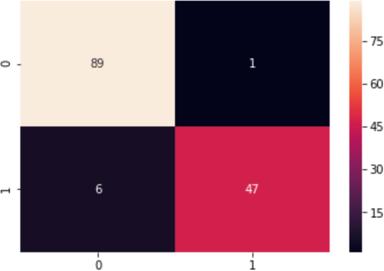
3 minute read
Kendall Lemons
Mentor: Indika Rathnathungalage Department of Mathematics
Introduction: Breast cancer is considered the most common type of cancer among women (World cancer report, 2008). It estimates that 23 out of 124 women will die due to breast cancer annually (Cancer Statistics Review, 2012). Therefore, early diagnose of breast cancer is critically important. Mammography, Fine Needle Aspiration (FNA), and Surgical biopsy are considered as some of the main techniques to diagnose breast cancer. Out of these techniques, the FNA is considered the essential diagnostic technique to detect breast cancer in the early stages (Fiuzy et al., 2012). Fiuzy et al. (2012) introduced a novel algorithm that can detect breast cancer using an artificial intelligence system and Fine Needle Aspiration (FNA). They tested 212 patient samples (malignant) and 357 healthy samples (benign) and used Evolutionary Algorithm (EA) with the Genetic Algorithm (GA) to separate malignant from benign samples. Daoud and Mayo (2019) discussed the use of machine learning techniques practical issues related to cancer prediction. Lemons (2020) use machine learning approaches to diagnose breast cancer. Further information related to FNA and breast cancer can be found. Fiuzy et al. (2012) and Saxena and Burse (2012). A breast cancer diagnosis can be seen as a classical binary data classification problem. In this study, we aim to use three artificial intelligence techniques to classify breast cancer patients based on the characteristics of their biopsies taken from the FNA technique. We use Neural Networks, Naïve Bayes algorithm, and Random Forest algorithms to construct classification models to classify breast cancer data and compare each algorithm's accuracy. Methodology: The construction of the classification model comprises several stages. At the first stage, the raw data is preprocessed and converted to structured data before constructing the learning algorithm. In this study, we use Neural Networks, Naïve Bayes algorithm, and Random Forest techniques. These algorithms are considered as three widely known artificial intelligence approaches with successes in other classification problems. In the next stage, a candidate model is constructed with the use of the algorithms mentioned above. Finally, this candidate model is modified until we obtain the final data classification model. In this study, we use Breast Cancer Wisconsin dataset, which was obtained from a publicly available source. There are details of biopsies of the breast taken from 569 patients comprising of 31 features and labeled classes [Benign, Malignant]. Some of the biopsies' features include radius, texture, perimeter, area, smoothness, compactness, etc. Results and Discussions: According to the experimental outcomes, the Neural Network classifier outperforms its counterparts, while Naïve Bayes and Random Forest follow closely after that. The classification algorithm's accuracy can be improved by the proper selection of the involved parameters. The following figures compare the accuracy of diagnosis for each selected algorithm.
Conclusion The impact of artificial intelligence on healthcare decision-making has the chance to change the industry dramatically. Healthcare is an industry with a lot of inefficiencies; perhaps one of the most pronounced is the issue of misdiagnosis. With AI approaches, healthcare can begin to make strides in the areas of misdiagnosis, as there are between 30,000 – 60,000 deaths
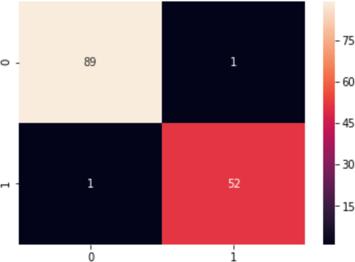
annually due to misdiagnosis of diseases and disorders. When using AI techniques, it is essential to select appropriate algorithms and properly select parameters to optimize the classification accuracy.
References:
Daoud M., Mayo M. (2019). A survey of neural network-based cancer prediction models from microarray data, Artificial Intelligence in Medicine, Volume 97, Pages 204-214. IARC. World cancer report: International agency for research on cancer. Lyon, 2008 Lemons, K., 2020. A Comparison Between Naïve Bayes and Random Forest to Predict Breast Cancer. International Journal of Undergraduate Research and Creative Activities, 12(1), pp.1–5. Shimizu, H., & Nakayama, K. I. (2020). Artificial intelligence in oncology. Cancer Science, 111(5), 1452–1460. Shweta Saxena, Kavita Burse: A Survey on Neural Net-work Techniques for Classification of Breast Cancer Data. International Journal of Engineering and Advanced Technology, 2012