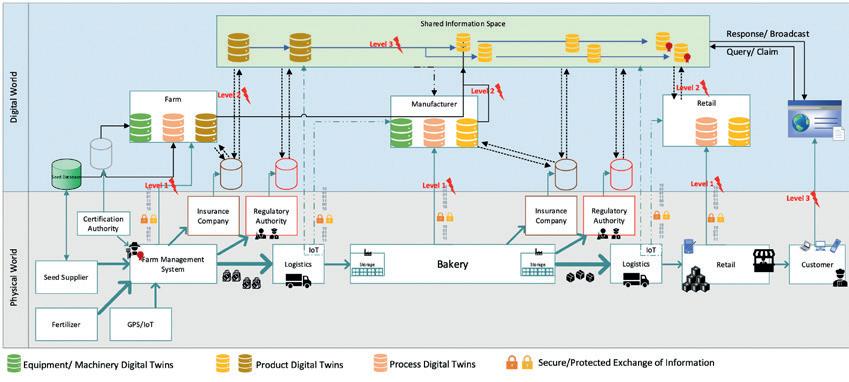
15 minute read
Digitization: Digitizing food supply chains
from f2m Automation Book
by landmagd
Digitizing food supply chains
+Why do we need more transparency in supply chains?
The global food supply network that provides us with our daily food is the world’s most extensive and most important critical infrastructure. This implies that we need to trust this infrastructure and its services. Unfortunately, we still see a substantial number of food safety incidents. In the fourth quarter of 2020 alone, the International Food Safety Authorities Network (INFOSAN), developed by the World Health Organization (WHO) and United Nations Food and Agriculture Organization (FAO), was involved in 23 food safety events - about half of them in Europe (1). Unsafe food still has a substantial adverse effect on our health and economy (2): one out of ten persons is affected, and about 420,000 die each year because of unsafe food, with children under 5 carrying 40% of the foodborne disease burden, from which 125,000 die every year.
Another threat to consumer trust is food fraud, referring to “the mislabeling, concealment, diversion, dilution, substitution/adulteration, unapproved enhancement, counterfeiting or grey market production/theft/diversion of foods” (3). Annual damages in Europe account for approximately EUR 8 to 12 billion. In 2019, cereal & bakery products appeared among the 10 top product categories in the annual report of the EU Food Fraud Network (4); the supply material category ’fats & oils‘ is leading these statistics and has seen an increase from 29 inquiries in 2018 to 44 in 2019. A particular type of fraud, organic food fraud, which is driven by the profit from the high price tag of this kind of food, is on the rise. In 2018, the European Commission launched the OPSON VIII operation in collaboration with Europol (5); this operation led to 63 fraud inquiries and flagged 90,000 tons of organic products as suspicious, of which 16,000 tons have been downgraded to conventional food products by the authorities.
One can also observe an increasing consumer interest in their food sources (6) and growing demand for individualized products and transparency in food supply chains. The benefit of more transparency is apparent as (7): + Consumers can make better-informed buying decisions + Traceability is improved, thus reducing the impact of recalls, accelerating the analysis of root causes, reducing fraud, and simplifying processes for audits and export control + Sustainability is improved by tracking resource consumption, CO2 footprint and waste, by identifying critical process steps in the supply chain, and by eliminating them with appropriate measures
Technologies enabling transparency in food supply chains include data acquisition technologies, Internet of Things (IoT), platforms for managing IoT generated data and big data technology (7). IoT is used to collect data from supply chain processes, these data are then stored, processed, and analyzed on an IoT platform like
Figure 1: Exemplary bakery supply chain
MindSphere1 In turn, blockchain stores transactions, therefore it must be protected from manipulation. Equally important, especially for automated analysis, are digital twins of food processes (8). They describe processes and products and their relationships in the supply chain and give data points a meaning.
In the following, we focus on a few innovative aspects related to data privacy, supply chain integrity, and data models for digital twins.
Data privacy and supply chain integrity
Digitalization is the pre-requisite for a data privacy and integrity concept for organic food supply chains. It may comprise of digital twins of each involved stakeholder, as shown in Figure 1: The bottom half shows the different actors like farmers, bakeries, logistics, retailers and customers, but also suppliers, certification bodies and insurance companies. All of them exchange products or information or both. On top, the digital world is shown with digital twins of equipment and machinery, products, and processes. They are typically stored in databases and part of them can be exchanged with business partners, agencies, and customers. This exchange is usually done via a shared information space shown on the very top of Figure 1. Ensuring integrity in supply chains typically involves three qualitatively distinct levels: 1)Level 1 ‘Reality gap’: ensures the data in the digital space correctly represents reality 2)Level 2 ‘Hackers’: ensures digital data is authenticated and cannot be changed or removed 3)Level 3 ‘Semantics’: ensures data is interpreted the right way and appropriate action is taken
The classical means to enforce Level 1 is a threat of legal consequences for providing and signing false assertions. Therefore we propose electronic signatures that can be checked automatically and thereby create more reliability. Additionally, the use of Biomarker Technology is an important pillar to ensure 1). The Biomarker Technology is a device that can check and assert if a food item is organic or not (10). The biomarker device confirms its measurements directly with an electronic signature of its own, eliminating the risk of somebody reporting embellished results.
The system can be further extended by including check sums, such as hash of measurements at different stages, and other checks across tenants (on a trusted cloud, with homomorphic encryption in perspective). Examples are described in a recent paper on olive oil fraud (11): 32 cases of fraud have been reported from September 2016 until December 2019, of which 11 concerned
¹ https://siemens.mindsphere.io/en/industrial-iot?gclid=EAIaIQobChMIl9vf6a-c8gIV2oODBx0QIQhrEAAYASAAEgIBQPD_BwE
mislabeling, 4 untrue origin, 16 substitution, 6 dilution, 5 intentional distribution of contaminated products/counterfeiting and 1 was related to theft. The true origin, for example, can be detected by establishing a specific databank of isotopic values similar to that for wine. These values denote the ratio of e.g. hydrogen to deuterium or 13C to 12C and can be measured with isotope ratio mass spectroscopy.
To ensure Level 2, a concept that relies on hashing, signing, and linking all messages on the sender and receiver’s side is required. With this concept, all directly involved parties can prove (without doubt) mathematically, at any time, that a message was sent/accepted by a specific entity and not changed afterward. Additionally, the generated signature chains can be anchored in the public distributed ledger, such as bitcoin blockchain, at regular intervals (e.g. once a day) or if somebody rejects reception (s. Figure 2). So, if some or even all parties colluded later to change or hide something, they cannot do so without this being automatically revealed. So, the regulator can automate big parts of the audit process and let it happen continuously.
The public Blockchain entry entails no data directly, but only random-looking numbers, i.e. hash values (numeric values of a fixed length that uniquely identifies data). Therefore, it is necessary that at least one of the parties still has the data, usually the owner of the data.
To ensure Level 3, the data must be interpreted with standardized ontologies. In the food industry, many different ontology standards have already been developed. Some standards are for particular use cases, but also some generic standards are available that can and should be used for a shared understanding and better exchange within the food industry. So far, none of the existing ontology standards suit the requirements of a digital twin for the supply chain. Therefore the following section introduces a new Ontology.
Ontologies for digital twins
Digitizing food supply chains heavily relies on digital twins of supply chain processes and digital twins of products. Examples of supply chain processes are not only transport, processing, and storage of food, but also lab tests of samples for quality management. Digital twins of products typically contain information about the specification of the product and its history along the supply chain. Therefore, digital twins of products and supply chain processes are closely linked. Standardized data models for these digital twins are fundamental for several reasons: + The interpretation of data in digital twins is more effortless and allows for analysis by generic tools which understand the data model; + The exchange of information represented by digital twins between stakeholders along the supply chains is simplified and can be done entirely electronically without manual examination and translation by humans; + Relationships between supply chain processes as well as between products and supply chain processes allow the automated analysis of root causes in case of contamination or fraud.
Such standardized models specify entities and their relationships using semantic Web technologies such as RDF (12), RDFS (13) and OWL (14). In a project2 co-funded by EIT Food3 addressing
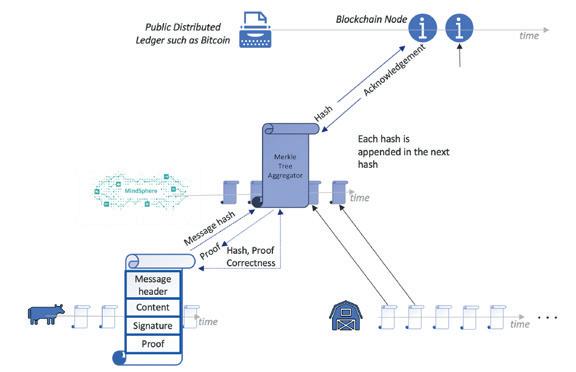
© Siemens
Figure 2: Basic supply chain integrity and privacy concept
2 www.eitfood.eu/projects/the-development-of-organic-supply-chains-that-drive-fair-transparent-and-healthy-options-for-the-consumer 3 www.eitfood.eu
INTERVIEW DIGITIZING FOOD SUPPLY CHAINS
Figure 3: The ontologies OBSC and OVSC specify the ontology OFSC and inherit its basic concepts organic supply chains for meat and leeks, several standards were considered in order to create the systematic base for a digital twin: 1. The ISA standard ISA-88 (15) (adopted as IEC 61512-1) was considered as it enables various structuring examples and labeling of unit operations. The classification into enterprise, enterprise site, work area, work centers, work units was applied to design comparable, standardized structures in the generic model.
Thus, it becomes possible to create a physical model of each production process where each work unit forms its own digital twin. The classification enables nearly every user to map their partners of the supply chain. The generic model can map from small suppliers, like farmers who supply the grain, to big flour mills and warehouses that belong to large groups or the bakery shop that sells the product in the end. In addition, the overlying corporate structure is also mapped to the standardized model. 2. The descriptions for modeling food products contained in the model are also based on established standards. In concrete terms, the Codex
Alimentarius (16), LanguaL (17), and the Food
Additive Classification (18) of the European
Community were used for this purpose. 3. Concepts of FoodOn (19), which is based on
LanguaL (17), guarantee an even broader use.
FoodOn currently represents the most powerful and massive ontology for modeling knowledge considering food sources, derived food products and some related processes. These standards were used to develop a hierarchical approach for digital twins in supply chains (see Figure 3). The first ontology, “Organic Food Supply Chain” (OFSC), is a generic ontology that contains the structure and main concepts about organic supply chains. The other two ontologies are specific for the two different product groups, beef and leek, considered in this project. A similar ontology can be developed for the supply chains of bakeries and their products.
The link to the integrity concept for food supply chains described above is achieved by including information, which is stored in the blockchain, also in the digital twin data model. A simple query using the product id allows the organic status of the product to be checked: the ontology helps to identify all instances of supply chain processes related to this particular product; information that is stored in the blockchain is queried from there.
Case examples
Seamless traceability at Hochdorf Swiss Nutrition AG (9) We describe this case as an example of traceability inside a production facility. Due to regulation, this is mandatory here. However, it is also an example of a highly automated and complex data acquisition process. Similarly automated data acquisition in any production system substantially simplifies the extension of traceability solutions along whole supply chains also involving suppliers and customers.
Hochdorf Swiss Nutrition AG4 produces food from milk, oilseeds, and cereals. In their new 30-meterhigh production tower, one of the largest of its kind in Europe, around 75 million liters of milk are processed each year into around 30,000 tons of baby food. Precise process control around the clock guarantees that the milk powder has the right consistency at the end and does not become too dusty or too moist.
The high hygiene and quality requirements for baby food require reliable 24-hour monitoring of the plant and complete traceability of the entire
Ontology OBSC Ontology OFSC
Ontology OVSC
© Siemens
4 www.hochdorf.com

© Siemens
production process. This is achieved with modern technology from Siemens. A total of 663 digital and 637 analog sensors continuously record parameters such as pressure, temperature, or filling levels. In addition, 300 motors provide the drive, and 1,700 valves regulate the pressure and flow rates of the media and ingredients required for production (see Figure 4).
All production data is stored on a long-term basis. Data processing and automation are carried out via 10 Simatic controllers with 64 peripheral stations. The system produces continuously for one to two weeks in a 24-hour operation. All process variables are continuously recorded in the control room, and crucial key data is automatically stored on a central server. This procedure ensures complete traceability of the entire production process – all data can still be retrieved years later. Potential applications of such data in bakeries include rapid detection of problems in the process, and improvement of the production process using data analytics.
Potato chips with a certificate show their origin
Siemens is working on an intelligent solution to deal with recalls more quickly and specifically in the future. The open, cloud-based MindSphere IoT system will enable suppliers, distributors, and manufacturers to collect data at every stage of the transport and production chain and store it in the Siemens blockchain.
This makes it possible to narrow down a recall to a specific batch or production day. The risk of contaminated food entering the supermarket can thus be minimized as much as the unnecessary effort and costs for a reasonless recall.
In a possible scenario, for example, a Frankfurtbased manufacturer of potato chips with an organic certificate who obtains his potatoes from Germany, the salt from France, and the sunflower oil from Italy would have immediate access to all relevant information: from the cultivation of organic potatoes and other ingredients, their storage and transport, processing such as cutting, frying, and seasoning to packaging and distribution at the retailer.
At the end of the day, the consumer is provided with a complete information chain that can be viewed at any time and guarantees that his chips consist of, for example, 100% organically grown potatoes and that they have been processed under optimal conditions.
Figure 4: Numerous sensors in the production plant record all parameters. Important key data is stored automatically. This ensures complete traceability of the entire baby food production process
The transparent supply chain for food traceability ensures that products and their ingredients are safe and genuine. Wholesalers and supermarkets receive detailed information on where they were last stored. The manufacturer can identify the origin of the ingredients used at any time and obtain detailed information about their producers. The unchangeable time stamps provide all ingredients with a forgery-proof best-before-date. +++
Authors
Rudolf Sollacher1, Aliza Maftun1 , Michael Fiegert1, Alastair Orchard2 , Kilian Vernickel3, Paul Weber4
1 Siemens AG, Technology, Otto-Hahn-Ring 6, 81739 Munich, Germany 2 Siemens Industry Software S.r.l., Via Enrico Melen, 83, 16152 Genova GE, Italy 3 Fraunhofer Institute for Casting, Composite and Processing Technology IGCV, Am Technologiezentrum 10, 86159 Augsburg, Germany 4 Fraunhofer Institute for Process Engineering and Packaging IVV, Heidelberger Str. 20, 01189 Dresden, Germany
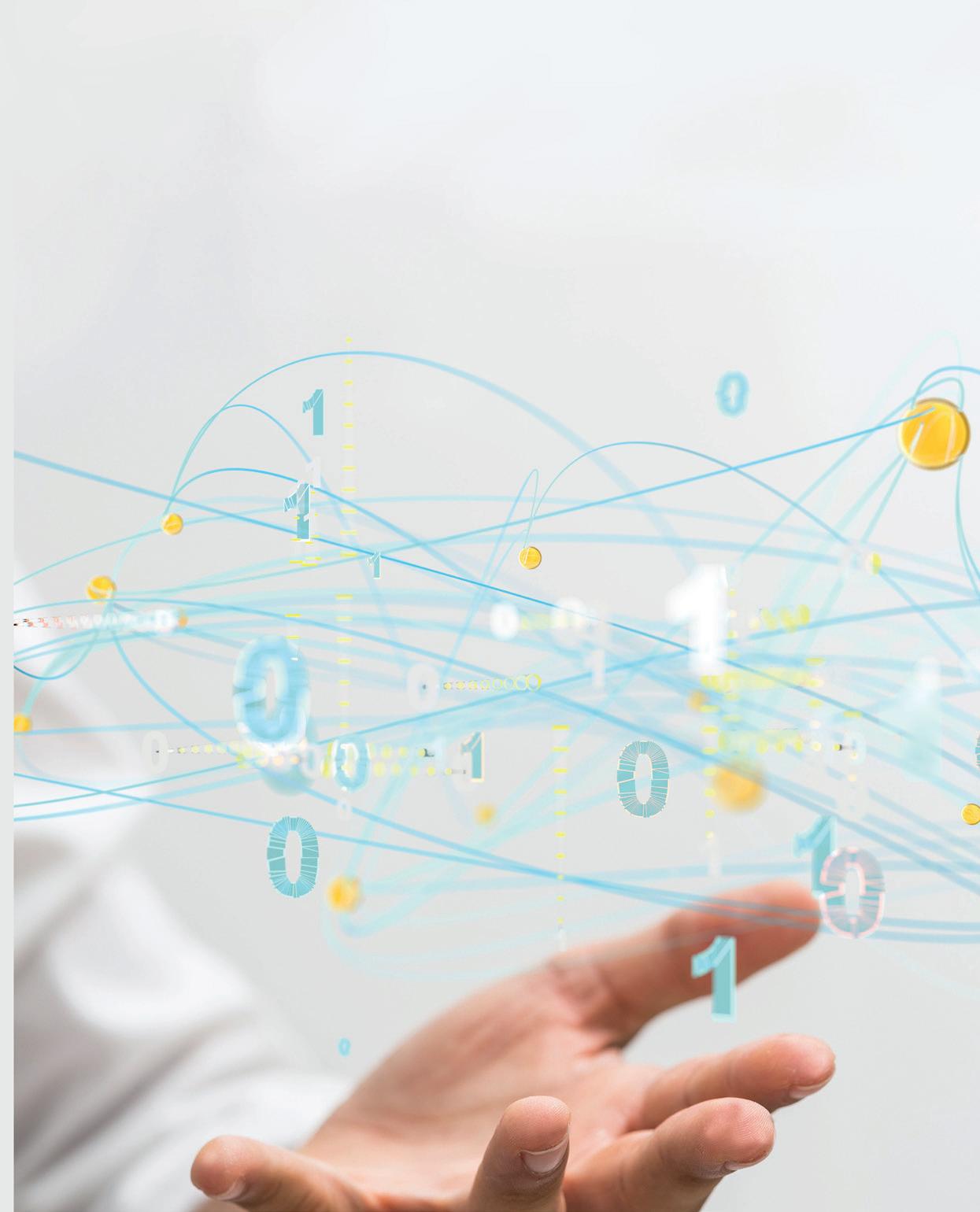
Bibliography
1. INFOSAN. INFOSAN Quarterly Summary, 2020 #4. World Health
Organization. [Online] January 27, 2021. [Cited: March 10, 2021.] www.who.int/news/item/27-01-2021-infosan-quarterlysummary-2020-4. 2. Paul Garwood; Zoie Jones. Food safety is everyone’s business.
World Health Organization. [Online] June 6, 2019. [Cited: March 10, 2021.] www.who.int/news/item/06-06-2019-food-safety-iseveryones-business. 3. Organic Food Fraud in the EU: Meaning, Examples & Prevention. foodcircle. [Online] [Cited: March 10, 2021.] www. foodcircle.com/magazine/organic-food-fraud-eu-meaningexamples-prevention. 4. Commission, European. 2019 Annual Report: The EU Food Fraud
Network and the Administrative Assistance and Cooperation
System. Luxembourg: Publications Office of the European
Union, 2020. ISBN 978-92-76-18809-4. 5. DG SANTE Unit G5; DG AGRI Unit B4. OPSON VIII DEBRIEFING: organic targeted action. [Online] [Cited: March 11, 2021.] https:// ec.europa.eu/food/sites/food/files/safety/docs/food-fraudreports_20191125_pres01.pdf. 6. How consumer demand for transparency is shaping the food industry. [Online] Label Insight, 2016. [Cited: August 6, 2021.] www.labelinsight.com/hubfs/Label_Insight-Food-Revolution-Study.pdf?hsCtaTracking=fc71fa82-7e0b-4b05-b2b4de1ade992d33%7C95a8befc-d0cc-4b8b-8102-529d937eb427. 7. Transparency in food supply chains: A review of enabling technology solutions. Astill, J., et al. s.l.: Elsevier BV, 2019, Trends in
Food Science & Technology, Vol. 91, pp. 240-247. 8. Pieter Verboven; Thijs Defraeye; Ashim K Datta; Bart Nicolai.
Digital twins of food process operations: the next step for food process models? [ed.] Pedro ED Augusto. Current Opinion in Food Science. March 19, 2020, Vol. 35, pp. 79-87. 9. Blockchain in the food and beverage industry. s.l. : Siemens
AG, 2019. 10. High-resolution mass spectrometry-based metabolomics for the discrimination between organic and conventional crops: A review. Mihailova, A., Kelly, S. D., Chevallier, O. P., Elliott, C.
T., Maestroni, B. M. & Cannavan, A. Apr 2021, Trends in Food
Science and Technology, Vol. 110, pp. 142-154. 11. Emerging trends in olive oil fraud and possible countermeasures. Casadei, E., et al. s.l.: Elsevier BV, 2021, Food Control, Vol. 124, p. 107902. 12. A comparison of RDF query languages. International Semantic. Haase, P., et al. Berlin: Springer, 2004. International
Semantic Web Conference. pp. 502–517. 13. Hendler, Dean Allemang and Jim. Semantic Web for the Working Ontologist – Effective Modeling in RDFS and OWL. s.l.:
Elsevier Inc., 2011. 14. S., Bechhofer. OWL: Web Ontology Language. [ed.] ÖZSU M.T. LIU
L. Encyclopedia of Database Systems. Boston, MA : Springer. 15. Exchange General, Technical and Business Information. ISA88. [Online] The International Society of Automation. [Cited: 08 08, 2021.] www.isa-88.com/. 16. Codex Classification Of Foods And Animal. s.l.: CODEX ALIMEN-
TARIUS COMMISSION, 2006. 17. The LanguaL 2017™ Thesaurus – Details for descriptor K0020. [Online] Danish Food Informatics. [Cited: 08 08, 2021.] www. langual.org/langual_thesaurus.asp?termid=K0020&haschildr en=True&o. 18. GSFA Online. CODEX GENERAL STANDARD FOR FOOD ADDITIVES (GSFA) ONLINE DATABASE. [Online] FAO/WHO. [Cited: 08 08, 2021.] www.fao.org/gsfaonline/index.html. 19. Hsiao, William, et al. FoodOn: A farm to fork ontology. [Online] [Cited: 08 08, 2021.] https://foodon.org/. 20. A study on adulteration in cereals and bakery products from
Poland including a review of definitions. Kowalska, A.; Soon,
J. M.; Manning, L. s.l.: Elsevier BV, 2018, Food Control, Vol. 92, pp. 348-356. 21. FTTO: An example of Food Ontology for traceability purpose. Teresa Pizzuti, Giovanni Mirabelli. 2013. 2013 IEEE 7th
International Conference on Intelligent Data Acquisition and
Advanced Computing Systems: Technology and Applications (IDAACS). pp. 281–286.