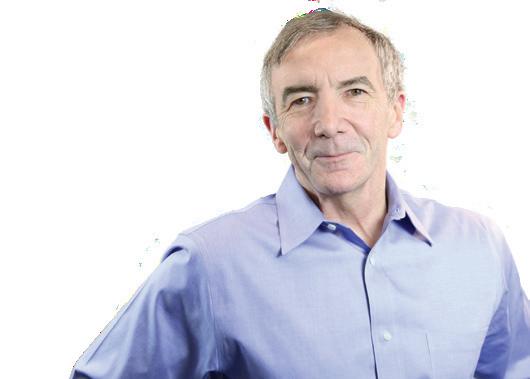
2 minute read
Teschler on Topic
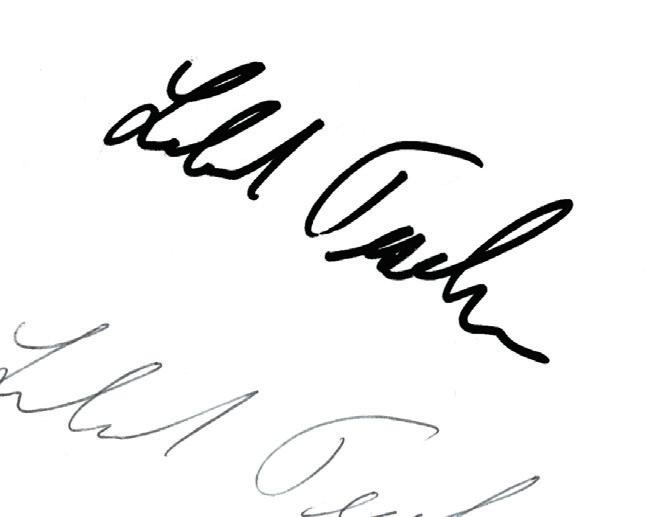
When trust in models leads to bad outcomes
Three cheers for mathematical models, especially those that help sort out complicated engineering problems. No question that engineers emerge from their undergraduate years with a healthy respect for the benefits of modeling. After all, math models are a great help not only in engineering but also in decision making. There they help avoid over confidence and weigh data evenly to help eliminate human biases.
But a misapplication of math models can also lead to dubious results and misleading predictions. It is correct to apply math modeling when the behavior of the system doesn’t change, as in physics where F always equals mA. But all bets are off when people can influence the outcome of the thing you’re trying to model.
Hold this thought as you consider recent proposals for U.S. infrastructure spending. Current plans call for spending $85 billion to modernize transit systems and $80 billion for repairing, improving and expanding Amtrak. Airports, ports and waterways would also get some of the funding.
One major problem with this idea is that uses of transit and transport systems change over time and sometimes drastically so. A cautionary tale can be drawn from a case in Scotland. There consultants put together a model predicting that a tram built between the center of Edinburgh and its nearby airport would easily pay for itself. In fact, the tram project, which is only partly complete, cost about double what was projected and has generated small losses. A public inquiry into the bungled construction has been proceeding for about as long as it took to build the 8.7-mile section.
Somehow, project planners apparently missed the existence of a long-established bus service running between the airport and the city that is cheaper and quicker than the tram would be. Nevertheless, they allowed for uncertainties in their predictions by employing Monte Carlo simulations. As a quick review, Monte Carlo is a way of estimating joint probability distributions of multiple variables when you know each variable’s underlying probability distribution. Importantly, though, probability distributions can be inferred only when the observations used come out of an underlying process that doesn’t change—in the parlance of statistics, it must be stationary.
Edinburgh tram consultants ginned up many sets of alternatives to the construction and operation costs they came up with and generated a probability distribution of possible outcomes predicting all would be well. But critics argue the modelers really had no idea what the underlying probability distributions looked like; it was very much a case of garbage in, garbage out. And a key flaw in these exercises was in assuming the future would resemble the present. The transit situation being modeled was anything but stationary. The result: Costs and revenues of the unfinished tram project came out to be many standard deviations away from what the modelers expected.
That brings us back to U.S. infrastructure spending. Undoubtedly the proponents have prepared models predicting the money will be well spent. But as the Scots learned the hard way, models are only as good as their underlying data, and only as good as their modelers.
Thus when it comes to modeling, the lesson from the recent pandemic is how unstationary the trends and practices in our society really are. DW
Leland Teschler • Executive Editor lteschler@wtwhmedia.com
On Twitter @ DW_LeeTeschler