
7 minute read
The FDT integration standard is set to transition into an information exchange platform which will enable cloud-based asset monitoring for field devices
WIRELESS CONDITION MONITORING
WIRELESS CONDITION MONITORING
Advertisement
Wireless sensors can help discover maintenance issues before they happen by supplying real-time information, says John Bernet. C ondition monitoring is the best way to proactively determine when machines need maintenance. Collecting real-time data on an asset’s condition provides an accurate, up-to-date picture of asset health. Regardless of the type of asset, condition monitoring is the best solution to reduce unplanned downtime and production gaps. Machines monitored by wireless sensors can let a user know the machine needs to be inspected or maintained. When equipment is continuously monitored with wireless sensors, users are alerted to changes or deviations from the norm. Once installed, they monitor assets 24/7 and trigger alarms when conditions reach certain thresholds.
Wireless sensors easy to install, even on assets in dangerous or hard-to-reach places and once in place they avoid the need for repeated trips to take measurements.
The next steps are to diagnose the potential fault and its severity so users can plan the best corrective action. Numerous technologies can detect machine faults and their severity. By matching the asset and the company’s needs and budget with available technologies, users can boost reliability and maximise maintenance dollars and resources. Condition-monitoring methods Predictive technology methods that detect potential faults early are not always the best choice. Striking a balance between the amount of warning about the fault and the time and money needed to spend to diagnose the fault is key. So, what condition-monitoring methods might fit the company’s needs? Here are some technical considerations for four common methods: 1. Oil analysis is capable of detecting faults early. Monitoring lubricant, wear, and contamination offers a multifaceted look at asset condition and can alert users to potential issues before any actual damage occurs. Oil analysis can be useful for low-speed machinery (<5 rpm), where vibration analysis may be difficult. Obtaining oil samples and interpreting oil analysis data can be complex, and using a lab brings additional costs. Not all assets have oil that can be analysed. 2. Ultrasound analysis can discover things oil analysis can’t, such as bearing wear or fatigue so early that it is often too soon to replace the bearings. Increased decibel readings can indicate a potential fault resulting from friction. Ultrasound can detect leaks by identifying sounds at frequencies that wouldn’t be heard in a plant’s ambient noise. Ultrasound can be useful for low-speed machines. 3. Vibration monitoring analysis is well established, so vibration patterns and anomalies are well documented. Vibration analysis also can identify the most common mechanical faults. It identifies faults 12 to 18 months in advance, which allows of time to react while there is still life in the asset. 4. Thermography can uncover electrical hot spots, faulty connections, overheated bearings, pipe blockages, issues with tank levels and other issues. Use vibration combined with thermography for rotating machines to avoid asset damage. Wireless sensors can communicate realtime information related to all of these methods. By monitoring measurable conditions, such as capacity, temperature, pressure, or sound, and sending a signal when the relevant quality changes or reaches a certain threshold, wireless sensors make it possible to users to know about potential faults right away. When making changes to a maintenance program, a best practice is to start with a small pilot program and grow it over time, rather than attempting to change everything at once. Recommended steps include: 1. Conduct a criticality analysis. It makes sense to keep the closest eye on the assets that have the most significant impact on the bottom line. If they aren’t being continuously monitored already, these assets are good candidates for wireless sensors. 2. Identify probable asset failures. 3. Decide on the technology best suited to identifying these failure modes, keeping in mind the team’s experience and the budget. 4. Trend and analyse the data from the wireless sensors to plan and execute maintenance actions at the most advantageous times, reducing unplanned downtime and extending the life of the assets. !
John Bernet, CMRP, is a mechanical application and product specialist at Fluke Corp.
This article originally appeared on www.controleng.com
The ultra-compact performance class: ultra-small and ultra-powerful
C6015 C6017 C6030 C6032
www.beckhoff.com/C60xx
Beckhoff opens up new applications with this generation of extremely compact and fl exible Industrial PCs, from the C6015 entry-level model to the powerful C6032 with extended interfaces. Which ultra-compact model suits your application?
Ultra-compact: C6015, C6017 processor: Intel Atom ® , 1, 2 or 4 cores C6015 interfaces: 2 Ethernet, 2 USB, 1 DisplayPort C6017 interfaces: 4 Ethernet, 4 USB, 1 DisplayPort main memory: up to 4 GB DDR3L RAM
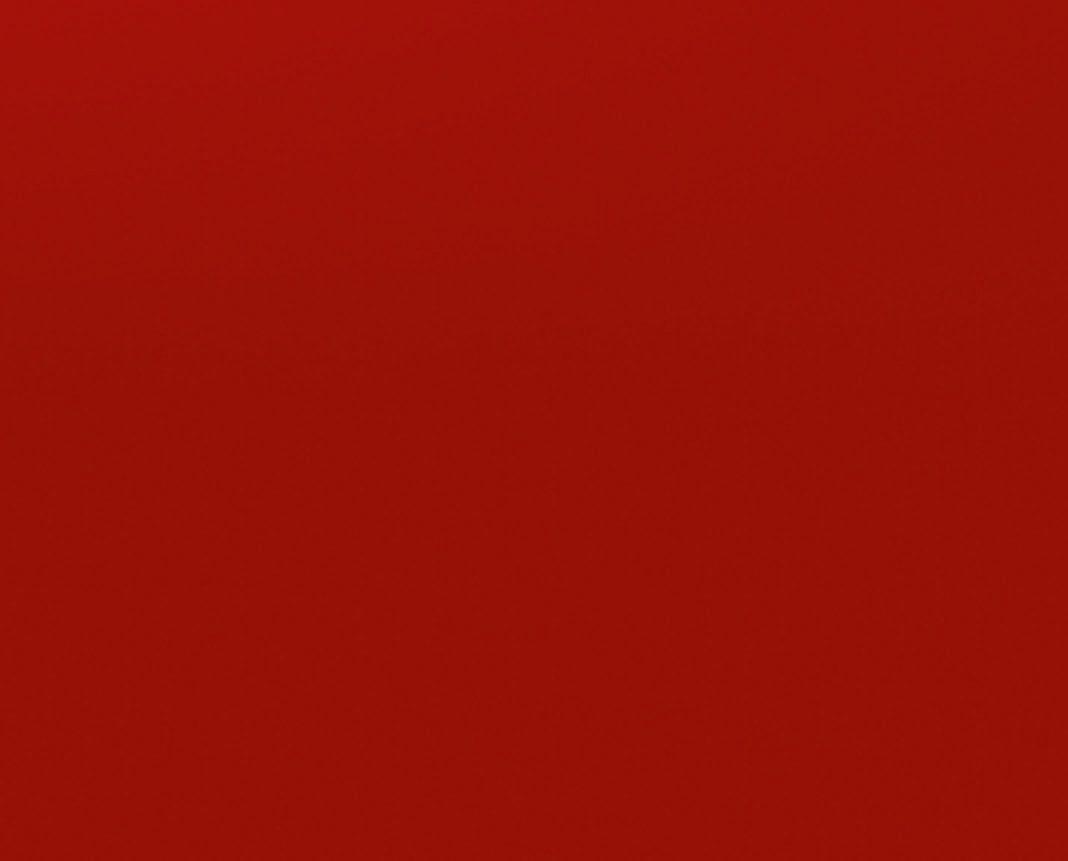
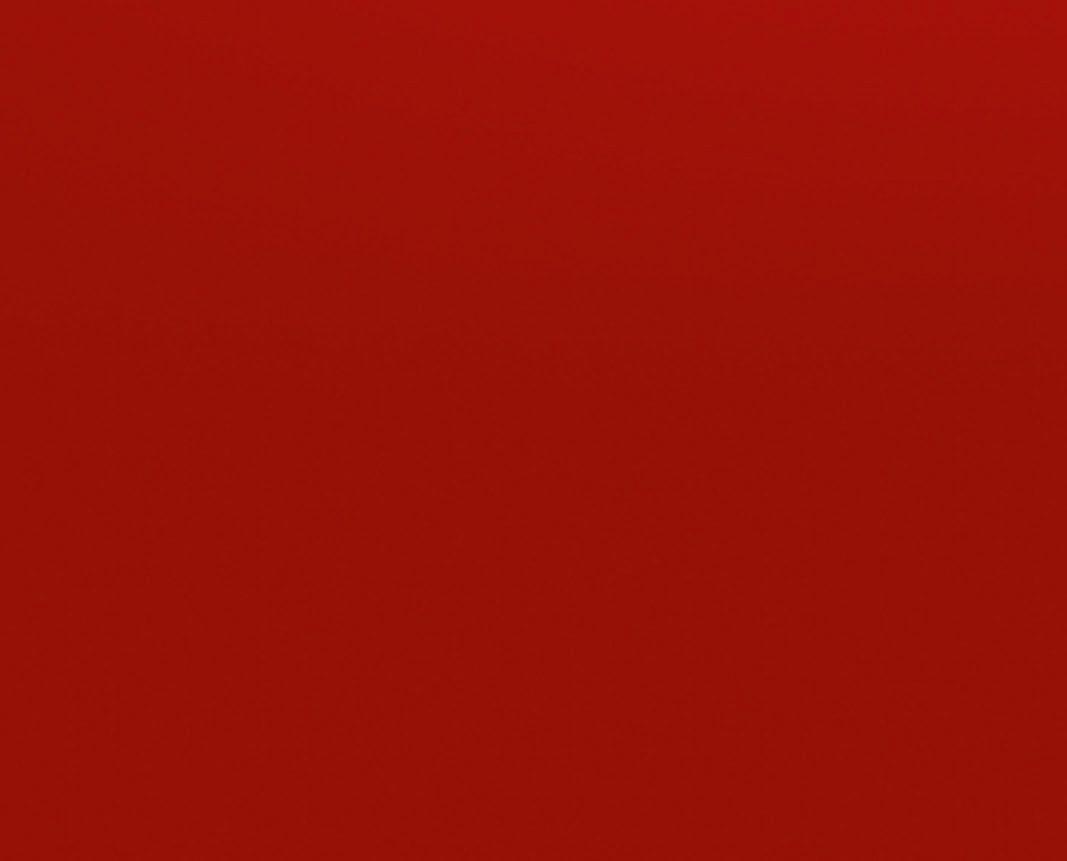
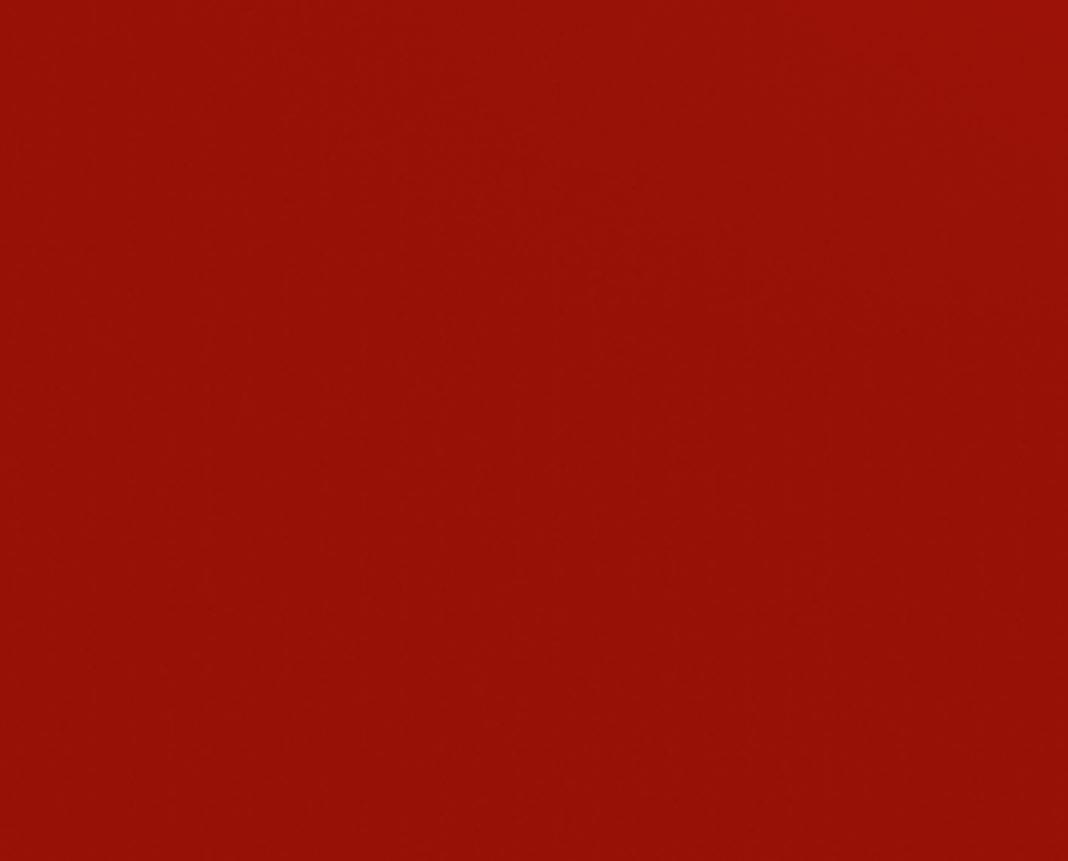
Ultra-high performance: C6030, C6032 processor: up to Intel ® Core™ i7 with four 3.6 GHz cores C6030 interfaces: 4 Ethernet, 4 USB, 2 DisplayPort C6032 interfaces: 4 Ethernet, 4 USB, 2 DisplayPort, 2 PCIe compact module slots main memory: up to 32 GB DDR4 RAM
DATA ANALYSIS
GAINING INSIGHT FROM DATA
Michael Risse believes that there are three main trends driving faster insights from manufacturing big data. B ig data is the raw material used by analytics tools to extract value or insight. Today, the pressure to gain insight from data is so pervasive that ‘analytics’ has become a throw-away term in marketing material for all types of software: visualisation, spreadsheets, business intelligence, and dashboards – and always along with the promise of ‘actionable insights’. And analytics comes in many forms: descriptive, predictive, diagnostic, interactive, prescriptive, basic, real-time, historical, and root cause as examples.
However, research on the state of analytics within manufacturing organisations shows a gap between the current and desired state of adoption. Simply put, organisations do not have timely access to the insights they need to drive improved business and production outcomes. With data volumes increasing, this gap will widen as the data rich but information poor (DRIP) problem gets worse over time.
There are, however, three trends in analytics with the potential to keep up with the innovations in data creation and storage associated with IIoT and big data in general. Process Industries – has the following explanation of the opportunity from new insights in data. ‘Analytics can improve performance tremendously while reducing costs: the value, spread over thousands of opportunities, can be worth tens or even hundreds of millions of euros across a company’s site network… Manufacturers must therefore embark on an analytics-transformation effort that reaches all the way from the shop-floor operators (who steer processes) to process engineers (who use deep insights that will drive the next wave of improvements) to managers (who constantly oversee performance).’
Applying data science innovation to analytics may also be referred to as ‘augmented’ or ‘accelerated’ in the sense that the user experience is improved through innovations that improve the time to outcomes.
A number of scenarios come into focus with the deployment of advanced analytics solutions. For example, predictive analytics on equipment can warn of impending failure. By taking a deeper look into near real-time data, engineers can make small adjustments and shave 10 minutes off a batch cycle time, increasing the annual number of batches by hundreds. Management can receive near real-time alerts about commodity pricing, allowing them to make decisions to boost plant profitability.
The cloud The second change is the application of massive amounts of analytics capacity within the manufacturing sector using the cloud. For the first time, Google, Amazon, and Microsoft all had a significant presence at IHS’s CERA Week event in March Amazon Web Services (AWS) presently has 50 job openings for employees with oil and gas expertise, to add to a roster of existing employees with experience at GE, AVEVA, and other industrial automation vendors. Microsoft and Google are similarly recruiting from across the automation ecosystem.
What this means for manufacturing organisations is more cloud-based options to provide faster time to implement analytics solutions via an elastic infrastructure that can grow and shrink according to customer requirements, along with greater agility and the promises of lower computing costs. While cloud deployment is not a requirement for advanced analytics deployments and many customers either choose or are tethered to on-premise deployments, it brings another opportunity to organisations. This will mean a change
Three trends The first of these is advanced analytics as a new and distinct set of offerings. ‘Advanced’ is the term of choice of industry analyst Gartner and management consulting firm McKinsey & Company. Their definitions for advance analytics focus on the integration of innovations in machine learning, artificial intelligence, and data science into analytics products. The McKinsey report on advanced analytics, for example – Buried Treasure: Advanced Analytics in
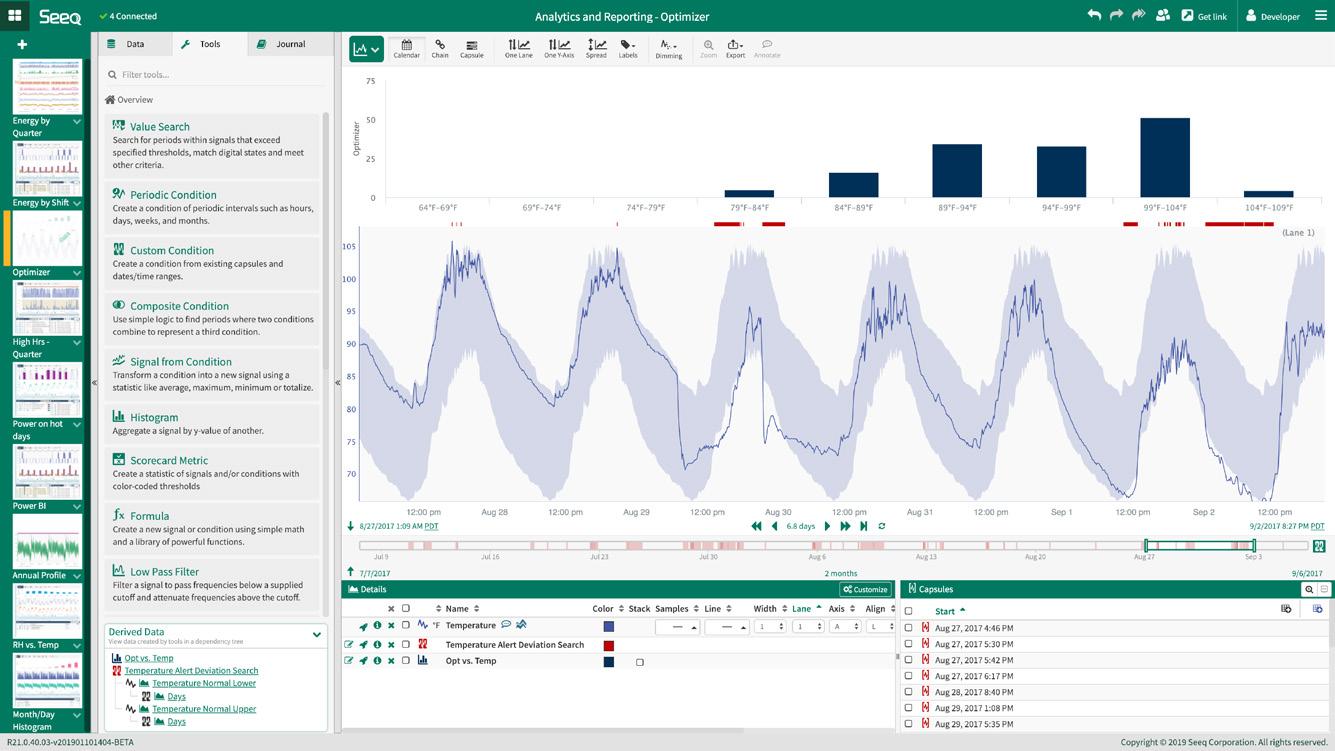
SOMETIMES THE CHOICE IS DIFFICULT …
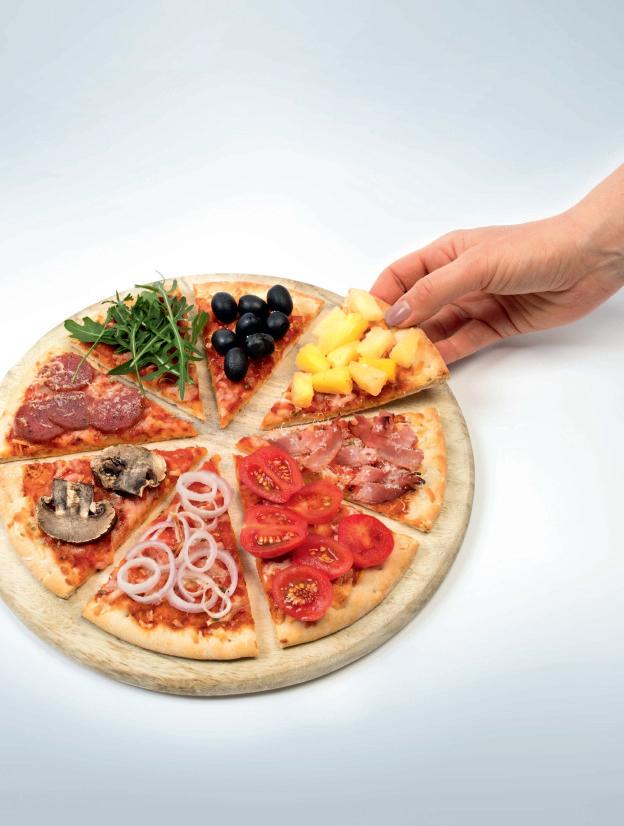