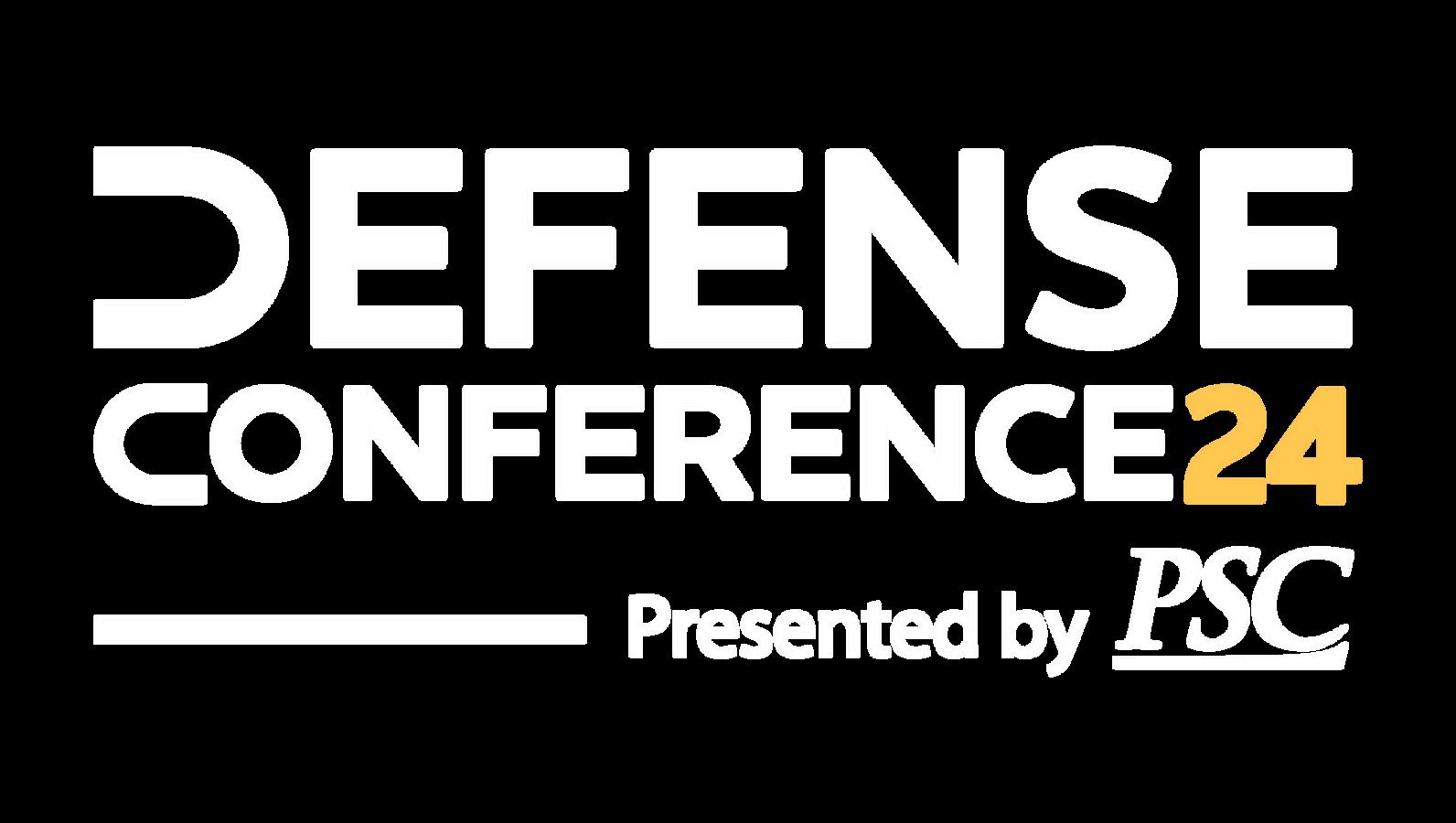
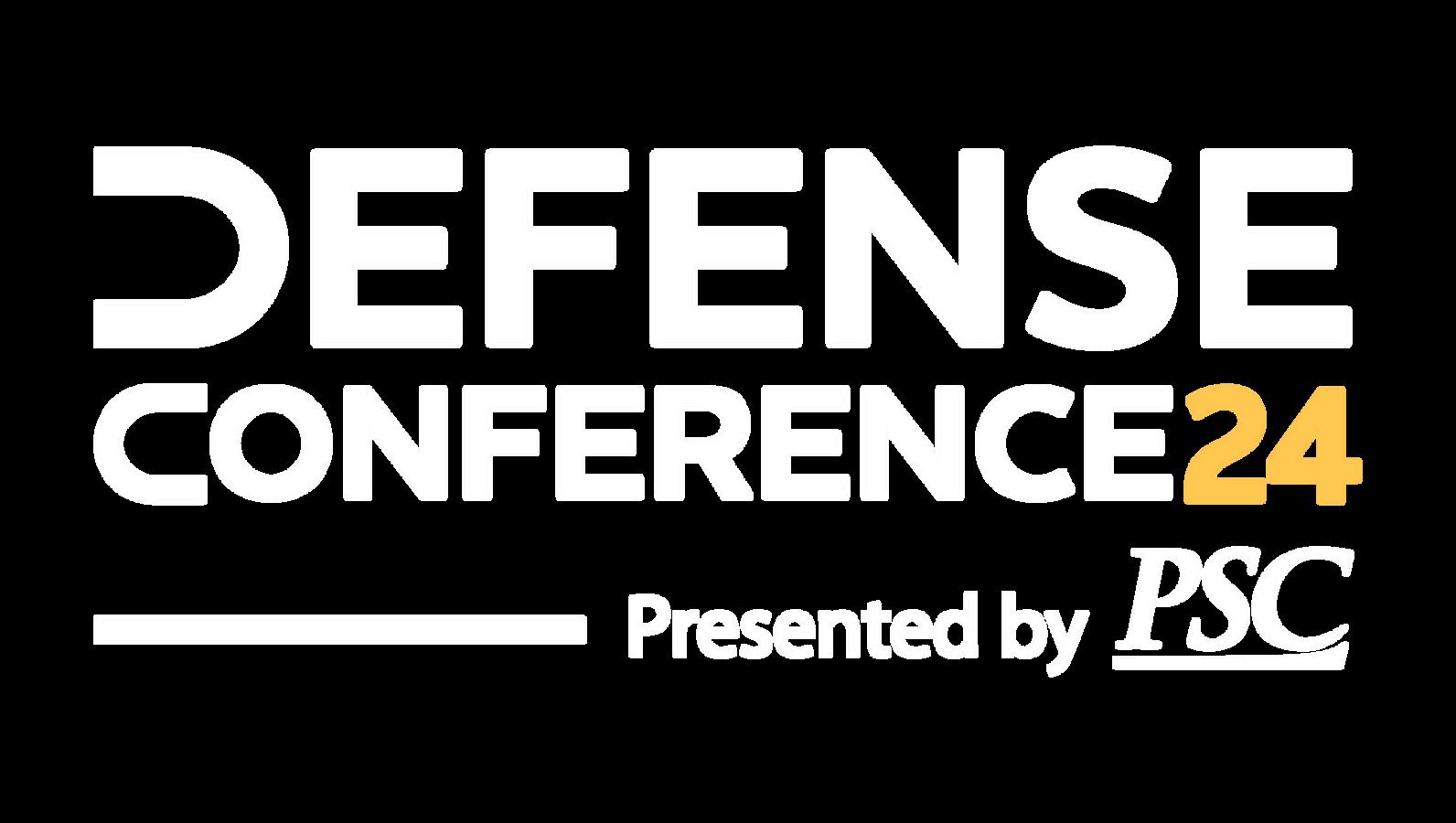
THOUGHT LEADERSHIP COMPENDIUM
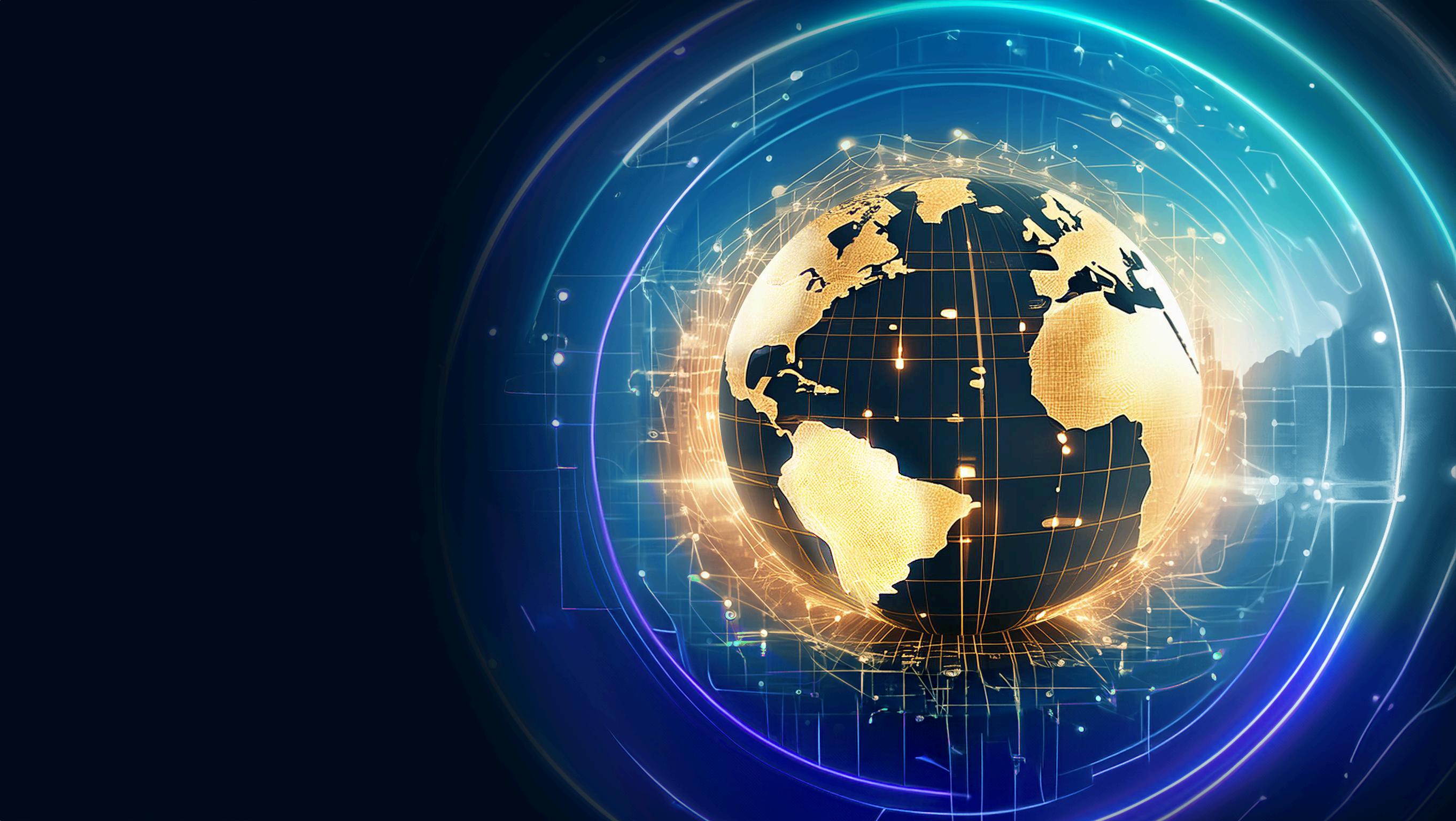
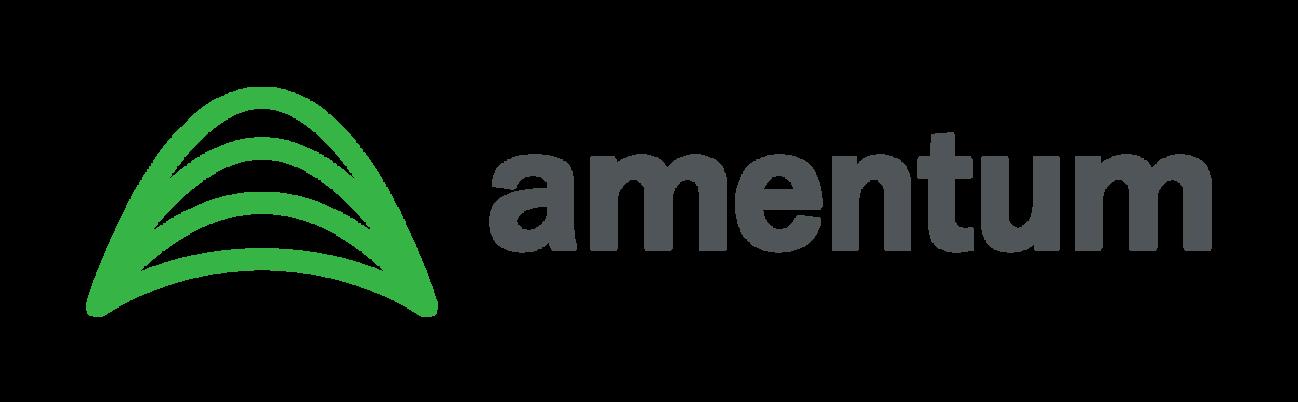
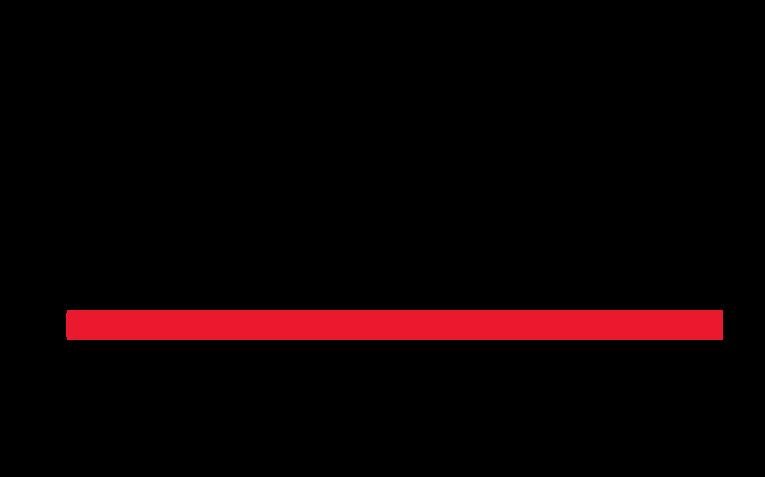
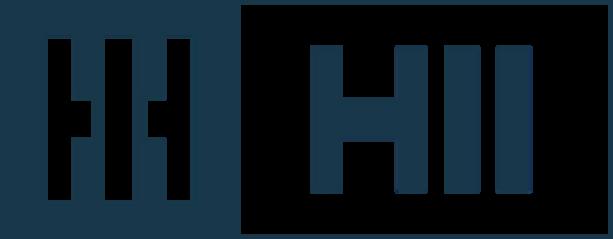
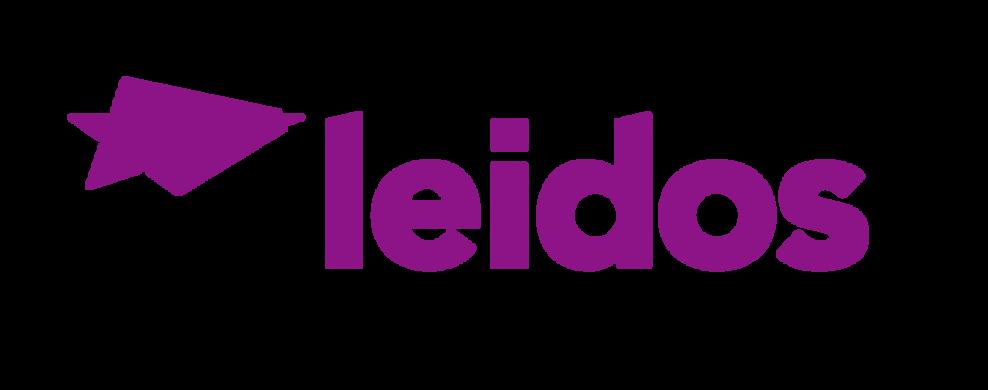
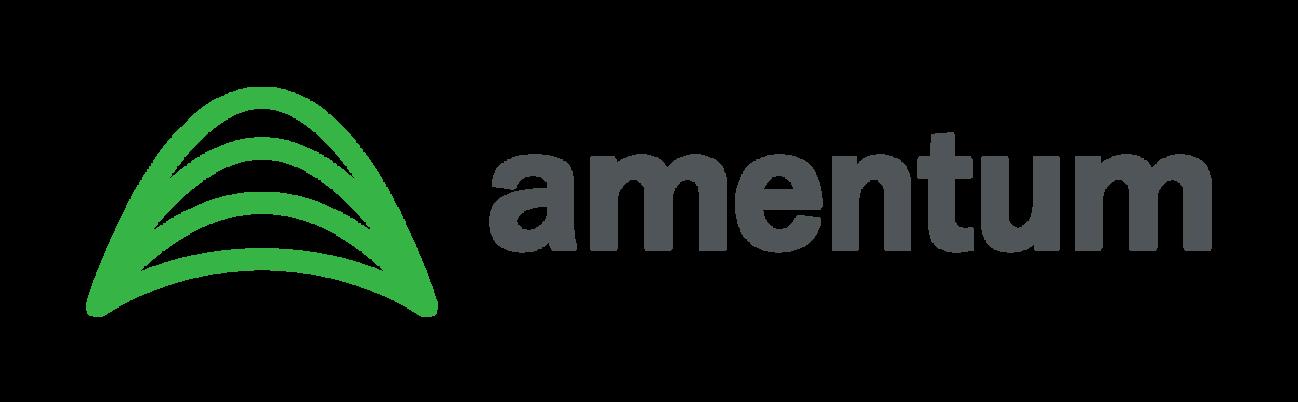
Amentum
Demystifying Artificial Intelligence
Understanding Its True Nature and Scope
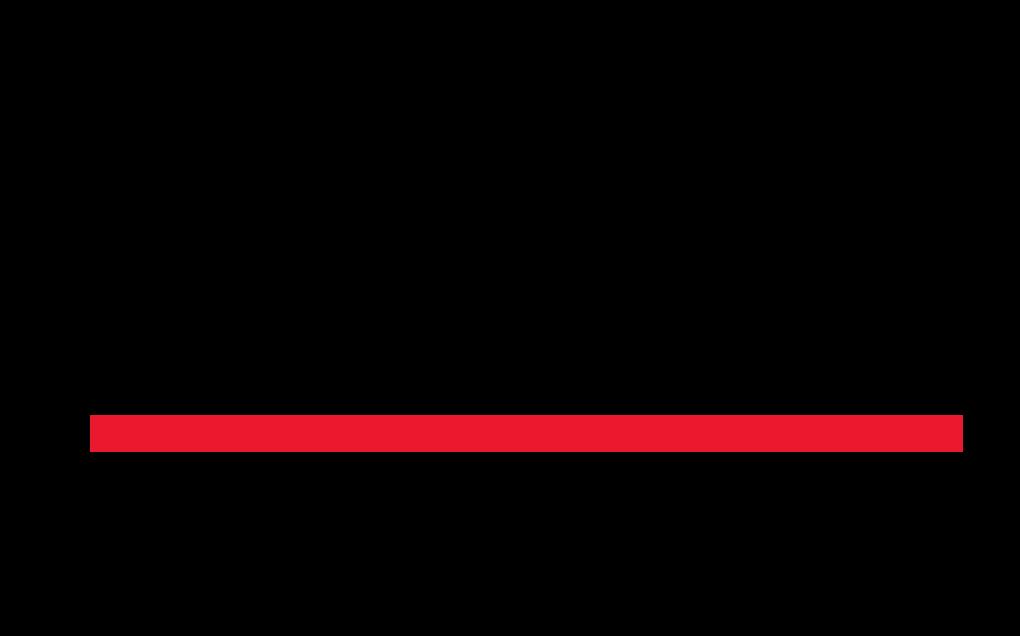
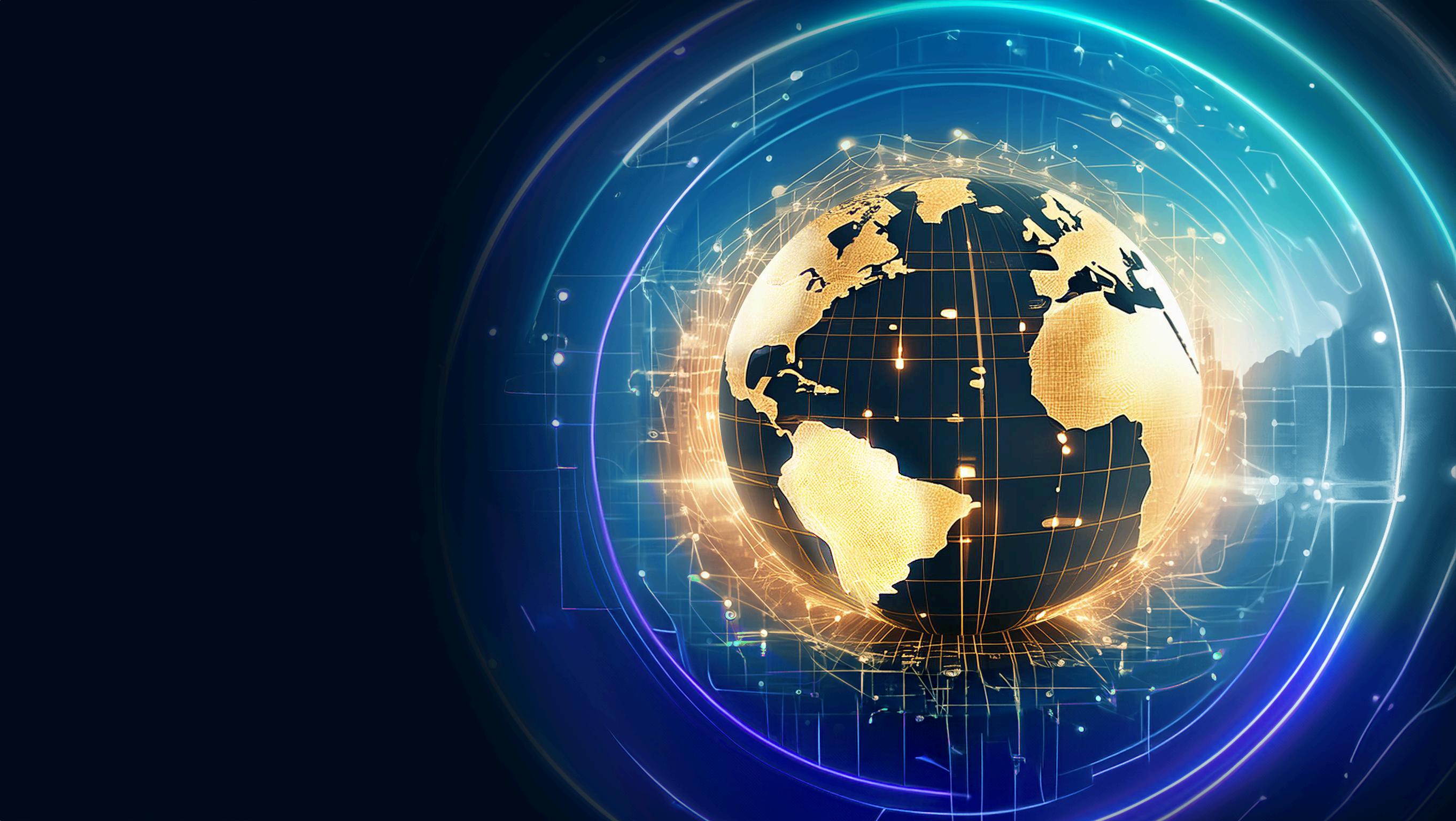
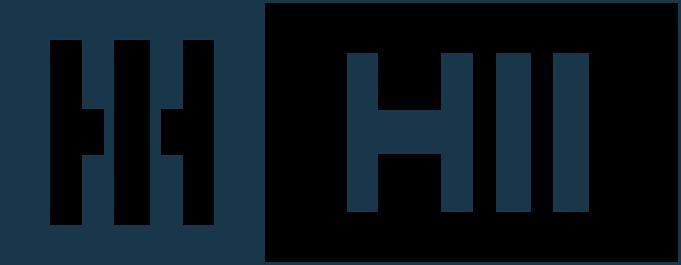
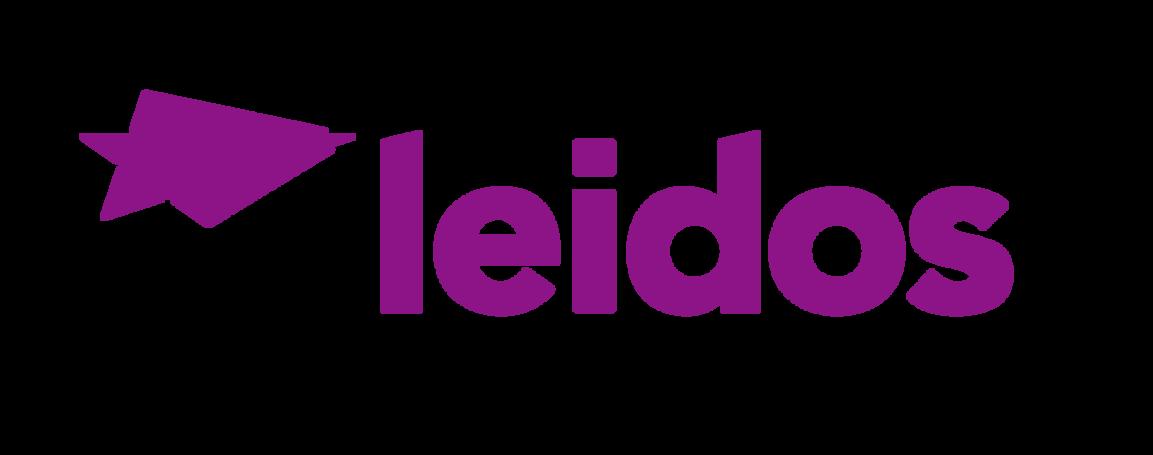

Demystifying Artificial Intelligence
Understanding Its True Nature and Scope
Introduction
As we advance further into the digital age, the realm of Artificial Intelligence (AI) has transitioned from being a mere element of science fiction to a vital part of our everyday life, sparking both fascination and trepidation. This article is part of a comprehensive series designed to illuminate the multifaceted world of AI, cutting through the haze of myths and misconceptions, and offering a clear, precise comprehension of its true essence. Our objective is to present an accurate depiction of AI's capabilities and limitations, to provide insights into the real-world implications of key AI topics, including Deepfakes, Large Language Models, Autonomy and Robotics, and the future of AI. Each article in the series will focus on a specific aspect of AI, offering guidance and principles for its effective and responsible implementation in the modern world.
Transformative Potential of AI
AI's transformative power stems from its unparalleled ability to process and analyze vast amounts of data, far exceeding human capabilities. This rapidly advancing technology is not only creating new opportunities but also giving birth to new industries. Globally, organizations are recognizing AI’s profound potential beyond just enhancing services and streamlining operations. It's reshaping decision-making processes, especially in government sectors, where AI is crucial for efficient public service and robust security. For instance, its predictive analytics capabilities are employed to foresee social events, manage traffic efficiently, and reinforce public safety measures. This expanding role of AI underscores its significance as a key driver of innovation and societal advancement.
As AI continues to evolve, its scope is widening, finding applications across diverse sectors such as healthcare, entertainment, finance, and transportation. Our growing reliance on AI to automate routine tasks, analyze extensive datasets, and rapidly execute complex computations is a testament to its potential to transform industries and everyday life. AI's ability to uncover previously unseen patterns in data makes it a powerful tool for innovation and efficiency, paving the way for groundbreaking advancements in various fields.
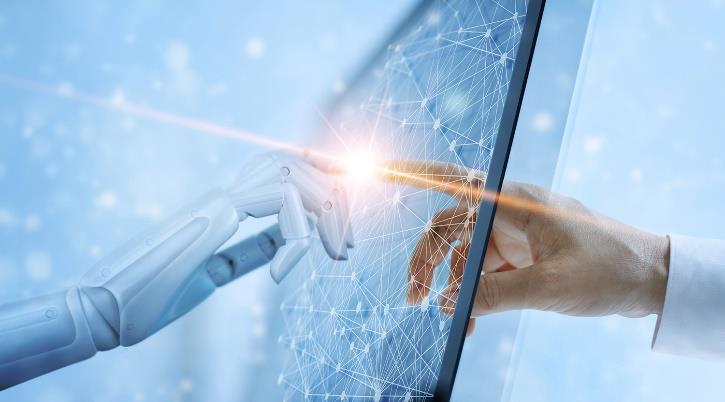
Emphasizing AI's national significance, the White House's executive order [1] to expedite AI development and deployment highlights its strategic importance in maintaining global competitiveness, security, and technological leadership. This directive is not merely about technological advancement but underscores the need for a responsible vision for implementing AI; maintaining a security posture that protects sensitive data while being agile enough to allow us to collectively innovate. Additionally, we need a design framework that ensures AI development is aligned with the public interest and democratic values, recognizing AI's potential to influence societal structures and norms.
Key Milestones in AI Evolution
The journey of AI from its beginnings to its current state as a transformative technology illustrates a field that is constantly evolving and expanding its boundaries. The history of AI is not just a technical narrative but also a reflection of our changing understanding of intelligence and cognition. As AI continues to advance, it promises to redefine what is possible in technology, while also posing significant challenges and ethical considerations that
must be addressed. The development of AI has been marked by several key milestones, each signifying a leap forward in theory, technology, and application.
The Inception and Theoretical Foundations (1940s – 1950s)
The journey of AI began in the 1940s with the 'Turing Test' by Alan Turing, a criterion for assessing intelligence in machines. This period also saw the development of the first computers and the theoretical underpinnings of AI, including the creation of artificial neurons by McCulloch and Pitts in 1943. The Dartmouth Conference in 1956, where the term "artificial intelligence" was coined, marks the formal birth of the field.
Logic-Based AI (1950s – 1960s)
This era witnessed significant progress in AI, with researchers developing early AI programs like Samuel's checkers program and Newell and Simon's Logic Theorist. There was a growing focus on using logic and rulebased systems to simulate human problem-solving and decision-making processes.
Expert Systems (1970s – 1980s)
A major breakthrough in AI was the development of expert systems, which are algorithms that emulate the decision-making ability of a human expert. These systems were particularly successful in fields such as medical diagnosis, geology, and chemistry. The success of expert systems led to a surge in AI research and applications, making AI a commercially viable field.
Probabilistic Reasoning (1980s – 1990s)
One limitation of purely logic-based AI is that it cannot accurately describe uncertainty in the world. This limitation led to the integration of probabilistic methods, which can capture event uncertainty in a predictable fashion. This shift was crucial in dealing with uncertainty in real-world environments, leading to more robust AI applications. The development of algorithms like the Bayesian networks significantly advanced this field.
Machine Learning and Neural Networks (2000s – Present)
In the 2000s, the fusion of increased computational power and large datasets reinvigorated interest in neural networks, particularly in machine learning. This era witnessed significant strides in computer vision and natural language processing. AlexNet, a deep neural network that emerged at this time, significantly advanced image classification. Additionally, the development of AlphaGo, renowned for defeating world champion Go player Lee Sedol, showcased AI's advanced strategic capabilities. These advancements highlight machine learning's impact on complex problem-solving and real-world applications like autonomous vehicles.
Current State of AI Technology
AI's evolution mirrors two important concepts: Amara's Law and the Gartner Hype Cycle. Amara's Law posits that we tend to overestimate technology's impact in the short term but underestimate its effect in the long run. The Gartner Hype Cycle visualizes the stages of hype surrounding new technologies:
• Innovation Trigger: A breakthrough sparks interest and early hype.
• Peak of Inflated Expectations: Excitement reaches a fever pitch with potentially unrealistic expectations.
• Trough of Disillusionment: The technology fails to live up to the hype, and interest wanes.
• Slope of Enlightenment: Practical applications emerge, and the technology's potential becomes clearer.
• Plateau of Productivity: Mainstream adoption as benefits solidify.
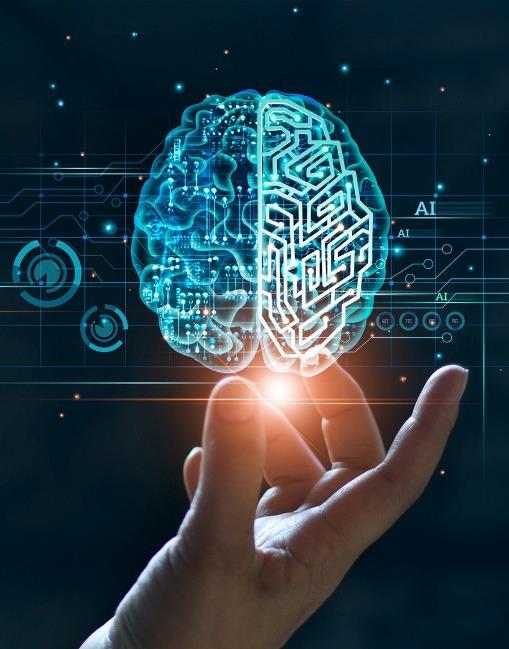
Let's examine how these concepts apply to the current AI landscape:
1. Deep Learning Dominance
Deep learning has moved beyond the hype stage and into productive use across various industries. Its power in pattern recognition and decision-making is undeniable.
2. Generative AI and Large Language Models (LLMs)
LLMs have captured public imagination with their conversational abilities. The recent Sparks paper highlights impressive capabilities but also key limitations, and a follow up paper emphasizing that we're still far from true Artificial General Intelligence (AGI), by making a LLM forget its knowledge about Harry Potter. As pointed out, LLMs are at the peak of inflated expectations. AI's ability to create new content is rapidly advancing. While sparking excitement and innovation, issues of intellectual property, disinformation, and ethical use abound, and are likely to have mild but notable impact on its adoption.
3. AI Democratization
The growing accessibility of AI tools lowers the barrier to entry. However, understanding the technology's principles remains crucial for responsible and effective use. The notion of AI Democratization is entering the slope of enlightenment phase of the hype cycle.
4. Focus on Explainability
Efforts to make AI decisions more transparent are moving from research to practice. Explainable AI is essential for building trust, especially in high-stakes domains. Explainable AI may still be 2 – 5 years away and is transiting through the trough of disillusionment.
5. AI in Robotics
Robotics is steadily progressing towards more flexible and capable robots. AI-powered perception and control systems are enhancing adaptability and dexterity. Drones are becoming essential at the squadron level. While the technology still has 5 – 10 years to go, the promise of AI in robotics is entering the slope of enlightenment.
Conclusion
Although there are possibilities for profound long-term transformation across many different sectors, it's essential to navigate these times with a critical eye, discerning between transformative potential and unrealistic expectations. It is necessary to maintain a sensible approach, getting started with use cases that can take advantage of structured environments capable of processing large amounts of complex data in ways that humans cannot. When adopting AI to handle these use cases, it is important that we don’t lose the nuances of the old process. AI works best today by working alongside of humans, not instead of them. In this way we can enable democratization of use cases, while maintaining low risk to organizations by not over automating to the point that we lose control of the process. Amentum remains excited to keep taking steps to harness to power of AI as we strive to make a smarter, cleaner and safer world.
Contact Us
Seth Eaton VP of Technology seth.eaton@amentum.com
Dr. Sam Nazari
DARPA Research and Development, ES&T sam.nazari@amentum.com
Dr. Kim Wilcher
Mechanical Engineer, ES&T kimberly.wilcher@amentum.com
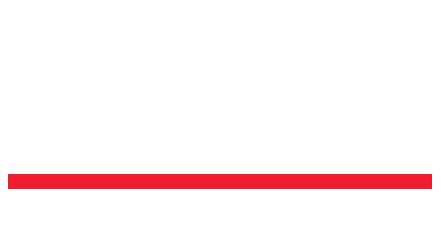
How SOF Sharpens its Edge in the Digital Technology Era
EMBRACING RISK IN THE FACE OF MILITARY DISRUPTION
By Lt. Gen. Michael K. Nagata, U.S. Army (Ret.) Strategic Advisor
CACI
Senior Vice President
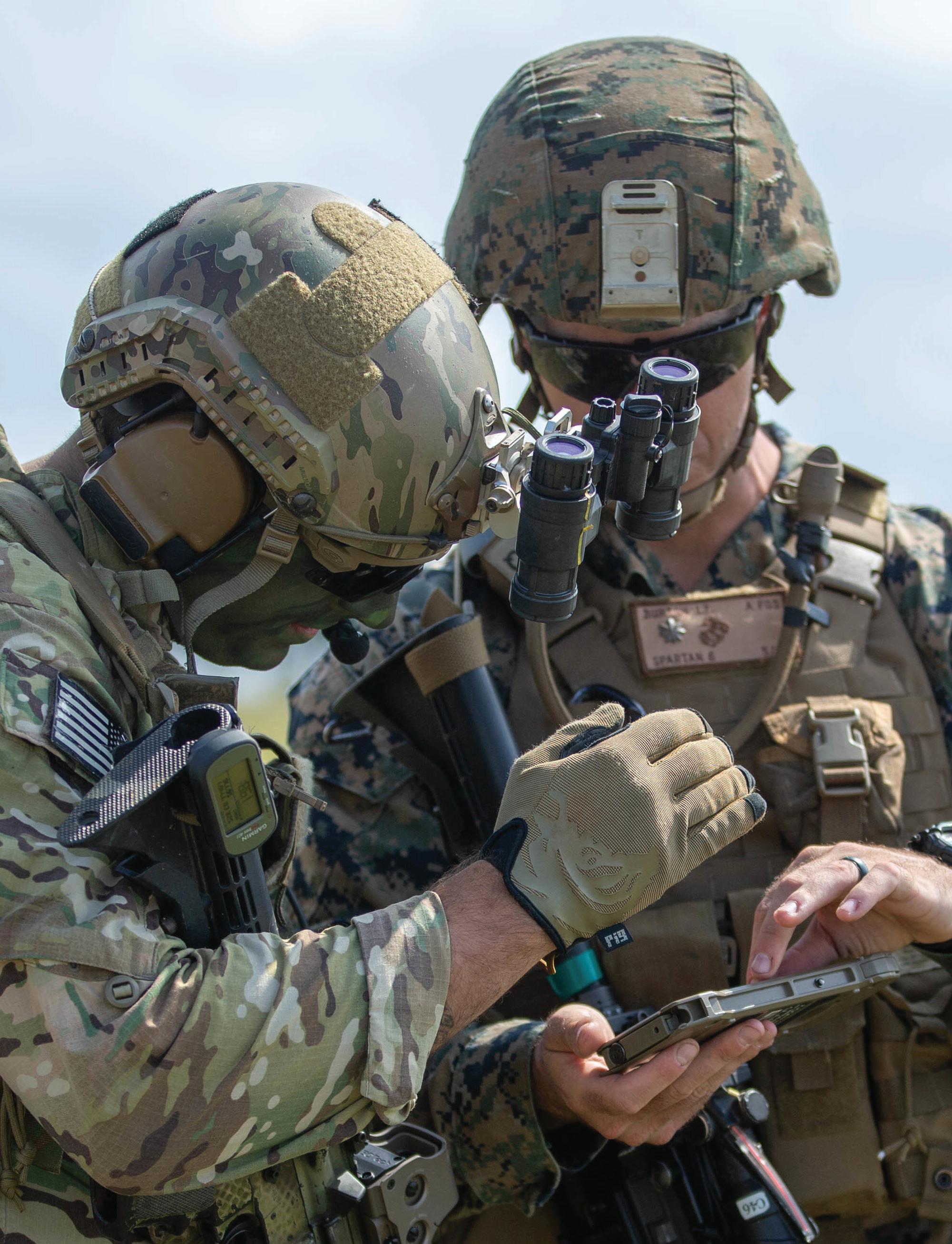
During the period roughly between the 10th and 12th Century, Chinese-invented explosive powders, intended originally for entertainment purposes such as fireworks, were moving into Europe. But as this new “technology” migrated west, people saw its potential as a weapon of war, particularly with the discovery of its ability to propel projectiles. The emergence of explosives and gunpowder began a rapid race of discovery and invention, leading to firearms, cannons, missiles, and beyond—ultimately changing the face of mankind. All the nations, kingdoms, and empires that embraced the power of explosives survived. Those that did not no longer exist.
Today, we are going through another “gunpowder moment” but today’s game-changing element is not chemical; it is the rapid proliferation of digital technology. Whether we realize it or not, the US government broadly, and US national security agencies such as DoD specifically, must ask themselves whether we will be the most eager, the most risk-tolerant, and the most flexible in embracing the quickly increasing power of digital technology—and therefore survive and thrive—or will we disappear like the ancient kingdoms and empires that were too slow to adapt?
Today’s analog of this ancient history has been the constantly accelerating proliferation of powerful and disruptive digital technologies which, for the past several decades have profoundly altered modern warfare already. Examples include:
• Terror and nation-state proxy groups now employing sophisticated drone technology.
• Russian forces conducting prolific digital electronic warfare and information operations. China’s ongoing development and proliferation of space-based sensors and weaponry.
• The Islamic State, in all of its many forms across Asia, the Middle East, and Africa, and its ability to subvert, dissuade, and influence its adversaries, and recruit large volumes of new adherents, through numerous highly effective social media campaigns that no nation state has been able to match or strategically counter.
These examples all showcase how technology is fundamentally altering how every modern adversary will operate and fight.
Just as importantly, it is not these governments or groups that are developing these cutting edge, technology-based abilities. Instead, it is commercial industry that is creating the vast preponderance of these tools and applications that will increasingly dominate future battlefields.
Meanwhile, the U.S. military still largely operates within an elaborate and lengthy government procurement process designed to focus on traditional, multi-million-dollar platforms, and seek minimal risk in doing so. Some of these practices are decades old. This stands in stark contrast to the speed with which commercial industry rapidly develops
and fields new hardware, firmware, and most importantly, software. This contrast illuminates a still unresolved clash between today’s military need to rapidly adopt and employ 21st Century “technology age” capabilities, versus much slower government processes that were designed to procure, distribute, train for, troubleshoot, and employ “industrial age” capabilities.
Unfortunately, existing US government concepts, practices, and bureaucracies too often run counter to the speed at which digital technology evolves. Probably the most vivid contemporary example is our adversaries’ rapid development and fielding of large numbers of increasingly capable drones at increasingly affordable price points. Meanwhile, U.S. forces must sometimes shoot down a $50,000 drone with a million-dollar missile. This imbalance today is often unavoidable, yet also unsustainable and unwise.
Fortunately, there are places in government where the need to accelerate the adoption of alternative, hightechnology solutions can be nourished, accelerated, and thereby put modern, technology-based capabilities in the hands of the warfighter far faster. High on this list are US Special Operations Forces (USSOF). The US Special Operations Command (USSOCOM) has a long tradition of being an “early adopter” of new capabilities. Because USSOF has always had to be effective and survivable while operating in very small teams in volatile or dangerous environments, this community has always placed a cultural premium on the willingness to “try new things and new abilities” even if not yet completely vetted or tested. The philosophy in USSOF that I grew up with as a young Special Forces officer was often described with words like, “Just give me the “80%” new solution now... I will fill in the shortcomings by improvising, adapting, and overcoming whatever parts don’t work so well.” Several more modern examples come to mind.
USSOF were among the first to start employing the lightweight ballistic helmets now in wide use across both US military and US law enforcement communities. They were the first to pioneer the use of civilian “Tandem Skydiving” parachuting into a new form of military free-fall to bring both untrained personnel and military K-9 dogs into combat environments that were otherwise unreachable for either.
Accordingly, America’s SOF community has the potential to be a helpful beacon for many others in government to follow. While striving to never be cavalier about safety or avoiding unnecessary harm or risk, this spirit of early
adoption and rapid experimentation also drove the creation of a more flexible and adaptive acquisition philosophy and set of practices in USSOF that has served the needs of its operators and forces very well during the past two decades of unrelenting combat adaptation across at least three continents.
And yet, the SOF community will still face many of the same challenges that all government elements will face. High among these is the stark reality that, as military service members and government employees of all types become increasingly dependent on emerging technology, the boundary between which tasks are inherently governmental and which can only be done by industry will increasingly blur. The day is coming when Special Forces A-Teams, SEAL platoons, and Ranger Companies can only succeed if they have “robots” embedded in their formation. How will the US government ensure effective training and education for its formations and people, when simultaneously the hardware, software, and firmware of their most important capabilities are changing at increasing speeds, and in ways best understood by Industry experts?
Imagine a swimming robot that can do powerful, useful activities that advance the mission of a SEAL platoon. But every week, the graphic user interface changes and the robot receives several new, software-driven capabilities. Imagine that same platoon receiving a new box to mount on the back of the swimming robot that will dramatically expand its abilities. Who will train and educate the platoon to install, use, troubleshoot, and maintain these emerging technologies? As training and education become more and more focused on technology-based capabilities, changing at ever-increasing speeds, it is unlikely that government alone can keep up. This is where industry can and must play a vital role, creating substantial changes in how America’s national security practitioners of all types will train on and understand new technology-based capabilities.
More and more US military leaders are understanding that they cannot and must not rely on our past success or traditional practices to ensure our future national security. While traditional “gunpowder-based” types of capabilities will always remain important, it is the rapid and expanding emergence of new, powerful digital technologies that will determine the future balance-of-power around the world, and America’s place in it. For us, this challenge requires a clarion call that ironically might be best illustrated by something an American President in the 1800’s, long before the Internet, once said.
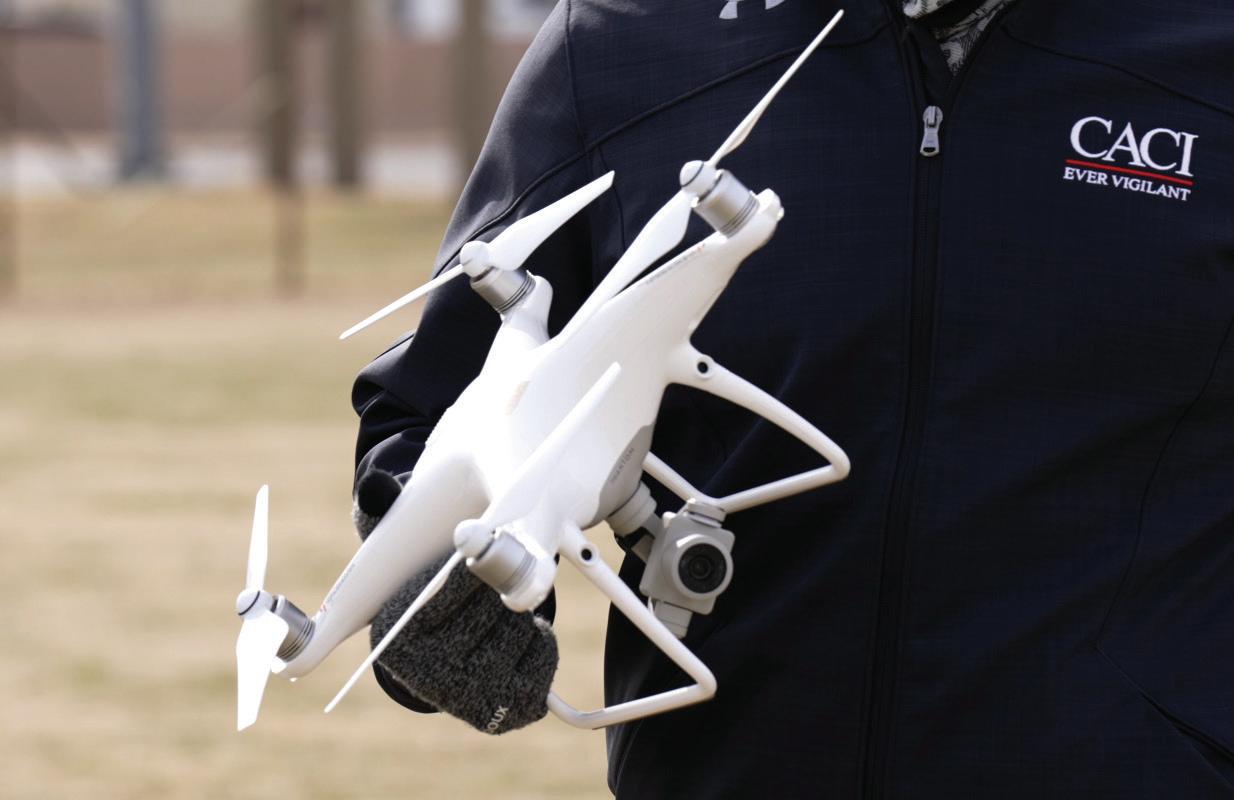
“The dogmas of the quiet past are inadequate to the stormy present. The occasion is piled high with difficulty, and we must rise with the occasion. As our case is new, we must think anew and act anew. We must disenthrall ourselves, and then we shall save our country.”
— ABRAHAM LINCOLN
ABOUT CACI
At CACI International Inc (NYSE: CACI), our 23,000 talented and dynamic employees are ever vigilant in delivering distinctive expertise and differentiated technology to meet our customers’ greatest challenges in national security and Government modernization. We are a company of good character, relentless innovation, and long-standing excellence. Our culture drives our success and earns us recognition as a Fortune World’s Most Admired Company. CACI is a member of the Fortune 1000 Largest Companies, the Russell 1000 Index, and the S&P MidCap 400 Index.
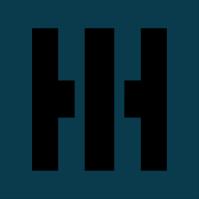
Digital Twin Trust
[2] R. Capozzi, A. Costa and I. Friedrichs, "Creating Virtual World Environments for Ocean Vehicles," in
1. V&V Project Overview
Digital twinsarea virtual representation of a physical asset, its environment, and its processes. They are increasingly employed by companies looking to decrease design, schedule, and cost risk by rapidly iterating through use cases of an asset in its virtual form. As with any engineering approximation, digital twins are not perfect substitutes for reality and there is a constant balancing of risk when using outputs from a digital twin to make real world decisions. To build trust, a validation and verification framework is proposed in [1] that can be applied to any digital twin. The goal of this document isto demonstratetheapplication of that framework to an existing digital twin used by Unmanned Systems (UxS), a business group within HII’s Mission Technologies division.
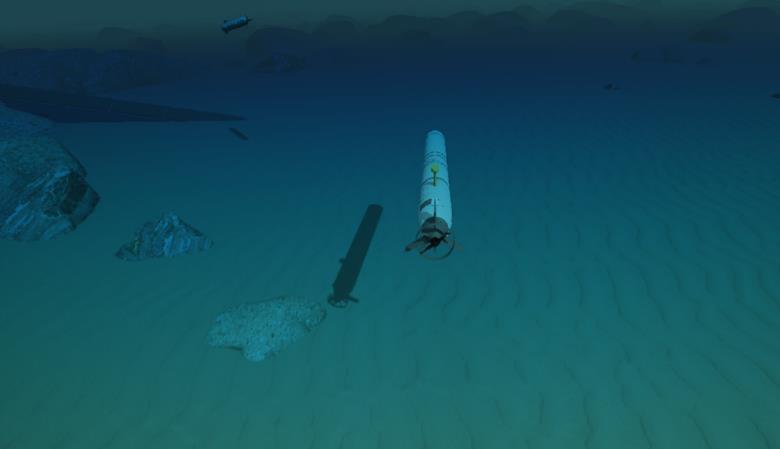
2. HII Unmanned Systems Digital Twin Overview
UxS designs, builds, and sustains unmanned underwater vehicles (UUVs). Due to the complexities of designing robotsto withstand harsh ocean environments, aUUV digital twin (DT) has been developed to aid in the decision-making process. The DT is a physics-based representation of a UUV, its processes, and its environment [2] The DT user can vary those representations to obtain useful output that would otherwise be prohibitively time consuming, expensive, or impossible to generate in the real world.
The UxS UUV DT iscomprised of four major components: (1) the virtual world, (2) the vehicle model, (3)sensor models, and (4) the hydrodynamic physics solver. The virtual world is populated with bathymetry maps, ocean turbulence, water density profiles, sea state effects and physical obstacles in an integrated framework built on Unreal Engine and supporting processing nodes. The vehicle model is represented by the hydrodynamic hull signature and actuators populated from a suite of high-fidelity computational fluid dynamics(CFD) simulations. This vehicle is outfitted with simulated sensor models developed as mathematical representations of the sensor functions with specifications applied based on vendor datasheets and real-world data. Thesimulated vehicle interacts with the virtual world through its sensors and hydrodynamic properties. The high-fidelity physics solver uses these interactions to solve a set of non-linear equations integrated in time for the dynamic vehicle state. This DT is developed in a very modular framework that allows models to be used when needed and combined to represent various vehicle configurations. Each model publishes its output information onto the data distribution service (DDS) busfor any other model to subscribeto. The following sections will detail some of the significant DT components and processes through the ontology presented in [1]
2.1. Model Library
The model library is a collection of model elements that can be combined to represent the digital twin. For the UxS UUV DT, models are essentially the discretization of the real world into its virtual, mathematical counterparts. Each model has a distinct purpose in the virtual world and when combined, form a complete representation of the UUV. Models include the physics solver, propeller, depth sensor, GPS, and visualization system.
Figure 1: Simulated small- and medium-class UUVs operating in HII's UUV Digital Twin over local bathymetry
The fidelity of each model varies, both in softwarecomplexity and digital twin classification level. Many of themodelsare classified as digital twin prototypesbecausethey do not exchange information with the corresponding real-world systems. Other models have modes to allow connections to real hardware and are an example of a complete digital twin. How the models are linked together and deployed influences the overall complexity of the DT.
2.2. Digital Twin Configuration
A digital twin configuration isaset of modelsthat are linked in a certain way to run a simulation. Due to the modular nature of the DT, many configurations can be formed depending on the use case. The configuration referenced throughout this report is Software-in-the-Loop (SIL), which links the digital twin with a processor running vehicle control software through communication protocols like ethernet and serial.
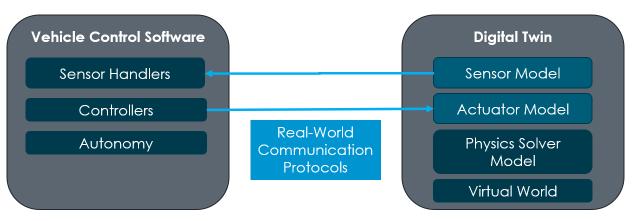
2.3. Use-Case for this Report
When performing validation and verification on a digital twin, the use case is an essential aspect to establish up front, as it has a large impact on whether the V&V activities provide acceptable results. Exampleusecases for the UUV DTinclude use as a testbed for developing autonomy algorithms,training autonomy algorithms, troubleshooting hardware, feasibility studies for new intended vehicle operations, or operator training. These would each pose very different V&V tests and success criteria.
This paper will discuss the V&V for the digital twin of one of HII’s small class UUVs when used for design and testing of the vehicle control software. This DT applications uses a SIL configuration introduced in Section 2.2 The V&V of individual models will be discussed in the context of the overall digital twin, as its modular nature lends itself to activities on a model-by-model basis.
3. Verification and Validation Processes
This section will cover the process implemented by the Modeling and Simulation team to complete validation and verification activitiesduring thedevelopment and testing of thedigital twin, following the framework laid out in [1]. The result of this process is a V&V report, one per individual model and one per DT configuration use case.
3.1. New Feature Identification & Use-Case
When a manufacturing contract is established, the modeling and simulation team works closely with the systems and software engineering teams to determine the digital twin requirements. New models or upgrades to existing models are identified to support the new vehicle variant. Requirements and development aretracked using Jamaand Jira(workflow and requirements management) tools and linked to the final V&V report created by the team.
For the test case discussed in this report, the digital twin must mimic the dynamics, processes, and environment of a small class UUV. If the digital twin data is proven to accurately represent the real
Figure 2: Software-in-the-Loop Architecture
vehicle’smotion through theenvironment and all feedback from sensorsand processes, thesoftware team (DT users) can use the digital twin to test vehicle control code in the virtual environment. Table 1 contains a single requirement as an example for this use-case. These requirements are the result of discussion between the DT Users and the DT developers.
Table 1: UUV Digital Twin Requirement Example for Vehicle Control Software Testing Use-Case
Requirement
UUV DT shall capture similar steady state flight to actual vehicle.
Acceptance Criteria Metrics
Pitch response percent error as compared to sea data is lower than maximum threshold percent error required per vehicle specifications.
Pitch response from the simulated vs actual vehicle log files.
The requirements for individual model V&V activities can differ in format from the use-case requirements. Many sensor requirements are driven by vendor specification sheets and communication protocols with vehicle control code, while actuator requirements are based on higher order modeling inputs. In the next phase of the report, the validation of an individual model will be discussed.
3.2. Criticality
Before beginning the validation and verification activities, a criticality assessment is completed for each model or digital twin configuration. This determines how rigorous the V&V process should be. The assessment looksat variouscriteriaabout themodel’simportancein theoverall digital twin configuration, such as number of other modelsitsdataimpacts, theeffect that model failurehas on the overall outcome, and whether similar data is available or has been used for decision making in the past.
The resulting consequence level is combined with the results integration level and supplementary information availability level to calculate a model influence level. This is required to determine overall criticality of themodel or digital twin configurationand thusdetermine the magnitude of V&V required.
3.3. Conceptual Model Validation
Conceptual model validation answersthequestion of whether the theories and assumptions used to create the model are correct and if the model representation of the real-world component is reasonable for the use-case. The goal of this V&V activity is to document this information before and during model development in a standardized format, as both V&V report content and a resource for future work. The conceptual model validation also clearly boundstheproblem spaceand use-case of each model, providing hard evidencewhen discussing applications of the overall digital twin. The UUV Fin model is presented below as an example case for the conceptual model validation process.
3.3.1.
Actuator Model
A UUV’s finsand propeller arethe main actuators of the UUV. The fins are a specialized model in the UxS DT because the model content is largely driven by computational fluid dynamics (CFD) modeling completed based on the specific fin dimensions. This CFD output drives the lift and drag coefficients (Cd and Cl) based on angleof attack (AoA) that feed into theUxS DT fin model, along with lift and drag force equations. These model development inputsarecompleted once and inform the developers who are writing the model. A different set of inputsisrequired to run the model. These include fin position commands, vehicle velocities, rates and wave elevation and density from the environmental state. The outputs from the model include lift force, drag force, and induced moments.
3.4.
Model Verification
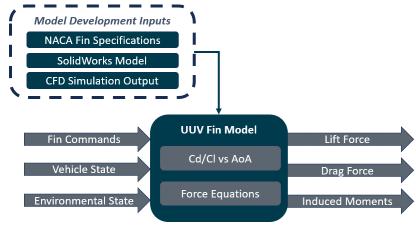
The next process implemented aspart of theUxS DTframework is model verification, which determines if themodel implementation sufficiently satisfies the requirements of the use case. Model verification is also documented as part of the individual model V&V process for the UxS DT.
3.4.1
Software Quality Assurance
The UxS Modeling and Simulation team is the primary digital twin developer and is responsible for conducting softwarequality assurance. At the beginning of the model development process, a new ticket is created in Jira and assigned. This ticket serves as a compilation platform for any information, bugs, anomalies, and testing sequences during the model development. The team also uses GitLab for configuration management of thedigital twin sourcecode. Individual branches are used by developers to make and test any new model, before merging work into the main branch. A detailed code review is completed at the merge stage, and approval is needed from two team members before any change is accepted. Additionally, tagged versions of the digital twin code base are created and saved after major changes. These Jiratickets, GitLab merge requests, and any other development work are included in the model V&V document as part of model verification.
3.4.2
Numerical Algorithm Verification
The modeling and simulation team is also responsible for numerical algorithm verification, which determinesthecorrectnessof thealgorithmsimplemented in themodel code. Theverification rigor in this category scales depending on the model complexity. Models like a GPS or a depth sensor implement straightforward formulas, therefore automated unit tests are sufficient for verification. Unit tests are implemented for all models as baseline testing that output simple binary pass/fail reports.
The UUV fin model outlined in Section 4.3.1 has a more complicated formulation, so the developer also conducts integration tests using the autopilot configuration to verify the model. An example mission includes a sandy flat seafloor, no environmental effects, and commands the vehicle to fly a box or lawnmower pattern. Visual inspection is used to verify that the vehicle dives, surfaces, turns left, and turns right when commanded to do so. Evidence from the unit tests and baseline autopilot missions is gathered and submitted as part of each model’s V&V document.
Figure 3: UUV Fin Inputs, Outputs, and Model Content
3.5. Results Validation Activities
The last process conducted as part of theUxS V&V activitiesisresult validation, which determines if the digital twin isan accurate representation of thereal-world problem.A key component of resultsvalidation is comparison with real-world data, so thepredictivecapability of thedigital twin can beunderstood. This process iscompleted by theDT developer, but input may be needed from SME’s and the DT users when performing analysis.
At thispoint, thefocusshiftsfrom theindividual model level to theoverall digital twin configuration. The entiredigital twin must beexercised and compared to real world datato determine if it meets the use case criteria. For theexampleusecase, thedigital twin is used to test the small class UUV control code in the virtual environment. Since ample sea data exists for the UUV, a software-in-the-loop mission can be completed that mimicsconditionsfrom thereal world. By comparing the outputs from the two missions, the digital twin’s predictive capability is established.
The general SIL validation process can be broken down into three major steps. The first is establishing communications between the vehicle control software and the virtual sensors and actuators running as part of the digital twin configuration.
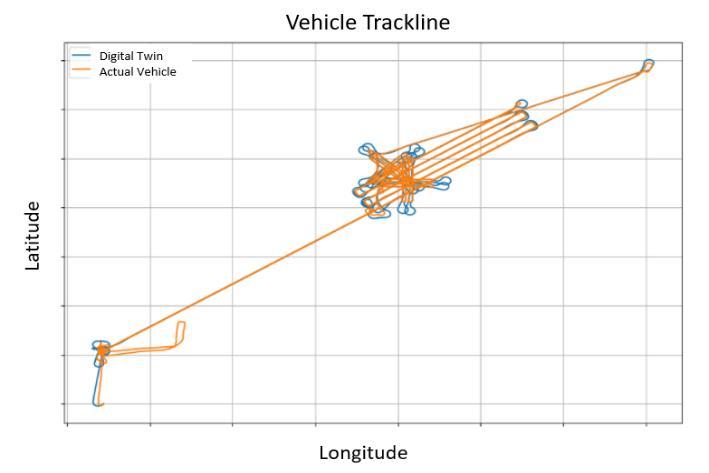
During this step, any errors in the sensor messaging format can be identified and fixed. Once the vehicle control code is working with the digital twin code, the mission starts. At this point, any major dynamics discrepancies are analyzed real time. For example, inability to diveor major pitch oscillationswould merit a pause in simulation and for the developers to revisit the digital twin models to identify bugs. Once the mission is completed, detailed post mission analysis (PMA) is conducted. The PMA determines the dynamic performance of the digital twin vs the realworld referent data and drives the conclusions about the digital twin’s predictive capability.
4. V&V Recommendations
Lastly, thedigital twin use-case requirementsarerevisited, and formal recommendationsaredocumented. Based on thedata generated by thevalidation and verification activities, an assessment is made about the use of the digital twin. Any requirements that are not achieved are discussed between the DT User/Sponsor and theDT Developer for thespecificuse-caseto identify error sourcesand next steps. This can often lead to updates to individual models in the DT or a reduction of the use-case scope.
5. Conclusion
Whileno digital twin can ever be aperfect representation of thereal world, the goal of this framework is to providestructured, reasonableguidelinesfor interpreting digital twin outputs and clearly understanding when they are applicable. One of the most important aspects of any engineering work is communicating the results with project sponsors and stakeholders. The individual model and integrated digital twin use case V&V reports that are generated by the modeling and simulation team will be indispensable documentation for ensuring confidence in the ability to use the DT as a tool for vehicle design, integration, and test.
Figure 4: Example PMA Data Comparison Between DT and Referent Vehicle
Converged Cyber AI
A Paradigm Shift in Cybersecurity
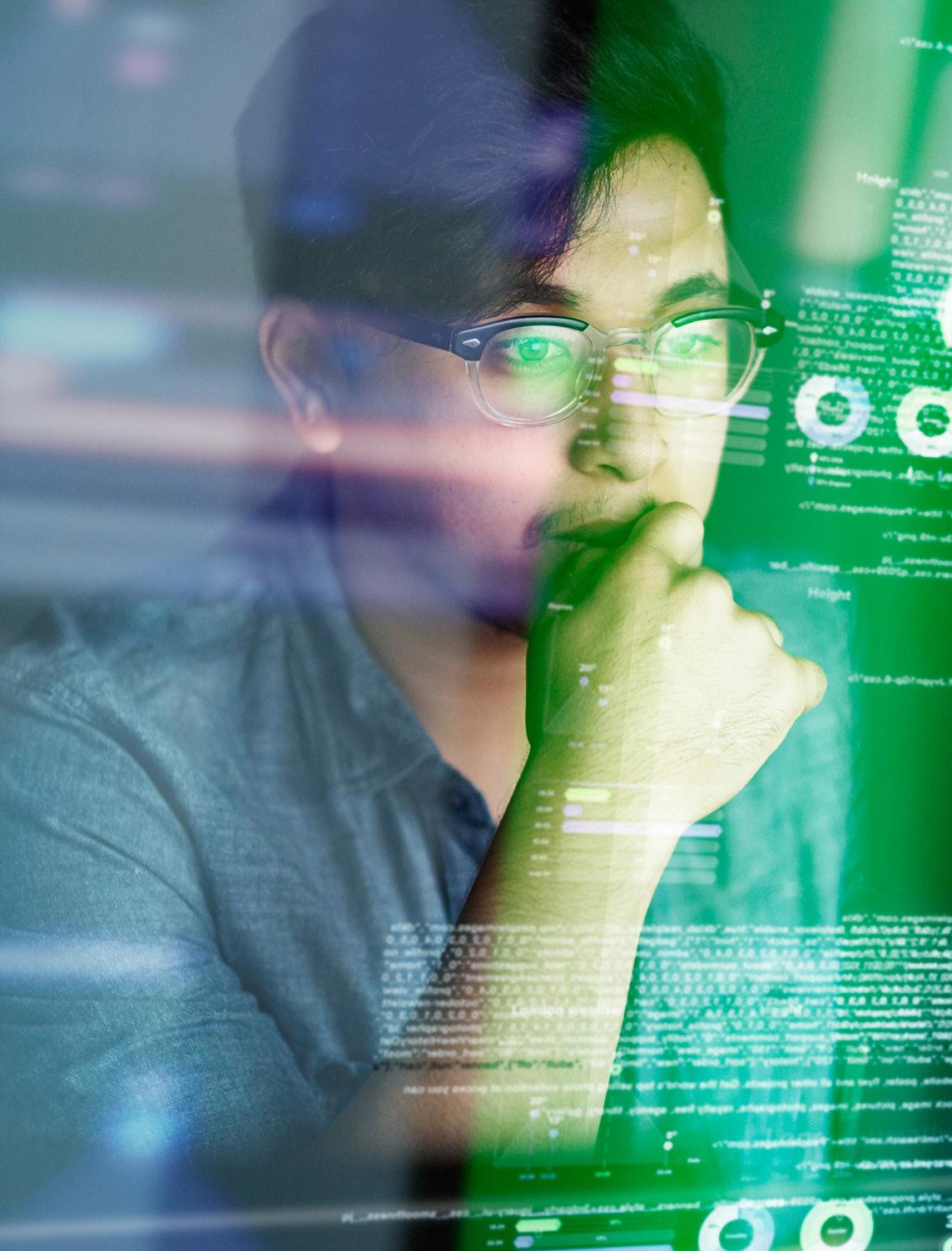
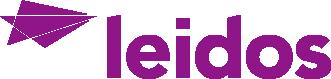
THE ADVANCEMENT OF GENERATIVE AI capabilities presents enormous potential for modernizing government operations but it also introduces new security gaps. While automation allows developers to move from concept to minimum viable product faster than ever, adversaries are developing similar AI-enabled techniques to discover and exploit security vulnerabilities.
To maximize generative AI benefits while minimizing threats, cybersecurity solutions must leverage AI as a first thought, not an afterthought. An AI-centric approach will enhance agencies’ abilities to both identify novel attacks and prepare to defend against them. To that end, Leidos is developing innovative converged cyber AI solutions with broad applications across government, from digital modernization to enhancing cyber-physical systems.
Generative AI for government
“The phrase ‘transformational technology’ is often overused,” says Bobby Scharmann, principal investigator for converged cyber AI at Leidos. “But with generative AI, it’s really appropriately used — there’s broad applicability in virtually every sector of our culture, from music and education and movies to improving the way humans and machines interact with each other.”
In the government space, generative AI has the potential to help solve the constant need to do more without an increased budget. Automated processes give human experts more time for deeper analysis and innovation. While fears around being replaced by AI have contributed to an often binary view of AI — that a task is either manual or entirely co-opted by AI — it’s more of a spectrum, with augmented decision-making as a sweet spot.
“Every analyst needs ways to help them get through the noise to the important information specifically relevant to them, so that they can make decisions instead of spending 95% of their day sifting through data and searching for what’s relevant,” says Robert Allen, a research scientist and solutions architect at Leidos.
In the software development lifecycle, the augmentative capabilities of generative AI can help developers write new code faster than ever, greatly increasing speed to delivery. However, research has also found that code
written with AI assistance tends to be buggier and less secure than traditional methods.
“It’s an important consideration that while you’re potentially putting out more systems faster, they are inherently less secure in some areas,” Scharmann says, “unless you have a security-first mindset throughout the development process.”
A cybersecurity paradigm shift
For Leidos, this mindset represents a paradigm shift. Since the cybersecurity field was established decades ago, solutions have largely been increasingly advanced versions of the same rule-based or signature-based methods. Though they’ve grown more complex and layered over time, there are still gaps that can be exploited, which then must be filled with new heuristic updates.
Generative AI, and its ability to evolve and adapt in ways rule-based methods can’t, offers gamechanging possibilities, but government leaders are understandably proceeding with caution. Industry partnerships offer reassurance and guidance as agencies move into uncharted territory.

Rule-based firewalls and other protections are familiar and predictable, “but what we need to combat an evolving AI-driven attack is a defense that evolves over time with AI. However, you don’t actually have full control over what the AI-enabled defense is,” says Meghan Good, senior vice president for technology integration at Leidos. “And that lack of visibility and explainability feels risky. We’re helping customers work through that risk.”
Given the sensitivity of government data, systems and infrastructure, technology leaders must take care in adopting new technologies. Leidos offers the benefit of innovative proving grounds and testing capabilities to hone new solutions before deployment. The key to developing cutting-edge converged cyber AI solutions is establishing learning environments that focus on: 1) all layers of security — perimeter, network, endpoints — and 2) offensive and defensive perspectives, or purple-teaming.
“Those two perspectives working together is really what strengthens the capability,” Allen says. “You might think that you have a good defensive product, but if you’re not actually testing that with an evasion capability, then you can’t strengthen it. The whole point is to have a system that’s learning from its adversary and improving on both sides over time.”
This is where AI can help in proactively discovering evasion techniques and vulnerabilities. Then, leveraging the same techniques, you can reinforce a system’s defensive posture. Through adopting a first principles approach to AI, breaking it down to its most fundamental interactions and objectives, Leidos is developing dynamic solutions with broad applicability.
“If a given capability is developed for an enterprise IT system, that doesn’t mean that the same underlying principles and underlying models are irrelevant in an embedded low-SWaP environment,” Scharmann says. “The deployment environments are different, but the
underlying fundamentals and characteristics are often very much transferable.”
Getting into an attacker’s mindset is difficult, especially when they’re leveraging their own AI-driven tools and exploits at the same time. AI offers ways to enhance both offense and defense skills beyond human capabilities.
“Leveraging AI/ML techniques allows you to discover novel and innovative approaches that otherwise might be subject to the imagination of the person codifying your more heuristic-based rules,” Scharmann says. “It allows you to branch out beyond just their imagination.”
Novel solutions for sophisticated threats
Leidos teams are taking a variety of innovative approaches to converged cyber AI solutions with applications across enterprise IT, cyber-physical systems, Internet of Things devices and more.
Among their latest research and solutions:
Generating training data: High-quality data is essential to training AI models in cyber attack detection, but there isn’t always enough data available that is representative of a particular attack type. “Our capability allows us to generate synthetic data that is higher quality than existing state-of-the-art methods out there,” Scharmann says.
TabMT, recently highlighted in a paper published by the prestigious Conference on Neural Information Processing Systems (NeurIPS), can take a small sample of data and create unlimited additional tabular data. It can also anonymize and later scrub the input data sample while still adhering closely to it, which is particularly beneficial given the high level of sensitivity and confidentiality required for handling government data.
“The whole point is to have a system that’s in effect learning from its adversary and improving on both sides over time.”
Robert Allen
Research scientist & solutions architect, Leidos
Understanding networks in context: Current enterprise network audits fall short when it comes to contextualizing risks. Leidos’ AI-driven network exploration aims to improve such practices by identifying attack paths to key cyber terrain and the exploits attackers may leverage to traverse them, creating invaluable situational awareness. Identifying the easiest exploitation paths to sensitive network segments or resources highlights the greatest risks to the network and essentially creates a map that can be used in the event of a compromise to identify where an attacker might traverse next to access valuable resources.
“It’s like going from a list of, ‘we have all of these assets in our environment’ to ‘we fully understand what the relationships are between them right now and the changes that are happening to them over time,’” Good says.
Enhancing existing rule-based systems: Leidos uses AI to discover rule-set gaps and automate defenses against potential attacks. From an offense perspective, it augments human capabilities with thousands of highconfidence, logic-preserving bypass approaches, while from a defensive perspective, it provides automated patching beyond signature-based methods.
“We look at our perimeters differently and take an adversarial, evasive approach through what we currently have deployed, whether that’s different layers of firewalls or zero trust network access layers,” Good says. “What malicious activity is still making it through from an evasion perspective? And then how do we proactively update our alerting capabilities to better look for these evasive attempts and make sure we’re detecting things before they occur in the wild?”
Testing AI solutions in relevant environments: To provide a safe way to do evasion and defensive testing using new products and capabilities, Leidos developed CastleClone, a cyber range solution that enables organizations to create digital twins of their environments and networks. The clones can be used for everything from penetration testing and malware analysis to training employees and new product assessments, all tailored to the organization’s unique needs.
CastleClone and digital twins can help ease fears or uncertainties around AI adoption because they offer government leaders a low-risk, custom environment for seeing firsthand how new tools and methods play out.
Building on top of leading commercial AI: To further enhance exploration and development, Leidos also
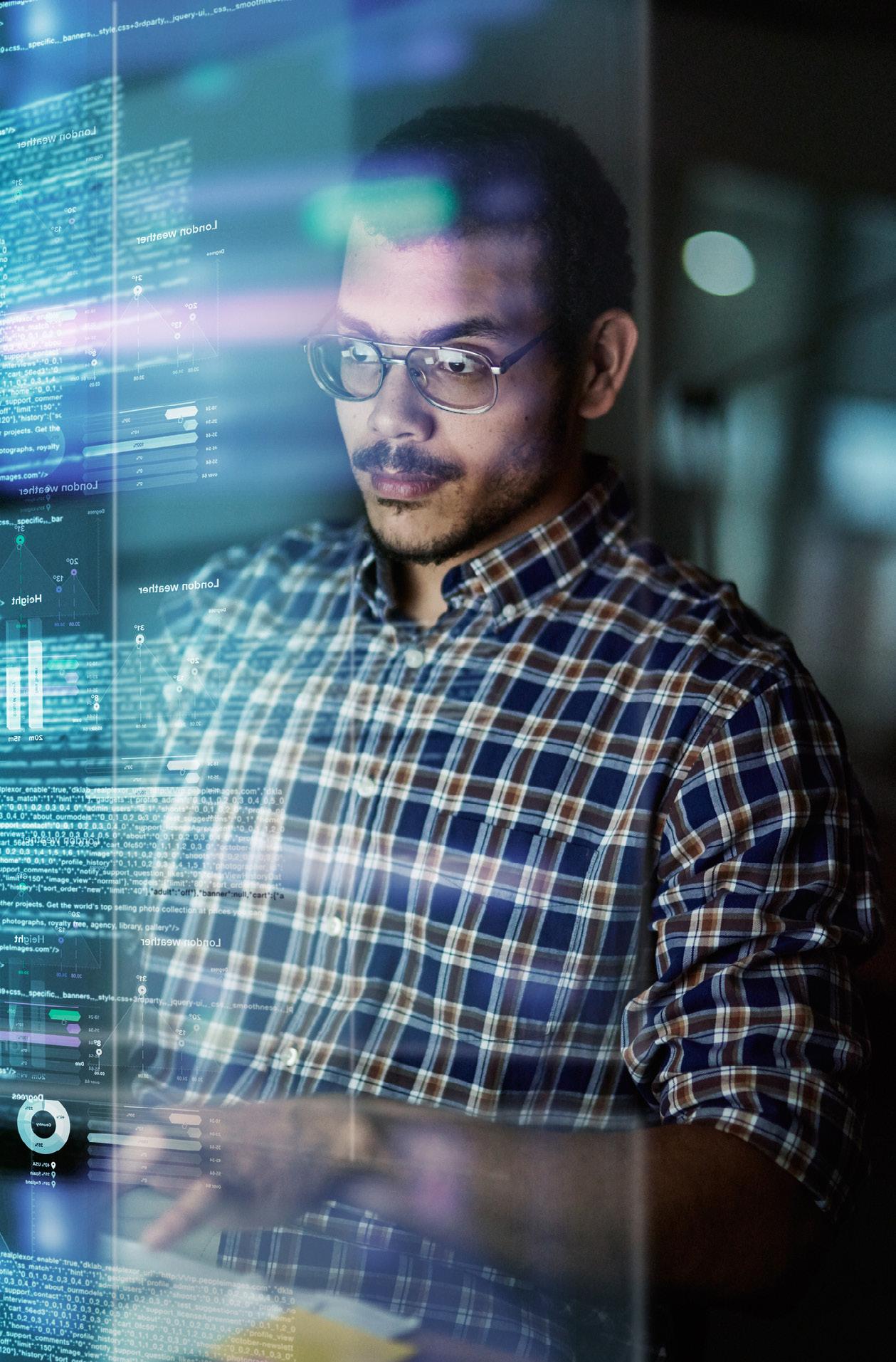
“We look at our perimeters differently and take an adversarial, evasive approach through what we currently have deployed, whether that’s different layers of firewalls or zero trust network access layers.”
Meghan Good Senior Vice President, Technology Integration
partners with leading commercial technology providers. Accelerating innovation doesn’t mean creating every piece from scratch but rather leveraging the best of existing solutions to create new capabilities. For example, Leidos has teamed up with code intelligence platform Sourcegraph to introduce secure, generative AI-enabled software development tools to government customers.
Good also highlights a partnership with Moveworks, a chatbot solution powered by GPT-class machine learning models with a conversational interface that works across a variety of systems.
“It makes it so you can get to information that was previously in silos,” Good says. “A capability like this is able to federate queries and search across and make it so that your data is really actionable.”
Keeping pace with evolving threats
As agency leaders continue to explore ways to incorporate generative AI into their cybersecurity solutions, Leidos offers expertise and guidance on how to approach it in a measured and strategic way. While it may be a transformational technology, that transformation doesn’t have to happen overnight.
“As part of our trusted AI approach, we start by analyzing and figuring out what we can do to better
assist someone, then how we’re going to augment them in the future, all before going into more autonomous operations,” Good says. “By going through those steps, by using digital twins and proxies, you can understand what the AI is doing and evaluate the risks before deploying it into production.”
Cybersecurity will always be a challenge in government, but a commitment to exploring novel technologies and solutions makes that challenge manageable. Staying ahead of adversaries is critical and increasingly complex. Generative AI solutions provide the opportunity to augment capabilities beyond what humans can do alone, enabling faster development and more robust security measures.
“We constantly need to be evolving, because our networks are evolving every day,” Good says. “We need a more dynamic way to look at our environments, and that’s the capability that we’re building.”
Learn more about how Leidos can help your agency successfully implement generative AI.
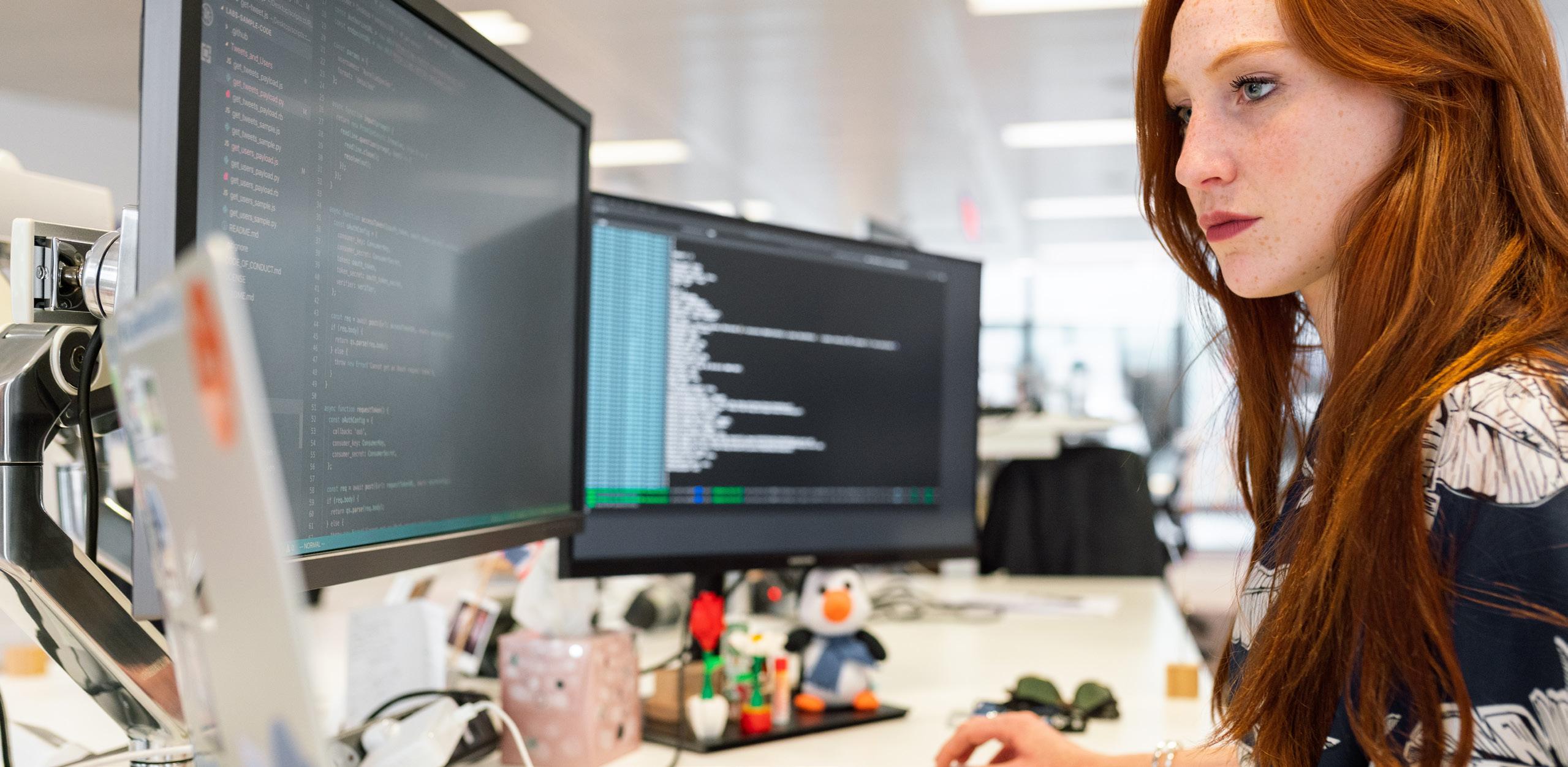
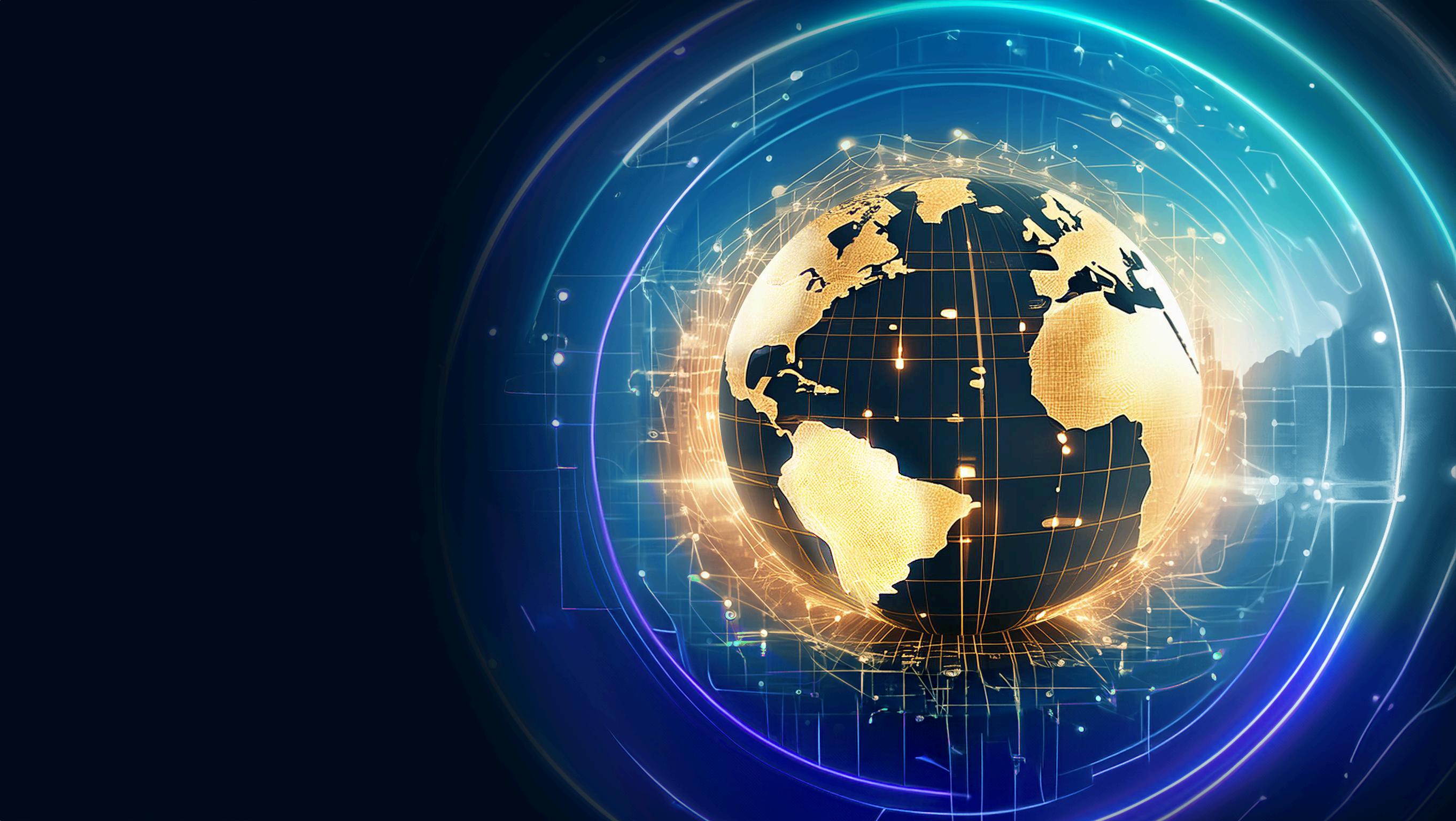
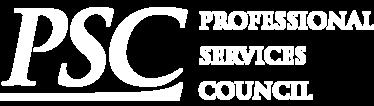