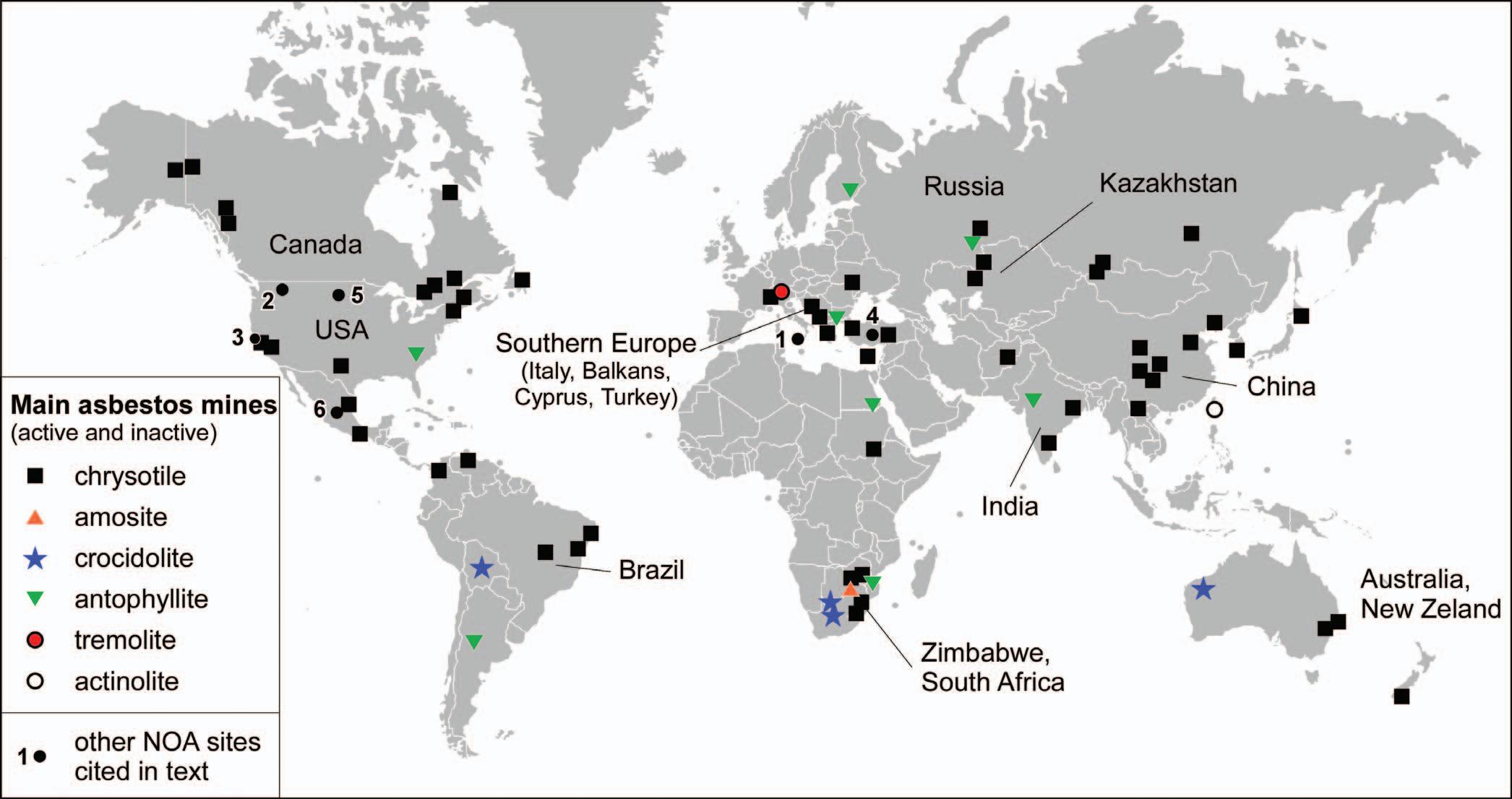
22 minute read
New Tools for the Evaluation of Asbestos-Related Risk during Excavation in an NOA-Rich Geological Setting
from EEG Journal - February 2020 Vol. XXVI, No. I (2)
by Association of Environmental & Engineering Geologists (AEG)
FRANCESCO TURCI* Department of Chemistry and “G. Scansetti” Interdepartmental Center for Studies on Asbestos and Other Toxic Particulates, University of Torino, Via Pietro Giuria, 7, 10125 Torino, Italy
CHIARA AVATANEO SERENA BOTTA IGOR MARCELLI Geological Risk Analysis Gi-RES Srl, Via Gottardo 223, 10154 Torino, Italy
LUCA BARALE National Research Council of Italy, Institute of Geosciences and Earth Resources, Via Valperga Caluso, 35, 10125 Torino, Italy
MAURA TOMATIS Department of Chemistry and “G. Scansetti” Interdepartmental Center for Studies on Asbestos and other Toxic Particulates, University of Torino, Via Pietro Giuria, 7, 10125 Torino, Italy
ROBERTO COSSIO Department of Earth Sciences and “G. Scansetti” Interdepartmental Center for Studies on Asbestos and Other Toxic Particulates, University of Torino, Via Valperga Caluso, 35, 10125 Torino, Italy
SERGIO TALLONE FABRIZIO PIANA National Research Council of Italy, Institute of Geosciences and Earth Resources, Via Valperga Caluso, 35, 10125 Torino, Italy
ROBERTO COMPAGNONI Department of Earth Sciences and “G. Scansetti” Interdepartmental Center for Studies on Asbestos and Other Toxic Particulates, University of Torino, Via Valperga Caluso, 35, 10125 Torino, Italy
Key Terms: Naturally Occurring Asbestos, Asbestos Analysis, Rock Tunneling, Sampling, SEM-EDS, Ligurian Alps, Asbestos
ABSTRACT
The presence of naturally occurring asbestos (NOA) in many areas worldwide requires an enhanced geological risk evaluation to ensure workplace safety from asbestos during large construction projects. Due to the complexity of the geological risk definition, health and safety regulations for working with asbestos-bearing
*Corresponding author email: francesco.turci@unito.it
materials are often not enforceable in NOA settings. Therefore, to correctly estimate the risk of NOA in these scenarios, new procedures are urgently needed to provide (1) a detailed geological model representative of the possible presence of the asbestos, (2) representative sampling, and (3) a reliable quantitative determination of asbestos content in rocks. This work aims to discuss the improvements on the two latter points specifically developed during the design of the “Gronda di Genova” project, a 50-km-long tunnel bypass partially designed in the NOA-bearing meta-ophiolites of the Ligurian Alps and ophiolites of the northern Apennines in Italy. Implementation of Gy’s theory on sampling was used to maintain statistical validity during sample processing from the primary sample to the analytical
Figure 1. Worldwide distribution of active and inactive asbestos mining sites. Some NOA occurrences cited in the text are also indicated: 1, Biancavilla, Italy; 2, Libby, Montana; 3, Franciscan Complex, California; 4, 5, 6, erionite sites in Turkey (Cappadocia), the United States (Dunn County, North Dakota), and Mexico (Tierra Blanca de Abajo), respectively. Data from “Asbestos. Overview and Handling Recommendations” (Deutsche Gesellschaft für Technische Zusammenarbeit, 1996) and Virta (2002).
sample and is here described. The scanning electron microscopy/energy dispersive spectroscopy procedure for the quantification of NOA was improved with an error analysis delivering the minimum number of fibers to be measured to achieve the best analytical results.
INTRODUCTION
Asbestos is a human carcinogen currently causing the majority of occupational lung cancers and several other malignant and non-neoplastic pathologies (International Agency for Research on Cancer, 2012). The occupational exposure to asbestos, a set of six minerals defined by the World Health Organization/International Agency for Research on Cancer, the National Institute for Occupational Safety and Health, the European Union, and many other international agencies worldwide, is unambiguously correlated to an increase of mesothelioma, a fatal malignancy currently causing more than 100,000 deaths per year worldwide. Asbestos are not the only minerals to have a fibrous habit. More than 300 minerals are known to occur in fibrous form (Skinner et al., 1988), and many of them share toxic effects with asbestos (Baumann et al., 2013). A more inclusive definition of these fibers that might have an impact on human health is urgently needed, and the definitions of elongated mineral particles and naturally occurring asbestos (NOA) are currently debated (Gunter, 2018). The term NOA is used here to define asbestos and other fibrous minerals (mainly fibrous antigorite) that occur in the investigated area. In many industrialized countries, the ban on or the strong regulatory limitations to asbestos use have drastically reduced the exposure to asbestos fibers, though many low- and middle-income countries are currently mining and using asbestos. In the western Alps, NOA occur as rockforming minerals in many rocks of the ophiolitic suite, including green-colored serpentinites derived from the hydration of the peridotites. Excavation, tunneling, construction, and generally all anthropic activities carried out in ophiolitic rocks may occasionally release NOA. During unintended or designed disturbance of natural asbestos-rich areas, such as agricultural activities (Turci et al., 2016), road traffic (Baumann et al., 2011; Petriglieri et al., 2019), and tunnel construction (Turci et al., 2015; Gaggero et al., 2017; and Barale et al., this issue), the workers and the population at large might incur occupational or environmental exposure to NOA (Hendrickx, 2009). To gain insight into the possibility of the above scenario occurring worldwide, we could look at geographic areas where asbestos minerals were abundant enough to be commercially exploited (Figure 1). Figure 1 also highlights the
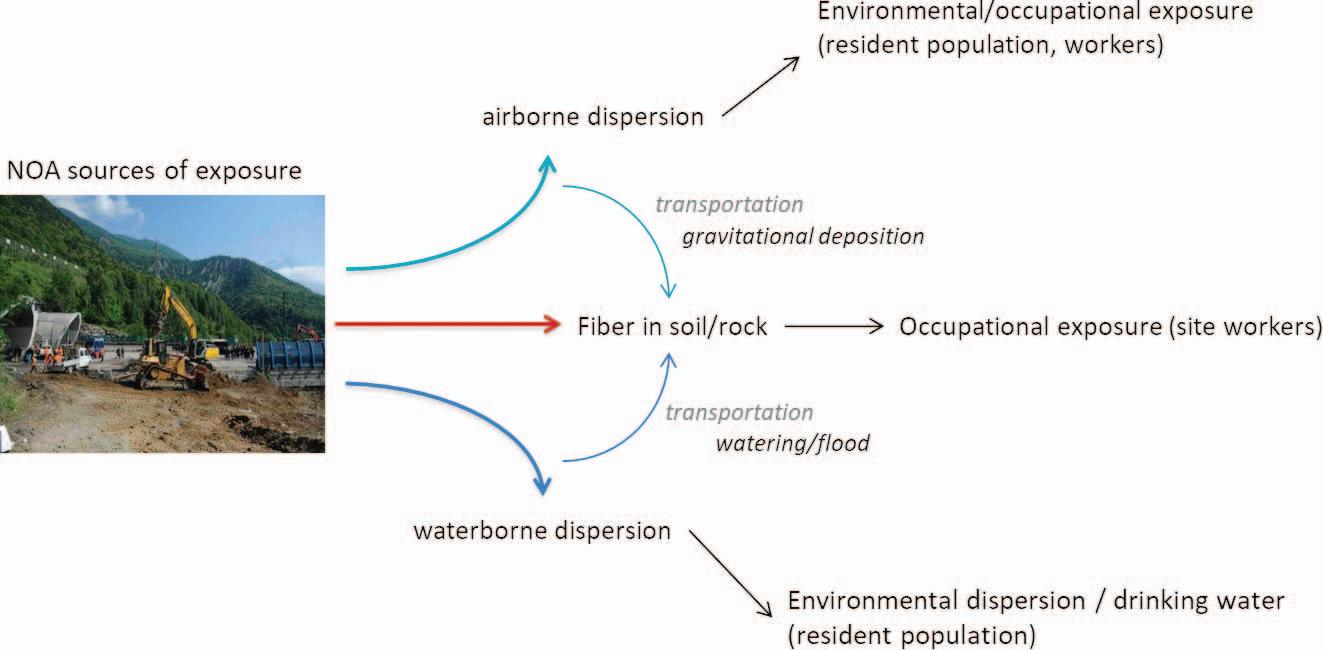
Figure 2. Schematic representation of the possible dispersion routes and human exposures from NOA sources during large construction projects.
occurrence of fibrous minerals that were never exploited commercially but where health-related issues were recorded by mesothelioma epidemiology, including the occurrence of fluoroedenite, in Biancavilla, Sicily, Italy; fibrous amphibole in Libby, Montana; and erionite in Turkey, the United States, and Mexico (Case and Marinaccio, 2017, and references therein). Fibrous glaucophane and winchite, found in the blueschist Franciscan Formation in California, are also marked after the work of Erskine and Bailey (2018).
Although not all fibrous minerals may constitute a risk to human health, NOA risk should properly be assessed (Gualtieri, 2018) during the design and the realization of construction works that may liberate fibers in both the workplace and the environment. Appropriate risk assessment has to be carried out prior to and during excavation/construction in NOA-rich sites. Specifically, NOA mobilization from rock/soil to waters and air and vice versa has to be taken into account (Figure 2), and site-specific health and environmental protection measures have to be enforced to limit airborne and waterborne dispersion of the fibers.
The greatest challenge posed by NOA is indeed due to their spatial localization and quantification in rocks. The first challenge requires the adoption of an asbestos-oriented geological model, such as the approach used for the design of the “Gronda di Genova” highway bypass and described in this special issue (Barale et al., this issue). The NOA quantification in situ generates further challenges, including (1) a harmonized definition of NOA, (2) a reliable sampling methodology, and (3) a proper analytical procedure. The first point is currently under consideration by two independent international panels within the International Mineralogical Association and the International Association of Engineering Geology and the Environment. This work deals with the two latter points in the specific setting of the Gronda di Genova project. A parallel approach can be found in the high-speed railway project designed in a similar geological context (Clerici, 2018).
RESULTS AND DISCUSSION
Sampling Methodology
To obtain a reliable measure, a proper sampling method is required, and although few analysts are aware of the availability of the theory of sampling, it is useful to remember that the analyst’s work is useless if a sample is biased. Specifically, the sample variance of a poorly designed sampling method might easily be up to 1,000%, while analytical methods are usually required to have a variance lower than 1%. All sampling errors derive from the heterogeneity of the material to sample, and the specific case of a NOA-bearing outcrop, the distributional heterogeneity (i.e., the spatial distribution of the constituent elements of the rock with respect to NOA) is very high. NOA minerals are indeed confined in
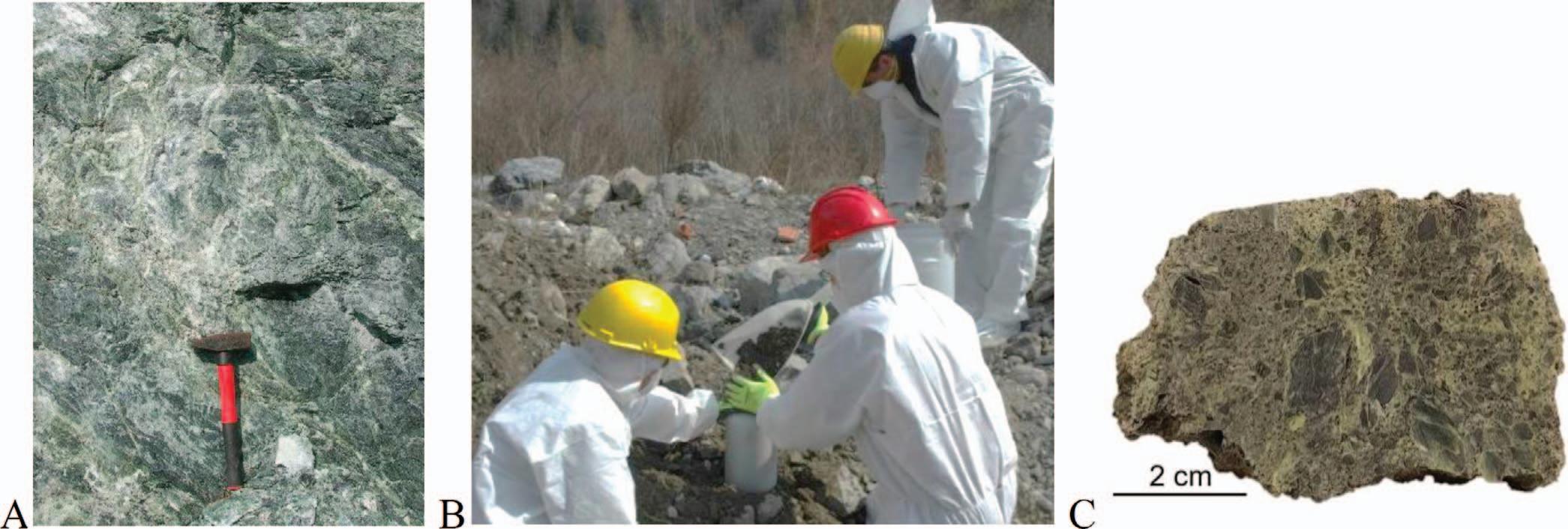
Figure 3. Sampling site (A) and primary field samples (soil and hand sample from drill core, B and C, respectively), from the Gronda di Genova highway bypass.
micrometric veins, irregularly distributed along a network of fractures, within the rock unit. During the evaluation of the NOA occurrence for the Gronda di Genova project, two main scenarios were taken into consideration, and two different sampling approaches were designed: (1) a “preventive evaluation” was used to define, during the preliminary stages of the project, the volume of excavated rocks where NOA could occur above the regulatory limit (Botta et al., 2019), and (2) an “ongoing quantification” was designed to be implemented throughout the project lifetime and to deliver real-time quantitative assessment of NOA in the excavated rock and soil. The sampling methodology adopted during the preventive evaluation, thoroughly described in Barale et al. (this issue), included the definition of site-specific NOA petrofacies. All the lithotypes identified in the study area were represented by at least one sample (logically, more abundant lithotypes were granted a higher number of samples than the rarest ones). The statistical representativeness was achieved by collecting primary samples from the defined petrofacies for each of the structural domains distributed along the tunnel layout. That approach was also followed on portions of drill cores from the geognostic survey as well as on soil samples collected by geologists during the field survey (Figure 3).
In all cases, a reasoned non-random sampling approach was chosen. Despite obvious limitations, a non-stochastic approach offers the advantage of identifying discontinuities between lithotypes and collecting representative portions of each fundamental asbestos-bearing rock type, consisting of recurrent lithological and structural features controlling asbestos occurrence: the NOA petrofacies (Piana et al., 2019). Only a non-stochastic sampling, carried out by a trained geologist, allows the composite sample to be representative of the entire spectrum of homogeneous petrofacies occurring in the primary sample. To take into account such intrinsic heterogeneity, the size and mass reduction steps required to create the analytical sample were carried out with an approach derived from Pierre Gy’s theory (Gy, 1967, 1971, 1998). Gy’s theory and the theory of sampling in general usefully describe heterogeneity with two terms: the constitutional and the distributional heterogeneity. These terms have to be defined to quantify the minimum mass of the analytical sample that correctly represents the primary (or composite) sample collected in the field (Belardi et al., 2018). A detailed discussion of constitutional and distributional heterogeneity in the present case study is beyond the purpose of this work and will be described in a following paper. However, it is known that heterogeneity is related to some fundamental factors of the investigated material. In the case of solid fragments, the heterogeneity can be described by an invariant term H expressed as the product of five descriptive parameters
H = cβ f gd 3 ,
where c is the constitution parameter (g/cm 3 ) and depends on the amount of the analyte of interest and its relative density; fis the shape factor (dimensionless) and depends on the shape of grains; g is the size range factor (dimensionless) and describes the dimensional heterogeneity; and dis the top-particle size (cm), which is also used to calculate β, the liberation parameter (dimensionless), as the square root of the d lib /d ratio, where d lib is the liberation diameter (cm), the size of grains at which all the analyte is liberated. During the Gronda di Genova project, the descriptive factor values were used (Table 1).
As the variance of the sampling error (σ 2 ) is a function of heterogeneity (H), Gy’s approach conveniently correlates the minimum mass of the material to be
Descriptive Factors Factor Description and Range Values Used in the Study
Constitution parameter, c
Particle shape factor, f Size range factor, g
Liberation factor, β
It depends on the mineralogical composition of the material. For a two-component system, the parameter is calculated using the following equation: c = (1−a) a [(1 − a)ρ 1 + aρ 2 ], where a is the expected value of the analyte and ρ 1 and ρ 2 are the analyte and matrix density, respectively.
From round particles (0.4–0.5) to lamellar ones (0.1) From non-calibrated material (0.25) to material selected by means of sieves (0.55). For an analyte free from matrix, the factor is around 0.4–0.8; if the particle top size (d) is higher than the liberation size (d lib ), the factor is around 0.05–0.02. c = 2,697 Setting a as the threshold limit for asbestos detection (1,000 ppm), ρ 1 = 2.7 g/cm 3 (average asbestos density, considering chrysotile more abundant than amphiboles) and ρ 1,2 = 2.7 g/cm 3 (matrix and NOA average density) f= 0.30 g = 0.25
β = 0.05, determined by posing the particle top size
sampled (M S ) with the error introduced during the sampling:
σ 2 ∼ c β f gd 3 M S . As all the descriptive parameters are strictly positive, Gy’s theory allows us to set an acceptable sampling error and obtain the minimum sampling mass (M S ) for a given top-particle size (d). To correctly operate in real-case scenarios, d is often adjusted to meet the practical and theoretical constraints of the system investigated, and sample preparation procedures can be ad hoc designed as a series of alternating size (d) and mass (M S ) reductions (Figure 4A). Specifically, the primary sample collected in the field (ca. 1 kg) was size reduced to d = 1 mm and mass reduced to 16 g; a further size reduction to d = 0.1 mm was completed with a mass reduction to ca. 5 mg (Figure 4B, curve a) obtained by an automatic rotational splitter. The second sampling methodology was designed to collect samples for the quantitative analyses of NOA content throughout the project lifetime. That sampling again had to deal with several types of materials to be collected and required different sampling strategies. Sampling strategies were designed to collect samples mainly from the output slurry of the tunnel boring machine, but ad hoc sampling was designed also for excavation faces, heaps, and drill cores. As per the previous case, the distributional heterogeneity has to be taken into account, and the primary samples have to be reduced by a series of alternating size (d) and mass (M S ) reduction steps (Figure 4B, curves b and c). The recursive steps allow production of a statistically representative analytical sample of few milligrams, suitable for the quantitative scanning electron microscopy/energy dispersive spectroscopy (SEM-EDS) assessment of NOA, from the original 200–300 kg of rocks.
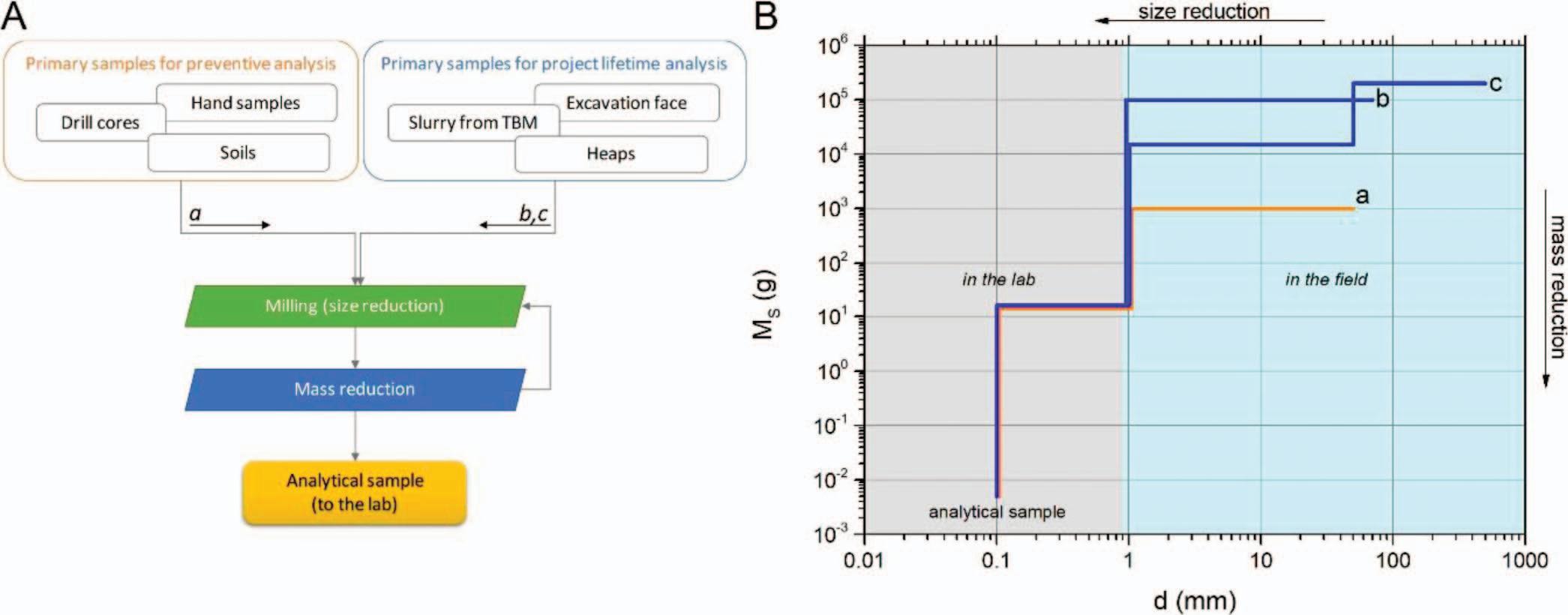
Figure 4. General scheme (A) and project-specific size and mass reduction curves (B) adopted during the study. The analytical samples were obtained from primary samples following Gy’s theory to maintain representativeness in each mass reduction step.
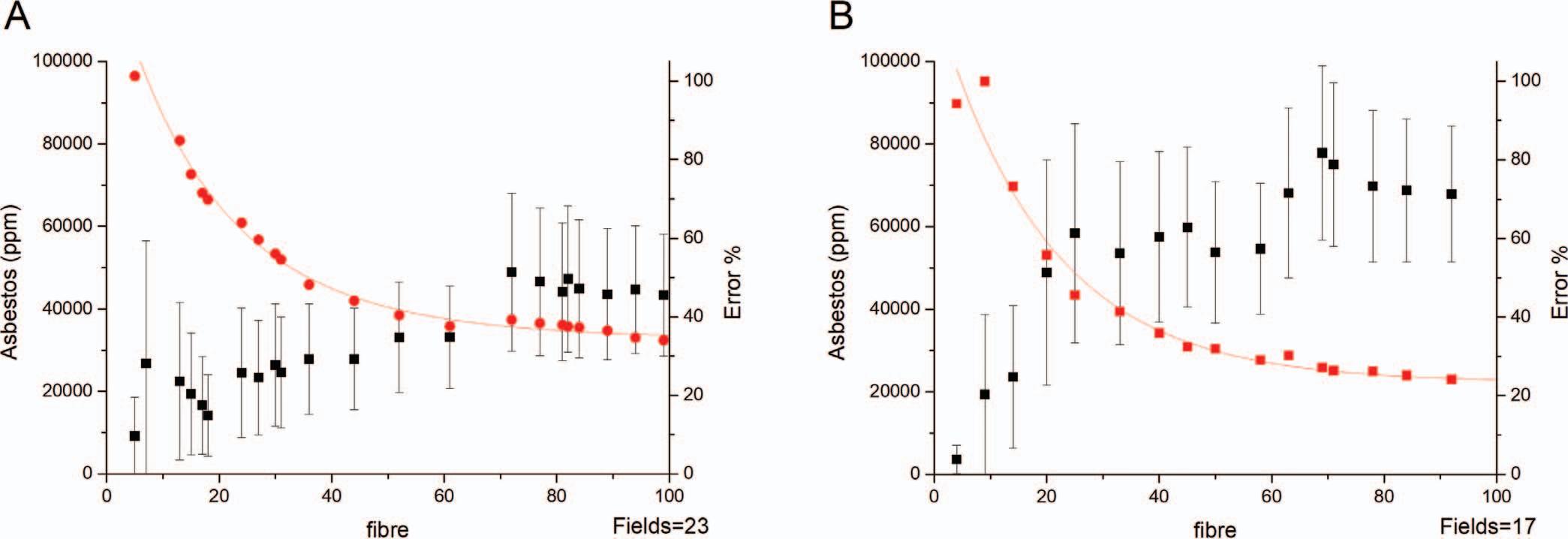
Figure 5. Evolution of the measured NOA concentrations (black squares, ppm) and the relative errors of the measures (red squares, %) plotted against the number of fibers detected on the membrane of two independent samples, A and B. In both cases, when the number of measured fibers (N) increases, the error progressively decreases with a plateau effect and stabilizes on a value that is dependent on the dimensional variability of the fibers on the membrane.
Quantitative Measurement of NOA
The analytical samples obtained were quantified by adopting the only methodology, SEM-EDS, able to discriminate between a mineral with either fibrous or massive habit, to resolve structures of 100–200 nm, to gain insight into the elemental composition of the minerals detected, and to investigate a statistically relevant portion of the sample to achieve 100-ppm sensitivity. The method is described in the Italian legislation on asbestos (DM 6/9/94, Annex 1B), which is similar to ISO 14966 (2002), but it extends the quantification of the fiber concentration from a per-number to a per-mass basis. About 5 mg of the fine analytical sample are weighed and suspended in a water solution containing 0.1% surfactant. A known amount of the suspension is transferred to a polycarbonate membrane, dried, and prepared for electron microscopy. Quantitative NOA analyses are carried out manually by scanning a known area of the membrane and measuring the dimensions (length and diameter) of each NOA fiber detected on the membrane. The observed area of the membrane is clearly a key parameter for the statistical significance of the measure. For each fiber detected, length and diameter are used to approximate the fiber to a cylinder, derive its volume, and calculate its weight by multiplying the volume by the average density of the mineral (2.6 and 3.0 g/cm 3 for serpentine and amphiboles, respectively). Despite SEM-EDS being among the most sensitive quantitative analyses of fibers, it often generates data with a high error due to some instrumental- and sample-related factors. Specifically, (1) due to the manual operational setting, only 0.4% to 0.6% of the membrane can be observed; (2) the discrimination between fibrous and non-fibrous mineral is subjective, and a harmonized definition of fibers is urgently needed; and (3) a fiber or, even worse, an aggregate of fibers cannot always be properly approximated to a cylinder. While the two latter points may be solved by working on the analytical definition of NOA, the former issue can be tackled by analyzing the variation of the error during the measurement phase. The experimental error of quantitative SEMEDS analysis of NOA is essentially due to the fiber sampling statistics generated by reading of the filter, as the number of fibers sampled on a given surface is approximated by a Poisson distribution if the fibers are randomly distributed on the membrane. Furthermore, the error is dependent on the dispersion of the grainsize distribution of the fibers, generally well described by a lognormal distribution. The experimental error C on the fiber concentration C is
C ≈ C × ⎛ ⎜ ⎜ ⎝ 1 √ N + i ( ¯ f− f i ) 2 N· ( N−1 ) ¯ f ⎞ ⎟ ⎟ ⎠ ,
where C is the concentration (ppm) of the fibers in the sample; N the number of identified fibers; ¯ f is the average weight of an asbestos fiber determined as the average of the weights of the identified N asbestos fibers; and f i the weight of the ith counted fiber. It can be observed that the main contribution to the error is due to the number of fibers analyzed for low numbers of fibers (N < 30). As the number of fibers increases, the contribution of the term 1/ √ N becomes negligible with respect to the standard deviation included in the second term of the equation. The standard deviation is still influenced by the

Figure 6. SEM images of (A) a representative NOA complex aggregate of fibers and (B) an asbestos bundle of uncertain diameter and apparent density evidenced during the quantitative analysis of NOA in ophiolitic rock from the Gronda di Genova project.
number of fibers detected, but it strongly depends on the dispersion of the weights of the individual fibers analyzed ( ¯ f − f i ) 2 . The dispersion of the weights is intrinsically related to the dimensional variability of the sample on the membrane, and it can be reduced only by narrowing the particle size distribution during the preparation phases. To evaluate the evolution of the experimental error, the NOA concentrations (black squares) and the relative errors of the measures (red squares) are reported as a function of the number of the fibers detected (Figure 5). As the number of the fibers measured increases, the calculated NOA concentration varies quite unpredictably due to inclusion of outliers in the distribution. Conversely, the relative error of the measure progressively decreases with the increase in measured fibers, producing a plateau effect, and stabilizes to a value that is independent on the number of the fibers detected; that is, it cannot be further reduced by analyzing a larger portion of the membrane. For large numbers of fibers, the error is indeed related only to the dimensional variability of the fiber masses, hence their sizes, described by the second term of the error equation. By performing the error evaluation in parallel with SEM-EDS measurement, we estimated the minimum number of fibers to be analyzed to achieve the best analytical result in terms of experimental error of the measure.
The error-minimizing approach can be particularly effective if integrated in unattended SEM-EDS analyses of asbestos concentrations, described in a previous work (Cossio et al., 2018).
To further increase the reliability and interlaboratory reproducibility of SEM-EDS quantitative analysis of NOA, a few other issues have to be solved. Future research will include the non-trivial approximation to a geometrical shape of complex fiber aggregates, which often occur during microscopic analysis (Figure 6A), and the apparent density and the diameter of large bundles, in which inter-crystal spaces cannot be disregarded when evaluating the total bundle volume (Figure 6B).
CONCLUSIONS
To correctly predict the risk of naturally occurring asbestos in large construction projects, a methodology for reliable sampling and quantitative analysis has to be sought. In this article, we described the approaches used in the Gronda di Genova highway bypass to achieve a reliable sampling statistic and methodology to minimize the analytical error during SEM-EDS quantitative analysis of NOA. An implementation of Gy’s theory allowed us to define the minimum quantity of sample and the size and mass reduction curves to maintain statistical representativeness throughout the sample processing steps. An error-minimizing approach was adopted by investigating the variation of error during the NOA analysis and allowed us to optimize the analytical time without affecting accuracy.
ACKNOWLEDGMENTS
This research was developed in the frame of the Gronda di Genova research project between University of Torino, Institute of Environmental Geology and Geoengineering-National Research Council (IGGCNR), GiRES srl and SPEA Engineering S.p.A., Genoa, Italy, under contract no. 400006258/2018. The authors are indebted to Dr. G. Belardi,
Dr. F. Trapasso, and Dr. D. Passeri, IGG-CNR, Monterotondo, Rome, for carrying out comminution experiments and for the fruitful discussion on the theory of sampling. The authors kindly acknowledge Dr. J. R. Petriglieri for reviewing the manuscript. The second author is indebted to Agenzia Regionale per la Protezione Ambientale (ARPA) Valle d’Aosta for funding her scholarship.
REFERENCES
Barale, L.; Piana, F.; Compagnoni, R.; Tallone, S.; Avataneo, C.; Botta, S.; Irace, A.; Marcelli, I.; Cossio, R.; Mosca, P.; and Turci, F., 2019, Geological model for NOA content prediction in the rock excavation of a long tunnel (“Gronda di Genoa” project—NW Italy): Environmental and Engineering Geoscience, this issue. Baumann, F.; Ambrosi, J.-P.; and Carbone, M., 2013, Asbestos is not just asbestos: An unrecognised health hazard: Lancet Oncology, Vol. 14, No. 7, pp. 576–578. Baumann, F.; Maurizot, P.; Mangeas, M.; Ambrosi, J.-P.; Douwes, J.; and Robineau, B. P., 2011, Pleural mesothelioma in New Caledonia: Associations with environmental risk factors: Environmental. Health Perspectives, Vol. 119, No. 5, pp. 695–700. Belardi, G.; Vignaroli, G.; Trapasso, F.; Pacella, A.; and Passeri, D., 2018, Detecting asbestos fibres and cleavage fragments produced after mechanical tests on ophiolite rocks: Clues for the asbestos hazard evaluation: Journal of Mediterranean Earth Sciences, Vol. 10, pp. 63–78. Botta, S.; Avataneo, C.; Barale, L.; Compagnoni, R.; Cossio, R.; Marcelli, I.; Piana, F.; Tallone, S.; and Turci, F., 2019, Petrofacies for the prediction of NOA content in rocks: Application to the “Gronda di Genova” tunnelling project: Bulletin of Engineering Geology and the Environment, pp. 1–20. Case, B. W. and Marinaccio, A., 2017, Epidemiological approaches to health effects of mineral fibres: Development of knowledge and current practice. In A. F. Gualtieri (Editor), Mineral Fibres: Crystal Chemistry, Chemical-Physical Properties, Biological Interaction and Toxicity, Vol. 18: EMU Book, London, UK, pp. 367–416. Clerici, C., 2018, Procedure di campionamento del fronte nello scavo di gallerie con l’eventuale presenza di rocce potenzialmente contenenti amianto. In: Protocollo Gestione Amianto per Il Terzo Valico Ferroviario Dei Giovi: Osservatorio Ambientale per il Terzo Valico Ferroviario dei Giovi, Alessandria (I), pp. 1–32. (In Italian) Cossio, R.; Albonico, C.; Zanella, A.; Fraterrigo-Garofalo, S.; Avataneo, C.; Compagnoni, R.; and Turci, F., 2018, Innovative unattended SEM-EDS analysis for asbestos fiber quantification: Talanta, Vol. 190, pp. 158–166. Decreto Ministeriale 6/9/94, Ministero della Sanità (G.U. n.288). Annex 1: Normative e metodologie tecniche per la valutazione del rischio, la bonifica, il controllo e la manutenzione dei materiali contenenti amianto presenti negli edifici (in Italian) Deutsche Gesellschaft für Technische Zusammenarbeit, 1996, Asbestos: Overview and Handling Recommendations: Vieweg, Braunschweig (D), 203 p. Hendrickx, M., 2009, Naturally occurring asbestos in eastern Australia: A review of geological occurrence, disturbance and mesothelioma risk: Environmental Geology, Vol. 57, pp. 909–926. Internationsl Standard Organization ISO 14966, 2002 Ambient air: determination of numerical concentration of inorganic fibrous particle – scanning electron microscopy method. Erskine, B. G. and Bailey, M., 2018, Characterization of asbestiform glaucophane-winchite in the Franciscan Complex blueschist, northern Diablo Range, California: Toxicology and Applied Pharmacology, Vol. 361, pp. 3–13. Gaggero, L.; Sanguineti, E.; Yus González, A.; Militello, G. M.; Scuderi, A.; and Parisi, G., 2017, Airborne asbestos fibres monitoring in tunnel excavation: Journal of Environmental Management, Vol. 196, pp. 583–593. Gualtieri, A. F., 2018, Towards a quantitative model to predict the toxicity/pathogenicity potential of mineral fibers: Toxicology and Applied Pharmacology, Vol. 361, pp. 89–98. Gunter, M. E., 2018, Elongate mineral particles in the natural environment. Toxicology and Applied Pharmacology, Vol. 361, pp. 157–164. Gy, P., 1967, L’échantillonnage des minerais en vrac, Tome 1: Societe de l’industrie minerale, Paris. Gy, P., 1971, L’échantillonnage des minerais en vrac, Tome 2: Societe de l’industrie minerale, Paris. Gy, P., 1998, Sampling for Analytical Purposes: John Wiley and Sons, New York, 172 p. International Agency for Research on Cancer, 2012, Asbestos (chrysotile, amosite, crocidolite, tremolite, actinolite, and anthophyllite): IARC Monographs on the Evaluation of Carcinogenic Risks to Humans, Vol. 100C, pp. 219–309. Petriglieri, J. R.; Laporte-Magoni, C.; Gunkel-Grillon, P.; Tribaudino, M.; Bersani, D.; Sala, O.; Le Mestre, M.; Vigliaturo, R.; Bursi Gandolfi, N.; and SalvioliMariani, E., 2019, Mineral fibres and environmental monitoring: a comparison of different analytical strategies in New Caledonia: Geoscience Frontiers, in press. Piana, F.; Barale, L.; Botta, S.; Compagnoni, R.; Fidelibus, C.; Tallone, S.; Avataneo, C.; Cossio, R.; and Turci, F., 2019, Direct and indirect assessment of the amount of naturally occurring asbestos in fractured rocks. Accepted for publication Bol. Geo Min. Skinner, H. C. W.; Ross, M.; and Frondel, C., 1988, Asbestos and Other Fibrous Materials: Mineralogy, Crystal Chemistry, and Health Effects: Oxford University Press, Oxford. Turci, F.; Compagnoni, R.; Piana, F.; Delle Piane, L.; Tomatis, M.; Fubini, B.; Tallone, S.; Fuoco, S.; and Bergamini, M., 2015, Geological and analytical procedures for the evaluation of asbestos-related risk in underground and surface rock excavation. In: Engineering Geology for Society and Territory, Vol. 5: Springer, Berlin, pp. 619–622. Turci, F.; Favero-longo, S. E.; Gazzano, C.; Tomatis, M.; Gentile-Garofalo, L.; and Bergamini, M., 2016, Assessment of asbestos exposure during a simulated agricultural activity in the proximity of the former asbestos mine of Balangero, Italy: Journal of Hazardous Materials, Vol. 308, pp. 321–327. Virta, R. L., 2002, Asbestos: Geology, Mineralogy, Mining, and Uses: U.S. Department of the Interior, U.S. Geological Survey, Washington, DC, 28 p.