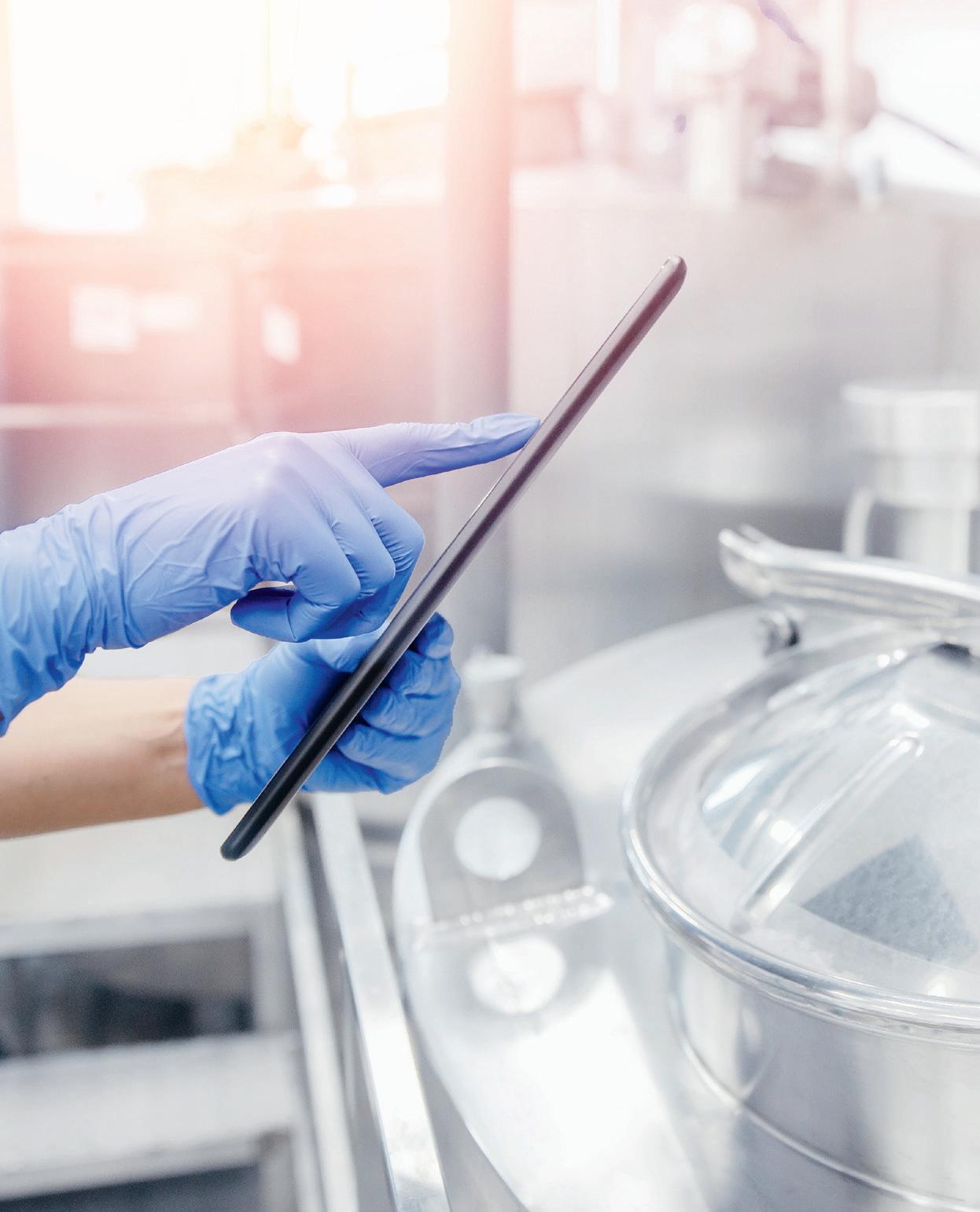
14 minute read
Digital twins: Digital twins in baking process automation
from f2m Automation Book
by landmagd
Digital twins in baking process automation
In a digitization project, it is important to first consider several use cases, because they typically require the most effort, while the implementation of additional use cases promises additional revenue at a lower cost.
+What is a digital twin? The industry has seen a couple of periods, each of which introduced innovations leading to a substantial increase in productivity. The introduction of mechanical machines, mass production and electrification and finally automation using electronics and information technology were previous such periods. Now we are in the era of digitization, the advent of which had already been foreseen in 1991 (1) and which is a core innovation of Industry 4.0, a high-tech strategy originally developed in Germany (2). Digitization is about creating digital twins of physical objects, maintaining them, and using them for improving processes related to these objects.
A digital twin encompasses all possible information about a product, its production and possibly also its performance throughout its complete lifecycle. Let us illustrate this with bread production: + The general digital twin of a batch of bread may consist of the base recipe, the formulation related to a specific production line and any kind of data collected during production, analytics, and finally transport and distribution.
It may also include simulation models relevant for product design or production, and even consumer feedback may be part of it. + The production line in a bakery producing this bread also has a digital twin. It is a combination of digital twins of its stations and machines. These may include 3-dimensional CAD models used for hardware design; simulation models of different granularity ranging from detailed 3-dimensional simulations to 1-dimensional process flow simulations; models of its control to be used in e.g. production design and model-predictive control; a track of data is collected during operation for monitoring and analytics.
The benefit of using digital twins is manifold: The design of a product and its production can be made faster and cheaper using simulation instead of prototyping; the degree of automation can be increased up to autonomous production (3); the transparency of production can be increased leading to more informed and optimal decisions; processes in the supply chain or related to audits can be simplified substantially.
In most cases, the main hurdle is the collection of sufficient data points and the integration of several, usually isolated data sources. Digital twins can be stored and used in the cloud, on-premise, but can also be embedded (4). A particular decision typically depends on requirements concerning data privacy and real-time performance. Using digital twins in the cloud is becoming more and more attractive, especially for remote monitoring, analysis and computationally extensive
applications1. On-premise infrastructure is not available everywhere; it is typically a choice for those who don’t trust cloud solutions. Using digital twins in embedded systems is recommended for applications where a rapid and reliable reaction is needed; examples are preprocessing key performance indicators (KPIs) for real-time monitoring or image pre-processing.
In the following, we will present examples from projects co-funded by EIT Food2, an innovation initiative funded by the European Institute of Innovation and Technology (EIT), and from a customer project at Siemens.
Collecting data from production
A very important step in a digitization project is the collection of data from production. The effort and time needed for this strongly depend on the existing automation infrastructure. A necessary precondition is accessible communication links to sensors or controllers. Not having that implies an investment in the corresponding infrastructure like controllers and an automation network. Here, we want to present an example showing how fast and seamless the collection of data can be carried out even in brownfield if this precondition is fulfilled.
In 2018, Nestlé Wagner GmbH asked Siemens for support in enhancing the efficiency of the existing machine park through the changeover to Industry 4.0 in accordance with the company's productivity policies, and at a reasonable cost. A proof of concept was implemented in the plant in Nonnweiler-Otzenhausen, Saarland, Germany, where amongst others the popular Wagner stone-baked pizza specialties are produced. The following requirements had to be fulfilled: + No modifications of machine controller are allowed. This would inevitably result in a change of the machine software and could cause unexpected machine behavior. + The new system must comply with the Nestlé
Wagner safety regulations. + No cloud-based solution may be used. All data must be stored locally. + It must be compliant with the General Data
Protection Regulation (GDPR). + The tool must be integrable into corporate IT. + It must be quickly integrable within the structure and be able to quickly supply data. + The system must be easily and intuitively usable.
The solution is based on SIMATIC WinCC OA IOT Suite3 combining reliable, industrial-grade hardware with proven software and intuitive apps for establishing connections, setting controllers, and displaying information. The proof of concept deployed at the production line of Nestlé Wagner is currently comprised of a central system controller, the so-called On Premise Administration (OPA) and the IOT box. OPA hosts system infrastructure including system administration and apps, equipment, and updates management. The IOT box is connected to a SIEMENS S7 controller through an Ethernet network, and directly processes the collected values and feeds them to the appropriate apps. Two apps are currently in use at Nestlé Wagner: one displaying the machine performance indicators and another, a dashboard app, which is freely configurable. The technician or person in charge of the machine opens the app and can view the current values on their tablet (s. Figure 1).
The IOT box can also be connected to different other devices for collecting their data and integrating them into a consistent data model. In most cases, data are collected from PLCs, but there are also drivers available for communicating directly with e.g. sensors. Data collection and pre-processing is directly configured; the corresponding apps providing functionality are downloaded to the IOT box. The apps calculate
1 https://news.microsoft.com/2021/05/13/mars-and-microsoft-work-together-to-accelerate-mars-digital-transformation-and1 reimagine-business-operations-associate-experience-and-consumer-engagement/ 2 www.eitfood.eu/ 3 https://new.siemens.com/global/en/products/automation/industry-software/automation-software/scada/simatic-wincc-oa/ 1 simatic-wincc-oa-iot-suite.html
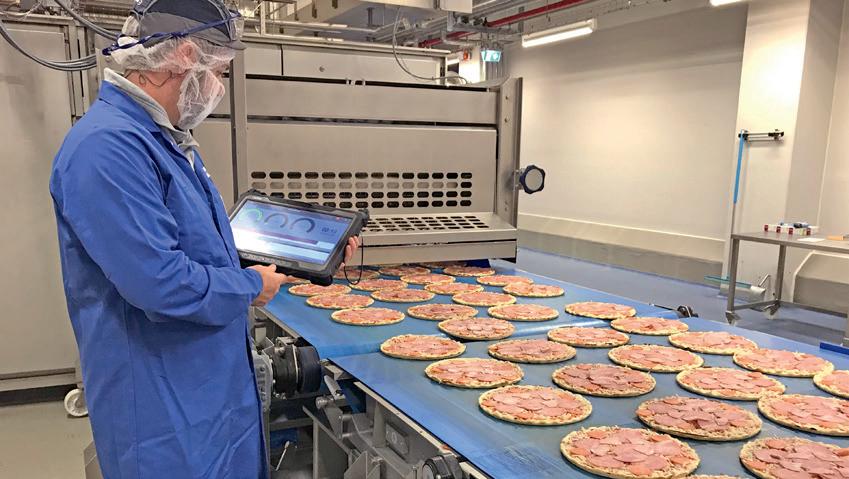
© Siemens Figure 1: Data retrieved from a production line at Nestlé Wagner GmbH are shown on a tablet
different KPIs from e.g. the shift and provide them as aggregated data. Commissioning the IOT box for this application entails the integration of data from different PLCs, setup of the apps and providing them with the appropriate data. Through the ease of use, this process can be done by an electrician in less than one hour.
Nestlé Wagner is planning to deploy IOT Suite also on the remaining lines comprising production, packaging and reader equipment. Apart from gaining transparency on their production, a major goal is to reduce machine faults by getting more transparency through the data collection and used apps.
Digital twins of product and production
A digital twin of production has been used in HealthSnaP⁴, an innovation project co-funded by EIT Food. This project aimed at supporting healthy eating by developing a new food manufacturing technology and B2C business concept for at-site customized production of healthy snacks. The result was a prototype of a machine of the size of a typical vending machine hosting a complete production line for snack production. The production process starts with collecting all needed ingredients, mixing them, extruding sticks to a transportation plate, baking the sticks in an oven, and putting them into a cup. The cup – and an additional dip – are collected by the consumer in the end.
Figure 2 shows the simplified layout of the machine. On the left in the background are the containers (green) for the ingredients water, oil, premixed dry base ingredients and optional boosts. In the foreground are the mixing and extrusion unit (blue). On the right side in the
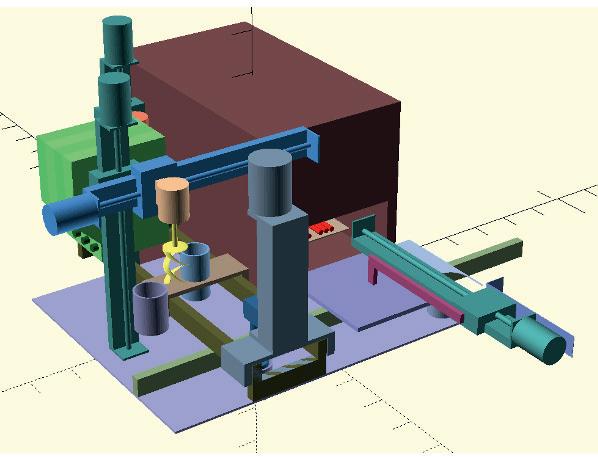
© Siemens Figure 2: Simplified 3D-layout of the machine prototype
4 www.eitfood.eu/projects/health-snap
INTERVIEW DIGITAL TWINS IN BAKING PROCESS AUTOMATION
Velocity
Pressure
Die Angle 60°
90°
120° 1 mm/s 3 mm/s 5 mm/s
kPa
53.0 (45.9)
46.8 (43.9)
48.6 (49.4) kPa
91.9 (82.4)
81.3 (87.8)
84.4 (82.3) kPa
119.0 (125.3)
105.08 (107.3)
109.0 (106.8)
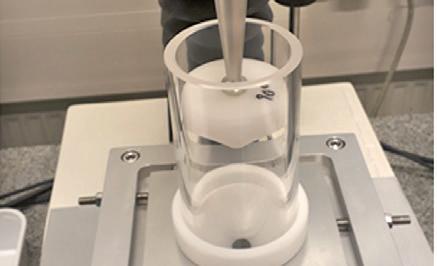
Figure 3: (left) comparison of measured mean extrusion pressure, in brackets, and mean extrusion pressure derived from the numerical simulations; (right) experimental setup for extrusion measurements for an extrusion angle of 90° background is the oven (brown) and in front of it is the unit moving snack sticks into and out of the oven and into the cup.
The 3D CAD-model not only contains shapes and dimensions of all components, but also a semantic context for them. This imposes constraints on the position of components and on their dimensions. For example, the maximum number and size of the snack sticks impose a minimum size of the mixing cup; this determines the size of the mixer and the extruder and – consequently – the size of the cup for cleaning the mixer and extruder. The 3D CAD-model also allows for simulations of the motions of the components. Any potential collision of components enforces a new arrangement of all components. This can be done automatically using a suitable solver for all these constraint equations. The benefit is a much faster design process.
Some components must be designed specifically for the product type. A good example is the mixing and extrusion unit. In the first phase of the design, a single hole extruder has been studied experimentally to evaluate the effect of the extrusion speed and die angle on the resulting dough sticks. The experimental setup is shown in Figure 3 right for an extrusion angle of 90°. A parametric model of a quarter of the extruder was created using the Siemens StarCCM+⁵ simulation solver. The die-angle is parametrized and a new model for a new angle can be generated with a few mouse clicks. The experimental measurements of extrusion speed and extrusion force were used to calibrate a Power-Law model for non-Newtonian fluids (5). The results obtained for three die angles and three extrusion speeds show a good agreement with the measurements with a maximum error of 15%. The results are displayed in Figure 3 left. The numerical results are used to determine at which exit speed (different from the extrusion speed) the sticks start to sag, and the ‘cylindrical’ shape is compromised. In this way, an optimal exit speed is determined which can be used for evaluating different designs. For more accurate analysis and complex extrusion geometries, precise calibration of the material model is needed. This is achieved using rheology measurements of the dough which was not possible in this project.
Simulations also help in determining optimal parameters for production steps. This has been done for the baking process, also with Siemens StarCCM+. To support the production steps, the baking process in the oven has been simulated in three dimensions including models for the baking plate and the bread sticks. To reduce model size only half of the oven was modeled, as a symmetric set-up was assumed. The bread sticks’ properties, as well as the air properties, are temperaturedependent. The sticks are considered solid and no deformation is allowed. The cross-section of the sticks allows for contact between the sticks and the plate. The model setup is presented in Figure 4 (left). The condition for the sticks to be reached in the baking process (=‘baked condition’)
5 www.plm.automation.siemens.com/global/en/products/simcenter/STAR-CCM.html
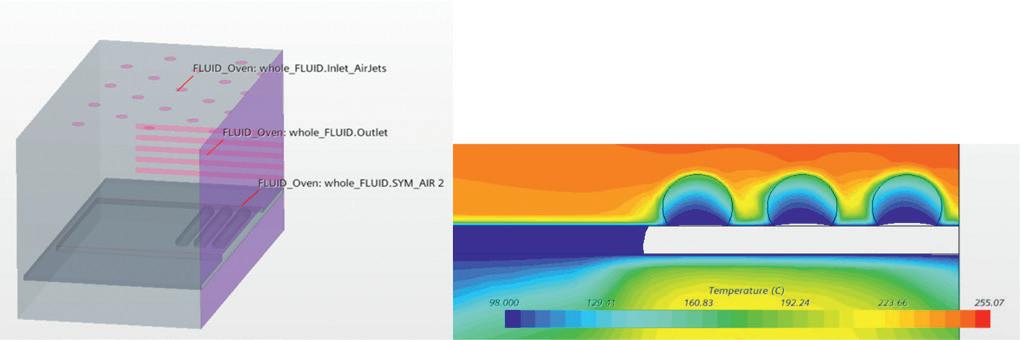
© Siemens
has been defined as having a temperature above 98°C everywhere and between 150 and 180°C on the crust to obtain a golden color, but avoiding burning. Using this definition several scenarios were investigated. The aim was to determine the necessary baking time for the sticks under different starting conditions (dough temperature, plate temperature, oven air temperature). A target baking time of under 60 seconds was set from the requirements coming from the whole machine design.
In the first phase a dummy material for the baking tray was assumed, as the final configuration was not yet specified and time would not be lost in setting up the model. Based on this model the digital twin simulation model was generated and possible scenarios to be investigated were defined. Once the final material for the tray was defined, the simulations were executed in less than one day. A minimum baking time of 35 seconds was necessary to get the golden color on the sticks. The baking time was driven by the crust conditions, if the aluminum plate inside the oven was already hot (the machine has been working for some time) and by the temperature at the contact between plate and sticks, if the plate was well below oven temperature (first batch after a long pause). Figure 4 (right) presents the configuration a few seconds before the baked condition was reached, the part of the sticks in contact with the plate is the last to reach baked conditions. For all investigated conditions a baked condition could be reached in a maximum of 52 seconds. The baking time could be made dependent on the oven conditions (continuous usage or paused).
Finally, 1d-process flow simulations using Tecnomatix Plant Simulation from Siemens allow for the evaluation of the design and investigating
Figure 4: (left) Simulation setup. The hot air injection holes are visible at the top. The three bread sticks lay on a removable Teflon plate on top of a fixed aluminum tray. (right) Mid-section of the sticks temperature at reached baked conditions. White areas represent temperatures below 98°C
Figure 5: Material flow simulation model of a production process
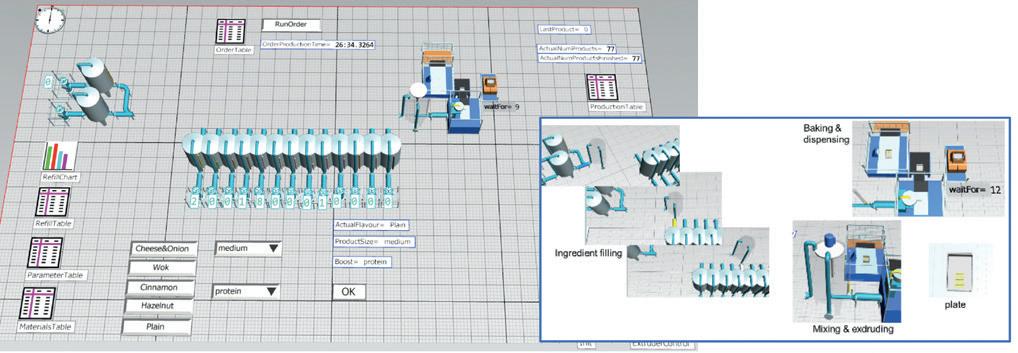
© Siemens
INTERVIEW DIGITAL TWINS IN BAKING PROCESS AUTOMATION
design options (see Figure 5). Based on the recipes with the individual customized ingredients the production schedule can be dynamically defined and tested. With the configuration of specific process parameters like motor speeds or conveyor velocities, as well as variants in the cleaning process such as by an additional mixer or in the dispensing process with several plates, the production time of a specific product can be evaluated. Also, with this, stress tests by a specific
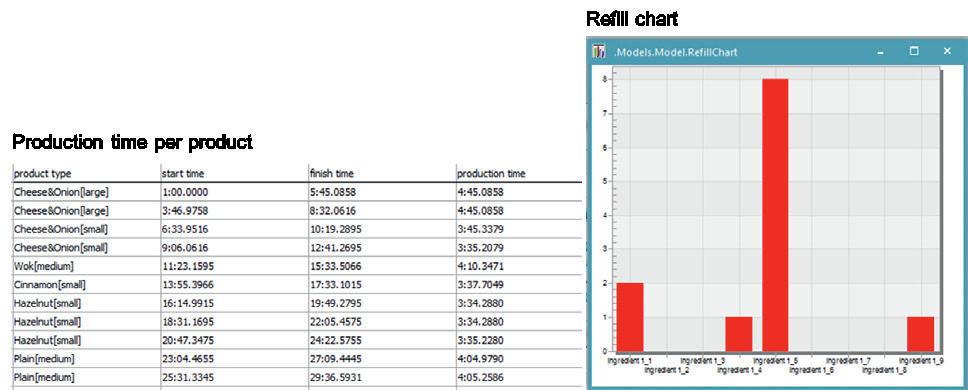
Figure 6: Some simulation results, e.g. production time and necessary ingredient refill for a given number of orders
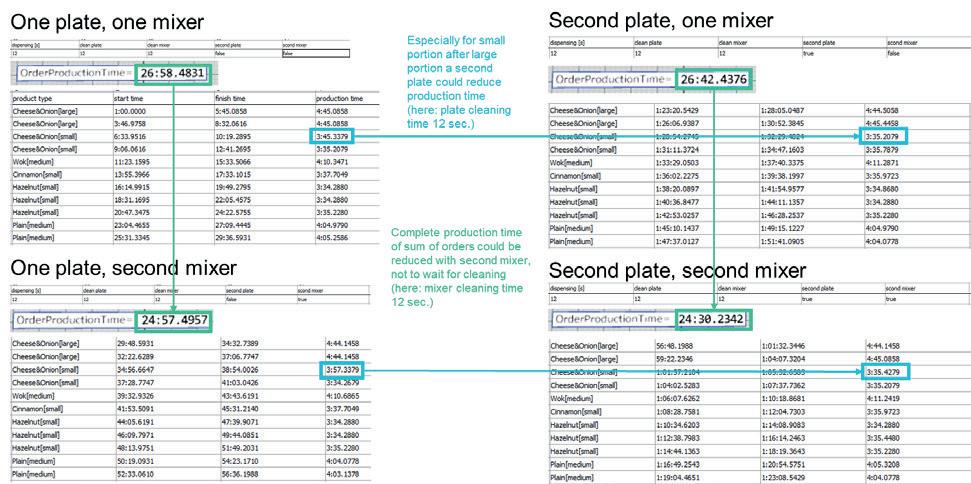
Figure 7: Example of some experiments and their interpretation
combination of orders are possible in order to identify ingredient availability and bottlenecks in material and process flow.
Results from simulation such as production time or refill chart (see Figure 6) can then be used to improve the process. Figure 7 shows an evaluation of adding a second plate or a second mixer. While a second plate does not improve production time substantially, a second mixer can reduce the order production time by about 8%.
This evaluation gives hints for process optimization regarding design decisions: Adding a second mixer shortens the total production time for the orders listed in Figure 7 by about two minutes corresponding to about 8%. Adding a second plate has a minor effect on the total production time but can notably reduce production time for a small portion produced after a large portion; for the marked example in Figure 7 this is a reduction of 10 seconds in the case of one mixer and about 20 seconds in the case of a second mixer, corresponding to about 5%, respectively 10%.
There are plenty of other applications for such simulations: Examples are (i) determining optimal dimensions of ingredient containers, (ii) handling of production processes with the prediction of production time for a customer's order or (iii) giving alarms in advance e.g. in case of refill.
+++
Acknowledgment This contribution has been co-funded by EIT Food for the project entitled “Health SnaP” (www.eitfood.eu/projects/health-snap).
Authors
Rudolf Sollacher¹, Wendelin Feiten¹, Birgit Obst¹, Arianna Bosco¹, Stefan Boschert¹, Justina Yoo², Heiko Soehner²
¹Siemens AG, Otto-Hahn-Ring 6, 81739 Munich, Germany ²Siemens AG, Gleiwitzer Str. 555, 90475 Nuremberg, Germany
Literature
[1] Gelernter, David Hillel. Mirror Worlds: or the Day Software
Puts the Universe in a Shoebox—How It Will Happen and
What It Will Mean. Oxford; New York : Oxford University
Press, 1991. [2] Henning Kagermann; Wolf-Dieter Lukas; Wolfgang Wahlster. Industrie 4.0: Mit dem Internet der Dinge auf dem
Weg zur 4. industriellen Revolution. https://web.archive. org/. [Online] April 01, 2011. [Cited: March 04, 2013.] https://web.archive.org/web/20130304101009/http:// www.vdi-nachrichten.com/artikel/Industrie-4-0-Mit-dem-
Internet-der-Dinge-auf-dem-Weg-zur-4-industriellen-
Revolution/52570/1. [3] About The Importance of Autonomy and Digital Twins for the Future of Manufacturing. Rosen, Roland; von Wichert,
Georg; Lo, George; Bettenhausen, Kurt D. 2015. IFAC-PapersOnLine. Vol. 48, pp. 567-572. [4] Next Generation Digital Twin. Stefan Boschert; Christoph
Heinrich; Roland Rosen. [ed.] I. Horváth, J.P. Suárez Rivero and P.M. Hernández Castellano. Las Palmas de Gran
Canaria, : s.n., 2018. Proceedings of TMCE 2018, 7-11 May, 2018. pp. 209-218. [5] H. A. Barnes, J. F. Hutton, K. Walters. An Introduction to
Rheology. s.l. : Elsevier Science, 1989. [6] Florian T. Hecker, Marc Stanke, Thomas Becker, Bernd
Hitzmann. Application of a modified GA, ACO and a random search procedure to solve the production scheduling of a case study bakery. Expert Systems with Applications. 2014, 41, pp. 5882–5891.