Limitations of Applying Summary Results of Clinical Trials to Individual Patients: The Need for Risk Stratification
Editorial Summary
One of the limiting factors in contemporary wound care research is the elidation and ignorance of the pathologies with wound care data. This leads to the use of statistical tools that cannot deal with the nature of the data. This article explores some of the problems and solutions with the application of wound care data, and outlines the need for risk stratification.
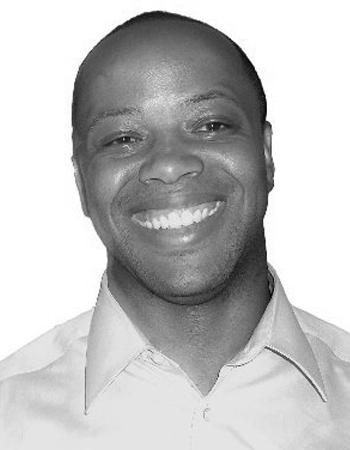
Introduction
When I began writing this article I originally titled it ‘The Pathology of Wound Care Data’, but the title did not seem quite right. Pathology refers to the ‘study of causes and effects of the disease or injury’; this was not quite what I wanted to write about regarding wound care data. The more I thought about it, pathophysiology seemed to fit better. Pathophysiology is the study of a ‘disordered physiological processes that cause, result from, or otherwise are otherwise associated with a disease or injury’.
Wound care data in particular, but data in general, can be thought of as the result of a data generating process in the real world. In a perfect world, researchers design experiments where the data generating process is not one of these ‘disordered physiological processes’; however, in the real world, wound care data is often ‘diseased or injured’. Wound care data possesses many statistical pathologies that make analysis and inference difficult, and if not identified and treated, these pathologies can cause analysts and researchers to arrive at conclusions that range from completely wrong, to less correct than they could be.
I have often thought of creating something like a DSM-4 (The Diagnostic and Statistical Manual of Mental Disorders, fourth edition, text revision (DSM-IV-TR) (American Psychiatric Association [APA], 2000) is a compendium of mental disorders, a listing of the criteria used to diagnose them, and a detailed system for their definition, organization, and classification) of wound care data; a guide on how to identify
these statistical pathologies, and how to attend to them in a principled and ethical manner. This article is an abridged version of such a guide, where we focus mostly on the diagnosis and description of the pathologies that I come across most often in my work: censoring and truncation, measurement error, fat tails, and zero-inflation.
Censoring and Truncation
Censoring occurs when the value of an observation is only partly known. An example of censoring, when the number of observations is equal to one, would be an unstageable pressure ulcer. As there is an obstruction to viewing the underlying tissue, the clinician cannot determine whether the wound is actually a stage 3 or 4 pressure ulcer, and thus the ‘unstageable moniker’ is used, which explicitly indicates that censoring has occurred. Carrying this idea forward, one could think of a deep tissue injury as a censored stage 1, 2, 3, or 4 pressure ulcer.
Censoring most often occurs in the longitudinal analysis of wound care data; longitudinal wound care data comprises repeated measurements of the same wounds over time. The most often used longitudinal design is a pre-post test (one repeat measurement), where there is one initial baseline measurement, and then one single follow-up measurement. When there is more than one repeat measurement, we call this a longitudinal cohort study. In the context of longitudinal data, there are 3 types of censoring: left-censoring, right-censoring, and interval-censoring. Interval-censoring can combine with left or right censoring, and a wound can have multiple occurrences of
interval-censoring. Truncation is related to but not the same as censoring, insofar as there is no missing information about the wound itself, only the absence of data during the period of interest. When it relates to longitudinal data, truncation occurs when the wound heals prior to the start of the study window. For example, let’s say that our study has two screening visits, and the wound is initially observed at the first screening visit and heals by the second screening visit; that wound would be truncated, relative to the study window.
Censoring can be attended to by using imputation methods to estimate the true value of the measurement(s) at the missing time step(s). Methods range from the naive, such as linear interpolation, to the sophisticated such as hierarchical models, Gaussian processes, or neural nets.1
Measurement Error
Measurement error occurs when the recorded values of an observation are different from the true value. The most common example is a simple wound measurement; any wound has an empirical value for its area, however, there are many ways to measure the wound, both analog and digital. Inherent in all of them is the fact that there is a gap between the empirical (true value) and what is measured. Even if the same method is used to measure the wound, say measurement tape, the measurements will be slightly different depending on who is performing the measurement.
It is good practice to assume the omnipresence of measurement error and to be reasonably skeptical of data. Measurement error can be attended to by using exploratory data analysis, and Bayesian inference, including but not limited to Bayesian Hierarchical models, to estimate true values.2
Fat Tails
Fat tails occur when the empirical distribution of data exhibits a large skewness to one or both sides. Generally, in wound care, the skewness is to the right, such as in the representation of a cohort’s wound time-to-heal (TTH) in days. Fat-tailed distributions, unlike normal distributions, cannot be described fully using just the mean (or average) and standard
deviation.
In fact, the notion of mean and standard deviation in fat-tailed distribution does not connote the same thing as in a normal distribution; in a normal distribution, the mean describes the central point of the data, and the standard deviation (or sigma) is the spread of the data around that distribution. Of values, 68% will fall within one sigma of the mean, 95% within 2 sigmas, and 99.7% within 3 sigmas. These implications don't hold if the empirical distribution of the data is fat-tailed, and pretending as if they do leads to a myriad of inferential problems.
Fat tailed distributions abound in wound care data. We see it not only in TTH, but in the starting area of wounds in a cohort, proportion of high risk patients who develop state 2 - 4 pressure ulcers, total cost of wound care, daily cost of wound care, etc. We even routinely run into our friend, the Pareto distribution, or as it is commonly referred to, the ‘80 - 20 rule’, when describing the share of costs related to a large population of wounds. For example, 20% of wounds result in 80% of wound care costs to an insurer, or 2% of wound care cases result in 30% of a nursing home’s medical malpractice costs.
Fat tails are not amenable to the traditional way of analyzing data. That is to say, the mean and standard deviation are meaningless in describing and analyzing this type of data. The mean is not the parameter of interest, but the extremes, as they generate most of the consequences. The best approach to understanding fat tailed distributions is through probabilistic models that estimate parameters or values of interest.3
Zero-Inflation
Zero-inflation occurs when data exhibits a disproportionately large number of zeros in its empirical distribution. Zero-inflation generally suggests one of two things; first, that there are two processes captured in the data, or second, that left-censoring is occurring in a significant part of the sample.
Let’s discuss the first case; say a nursing home collects data for a weekly count of new nosocomial pressure ulcers. They find that in 20 of 52 weeks there were no new nosocomial pressure ulcers, and in the remaining weeks between 1