend,thispapercombinestheobjectiveweight
Symmetry 2017, 9,106 9of20
andsubjectiveweightacquisitionmethodsdefinedabovetoreflectmorerealisticweightinformationin theintegratedweightinformationestimationprocedureofthenewlyextendedWASPAStechnique.Itis wellknownthataPAoperatorcaneffectivelyrevealtherelationshipamongalternativesbycalculating supportdegreesfromothers[67].ThePAoperatorisgeneralizedintotheWASPASmethodtooptimize theaggregatedWASPASmeasuredeterminationprocedureinthispaper.Considerthehumanbeings’ expressionpreferenceandtheuncertaintytheyfacedwith,thispaperproposesanextendedWASPAS techniqueinwhichthreeproceduresarefeasiblyimplementedunderINSscontext.Theframeworkof theextendedtechniqueisdisplayedinFigure 1
Symmetry 2017, 9, 106 11 of 20
Figure 1. The framework of the extended WASPAS technique.
Figure1. TheframeworkoftheextendedWASPAStechnique.
4. Case Study
AssumethataMCDMprobleminwhichapermutationof m alternatives {A1, ··· , Ai, ··· , Am } areevaluatedunderapermutationof n criteria C1, ··· , Cj, ··· , Cn
.Theperformanceevaluation ofthe i thalternativeonthe j thcriteriaisassessedbytheINSsdenotedby aij =
4.1. Problem Description
TL ij , TU ij , I L ij , IU ij , FL ij , FU ij
.Themainproceduresareoutlinedasfollows:
Step1. Constructthedecisionmatrix.
With the increasing concern of the regional competition and circumstance protection, solar-wind power station contributes to converting solar or wind resources into power, and further generating electric energy. When assuming that government planners want to build a solar-wind power station for better serving regional socio-economy, a good station construction location should be selected. A group of experts are invited to consist a working team for evaluating four locations Ai () 1,2,,4 i = with respect to various influential factors utilizing numerical rating in the range from 0 to 1. According to relative literature research, this paper concludes its influential factors into five criteria in Table 2. In practical assessment procedure, the evaluation rating not only contains the numerical rating but also the emotional tendency of DMs. For a candidate location i under certain criteria j , the positive maximum evaluation among all the DMs is treated as the
LetaDMprovideperformanceestimationofeveryalternativewithrespecttoallthecriteria, whichisshownas
Step2. Derivethenormalizeddecisionmatrix.
UtilizeEquation(1)inDefinition4toconverttheevaluationundercostcriteriatobenefit criteria.Forconvenience,thenormalizedevaluationsforthe ithalternativewithrespecttothe jthcostcriteriaarealsodenotedby aij = TL ij , TU ij , I L ij , IU ij , FL ij , FU ij
Step3. Calculatetheobjectivecriteriaweight.
UseEquations(16)and(17)tocalculatetheobjectiveweight ω ∗ j foreachcriteriabythe maximizingdeviationmethod.
Step4. Estimatethesubjectivecriteriaweight.
ConducttheproceduresproposedinSection 3.2,andestimatethesubjectivecriteriaweight ωj foreachcriteria.
Step5. Computetheintegratedcriteriaweight.
CombinetheobjectiveandsubjectiveweightsgeneratedfromStep3andStep4,theintegrated criteriaweight ωj isshownas ωj = λω ∗ j + (1 λ)ωj ,(20) inwhich λ istheaggregationparameteralteringin [0,1].
Step6. Calculatesumtotalrelativeimportanceofalternative.
IncorporatetheINPWAoperatordefinedinEquation(9),thesumtotalrelativeimportanceof alternative i iscalculatedbyEquation(21).
Qi = INPWA(a1, a2, , an ).(21)
Step7. Calculateproducttotalrelativeimportanceofalternative.
RefertotheINPGWAoperatorinEquation(8),theproducttotalrelativeimportancefor alternative i isdefinedas
Qi = INPGWA(a1, a2, , an ).(22)
Step8. DeterminetheaggregatedWASPASmeasureforeachalternative.
Aggregate Qi and Q ,thefinalWASPASmeasurecanbedeterminedbytheequationas follows:
Qj = θQi + (1 θ)Qi ,(23)
inwhich θ istheparametertoadjusttheproportionofWSMandWPMintheWASPAS techniquealteringin [0,1].When θ = 1,theWASPAStechniqueisdegeneratedtoWSM.When θ = 0,theWASPAStechniqueisdegeneratedtoWPM.
Step9. Generatethescore,accuracyandcertaintyfunctionvaluesforeachalternative.
Obtainthescore,accuracyandcertaintyfunctionvalues S(ai ), H(ai ) and B(ai ) foreach alternativeutilizingEquation(1)inDefinition7.
Step10. Constructthelikelihoodmatrix.
Constructthepossibilitymatrixofthescorefunctionvalue S(ai ) accordingtoEquation(2), whichisshownasfollows: PS S(ai ) ≥ S aj =
pS 11 pS 12 pS 1n pS 21 pS 22 pS 2n . pS n1 pS n2 pS nn
whoseelements pS ij representsthepossibilitydegreeof S(ai ) ≥ S aj .If pS ij = 0.5,then calculatethepossibilitydegreeof H(ai ) ≥ H aj characterizedby pH ij .If pH ij = 0.5,then calculatethepossibilitydegreeof B(ai ) ≥ B aj characterizedby pB ij.Obtaintheranking vector pi accordingtoEquation(4). Step11. Rankthealternativesandselectthegoodlocation.
Rankallthealternativesandselectthegoodlocationaccordingtothedescendingorderof pi (i = 1,2, ··· , m)
4.CaseStudy
4.1.ProblemDescription
Withtheincreasingconcernoftheregionalcompetitionandcircumstanceprotection,solar-wind powerstationcontributestoconvertingsolarorwindresourcesintopower,andfurthergenerating electricenergy.Whenassumingthatgovernmentplannerswanttobuildasolar-windpower stationforbetterservingregionalsocio-economy,agoodstationconstructionlocationshouldbe selected.Agroupofexpertsareinvitedtoconsistaworkingteamforevaluatingfourlocations Ai (i = 1,2, ,4) withrespecttovariousinfluentialfactorsutilizingnumericalratingintherange from0to1.Accordingtorelativeliteratureresearch,thispaperconcludesitsinfluentialfactorsinto fivecriteriainTable 2.Inpracticalassessmentprocedure,theevaluationratingnotonlycontains thenumericalratingbutalsotheemotionaltendencyofDMs.Foracandidatelocation i under certaincriteria j,thepositivemaximumevaluationamongalltheDMsistreatedasthe TU ij inthe INS aij = TL ij , TU ij , I L ij , IU ij , FL ij , FU ij ,andthenegativeminimumevaluationoftheentireworking teamistreatedas FL ij intheINS.Forinstance,whenratingforlocation A1 withrespecttoeconomic factors C2,themaximumandminimumevaluationfromalltheexperts,whodeemitisappropriate toconstructthestationhere,are0.5,0.3,respectively;themaximumandminimumofinappropriate evaluationare0.8,0.2;themaximumandminimumoftheotherexpertsare0.3,0.2;then,theINS canbeexpressedas a12 = [0.3,0.5], [0.2,0.8], [0.2,0.3] .Basedonthisprinciple,finaldecisionmatrix A = Aij m×n involvingthesyntheticevaluationinformationfromalltheDMsintheworkinggroup isderivedasfollows:
[0.7,0.8], [0.5,0.7], [0.1,0.2] [0.3,0.5], [0.2,0.8], [0.2,0.3] [0.6,0.8], [0.4,0.5], [0.3,0.3] [0.5,0.7], [0.3,0.5], [0.1,0.3] [0.8,0.8], [0.4,0.6], [0.1,0.2] [0.6,0.6], [0.2,0.3], [0.4,0.5] [0.7,0.9], [0.3,0.4], [0.2,0.2] [0.6,0.8], [0.4,0.4], [0.2,0.4] [0.4,0.6], [0.2,0.2], [0.2,0.4] [0.4,0.5], [0.5,0.6], [0.4,0.4] [0.6,0.7], [0.4,0.5], [0.4,0.5] [0.6,0.7], [0.4,0.6], [0.3,0.4] [0.5,0.6], [0.3,0.4], [0.4,0.5] [0.8,0.9], [0.3,0.4], [0.1,0.2] [0.7,0.8], [0.6,0.7], [0.1,0.2] [0.6,0.7], [0.7,0.8], [0.2,0.3] [0.7,0.8], [0.5,0.6], [0.1,0.2] [0.5,0.6], [0.5,0.6], [0.2,0.3] [0.8,0.9], [0.3,0.4], [0.1,0.2] [0.5,0.7], [0.5,0.6], [0.2,0.3]
Table2. Criteriainformationandrelativedescription.
Criteria Cj Description
Naturalresources C1
Economicfactors C2
Trafficconditions C3
Environmentalfactors C4
Socialfactors C5
Naturalresourcesincludevariousindicatorsrelatedtowindandsolar resourcesinthelocation.
Economicfactorsbrieflymeasurethecostduringtheengineering construction,operationandmaintenanceprocedures.
Trafficconditionsreflectthetrafficconveniencetothelocationduring theengineeringconstruction,operationandmaintenanceprocedures.
Environmentalfactorsreflecttheenvironmentdestructionduringthe engineeringconstructionandoperationprocedures.
Socialfactorsreflecttheattitudeofthelocalresidentstotheengineering.
4.2.TheSelectionofSolar-WindPowerStationLocation
Thespecificproceduresofseekingagoodsolar-windpowerstationlocationwiththeextended WASPAStechniqueareimplementedasfollows:
Step1. Constructthedecisionmatrix.
ThedecisionmatrixisconstructedbytheillustrationinSection 4.1,whichisshownas A = Aij 4×5 above. Step2. Derivethenormalizeddecisionmatrix.
ReferringtothecriteriadescriptioninTable 2, C2 and C4 arecostcriteria.UtilizeEquation(1) inDefinition4,thenormalizeddecisionmatrixcanbeobtainedas A = Aij 4×5 =
[0.7,0.8], [0.5,0.7], [0.1,0.2] [0.2,0.3], [0.2,0.8], [0.3,0.5] [0.6,0.8], [0.4,0.5], [0.3,0.3] [0.1,0.3], [0.5,0.7], [0.5,0.7] [0.8,0.8], [0.4,0.6], [0.1,0.2] [0.4,0.5], [0.7,0.8], [0.6,0.6] [0.7,0.9], [0.3,0.4], [0.2,0.2] [0.2,0.4], [0.6,0.6], [0.6,0.8] [0.4,0.6], [0.2,0.2], [0.2,0.4] [0.4,0.4], [0.4,0.5], [0.4,0.5] [0.6,0.7], [0.4,0.5], [0.4,0.5] [0.6,0.7], [0.4,0.6], [0.3,0.4] [0.4,0.5], [0.6,0.7], [0.5,0.6] [0.8,0.9], [0.3,0.4], [0.1,0.2] [0.7,0.8], [0.6,0.7], [0.1,0.2] [0.2,0.3], [0.2,0.3], [0.6,0.7] [0.7,0.8], [0.5,0.6], [0.1,0.2] [0.5,0.6], [0.5,0.6], [0.2,0.3] [0.1,0.2], [0.6,0.7], [0.8,0.9] [0.5,0.7], [0.5,0.6], [0.2,0.3]
Step3. Calculatetheobjectivecriteriaweight.
4×5
UseEquations(16)and(17),thentheobjectiveweight ω ∗ j foreachcriteriacanbecalculatedas ω ∗ 1 = 0.1259, ω ∗ 2 = 0.2122, ω ∗ 3 = 0.2086, ω ∗ 4 = 0.2698, ω ∗ 5 = 0.1835.
Step4. Estimatethesubjectivecriteriaweight. Assumethattheaggregationparameter λ = 0.5,andtheorderrelationofallthecriteriais C4 C2 C1 C5 C3 judgingfromDMs’subjectiveexperience.Referringtotherelative importancedegreeindexamongadjacentcriteriainTable 1,thesubjectivecriteriaweight foreachcriteriaisestimatedas ω1 = 0.3533, ω2 = 0.2945, ω3 = 0.1840, ω4 = 0.1022 and ω5 = 0.0639.
Step5. Computetheintegratedcriteriaweight. CombinetheobjectiveandsubjectiveweightsgeneratedfromStep3andStep4,theintegrated weightofcriteriaisshownas ω1 = 0.2396, ω2 = 0.2534, ω3 = 0.1963, ω4 = 0.1860 and ω5 = 0.1237.
Step6. Calculatesumtotalrelativeimportanceofalternative.
IncorporatetheINPWAoperatordefinedinEquation(9),thesumtotalrelativeimportanceof allalternativesare
Q1 = [0.4986,0.6171], [0.3084,0.6468], [0.1866,0.3342] ,
Q2 = [0.4236,0.6255], [0.4479,0.5977], [0.3746,0.4618] ,
Q3 = [0.6136,0.6642], [0.5212,0.6680], [0.2643,0.3665]
Q4 = [0.4867,0.7214], [0.4433,0.5128], [0.3468,0.4122]
Step7. Calculateproducttotalrelativeimportanceofalternative.
RefertotheINPGWAoperatorinEquation(8),theproducttotalrelativeimportancefor alternativesare
Q1 = [0.3816,0.4983], [0.3562,0.7138], [0.2219,0.3815] ,
Q2 = [0.2638,0.4970], [0.4566,0.6189], [0.4046,0.5409] ,
Q3 = [0.5184,0.6005], [0.5784,0.7099], [0.4203,0.4605]
Q4 = [0.3533,0.5616], [0.4852,0.5355], [0.4509,0.6134]
Step8. DeterminetheaggregatedWASPASmeasureforeachalternative.
Let θ = 0.5,thenthefinalWASPASmeasurecanbederivedas
Q1 = [0.4401,0.5577], [0.3323,0.6803], [0.2043,0.3579] ,
Q2 = [0.3437,0.5613], [0.4523,0.6083], [0.3896,0.5014] ,
Q3 = [0.5660,0.6324], [0.5498,0.6889], [0.3423,0.4135]
Q4 = [0.4200,0.6415], [0.4643,0.5241], [0.3989,0.5129]
Step9. Generatethescore,accuracyandcertaintyfunctionvaluesforeachalternative.
Foreachalternative,thescore,accuracyandcertaintyfunctionvalues S(ai ), H(ai ) and B(ai ) areshowninTable 3
Table3. Relativefunctionvaluesofalternatives.
Ai S(ai ) H(ai ) B(ai )
A1 [1.4020,2.0212][0.1998,0.2359][0.4401,0.5577]
A2 [1.2341,1.7194][ 0.0459,0.0599][0.3437,0.5613]
A3 [1.4636,1.7402][0.2189,0.2237][0.5660,0.6324]
A4 [1.3831,1.7783][0.0212,0.1286][0.4200,0.6415]
Step10. Constructthelikelihoodmatrix.
Constructthepossibilitymatrixofthescorefunctionvalue S(ai ) accordingtoEquation(2), whichisshownasfollows: PS S(ai ) ≥ S a
0.50.71260.62240.6290 0.71260.50.33580.3820 0.62240.33580.50.5315 0.62900.38200.53150.5
Andtherankingvectorcanbeobtainedas p1 = 2.5981, p2 = 2.1977, p3 = 2.2423 and p4 = 2.2819. Step11. Rankthealternativesandselectthegoodlocation. Therankingorderofallthealternativesis A1 A4 A3 A2 andthegoodlocationis A1
5.SensitivityAnalysisandComparisonAnalysis
Inthissection,comparisonanalysisandsensitivityanalysisareconductedtofurthertestify theeffectivenessandreliabilityoftheextendedWASPAStechniquewithexistingmethodsonthe sameexample.
5.1.SensitivityAnalysisandDiscussion
Todemonstratetheimpactofdifferentaggregationparameter λ andtheproportionadjustment parameter θ onthefinallocationselectionresults,weconductthesensitivityanalysisonthesame examplewithfive λ(0,0.2,0.5,0.8,1) valuesandfive θ(0,0.2,0.5,0.8,1) valuessimultaneously.The rankingresultsfordifferent λ and θ aredisplayedinTable 4. Table4. Rankingresultsfordifferent λ and θ λθ =
θ = 0.5 θ = 0.8 θ = 1 0 A1 A4
2 A1 A4 A3 A2 A1 A4 A3 A2 A1 A4 A3 A2 A1 A4 A3 A2 0.2 A4 A1 A3 A2 A4 A1 A3 A2 A1 A4 A3 A2 A1 A4 A3 A2 A1 A4 A3 A2 0.5 A4 A1 A3 A2 A4 A1 A3 A2 A4 A1 A3 A2 A4 A1 A3 A2 A4 A1 A3 A2 0.8 A4 A1 A3 A2 A4 A1 A3 A2 A1 A4 A3 A2 A1 A4 A3 A2 A1 A4 A3 A2 1 A4 A1 A3 A2 A4 A1 A3 A2 A4 A1 A3 A2 A4 A1 A3 A2 A4 A1 A3 A2
Thevariationof λ representstheinfluenceofcriteriaweightintegratedbyobjectiveandsubjective weightstofinalselectionresults.Inaddition,alteringthe θ valuesmeansthatthechangefromsum totalrelativeimportancetoproducttotalrelativeimportance.SynthetizingwhatisshowninTable 4, itcanbeinferredthatdifferent λ and θ valuesindeedaffectfinalselectionresults.When λ = 0 and λ = 1,theintegratedcriteriaweightiscompletesubjectiveorobjectiveweight,andtherankingorder hasdifferentselectionresults A1 and A4 undertheseoppositecontext.Furthermore,nomatterhow thevariationof θ values,therankingorderremainsallthesameinthesecircumstances.When λ = 0.2 and λ = 0.8,theproportionadjustmentparameter θ valuesmakehugedifferencestotherankingorder. Therankingresultyields A4 A1 A3 A2 when 0 ≤ θ < 0.5,whileityields A1 A4 A3 A2 when 0.5 ≤ θ ≤ 1.Inviewoftheidenticalchangeabletendencyofrankingorder,itisapparentthat thegradualincreaseoftheadjustmentproportion θ clearlyaffectstheorderwhendifferentimportance oftheobjectiveandsubjectiveweightsintheintegratedweight.However,whentheimportanceof theobjectiveandsubjectiveweightsintheintegratedweightaretotallyequivalent,therankingorder keeps A4 A1 A3 A2 allthetime.
Obviously,theserankingorderandselectionresultsrevealthatdifferent λ and θ valueshave aneffectonthedecision-makingprocedure.Itfurtherindicatesthatthecombinationofobjectiveand subjectiveweights,andtheintegrationofsumtotalrelativeimportanceandproducttotalrelative importanceemphasizetheinfluencetotheselectionprocedure.Integratedthespecificmeaningof λ and θ withtheirroleplayinginthedecision-makingprocedures,thelocationselectionbecomes adynamicprocedurebysettingdifferentparametervaluesderivedfrompracticalcontext.
5.2.ComparisonAnalysisandDiscussion
Thissubsectionfurthervalidatestheeffectivenessandreliabilityofthenewlyproposedmethod bycomparingitwithdifferentexistingmethodsontheidenticalillustrativeexample.Theranking resultsareshowninTable 5.Asthereexistsnorelativecriteriaweightobtainmentmethodin[34,62,68]
chosenforcomparison,weusetheintegratedcriteriaweightobtainedinthispaperasthecriteria weightinformationforthecomparisonconvenience.
Table5. Rankingresultswithdifferentexistingmethods.
MethodsRankingResults
Similaritymeasurein[62]
Methodusedweightedarithmeticaggregationoperatorin[68]
Methodusedweightedgeometricaggregationoperatorin[68]
A3 A4 A2 A1
A4 A2 A3 A1 AnextendedTOPSISmethodin[69]
A4 A1 A3 A2
A4 A1 A3 A2 ClassicalWASPASmethodin[34]
A4 A1 A3 A2 Theproposedmethod A1 A4 A3 A2
(1) Themethodin[62]generatestheselectionresultbyimplementingtwosteps.Firstly,confirm theidealalternativesfordifferenttypeofcriteria.Subsequently,derivetheresultbysimilarity measures.Accordingtothefirstsimilaritymeasureinthatliterature,thesimilaritymeasures areobtainedas S∗ 1 (A∗ , A1) = 0.8178, S∗ 1 (A∗ , A2) = 0.8705, S∗ 1 (A∗ , A3) = 0.8692 and S∗ 1 (A∗ , A4) = 0.9057.
(2) Inthemethodof[69],maximizingdeviationmethodisutilizedtoderiveobjectiveweights.Then, basedontheidealofTOPSISmethod,alternativesarerankedbytherelativeclosenesscoefficient. Conducttheseprocedures,relativeclosenesscoefficientcanbecalculatedas RCC1 = 0.5226, RCC2 = 0.5186, RCC3 = 0.5190and RCC4 = 0.5189.
(3) Theprocedureofthemethodin[68]canbebrieflyclassifiedintoaggregationprocessandranking process.Therankingprocedurein[68]isidenticalwithourproposedmethod.Basedonweighted arithmeticaggregationoperatororweightedgeometricaggregationoperator,thetotalscoreare obtainedas ps = [2.2997,2.0098,2.0589,2.5926] or ps = [2.5804,2.2751,2.3728,2.9288] withthe samerankingorder A4 A1 A3 A2.
(4) ClassicalWASPASmethodin[34]generatesthefinalWASPASmeasurebyaggregatingweighted arithmeticaggregationoperatorandweightedgeometricaggregationoperator.Tobettercompare theclassicalWASPASmethodwithourmethod,thealternativesarerankedbytheranking procedureinourpaper.Then,rankingvectorcanbeobtainedas p1 = 2.4449, p2 = 2.1483, p3 = 2.2189and p4 = 2.7681.
ItcanbeeasilyinferredfromTable 5 thatdifferentmethodsgeneratetotallydistinctselection results.Especially,theordersequencederivedfromthemethodsin[62,69]isentirelyoppositetoours. Thegoodlocationselectionfor[62,69]andourpaperare A4, A3 and A1,respectively.Theprimary reasonforthatphenomenonreliesonthatthesemethodswereproposedbasedoncompletelydifferent ideal.Itresultsintheinconsistentsequencebetweeneachpairofalternativesinthesemethods.Both thesimilaritymeasuresandTOPSISmethodrankthealternativesontheidealofdistance.Forabetter comparisonanalysis,weadoptHammingdistanceduringthecalculationprocedure.Moreover,the methodin[62]neglectstherelationofcriteriatoeachother,whilethemethodin[69]onlyconsidersthe objectivecriteriaweightwithoutfocusingonthesubjectivepreferenceofDMs.Theproposedmethod emphasizesthesupportrelationfromothercriteriainthecourseofWASPASmeasuredetermination. Itadequatelyconsiderstheobjectivecriteriaweightsandsubjectiveexperiencejudgementfrom DMsbyintegratedcriteriaweight.Theselection A4 and A3 cannotreflectrealisticsituationwithout consideringthesubjectivecriteriaduringtheMCDMprocedure.Inaddition,thesecharacteristics ensuretheeffectivenessandreliabilityofthelocationselection A1 derivedfromourmethod.Although boththemethodin[68]andourmethodutilizeanidenticalrankingmethod,theaggregationoperators causehugedifferencestothefinallocationselection.Whenusingoneoftheweightedarithmetic aggregationoperatororweightedgeometricaggregationoperatorin[68],ityieldsidenticalselection result A4.However,theproposedmethodnotonlycontainstheadvantageofPAoperator,butalso effectivelyutilizesthecombinationofINPGWAandINPWAratherthanapplyingthemrespectively.
Besides,theextendedWASPAStechniquerevealsitssuperioritybyimplementingthreeproceduresto assuretheselectionresult A1 rationalandconvincing.
Utilizingidenticalaggregationoperator,methodsin[34,68]providethetotallysameranking order A4 A1 A3 A2 withidenticallocationselection A4.Itindicatesthatthesuperiorityofthe classicalWASPASmethodcannotbeinferredbecausethereexistsnorankingorselectiondiscrepancy whenusingcompletelydifferentmethods.Itisobviousthattherankingandselectionresultsofthe classicalWASPASmethodandtheproposedmethodexistsdiscrepancy.Theorderof A1 and A4 is oppositeinthosemethods.Thatis,thoughboththeclassicalandextendedWASPASmethodshavethe sameprocedureinwhichtheWASPASmeasureareaggregatedbytwokindsofoperators,theextended WASPASmethodreflectsamorerationalselectionresultbynewlyincorporatingtheadvantageofPA operatorsinthat.Furthermore,theintegratedweightinformationestimationprocedurefurtherensures theselectionoftheextendedWASPASmethodscientific.Theidenticalorderbetweenalternatives A3 and A2 inthesemethodscanverifytheeffectivenessoftheextendedone.
Fromabovediscussion,primaryhighlightsofthenewlyextendedWASPAStechniquecanbe simplysummarizedintothefollowingpoints.
(1) Itcaneffectivelymanagethesolar-windpowerstationlocationproblemviaembeddingthree proceduresintothenewlyextendedWASPAStechnique.DuringtheWASPAStechnique implementprocess,arationallocationselectionresultwillbegeneratedbyincorporatingthe advantagesofrelevantmethodsintheseprocedures.
(2) Withthemaximizingderivationmethod,objectivecriteriaweightscanbesimplydeterminedno matterunderthecriteriaweightscompletelyunknownorincompletecircumstances.Apartfrom theobjectivecriteriaweights,subjectiveweights,whichfullyreflectthesubjectivepreference underpractice,canbeobtainedwithG1.Theintegratedcriteriaweightisthecombinationofthe objectiveandsubjectiveweights,andcanadequatelyrepresentmorerealisticsituation.
(3) Differentaggregationparameter λ andtheproportionadjustmentparameter θ facilitatethe wholeproceduresadynamicselection.Theparametersettingisbasedontherequirementofreal applicationandsubjectivepreferenceofDMs,whichmakestheextendedWASPAStechnique feasibleindealingwiththereality.
6.Conclusions
Agoodlocationofthesolar-windpowerstationcanaffectregionalcompetitivenessanddirect futuredevelopmenttoagreatextent.Facedwithmultipleuncertaintiesinreality,thelocationselection caseisconsideredwithintheINSscircumstancesfortacklingsuchchallenges.Recognizedasacomplex MCDMprocedure,theselectioninthispaperissettledbyanextendedWASPAStechniquecontaining threeprocedurestoreinforceitsapplicabilitytorealsituation.Formodellingmorerealisticinformation, somemodificationsaremadeintheclassicalWASPASmethodespeciallyutilizingtheobjectiveand subjectivecriteriaweightintegratedweightinformation.Itsstrengthshavebeenadequatelydiscussed viacomparisonanalysisandsensitivityanalysis.
Highlightsoftheextendedtechniquecanbebrieflysummarizedinthreeaspects.Firstly,itensures arelativelyrationalandscientificresultbyincorporatingthreeproceduresintotheframeworkofthe technique.Secondly,theintegratedweightinformationreflectsmorerealisticweightinformation withthecombinationoftheobjectiveandsubjectivecriteriaweightinformation.Thirdly,more practicalcontextscanbereflectedbyalteringtheaggregationparameterandtheproportionadjustment parameterduringperformingrelevantprocedures.Althoughthelocationselectioncasecanbewell measuredbythecriteriadimensionsinthispaper,potentialworkshouldalsobefocusedonspecific sub-criteriainformationforreachingamorepromisingsolution.
Symmetry 2017, 9,106
Acknowledgments: ThisworkwassupportedbytheNationalNaturalScienceFoundationofChina (No.71571193),andsupportedbytheFundamentalResearchFundsfortheCentralUniversitiesofCentral SouthUniversity(No.2017zzts284).
AuthorContributions: Jian-qiangWangandRu-xinNieconceivedandworkedtogethertoachievethiswork, Ru-xinNiewrotethepaper,Hong-yuZhangmadecontributiontothecasestudy.
ConflictsofInterest: Theauthorsdeclarenoconflictofinterest.
References
1. Khare,V.;Nema,S.;Baredar,P.StatusofsolarwindrenewableenergyinIndia. Renew.Sustain.EnergyRev. 2013, 27,1–10.[CrossRef]
2. Khare,V.;Nema,S.;Baredar,P.Solar–windhybridrenewableenergysystem:Areview. Renew.Sustain. EnergyRev. 2016, 58,23–33.[CrossRef]
3. Kazem,H.A.;Al-Badi,H.A.S.;AlBusaidi,A.S.;Chaichan,M.T.Optimumdesignandevaluationofhybrid solar/wind/dieselpowersystemforMasirahIsland. Environ.Dev.Sustain. 2016,1–18.[CrossRef]
4. Petrakopoulou,F.;Robinson,A.;Loizidou,M.Simulationandevaluationofahybridconcentrating-solar andwindpowerplantforenergyautonomyonislands. Renew.Energy 2016, 96,863–871.[CrossRef]
5. Jahangiri,M.;Ghaderi,R.;Haghani,A.;Nematollahi,O.Findingthebestlocationsforestablishmentof solar-windpowerstationsinMiddle-EastusingGIS:Areview. Renew.Sustain.EnergyRev. 2016, 66,38–52. [CrossRef]
6. Lee,A.H.I.;Kang,H.Y.;Liou,Y.J.Ahybridmultiple-criteriadecision-makingapproachforphotovoltaicsolar plantlocationselection. Sustainability 2017, 9,184.[CrossRef]
7. Roy,B. MulticriteriaMethodologyforDecisionAiding;SpringerScience&BusinessMedia:Dordrecht, TheNetherlands,2013.
8. Roy,B.Paradigmsandchallenges.In MultipleCriteriaDecisionAnalysis:STATEoftheArtSurveys;Figueira,J., Greco,S.,Ehrgott,M.,Eds.;Springer:NewYork,NY,USA,2005;Volume78,pp.3–24.
9. Roy,B.;Słowi´nski,R.Questionsguidingthechoiceofamulticriteriadecisionaidingmethod. EUROJ. Decis.Process. 2013, 1,69–97.[CrossRef]
10. Abudeif,A.M.;AbdelMoneim,A.A.;Farrag,A.F.Multicriteriadecisionanalysisbasedonanalytichierarchy processinGISenvironmentforsitingnuclearpowerplantinEgypt. Ann.Nucl.Energy 2015, 75,682–692. [CrossRef]
11. Latinopoulos,D.;Kechagia,K.AGIS-basedmulti-criteriaevaluationforwindfarmsiteselection.Aregional scaleapplicationinGreece. Renew.Energy 2015, 78,550–560.[CrossRef]
12. Liu,J.;Xu,F.;Lin,S.Siteselectionofphotovoltaicpowerplantsinavaluechainbasedongreycumulative prospecttheoryforsustainability:AcasestudyinNorthwestChina. J.Clean.Prod. 2017, 148,386–397. [CrossRef]
13. Wu,Y.;Chen,K.;Zeng,B.;Yang,M.;Li,L.;Zhang,H.Aclouddecisionframeworkinpure2-tuplelinguistic settinganditsapplicationforlow-speedwindfarmsiteselection. J.Clean.Prod. 2017, 142,2154–2165. [CrossRef]
14. Wu,Y.;Zhang,J.;Yuan,J.;Geng,S.;Zhang,H.Studyofdecisionframeworkofoffshorewindpower stationsiteselectionbasedonELECTRE-IIIunderintuitionisticfuzzyenvironment:AcaseofChina. EnergyConvers.Manag. 2016, 113,66–81.[CrossRef]
15. Jun,D.;Tian-tian,F.;Yi-sheng,Y.;Yu,M.Macro-siteselectionofwind/solarhybridpowerstationbasedon ELECTRE-II. Renew.Sustain.EnergyRev. 2014, 35,194–204.[CrossRef]
16. Yunna,W.;Geng,S.Multi-criteriadecisionmakingonselectionofsolar-windhybridpowerstationlocation: AcaseofChina. EnergyConvers.Manag. 2014, 81,527–533.[CrossRef]
17. Zhang,H.Y.;Peng,H.G.;Wang,J.;Wang,J.Q.Anextendedoutrankingapproachformulti-criteria decision-makingproblemswithlinguisticintuitionisticfuzzynumbers. Appl.SoftComput. 2017, 59,462–474. [CrossRef]
18. Turksen,I.B.Intervalvaluedfuzzysetsbasedonnormalforms. FuzzySetsSyst. 1986, 20,191–210.[CrossRef]
19. Nie,R.;Wang,J.;Li,L.2-tuplelinguisticintuitionisticpreferencerelationanditsapplicationinsustainable locationplanningvotingsystem. J.Intell.FuzzySyst. 2017.[CrossRef]
Symmetry 2017, 9,106
20.
Cao,Y.X.;Zhou,H.;Wang,J.Q.Anapproachtointerval-valuedintuitionisticstochasticmulti-criteria decision-makingusingsetpairanalysis. Int.J.Mach.Learn.Cybern. 2016,1–12.[CrossRef]
21. Tian,Z.P.;Wang,J.;Wang,J.Q.;Zhang,H.Y.AnimprovedMULTIMOORAapproachformulti-criteria decision-makingbasedoninterdependentinputsofsimplifiedneutrosophiclinguisticinformation. NeuralComput.Appl. 2016.[CrossRef]
22. Smarandache,F. Neutrosophy:NeutrosophicProbability,Set,andLogic;AmericanResearchPress:Rehoboth, DE,USA,1998;pp.1–105.
23. Peng,H.;Zhang,H.;Wang,J.Probabilitymulti-valuedneutrosophicsetsanditsapplicationinmulti-criteria groupdecision-makingproblems. NeuralComput.Appl. 2016,1–21.[CrossRef]
24. Tian,Z.P.;Wang,J.;Wang,J.Q.;Zhang,H.Y.Simplifiedneutrosophiclinguisticmulti-criteriagroup decision-makingapproachtogreenproductdevelopment. GroupDecis.Negot. 2017, 26,597–627.[CrossRef]
25. Smarandache,F.AUnifyingFieldinLogics:NeutrosophicLogic. Mult.Log. 1999, 8,489–503.
26. Liu,C.Intervalneutrosophicfuzzystochasticmulti-criteriadecision-makingmethodsbasedonMYCIN certaintyfactorandprospecttheory. Rev.Tec.Fac.Ing.Univ.DelZulia 2017, 39,52–58.
27.
Ma,H.;Hu,Z.G.;Li,K.Q.;Zhang,H.Y.Towardtrustworthycloudserviceselection:Atime-awareapproach usingintervalneutrosophicset. ParallelDistrib.Comput. 2016, 96,75–94.[CrossRef]
28. Reddy,R.;Reddy,D.;Krishnaiah,G.LeansupplierselectionbasedonhybridMCGDMapproachusing intervalvaluedneutrosophicsets:Acasestudy. Int.J.Innov.Res.Dev. 2016, 5,291–296.
29.
Ma,Y.X.;Wang,J.Q.;Wang,J.;Wu,X.H.Anintervalneutrosophiclinguisticmulti-criteriagroup decision-makingmethodanditsapplicationinselectingmedicaltreatmentoptions. NeuralComput.Appl. 2016,1–21.[CrossRef]
30.
Tian,Z.P.;Zhang,H.Y.;Wang,J.;Wang,J.Q.;Chen,X.H.Multi-criteriadecision-makingmethodbasedon across-entropywithintervalneutrosophicsets. Int.J.Syst.Sci. 2016, 47,3598–3608.[CrossRef]
31. Peng,J.J.;Wang,J.Q.;Yang,L.J.;Qian,J.Anovelmulti-criteriagroupdecision-makingapproachusing simplifiedneutrosophicinformation. Int.J.Uncertain.Quantif. 2017.[CrossRef]
32. Wu,X.H.;Wang,J.Q.Cross-entropymeasuresofmulti-valuedneutrosophicsetsanditsapplicationin selectingmiddle-levelmanager. Int.J.Uncertain.Quantif. 2017, 2,155–172.[CrossRef]
33.
Tian,Z.P.;Wang,J.;Zhang,H.Y.;Wang,J.Q.Multi-criteriadecision-makingbasedongeneralizedprioritized aggregationoperatorsundersimplifiedneutrosophicuncertainlinguisticenvironment. Int.J.Mach. Learn.Cybern. 2016,1–17.[CrossRef]
34.
35.
36.
Zavadskas,E.K.;Turskis,Z.;Antucheviciene,J.;Zakarevicius,A.Optimizationofweightedaggregatedsum productassessment. Electron.Electr.Eng. 2012, 122,1–3.[CrossRef]
Zavadskas,E.K.;Kalibatas,D.;Kalibatiene,D.Amulti-attributeassessmentusingWASPASforchoosing anoptimalindoorenvironment. Arch.Civ.Mech.Eng. 2016, 16,76–85.[CrossRef]
Zavadskas,E.K.;Baušys,R.;Stanujkic,D.;Magdalinovic-Kalinovic,M.Selectionoflead-zincflotationcircuit designbyapplyingWASPASmethodwithsingle-valuedneutrosophicset. ActaMontan.Slovaca 2017, 21, 85–92.
37. Bagoˇcius,V.;Zavadskas,E.K.;Turskis,Z.Multi-personselectionofthebestwindturbinebasedonthe multi-criteriaintegratedadditive-multiplicativeutilityfunction. J.Civ.Eng.Manag. 2014, 20,590–599. [CrossRef]
38. Zavadskas,E.K.;Baušys,R.;Lazauskas,M.Sustainableassessmentofalternativesitesfortheconstructionof awasteincinerationplantbyapplyingWASPASmethodwithsingle-valuedneutrosophicset. Sustainability 2015, 7,15923–15936.[CrossRef]
39. Baušys,R.;Juodagalviene,B.GaragelocationselectionforresidentialhousebyWASPAS-SVNSmethod. J.Civ.Eng.Manag. 2017, 23,421–429.[CrossRef]
40. Zavadskas,E.K.;Antucheviciene,J.;Saparauskas,J.;Turskis,Z.MCDMmethodsWASPASand MULTIMOORA:Verificationofrobustnessofmethodswhenassessingalternativesolutions. Econ.Comput. Econ.Cybern.Stud.Res. 2013, 47,5–20.
41. Džiugaitetumeniene,R.;Lapinskiene,V.Themulticriteriaassessmentmodelforanenergysupplysystemof alowenergyhouse. Eng.Struct.Technol. 2014, 6,33–41.[CrossRef]
42. Vafaeipour,M.;Zolfani,S.H.;Derakhti,A.;Eshkalag,M.K.Assessmentofregionspriorityforimplementation ofsolarprojectsinIran:Newapplicationofahybridmulti-criteriadecisionmakingapproach. EnergyConvers.Manag. 2014, 86,653–663.[CrossRef]
Symmetry 2017, 9,106
43. Ghorabaee,M.K.;Amiri,M.;Zavadskas,E.K.;Antucheviˇciene,J.Assessmentofthird-partylogistics providersusingaCRITIC–WASPASapproachwithintervaltype-2fuzzysets. Transport 2017, 32,66–78. [CrossRef]
44.
Zavadskas,E.K.;Turskis,Z.;Antucheviciene,J.Selectingacontractorbyusinganovelmethodfor multipleattributeanalysis:Weightedaggregatedsumproductassessmentwithgreyvalues(WASPASG). Stud.Inform.Control. 2015, 24,141–150.[CrossRef]
45. Mardani,A.;Nilashi,M.;Zakuan,N.;Loganathan,N.;Soheilirad,S.;Saman,M.Z.M.;Ibrahim,O. Asystematicreviewandmeta-AnalysisofSWARAandWASPASmethods:Theoryandapplicationswith recentfuzzydevelopments. Appl.SoftComput. 2017, 57,265–292.[CrossRef]
46. Zavadskas,E.K.;Antucheviciene,J.;RazaviHajiagha,S.H.;Hashemi,S.S.Extensionofweightedaggregated sumproductassessmentwithinterval-valuedintuitionisticfuzzynumbers(WASPAS-IVIF). Appl.SoftComput. 2014, 24,1013–1021.[CrossRef]
47. Wei,G.W.Maximizingdeviationmethodformultipleattributedecisionmakinginintuitionisticfuzzysetting. Knowl.BasedSyst. 2008, 21,833–836.[CrossRef]
48. ¸Sahin,R.;Liu,P.Maximizingdeviationmethodforneutrosophicmultipleattributedecisionmakingwith incompleteweightinformation. NeuralComput.Appl. 2016, 27,2017–2029.[CrossRef]
49. Gitinavard,H.;Mousavi,S.M.;Vahdani,B.Anewmulti-criteriaweightingandrankingmodelfor groupdecision-makinganalysisbasedoninterval-valuedhesitantfuzzysetstoselectionproblems. NeuralComput.Appl. 2016, 27,1593–1605.[CrossRef]
50. Xu,Z.;Zhang,X.Hesitantfuzzymulti-attributedecisionmakingbasedonTOPSISwithincompleteweight information. Knowl.BasedSyst. 2013, 52,53–64.[CrossRef]
51. Chen,Z.;Yang,W.Anewmultipleattributegroupdecisionmakingmethodinintuitionisticfuzzysetting. Appl.Math.Model. 2011, 35,4424–4437.[CrossRef]
52. Nguyen,H.T.;Md,D.S.;Nukman,Y.;Aoyama,H.;Case,K.Anintegratedapproachoffuzzylinguistic preferencebasedAHPandfuzzyCOPRASformachinetoolevaluation. PLoSONE 2015, 10,e133599. [CrossRef][PubMed]
53. Zhang,W.;Xu,Y.;Wang,H.Aconsensusreachingmodelfor2-tuplelinguisticmultipleattributegroup decisionmakingwithincompleteweightinformation. Int.J.Syst.Sci. 2016, 47,389–405.[CrossRef]
54. Ruan,C.;Yang,J.Softwarequalityevaluationmodelbasedonweightedmutationratecorrection incompletionG1combinationweights. Math.Probl.Eng. 2014, 2014,1–9.[CrossRef]
55. Wang,J.;Li,Y.;Zhou,Y.Intervalnumberoptimizationforhouseholdloadschedulingwithuncertainty. EnergyBuild. 2016, 130,613–624.[CrossRef]
56.
Xu,Z.Dependentuncertainorderedweightedaggregationoperators. Inf.Fusion 2008, 9,310–316.[CrossRef]
57. Wang,J.;Yang,Y.;Li,L.Multi-criteriadecision-makingmethodbasedonsingle-valuedneutrosophic linguisticMaclaurinsymmetricmeanoperators. NeuralComput.Appl. 2016,1–19.[CrossRef]
58. Liang,R.X.;Wang,J.Q.;Li,L.Multi-criteriagroupdecision-makingmethodbasedoninterdependentinputs ofsingle-valuedtrapezoidalneutrosophicinformation. NeuralComput.Appl. 2016,1–20.[CrossRef]
59. Broumi,S.;Smarandache,F.Newoperationsonintervalneutrosophicsets. J.NewTheory 2015, 2,62–71.
60.
61.
62.
Liu,P.;Wang,Y.IntervalneutrosophicprioritizedOWAoperatoranditsapplicationtomultipleattribute decisionmaking. J.Syst.Sci.Complex. 2015, 3,681–697.[CrossRef]
Liu,P.;Tang,G.Somepowergeneralizedaggregationoperatorsbasedontheintervalneutrosophicsetsand theirapplicationtodecisionmaking. J.Intell.FuzzySyst. 2016, 30,2517–2528.[CrossRef]
Ye,J.Similaritymeasuresbetweenintervalneutrosophicsetsandtheirapplicationsinmulticriteria decision-making. J.Intell.FuzzySyst. 2014, 26,165–172.
63. Peng,J.J.;Wang,J.Q.;Wang,J.;Zhang,H.Y.;Chen,X.H.Simplifiedneutrosophicsetsandtheirapplications inmulti-criteriagroupdecision-makingproblems. Int.J.Syst.Sci. 2016, 47,2342–2358.[CrossRef]
64. Yingming,W.Usingthemethodofmaximizingdeviationtomakedecisionformultiindices. J.Syst. Eng.Electron. 1997, 8,21–26.
65. Xu,F.;Liu,J.;Lin,S.;Yuan,J.AVIKOR-basedapproachforassessingtheserviceperformanceofelectric vehiclesharingprograms:AcasestudyinBeijing. J.Clean.Prod. 2017, 148,254–267.[CrossRef]
66. Chakraborty,S.;Zavadskas,E.K.ApplicationsofWASPASmethodinmanufacturingdecisionmaking. Informatica 2014, 25,1–20.[CrossRef]
67. Wang,L.;Shen,Q.;Zhu,L.DualhesitantfuzzypoweraggregationoperatorsbasedonArchimedean t-conormandt-normandtheirapplicationtomultipleattributegroupdecisionmaking. Appl.SoftComput. 2016, 38,23–50.[CrossRef]
68. Zhao,A.;Du,J.;Guan,H.Intervalvaluedneutrosophicsetsandmulti-attributedecision-makingbasedon generalizedweightedaggregationoperator. J.Intell.FuzzySyst. 2015, 6,2697–2706.
69. Chi,P.;Liu,P.AnextendedTOPSISmethodforthemultipleattributedecisionmakingproblemsbasedon intervalneutrosophicset. NeutrosophicSetsSyst. 2013, 1,63–70.
© 2017bytheauthors.LicenseeMDPI,Basel,Switzerland.Thisarticleisanopenaccess articledistributedunderthetermsandconditionsoftheCreativeCommonsAttribution (CCBY)license(http://creativecommons.org/licenses/by/4.0/).
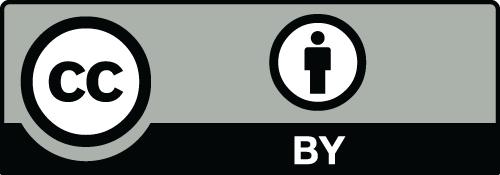