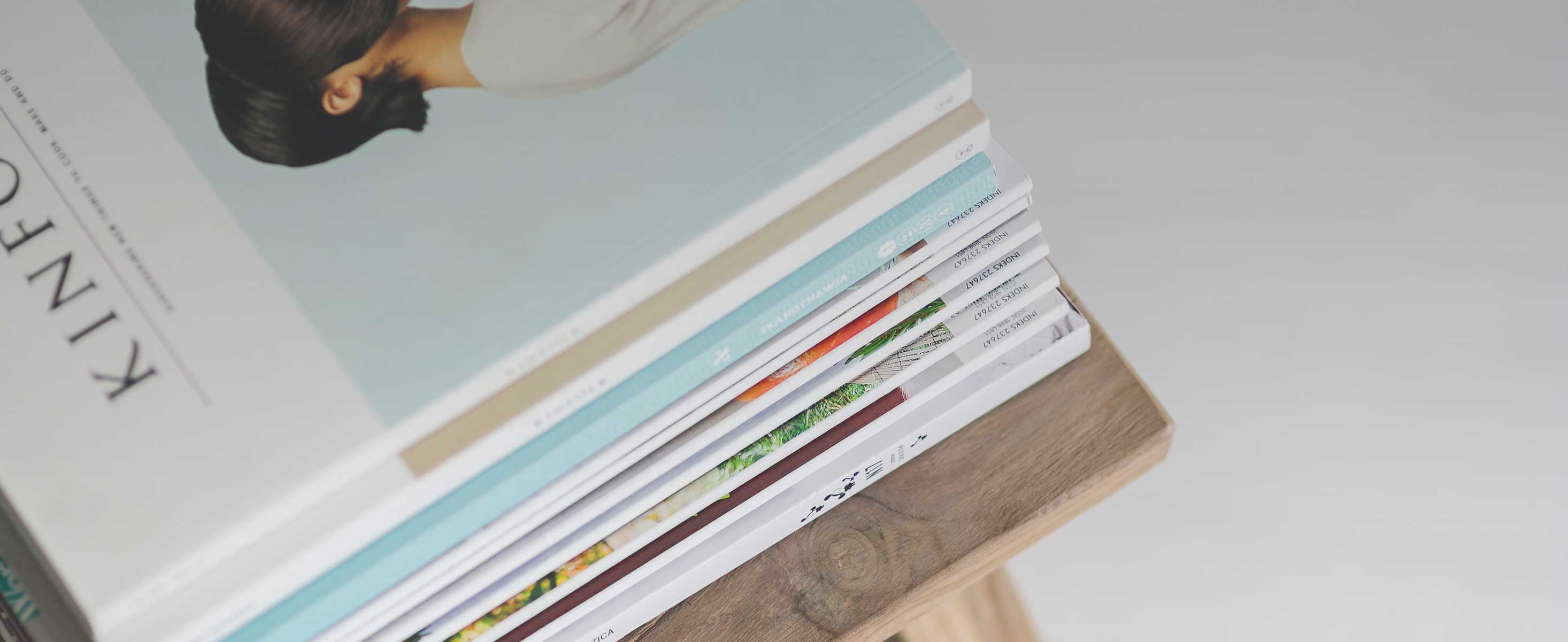
2 minute read
Edgar R. Mendoza
Dynamic Algorithms for Time-to-Event Processes Edgar R. Mendoza
Mentor: Emmanuel Appiah Department of Mathematics and Department of Chemical Engineering
Introduction: In the survival and reliability data analysis, parametric and nonparametric methods are applied to estimate the hazard/risk rate and survival functions [4, 6]. A parametric approach assumes that the underlying survival distribution belongs to some specific family of distributions (e.g., normal, Weibull, exponential). On the other hand, a nonparametric approach is centered around the best-fitting member of a class of survival distribution functions [5]. Moreover, Kaplan-Meier (KME) [5] and NelsonAalen [1, 8] type nonparametric approach does not assume neither distribution class, nor closed-form distributions. In fact, it just depends on a data. The Kaplan-Meier and Nelson-Aalen type nonparametric estimation approaches are systematically analyzed by the totally discrete-time hybrid dynamic modeling process in [2]. In the existing literature [4, 6], the closed-form expression for a survival function is based on probabilistic analysis. The closed-form representation of the survival function coupled with the mathematical statistics method (parametric approach) is used to estimate survival and hazard/risk rate functions. In fact, the parametric approach/model has advantages of simplicity, the availability of likelihood-based inference procedures and the ease of use for a description, comparison, prediction, or decision [6]. The goal of this research project is to develop new mathematical models and computational tools for time-to-event dynamic processes in biological, engineering, epidemiological, financial, medical, military, and social sciences. Materials and Methods: Algorithms developed will be validated by applying them to real-world data sets. Conclusion(s) or Summary: In this work, we hope to attempt the following: 1. develop an innovative alternative dynamic modeling approach for time-to-event processes. 2. Introduce time-dependent covariates (external and internal) in the developed models and consider more complex time-to-event dynamic studies. 3. introduction of discrete-time dynamic intervention process 4. formulation of continuous and discrete-time interconnected dynamic system 5. introduction of conceptual and computational state and parameter estimation procedures
References:
1. Odd Aalen. Nonparametric inference for a family of counting processes. The Annals of Statistics, pages 701–726, 1978. 2. EA Appiah and GS Ladde. Linear hybrid deterministic dynamic modeling for time-to-event processes: State and parameter estimations. International Journal of Statistics and Probability, 5(6):32, 2016.
3. David W Hosmer, Stanley Lemeshow, and Susanne May. Applied survival analysis. 2011.John D Kalbfleisch and Ross L Prentice. The statistical analysis of failure time data, volume 360. John Wiley & Sons, New Jersey, 2011. 4. Edward L Kaplan and Paul Meier. Nonparametric estimation from incomplete observations. Journal of the American statistical association, 53(282):457–481, 1958. 5. Jerald F Lawless. Statistical models and methods for lifetime data, volume 362. John Wiley & Sons, New Jersey, 2011. 6. Ganesh Malla and Hari Mukerjee. A new piecewise exponential estimator of a survival function. Statistics & probability letters, 80(23):1911–1917, 2010. 7. Wayne Nelson. Hazard plotting for incomplete failure data. Journal of Quality Technology, 1(1), 1969. 8. Olusegun M Otunuga, Gangaram S Ladde, and Nathan G Ladde. Local lagged adapted generalized method of moments and applications. Stochastic Analysis and Applications, pages 1–34, 2016. 9. Josef Schmee and Wayne Nelson. Estimates and approximate confidence limits for (log) normal life distributions from singly censored samples by maximum likelihood. Technical report, DTIC Document, 1977.