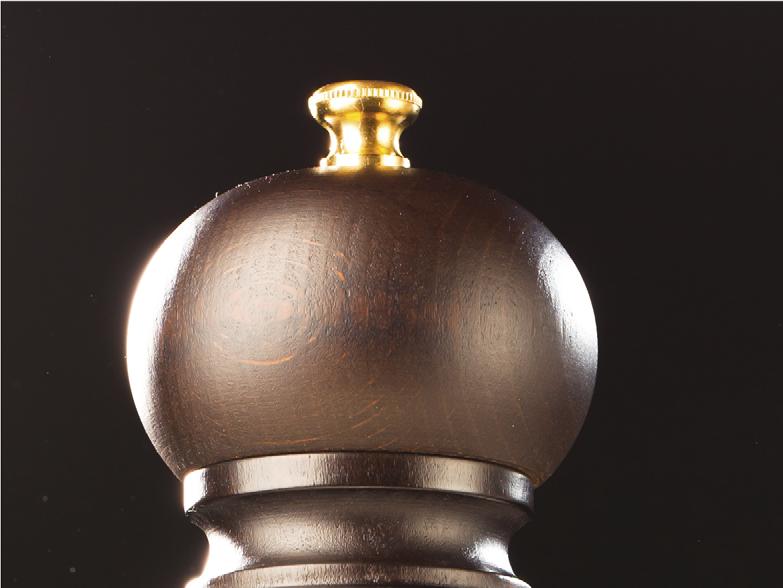
8 minute read
Putting Reliability Through The Mill

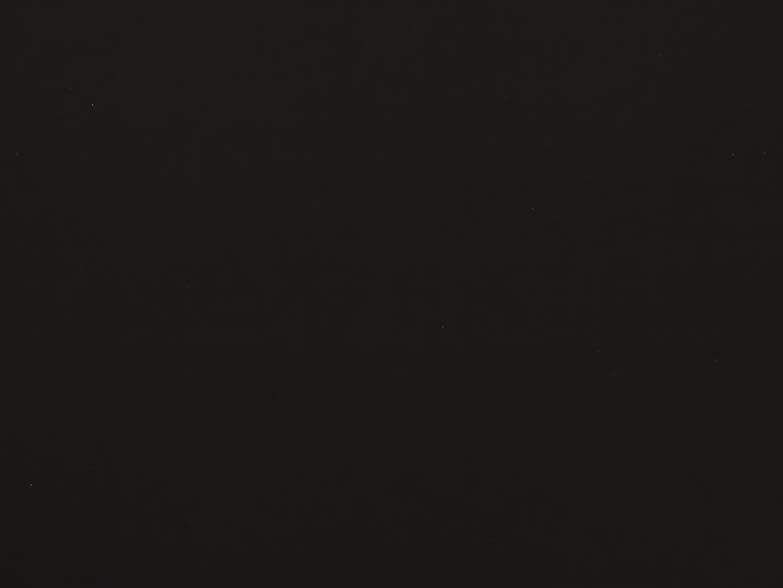
Farrukh Yaqub, SKF Australia, discusses the advances in condition monitoring and data analytics that are helping owners to optimise the reliability, availability, and cost of grinding operations.
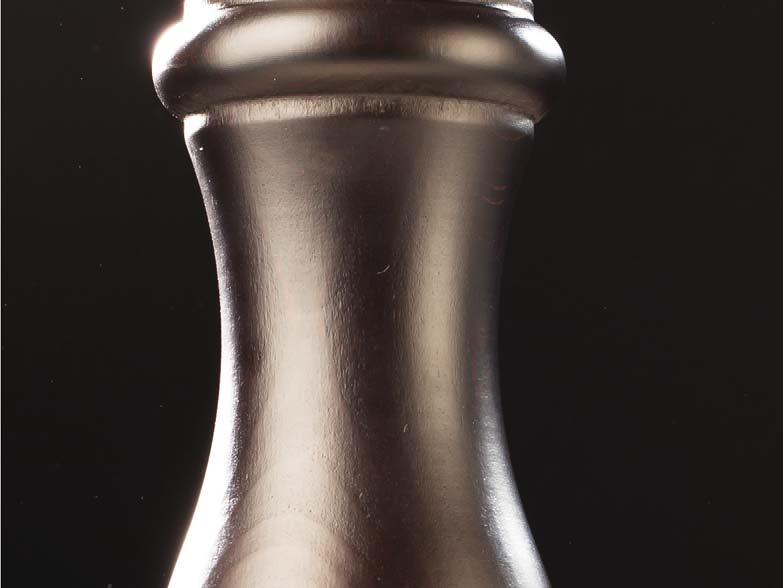
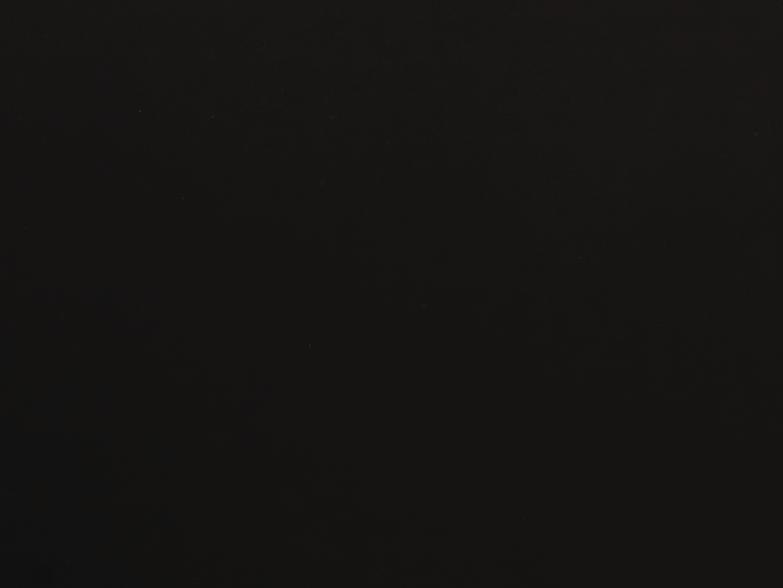
Grinding is a critical process in the mining value chain. The performance of downstream processes in the production of copper, iron, gold, and cement all depend on consistent grinding of input materials. Grinding mill throughput also plays a key role on the overall productivity and profitability of a mine.
In recent years, grinding mills have become the focus of increased attention. To maximise yields and exploit lower quality ores, owners need their grinding mills to work harder and perform more consistently. Grinding is also a highly energy-intensive process, making mills a target for eff iciency improvement eff orts aimed at cutting operating costs and reducing the carbon footprint of materials production.
Against this backdrop, the use of predictive technologies in grinding mills is growing, as owners seek ways to better understand the performance and reliability of their assets. By measuring a range of physical characteristics – such as temperature, vibration levels, and the position of key components – condition monitoring systems aim to provide early warning of wear, breakages, and other problems that could aff ect process performance or lead to unscheduled shutdowns.
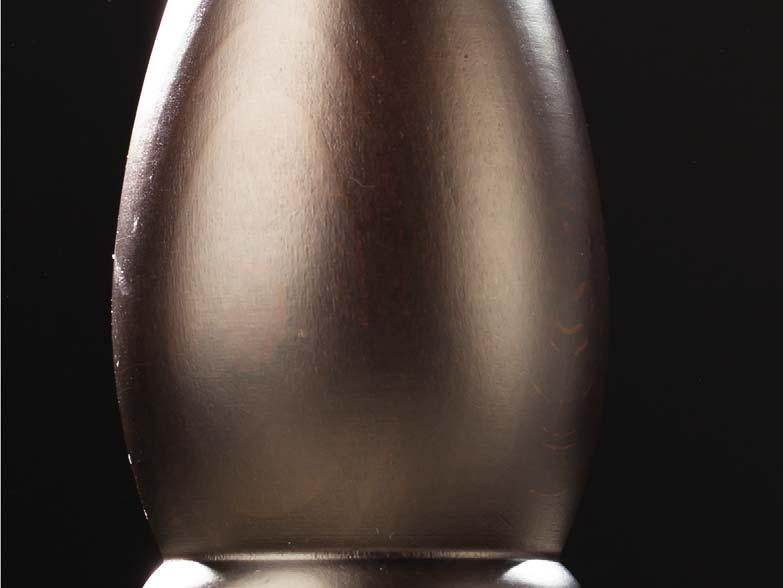
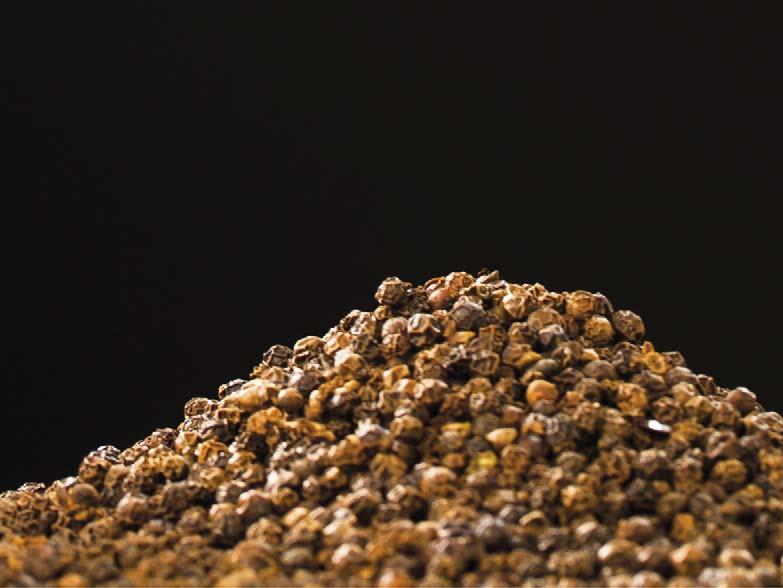
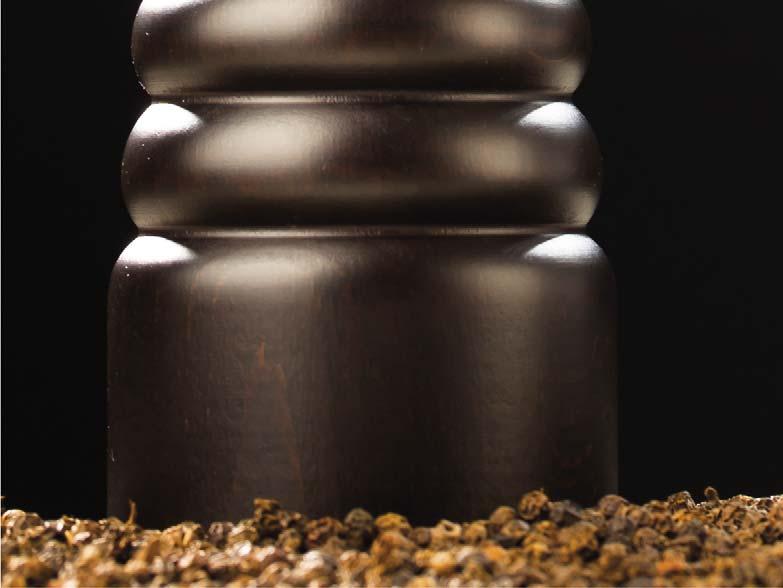
At its simplest, condition monitoring involves periodic manual measurements using hand-held data collectors, such as temperature or vibration probes. Operators or their contractors may make a series of measurements on a monthly basis, increasing that frequency if anomalies in the data suggest cause for concern.
More recently, digitalisation and the growth of the Industrial Internet of Things (IIoT) technologies has enabled the development of more automated monitoring solutions. Networks of sensors permanently attached to machines can continuously record data in conjunction with the process parameters and transmit to a central on or offsite location for data analytics, diagnostics, and prognostics.
Continuous condition monitoring has a number of advantages for owners: automated data collection is consistent, reliable, cheaper, and safer than manual monitoring. It also reduces the mobilisation cost to remote locations and allows on-site operations and maintenance personnel to spend more time on higher-value tasks. Permanently installed systems also pick-up problems far in advance. If an issue develops in the hours or days after a round of manual measurements, it may not be spotted for several weeks.
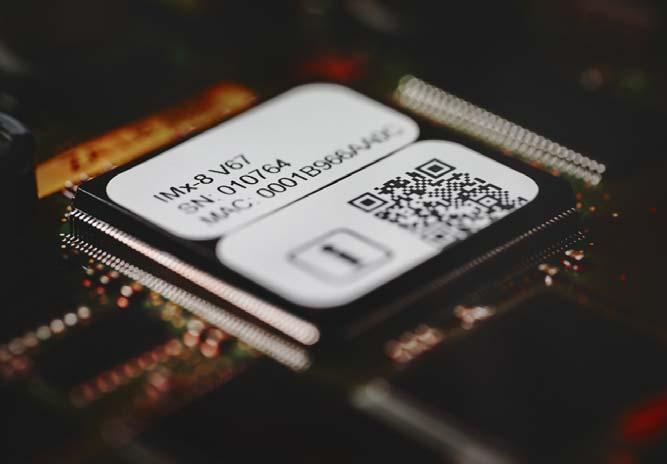
Figure 1. Continuous condition monitoring can pick-up problems in advance.
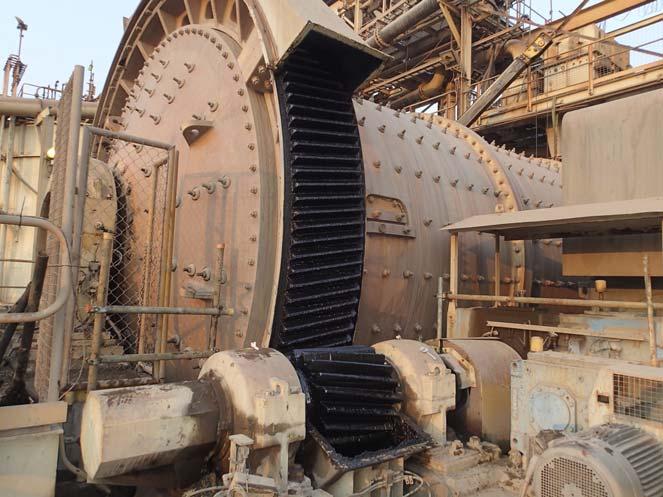
Figure 2. SKF has used decades of experience to specifically design mill monitoring systems (MMS).
Data overload
Modern wired condition monitoring systems create a different set of challenges, however. The most significant of those is the sheer volume of data. Together, the sensors installed on a mill can generate millions of data points every month. Combing manually through all that data to spot potential problems is too much of a task for even the most experienced data analyst.
To overcome that issue, today’s most advanced mill condition monitoring systems use sophisticated analytical techniques to automate significant parts of their data processing. Reliability engineers have access to a large, and growing, set of analytical tools that allow them to filter data in different ways, identify transient events or long-term trends, and detect faint but important signals in complex, noisy data. Selecting and using the most appropriate tools for a grinding mill requires a combination of advanced analytical skills and deep knowledge of equipment performance and health.
SKF has been involved in the design and analysis of rotating equipment for more than 110 years, and the company has decades of experience in condition monitoring – using both hand-held and permanently installed technology. In the mining and materials processing sectors, SKF's specifically designed mill monitoring systems (MMS') are deployed around the globe.
The mill monitoring system
While they share a number of common components, the company’s mill monitoring systems are customised to meet the requirements of each mill and each end-user. One key design decision is the number and type of sensors to install on the machine, and that depends on the design of the mill and associated failure modes.
Depending upon the design, drive assembly and failure modes, SKF’s customised solution provides robust predictability through multiple sensing arrangements, which include: vibration, temperature, pressure, gap, and process data.
MMS' not only collect data through their array of sensors, but they also interface with control systems to streamline data collection in conjunction with process and production parameters. That interface has a critical role to play in the overall performance of the condition monitoring system, as it provides context that aids the interpretation of the sensor data.
The operational data is important because condition monitoring data points – such as temperatures, gaps, and vibration levels – are strongly affected by the mill’s working conditions. Differences in load, material characteristics, and operating speeds will impact the sensor readings. The thickness of the oil film in a hydrostatic bearing will depend on the pressure generated by the lubrication pump, for example.
When the MMS is interfaced with the control systems, it can make decisions about whether the sensor data it is receiving is providing a useful picture of the mill’s condition. Typically, reliability engineers want to look at data from steady state operation, and the fieldbus
connection allows this to be determined automatically. Data recorded during transient events, such as start up or shut down procedures, is filtered through simultaneous sampling of all variables.
On the ground and in the cloud
Data analysis in the mill monitoring system is carried out in two places: on-site within the data acquisition system, and off-site in the SKF cloud. Splitting the work this way means the system can respond quickly to urgent events, while also taking advantage of the greater computing power, and human expertise, available at SKF’s condition monitoring centres around the world.
In normal use, the on-site system will detect times when the mill is operating at a suitable steady state, then transmit selected sensor data over a secure internet connection to the off-site servers. The on-site system also triggers an alarm if there is an abnormality in the data, in addition to capturing pre and post event data. In such cases, the system also sends the sensors’ data to the SKF servers.
It is at the SKF condition monitoring centres that the analytical heavy lifting is done. Software at the centres continually filters and monitors the incoming data, hunting for signals that could indicate a developing problem at the mill. The analytical techniques used are based on the knowledge of the possible failure modes in different types of equipment, and the effect of such failures on sensor readings.
While many condition monitoring tools are based on explicit rules, coded into the system by experienced reliability engineers, the most advanced systems are now using artificial intelligence and machine learning techniques to increase their ability to detect potential issues. In this approach, the monitoring system uses the data it collects to build a ‘digital twin’ of the grinding mill. This digital twin is a representation of the range of data generated by normal operations. Comparing new data against this historical baseline, the artificial intelligence (AI) system can flag unusual events, even if it has not been explicitly told to look for them, and ask the reliability specialists to take a closer look at the data.
When one of the MMS’ analytical tools identifies a potential issue, the next step involves human expertise. An SKF reliability specialist will look to the data, using their experience and judgement to determine the most appropriate action. Their recommendation will depend on the nature of and severity of the issue.
Some problems require quick, but non-disruptive interventions. Data from a bearing might indicate lubricant starvation, for example, and the condition monitoring team will suggest that the mill operations personnel check or adjust its lubrication systems.
In other cases, the data may indicate wear or damage to a component. Here, the SKF specialist will make
Figure 3. Advanced MMS are increasingly used to enable sustainable long-term performance improvements and optimise production. recommendations for future interventions. Is the problem mild enough to wait until the next scheduled overhaul, or should the operator plan an earlier shutdown to replace the part? Based on the specialists’ feedback, the AI and machine learning (ML) system adapts and tunes itself for future diagnostics and prognostics.
From predictability to performance
Maintenance decisions are made in collaboration with the mill operator, and they require the costs and benefits of different intervention strategies to be carefully balanced. Running a component to the end of its safe life may be the least disruptive option, for example, but earlier replacement may allow the worn part to be remanufactured and reinstalled at a later date. For large, high value components such as trunnion bearings, remanufacturing offers significant cost savings for operators. Remanufacturing also has environmental benefits, requiring fewer materials and less energy than the production of a new item. With colleagues in SKF AI, SKF is already working on new approaches that automate more of the reliability analysis and which allow the company to make more accurate predictions and actionable recommendations for its clients. Advanced MMS' are not just helping owners to optimise the short and medium-term reliability and cost-effectiveness of their assets. Increasingly, they are also being used to enable sustainable long-term performance improvements and optimise production, while having reliable rotation. By collecting data from multiple mills, reliability experts can find reoccurring problems and identify changes to equipment, operations, and maintenance processes that prevent them. Furthermore, the more data these systems collect, the smarter and more sophisticated their analytical tools become.
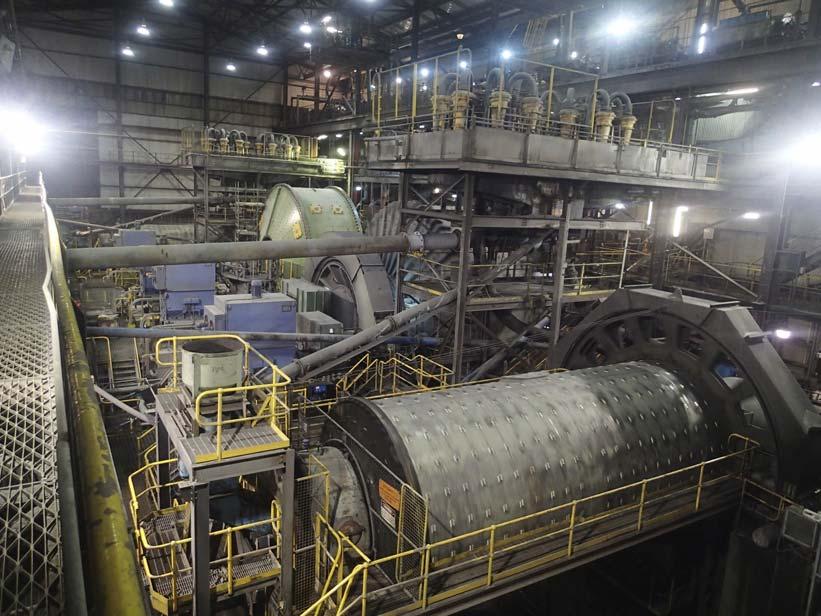
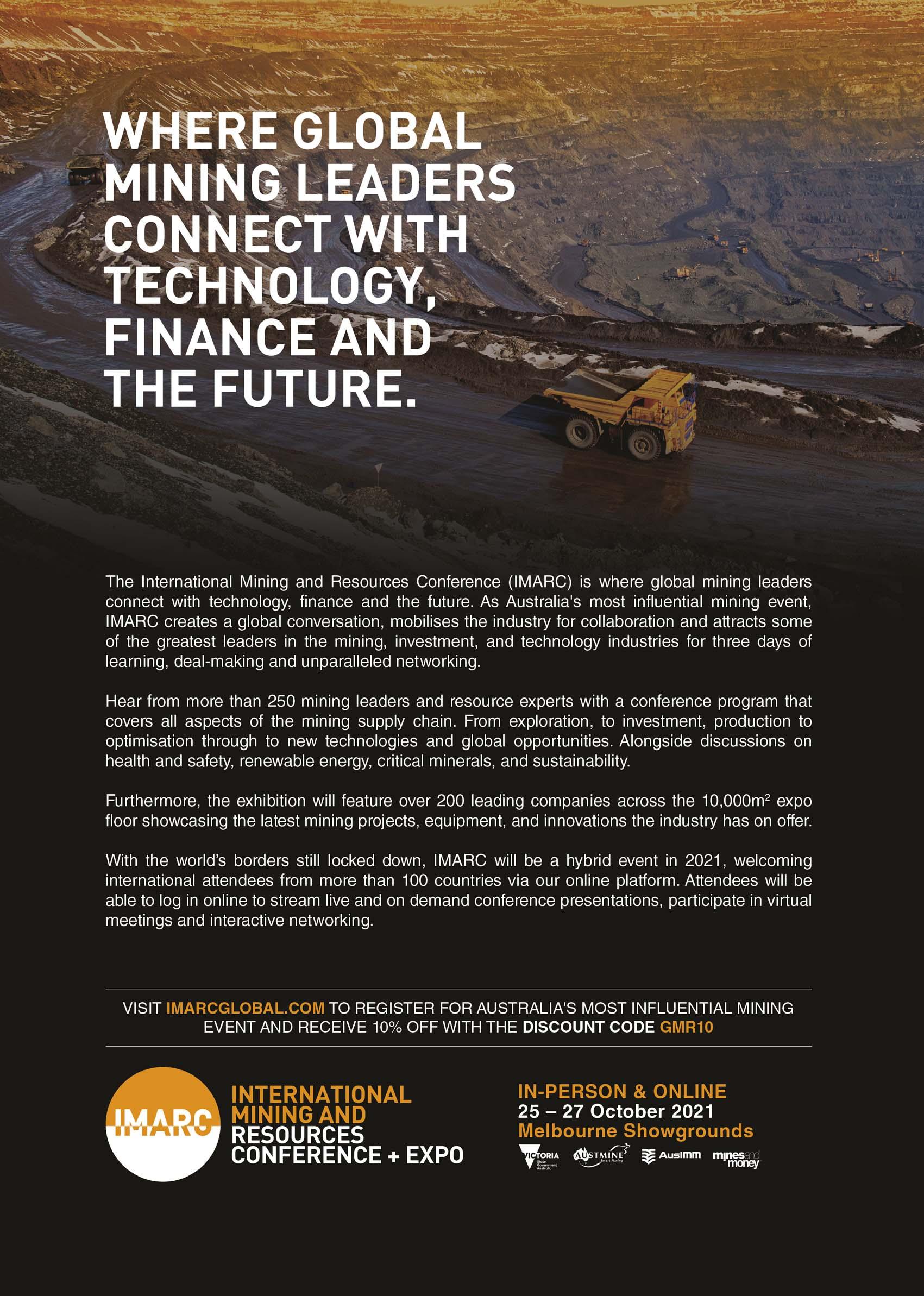