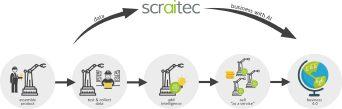
3 minute read
AI at the edge has a very small footprint
ARTIFICIAL INTELLIGENCE
AI at the edge has a small footprint
Advertisement
At a recent Festo press event, Tanja Maaß, managing director at Resolto Informatik, spoke about the benefits of bringing artificial intelligence directly to the machine. Suzanne Gill reports.
While artificial Intelligence detect anomalies to lower the risk of (AI) is starting to play breaking a contract through machine a much bigger role in failure.” Taking this thinking to the industry, Maaß was quick next stage, Maaß pointed out that if it to point out that the requirements was possible to offer a 100% guarantee of the machine builder (OEM) and that a machine will always be available end-user will be very different. She it could make the product-as-a-service said: “For the end-user, AI is probably model more attractive to end-users. most often associated with predictive Achieving 100% guaranteed machine maintenance applications. But it could availability requires real-time data have many more uses – helping speed and Maaß pointed out that any data up production lines or to reduce waste, analysed in the cloud will only ever for example. answer questions retrospectively. “Even
“End users could also use AI if you are monitoring data continuously technology to help as part of an in the cloud it will still always have a intelligent energy optimisation solution slight latency to it,” she said. “Historical and for peak load forecasting. Early data can only ever answer questions anomaly detection is another good about an event that has already application for AI. Using an algorithm happened. in place of a programme will result in “Live data, monitored close to the a system that is able to learn through field, however, allows us to predict experience to identify anomalies in a what will happen. These real-time process.” machine learning solutions are able
In the machine building sector AI can to learn healthy behaviour patterns be utilised to create service offerings. and recognise emerging unhealthy Maaß explained: “As margins on behaviour patterns. With an AI hardware offerings reduce, having the algorithm it should be possible to ability to offer a service would give not only ask for recommendations to OEMs a valuable new repeating income prevent potential events, but to ask it stream and the use of AI will help to act automatically to avoid an event.”
The SCRAITEC platform from Resolto Informatik is able to collect data, analyse it, optimise it and then present the solution in some way. Since the company was acquired by Festo several years ago, it has focused on applications that use SCRAITEC algorithms on Festo hardware, such as the CPX controller and the IoT gateway.
In one such application, Resolto was approached to provide a predictive maintenance solution to allow an automotive company to predict wear and cycle time deterioration on pneumatic clamping units. This application quickly turned into an optimisation solution. “When we applied the data to SCRAITEC it identified an unexpected unhealthy behaviour pattern in one part which was found to be losing the company the equivalent of one car every day. SCRAITEC on a Festo CPX E CEC controller – with no cloud connectivity – has learned the normal operative state, regardless of the clamping unit type, and is now able to offer an early indication of anomalies which will lead to clamping unit failure.
In another application, for a household electrical appliance company, Resolto was approached to find a solution to poor welds of washing machine drums. “We needed to look at data from the SAP system in addition to the plant-level data,” said Maaß. “This identified a problem linked to where the steel came from. The solution sees SCRAITEC cross-reference the origin of steel in a drum and automatically make the necessary heat and timing adjustments to the welding process for each drum.”
In conclusion, Maaß pointed out that it often comes as a surprise to many that AI need not be a resource intensive application. She said: “Using a trained algorithm will have a very small footprint and requires minimal hardware for interpretation. If you have a device running close to a machine and it is well trained and has local feedback, there is probably no need to ever connect it to a central system.” !