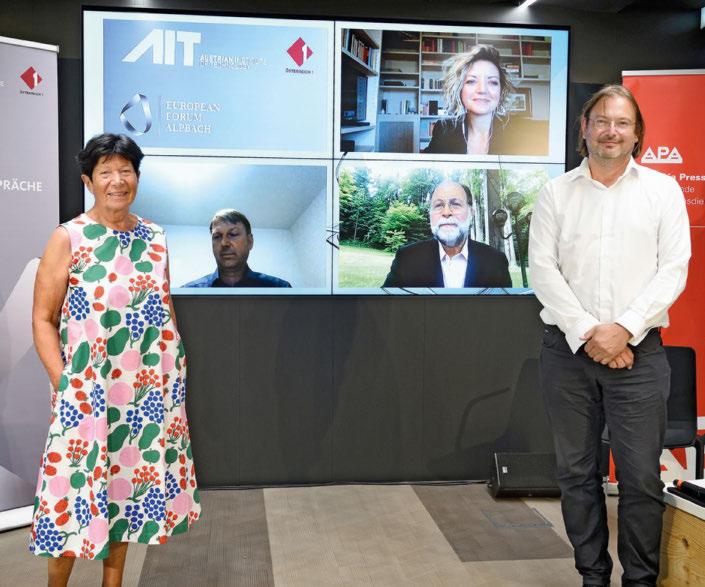
6 minute read
COMPLEXITY SCIENCE – CORONA
COMPLEXITY SCIENCE – CORONA AND THE CONSEQUENCES
Vittoria Colizza (Sorbonne), Ricardo Hausmann (Harvard), Dirk Helbing (ETH Zürich), Stefan Thurner (CSH) // Chair: Helga Nowotny (RTD-Council)
Supported by Complexity Science Hub Vienna
Schon früh in der Corona-Krise versuchten wissenschaftliche Disziplinen wie die Complexity Science, Modelle und Vorhersagen zur Eindämmung des Virus zu erstellen, so Helga Nowotny vom österreichischen Rat für Forschung und Technologieentwicklung in ihrer thematischen Einführung. Die Pandemie hat laut Dirk Helbing, Professor of Computational Social Science an der ETH Zürich, klar die Grenzen von Big Data und AI aufgezeigt. Die AI-Vision, dass künftig Daten für sich selbst sprechen, bewahrheitete sich nicht. Vorhersagen über die Pandemie zu treffen, war eine große Herausforderung. Helbing kritisierte generell AI-Methoden, mit denen etwa Flughafenpassagiere überwacht und Terroristen gesucht werden. Die Fehlerraten lagen bei 99 Prozent bzw. gab es 100.000 falsche Positivmeldungen für jeden realen Terroristen. Das Problem sei, dass Algorithmen statistische Beziehungen in kausale Zusammenhänge verwandeln können. „Wir sollten AI und Computer Science anders nutzen“, forderte Helbing. Mit Smartphones und ihren Sensoren können etwa kollaborative Sicherheitsnetzwerke gebildet werden, mit denen schon Erdbeben detektiert wurden. Helbings Vision: Der Aufbau einer digitalen Demokratie. Hier spiele der freie Zugriff auf Daten mit Open Source und Open Data eine wichtige Rolle. Laut Helga Nowotny ist ein kollektiver Lernprozess erforderlich, eine neue Kultur, wie man mit Big Data umgehe: „Wir müssen wissen, wo Daten erzeugt und in welchem Kontext sie gesammelt werden, sonst kann es falsch laufen.“ Mit diesem Lernen müsste schon früh in der Schule begonnen werden. Vittoria Colizza, Research Director bei INSERM und der University of Sorbonne, Faculty of Medicine, in Paris, sprach zum Thema „Chasing Covid-19 Pandemie through modeling” und teilte ihre Erfahrungen der letzten Monate. Anfangs gab es keine Daten und Erfahrungen. Mit mathematischen Modellen wurde versucht, Klarheit zu schaffen. „Wir hätten auch mehr aus den Erfahrungen anderer Länder lernen sollen“, so Colizza, da habe es eine gewisse nationale Arroganz gegeben. Ricardo Hausmann, Ökonom an der John F. Kennedy School of Government der Harvard University, verwendet Complexity Science, um Wachstum in Entwicklungsländern und ökonomische Krisen zu erforschen. „Ich sehe Gesellschaften wie ein soziales Gehirn, in dem unterschiedliche Teile spezialisiertes Wissen erlangen, das vernetzt werden muss, um es zu nutzen“, so Hausmann. Er untersucht, wie diese Netzwerke wachsen. Seine Erforschung von Wirtschaftszusammenbrüchen hat ergeben, dass die Erholungsgeschwindigkeit vor allem von der Resilienz der Orte abhänge - und diese wiederum vom Zustand der gesellschaftlichen Netzwerke. Um Resilienz in einer Gesellschaft zu schaffen, bedarf es aber perfekt auf die jeweilige Situation angepasste Maßnahmen. Ein Gutes hat die Corona-Krise laut Stefan Thurner, Leiter der Abteilung Science of Complex Systems an der Medizinischen Universität Wien und Präsident des Complexity Science Hub Vienna: Es gab erstmals den Schritt in Richtung einer evidenzbasierten Politik. Im CSH wurden am Anfang der Krise alle laufenden Projekte unterbrochen, um 18 Teams zur Lösung von 18 Problemen zu bilden – etwa um die Rolle der Kontaktnetzwerke oder ökonomische Effekte besser zu verstehen. Zugleich wurde ab April mit Grafiken zu den Infektionsraten und einer Corona-Ampel die Öffentlichkeit informiert. Was nicht funktioniert habe, war der Zugang zu den entsprechenden Daten. Da mangelte es oft an der Datenqualität, eingespielten Datenprozeduren und dem Teilen der Daten. „50 Prozent der Projekte scheiterten, da wir die Daten nicht in der passenden Art nutzen konnten“, sagte Thurner. Ohne korrekte Daten könne Digitalisierung nicht funktionieren, warnte der Komplexitätsforscher: „Wir müssen lernen, mit Daten richtig umzugehen.“
Helga Nowotny (Austrian Council for Research and Technology Development, Former President ERC European
Research Council), and Stefan Thurner (Head of Complexity Science Hub Vienna, MedUni Vienna) (in the foreground);
Vittoria Colizza (INSERM and University of Sorbonne), Ricardo Hausmann (Harvard University) and Dirk Helbing (ETH Zürich) (bottom left on the screen)
Already early on in the corona crisis, scientific disciplines such as Complexity Science attempted to create models and predictions for the containment of the virus, stated Helga Nowotny of the Austrian Council for Research and Technology Development in her thematic introduction. According to Dirk Helbing, Professor of Computational Social Science at the ETH Zurich, the pandemic has clearly shown the limits of Big Data and AI. The AI vision that data would speak for themselves in the future has not come true. Making predictions about the pandemic has been a major challenge. Helbing generally criticized AI methods, such as those used to monitor airport passengers and to search for terrorists. The error rates were 99 percent, in other words there were 100,000 false positives for every real terrorist. The problem is that algorithms can transform relations into causal relationships. "We should use AI and computer science differently," demanded Helbing. For example, smartphones and their sensors can be used to create collaborative safety networks with which earthquakes have already been detected. Helbings´ vision: The creation of a digital democracy. Free access to data with open source and open data plays an important role here. According to Helga Nowotny, a collective learning process is needed, a new culture of how to deal with Big Data: "We have to know where data is generated and in what REVIEW #Efatec 2020 context it is collected, otherwise things can go wrong." This learning would have to already begin early in school. Vittoria Colizza, Research Director at INSERM and the University of Sorbonne, Faculty of Medicine, in Paris, spoke on the topic of "Chasing Covid-19 pandemic trough modeling" and shared her experiences of recent months. Initially, there was neither data nor experience. Mathematical models were used to try to create clarity. "We should also have learned more from the experiences of other countries," said Colizza, adding that there was a certain national arrogance. Ricardo Hausmann, an economist at Harvard University's John F. Kennedy School of Government, uses complexity science to study growth in developing countries, and economic crises. "I see societies as a social brain in which different parts acquire specialized knowledge that needs to be cross-linked in order to use it," says Hausmann. He studies how these networks grow. His research into economic collapses has shown that the speed of recovery depends above all on the resilience of places – which in turn depends on the condition of the social networks. To create resilience in a society, however, requires measures that are perfectly adapted to the respective situation. According to Stefan Thurner, Head of the Section for Science of Complex Systems at the Medical University of Vienna and President of the Complexity Science Hub Vienna, the corona crisis has one positive aspect: For the first time, there was a move toward evidence-based policies. At the CSH, all ongoing projects were interrupted at the beginning of the crisis to form 18 teams to solve 18 problems – e.g., to better understand the role of contact networks or economic effects. At the same time, starting in April, the public was informed by means of graphics on infection rates and a so-called Corona traffic light. What did not work was the access to the corresponding data. There was often a lack of data quality, well-established data procedures and data sharing. "50 percent of the projects failed because we could not use the data in the appropriate way," said Thurner. Without correct data, digitalisation will not work, the complexity researcher warned: "We must learn to use data correctly."