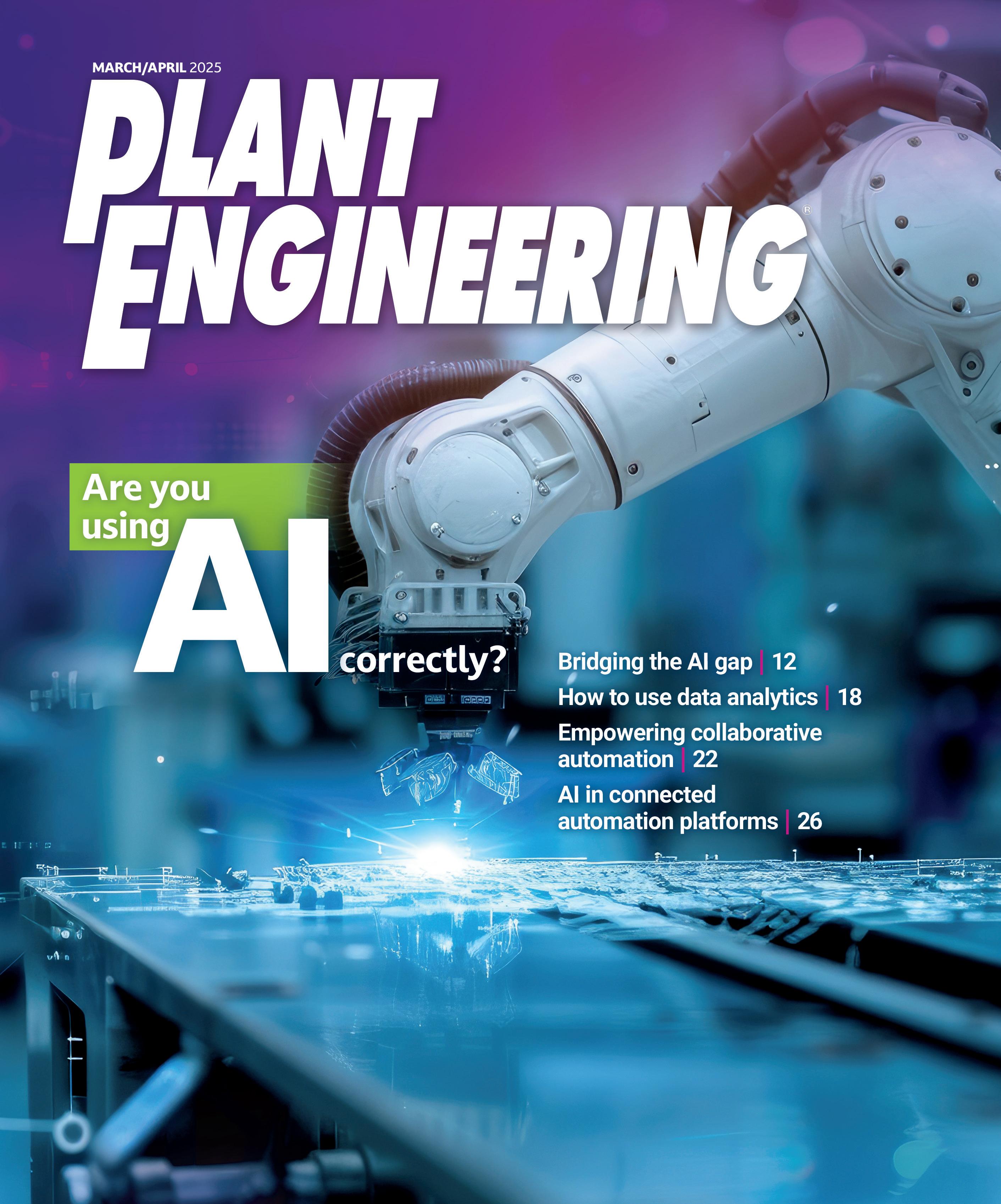

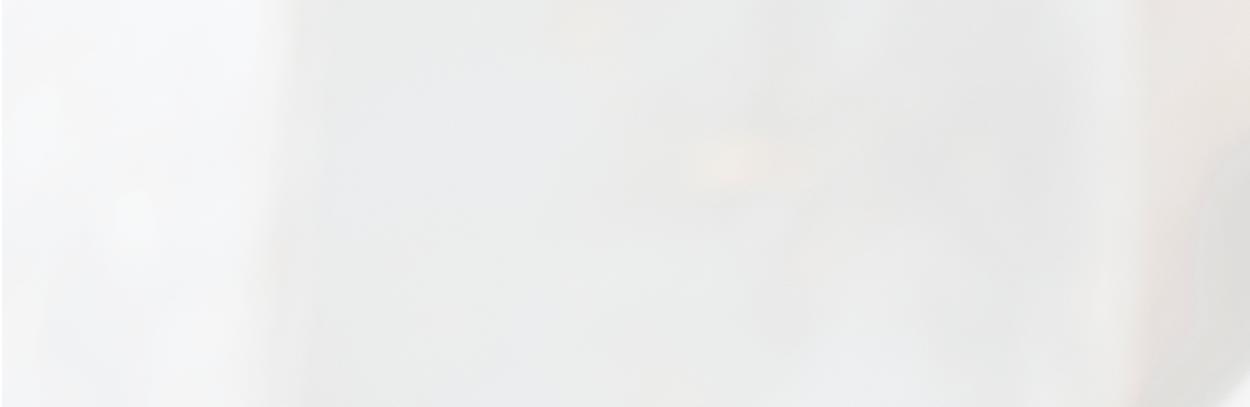
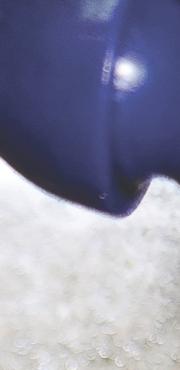
CITO MODULAR PROCESS VALVES STARTING AT $29.00 (SP-1000-BR)
CITO Modular Process Valves
CITO™ StackFlow™ series valves are a durable series of modular process valves that can stack together to form a valve bank assembly. Each module includes common inlets at each end and an outlet discharge at the bottom. Manual and solenoid valves are available, as well as distribution blocks that can all be included in the same assembly. All components are proudly made in the USA.
CITO StackFlow series manual ball or diaphragm process valves offer a high flow coefficient. These valve assemblies save space and allow for an easy-to-maintain system. Inlet and outlet port adapters are sold separately.
• Pressure rating: 5 to 100 psi (minimum pressure for manual valves 0 psi)
• Temperature rating: 20 to 160°F [-6 to 71°C]
• 24 VDC valve has an electrical override button
Also Available
General-Purpose Process Valves
Stacking/Manifold Process Valves NSF Potable Water Valves
Other components available include:
• End caps (blank or with integral temperature or pressure gauge)
• Inlet and outlet port adapters
• Outlet flow controls
• Check valves
• Flow indicators
No matter how many “ings” your process has, Productivity PLCs can handle them all while providing substantial cost savings. Whether you’d prefer a single controller for complete end-to-end control or a segmented control system with multiple controllers, the scalable Productivity PLC family has what you need for less.
This family offers three series of PLCs each with different I/O capacities but all using the same FREE advanced programming software, so you can easily scale your control hardware up or down depending on the application.
NEW! More discrete and relay I/O expansion modules have been added to the Productivity PLC family for even more affordable control options.
For the Productivity1000 PLC series:
• A 4-channel, high current relay output module with up to 7A/point and four Form C contacts, perfect for applications with higher current loads
For the Productivity2000 PLC series:
• A 6-channel, high current (7A/point) relay output module with both Form A and Form C contacts
• A 16-point low voltage discrete input module and 16-point low voltage discrete output module, ideal for devices that utilize transistor-transistorlogic (TTL) and voltage levels ranging from 3.3 to 5 VDC
Production processes, above all, must be safe, reliable and efficient. With our level and pressure measurement technology, you get exactly that. Durable sensors and accurate measured values make your work smarter, easier and more sustainable. Everything is possible. With VEGA. We don’t wait for
vega.com
5 | How can manufacturing plants best incorporate AI?
Our state of the industry study shows manufacturers are implementing artificial intelligence. What does that look like?
6 | Maintenance: Experts reveal these maintenance trends to watch
Our panel of experts reveals some of the top manufacturing maintenance trends.
12 | Bridging the AI gap: how to harness AI's potential
How can plant engineers overcome the gap between AI's potential and practical execution in the workplace?
18 | How to use data analytics to develop a winning manufacturing strategy
Data analytics help manufacturers increase uptime, reduce costs and improve quality, enhancing overall equipment effectiveness.
22 | How to harness physical AI to empower collaborative automation
Physical AI can empower end users and enhance existing applications performed by cobots.
26 | What is the role of AI in connected automation platforms?
A fit-for-purpose, seamless automation system is the most effective way to deliver AI solutions.
30 | Hazards in automated manufacturing systems and how to mitigate them
Automation enhances productivity and safety, but it introduces unique hazards that must be effectively managed.
36 | Predictive and preventive maintenance in the age of AI
Data-backed software and AI can help modern manufacturers predict and prevent problems.
40 | How to combat cyberattacks with advanced software technologies
Advanced software technologies can help manufacturers secure vulnerable infrastructure.
45 | Do your plant hardware and software really integrate?
How can manufacturers balance connectivity and the large capital expenditures to modernize?
Lubriplate’s ultra-high-performance, 100% synthetic lubricants have been engineered to provide unsurpassed performance in the most demanding plant environments. They provide a wide range of benefits designed to make your plant run better. Benefits include: extended lubrication intervals, lubrication consolidation through multiple application capability, reduced friction, extended machinery life and reduced downtime. Products include...
HIGH-PERFORMANCE SYNTHETIC GEAR OILS
SYNTHETIC AIR COMPRESSOR FLUIDS
SYNTHETIC HYDRAULIC FLUIDS
HIGH-PERFORMANCE SYNTHETIC GREASES
NSF H1 REGISTERED FOOD GRADE LUBRICANTS
ECO-FRIENDLY SYNTHETIC LUBRICANTS
SPRAY & SPECIALTY LUBRICANTS
AMARA ROZGUS, Editor-in-Chief ARozgus@WTWHMedia.com
SHERI KASPRZAK , Managing Editor SKasprzak@WTWHMedia.com
MICHAEL SMITH, Art Director MSmith@WTWHMedia.com
AMANDA PELLICCIONE, Marketing Research Manager A Pelliccione@WTWHMedia.com
SUSIE BAK, Staff Accountant SBak@WTWHMedia .com
H. LANDIS “LANNY” FLOYD, IEEE Life Fellow
JOHN GLENSKI, Principal, Automation & Digital Strategy, Plus Group, A Salas O'Brien Company
MATTHEW GOSS , PE, PMP, CEM, CEA, CDSM, LEED AP, Senior Vice President, CDM Smith
Are you a subject matter expert in one of these topics? Would you like to write an article on one of the topics below? If so, please submit an idea to:
https://tinyurl.com/PlantEngineeringSubmissions
• Asset management for energy efficiency
• Expert Q&A: Hazard protection, hazardous environments
• Expert Q&A: VFDs and VSDs
• Fall protection
• Lubrication
• Maintenance and energy management tools
• Motor calculations
• Predictive maintenance
Content For Engineers. WTWH Media focuses on engineers sharing with their peers. We welcome content submissions for all interested parties in engineering. We will use those materials online, on our Website, in print and in newsletters to keep engineers informed about the products, solutions and industry trends.
* https://tinyurl.com/PlantEngineeringSubmissions gives an overview of how to submit press releases, products, images and graphics, bylined feature articles, case studies, white papers and other media.
* Content should focus on helping engineers solve problems. Articles that are commercial in nature or that are critical of other products or organizations will be rejected. (Technology discussions and comparative tables may be accepted if nonpromotional and if contributor corroborates information with sources cited.)
* If the content meets criteria noted in guidelines, expect to see it first on the website. Content for enewsletters comes from content already available on the website. All content for print also will be online. All content that appears in the print magazine will appear as space permits, and we will indicate in print if more content from that article is available online.
* Deadlines for feature articles vary based on where it appears. Print-related content is due at least three months in advance of the publication date. Again, it is best to discuss all feature articles with the content manager prior to submission.
LEARN MORE AT: https://tinyurl.com/PlantEngineeringSubmissions
Share your knowledge and stories about improving operational efficiency.
Arecent “state of the industry” study conducted by Plant Engineering showed that 28% of respondents plan to implement artificial intelligence (AI) in the next 12 months to improve operational efficiency. But what does that look like?
It’s different for each plant, and difficult to put a “best practices” stamp on a plan. Implementation generally requires a variety of systems to talk to each other, which may mean updating portions of a plant’s software, sensors or other data-collection tools.
This relies heavily on sensors and other tools to collect information.
Amara Rozgus, Editor-in-Chief
Achieving operational efficiency could mean improving automation with AI, such as tapping into advancements in machine learning, cloud computing and natural language processing. It could also include automation as part of a more holistic approach to managing a manufacturing plant.
Automation in the form of robots is also a collaborative way to use AI. By incorporating these collaborative robots — or cobots — manufacturers can automate aspects of existing product manufacturing or even add new production options.
AI can be added to a plant’s predictive maintenance strategy, reducing the need for a break-fix approach. Plant managers can use data to review historical patterns and predict when or where a failure might occur.
Digital twins offer a digital replica of physical systems to allow engineers to conservatively simulate processes within a plant, allowing for process optimization that retains the ability to test new options without disruption. Depending on the size of the plant and the procurement volume, supply chain management can be brought to a new level with AI. The amount of data AI can comb through helps procurement accurately obtain products at the lowest price and correct time. Sourcing and negotiations can be handled by AI, too.
Customer service can be tackled by AI to ease operational efficiency, with basic questions fielded by chatbots and more complex questions managed by humans.
Finally, recruiting is one of the toughest workforce challenges for plant engineers. In the same study, 63% indicated they had difficulty hiring skilled technicians, and 22% said they struggled to retain experienced employees. While some may shun using AI, its incorporation into the hiring process, training or even knowledge retention can be invaluable.
Would you like to share your knowledge on any of these topics? We will be covering them over the next several months, so please contact me to share your story. PE
This panel of experts reveals some of the top manufacturing maintenance trends. From artificial intelligence and machine learning to predictive maintenance, you’ll want to watch and implement these strategies.
• Discover the latest trends in manufacturing maintenance.
• Learn ways in which organizations are using artificial intelligence (AI) and machine learning (ML) to better predict problems.
• Determine the best methods for avoiding downtime.
Question: What future trends should engineers, plant managers and designers expect for predictive and/or preventive maintenance, looking one two years ahead?
Greg Nolan: Compressors being an integral utility for most manufacturing, we are seeing an increased priority being given to both predictive and preventive maintenance (PM). Remote monitoring capabilities will continue to expand, allowing compressed air users to see more information regarding their compressed air system on their phone or tablet.
Stacey Jones: Two things: Enhanced condition monitoring with wired and wireless sensors. The use of sensors for condition monitoring is becoming more widespread. These sensors track performance and provide insights into potential issues like abnormal vibrations or heat, enabling proactive maintenance.
Second, increased adoption of predictive maintenance leveraging artificial intelligence/machine learning (AI/ML)-enabled technologies. With advancements in internet of things and AI technologies, more organizations will be leveraging real-time data to anticipate equipment failures before they occur. This approach can help to reduce downtime and extend asset life but change management will be critical to realize the true value of implementing these new technologies.
Mark Ruffin: Predictive maintenance (PdM)
strategies leverage real-time monitoring and analytics to anticipate failures before they occur, while preventive maintenance follows a scheduled approach to replace components at fixed intervals. Combining both methods ensures the best balance of cost efficiency and reliability.
As it relates to sealing products, temperature cycles can increase the likelihood of leaks. Extending the run times of units increases the likelihood of having process upsets that can cause temperature swings. Some sealing technologies are more forgiving of temperature swings than others. Engineers should work with their sealing product supplier to understand what product would be best for their application and implement assembly best practices. Having the right sealing product and installing it the correct way will provide the application with the reliability associated with longer run times.
Question: What’s the current trend in maintenance for industrial and manufacturing facilities?
Ruffin: Companies are focusing on reliability-centered maintenance (RCM) and predictive strategies that optimize uptime, reduce failures and ensure compliance with safety and environmental standards. These approaches rely on advanced monitoring, historical data and expert-driven analysis.
Additionally, there is an effort to extend turnaround cycles. Previously, a five-year cycle was considered relatively standard. Currently, it is not uncommon for units to run for six years between turnaround cycles.
Question: What are the fundamental distinctions between predictive and preventive maintenance and how do these approaches complement each other in an ideal maintenance strategy?
John Wilkerson: The fundamental distinction between preventive and PdM lies in their approach and application. Preventive maintenance follows
a manufacturer's recommended schedule based on a generic installation scenario. Essentially, if a machine is installed as the manufacturer specifies — or within acceptable variations — performing the recommended maintenance ensures reliability between service intervals.
PdM, on the other hand, uses data-driven insights — gathered through human expertise, technology or a combination of both — to monitor the actual performance of a machine. It identifies when the equipment is deviating from the manufacturer's baseline parameters and allows operators to take corrective action before issues escalate.
In an ideal maintenance strategy, these two approaches work in tandem. Preventive maintenance lays the foundation for reliable operation, while predictive maintenance provides the specificity needed to address the unique challenges of real-world applications, where equipment may not always operate under optimal conditions. By combining both methods organizations can maximize equipment reliability, minimize downtime and ensure maintenance activities are precisely aligned with the demands of each application.
Jones: PM involves regular, scheduled maintenance activities to prevent equipment failures while PdM uses real-time data and advanced analytics to detect equipment issues and predict when a potential failure may occur. Some equipment degrades and fails on a predictable frequency (like oil degradation or clogged filters) and PM is ideal. However, a large percentage of equipment failures (~80% per industry analysts) still happen randomly. To catch these, PdM has the advantage. For the best maintenance strategies, you need to use both to realize the following benefits:
• Holistic coverage: Combining PdM and PM ensures that both unexpected and predictable issues are addressed. PdM can catch anomalies that PM might miss, while PM ensures routine checks and upkeep.
• Optimized resource allocation: PdM helps prioritize critical maintenance tasks based on realtime data, while PM ensures that all equipment receives regular attention.
Ruffin: Businesses are adopting condition-based maintenance techniques, integrating sensor technology, machine learning and failure trend analysis to improve asset longevity and operational efficiency. The use of load-indicating studs is an example of performing maintenance based on data. If the stud
shows a significant loss in load, then a leak is more likely to happen. Retightening following safe and recommended practices, is a maintenance activity that can prevent the need for reactive maintenance.
Question: Describe the successes from using programs that incorporate predictive, preventive, reliability-centered or reactive maintenance.
Jones: There is a time and place for each maintenance approach. Deciding which strategy to apply depends on the criticality, cost and impact of the equipment. For high-criticality and high-impact equipment, both preventive and PdM technologies should be applied. However, for redundant or lowcost equipment, reactive maintenance may be more appropriate.
To determine which equipment to focus on, implementing an RCM approach is essential. Successful maintenance strategies begin with identifying which assets to prioritize in the maintenance budget. Next, research how this type of equipment has failed in the past — whether predictably or randomly. Based on this analysis, apply the level of PdM that best manages these risks.
By following these steps organizations can ensure that their maintenance efforts are both efficient and effective, ultimately leading to improved reliability and reduced costs.
Nolan: PdM is generally based on expected life cycles of a component and PM is usually based on the compressor manufacturer's recommended maintenance schedule. Using both aspects of maintenance and adjusting a maintenance schedule to account for environmental conditions and duty cycles, you end up with a more reliable compressor system that will limit the need for reactive maintenance that can cost the user production.
Wilkerson: Organizations that successfully implement a maintenance program incorporating predictive, preventive, reliability-centered and reactive maintenance enjoy significant benefits in equipment uptime, operational efficiency and cost savings. Each approach plays a unique role, but together they create a robust framework for addressing maintenance challenges proactively and effectively.
PdM, often powered by advanced technologies like AI-driven vibration analysis, helps detect subtle changes in equipment performance, such as bearing wear, before they escalate into major issues.
Continued on pg. 10
‘ Easily
most unscheduled downtime is caused by lack of regular maintenance...
’
Continued from pg. 7
PM provides a structured, routine approach to maintaining equipment reliability based on manufacturer recommendations. Meanwhile, RCM shifts the focus beyond warranty compliance to prioritize long-term operational consistency by tailoring strategies to specific applications.
The role of reactive maintenance, while less desirable, cannot be eliminated entirely. However, with the other three components in place, the frequency and costs of reactive responses are significantly reduced. For example, having a clear plan for emergency scenarios — such as knowing which rental service to call at 2 a.m. when critical equipment fails — ensures swift recovery and minimizes downtime. Companies that integrate all four approaches experience far fewer unplanned outages and lower out-of-pocket expenses tied to urgent repairs. By reducing costly reactive maintenance, these organizations increase uptime, maintain productivity and achieve a lower total cost of ownership for their equipment. This comprehensive strategy not only protects operational continuity but also drives measurable improvements in efficiency and reliability.
Question: What are some of the key challenges for improving maintenance at your facility?
tial issues early, schedule downtime efficiently and maintain equipment within manufacturer specifications. It’s worth noting that even facilities running 24/7 can schedule and manage downtime effectively with proper planning, reducing the long-term risks of unexpected breakdowns and costly repairs.
Question: How does your team incorporate maintenance, repair and operations (MRO) seamlessly?
Wilkerson: Incorporating MRO seamlessly requires a holistic, team-centered approach where every stakeholder — regardless of role — contributes to the upkeep and reliability of equipment. At Kaishan USA, we’ve observed that the most successful teams foster a culture of collaboration and empowerment across all levels of the organization, from operators and engineers to plant management and even security personnel.
u
Maintenance insights
uArtificial intelligence (AI) and machine learning (ML) are playing a larger role in maintenance initiatives.
uReal-time monitoring and analytics can help predict equipment failures before they happen.
uPreparing for downtime can be a real challenge for many organizations.
Wilkerson: One of the key challenges in improving maintenance is scheduling downtime. Even in facilities that don’t run three shifts or operate around the clock, there’s often resistance to planned downtime. It stems from the perception that any halt in operations, even temporarily, disrupts productivity. This mindset frequently leads to delays in addressing maintenance needs, especially as equipment ages or personnel roles change.
The challenge is compounded by human nature’s tendency to favor reactive over preventive behavior. Much like avoiding a doctor’s visit until a problem arises, many facilities postpone maintenance until a failure occurs. This reactive approach often results in higher costs and longer disruptions compared to proactive strategies.
Overcoming this requires a cultural shift toward proactive planning and the integration of predictive, preventive and RCM practices. By embedding these practices into operations, facilities can identify poten-
A prime example is when security staff, who routinely pass by machinery during their shifts, are encouraged to report unusual sounds or changes. In one case, this proactive observation by a security guard triggered a chain of actions involving operators, maintenance personnel, engineering and the vendor. This synergy allowed the issue to be identified and resolved before it caused downtime or further damage — an outcome made possible because every individual felt empowered to act.
The key to seamless MRO integration lies in ensuring that responsibilities and communication channels are shared across departments. When everyone in the organization is engaged in the maintenance process and aligned toward the same goal of reliability, it minimizes silos and accelerates problem resolution. Ultimately, this collaborative approach reduces downtime, improves efficiency and fosters a proactive maintenance culture that benefits the entire operation.
Ruffin: Maintenance teams usually comprise 10% to 30% of a company’s workforce and their efficiency depends on access to reliable data, high-quality components and advanced diagnostic tools. However, for these resources to be effective, they must be coordinated through proper planning, scheduling and purchasing. Often, suppliers are left out of this planning process, resulting in delayed material deliveries or last-minute expedited orders. PE
John Glenski, Salas O’Brien, Cincinnati; and Tony Pica, Salas O’Brien, Pittsburgh
Learn how plant engineers can overcome the gap between artificial intelligence (AI) potential and practical execution in the workplace.
For plant engineers, the idea of leveraging artificial intelligence (AI) isn’t new. Conversations about predictive analytics, autonomous systems and digital twins have dominated industry discussions for years. But for many, the enthusiasm for AI’s potential has been met with equal parts frustration. Why? Because often the promise of AI feels disconnected from the realities of factory floors, where equipment, processes and people are intertwined in day-to-day operations.
• Identify practical applications of AI in plant operations, including maintenance, supply chain and customer service, to enhance efficiency and reduce downtime.
The gap between knowing what AI can do and making it work in real-world settings and sharing that with management can feel overwhelming. Plant engineers find themselves asking the same questions: How do we transition from reactive to predictive maintenance? Can digital twins truly optimize processes and prevent downtime? How do we ensure AI doesn’t overcomplicate supply chains or create more problems than it solves?
• Evaluate challenges and solutions for integrating AI tools into existing workflows to maximize benefits.
• Develop strategies to leverage AI for predictive insights, process optimization and talent acquisition, ensuring alignment with organizational goals.
These are not theoretical concerns — they’re practical ones. And they deserve practical answers. The focus is on how plant engineers can move beyond abstract concepts and buzzwords to actionable solutions, uncovering how AI can solve real-world challenges while enhancing efficiency and resilience.
For plant engineers, minimizing downtime is a goal — but achieving it requires more than just predictive maintenance. Enter AI-enabled digital twins, a simulation that transforms process optimization from a trial-and-error exercise into a virtual science. By creating a digital replica of physical systems, digital twins allow engineers to simulate scenarios, refine processes and predict outcomes without disrupting operations.
How it works:
• A digital twin is a virtual model of a physical system, from a single machine to an entire production line. The size of the simulation allows for great flexibility. Once you build a foundation, you can add on more as your budget allows and you can also maintain to simulate future scenarios.
• Powered by real-time data collected through internet of things (IoT) sensors, the twin simulates conditions, evaluates performance and identifies inefficiencies.
• Engineers can ask "what if" questions without costly experiments in the physical world.
For example, Salas O’Brien created a simulation model for a food and beverage client’s facility. The goal was to test planned process upgrades and identify gaps in existing planning. The simulation covers bulk liquid receiving, tank farm storage, blending, packaging and shipping. Using the model, the client visualized changes in real-time and obtained simulated data, changing the original proposed plan considering the data enabling successful and efficient upgrades.
Once in place, digital twins not only enhance process efficiency but also accelerate innovation.
• Complexity in model creation: Building a comprehensive digital twin requires extensive data, but starting with high-priority systems and scaling up gradually can mitigate this complexity. Pricing for development is typically based on the complexity of the scenarios.
• Integration with existing tools: Ensuring digital twins communicate seamlessly with ERP or MES systems is critical. Selecting open, interoperable platforms helps.
• Data accuracy: Regular calibration and realtime validation ensure the twin remains reliable.
For many plants, maintenance strategies still follow a reactive or scheduled approach. Equipment runs until it fails or maintenance occurs at predetermined intervals, regardless of the asset’s actual condition. While these methods are familiar and straightforward, they come with significant downsides: unplanned downtime, unnecessary costs and even safety risks.
Predictive maintenance, powered by AI, flips this paradigm by enabling a data-driven approach.
Instead of waiting for failure or relying on static schedules, AI models analyze sensor data, machine performance trends and historical patterns to predict when and why a failure is likely to occur.
• Sensors installed on critical equipment continuously monitor key parameters like vibration, temperature and pressure.
• AI algorithms process this data in real time, looking for patterns or anomalies that signal potential failure.
• AI delivers actionable insights via alerts and maintenance recommendations, allowing teams to address issues before they escalate.
Consider a pharmaceutical plant where unplanned downtime from a variable frequency device disrupts production schedules. By implementing predictive maintenance with AI, the plant can monitor motor wear and anomalies. When the AI system detects a deviation from the normal range, it triggers an alert, enabling the maintenance team to replace the motor during scheduled downtime, avoiding costly disruptions. This capability is available from all the major automation suppliers including Rockwell’s FactoryTalk Analytics GuardianAI, Siemens’ Senseye Predictive Maintenance
and the ABB Ability Genix Asset Performance Management Suite.
Overcoming barriers to adoption includes:
• Integration with legacy systems: Many plants rely on older equipment without built-in sensors. Retrofitting these machines with predicted devices can bridge the gap and unlock those capabilities.
‘For plant engineers, embracing AI in sourcing isn’t just about efficiency; it’s about maintaining a competitive edge. ’
• Cultural shift: Teams accustomed to traditional maintenance methods may hesitate to trust AI-driven insights. Education and pilot programs can demonstrate the technology’s value, fostering buy-in.
• Cost justification: While the upfront investment in AI and IoT sensors can be significant, the return on investment from reduced downtime, extended asset life and lower maintenance costs often outweighs the expense.
By embracing predictive maintenance, plant engineers can transition from firefighting to planned action. This shift doesn’t just save money — it empowers teams to plan confidently and focus on proactive improvements.
AI insights
uThis covers five key areas where AI can be implemented effectively: predictive maintenance, process optimization with digital twins, supply chain management, customer service and recruiting.
uThrough numerous examples and actionable insights, the article demonstrates how AI can improve efficiency, enhance decision-making and drive operational resilience, offering practical solutions to realworld challenges.
For plant engineers, sourcing and procurement is both a lifeline and a source of constant headaches. Material shortages, unpredictable lead times and fluctuating costs can disrupt production schedules and undermine operational efficiency. AI is emerging as a transformative force, offering plant engineers the tools to navigate these complexities with precision and agility.
How it works:
• AI is currently being used to “automate” the sourcing and negotiation process largely on frequently purchased commodities and commercially off the shelf products and services.
• This is a time saver for teams that have a large volume of commodity-based purchasing demand.
• The availability of market intel and pricing data allows the AI program to automatically negotiate or offer the optimized market price to competing suppliers with a high degree of accuracy and expediency.
As an example, a negotiated bulk fuel agreement was reached with a national diesel fuel provider to supply company tanks at a cost-plus price that provided immediate savings. Additionally, company fuel tanks were outfitted with technology that compared the fuel level in the tank with the current cost of market prices. Fuel was purchased either the same day or the next based on financial projections stemming from the petroleum markets. The technology at the tank was a transducer, which triggered the AI to analyze and predict immediate and future fuel price trends to optimize spend management in this category.
• Expect trial and error with the setup: The key criteria to achieving success in this process is to have clearly defined parameters, specifications and rules for the AI program to perform to expectations and deliver value add results through increased savings and cost avoidance.
• Complexity requires oversight: More complex engineered solutions require a greater degree of human oversight and engagement.
• Processes and procedures need to be managed: AI isn’t a “cure all.” It’s a tool that has to be managed. Consider it as an augmentation to your normal work with markets and suppliers.
For plant engineers, embracing AI in sourcing isn’t just about efficiency; it’s about maintaining a competitive edge.
While customer service might not seem like a traditional focus for plant engineers, its impact on overall operations is undeniable. Customer satisfaction drives repeat business and ensuring timely, accurate communication with customers is crucial. AI offers plant engineers tools to streamline customer interactions, resolve issues faster and align customer demands with operational capabilities.
How it works:
• AI systems like chatbots or virtual assistants use natural language processing to understand and respond to customer inquiries in real time.
• Machine learning analyzes customer data to forecast order trends, anticipate questions and recommend personalized solutions.
• AI integrates with ERP systems, connecting customer service platforms with plant operations to provide real-time updates on production schedules and inventory.
A packaging manufacturer frequently dealt with delays in responding to customer inquiries about order statuses. After implementing an AI-powered chatbot integrated with the enterprise resource planning (ERP) system, customers received instant updates on order progress, delivery timelines and options for expedited shipping. This improved satisfaction while allowing plant teams to focus on operations.
Overcoming barriers to adoption:
• Data accuracy: AI relies on accurate underlying data. Regular updates to ERP and customer relationship management systems ensure reliable responses.
• Customization needs: Generic AI solutions may not meet specific operational requirements. Customizable platforms align better with unique workflows.
• Customer trust: Some customers may distrust automated systems. Combining AI with access to human representatives balances efficiency with reassurance.
AI enhances, rather than replaces, customer service teams. By managing routine tasks, AI frees up human representatives for more complex inquiries, improving both efficiency and customer relationships.
Recruiting skilled talent is one of the toughest challenges for plant engineers. As the manufacturing sector evolves, it becomes challenging to find workers who possess the right blend of technical expertise and adaptability. AI-powered recruiting tools are reshaping how plants attract, assess and onboard top talent, making the hiring process faster, more accurate and less resource-intensive.
How it works:
• AI analyzes job descriptions to ensure they’re inclusive, clear and targeted to desired skills.
• AI systems scan resumes and applications, identifying top candidates based on criteria such as certifications, experience or relevant skills.
• AI tools automate candidate communication, scheduling interviews and responding to queries through chatbots or email automation.
In one case, a large equipment manufacturer struggled to fill maintenance technician roles. By implementing an AI-powered recruiting platform, the company automated resume screening, matched candidates with job openings based on skills and experience and broadened the talent pool. The result was a 30% reduction in time-tohire and better-qualified hires.
Overcoming barriers to adoption:
• Bias in algorithms: Historical data may introduce bias into AI systems. Regular audits and updates ensure fairness and inclusivity.
• Integration with current processes: Introducing AI into recruiting workflows may require training. Starting with pilot projects helps smooth the transition.
• Balancing automation with human touch: While AI handles administrative tasks, human involvement remains vital for evaluating cultural fit and building rapport.
AI speeds up hiring and identifies talent that might otherwise go unnoticed. For plant engineers, this means reducing downtime from understaffing and fostering innovation through diverse, qualified teams.
Getting AI from theoretical to practical
AI offers transformative potential for plant engineering, but its true value lies in practical execution. To bridge the gap between theory and real-world application, plant engineers should start small and focused. Identify specific AI projects that address well-defined challenges, such as reducing
downtime or improving supply chain visibility and implement them as controlled experiments within a single department or system.
For example, pilot an AI-driven predictive maintenance solution on a critical piece of equipment before scaling it plant-wide. Similarly, test an AI-powered recruiting platform with one job category or implement digital twins for one production line to refine processes.
This incremental approach minimizes risk, delivers quick wins and builds confidence in AI tools. As these smaller initiatives prove successful, they can be integrated into broader workflows, creating a foundation for enterprise-wide adoption.
By adopting this phased strategy, plants can unlock AI’s full potential while maintaining agility, competitiveness and readiness for the evolving demands of manufacturing. PE
John Glenski is Principal and Senior Director of Automation & Digital at Salas O’Brien. Tony Pica is Vice President of Procurement at Salas O’Brien.
AI AND MACHINE LEARNING
Sam Hoff, Patti Engineering, Auburn Hills, Michigan
Artificial intelligence-based tools provide remarkable insights leading to improvements in any domain that can be characterized with data.
DArduino's OPTA facilitates the retrieval of valuable diagnostics data from older control systems without requiring modification. Courtesy: Arduino
ata analytics can help organizations achieve remarkable results, and the data sets that fuel it are an integral part of this winning strategy. These artificial intelligence (AI)-based tools have become widely available to mathematically model, characterize and optimize nearly every part of a manufacturing operation.
Fueled by diverse data sets that describe the system, abundant in any manufacturing facility, the tools’ insights can show how to increase uptime, reduce costs and improve quality — all facets of overall equipment effectiveness (OEE). They do so by facilitating system diagnostics, predicting maintenance needs prior to equipment failure. While manufacturers commonly struggle with data accessibility and useability, investing in foundational steps to overcome this hurdle is essential for maintaining current and future competitiveness.
Machine learning, a subset of AI, employs vast data sets pooled from diverse parts of a system to train a model using sophisticated algorithms that mathematically describe its behavior. Once properly trained, the model can be used for insights in many different capacities, pulling together relationships among variables that may otherwise go unnoticed.
Data analytics is particularly well-suited to manufacturing because facilities already generate large amounts of data from various sources across the operation, including sensors, equipment performance metrics and operational efficiencies. When used effectively, machine learning algorithms can turn this data into actionable insights, leading to predictive maintenance, process optimization and better quality control.
A common vexing automation challenge faced by manufacturers involves prolonged downtime associated with unclear equipment failures. These incidents result in a cascade of stressful, reactive engineering and maintenance efforts and a significant loss of productivity.
Data analytics addresses this problem. By analyzing historical data, machine learning algorithms can identify the patterns that previously led to faults from past incidents. This capability not only speeds up diagnostics but also helps manufacturers transition from reactive to proactive maintenance, enabling them to predict issues and plan for their remediation prior to an expensive failure.
Consider a recent project Patti Engineering completed on behalf of a large industrial manufacturer that suffered a major press failure, halting production and ultimately costing the company more than $1 million in repairs. Had they known that the servo drive controlling the press was on the verge of failure, the issue could have been fixed for around $10,000.
Predictive maintenance strategies provide exactly this kind of foresight. Implementation involves adding sensors to the system, each providing ongo-
ing data describing the machine’s overall health. In the case of the press’s servo drive, vibration sensors (accelerometers) positioned close to the bearing housings of the servo motor may have measured changes in vibration possibly stemming from bearing wear. Likewise, a temperature sensor positioned near the motor’s windings may have measured higher operating temperatures indicative of increased winding resistivity or insulation breakdown. Overheating faults can also occur when the power electronics within a drive begin to fail, so a sensor monitoring its ambient operating temperature may have noticed it increasing.
In conjunction with other relevant system data sets (for example, power consumption), the sensors’ data points can be analyzed and modeled by various machine learning-based platforms providing manufacturers with insights into anomaly behavior well ahead of a dramatic failure like that of the press. Cloud-based analytics platforms from Amazon Web Services (AWS) or Microsoft Azure can be used for this purpose. As a result, industrial maintenance transitions to a streamlined proactive approach that minimizes downtime, reduces repair costs and maximizes equipment lifespan.
Patti Engineering’s approach to sensor integration is strategic with the goal of maximizing data accuracy while minimizing additional system complexity.
• It’s important to use the minimum number of sensors needed to characterize a system’s behavior, minimizing increases to the overall system’s complexity.
• Sensors should be integrated directly into the process, when possible, rather than positioning them in parallel. This approach often requires modifying the programmable logic controller program to route data to the selected cloud-based analytics platform. However, it ensures the collected data accurately reflects current operating conditions. In contrast, a sensor placed in parallel and not directly affecting production may fail without detection for extended periods, resulting in bad data and inaccurate models and insights.
‘By analyzing historical data, machine learning algorithms can identify the patterns that previously led to faults from past incidents.’
While the benefits of advanced analytics-based applications are clear, they require access to clean, ready-to-use data that can accurately describe and therefore model the target system. Within the manufacturing industry, the hurdles to achieving this data-readiness are multi-faceted. Disparate and siloed data sources using different standards, protocols and formats with potentially differing reporting rates and accuracies must be addressed.
This challenge is uniquely suited to system integrators as they possess comprehensive knowledge of diverse vendors’ equipment and platforms and are skilled in creating interoperable systems.
• Understand how data analytics can improve diagnostics, shifting manufacturers from reactive to predictive maintenance, minimizing downtime and repair costs.
• Learn effective strategies for integrating legacy systems without modifying them to retrieve valuable diagnostics data.
• Understand how system integrators are uniquely suited to resolve complex data integration challenges.
uData analytics can help organizations understand disparate data sets to form a cohesive view of manufacturing floor performance.
uMachine learning can help manufacturers predict when problems could arise, saving downtime and money.
uSensor placement plays a significant role in data analytics outcomes as collected data must accurately reflect operating conditions.
Another challenge to data integration within industrial manufacturing is the prevalence of legacy systems. Because they were not originally designed with current data needs in mind, the required data sets often do not natively exist within these systems. Compounding the problem, legacy systems are more prone to downtime events of unclear origin due to their age. Determining the cause of these events takes significant time, which takes a large toll on the manufacturer’s OEE.
To address this common problem, Patti Engineering partnered with Arduino. Arduino’s Opta facilitates the retrieval of valuable diagnostics data from older control systems without requiring their modification. Opta does what a legacy control system can’t do — collect, store, perform edge analysis and integrate data with modern aggregation platforms. It’s a tool that allows manufacturers to obtain better system diagnostics on legacy systems without immediately investing in a full controls upgrade.
AI-based simulation tools have the potential to deliver insights about a complex system’s operational efficiency that might otherwise be too difficult to discern. These tools not only facilitate root cause fault analysis but also foster predictive maintenance approaches that can prevent costly downtime. As a result, manufacturers that embrace AI-based tools will gain a competitive advantage by more effectively addressing a wide range of complex manufacturing challenges.
By relying on system integrators for implementing foundational data integration strategies, including careful sensor use and other technologies that seamlessly interface with legacy systems, manufacturers can employ a winning strategy — consistently outperforming expectations, improving productivity and thriving amid resource constraints. PE
Sam Hoff is the founder and chief executive officer of Patti Engineering.
From mobile robots and automated storage to picking, palletizing, and sortation systems, warehouse operators have a wide range of options to choose from. To get started or scale up with automation, end users need to evaluate their own processes and environments, find the best fit for their applications, and deploy and manage multiple systems.
Automated Warehouse Week will provide guidance, with expert insights into the evolving technologies, use cases, and business best practices.
Robotics Engineering Week features keynotes and panels, delivered by the leading minds in robotics and automation, addressing the most critical issues facing the commercial robotics developers of today. REGISTER TODAY roboweeks.com
Andrew Pether, Universal Robots, North Reading, Massachusetts
Physical artificial intelligence (AI) development, the use of AI and machine learning technologies with robotics will accelerate rapidly over the next few years. How can physical AI benefit end users and enhance existing applications already performed by cobots?
Industrial and manufacturing plants are entering an era of advanced, artificial intelligence (AI)-boosted robotics where robots can learn from and adapt to their surroundings in realtime. This opens new possibilities for complex collaborative tasks, transforming robots from tools into intelligent partners.
Physical AI development — the use of AI and machine learning technologies with robotics — will change the way people program robots. AI will handle the line-by-line programming of motions and inputs/outputs, allowing users to command higher-level robot behaviors to complete tasks.
cobot makers to merge the flexibility of AI with mature programming paradigms. The end-result is a range of solutions that can handle workspace variance and provide the speed and precision that industrial customers expect.
AI will largely eliminate the need for experts when deploying robots. We will still need robotics engineers, integrators and other skilled experts in the future, but there can’t be an expert on every factory floor. AI will remove some of the hurdles around robotics expertise, accelerating the introduction of robots in the process.
u
• Learn how physical AI enhances collaborative automation.
• Discover examples of physical AI in collaborative applications.
• Explore progress in the physical AI space.
Imitation/reinforcement learning, vision language action models and robotics foundation models are promising research areas for physical AI. This research will simplify how we control and interact with robots and create solutions to tasks that are currently difficult to achieve with robots, such as handling textiles and connecting flexible cables.
If you’re a manufacturer who has just begun a collaborative automation journey, it might be hard to see how AI can help near-term. But AI allows
Meanwhile, generative AI can help create standardized solutions. The challenges faced by the automation industry are similar in many companies. Generative AI allows industry to standardize both problems and solutions, creating more reusable robot behaviors. This will eliminate the need to reinvent the wheel every time a new robot is installed.
AI also boosts robots’ ability in unpredictable and dynamic environments. Vision technology with real-time feedback from 3D cameras is a huge enabler of autonomous navigation and obstacle detection. This creates possibilities for introducing robots outside of the structured environment of a factory floor. One example is in construction where robots must handle project variations while working side-by-side with workers.
FIGURE 1: Universal Robots launched the AI Accelerator. The toolkit is enabled by UR's next-generation software platform PolyScope X and is powered by NVIDIA Isaac accelerated libraries and AI models, running on the NVIDIA Jetson AGX Orin system-on-module. The toolkit also includes the Orbbec Gemini 335Lg 3D camera. Depicted here from the ROSCon exhibition 2024 is a demo of the AI Accelerator with a computer numerical control machine tending application using a UR5e. Courtesy: Universal Robots
Machine vision is widely deployed in manufacturing environments, primarily for locating and inspecting parts. However, fewer than 20% of today’s cobot applications use vision systems. That’s because most non-AI vision systems require expert configuration, making them complex and costly to program and maintain. As a result, most industrial users prefer to spend time and money securing their parts rather than benefiting from the increased flexibility that vision enables.
When a person sees a familiar object, they can still recognize it when it has a different surface finish, under different lighting conditions, against a different background or even when it’s a slightly different size and shape. This level of visual intelligence is hard for traditional vision systems that rely heavily on high contrast between background and object and repeatable size and shape of object to detect it.
Deep-learning vision systems allow us to train this kind of variability into a single model so it can
‘Vision technology with real-time feedback from 3D cameras is a huge enabler of autonomous navigation and obstacle detection.’
handle myriad environmental variations. It also means it’s no longer necessary for a user to obtain hundreds or thousands of images to train a model. A wide range of off-the-shelf, pre-trained models are available with permissive licensing that can be repurposed for a wide range of industrial tasks with only a short retraining process that typically requires just 50 images that can be automatically segmented and labelled.
NVIDIA's Isaac ROS and Isaac manipulator make it easy to bring advanced perception capabilities into existing robot programs. Use cases include:
uPhysical AI can remove barriers that currently exist to fully automating manufacturing processes.
uSoftware can program robots to automate processes.
u AI will enable robot manufacturers to build robots that are intuitive, user-friendly and adaptable to diverse production environments.
• Object detection: Used to locate and pick up objects in the robot workspace, reducing the need for rigid mechanical fixtures.
• Workspace check: An inspection capability used to find out “Is this thing in the workspace in the state that it should be for the robot to complete its task?” In a computer numerical control machine-tending application, for example, this capability ensures the work holding is clear and the tools in the machine are intact and clean.
‘It is not the robot's fault, but traditional automation is extremely reliant on things remaining exactly as they were when their program was created.’
• Workspace realignment: It makes sense to move robots around production environments to complete different tasks at different times. However, realigning to a workspace can be difficult because it requires precise placement of the robot and/or reteaching all the coordinate frames. A camera at the end of a robot arm automates this process so the robot can check where it is relative to the rest of the workspace and carry on with minimal fuss.
• Path planning: Plotting waypoints across the workspace to produce the optimal trajectory for a robot to get around and in and out of machines can be tricky, especially for end users new to automation. Automatic path planning makes this process much easier. But difficulties in providing the path planner with a detailed model of the robot environment is an obstacle to widespread adoption. AI can also help here, and new functionality is expected in 2025 to deal with this issue.
Complex warehouse requirements and dynamic environments can benefit from using 3D machine vision with AI to precisely identify, pick up and deliver pallets. The MiR1200 Pallet Jack solution, for example, is trained on more than 1.2 million real and synthetic images and combines data from four red, green, blue and depth (RGBD) cameras to enable fast and precise pallet handling.
The solution combines MiR’s autonomous mobile robot hardware with the NVIDIA Jetson platform. Jetson provides the advanced computing required to handle so much data in real time. By fusing feedback from RGBD cameras and 3D light detection and ranging, the robot can detect obstacles in 3D space for fully autonomous navigation.
Many times, when automated cells fail unexpectedly, the issues are caused by the world around the robot not being fixed in place well enough for the robot to do its job. It is not the robot's fault, but traditional automation is extremely reliant on things remaining exactly as they were when their program was created. AI enables greater flexibility and adaptability, creating an opportunity to make cobots more flexible and to prevent many issues from ever occurring.
Many businesses, particularly small- and medium-sized manufacturers, face barriers such as
‘Comparisons with the development of self-driving cars are useful, particularly the five stages that describe the transition from manual driving to fully autonomous driving.’
limited resources and technical expertise. AI allows robot manufacturers to build robots that are even more intuitive, user-friendly and adaptable to diverse production environments.
By prioritizing simplicity, reliability and real-world functionality, manufacturers can introduce AI without the steep learning curve or infrastructure overhaul often associated with new technologies.
Some cobot makers have barely incorporated AI into their offerings. But even companies that have begun providing AI capabilities still have a considerable way to go. Comparisons with the development of self-driving cars are useful, particularly the five stages that describe the transition from manual driving to fully autonomous driving.
Currently, self-driving car industry isn’t on level 5, (full driving automation) but there are a lot of level 2 (partial driving automation), 3 (conditional driving automation) or 4 (high driving automation) technologies in various stages of development, such as adaptive cruise control, which has turned a manual process into a semi-automated process, making driving smoother, easier and safer.
The same goes for industrial robots, including cobots. AI will one day lead to level 5 robots that can think and figure out how to solve problems by themselves without prior programming. That day has not arrived, but we are already seeing plenty of breakthroughs on levels 2, 3 and 4 that provide real value to businesses.
One example from logistics is a new solution that allows cobots to perform order picking autonomously. When compared to manual processes, this significantly enhances the speed and accuracy of order fulfilment in warehouses and logistics centers.
That example is not level 5, but it is an intermediate-stage technological innovation that is already delivering a lot of value to industry. PE
Andrew Pether is the innovation manager and perception team lead at Universal Robots.
RUBBER
Claudio Fayad, Emerson; and Steve Williams, AspenTech
The most effective artificial intelligence solutions will be those delivered as part of a fit-for-purpose, seamless automation system.
There can be little doubt that the world — and industrial manufacturing in particular — is in a state of rapid transformation and artificial intelligence (AI) is at the center of that change. AI has been embedded in select industrial manufacturing technology for years, helping power early automation and emerging data analytics solutions. However, technology has finally reached an inflection point.
Improvements in machine learning and cloud computing have been combined with technological advances in natural language processing, data
analytics and generative AI. These advances have dramatically increased the scope and scale of AI solutions, delivering technologies that are more powerful, personalized and efficient.
The possibilities are both broad and complex. AI-driven automation and predictive maintenance solutions are forming an increasingly powerful foundation upon which organizations can improve their processes and workflows to promote operational excellence. Simultaneously, AI is the driving force behind robust analytics tools that compare, combine and even coordinate production and market data to drive better financial choices and mitigate risk across an organization’s entire enterprise. Such capabilities are only a small sampling of the existing and emerging benefits of modern AI solutions (see Figure 1).
Ultimately, businesses will increasingly adopt new AI solutions to improve how they operate, whether those solutions are fit-for-purpose and standalone or embedded elements of existing automation solutions.
However, in both cases, doing so effectively will mean finding ways to navigate an increasingly broad and complex landscape of solutions, each with its own capabilities and requirements. Therefore, it is worthwhile to consider how AI technologies can be implemented as part of a seamlessly integrated holistic solution across operations and the enterprise.
The world has seen dramatic change in just the past two decades and the manufacturing industry has changed along with it. Technology advances, increasing mobility and globalization, geopoliti-
cal shifts and more have created a volatile, uncertain, complex and ambiguous environment that has increased competitive pressures. As demand, supply chains, workforces and other key enablers of efficient operations continue to evolve, manufacturing organizations are shifting their strategies to an approach focused on flexibility, monitoring demand and changing production as necessary to meet changing marketplace needs (see Figure 2).
Demographic change is creating significant challenges for the manufacturing industry. As an entire generation of expert personnel leaves the workforce, decades of institutional knowledge depart with them. Gone are the days of operators and technicians with decades of experience walking through the plant and identifying a worn bearing from an unusual sound or flagging a lubrication issue due to a strange smell.
As manufacturing organizations rise to meet the new challenges of a changing world and shifting demographics, they will increasingly rely on automation to develop pathways for increased performance and profitability. AI tools at the heart of these automation solutions will help companies unlock new production efficiencies to drive continual optimization — both of personnel and processes — helping them capture operational excellence and competitive advantage.
Increasing agility: As organizations cope with an increasing need to adapt to shifting business conditions and opportunities, they will leverage AI tools to increase their agility, both at the individual plant level and across the enterprise. Many plants will move away from the traditional model of producing one blockbuster product 24/7, opting instead for a more flexible approach focused on monitoring demand and changing production lines as necessary to meet those needs. Doing so — particularly with fewer experienced operators in the control room — will drive an increased reliance on automation as teams safely move production from state to state.
Tools like advanced process control, predictive maintenance, dynamic and state-based alarming and more will be essential to guide operators through transitions in their flexible environments. Supported with embedded AI, these tools will help teams lock in best practices and ensure safer and more efficient execution of their complex opera-
tions, regardless of who is in the control room. In addition, closed-loop planning and scheduling will tightly integrate with advanced process control and AI-driven forecasting tools to continually optimize operations across both plants and the enterprise.
In-context guidance: It can take years or even decades to bring operators and technicians up to expert levels. But when a plant experiences a complex operational state — such as a startup, shutdown or unexpected process deviation — safety and efficiency needs require workers who can make fast, wise decisions. To close the ever-increasing experience gap and enable personnel to quickly perform at a higher level, many organizations will look to advanced AI tools to provide expert guidance, assisting operators, technicians and engineers through complex decisions to drive the best results.
To meet this need, many automation suppliers are already employing process performance modeling with a network of online models to provide automated, 24x7 insights to propose critical adjustments to human operators for their review and implementation. Moreover, automation solutions providers are increasingly building intelligent virtual advisers into automation systems to help enhance decision making with natural language insights into closed-loop relationships, making it easier to maintain optimum process performance and safety.
Automation-enhanced productivity: The challenges of a shrinking pool of expertise are not limited to the plant floor but also associated with engineering. Those organizations retaining expert
‘AI tools at the heart of these automation solutions will help companies unlock new production efficiencies.’
• Explain how advancements in artificial intelligence (AI), including machine learning, cloud computing and natural language processing, are transforming industrial manufacturing.
• Identify how AI tools, such as predictive maintenance and advanced process control, enable operational efficiency and flexibility in manufacturing.
• Analyze the benefits of integrating AI into holistic automation systems to overcome challenges and drive innovation.
FIGURE 3: Industrial artificial intelligence tools drive value by increasing automation, improving agility and providing guidance to support and upskill personnel of all experience levels. Courtesy: Emerson and AspenTech
personnel are finding them quickly overtaxed, so they are looking for ways to help operators understand what actions are required and what the automation is doing at any given stage of the process. In addition, organizations are looking to help engineers work more efficiently and effectively using expert guided creation of models to quickly optimize plants both offline and online.
The organizations using AI most effectively have discovered that the most valuable tools are those deployed as an integrated part of their end-to-end automation solutions. Such solutions leverage deep industry expertise to help cut through the challenges of deploying and integrating AI into operational technology (OT) environments and to drive increased benefits and faster return on investment. The best AI tools are seamlessly integrated into software operations because operations teams already trust their automation software to help them deliver operational excellence.
That trust, in turn, leads to faster adoption and a seamless user experience — operators and technicians continue using the tools they know and trust, gaining AI enhancement without the need for additional knowledge or training. At the heart of this powerful, embedded-AI automation is an enterprise operations platform built on a boundless automation vision providing seamless data mobility from the intelligent field through the edge and into the cloud.
AI-ready automation will require a rich OT data ecosystem that not only drives copious amounts of data to and from any automation technology across the enterprise, but does so while preserving rich context, broad and secure connectivity and layered analytics.
‘AI will unlock unimagined
flexibility, safety, sustainability and performance.
’
AI-enabled adaptive process control can help simplify process transitions in both routine and emergency situations, while helping operators better understand exactly what is happening. In addition, engineering tools with embedded machine learning help engineers develop more accurate and sustainable advanced process control models. Leveraging embedded AI, users can more quickly manage configuration, monitoring and optimization.
AI tools are an increasingly critical element of a successful industrial manufacturing business strategy in a changing marketplace. However, as teams start implementing a wide array of disparate AI tools from a variety of suppliers, they often quickly discover challenges to effective deployment. To get the most out of any AI solution, organizations should seek out technological offerings that deliver AI tools as part of a holistic automation package.
If data is trapped in various silos across the enterprise or is delivered without the rich context necessary to turn large data into smart data, AI tools will struggle to generate trustworthy, actionable insights. An enterprise operations platform designed as part of a boundless automation vision delivers the data mobility — with rich context — necessary to power embedded AI tools. Built on an OT data ecosystem, seamlessly integrated enterprise operations platforms help overcome data silos by allowing contextualized data to move freely without the need for complex, custom engineering between solutions.
Yet, even among embedded AI solutions, not just any software will provide the game-changing results industrial manufacturers require to compete in a more complex marketplace. Purpose-built industrial AI solutions leverage engineering fundamentals, deep asset knowledge and industry experience to drive better experiences and results. The most powerful tools combine the speed and flexi-
bility of a data-driven approach with first principles models to provide guardrails, robustness and trusted results.
Under such practical constraints, options that are not practically possible in the plant are prevented. Hybrid models can run simulations thousands of times, but still ensure key constraints are obeyed. Such a solution helps reduce the time users spend validating because models are constrained by reality.
In fact, one of the key differentiators of fit-forpurpose industrial AI is that the tools are already trusted by users to help them work more effectively on their highest-value tasks. The most advanced industrial AI tools offer a robustness that no generic AI solution can match because they have been embedded in key automation tools for years — generating design examples in engineering, enhancing models in digital twin simulation software, tracking equipment performance and predicting breakdowns as part of predictive maintenance solutions and more (see Figure 3).
When combined by design, automation tools and embedded industrial AI naturally deliver a holistic solution by enhancing automation of business processes while providing seamless integration of AI automation infrastructure. Such solutions deliver end-to-end functionality that provides value not just in an individual stage, but across the entire technology life cycle.
For example, in the earliest stages, instead of requiring users to drag and drop control elements or to prepare spreadsheets to be imported to engineering automation systems, AI tools are gradually gaining the capacity to use natural language to present configurations that users can approve or modify. Later, during operations, AI guidance can help users combine real-time data, historical data — and unstructured information like documents, control narratives and more — to help operators understand what is happening in the plant.
AI tools can easily monitor hundreds of displays simultaneously and then use natural language to help operators understand what is going on behind the scenes of the automation — such as when an advanced process control algorithm automatically changes states or setpoints for a reason that is not immediately clear. And even at end of life, embedded AI technologies can help convert
legacy code to new control system logic to simplify and streamline modernization (see Figure 4).
As they increase in power and capability, AI tools will fundamentally redefine the way industrial manufacturers operate. AI will unlock unimagined flexibility, safety, sustainability and performance, helping organizations navigate the market and demographic challenges that are barriers to operational excellence.
The same industrial AI tools that have been embedded in automation solutions for years will be a key part of those solutions, evolving and scaling alongside key automation tools to provide users with an intuitive, trustworthy way to continue leveraging AI for increased efficiency and decision support. It will be tightly integrated technologies, delivered as part of an organization’s seamless and comprehensive enterprise operations platform that will truly unlock a step-change in operation.
The foundation for such a technological leap is already available today and it will continue to improve and scale in the coming years. Now is the time to get on board and capture the innovation capabilities that will drive competitive advantage for years to come. PE
FIGURE 4: Modern artificial intelligence tools can automate configuration, coding and testing to drive faster modernization. Courtesy: Emerson and AspenTech
Claudio Fayad is Vice President of Technology of Emerson’s Process Systems and Solutions business.
Steve Williams is Vice President of Product Management, Portfolio Product Strategy at AspenTech.
AI insights
uArtificial intelligence (AI)-driven tools like predictive maintenance and advanced process control are transforming operational efficiency in industrial manufacturing. uIntegration of AI with automation systems addresses challenges, enhances flexibility and drives innovation across the industry.
SAFETY AND PPE
Herbert Post, Tradesafe, Las Vegas
While automation enhances productivity and safety, it also introduces unique hazards that must be effectively managed.
Automation enhances productivity and safety, but it also introduces unique hazards that must be effectively managed. One such example occurred in June 2016 when a 20-year-old machine operator at a manufacturing facility lost her life. A robot unexpectedly reactivated while she and her colleagues were clearing a sensor fault. This tragic incident, resulting in more than $1.3 million in penalties and criminal charges, starkly illustrates the potentially devastating consequences of inadequate safety protocols in automated manufacturing environments.
• Identify the specific hazards associated with automated manufacturing systems.
• Analyze the causes and potential impacts of these hazards on operations and personnel.
• Implement effective mitigation strategies and safety measures to minimize risks in automated environments.
Automated manufacturing systems are computer-controlled machines, equipment and processes that perform manufacturing operations with minimal human intervention. These systems integrate various technologies including robotics, programmable logic controllers (PLCs), sensors and computer-aided manufacturing software to execute precise, repetitive tasks. Automated manufacturing systems handle operations such as assembly, material handling, welding, painting and quality inspection, enabling manufacturers to achieve consistent quality and higher production rates.
These systems offer numerous benefits including increased productivity, improved product quality, reduced labor costs and enhanced workplace ergonomics by relieving workers from repetitive or physically demanding tasks. Additionally,
modern automated systems can collect and analyze production data in real-time, allowing for predictive maintenance and continuous process optimization.
Despite these advantages, the complexity of automated manufacturing systems, combined with the high-speed operation of powerful machinery and the necessity for human-machine interaction, creates unique safety challenges that can result in serious injuries or fatalities when not properly addressed. As demonstrated by incidents like the one mentioned earlier, a simple system malfunction can have devastating consequences, making it critical to know the hazards in these systems and how to mitigate them.
While there could be many safety challenges in automated manufacturing environments, especially those specific to different industries, we summed up the major ones with examples of how they could occur (see Figure 1).
Impact, collision or other struck-by or caught-between hazards: These hazards occur when workers are hit by moving machine parts, ejected materials or caught between a moving and a fixed part of automated equipment. In robotic assembly lines, for instance, a worker performing maintenance might be struck by an unexpected robotic arm movement if the system's safety interlocks fail or proper lockout/tagout procedures aren't followed. Similarly, automated guided vehicles in material handling operations can collide with workers if their proximity sensors malfunction or if designated safety zones are not properly established and maintained.
Crushing and trapping hazards: Crushing and trapping hazards arise when body parts or entire bodies are caught and compressed between moving machine components or between machines and fixed structures. A prime example is seen in automated palletizing systems, where workers can be crushed if they enter the robot's operational space during a malfunction clearance without properly disabling the system. The incident mentioned earlier about the 20-year-old machine operator tragically illustrates this hazard, where a worker was fatally crushed inside a robotic cell when the system unexpectedly reactivated during fault clearing.
Electrical hazards: Electrical hazards involve risks of shock, electrocution or burns from exposed electrical components or system malfunctions. These hazards commonly arise during the maintenance of automated systems when workers need to access control panels or when troubleshooting electrical components. For example, a technician working on a PLC cabinet might be exposed to live electrical contacts if proper isolation procedures aren't followed or damaged insulation on robot power cables could create shock risks during routine operations.
Slipping, tripping and falling hazards: These hazards involve risks associated with walking surfaces, workspace organization and elevation
Courtesy: Tradesafe
changes in automated manufacturing environments. Within automated facilities, workers can trip over exposed cables, slip on lubricant leaks from automated machinery or fall from elevated platforms while accessing automated storage and retrieval systems. For instance, an operator might slip on hydraulic fluid leaked from an automated press or trip over sensor cables that weren't properly secured during system installation or maintenance.
Cybersecurity hazards: Cybersecurity hazards represent risks associated with unauthorized access, control or manipulation of automated manufacturing systems through digital means. Modern automated systems are increasingly connected to networks for monitoring and control, making them vulnerable to cyberattacks. For example, Trend Micro found out in its survey that 61% of smart factories across the United States, Germany and Japan have experienced cyber incidents, with most of them experiencing system outages as a result. Almost half of these manufacturers said outages lasted more than four days.
‘Despite these advantages, the complexity of automated manufacturing systems... creates unique safety challenges that can result in serious injuries or fatalities when not properly addressed.’
Control errors
Environmental sources
Human errors
Improper assembly and installation
Mechanical failures
Power system failures or malfunctions
Time pressure
Unauthorized access
TABLE 1: A table summarizing the common causes of hazards in automated environments. Courtesy: Tradesafe
Injured when safety systems fail to detect their presence or automated equipment moves unexpectedly
Health issues or face increased accident risks
Injuries or fatalities when systems behave unpredictably
Risks from improperly secured components, inadequate guarding or compromised safety systems
Struck by broken components, caught in failed mechanisms or exposed to hazardous materials
Injured during sudden system shutdowns or when equipment unexpectedly restarts
Injuries when they take shortcuts or bypass safety protocols
Serious injuries when they encounter hazards they're not prepared to handle or recognize
These major hazards can be caused by different factors. The Occupational Safety and Health Administration listed the common causes or sources of hazards in an automated environment. Table 1 shows the major cybersecurity hazard causes and their impact on personnel and operations.
Now that we have identified the major hazards in automated manufacturing environments and their major causes, here are comprehensive strategies for risk mitigation with practical examples of how to implement them.
Implement the hierarchy of controls:
The hierarchy of controls (see Figure 2) is a widely known systematic approach to managing workplace hazards, including hazards in automated environments, by implementing control measures in order of effectiveness, from most to least effective.
• Elimination: Physically remove the hazard from the workplace.
• Substitution: Replace hazardous processes or materials with less-hazardous ones.
Production stoppages, quality issues and extensive troubleshooting
Premature equipment wear, sensor failures and quality control issues
Costly downtime and potential equipment damage
Chronic operational issues, increased maintenance requirements and potential system failures
Extended shutdowns for repairs and potentially damaging other systems
Damage sensitive electronic components, corrupt system programming and require extensive system revalidation
Production quality suffers and increased accident rates and regulatory interventions
Equipment damage, process disruptions and compromised quality control systems
• Engineering controls: Install physical barriers, machine guards or safety devices to isolate people from hazards.
• Administrative controls: Change how people work through procedures, training and warning signs.
• Personal protective equipment (PPE): Provide workers with equipment to protect against hazards.
For example, in an automated welding cell, elimination might involve redesigning the process to use mechanical joining instead of welding where possible. Engineering controls include installing light curtains and physical barriers. Administrative controls include implementing standard operating procedures. PPE includes providing welding masks and fire-resistant clothing.
Establish competency-based training program: A competency-based training program ensures workers have the knowledge and skills needed to safely operate and maintain automated systems through structured learning and assessment. This approach focuses on measurable outcomes and practical demonstration of skills rather than just completion of training hours.
For instance, a robotics maintenance technician must complete theoretical training on robot operation, demonstrate proper lockout/tagout procedures and pass both written and practical assessments before being certified to work on robotic systems independently. Regular refresher training and competency checks ensure skills remain current.
Execute preventive maintenance strategy: A preventive maintenance strategy involves scheduled inspections, testing and maintenance of automated equipment based on manufacturer recommendations and operational experience. This proactive approach helps identify and address potential issues before they lead to failures or safety incidents.
Implementing a computerized maintenance management system might, for example, schedule regular checks of safety interlocks, emergency stops and critical components of robotic cells, with maintenance tasks tracked and documented electronically.
Develop and maintain documentation systems: Documentation systems provide structured methods for creating, updating and accessing critical safety information, procedures and records. These systems ensure consistent application of safe-
‘Administrative controls include implementing standard operating procedures. PPE includes providing welding masks and fire-resistant clothing.’
ty practices and provide traceable evidence of safety compliance.
In this case, creating a digital documentation platform that houses all standard operating procedures, risk assessments, maintenance records and incident reports, with version control and regular review cycles to keep information current.
Implement emergency response protocols: Emergency response protocols establish predetermined procedures for handling various types of incidents or accidents in automated manufacturing environments. These protocols ensure quick and coordinated responses to emergencies to minimize injury and damage.
For example, developing and regularly practicing procedures for different emergency scenarios, such as robot malfunctions or worker entrapment,
Automated manufacturing systems insights
uAutomated manufacturing systems can enhance a facility’s safety protocols.
uWorkers and automated systems, including robots, create unique safety hazards. Potential risks include mechanical failures, software glitches and human-machine interaction issues that can lead to accidents or production downtime.
uGiven these new hazards, manufacturers must develop protocols to protect workers.
including clear roles for emergency response teams and evacuation procedures.
Conduct regular safety assessments: Regular safety assessments involve systematic evaluations of workplace hazards, control measures and safety performance indicators. These assessments help identify gaps in safety systems and opportunities for improvement.
For instance, managers can conduct monthly safety walks with a cross-functional team to inspect automated equipment, observe work practices, review incident reports and assess the effectiveness of existing safety measures, with findings documented and tracked to completion (see Figure 3).
As manufacturing automation continues to evolve with artificial intelligence, collaborative robots and interconnected systems, the approach
to safety must evolve in parallel. Technologies like predictive analytics, digital twins and augmented reality are already transforming how we identify and mitigate hazards. Manufacturers who embrace these automation systems while maintaining rigorous safety protocols will be best positioned to prevent accidents, protect workers and optimize operations.
The true measure of success in automated manufacturing safety is creating a culture where continuous improvement in safety practices becomes as ingrained as the manufacturing processes themselves. As the tragic 2016 fatality reminds us, the cost of complacency in automated environments can be devastating. The future belongs to organizations that view safety not as a constraint on automation but as an essential driver of innovation. PE
Herbert Post is vice president of Tradesafe.
PREDICTIVE AND PREVENTIVE MAINTENANCE
Eric Whitley, L2L, Brigham City, Utah
Manufacturers can mitigate unplanned downtime by adhering to robust predictive and preventive maintenance processes, supplemented by the right data-backed software and artificial intelligence.
Keeping your equipment up and running safely is no small feat, especially considering the costs associated with maintenance and downtime. Unplanned downtime costs manufacturers an estimated $50 billion every year, which means the right approach to maintenance can make or break a business.
But waiting for total equipment failure and fixing it with reactive maintenance isn’t a sustainable solution. This can lead to missed quotas during key manufacturing cycles, shortened lifespans of critical equipment and overworked maintenance technicians.
• Gain a deeper understanding of preventive maintenance and the benefits it poses.
• Learn how to leverage data — especially through the use of artificial intelligence (AI) — to streamline and improve both predictive and preventive maintenance.
To stay one step ahead of potential equipment failure, manufacturers should implement a combination of predictive and preventive maintenance. And as artificial intelligence (AI)-powered technology becomes more widely accessible, it’s enhancing maintenance processes across the board.
Predictive maintenance helps manufacturers stay ahead of necessary repairs by monitoring assets and component conditions in real time. With system-generated data triggering repair notifications, teams can jump in to perform maintenance once sensors, programmable logic controllers and other applications notify them that a part of their equipment is failing or about to fail.
In other words, it’s a smart, AI-based way to tell when your equipment needs repairs.
Predictive maintenance happens in three key phases.
1. Inspection: These are processes you go through on a regular basis to monitor the condition of your machine’s components. Frequent inspections may include vibration, temperature and oil analyses. Another approach involves real-time monitoring of things like pressures, flow rates and temperatures via measuring devices attached to the equipment itself.
2. Direction: New predictive maintenance platforms are able to use real-time data analysis to give organizations quicker decision-making capabilities. Once you’ve accumulated enough data from your regular inspections, it’s time to decipher what that information is telling you. What are the failure criteria for this equipment? Establish parameters based on past performance history, manufacturer recommendations and thorough failure mode and effects analysis to build your inspection criteria.
3. Corrective actions: Define a maintenance plan for this equipment based on your data analysis and your set parameters. Adhere to the plan and complete the maintenance needed during strategically planned downtime. AI-powered software can assist here by analyzing this data and making proactive recommendations for corrective actions.
Predictive maintenance offers a wide range of benefits to an organization. First, it cuts down on costs by prolonging the life of your assets and reducing the need for spare parts storage. Instead of cluttering your warehouse with extra components, predictive maintenance inspections allow you to order parts as needed when potential problems are detected. Furthermore, when inspections are performed during normal runtime or equipment is monitored in real time, you’ll save on labor costs associated with production downtime.
‘Complex systems, large-scale machinery and safety-critical equipment would be better suited for a predictive maintenance program.’
Predictive maintenance works best when installed in your most critical, high-value machines. Consider every piece of equipment you have onsite: If this specific piece of equipment fails, how much do you stand to lose when it’s taken out of commission?
Installing predictive maintenance software won’t provide much value if the asset it’s monitoring isn’t suitable. For example, say you’re keeping tabs on a large fan in a curing unit. It’s a comparatively cheap piece of equipment performing a relatively low-value task — which means the cost of predictive maintenance might not be practical.
On the other hand, complex systems, large-scale machinery and safety-critical equipment would be better suited for a predictive maintenance program. For other assets, you’ll want to consider implementing preventive maintenance.
Preventive maintenance is all about proactivity. This strategy relies on regular, scheduled maintenance efforts to prevent unexpected failures and breakdowns. These systems are quite common today due to their low cost and ease of imple-
mentation, but many facilities desperately need to streamline their approach with the help of digital technology.
Preventive maintenance is especially crucial for industries that rely on complex and expensive machinery like manufacturing. In practice, it involves adhering to a systematic schedule based on usage metrics or a predetermined timeline. Common applications include:
• Lubrication
• Cleaning
• Inspections
• Adjustments
• Part replacements
• Calibration
• Testing
• Software updates
• Electrical system checks
• Safety checks
There are two main types of preventive maintenance, both of which require thoughtful planning and detailed documentation of maintenance history to ensure future success. Most manufactur-
Maintenance insights
uLet’s explore what predictive and preventive maintenance approaches entail, the equipment best suited for each and how optimizing them via AI-powered connected workforce technology can maximize factory uptime. uThis piece defines predictive and preventive maintenance and explores how manufacturers should be leveraging AI-powered tools to improve these processes.
FIGURE 2: The fivephase workflow for preventive maintenance involves choosing the asset and picking a usage-based or time-based interval and deciding when to repair based on regular inspections.
Courtesy: L2L
ers will use a combination of the two based on the needs and operating conditions of different assets.
• Cycle-based preventive maintenance: This approach is dictated by how many production cycles an asset has gone through. It serves machines based on how much they’re used rather than following a time-bound schedule. By tracking metrics like products made or hours active, teams can prevent excessive wear and tear, reduce excessive maintenance and optimize resource allocation. An AI-powered connected workforce platform can track production and maintenance data automatically to make this process seamless within one system. Examples include scheduling a robotic arm for maintenance after it completes 80,000 cycles or checking packaging equipment for wear after it has sealed 50,000 boxes.
• Calendar-based preventive maintenance: This approach is determined by standard calendar dates. Repairs begin after a set amount of time
‘Implementing preventive maintenance software can help keep track of what needs to be done and execute standardized procedures.’
has passed, whether that’s daily, weekly, monthly or yearly. The exact interval will depend on the tool in question, how frequently it’s used, its operating conditions and existing regulations from governing bodies. For example, safety equipment like fire extinguishers and emergency lights need to be checked and tested every six months to comply with safety regulations. Meanwhile, heating, ventilation and air conditioning systems may need to be serviced annually in preparation for their peak usage seasons.
To get the most out of preventive maintenance, each facility needs a strategic approach. Identify and prioritize assets based on their production impact — focus on the equipment that would cause the biggest operational disruptions or safety hazards. The assets best suited for preventive maintenance will be those that are critical to operations and monitored for regulatory compliance. Assets with high repair or replacement costs and predictable wear and tear are suitable candidates as well.
Once you’ve settled on which equipment qualifies, create a plan complete with tailored schedules, procedures and checklists for each asset. Implementing preventive maintenance software can help keep track of what needs to be done and execute standardized procedures. Your maintenance teams need to be adequately trained on and equipped with the right tools and parts to complete the tasks you assign them. Introducing the proper software is one such method to ensure they’re set up for success and it’ll allow for a deeper analysis of the information and data at hand.
Data is the best defense we have against unplanned downtime and repair costs. Knowing exactly what, when and how to perform maintenance on your equipment is vital — but keeping track of all this information without the assistance of software is nearly impossible in today’s digital-first environment.
AI-powered connected workforce platforms with predictive and preventive maintenance software baked in have emerged as a solution. Such platforms empower skilled trades with the information they need to act efficiently and effectively by giving them the ability to plan, schedule, run and track maintenance activities through a centralized system. By notifying technicians when a piece of equip-
ment needs to be inspected, this technology helps manual processes and documentation, leaders can implement transparent and efficient maintenance procedures for every piece of equipment in operation.
Types of assests suited for preventive maintenance other isolated equipment. Like fall protection requirements, If any damage is spotted, employees should tag it, remove the PPE from service, and report it to management.
Assets better suited for preventive maintenance include those with high repair and replacement costs and those that need to be regularly monitored for damage. Courtesy: L2L
Training is another crucial factor. Ensure the team follows a detailed and regular training schedule to educate on the hazards associated with arc flash and how to properly use the equipment. This is a good place to revisit the equipment selection criteria, especially after there has been any upgrades or changes to the electrical system.
Lastly, fostering a company culture towards safety can greatly improve employee safety. Each person is their own safety critic and having safe operations top of mind is going to mitigate the risk of serious injury or death on any job site. PE
Reactive maintenance will always be necessary to some extent, but manufacturers can cut down on their need to play catch-up by investing in tools that enable a reliable blend of predictive and pre-
Eric Whitley is the Director of Industrial Transformation at L2L.
Jarred Richter is an electrical technologist at Hedgehog Technologies, a CFE Media and Technology content partner.
Cody P. Bann and David Nolan, SmartSights, Austin, Texas
Advanced software technology ensures critical infrastructures remain resilient against cyberattacks and enables real-time collaboration between operators, engineers and technicians who resolve process issues.
The need for strong cybersecurity software to identify and prevent attacks is paramount. Several sectors are particularly vulnerable to these threats and have been identified by the United States government as critical infrastructures (CIs). Those include:
• Transportation
• Oil and gas production and storage
• Water supply
• Emergency services
• Government services
• Banking and finance
• Electrical power
• Telecommunications
• Chemical
• Commercial facilities
• Manufacturing
• Defense
• Understand how capturing data from machine assets helps continuous operational improvement.
• Learn the importance of real-time data.
• Discover how organizations can manage critical infrastructure with a thoughtful approach to remote access that exposes alerts and insights in context rather than open access.
These threats present unique challenges.
Critical infrastructure faced a 30% increase in cyberattacks in just one year, showing how outdated frameworks that support vital sectors can affect the essential sectors we rely on, such as energy, water, transportation, healthcare and finance, according to a KnowBe4 report. Moreover, according to a recent Kyndryl Readiness report, 44% of critical IT infrastructure is approaching end-of-life. This means almost half of the world’s most critical infrastructure
is more vulnerable to cyberattacks and at higher risk for prolonged outages.
None of these CI sectors are immune to cyberattacks and downtime. Recently, a cyber incident at the Port of Seattle and Seattle-Tacoma International Airport highlighted the ongoing vulnerabilities and how critical infrastructure disruptions or outages can impact crucial avenues for travel, logistics and even power. However, embracing cyber resiliency allows organizations to ensure that critical systems can continue to function during and after a cyber event.
Responding quickly to these incidents can minimize damage and restore operations. Different sectors of CI face unique challenges when it comes to cyber resilience, but all share a common need for stronger, more adaptive defenses.
One component of resiliency is embracing and integrating advanced software technologies.
The modern industrial environment requires addition¬al advanced technology to improve operational efficiencies, including process optimization, real-time analytics and automation. Integrating these is paramount for maintaining a competitive edge in an increasingly globalized market.
However, with this escalating need comes a rise in the risks of plant downtime and cyberattacks, which can significantly hamper competitiveness and productivity and negate the cost savings of automation. Continuous operational improvement starts with capturing data from machine assets. This data provides immediate insights for both people and sy stems, enabling them to make better, faster decisions and drive automation.
Once real-time process information is gathered, the next step is to define conditions of concern on those process variables. Supervisory control and data acquisition (SCADA) systems provide for such conditions to be defined and tracked, monitoring pro-
cess variables and surfacing active conditions to human-machine interfaces. These condition-based events and alarms add another level to optimizing the process. They facilitate greater situational awareness for plant operators by calling attention to irregularities and suboptimalities. Real-time process variables and alarm conditions can then be captured over time and calculations can be performed to improve planning and offer solutions to detect patterns in the data.
Real-time data is also critical to providing IT teams with insights about the security of a network or related topics, promptly acting on them and mitigate threats sooner.
A key theme of Open Platform Communications Unified Architecture (OPC UA), industry 4.0 and smart operations is greater connectivity — increasing connectivity between devices, industrial networks and physical assets and the cloud.
Growing connectivity allows for greater process transparency and the added potential for predictive analytics and sentinel alarm conditions. Smart plants allow potential issues to be addressed before they become problems, but only by extending that connectivity to the final hop — to the people who operate and optimize assets. Connecting devices to people and getting the right information with
increased sophistication of modeling to the right people at the right time is the strength of remote alarm notification software. Remote monitoring and alarm management enables operators to take on more proactive, hands-on tasks in the field or at the plant, without hiring additional staff.
Smart technology and OPC UA are reshaping the deployment of remote alarm notification software solutions and their connectivity. As assets and processes become more populated with smarter sensors and are increasingly modeled at lower operational levels, the ability of remote alarm notification software solutions to connect to both SCADA and non-SCADA information sources is increasingly important.
Advanced notification workflows will call for the synthesis of information from multiple OPC UA servers in response to unfolding alarm events in SCADA. It’s nice to know that a pressure sensor is registering a low alarm; it’s better to know that information in the context of the status of the valves and pumps associated with that pressure, the maintenance information for that asset and the downstream impact of the low pressure.
Increased connectivity and information modeling will empower decision-makers, enable faster response times and drive increased productivity. Smart technology ready alarm notification software solutions connect to both the leading SCADA packages and to OPC UA servers and provide for such advanced notification workflows.
FIGURE 1: Not only can OPC UA be used to relay the real-time values like sensor readings to SCADA HMI screens across vendors; it can also relay alarm conditions that SCADA builds on top of those variables, so that dangerous or costly deviations can be quickly identified and addressed.
Courtesy: SmartSights
FIGURE 2: OPC UA helps water utilities meet the challenge of secured exchange of structured data between devices, equipment and services. OPC UA is also designed to share that data upwards from the OT network to IT networks and to the cloud beyond. Courtesy: SmartSights
The Environmental Protection Agency (EPA) rescinded its previous mandate that required states to expand inspections of water systems to include cybersecurity threats. It encourages states to still review cybersecurity practices for public water systems on a voluntary basis under the sanitary survey or use another equivalent process.
The EPA regulation states that water utilities can conduct a self-assessment, or have it done by an approved third-party resource such as a Cybersecurity and Infrastructure Security Agency cybersecurity adviser through the EPA’s water sector cybersecurity evaluation program or a state-approved private sector technical assistance provider. Currently, the only approved third-party assessment resources are limited to the Department of Homeland Security, EPA and states, which makes this activity hard to scale across the breadth of water utilities across the country.
Cyberattack insights
uQuick response times are critical to recovering from cyberattacks.
uSmart technology and certain standards are helping reshape the response protocol to cyber threats.
uCritical infrastructures (Cis) are becoming more vulnerable each year to cybersecurity attacks.
The goal of sanitary surveys is to ensure that states effectively identify significant deficiencies and that public water systems (PWS) then correct those deficiencies — including cybersecurity-related weaknesses — that could impact safe drinking water. To help with this, the EPA is offering considerable technical assistance and support to states as well as to PWS to help close cybersecurity gaps.
While accurate, real-time data is pivotal to operations, harnessing this data effectively requires advanced technology and analytical capabilities. Vast amounts of data are collected for industry reporting, predictive maintenance and safety enhancements,
for example, but organizations may be challenged to effectively manage and analyze the data. While monitoring and alarms can improve system efficiency, they don’t automate the labor-intensive reporting process or provide much-need analytics that extract raw or summary values over a discrete period.
Automated third-party reporting software, however, tracks all areas in a production facility. The finished reports are then distributed directly to preferred destinations, which streamlines the decision-making process and enhances operational efficiency. The ability to harness this data effectively can lead to smarter decision-making, improved processes and a competitive edge. Analyzing historical data allows operations management to identify patterns, trends and anomalies that may otherwise go unnoticed. Historical data analytics can help companies transition from reactive to proactive planning and keep planning aligned with operations. As the data is collected it is summarized as key metrics and the final output is published into a formatted document accepted by regulatory agencies, such as for an EPA surface water treatment compliance report.
A robust alarm management system enhances operational efficiency and enables timely detection of failures. A comprehensive alarm system provides actionable information to the operator and assists in taking corrective action. Research has shown that a well-managed alarm system results in production
efficiency, improved product quality and better operator effectiveness.
Since 1991, alarm management standards have been published and currently the International Society of Automation 18.2 and International Electrotechnical Commission 62682 are the most widely accepted. The standards define a seven-step alarm management cycle program from identification to monitoring and assessment.
A cloud-connected alarm management system provides access to real-time alarms even when working remotely to decrease response times and help reduce unplanned downtime. Alarm audits and reporting provide an efficient means to document and track the history of individual alarms, consequences, response time and the action taken to mitigate the alarms. As this rationalization is performed, continued system-level monitoring and assessment reports validate that these efforts are driving real improvement.
Alarm management is a critical aspect of industrial operations, particularly in high-risk industries such as oil and gas, chemical processing and power generation. The ability to effectively manage alarms can prevent accidents, minimize downtime and improve overall operational efficiency.
A challenge with organizations’ varied networks is the secure, standardized exchange of data and information between devices, equipment and services, which is where OPC UA comes in. OPC UA is
an extensible, platform-independent standard that enables the secure exchange of information in industrial systems. It is compatible with Windows, macOS, Android and Linux and works on PCs, cloud-based infrastructures, programmable logical controllers, micro-controllers and cyber physical systems.
It doesn't stop there though; OPC UA is also designed to structure and share that data upward to IT networks and to the cloud beyond. It’s the comprehensive information modeling framework provided by OPC UA that allows it to relay context for information and go beyond simple data transmission, context that is critical for the data to be interpreted and used correctly by external systems. A process variable coming from a sensor value can be modeled with engineering units to allow proper interpretation, operational limits to validate modifications and adjust display and servicing information to provide an approximation of accuracy.
The security of cloud-based platforms has been a growing concern over the past decade. As OPC UA is a communication layer for machine-to-machine and machine-to-cloud communication, it operates with two security layers. The first layer sets up an encrypted channel connection between the client and server. This layer provides confidentiality, integrity through message signing and certificate-based authentication. The application layer then manages user authentication and user authorization.
OPC UA allows OPC to be used as a client or a server; it will provide data to various devices and applications to control equipment functions. OPC
FIGURE 3: Third-party reporting software streamlines operational and compliance reporting from data sources like SCADA, HMI, and historians. Courtesy: SmartSights
‘Analyzing historical data allows operations management to identify patterns, trends and anomalies that may otherwise go unnoticed.’
‘
Organizations require systems
to protect operational data from unwanted access and to keep track of significant system events.
UA server applications allow data exchange for machine-to-machine and PC-to-machine communication. OPC UA server uses include Remote Terminal Units (RTUs) field service applications. Another benefit of OPC UA is that assets can be upgraded to this as they are repaired by deployed edge gateway devices as budgets allow.
A key theme of OPC UA is increasing connectivity — increasing connectivity between devices, networks and physical assets and the cloud. Increasing connectivity allows for greater process transparency and the added potential for predictive analytics and sentinel alarm conditions. Additionally, beyond delivering alerts or metrics to people, this advanced technology can deliver information to other software systems to reduce unplanned downtime. Integrating issues management software with ticketing systems, for example, further enables real-time collaboration between operators and engineers.
“Failing to address cybersecurity risk in a proactive way can have devastating results. Failing to take reasonable measures and employ best practices to prevent, detect and swiftly respond to cyberattacks means that organizations and the people who run them will face greater damage — including technical, operational, financial and reputational harm — when the cyberattacks do occur,” said a report, “Cybersecurity Risk & Responsibility in the Water Sector,” from the American Water Works Association.
Organizations require systems to protect operational data from unwanted access and to keep track of significant system events. Although replacing legacy systems and networks can be extremely costly, it is essential to work with vendors and cybersecurity experts to implement updates and, if necessary, overhauls of outdated systems. Invoke the help of internal or external advisors to prioritize risk and develop a realistic approach and plan for enhancing cybersecurity. At a minimum, comply with basic standards including restricted physical and technical access, firewalls, logging and encryption.
Additionally, many SCADA systems are simply over-exposed to the internet by remote desktop applications (RDP) (e.g., TeamViewer). To provide process and asset information to operators, organizations have provided much more, ignoring the principle of least privilege and opening their control systems and their
hosts to remote desktop access by unnecessary parties. Advanced remote alarm notification software allows remote operators access to only the information they need from SCADA and not access to the SCADA itself or its operating system host. Such notification software is compatible with more secure, layered networks in which a series of firewalls provide added protection from attacks. This is done by deploying notification solutions alongside the SCADA system at the network's control level and using notification modalities that are not internet facing or distributing internet-facing notification processes to higher levels.
There are several steps that organizations should take to improve their cybersecurity:
• Update any software to the latest version.
• Deploy multifactor authentication.
• Use strong passwords to protect remote desktop protocol credentials.
• Ensure antivirus systems, spam filters and firewalls are up to date, properly configured and secure.
CIs should also take steps to secure any remote access software. They shouldn’t use unattended access features and IT leaders should configure the software such that the application and associated background services are stopped when not in use. Integrating these advanced software systems through the SCADA system is critical to further reducing cyberattacks.
Cyber resilience ensures this critical data is accessible and secure at the time of an attack. Whether it be customer data, financial information or licensed proprietary technologies, this resilient approach limits sensitive data exposure and loss during any incident.
According to McKinsey & Co.’s report, “Critical Resilience: Adapting Infrastructure to Repel Cyberthreats,” cyberattacks should be thought of as a certainty akin to the forces of nature. Just as engineers must consider the heaviest rains that a dam may need to contain in the next century, those digitizing infrastructure must plan for the worst in considering how an attacker might abuse or exploit systems that enable infrastructure monitoring and control. PE
Cody P. Bann is vice president of engineering at SmartSights. David Nolan is the industry solutions manager at SmartSights.
Scott Dowell, Wesco, Waxhaw, North Carolina
Learn how manufacturers can manage the balance between connectivity and the large capital expenditures to modernize and what plants will be able to do in the future as a result.
Manufacturers of all sizes are in the midst of a transformation, driven in part by the emergence of new technologies that can automate, enhance and speed their operations. For almost a decade, firms have been working to incorporate operational technology (OT) with information technology (IT) and have experienced incremental benefits that are impactful but only graze the surface of what’s possible.
Plant engineers have been advocating to blend the powers of plant hardware and software to generate a more far-reaching improvement and other departments are finally sitting up and taking notice.
As companies have begun to embrace industry 4.0, it has highlighted the opportunities that automation and industrial software platforms can provide. It’s not enough to simply have assets that are “connected” if they cannot provide any real visibility needed to modify operations, enhance production, spur efficiency or avoid downtime. And the information cannot live in a silo, rather the shop floor data needs to be delivered in real time throughout the enterprise for better integration and decision-making.
By establishing the infrastructure required to connect the hardware and software applications available on the plant floor to the data flows from business applications, manufacturers can finally tap into the vast amount of data generated from machines, sensors and operational analytics to connect the shop and top floors.
But how can manufacturers embrace this shift to industry 4.0 and focus on true connectivity while balancing large capital expenditures around
upgrading and modernizing your installed base production equipment? And what will this technical transition enable plants to do in the future?
Plant managers are working hard to stay on top of production, meet quality and compliance standards and maintain tight schedules, all while trying to keep costs down and capacity high. The operations side of the business is working to identify production opportunities, speed efficiency and reconcile planned production versus actual.
While collectively these two groups recognize they may not be leveraging all of the data that’s being collected from the plant floor, undergoing a digital transformation project to amplify their efforts can seem like a daunting task given all of the priorities already in play. But, leveraging all of the intelligence that’s already being collected across the plant floor and integrating it into an accessible way for all aspects of the business, is about working smarter, not harder. There’s certainly a lot of information to sift through; industrial manufacturers generate six different categories of data:
• Operational data, which includes machine performance information such as speed, pressure, temperature and torque measurements from various parts of machinery.
• Machine health and status data generated from sensors that can report on the overall operational status of equipment, such as wear and tear, heat generation and system integrity.
• Know the six types of data that industrial manufacturers generate and how they can be better used.
• Learn how data feeds can be unified cost effectively.
• Hear what plants that are investing in data contextualization and process optimization are achieving.
• Quality control data generated from production lines, sensors and vision systems as they collect information to ensure quality control and compliance with specifications.
uManufacturers are harnessing data-driven integration of operational and information technology to break down silos, enhance automation and optimize efficiency, enabling industry 4.0 advancements while balancing cost-effective modernization.
u It’s not enough for plants to simply have assets that are “connected” if they cannot provide any real visibility needed to modify operations, enhance production, spur efficiency or avoid downtime.
• Safety data including details on safety interlocks, operator presence and access control to ensure workplace safety and compliance.
• Energy consumption data that relies on smart sensors and devices to track energy. This data is used to identify energy-saving opportunities and to ensure sustainable operations.
• Network and communication data, which includes information about the performance and integrity of network communications within smart devices and components.
Much of that data goes unnoticed or underused because the right systems aren’t in place to make use of the information. As software continues to
‘
Advanced technical innovations have driven down the costs associated with these solutions, without the need for an extensive or expensive IT burden.
’
become a bigger part of the network infrastructure (OT environment), solutions are more refined and bundled to more seamlessly bring hardware and software together. Capitalizing on these advancements can allow you to replace the haphazardly connected assets and first-generation OT networks that many plants have today.
For some manufacturers, this may mean embedding industrial software on top of existing product lines or machines, while others may need to update an entire production line. Smaller, more modular application software and hardware platforms built on open architectures allow plants to work in an applike environment with data that resides on the edge or in the cloud as part of the plant’s connected environment. This low-code option can help bring plants online more quickly, with modules built out for various functions, like plant performance or scheduling.
However, the infrastructure is architected for your plant, truly connecting data and creating a solid operational foundation can deliver the insights needed to make industry 4.0 a reality. This can help expand the visibility and control of the plant's operations and enable the business to better understand and predict orders, address inventory needs and develop more realistic financial forecasts.
So, now for the million-dollar question: How can data feeds be unified in a cost-effective way?
Many manufacturers may be wary of making a major purchase that might fail to deliver. But advanced technical innovations have driven down the costs associated with these solutions, without the need for an extensive or expensive IT burden.
That’s good news given the complexity is one thing that hasn’t changed. Industrial environments
have a wide range of devices, vendors, network protocols and time series datasets that grow rapidly over time. This has driven the need for that information to be available to users in a self-service model.
As such, industrial data operations is a growing category of data management designed to address the agility required to manage industrial data at scale and rapidly deploy new data applications. What this means practically is that in many cases, instead of processing data on external servers, data is being processed at the "industrial edge” — close to where it is generated near the plant floor itself. Custom solutions that work at the edge can be built quickly and easily and don’t require heavy investment.
This new approach is a cost-effective way to break down data silos, improve data quality and glean better insights for critical decision making. These platforms simplify complex industrial data by making it easier to access from various sources, understand the context and use it in various applications and analytics that can drive operational value.
For plants that have already begun this journey of data contextualization and process optimization, what have they achieved?
The biggest pain points that have been alleviated are in four key areas: assets, production, workforce and data analytics.
• Assets: From maintenance to inventory management, use to energy efficiency, plants are dealing with a number of asset-level pain points. Some are financial — rising energy costs and high life cycle and maintenance costs and others are operational — inadequate planning, lack of inventory, unplanned down time due to machine failures and poor tracking. Automation and software integration have helped solve a number of these issues, including lowering energy usage and associated cost savings, optimizing resources and alleviating capacity constraints, streamlining operations and reducing unplanned downtime.
• Production: Plants are dealing with several production priorities, including improving throughput and quality control, while also reducing waste, which can impact the overall bottom line. Integrating software and automation can help ensure steady production output, enhance product quality by reducing error rates in production,
improve uniform quality across production batches and scale operations efficiently.
• Workforce: Labor shortages continue to impact plant operations and many of the issues plants face around workforce are closely linked — poor training and unsafe work sites lead to unsatisfied workers, high turnover and workforce shortages that hinder production. With automation, plants can invest in quality training programs for skills enhancements, provide a safe workplace with improved morale, address workforce shortages so that operations aren’t impacted and ultimately reduce turnover.
• Data analytics: One of the most significant areas of improvement is the ability to bring data out of the silos, speed decision making and improve predictive maintenance and data security. Automation has helped plants make more accurate decisions based on current insights through real-time data access, identify problems in the plant before they lead to major disruptions, bring together data sources for cohesive insights and build secure infrastructure to withstand cyber threats.
We’ve come a long way from the old network protocols. Today, manufacturers have the opportunity to make industry 4.0 a reality by capitalizing on technology innovations that can help them achieve their goals. With a focus on the continuous improvement of these integrations, industrial manufacturers can also ensure their business is prepared for the digital future and the integration of more capabilities in the years to come. PE
Scott Dowell is Senior Vice President and General Manager, Industrial and CIG, at Wesco.
2: With the infrastructure in place to connect the hardware and software applications available on the plant floor to the data flows from business applications, manufacturers can connect the shop and top floors and tap into the data from machines, sensors and operational analytics.
Courtesy: Wesco
VP, Sales
Matt
Sales Director
Allen Basis
Sales Account Manager
Brian
Sales Account Manager
Richard
Sales Account Manager
MWaddell@WTWHMedia.com 312-961-6840
abasis@wtwhmedia.com 609-664-1451
BGross@WTWHMedia.com 847-946-3668
RGroth@WTWHMedia.com 774-277-7266
Robert Levinger RLevinger@WTWHTMedia.com 516-209-8587
Sales Account Manager
Judy Pinsel 847-624-8418
JPinsel@WTWHmedia.com
Patrick Lynch, Senior Vice President, Sales & Strategy 847-452-1191, PLynch@WTWHMedia.com
McKenzie Burns, Marketing Manager MBurns@WTWHmedia.com
Courtney New, Program Manager, Content Studio CNew@WTWHMedia.com
Paul Brouch, Operations Manager 708-743-5278, PBrouch@WTWHMedia.com
Rick Ellis, Director, Audience Growth 303-246-1250, REllis@WTWHMedia.com
Custom reprints, print/electronic: Matt Claney, 216-860-5253, MClaney@WTWHMedia.com
Information: For a Media Kit or Editorial Calendar, go to https://www.plantengineering.com/advertise-with-us.
Letters to the editor: Please email us your opinions to ARozgus@WTWHMedia.com. Letters should include name, company and address, and may be edited.
From fractional to 600 HP, Yaskawa industrial drives cover all of your application needs, with an array of features that improve your operations.
ü Intuitive Mobile-Friendly Interaction
ü Fast Connection to Any Major Network Protocol
ü Safety - Standard
ü Best-in-class Efficiency
And don’t forget about everything else Yaskawa provides you with.
ü World-Class Quality and Reliability
ü Award Winning Customer Service
ü Product Lifetime Training
ü Free 24/7/365 Technical Support
Want to have all of that working for you? Contact Yaskawa today.