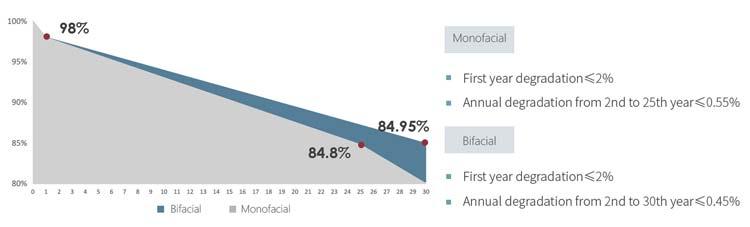
9 minute read
A design heads above the rest
Table 1. IEC standard algorithm vs inverter manufacturer recommended algorithm (calculated when minimum temperature is set to zero)
Tiger Pro 530 W Factory 2 500 W Factory 3 500 W
Open circuit voltage (V) 49.5 51 51.5
Number of components per string (based on IEC standards)
Number of components per string (calculated according to the inverter manufacturer) 28 27 27
29 28 27
Table 2. BOS cost comparison (assuming that the model and cost of the inverters are the same, the first year of the project generates the same amount of electricity)
120 MW Vietnam project Tiger Pro 530 W G12 500 W M6 445 W
Configuration information
First year (MWh) 200 200 200 200 200 200
Number of modules per string 30 29 30
Number of inverters 518 518 518
System voltage (V) 1500 1500 1500
Total number of modules
Total number of strings 226 416 240 000 266 667
7548 8276 8889
BOS cost
BOS cost (%) 0 +1.85% +2.31%
Table 3. Tracker cost comparisons between two modules provided by the mounting system manufacturer (moderate and high wind pressures)
Module length (mm) Number of modules per string
Recommended mounting system layout under moderate high wind pressure Total power Cost per watt Power level
2230 28
2267 28 2 × 28 (outside) and 2 × 42 (inside) 2 × 28 (outside) and 2 × 42 (inside) 44 520 0.03911 530
44 520 0.04038 530
Table 4. Simulation results of both PV plants in Vietnam with different inverters
Component model
Number of components 26 A maximum input current inverter 30 A maximum input current inverter
Tiger Pro bifacial 530 W Tiger Pro bifacial 530 W
16 200 16 200
Number of inverters
32 32
Hours of annual power generation
1650 1694 corresponding wind and snow loads, which indirectly reduces the cost of the fasteners for the mounting system.
According to the model provided by the mounting system manufacturer, the cost of a single watt for the mounting system is inversely proportional to the size of power in low wind pressure areas, thus the higher the power of modules, the lower the cost of a single watt for the mounting system. However, in high wind pressure areas, the cost of a single watt for the mounting system is positively related to the length of modules. Comparing Tiger Pro with modules from other manufacturers at the same power – according to the data provided by the tracker mounting system manufacturer – it is evident that although the component panels have the same power, the cost per watt of the mounting system also varies.
High compatibility between bifacial modules and inverters
Following multiple experiments, it is known that the shortcircuit current and working current of bifacial modules are much higher than those of mono-facial modules. At present, the maximum input current of maximum power point tracking (MPPT) of mainstream string inverters is 26 A, but when the back gain is more than 30% and the solar irradiance is very good (>1000W/m 2 ), the output current of the modules may be more than 13 A. If one MPPT of the inverter connects two groups of strings, the output of the modules will be lost. To bring higher power generation and lower power cost to clients, JinkoSolar has been in close communication with several mainstream inverter manufacturers to promote the launch of high current inverter products in response to JinkoSolar’s bifacial modules with high performance.
Higher production processes and product quality
From the testing in Vietnam, the company received the results seen in Table 4, from which it is clear that the inverters with high input current will be better optimised on the power generation of Tiger Pro products.
JinkoSolar’s modules have been rated as highly bankable PV module products in the industry, due to the high standard production process. In today’s 1500 V system era, high-quality module products have lower mismatch rates between groups and modules in long strings, which makes it easier for inverters to track to the maximum power point, especially in power stations that have been running for many years.
In summary, JinkoSolar’s Tiger Pro has demonstrated advantages in both cost reduction and compatibility on the system side, and this series of products may become one of the mainstream module selections in the new industry.
Technology components impacting LCOE
Along with the subsidy-free grid parity policy’s release of the National Development and Reform Commission and National Energy Administration in China, PV module power is upgrading unremittingly to meet the demand of the era
of subsidy-free grid parity. With rapid technology innovation, the diversity of technology type and the power class of PV module is increasing gradually among manufacturers over time. On 15 May 2020, JinkoSolar launched its latest PV module with 580 W maximum power and 21.21% efficiency. It indicates, to some extent, a rise of competition in the production capacity and performance of PV modules. Most mainstream manufacturers launch their high-efficiency modules one after another. Under this circumstance, more and more investors and EPCs are paying great attention to high-efficiency modules, especially after the release of JinkoSolar’s latest product. Therefore, the topic about how high-efficient modules achieve a lower LCOE in a PV system will spark a heated discussion on a wide-range power Figure 1. JinkoSolar’s new Tiger Pro module series. escalation.
From the perspective of a third party, TÜV NORD made a series of analyses and comparisons between the PV module Table 5. Basic information of the site produced by JinkoSolar and two other mainstream products Project site Golmud, Qinghai on the market. In the report, on the assumed condition of a 120 MW DC-side project, the economic performance of Project capacity 120 MW JinkoSolar’s 530W/535 W PV module is compared with others, GPS 94.55˚E 36.26˚N considering the aspects of a technical proposal review and financial income analysis. Regardless of the first-year power Annual irradiation hours 2195 generation gain, final revenue will be evaluated to meet the Altitude 2800 m demand of investors and EPCs. Annual average temperature 6.69˚C
To fairly and objectively compare the performance of the three modules applied to the project, TÜV NORD selected and analysed the same project site. The purpose of this was to limit the uncertain factors from the results, under the conditions of the same geographical location, type of power plant, meteorological conditions, on-grid electricity price, and tax policy.
For the convenience of comparison, Golmud in Qinghai, China was chosen as the project site. The annual irradiation of this area reaches up to 2195 hours, which belongs to the first-class light area in China. The annual temperature is low, with an average of 6.69˚C, Figure 2. JinkoSolar power degradation warranty profile. and the ground of the PV area is relatively flat.
Combined with TÜV NORD’s analogy analysis of existing projects on the market, the initial investment Table 6. Comparative LCOE case study analysis costs of the three module solutions can be estimated Case 1 Case 2 Case 3 and deduced, including the costs of pre-project Module model Jinko-530/535 XX-500 XX-450 development, EPC, and grid connection. Next, by Module power (W) 530/535 500 450 inputting the Jinko 530/535 W module’s pan-file into the Module efficiency 21.16% / 21.21% 20.90% 20.70% PV Syst software, the first year’s power generation can First year generation (MWh) 264 114 264 114 264 114 be calculated through simulation. At the same time, Modules number per string 26 25 26 to eliminate the loss of the curtailment and achieve a Inverters number 35 35 35 uniform power generation, the DC/AC ratio is uniformly DC/AC ratio 1.10 1.10 1.10 set as 1.10. In addition, it is also assumed that the three Inverter power (kWac) 3125 3125 3125 cases have the same degradation and O&M cost. In Modules number 227 006 240 625 267 362 conclusion, the results are as follows (Table 6): under the Strings number 8731 9625 10 284 established unified DC capacity, land availability and EPC per watt 4.0073 4.0710 4.1208 module price, case one of the Jinko 530/535 W module First year OM cost (¥/kW/y) 60.70 60.70 60.70 has greater advantages over cases two and three, such as LCOE and IRR. In light of the results from this Total investment (¥) 496 560 340 504 224 143 510 217 017 evaluation, the Jinko 530/535W module has presented Equity capital (¥) 148 968 102 151 267 243 153 065 105 market competitive advantages from technical and LCOE (¥/kWh) 0.2989 0.3028 0.3058 financial perspectives. Equity capital IRR 15.85% 15.39% 15.04%
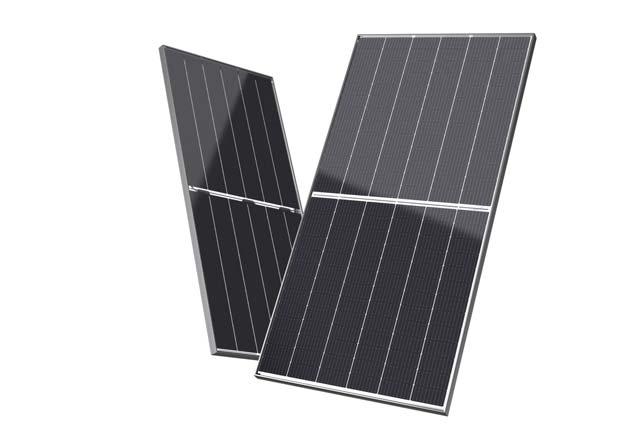
Figure 1. A 22 MW energy storage project in Cremzow, Germany, utilises Wärtsilä’s GEMS to meet the operational requirements of the power plant and to provide frequency regulation services to the German primary control reserve market.
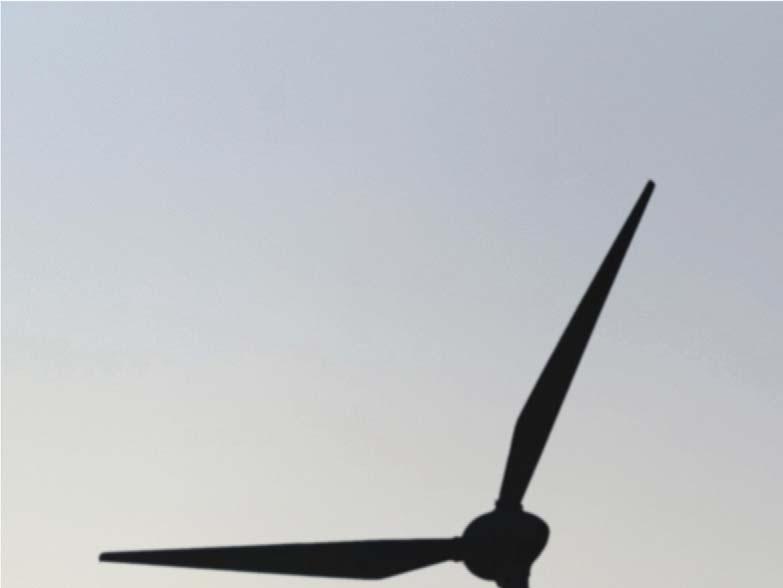
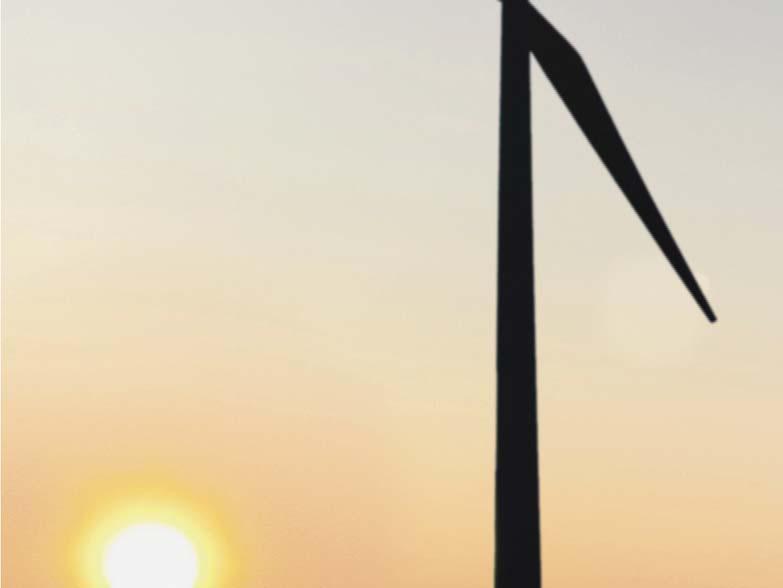
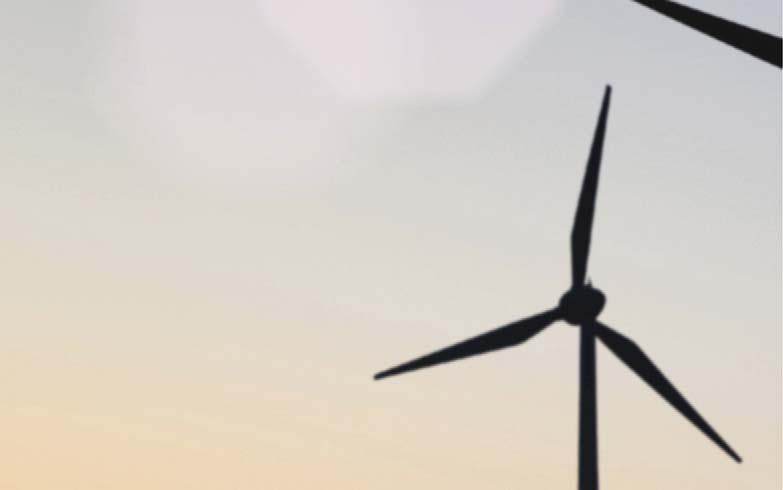
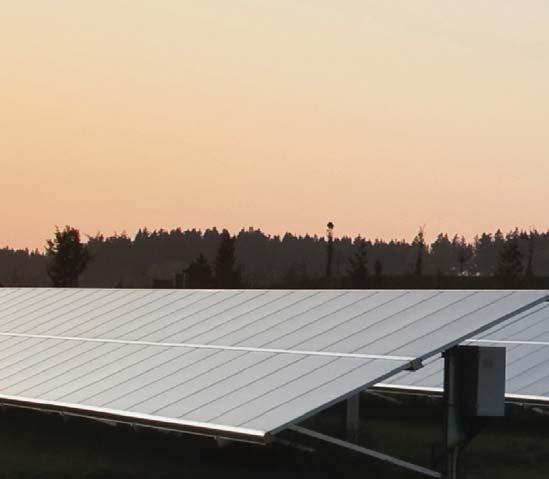
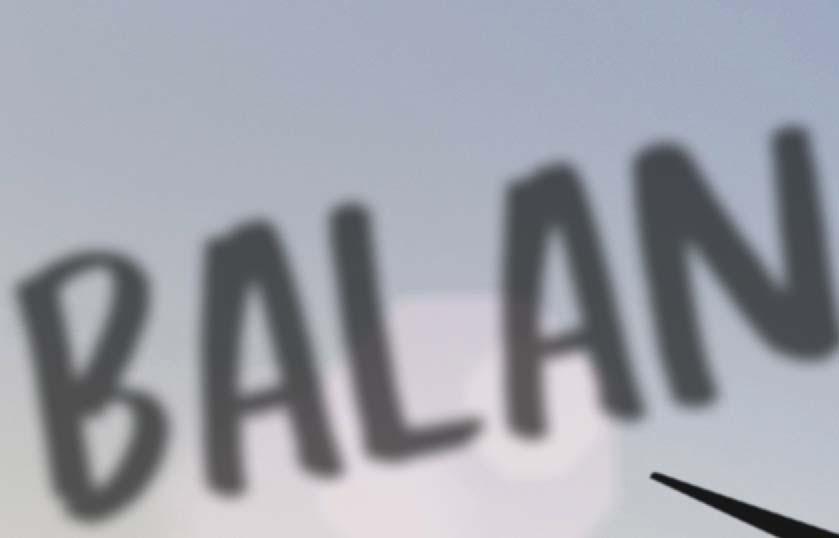
Energy storage, which helps manage renewables’ complexity, is itself becoming more complex as systems are able to serve different parties and incentive offerings. For large-scale solar and wind asset owners, utility-scale battery storage can offer heightened returns for these projects, yet for grid operators, battery storage offers much-needed grid stability. However, these value propositions can sometimes come into conflict, for example, when it is more financially valuable for the end customer to hold onto stored energy than supply it to a local grid in demand. Machine learning-enabled optimisation can help a battery system navigate the different energy storage value propositions and
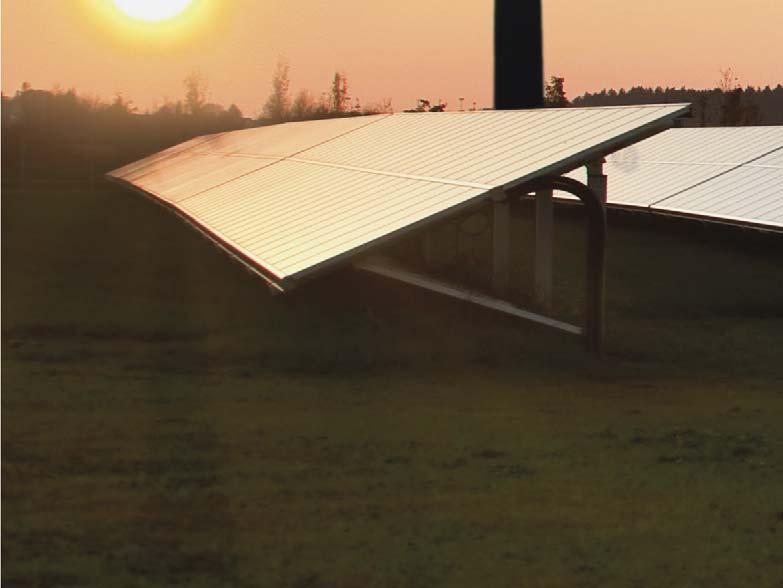
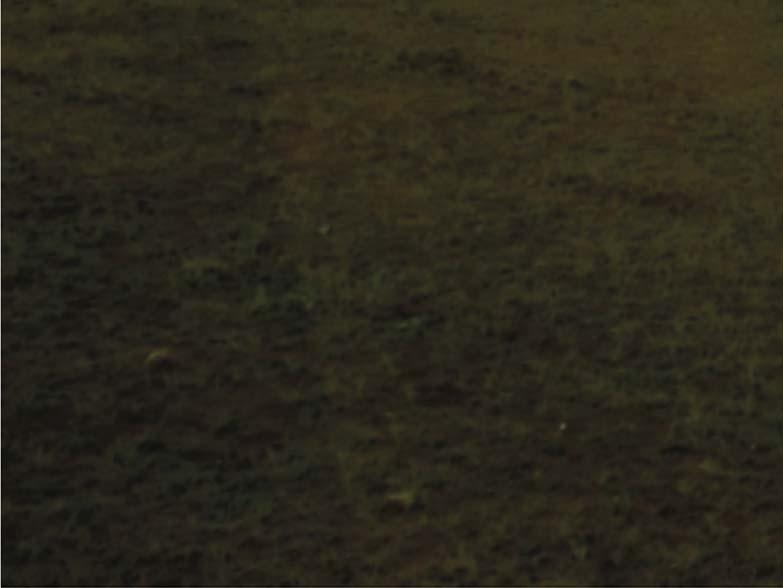
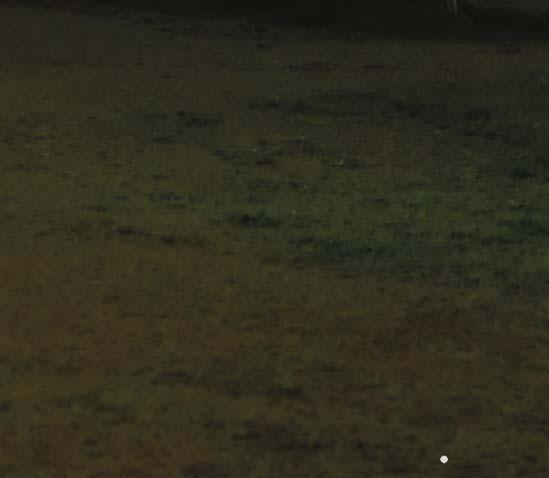
improve energy output for more automated grid management.
By applying machine learning, energy storage projects can rely on computer algorithms that establish contextual overrides built on years of experience, human guidance, and forecasted simulations. Machine learning then evaluates how the contextual override performed to improve the algorithm. Machine learning-enabled storage solutions carry a level of intelligence and capability to autonomously operate in ways that humans could not do alone. Energy storage software platforms use artificial intelligence and automated decision-making based on real-time and forecasted data from various
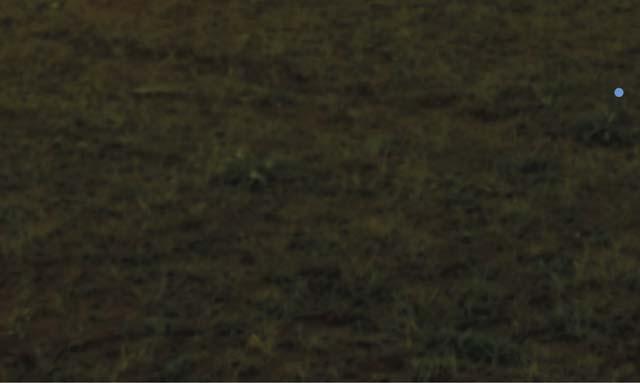
Sen Zhang, Wärtsilä Energy Storage and Optimisation, USA, discusses how the application of machine learning-enabled optimisation to energy storage projects can help batteries balance complicated and fast-changing priorities.
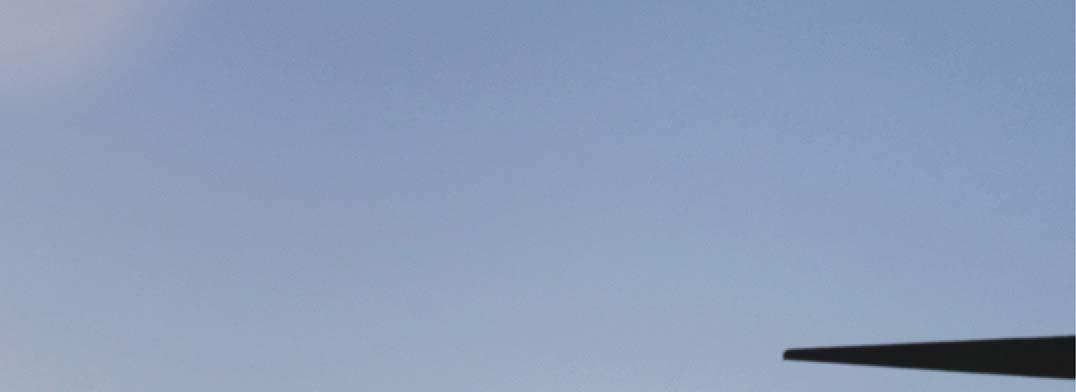
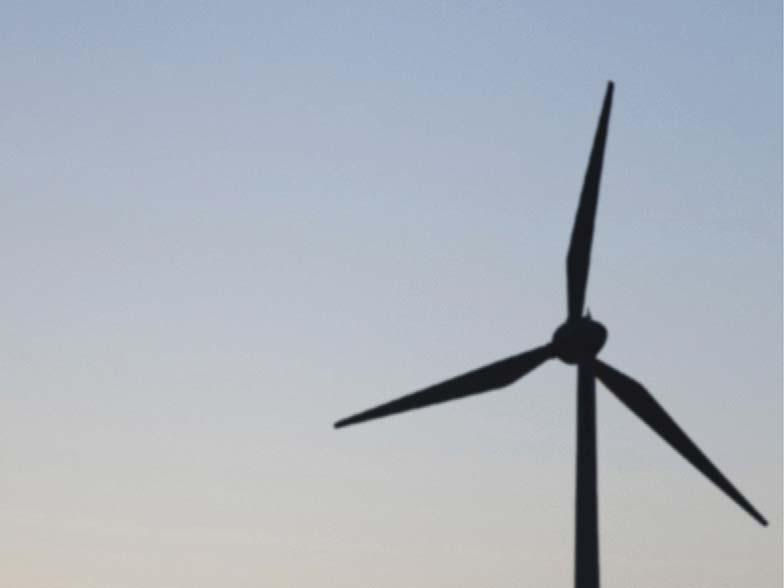
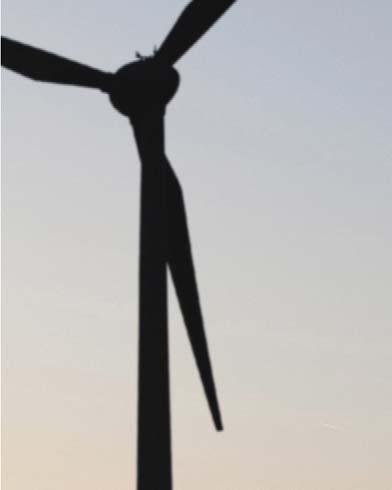
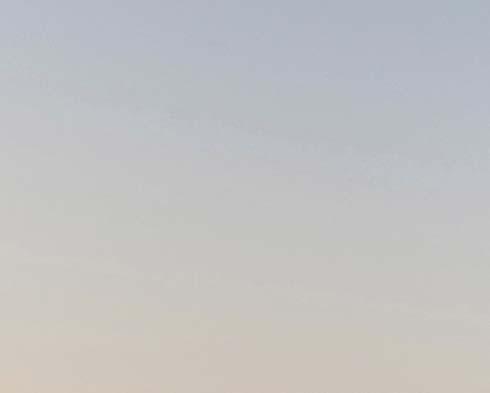
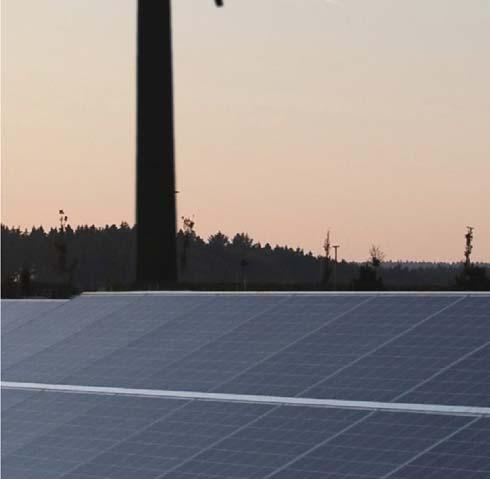
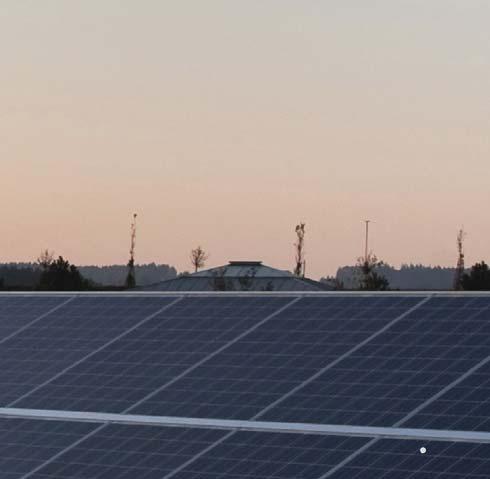
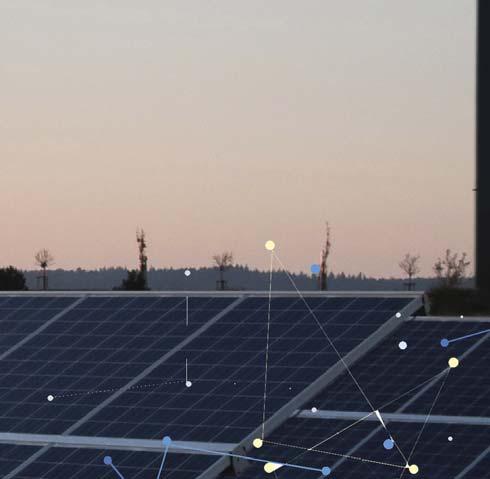
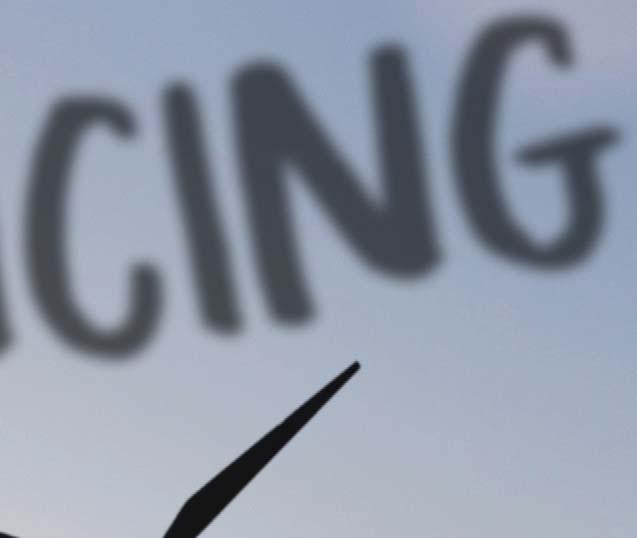
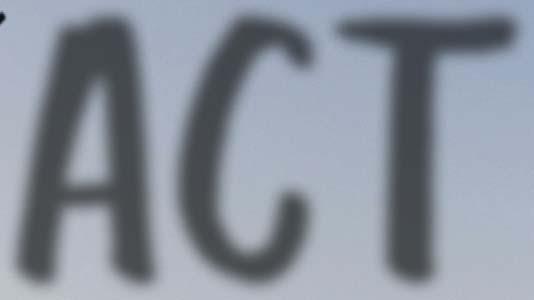
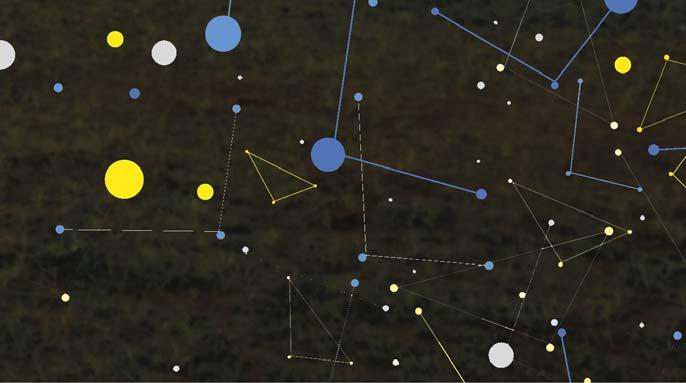
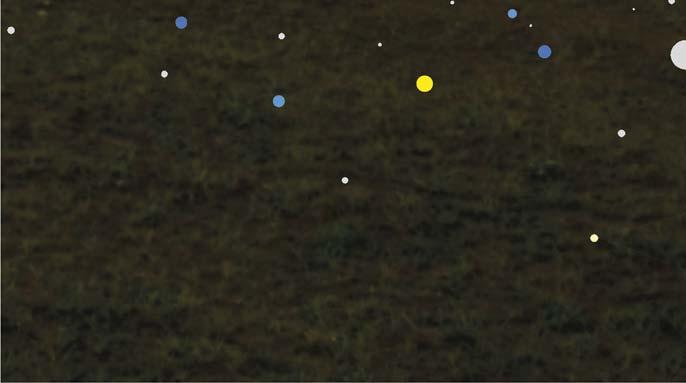
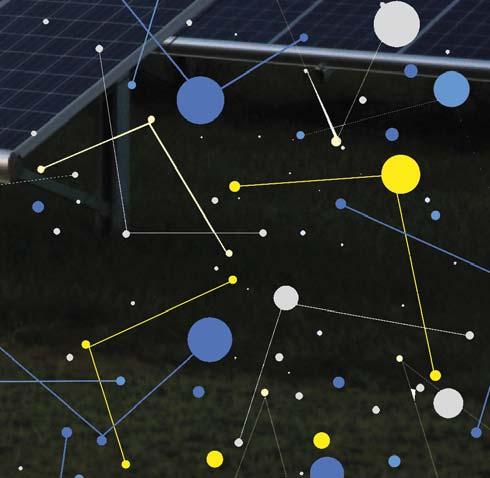
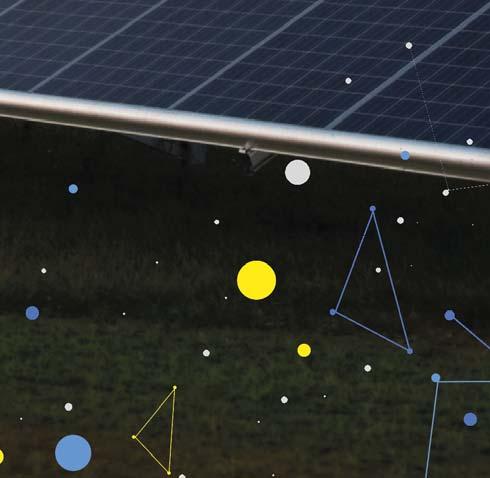
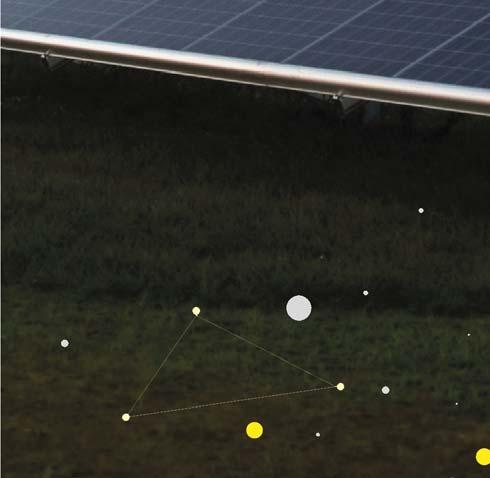