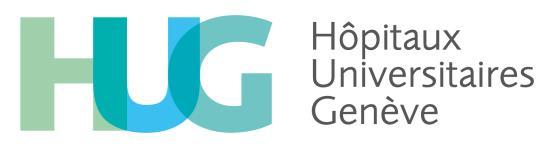
4 minute read
Projet 12 – « AVC, A Vos Citrons ! », Court-métrage d’une histoire vécue
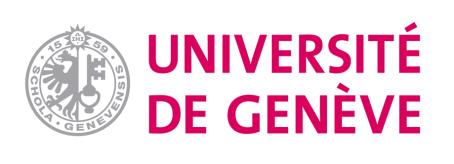
Projet 10 – Deep learning-assisted automated segmentation of lungs and COVID-19 lesions from chest CT images
Auteurs
Habib Zaidi, Département Diagnostique, HUG Isaac Shiri, Département Diagnostique, HUG Hossein Arabi, Département Diagnostique, HUG
Résumé du projet
Chest CT imaging has emerged as a promising tool for early diagnosis and longitudinal follow up of COVID-19 patients. However, quantitative analysis of clinical CT images through lung and infectious lesions segmentation remains challenging. We developed an automated segmentation of whole lungs and infectious regions using deep learning algorithms to implement a fast, consistent, robust and human error immune framework for lung and pneumonia lesion detection and quantification. A mean accuracy in lesions and lung segmentation larger than 0.91 and 0.98, respectively, was achieved, with a low amount of false negative and false positive rates.
Introduction
A wide range of radiological examinations, including CT scanning, are being employed for diagnostic and prognostic purposes to tackle the COVID-19 pandemic. A challenging problem which arises in clinical routine quantitative analysis of CT images is lung and infectious lesion segmentation owing to large inter/intra-observer variability and timeconsuming annotation process. Deep learning algorithms have been widely utilized in various medical image analysis problems owing to the promising results achieved. An Artificial intelligent solution with robust and consistent performance would enable efficient management of COVID-19 patients.
Innovation
Novel deep learning techniques are revolutionizing clinical practice and are now offering unique capabilities to the clinical imaging community and biomedical researchers at large. We set out to develop an automated segmentation of 3D whole lung and infectious lesions in COVID-19 patients using deep learning algorithms to enable fast, consistent, robust and human error immune framework for lung and pneumonia lesion detection and delineation. Due to the complex nature of the problem and high variability in lesion manifestation (appearance, size, location, boundaries), a novel deep learning-assisted image segmentation technique was proposed and implemented to enrich specific COVID-19 pneumonia features identification from CT images. Moreover, a large multicenter and multi-scanner dataset was collected for the development of a deep learning model enabling efficient COVID-19 patients management. The developed artificial intelligence platform was evaluated using a wide range of COVID-19 patients at different stages of the disease and diverse populations from multiple centers around the world.
Avantages
A number of studies reported that CT is a fast and highly sensitive approach for COVID-19 detection, segmentation and management. The proposed model enables whole lung and infectious lesions segmentation in very short time (few seconds) compared to time-
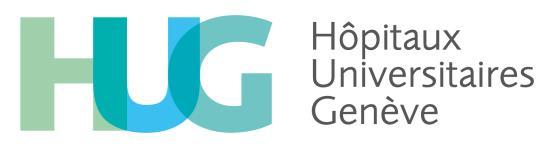
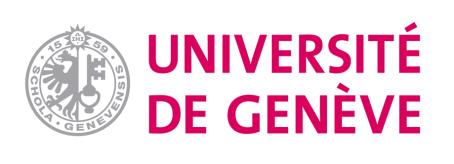
consuming (5 hours) and labor-intensive process if the task is performed manually by a radiologist. The developed artificial intelligence-based solution enables big data analysis of COVID-19 for automated assessment of progression/regression of pneumonia lesions. The tool allows automated calculation of the percentage of infectious and well aerated regions in the lungs, commonly performed by visual assessment. Applications are foreseen are not only in the area of detection and quantification but also in providing diagnostic and prognostic metrics. The acquired large-scale labelled datasets enables to build a robust and generalizable model to avoid overfitting.
Résultats préliminaires
The mean accuracy for lesions and lung segmentation is larger than 0.91 and 0.98, respectively, with a low amount of false negative and false positive rates. Our proposed model enabled image biomarker generation with clinically insignificant error (0.22±6.3%) derived from diverse multi-center multi-scanner COVID-19 patients dataset. The developed model exhibited noticeable performance variability across different COVID-19 patients gathered from different countries, centers, with various background, and at different stages of the disease. Regarding the complex manifestation of COVID-19 infection, the available state-of-the-art solutions in the literature for automated detection and quantification of pneumonia lesions faced serious challenges to achieve an accuracy of 0.90. The novel algorithm proposed in this work for COVID-19 pneumonia features identification outperformed state-of-the-art models proposed in the literature.
Développements
Though the proposed artificial intelligence-based solution was evaluated using multicenter, multi-scanner, multi-national datasets, including patients with diverse background, stages of the disease, a full-scale adaptation of this model requires further clinical assessment and fine-tuning to the specific characteristics and image acquisition parameters of a clinical center. This framework provides multiple clinically relevant image biomarkers for COVID-19 patients, capable of discriminating COVID-19 from bacterial/viral pneumonia and delivers additional metrics for implementation of prognostic models for COVID-19 patients (survival, hospital stay, ICU admission, risks, outcome).
Conclusion (si vous remportez un prix, comment l'utiliserez-vous pour faire avancer votre projet ?)
The proposed framework provides useful information for implementation of diagnostic and prognostic models specific to COVID-19. This should enable the development of a comprehensive pipeline for fully automated computer aided diagnostic/prognostic models for efficient clinical management of COVID-19 patients.