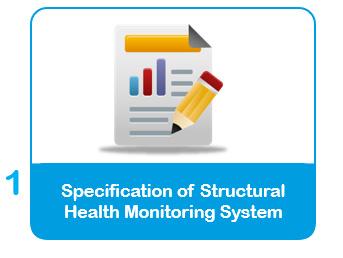
9 minute read
Using Digital Twins to Support a Safer and More Sustainable Industry
Digital twins have in recent years received increasingly more attention as their value creation has become more apparent when utilised in an efficient manner. More are realizing that an efficient use of digital twins might be the key to not only a more optimised future, but also in supporting other important aspects in the industry.
By Nadir Azam and Anders Andreasen, Ramboll
There are many understandings of what a digital twin is and what it is not. In this article, a digital twin is understood as a virtual representation of an object or system that potentially spans its lifecycle, can be updated from real-time data, and uses simulation, machine learning and reasoning to help decision-making.
A digital twin consists of several facets and layers, which may separately or combined contribute to increased value in their own way within different disciplines. In this article, we will focus on showing how digital twins specific for structural integrity, referred to in this article as True Digital Twin, and process simulations, referred to as Process Digital Twin, have been used to create value and support a safer, more optimised, and sustainable industry.
Why use digital twins?
There are several quantifiable and unquantifiable benefits of using a digital twin. The True Digital Twin technology, focusing on structural integrity of structures, has demonstrated its value throughout the past 25 years. It enables cost reduction, provides safer operation, and supports sustainability by allowing for optimisation of operational performance and life cycle management. These benefits are highly valuable in for example lifetime extension projects where the technology may be the differentiator between being able to reuse existing structures or having to decommission and build new ones.
The technology may at the same time provide invaluable information in time of need, for example during expected or unexpected critical events such as storms, ship impacts, vibrations etc.
The True Digital Twin technology allows for moving from costly and time-consuming experimental testing in the laboratory to cost-reducing full-scale testing in the field under real operational conditions, which enables safer and more cost-efficient operation of the asset.
Utilising a digital twin for process simulations can enable optimisation and offers unparalleled insight and facilitates increase in revenue, reduction in OPEX and more importantly reduced greenhouse gas emission footprint. The methodology presented in this article can be applied for brownfield optimisation as well as for basic design of greenfield facilities. In the design phase, the method can assist in choosing the best process scheme and selecting favourable operating conditions for minimisation of both CAPEX and OPEX. Another interesting and increasingly important area is lifetime extension and maximising the usability of existing facilities for tie-backs through detailed bottleneck identification studies.
Structural integrity: True Digital Twin
Ramboll combines digitalisation with innovative structural health monitoring to create the True Digital Twin for structures. The technology is a state-of-the-art methodology to facilitate coupling between the real physical conditions and their structural analysis models, which captures the real-life behaviour of structures in real-time.
The True Digital Twin technology has several applications within optimised operational performance and life cycle management.
Specific use cases include among others lifetime extension projects, root cause analyses, uncovering knowledge gaps, detection of changes, and predictive maintenance.
Methodology
The value creation expected from a True Digital Twin starts already in the specification of a structural health monitoring system as illustrated in figure 1. It is critical that the sensors defined are up to the required task and placed at the right locations to fulfil the overall purpose and objectives. There are several parameters to consider and evaluate when specifying a structural health monitoring system with the purpose of utilising it within structural integrity assessments. These parameters for the sensors include
for example: type, frequency range, accuracy, sensitivity, resolution, location of sensors and which post-processing methods will be utilised.
When the system is up and running, the real conditions are continuously identified using the Structural Health Monitoring System (SHMS), which provides information about the actual environmental loads and the corresponding structural response before the data from the monitoring system are analysed through a cloud computing solution. The True Digital Twin technology combines a variety of sensing technologies with an embedded measurement controller to capture, log and analyse real-time data.
The real-time data from the Structural Health Monitoring System (SHMS) is used to compare the real-time behaviour with the analysis model, and the analysis model is updated by using several novel methods to create a True Digital Twin, i.e. a structural analysis model that simulates the real-life behaviour of the structure.
Advanced analyses may now be performed using the True Digital Twin and assessment of the measured uncertainties associated with the analysis of structures, relating to elements such as quantification of model uncertainties and the direct link to Risk- and Reliability-Based Inspection planning (RBI). Continuous monitoring hence allows for continuous assessment of the structural integrity of the structures.
EXAMPLES OF USE
Lifetime extension The technology has been used in several lifetime extension projects throughout the years. The use of True Digital Twins in lifetime extension projects have resulted in large savings and sustainable reuse of structures, since quantifying the actual uncertainties leads to a safer and usually more optimised predictive risk-based inspection program.
Currently there are on-going projects where reusing oil & gas platforms as substations for offshore wind farms is being investigated and will also include this technology combined with advanced analyses.
Root cause analyses In cases where unexpected and unknown events occur, it might be invaluable to have measurement data from the events and being able to utilise it within structural integrity when it comes to understanding the root causes and how to mitigate these events from occurring in the future. Such events could for example include vibrations of either entire platforms or parts of a platform such as a topside module or flare tower. The technology has been used to monitor large structures to identify root causes of unexpected and unknown events, to provide an efficient mitigation strategy.
Process simulations: Process Digital Twin
The process digital twin is a process simulation representation of the chemical (and physical) processes taking place inside the plant process equipment. The process simulation invokes appropriate thermodynamic models of the fluid phase behaviour as well as correlations for transport properties etc. The process simulation combines several unit operations to make a digital representation of the real plant, which can be explored for several tasks such as for example: plant design, optimisation studies, diagnostics and troubleshooting, bottleneck identification and mitigation, training, safety studies, and what-if scenarios.
Process simulations have been used for decades as a representation of the real plant. However, extensive multi-parameter optimisation of complex process facilities is a more recent discipline, which has not yet achieved widespread foothold in the industry, especially where machine learning and multi-objective evolutionary algorithms are applied. In the following, we aim at describing the basic methodology and presenting some recent examples.
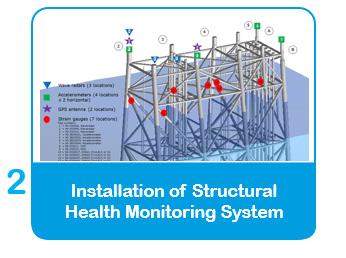
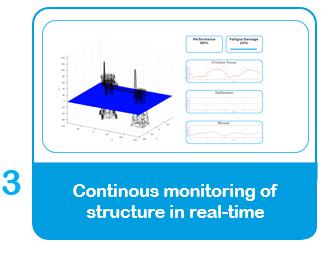
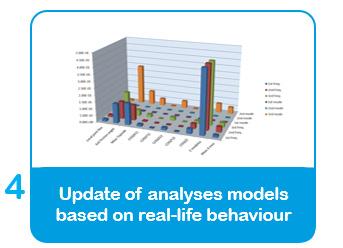
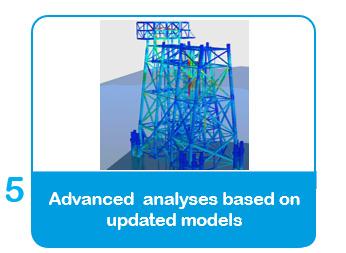
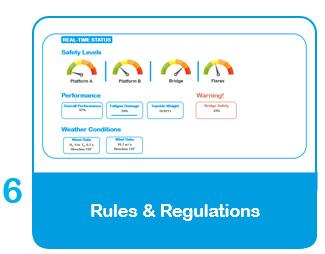
Methodology
The starting point is a high-fidelity model of the real plant. While the highest fidelity can be provided by a detailed process simulation model, a surrogate model may be a better choice, provided the model is well trained via e.g. supervised machine learning.
Using a surrogate, the insight of the model can be fully leveraged through massively parallel computations while minimising software license cost. The model is leveraged in combination with global optimisation methods. The number of variables of interest, which for example may include pressure, temperature, flow etc. from various places in the process, range from a few parameters up to hundreds of variables, with a typical range being 10-20 variables of interest.
The objective of the optimisation may for example be maximisation of a product yield, improved product quality, minimisation of production cost/OPEX, minimisation of emission-footprint / energy optimisation or any combination of these objectives. However, not all variables can be set freely due to natural limitations such as design pressure limits, design temperature limits, cooling/heating medium constraints etc. Constraints may include product quality such as crude oil export RVP/TVP, gas export quality, equipment constraints etc. All these limitations and constraints must be handled while seeking the best solution for the objectives.
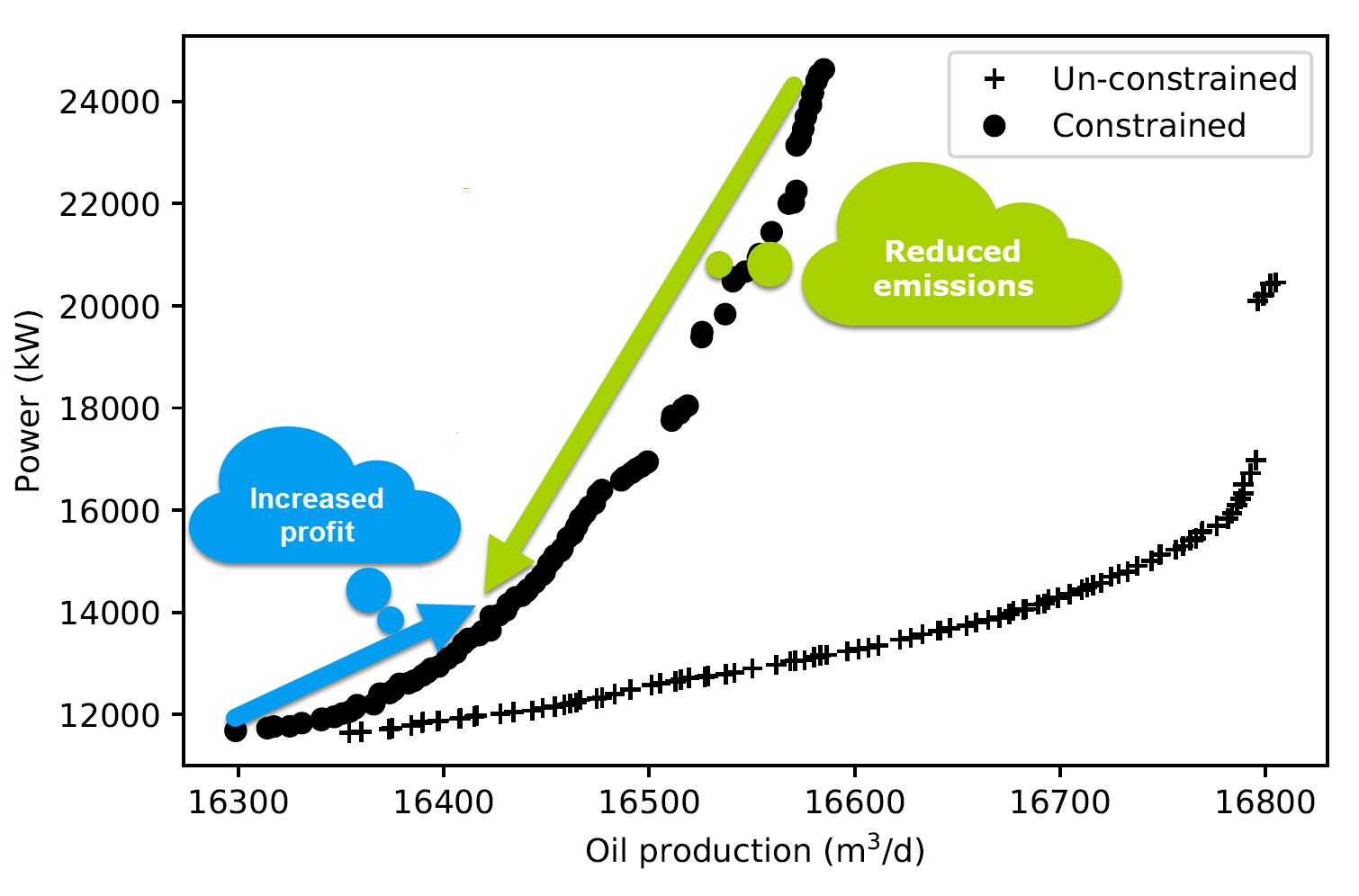
EXAMPLES OF USE
Increased product yield In various optimisation studies considering both full process simulation as well as surrogate models, the process digital twin approach has identified a significant optimisation potential for increased stabilised oil production. An increase of 0.1-1.3 % can be achieved, which over lifetime is a significant increase in revenue, and this is achieved without any hardware modifications, i.e. only obtained through changes in the operating parameters. The magnitude depends on for example the reservoir fluid and plant topology. Elucidating the optimisation potential requires a vast amount of simulation realisations, often 10,000-100,000 evaluations.
A main challenge is competing forces of different objectives. Often an increase in recoverable stable oil comes with an expense of increased power demand, see figure 2. Thus, the challenge is to identify the sweet spot that gives the most value for money - either the highest increase in production (starting from operating point A) at the lowest increase in energy demand or the highest reduction in energy demand for the lowest penalty in oil production (starting at point B). For processes not continuously optimised it may be possible to improve both parameters (starting at suboptimal point C).
Reducing separation gas plant footprint for NGL rich fluids During recent years, several projects including various development phases for reservoirs rich in NGL (natural gas liquids) have been executed, such as for example the Hejre and the Solsort fields in the Danish sector of the North Sea and the Yme re-development on the Norwegian continental shelf.
Such fluids are challenging since NGL may build up in the compression system and condensate recycle streams, increasing the power requirement substantially. For some fluids it may not be feasible to extract NGL offshore and it may be chosen to export excess NGL via either off-spec gas or off-spec crude. Using a process digital twin approach, it has been demonstrated that for a realistic hypothetical fluid, the topside energy requirement could be reduced by approx. 20% by choosing the gas export over the crude export for transport of NGL to shore for downstream extraction. This would translate into a significant downsizing of the entire compression system and gas treatment facilities with a significant concurrent reduction in CAPEX.
Authors
Nadir Azam
is Business Development Manager, working with digitalisation and innovation within structural integrity management for offshore structures. He has 15 years of experience in working with complex lifetime extension projects.
Figure 2: Pareto frontier for constrained and unconstrained multi-objective optimisation of an oil and gas separation plant
Anders Andreasen
is Technical Manager and chemical engineer, working with chemical process design for energy storage, carbon capture, utilisation & storage, Power-to-X, and gas production and utilisation.
Final reflections
We are still in the early days of digitalisation, but we believe it will be a vital part in creating an optimised transition from fossil fuels to renewables and support the green transition with accelerated technology-based knowledge.
Bright ideas. Sustainable change.
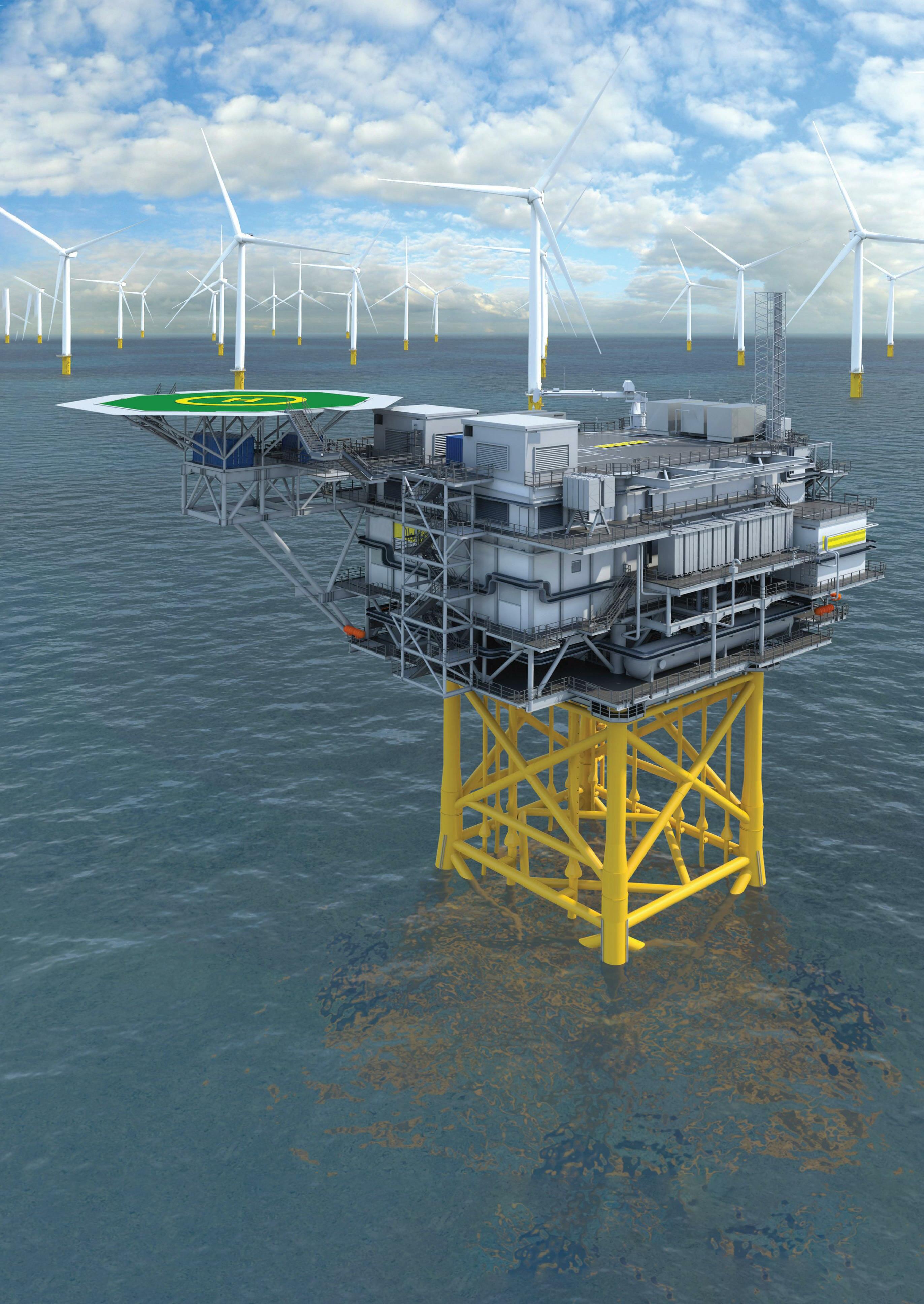