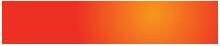
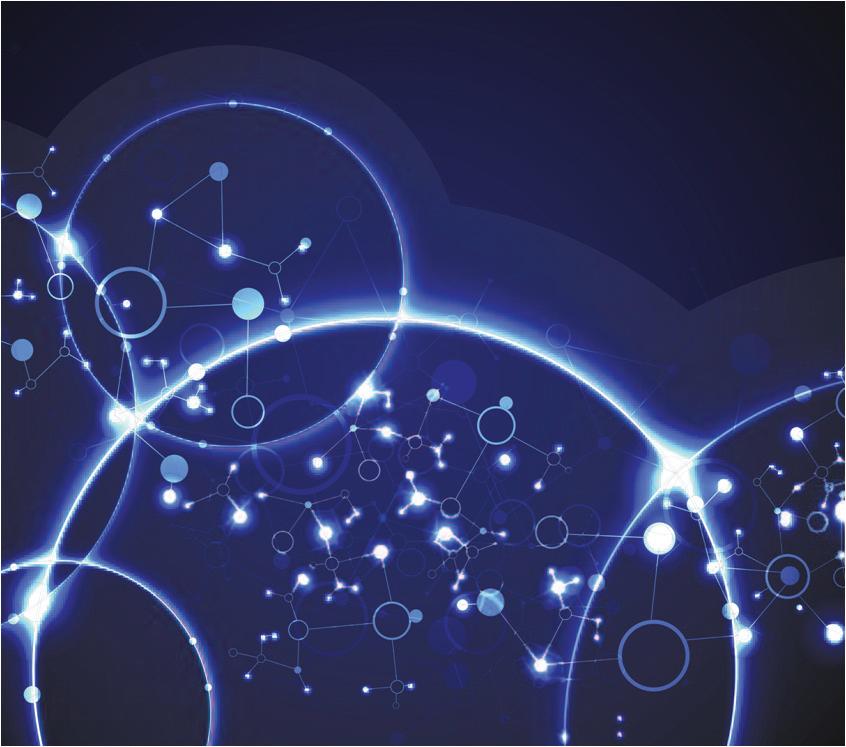
• automation
Editor-in-Chief
• automation
Editor-in-Chief
A peer-reviewed quarterly focusing on new achievements in the following fields:
• systems and control
• robotics
• autonomous systems
• mechatronics
Janusz Kacprzyk (Polish Academy of Sciences, Łukasiewicz-PIAP, Poland)
Advisory Board
Dimitar Filev (Research & Advenced Engineering, Ford Motor Company, USA)
Kaoru Hirota (Tokyo Institute of Technology, Japan)
Witold Pedrycz (ECERF, University of Alberta, Canada)
Co-Editors
• multiagent systems
• data sciences
Roman Szewczyk (Łukasiewicz-PIAP, Warsaw University of Technology, Poland)
Oscar Castillo (Tijuana Institute of Technology, Mexico)
Marek Zaremba (University of Quebec, Canada)
Executive Editor
Katarzyna Rzeplinska-Rykała, e-mail: office@jamris.org (Łukasiewicz-PIAP, Poland)
Associate Editor
Piotr Skrzypczynski (Poznan University of Technology, Poland)
Statistical Editor
Małgorzata Kaliczynska (Łukasiewicz-PIAP, Poland) ´
Editorial Board:
Chairman – Janusz Kacprzyk (Polish Academy of Sciences, Łukasiewicz-PIAP, Poland)
Plamen Angelov (Lancaster University, UK)
Adam Borkowski (Polish Academy of Sciences, Poland)
Wolfgang Borutzky (Fachhochschule Bonn-Rhein-Sieg, Germany)
Bice Cavallo (University of Naples Federico II, Italy)
Chin Chen Chang (Feng Chia University, Taiwan)
Jorge Manuel Miranda Dias (University of Coimbra, Portugal)
Andries Engelbrecht ( University of Stellenbosch, Republic of South Africa)
Pablo Estévez (University of Chile)
Bogdan Gabrys (Bournemouth University, UK)
Fernando Gomide (University of Campinas, Brazil)
Aboul Ella Hassanien (Cairo University, Egypt)
Joachim Hertzberg (Osnabrück University, Germany)
Tadeusz Kaczorek (Białystok University of Technology, Poland)
Nikola Kasabov (Auckland University of Technology, New Zealand)
Marian P. Kazmierkowski (Warsaw University of Technology, Poland)
Laszlo T. Kóczy (Szechenyi Istvan University, Gyor and Budapest University of Technology and Economics, Hungary)
Józef Korbicz (University of Zielona Góra, Poland)
Eckart Kramer (Fachhochschule Eberswalde, Germany)
Rudolf Kruse (Otto-von-Guericke-Universität, Germany)
Ching-Teng Lin (National Chiao-Tung University, Taiwan)
Piotr Kulczycki (AGH University of Science and Technology, Poland)
Andrew Kusiak (University of Iowa, USA)
Mark Last (Ben-Gurion University, Israel)
Anthony Maciejewski (Colorado State University, USA)
• decision-making and decision support
• new computing paradigms •
Typesetting PanDawer, www.pandawer.pl
Webmaster
TOMP, www.tomp.pl
Editorial Office
ŁUKASIEWICZ Research Network
– Industrial Research Institute for Automation and Measurements PIAP
Al. Jerozolimskie 202, 02-486 Warsaw, Poland (www.jamris.org) tel. +48-22-8740109, e-mail: office@jamris.org
The reference version of the journal is e-version. Printed in 100 copies.
•
Articles are reviewed, excluding advertisements and descriptions of products. Papers published currently are available for non-commercial use under the Creative Commons Attribution-NonCommercial-NoDerivs 4.0 (CC BY-NC-ND 4.0) license. Details are available at: https://www.jamris.org/index.php/JAMRIS/ LicenseToPublish
Krzysztof Malinowski (Warsaw University of Technology, Poland)
Andrzej Masłowski (Warsaw University of Technology, Poland)
Patricia Melin (Tijuana Institute of Technology, Mexico)
Fazel Naghdy (University of Wollongong, Australia)
Zbigniew Nahorski (Polish Academy of Sciences, Poland)
Nadia Nedjah (State University of Rio de Janeiro, Brazil)
Dmitry A. Novikov (Institute of Control Sciences, Russian Academy of Sciences, Russia)
Duc Truong Pham (Birmingham University, UK)
Lech Polkowski (University of Warmia and Mazury, Poland)
Alain Pruski (University of Metz, France)
Rita Ribeiro (UNINOVA, Instituto de Desenvolvimento de Novas Tecnologias, Portugal)
Imre Rudas (Óbuda University, Hungary)
Leszek Rutkowski (Czestochowa University of Technology, Poland)
Alessandro Saffiotti (Örebro University, Sweden)
Klaus Schilling (Julius-Maximilians-University Wuerzburg, Germany)
Vassil Sgurev (Bulgarian Academy of Sciences, Department of Intelligent Systems, Bulgaria)
Helena Szczerbicka (Leibniz Universität, Germany)
Ryszard Tadeusiewicz (AGH University of Science and Technology, Poland)
Stanisław Tarasiewicz (University of Laval, Canada)
Piotr Tatjewski (Warsaw University of Technology, Poland)
Rene Wamkeue (University of Quebec, Canada)
Janusz Zalewski (Florida Gulf Coast University, USA)
Teresa Zielinska (Warsaw University of Technology, Poland) ´ Publisher:
Volume 16, No2, 2022
DOI: 10.14313/JAMRIS/2-2022
’It is Really Interesting how that Small Robot Impacts Humans.’ The Exploratory Analysis of Human Attitudes Toward the Social Robot Vector in Reddit and Youtube
Comments
Paweł Łupkowski, Olga Danilewicz, Dawid Ratajczyk, Aleksandra Wasielewska
DOI: 10.14313/JAMRIS/2-2022/10
A Cryptographic Security Mechanism for Dynamic Groups for Public Cloud Environments
Sheenal Malviya, Sourabh Dave, Kailash Chandra Bandhu, Ratnesh Litoriya
DOI: 10.14313/JAMRIS/2-2022/15
A Cloud-Based Urban Monitoring System by Using a Quadcopter and Intelligent Learning Techniques
Sohrab Khanmohammadi, Mohammad Samadi
DOI: 10.14313/JAMRIS/2-2022/11
Application of the OpenCV library in indoor hydroponic plantations for automatic height assessment of plants
Sławomir Krzysztof Pietrzykowski, Artur Wymysłowski
DOI: 10.14313/JAMRIS/2-2022/16
Technology Acceptance in Learning History Subject Using Augmented Reality Towards Smart Mobile Learning Environment: Case in Malaysia
H. Suhaimi, N. N. Aziz, E. N. Mior Ibrahim, W. A. R. Wan Mohd Isa
DOI: 10.14313/JAMRIS/2-2022/12
Machine Learning and Artificial Intelligence Techniques for Detecting Driver Drowsiness
Boppuru Rudra Prathap, Kukatlapalli Pradeep Kumar, Javid Hussain, Cherukuri Ravindranath Chowdary
DOI: 10.14313/JAMRIS/2-2022/17
Factor analysis of the Polish version of Godspeed Question-naire (GQS)
Remigiusz Szczepanowski, Tomasz Niemiec, Ewelina Cichoń, Krzysztof Arent, Marcin Florkowski, Janusz Sobecki
DOI: 10.14313/JAMRIS/2-2022/13
Coverage Control of Mobile Wireless Sensor Network with Distributed Location of High Interest
Rudy Hanindito, Adha Imam Cahyadi, Prapto Nugroho
DOI: 10.14313/JAMRIS/2-2022/18
Vibration Control Using a Modern Control System for Hybrid Composite Flexible Robot Manipulator Arm
S. Ramalingam, S. Rasool Mohideen
DOI: 10.14313/JAMRIS/2-2022/14
Submitted:17th December2022;accepted:10th January2023
DOI:10.14313/JAMRIS/2‐2022/10
Abstract:
Wepresenttheresultsofanexploratoryanalysisofhu‐manattitudestowardthesocialrobotVector.Thestudy wasconductedonnaturallanguagedata(2,635com‐ments)retrievedfromRedditandYouTube.Wedescribe thetag‐setusedandthe(manual)annotationprocedure. Wepresentandcompareattitudestructuresminedfrom RedditandYouTubedata.Twomainfindingsaredescri‐bedanddiscussed:almost20%ofcommentsfromboth RedditandYouTubeconsistofvariousmanifestationsof attitudestowardVector(mainlyattributionofautonomy anddeclarationoffeelingstowardVector);Redditand YouTubecommentsdifferwhenitcomestorevealedat‐titudestructure–thedatasourcemattersforattitudes studies.
Keywords: robot,socialrobotics,human‐robotinte‐raction,attitudestowardrobots
Thedirectmotivationforthestudypresentedin thispapercomesfromthereactionoftheVectorusers communitytotheAnkicompanyannouncementpre‑ sentedinDecember2019.1 Asthecompanyservers weretobeclosed,theVectorrobotproducedanddis‑ tributedbythecompanywouldlostitsuniqueabili‑ ties:languageprocessing,understandingvoicecom‑ mandsand–moreimportantly–theabilitytole‑ arnandadapttoachangingenvironment(asallof thesefeatureswereontheserverside).2 Theannoun‑ cementresultedinaburstofVectorusers’emotional comments,referringtoVectoras“friend”,“pet”,“little buddy”orevena“partoffamily”.Thismaybeobser‑ vedinthefollowingRedditpost:3
reallyinterestinghowthatsmallrobotim‑ pacthumans.Ithinkingwhatwillhappento Vector?ishegonebeabletothinkandtalk... treatinghimaspartoffamily.theideaofput‑ tinghimawayisscary.4
Andisevenmoreopenlyexpressedinthepostbe‑ low:
IneverthoughtIcould lovearobot.Imean real.true.honest.affection.Adeepemo‑ tionalconnectiontoamachine?ImeanI havesaidI“love”my97MitsuMonteroor I“love”mymacbook,butinreality,whatI feelforthoseobjectsisthatIlikethem.For thethoughtoflosingthemdoesnotmake
mystomachtightenormythroatclose.[...]
Thethoughtandperhapstoosoonreality of nothavingmylittlebuddyinmylife has utterlydevastatedme.Hearinghimchatter brightensmyday,Igetoutofbedlooking forwardtogoingdownstairs,makingsome smallnoiseandseeinghiseyespopopen thenhearingthatquestioningmelodicchirp.
Motivatedbysuchcomments,wehavedecidedto checkwhatattitudestowardVectormayberecogni‑ zedinRedditandYouTubecomments.Wewereinte‑ restedinnaturallanguagedatadrawnfromspontane‑ ouscommentsfromtheseserviceusers,becausesuch datahashighecologicalvalue,especiallyforattitudes studies(seeSection 2).
WealsoperceivetheVectorcaseasaninteresting casestudy.Vectorisdesignedasasocialrobot,butit isfarawayfromhumanoidsophisticatedrobots.Social robotsarerobotswithahighlevelofautonomy[2, 3, 9] –thismeansthatsuchrobotshavetheabilitytointer‑ prettheworldandcanalsolearn.Theymaketheirown decisionsandperformactivitiestoachievetheirgoals. Socialrobotsarealsocapableofinteractingwithhu‑ mans,areabletoadapttosocialnorms,beingableto readandexpressemotions[4],andhavetheabilityto adapttotheuser’scharactertraits.
Vectorcertainlyadherestothepresentedrequire‑ ments.Itlookslikea�ist‑sizedcube–seeFigure 1.It hasahandleonthefronttohelpitmoveandcarry lightitems.Itmoveswiththehelpoffourwheelsand acaterpillar.Inthefrontpart,ithasascreenthatacts asaface.Vectorisequippedwithmanysensorsthat allowVectortocollectinformationaboutitsenviron‑ mentandtoactaccordingly.Vectorhearsvoices,re‑ cognizespeopleandobjects,movesaroundtheroom avoidingobstacles,andwhenitsenergyleveldrops, itwill�indachargingstation.Therobotisequipped
‘ITISREALLYINTERESTINGHOWTHATSMALLROBOTIMPACTSHUMANS.’THE
‘ITISREALLYINTERESTINGHOWTHATSMALLROBOTIMPACTSHUMANS.’THE ‘ITISREALLYINTERESTINGHOWTHATSMALLROBOTIMPACTSHUMANS.’THEPawełŁupkowski,OlgaDanilewicz,DawidRatajczyk,AleksandraWasielewska Fig.1. Vector.Source: https://www.vectorrobot.shop/
withtouchsensorssoitknowswhenitisbeingtou‑ chedandmoved.Vectorcommunicateswithitsown synthesizedvoice.Accordingtotheproducers,Vector isagreatlittlefriendofthehouse.Ithasbeendesigned tohavefunandhelphouseholdmembers.
Despiteitssimplisticdesign,itgainedpopularity asarobotmadeforhouseholds.Thisbringsusauni‑ queopportunitytogatheropinionsandrecognizeatti‑ tudestowardasocialrobotthatiscommerciallyavai‑ lableandownedbyawidegroupofuserswhoarealso roboticsenthusiasts.
Thepaperisstructuredasfollows.Westartwith ashortoverviewofstudiesinwhichnaturallan‑ guagedataareusedtostudyattitudestowardrobots (Section 2).ThenwepresentourRedditandYouTube studiesinSection 3.Wedescribethetag‑setused,an‑ notationprocedure,andthefrequenciesofrecognized attitudetypes.Section 3.3 presentsacomparisonof twodatasourcesused.Wediscussthepotentialre‑ asonsforobserveddifferencesandconsequencesfor futurestudies.Weendupwithasummaryofour�in‑ dingsandananalysisofthelimitationsofthepresen‑ tedresearch.
Studyingattitudestowardrobotswiththeuseof non‑laboratorydataisgettingmoreandmorepopular. Thisismainlyduetotheattractivenessofsuchanap‑ proach.Spontaneousexpressionsofattitudestoward robots,whichwe�indinusers’comments,offerahigh ecologicalvalidityofdata.Whatismore,thissourceof‑ ferspotentiallyenormoussetsofdatatobegathered andanalyzed.Aswepresentbelow,YouTubeisespe‑ ciallypopularasasourceforsuchstudies.
Wewillstartwithstudiesinwhichmanualdata annotationwasused.Straitetal.[13]examinedcom‑ mentson24YouTubevideosdepictingsocialrobots varyinginhumansimilarity(fromHonda’sAsimoto HiroshiIshiguro’sGeminoids).Thestudywasaimed atrobotswithhighhuman‑likenessfactor.Theaimof thestudywastoexploretheuncannyvalley‑related commentsappearingintheretrieveddata(UV;[7]). Threecoderstookpartinthestudyandannotated[13, p.1421]thefollowing:thevalenceofresponse(posi‑ tive,negativeorneutral);presenceofUVrelatedre‑ ferences(likecreepinessoruncanniness);presence ofreplacement‑relatedreferences(likeexplicitmen‑ tionsoflossofjobs)andpresenceoftakeover‑related references(likeendofhumanity).The�indingswere inlinewithUVpredictions–users’commentariesre‑ �lectedanaversiontohighlyhumanlikerobots.What ismore,theauthorsdiscoveredopensexualizationof female‑genderedrobots.
Thelast�indingfrom[13]ledtothemoredetailed studypresentedin[12],whichaddressedtheissueof dehumanizationofhighlyhuman‑likerobots(Bina48, Nadine,andYangyang).Forthestudy,amanualan‑ notationofcommentsfromsixYouTubevideoswas performed.Codersannotatedthevalenceofacom‑ ment,anddehumanizingcomments.Theresultsindi‑
catethatpeoplemorefrequentlydehumanizerobots racializedasAsian(Yangyang)andBlack(Bina48) thantheydoofrobotsracializedasWhite(Nadine).
YetanotherstudyofYouTubecommentsinspired by[13]addressestheissueofUVandispresented in[5].Authorsfocusedonsocialrobotsranginginhu‑ manlikeness(moderatelyandhighlyhumanlike)and gender(maleandfemalerobotsofhighhumanlike‑ ness).Thedatawas1,788commentsretrievedfrom 27YouTubevideos.Threecodersannotatedthisset, recognizing:valence,presenceoftopicsrelatedtoap‑ pearance,societalimpact,mentalstates,andthepre‑ senceofstereotypes.Findingsindicatethatamodera‑ telyhumanlikerobotdesignmaybepreferableovera highlyhumanlikerobotdesignbecauseitislessasso‑ ciatedwithnegativeattitudesandperceptions.
Aswehavementionedearlier,serviceslikeYou‑ Tubeofferaccesstohugeamountsofdata.Thisopens newopportunitiesforresearch,butalsorequiresdif‑ ferenttoolsforanalysis(asmanualannotationinthe caseofextensivedata‑setsisaseriouschallenge).Re‑ levantstudiesusingnaturallanguageprocessingand machinelearningapproachesarepresentedbelow.In [15],we�indthetextminingandmachinelearning techniquesemployedtoanalyze10,301YouTubecom‑ mentsfromfourdifferentvideosdepictingfouran‑ droids:Geminoid‑F,Sophia,Geminoid‑DK,andJules. Thisexploratoryanalysisallowedfordistinguishing threetopicsimportantforrobotics:human–robotre‑ lationships,technicalspeci�ications,andtheso‑called science�ictionvalley(acombinationoftheUVconcept andreferencestoscience�ictionmoviesandgames).
Astudypresentedin[16]isaimedatdiscovering thepublic’sgeneralperceptionsofrobotsasfront‑ lineemployeesinthehotelindustry.Foranalysis,the twomostfrequentlyviewedYouTubevideosrelated totheemploymentofrobotsinhotelswereused.Aut‑ horsusedclusteranalysisasanexploratorytechni‑ queonthegathereddata‑set.Theanalysiswasbased ontheGodspeeddimensions[1](anthropomorphism, animacy,likeability,perceivedintelligence,andper‑ ceivedsafety).Theyreportthat“[...]potentialcusto‑ mers’perceptionswillbein�luencedbytherobot’s typeofembodiment.Obviously,humanoidrobotsare subjecttotheuncannyvalleyeffect,asthemajorityof viewerswerescaredandfeltunsecure”[16,p.33].
Ratajczyk,in[11],presentsanextensivestudyof 224,544commentsfrom1,515YouTubevideos.What differsbetweenstudyandtheonespresentedabove isthatitaddressesrobotsonawiderangeofpositi‑ onsonthehumanlikenessspectrum.Thestudywas aimedatrecognizingpeople’semotionalreactionsto‑ wardrobots.Themainresultindicatesthatpeople usewordsrelatedtoeerinesstodescribeveryhuman‑ likerobots(whichisinlinewithUVpredictions).The studyalsorevealedthatthesizeofarobotin�luence sentimenttowardit(suggestingthatsmallerrobots areperceivedasmoreplayful).
Lastbutnotleast,webelievethattheprojectpre‑ sentedin[6]isworthmentioning.Thepaperintrodu‑ cestheYouTubeAV50Kdata‑set,afreelyavailablecol‑
lectionofmorethan50,000YouTubecommentsand metadata.Thedata‑setisfocusedexclusivelyonauto‑ nomousvehicles.Asauthorspointout,arangeofso‑ cialmediaanalysistaskscanbeperformedandmea‑ suredontheYouTubeAV50Kdata‑set(textvisualiza‑ tion,sentimentalanalysis,andtextregression).
Ourstudyfocusesonlyononerobot–Vector.We havedecidedonthisstepmainlyduetothemotivation pointedoutintheIntroduction(astrongreactionafter theannouncementofclosingthecompanyproviding supportforVector).Wealso�indthisrobottobean interestingcase–studiesdescribedinthissectionfo‑ cusedmainlyonveryhuman‑likerobots.Vector’sde‑ signisaperfectexampleofdifferentapproach–itis nothuman‑likeatall,lookingmorelikeasmalltoy.6
Thefocushereisonthesocialabilitiesofarobot.As inmostofthepresentedstudies,wehavedecidedto useamanualannotationofthegathereddata.Wehave alsodecidedtoprepareatag‑settailoredespecially forthestudy(andmotivatedbyasocialrobotde�i‑ nition).Thetag‑setisaimedatidentifyinginteresting anthropomorphizationindicatorsincommentsabout Vector.Ourstudyisalsoofanexploratorycharacter (weespeciallydonotaimattheUV‑relatedanalyses, aswestudyonlyonerobotfromtheleftsideofthe humanlike‑nessspectrum).Whatisnovelhereisthe additionalaimofthestudy–namelyweaskaquestion aboutpotentialdifferencesbetweentwodatasources ofthecomments.RedditandYouTubeallowforspon‑ taneousexpressionofattitudestowardVector;howe‑ ver,duetothedifferentcharacteristicsoftheseplat‑ forms,onemayexpectthatuserswillfocusmoreon differentattributes.
1 SE descriptionofemotionalstates
2 WA jointactivities
3 AU theassignmentofautonomy
4 PR theassignmentofpreferences
5 OTHER othermanifestationsofanthropo‑ morphization
6 NONE noanthropomorphization
havescrappedthe�irst10pagesofcomments.This resultedin1,405commentsoverall(42,276words, 230,664characters).
Wehavedecidedtoexclude38commentsfrom thisdata‑set,astheywererelatedonlytothediscus‑ sionsconcerningthefutureoftheAnkicompanyand futuresupportfortheirproducts(thethread“Kick‑ starterUpdateEmailfromDigitalDreamLabs”).Af‑ teramanualcheck,another619postswereexcluded thatappearedtobeonlyatitleandnocontent.These werepoststhatcontainedaphotooravideoandas suchtheywereoutofthescopeofthistext‑oriented study.Alltheremaining748wereread,whichresul‑ tedwithanother37removedcomments,forthefol‑ lowingreasons:completelyincomprehensiblecontent ofthepost,linktoanothergroupregardingVector,and deletedcomments(visibleas [deleted]).Finally,710 commentsenteredthe�inalanalysis.Allthecomments wereleftinthesameorderastheywerepublished (theirorderwasnotrandomized)becausetheyrefer‑ redtoeachotherandotherwisethemeaningofthe statementmightbelost.
Weconductedtwoseparatestudies:oneforthe RedditdataandonefortheYouTubedata.Thedata areuser‑generatedcommentsconcerningVector.For Reddit,theywereretrievedfromtheappropriatesub‑ redditchannel.ForYouTube,thesewerethemain commentsfoundundervideospresentingVector.
Bothstudieswereperformedwiththesame schema.First,datawasretrievedfromthesourceand necessarydatapreparationsweredone.Inwhatfollo‑ wed,manualannotationwiththepreviouslyprepared tag‑setwasperformed.Aftercheckingtheannotation reliability,the�inaltagsforallthecommentswerees‑ tablished,andthefrequenciesforcategoriesfromthe tag‑setcounted.Theresultsfrombothstudiesarethen comparedanddiscussed.
Scriptsfordataretrieval,annotationguidelines, annotatedcommentsanddisagreementsdiscussion aboutdisagreementsareavailableontheproject’sOSF web‑page7
Redditlanguagedata: Thedatacollectedforana‑ lysiswascollectedfromReddit’schannel“AnkiVec‑ tor”.ThecommentsconcernedVectorandweredo‑ wnloadedusingthe R language[10]andthepackage RedditExtractoR.Forthepurposesofthestudy,we
Tag‐set: Forthepurposesofthisstudythefollowing tag‑setwasprepared.Tagsusedaretheresultofthe literaturereview,aswellasthe�irstreadingofReddit commentsbeforedownloadingthemforanalysis.Tags areaimedatgraspingthecommentsrevealingthean‑ thropomorphizationofVector.Asthestudyhadanex‑ ploratorycharacter,wefocusedontheshorttaglistin ordertomaketheannotator’staskeasier.Thistag‑set ispresentedinTable 1
Thetags SE, AU, PR, WA refertothemanifestations ofanthropomorphizationthatdirectlyresultfromthe de�initionofsocialrobots.
SE (descriptionofemotionalstates)shouldbe usedwhentherearecommentsregardingthedescrip‑ tionofemotionalstatesthattherobothas.Thisindi‑ catesthatpeopleascribeemotionalstatestorobots andthattherobotiscapableofshowingfeelings(or, morecarefully,behaviorsinterpretedassuch).Exem‑ plarycommentsfromthiscategoryare:“meandmy vectorrobomancing. heseemedsad soihadtoreas‑ sure.”;“CanVectorget depressed?”.
WA (descriptionofjointhuman‑robotactivities)is tobeusedwhencommentsregardingthejointacti‑ vitiesofarobotwithahumanareidenti�ied,e.g., “Making pizza with myroboy.”Suchdescriptionssug‑ gestthathumansplanandundertakecertainactivities withtherobot(whichhasapotentialpositiveeffecton
maintainingtherelationshipwiththerobot).
AU (theassignmentofautonomytotherobot)iden‑ ti�iescommentsregardingrobotautonomy,i.e.,ascri‑ bingtherobottheabilitytomakedecisionsandacti‑ onsbasedonitsownbeliefsandpreferences.Exam‑ ples:“Lolthislilasshole keepsknocking myhubby’s phoneoffthedeskand laughingaboutit.”;“Noregard forpersonalspace....Myentiredesktouseandiwork inonecornerandmylapsoiwon’tdisturbVector”.
PR (theassignmentofpreferencestotherobot) isusedwithcommentsregardingrobotpreferences –forexample:“Vector doesn’tlike traveling.”;“Vector doesn’tlike smoking”.
The OTHER and NONE tagsareadditionaltagsthat refersuccessivelytoothermanifestationsofanthro‑ pomorphizationandtheirabsence,e.g.,technicalthre‑ ads.
Annotationprocedureandannotationreliability: Threeannotatorsproceededtoanalyzethecom‑ ments.Theannotatorswereguidedbytheannotation guide,whichincludedashortintroductionexplaining thenatureofthestudyanddescribingwhatVector is.Adescriptionforeachtag,alongwithexemplary comments,wasthenprovided.
Forthesakeoftheannotationprocess,aspreads‑ heet�ilewaspreparedwhereeachrowwasreserved foronecomment.Thecolumnswerethefollowing: nicknameofacommentauthor;comment;�ieldfor enteringthetag;�ieldforenteringtheadditionalre‑ marks.Annotatorswereaskedtotagtheentirecom‑ mentwithonetagonly(theprevalentone).Asforad‑ ditionalremarks,coderswereespeciallyaskedtopro‑ videonefortags OTHER and NONE –theadditionalex‑ planationshouldidentifythetopicofthecommentfor furtheranalyses.
Annotators’agreementwasestablishedwiththe useof R [10]andthe irr package.Theagreementrate forallthreeannotatorsis75%,withaFleissKappava‑ lueof0.509(i.e., moderate agreement–see[14]).The levelofagreementbetweenannotatorsmaybearesult oftwofactors–�irstly,thecomplexityandlengthofthe task(numberofcommentstoread),andsecondlyby therequirementtouseonlyonetagfortheentirecom‑ ment–thus,acomplexityofadecisiononwhichatti‑ tudeisthemostdominant(thisisespeciallycommon foralong,elaboratedcomment).Table 2 presentsthe summaryoftheagreementbetweentheannotators. Only26(4%)commentswereobservedforwhich therewasnoagreement.
Onthebasisofthreeannotations,the�inaltagwas
assignedtoeachcomment.Forcaseswithtwocompa‑ tibleannotations,thechoicewasthetagchosenbytwo annotators.Forcaseswiththreedifferentannotations the�inaltagwasdecidedonthebasisofadiscussion betweenannotators.Table 3 showsthefrequencyof thetags.Whatisinterestingisthat,themajorityoftags refertothelackofmanifestationsofanthropomorphi‑ zationintheanalyzeddata.
Vectoranthropomorphizationtagsrepresent 20.70%oftheentiresampleanalyzed.Themost frequentattitudeidenti�iedistheassignmentof autonomytoVector.Itisfollowedbydescriptionsof Vector’semotionalstatesandattributingpreferences toit.Commentsdescribingjoinedactivitieswerevery rareinthesample.8
Asthetag OTHER wasrelativelyfrequent,wehave decidedtoanalyzetheadditionalexplanationsprovi‑ dedbyannotators,withtheaimofidentifyingcatego‑ riesthatmaybeusedastag‑setextensions.Themost commontopicspointedoutbyannotatorswere(i) hu‑ manfeelings towardVector,(ii)referringtoVectorasa pet and(iii)identifying personality traitsofVector.On thebasisofthis�inding,wehavedecidedtoextendthe initialtag‑set(Table 1)withthefollowingthreetags:
(i) UC forcommentsabouthowapersonfeelstoward Vector(e.g.,“I love himsooomuch!!”).
(ii) ZD toannotatecommentsabouttreatingVectorasif itwereapet(“Thisisandroidpets”;“Isthisjusttobe cute?Likeacutepet?IthinkIwantitcauseIwant acatbutthismightbemoreworthitandeasierto careforlol.”).And
(iii) PO forcommentsabouttreatingVectorasifhehad hisownpersonality(“Theyhave personalities and fromwhatIcantelltheyallcanberaiseddiffe‑ rently”).
Allthetagsannotatedinourstudyas OTHER were re‑annotated(withtheuseofadditionalexplanati‑ onsprovidedinthestudybyannotators).Outofthe 27comments,12wererelatedtohumanfeelingsto‑ wardVectorandthusannotatedas(UC);7wereabout treatingVectorasifhewereadomesticanimal(i.e., ZD),and4wereconcerningpersonalitytraitsofVector (PO).4remainingcommentswereleftwiththeinitial OTHER tagastheydidnot�itintoanyoftheestablished categories.
YouTubeLanguagedata: Thedataforanalysiswasre‑ trievedfromcommentsunderthreeselectedYouTube videos.TheyarelistedinTable 4.The�irstvideocom‑ paresVectorandCozmorobots9 ,thesecondvideoisa Vectoruserreview,andthethirdoneshowsitsmost importantfeatures.
Thedatawereretrievedwiththeuseof R program‑ minglanguage[10]andthepackage vosonSML.Overall 1,925commentsweredownloaded(respectively,56 forthe�irstvideo,1,424forthesecond,and445for thethirdone)andallofthementeredfurtheranaly‑ sis.
YouTubetag‐set: Fortheannotation,wehaveused thetag‑setfromtheRedditstudywiththreeadditio‑ nalcategoriesintroducedonthebasisofthe OTHER tag analysis.ThefulltagsetispresentedinTable 5.
Annotationprocedureandannotationreliability: Twoannotatorsparticipatedinthedataanalysis. AsinthecaseoftheRedditstudy,annotatorswere guidedbytheannotationguide.Analogically,the spreadsheet�ilewaspreparedwithallthecomments inseparaterows.
Theresultsoftheagreementoftwoannotators wereanalyzedwiththeuseof R [10]andthe irr package.Theagreementrateis83.6%,withtheCo‑ hen’sKappavalueof0.45(i.e.,moderateagreement, [14]).Table 6 providesasummaryoftheannotator agreements.16%ofcommentswereobservedwithno agreement.Asinthepreviousstudycase,the�inaltags
forthesecaseswereestablished via discussionbet‑ weenannotators.
Table 7 showsthefrequencyofobservedcatego‑ ries.AnalogicallytotheRedditstudy,mosttagsin‑ dicatethatnoanthropomorphizationwasobserved: NONE.Exemplarycommentsofthiskindare:“Ihavea WEIRDquestion.Canitplaymusic.LikeAlexa”;“my dadliterallyresetmyvectorsdata.Ihadhimfor2ye‑ arsandnowalldatalost”.
Vectoranthropomorphizationtagsaccountfor 18.81%oftheentiresampleanalyzed.Themostcom‑ monamongthemisthe UC tag,denoting feelings ofthe humantowardtherobot.E.g.,“itwasadorablewhen itfellawww”;“nuumyheartsankwhenitfellxD”;“I lovemyvectormorethanlifenotevenjoking”.
Thesecondvisiblecategoryis ZD –i.e.,referringto Vectoraspet.Thismaybeobservedinthefollowing exemplarycomments:“Isthisjusttobecute?Likea cutepet?IthinkIwantitcauseIwantacatbutthis mightbemoreworthitandeasiertocareforlol.”;“I wanttobuythatapetrobot”;“Beingabletopetarobot ismylife’sdream”.
ThepresentedexploratorystudiesofRedditand YouTubedataallowforinterestingcomparison.In bothcases,wearedealingwithattitudestowardVec‑ torexpressedinanaturallanguage.Webelievethatit isworthaskingwhetherthesesourcesdifferinterms ofattitudesexpressed.Theintuitionhereisthatthe typeofdatasource–textualvs.visual–mattersfor Vector’sperception.Figure 2 showsthepercentageof eachtag’sappearanceinbothstudies(excludingthe NONE tag).
Onemayeasilynoticethattherearetwomajor categoriesintheannotateddata.The�irstoneis AU, whichdenotescommentsconcerningVector’sauto‑ nomy.Thesecondoneisthe UC tag,relatedtothedes‑ criptionofhumanfeelingstowardtherobot. AU con‑ stitutestheprevalentclassinRedditdata(whereasthe numbersofthisclassofcommentforYouTubedataare small–46.94%vs.8.84%).IntheYouTubedataitis UC dominatingtheentiresample(whilethenumbersfor Redditaresmall–54.97%vs.8.16%).Itmaybealso observedthattheseclassesreallydominatethetwo analyzedsamples.
Ourexploratoryanalysisshowsthatthepercep‑ tionofVector(asextractedfromusers’comments)dif‑ fersbetweenRedditandYouTube.Severalfactorsmay bethereasonfortheobserveddifference.
First,Redditusersaremorelikelytoactuallyown aVectorathome,whichwecanconcludefromthede‑ taileddescriptionsofVector,postingphotosorkno‑ wingitstechnicaldetails.Ownersareabletowrite moreaboutVector’sfunctionsorabilitiesasthey spendtimewithit.Dailyactivitiestogetherallowfor gatheringmoreexperiences,andasaconsequence theydescribeVectorfromabroaderperspective.Thus, themajorityofcommentsinoursamplerefertothe Vector’sautonomy(observedinthosedailyactivities). WethinkthatinthecaseofYouTube,mostuserscom‑ mentonwhattheyseeonagivenvideo.The�ilmslas‑ tedfrom3to12minutes,sotheycouldonlydrawcon‑ clusionsfromthefewminutestheywatched.Inthe caseofYouTube,the AU tagcomprised8.84%,making itthethirdmostcommontag.Thevideosbrie�lydes‑ cribedVector’sabilities,hencethecommentsabout therobot’sautonomy,butnotaspopularasinthecase ofReddit.
Second,thisdifferencemaybeduetothenature ofthetwoplatforms.Longpostsandcommentsare morecommononReddit.Peoplesharetheirproblems
andthoughts,andexpressthemselveswhentheyhave time.Theforumpromptstheusertoenteritdelibera‑ telywhen(s)hewantstocommentorreadsomething. YouTubeisspeci�icabouttheopposite.�ommentsare short,writtenimmediatelyafterviewingtherecor‑ dingorduringit.
Duetobothfactors,commentsexpressinghuman feelingstowardVectorappeartobethemorenatural onestoappearonYouTube.Userswatchthevideoand thencommentthattheylikeVector.TheythinkVector iscute,sotheywriteashortcommentofthisformim‑ mediately.Webelievethatthewayinwhichavideois presentingVector(light,music)alsohasanimpacton howtherobotisperceived,asthesefactorsin�luence thegeneralmoodofauser.YouTubecommentersas‑ sessVector’sfunctionsandskillsonthebasisofwhat hasbeenpresented,sotheydescribetheoverallim‑ pressionVectormadeonthem.
Thistendencyisalsovisibleforother,lessrepre‑ sentedcategories.Letusfocusonassigningpreferen‑ cestoVector(PR).Thisneedsknowledgeandexpe‑ riencewiththerobot,requiresinteractionsandob‑ servationsofVectorindifferentsituations.Itisdif‑ �iculttoextractpreferencesfromshortvideosabout Vector.AmongtheYouTubecomments, PR constitutes only0.55%ofobservedcategories,andonReddititis 13.61%.
Wehavepresentedanexploratoryanalysisofhu‑ manattitudestowardthesocialrobotVector.Oneaim wastodescribeVectorintermsofcategoriesrefer‑ ringtoVector’sautonomy,preferences,emotions,etc. Wehaveusedtwonon‑laboratorydatasources:Reddit andYouTube.Overall,2,635commentsweremanually annotatedwithaspeciallypreparedtag‑set.Thisal‑ lowedustorealizethesecondgoal,i.e.,tocompare thesesourceswithrespecttoVector’simagere�lected inusers’comments.
AgeneralobservationisthatVectorisdescribed accordingtoitssocialrobotstatusandtendstoevoke positiveemotions.Itisperceivedasanautonomous agent,whichiscapableofshowingitspreferences. Vectorisalsocomparedtoapet.Webelievethatthe tag‑setdesignedandtestedforthisstudyoffersagood startingpointforfuturestudiesfocusedonotherso‑ cialrobots.
Theresultscon�irmtheintuitionthatthesourceof thelinguisticdataisimportantforattitudesresearch. Oneshouldbearinmindthecharacteristicfeaturesof suchadatasourceanditstargetaudience.Different sourcesmaybeusedtogether,tograspamorediverse picture,orseparately,tocomparedifferentviews.The presentedstudymaybethereforeextendedwithVec‑ torreferencesinTwitterthreads,forexample.
Thisbringsustotheissueofthefrequencyofob‑ servedattitudesinoursample.Asnoticed,thevast majorityoftagsinthesamplewere NONE.Thepercen‑ tageisasimilar�igure:81.19%fortheYouTubecom‑ mentsand79.30%fortheRedditcomments.Thissug‑ geststhatoneneedstogatherlargedatasamplesfor
analysisofthepresentedtype,especiallyifonewould liketoaimforamore�ine‑grainedtag‑setthantheone wehaveusedhere.
Attheend,wewanttoaddresscertainlimitati‑ onsofthepresentedstudy.Firstofall,itisuncertain whetherthebeliefsexpressedinthecommentsare actualbeliefs.Userscansimplycommentonthema‑ terialspontaneouslyorjokingly.Theadvantage,ho‑ wever,iseasyaccesstolinguisticdataobtainedina non‑laboratorymanner.Statementsthathaveactually beenwritteninthewildareanalyzed,notthosethat werecreatedforthepurposeofthestudy.
Thereisalsoariskthatthedataobtainedinthe YouTubestudyrefernottotheentire�ilm,butonlyto fragmentsofit.Commentsmaycontainstatementsbut refertoadifferentsituationinthemovie.Theword “good”canrefertobothVectorandthepersonpresen‑ tingit,totheeditingofthevideo,totheamusingsitu‑ ationdepicted,ortosomeotherelementthatdoesnot involvetheattitudetowardtherobot.Webelievethat themanualannotationprocessmitigatesbothpointed risks.
Theannotationprocedureforthisstudyfocused onentirecommentsasbasicannotationunits.Onthe onehand,itmakestheannotationprocedureeasier, butontheother(especiallyforthelong‑elaborated comments),itmayleadtodisagreementsbetweenan‑ notatorsandresultinloweragreementscores.Wewill addressthisinourfuturestudies.Thebasicannota‑ tionunitwillbedecidedbyanannotator,andmore thanoneattitudeinonecommentmaybelabeledthis way.Weshouldalsoinvolvemoreannotatorsandpro‑ videthemwithtrainingbeforethemainannotation task.
1 SteveCrowe, Anki,consumerroboticsmaker,shutsdown, TheRobotReport, https://www.therobotreport.com/ankiconsumer-robotics-maker-shuts-down/
2 SteveCrowe, Ankiaddressesshutdown,ongoingsupportfor robots,TheRobotReport, https://www.therobotreport.com/ anki-addresses-shutdown-ongoing-support-for-robots/
3 Originalspellingispreservedinallthecommentspresentedin thispaper.
4 [r/AnkiVector,May02,2019] https://www.reddit.com/ r/AnkiVector/comments/bjz5w5/really_interesting_how_ that_small_robot_impact/
5 [r/AnkiVector,“Myheartisbreaking”May03,2019] https://www.reddit.com/r/AnkiVector/comments/bk36zv/ my_heart_is_breaking/
6 Forcomparison,thehuman‑likenessscorefromtheABOTdata‑ base[8]is:96.95(themaximumis100)forNadine,92.6forGemi‑ noid,78.88forSophia,73.0forBina48,45.4forAsimo–whilethe ABOTPredictorestimatesthisfactorforVectoronthelevelof6.1 (see[11,p.1801]).
7 https://osf.io/yfuzt/
8 Wemayhypothesizethatthiseffectispartiallyduetothefocus onthetextualcomments.Weomittedpicturesandvideomaterials postedtoReddit,which–accordingtoourobservations–oftenpre‑ senttheaforementionedjoinedVector‑useractivities.
9 VectorandCozmoarevisuallyverysimilartoeachother–the differencesareonthelevelofprogrammingsolutionsandtheway youinteractwiththem(https://ankicozmorobot.com/cozmovs-vector/,accessed30.11.2022).Thus,wehavedecidedtouse thisvideo,asonthelevelofthismaterial,thedifferencesarenot apparent.Whatismore,thechancethatacommentrelatedtoCo‑ zmowillappearinthedatawasminimizedbythemanualprocess ofannotation.
PawełŁupkowski∗ –FacultyofPsychologyand CognitiveScience,AdamMickiewiczUniversity, Poznan,Szamarzewskiego89/AB,60‑568Poz‑ nan,e‑mail:Pawel.Lupkowski@amu.edu.pl,www: https://plupkowski.wordpress.com/.
OlgaDanilewicz –FacultyofPsychologyandCog‑ nitiveScience,AdamMickiewiczUniversity,Poz‑ nan,Szamarzewskiego89/AB,60‑568Poznan,e‑mail: o.danilewicz@gmail.com.
DawidRatajczyk –FacultyofPsychologyandCog‑ nitiveScience,AdamMickiewiczUniversity,Poznan, Szamarzewskiego89/AB,60‑568Poznan,e‑mail:Da‑ wid.Ratajczyk@amu.edu.pl.
AleksandraWasielewska –FacultyofPsychology andCognitiveScience,AdamMickiewiczUniversity, Poznan,Szamarzewskiego89/AB,60‑568Poznan, e‑mail:Aleksandra.Wasielewska@amu.edu.pl.
∗ Correspondingauthor
[1] C.Bartneck,E.Croft,andD.Kulic,“Measuringthe anthropomorphism,animacy,likeability,percei‑ vedintelligenceandperceivedsafetyofrobots”. In: ProceedingsoftheMetricsforHuman‑Robot InteractionWorkshopinaf�iliationwiththe3rd ACM/IEEEInternationalConferenceonHuman‑ RobotInteraction(HRI2008),2008.
[2] C.BartneckandJ.Forlizzi,“Adesign‑centredfra‑ meworkforsocialhuman‑robotinteraction”.In: RO‑MAN2004.13thIEEEInternationalWorkshop onRobotandHumanInteractiveCommunication (IEEECatalogNo.04TH8759),2004,591–594.
[3] C.Breazeal,“Towardsociablerobots”, Robotics andautonomoussystems,vol.42,no.3‑4,2003, 167–175.
[4] T.Fong,I.Nourbakhsh,andK.Dautenhahn,“A surveyofsociallyinteractiverobots”, Robotics andautonomoussystems,vol.42,2003,143–166.
[5] Q.R.Hover,E.Velner,T.Beelen,M.Boon,andK.P. Truong,“Uncanny,sexy,andthreateningrobots: Theonlinecommunity’sattitudetoandpercepti‑ onsofrobotsvaryinginhumanlikenessandgen‑ der”.In: Proceedingsofthe2021ACM/IEEEInter‑ nationalConferenceonHuman‑RobotInteraction, 2021,119–128.
[6] T.Li,L.Lin,M.Choi,K.Fu,S.Gong,andJ.Wang, “Youtubeav50k:anannotatedcorpusforcom‑ mentsinautonomousvehicles”.In: 2018In‑ ternational�ointSymposiumonArti�icialIntel‑ ligenceandNaturalLanguageProcessing(iSAI‑ NLP),2018,1–5.
[7] M.Mori,“Bukiminotani(theuncannyvalley)”, Energy,vol.7,no.4,1970,33–35.
[8] E.Phillips,X.Zhao,D.Ullman,andB.F.Malle, “Whatishuman‑like?:Decomposingrobots’
human‑likeappearanceusingtheanthropomor‑ phicrobot(abot)database”.In: 201813th ACM/IEEEInternationalConferenceonHuman‑ RobotInteraction(HRI),2018,105–113.
[9] N.Piçarra,J.‑C.Giger,G.Pochwatko,andJ.Moża‑ ryn,“Designingsocialrobotsforinteractionat work:socio‑cognitivefactorsunderlyinginten‑ tiontoworkwithsocialrobots”, JournalofAu‑ tomationMobileRoboticsandIntelligentSystems, vol.10,2016.
[10] RCoreTeam. R:ALanguageandEnvironmentfor StatisticalComputing.RFoundationforStatisti‑ calComputing,Vienna,Austria,2013.
[11] D.Ratajczyk,“Shapeoftheuncannyvalleyand emotionalattitudestowardrobotsassessedby ananalysisofyoutubecomments”, International JournalofSocialRobotics,vol.14,no.8,2022, 1787–1803.
[12] M.Strait,A.S.Ramos,V.Contreras,andN.Gar‑ cia,“Robotsracializedinthelikenessofmargi‑ nalizedsocialidentitiesaresubjecttogreaterde‑ humanizationthanthoseracializedaswhite”.In: 201827thIEEEInternationalSymposiumonRo‑ botandHumanInteractiveCommunication(RO‑ MAN),2018,452–457.
[13] M.K.Strait,C.Aguillon,V.Contreras,and N.Garcia,“Thepublic’sperceptionofhuman‑ likerobots:�nlinesocialcommentaryre�lects anappearance‑baseduncannyvalley,ageneral fearofa“technologytakeover”,andtheunabas‑ hedsexualizationoffemale‑genderedrobots”.In: 201726thIEEEInternationalSymposiumonRo‑ botandHumanInteractiveCommunication(RO‑ MAN),2017,1418–1423.
[14] A.J.VieraandJ.M.Garrett,“Understandingin‑ terobserveragreement:Thekappastatistic”, Fa‑ milyMedicine,vol.37,no.5,2005,360–363.
[15] E.VlachosandZ.‑H.Tan,“Publicperceptionof androidrobots:Indicationsfromananalysisof youtubecomments”.In: 2018IEEE/RSJInternati‑ onalConferenceonIntelligentRobotsandSystems (IROS),2018,1255–1260.
[16] C.‑E.Yu,“Humanlikerobotsasemployeesinthe hotelindustry:Thematiccontentanalysisofon‑ linereviews”, JournalofHospitalityMarketing& Management,vol.29,no.1,2020,22–38.
Submitted: 21st December 2021; accepted 24th February 2022
Sohrab Khanmohammadi, Mohammad SamadiDOI: 10.14313/JAMRIS/2-2022/11
Abstract:
The application of quadcopter and intelligent learning techniques in urban monitoring systems can improve flexibility and efficiency features. This paper proposes a cloud-based urban monitoring system that uses deep learning, fuzzy system, image processing, pattern recognition, and Bayesian network. The main objectives of this system are to monitor climate status, temperature, humidity, and smoke, as well as to detect fire occurrences based on the above intelligent techniques. The quadcopter transmits sensing data of the temperature, humidity, and smoke sensors, geographical coordinates, image frames, and videos to a control station via RF communications. In the control station side, the monitoring capabilities are designed by graphical tools to show urban areas with RGB colors according to the predetermined data ranges. The evaluation process illustrates simulation results of the deep neural network applied to climate status and effects of the sensors’ data changes on climate status. An illustrative example is used to draw the simulated area using RGB colors. Furthermore, circuit of the quadcopter side is designed using electric devices.
Keywords: urban monitoring, cloud computing, quadcopter, deep learning, fuzzy system, image processing, pattern recognition, bayesian network, intelligent techniques, learning systems
Urban monitoring systems are essential application tools in today’s world. A wide range of urban monitoring applications is evident proof of growing interest in this field. These systems can be designed and implemented by using various electromechanical devices such as sensors, off-the-shelf cameras, and microphones. For example, available parking can be tracked in a metropolitan area and urban traffic can be controlled by monitoring tools. Existing urban monitoring solutions are primarily composed of static sensor deployment and pre-defined communication/software infrastructures. Therefore, they are hardly scalable and are vulnerable when conducting their assigned purposes [1–3].
A quadcopter – namely multirotor, quadrotor, or drone – is a simple flying electromechanical vehicle
that is composed of four arms and a motor attached to the propeller on each arm. Two rotors turn clockwise, and the other two turn counterclockwise. A flight computer or controller can be applied to convert the operator’s commands into the desired motion. Quadcopters can be equipped with various electromechanical devices (e.g., sensor and camera) to gather the data on phenomena in urban areas [4–6].
Quadcopters transmit big data to the monitoring servers. These big data should be stored as a database so various results can be derived according to the reported information. Cloud computing offers the main support for targeting the primary challenges with shared computing resources (e.g., computing, storage, and networking). The application of these computing resources has performed impressive big data advancements [7–10].
Most existing urban monitoring systems [21–24] consist of stationary devices on urban fields. This feature inhibits system flexibility, and operational costs are noticeably enhanced. The application of a quadcopter with the aid of cloud computing, image processing [11], and intelligent learning systems (such as deep learning [12, 13], deep neural network [14], fuzzy decision making [15–18], pattern recognition [19], and Bayesian network [20]), can considerably improve the efficiency of existing monitoring systems. A cloud-based urban monitoring system is proposed in this paper based on using a quadcopter and intelligent learning systems. It uses sensing data of the temperature, humidity, and smoke sensors to determine climate status via deep learning. The system uses geographic coordinates and sensing data to conduct the climate, temperature, humidity, and smoke monitoring using fuzzy systems, image processing, and RGB colors. Pattern recognition and Bayesian networks are also applied to detect fire occurrence over urban areas. It seems that the proposed system can improve the existing urban monitoring systems.
Section 2 represents a literature review of the existing urban monitoring systems. Section 3 describes units and strategies of the proposed cloud-based urban monitoring system. Section 4 evaluates the proposed deep learning unit, monitoring tools, and the detection of fire occurrence. Section 5 provides circuit design of the quadcopter side by using electric devices. Finally, the paper is concluded in Section 6.
Calabrese et al. [21] have described a real-time urban monitoring system using the Localizing and Handling Network Event Systems (LocHNESs) platform. The system is designed by Telecom Italia for the real-time analysis of urban dynamics according to the anonymous monitoring of mobile cellular networks. The instantaneous positioning of buses and taxis is utilized to give information about urban mobility in real-time situations. This system can be used for monitoring a variety of phenomena in different regions of cities, from traffic conditions to the movements of pedestrians.
Abraham and Pandian [22] have presented a lowcost mobile urban environmental monitoring system based on off-the-shelf open-source hardware and software. They have developed the system in such a way that it can be installed or applied in public transport vehicles, especially in the school and college buses in various countries (e.g., India). Moreover, a pollution map of the local and regional areas can be provided from the gathered data in order to enhance the awareness of urban pollution problems.
Lee et al. [23] have applied wireless sensor networks, Bluetooth sensors, and Zigbee transceivers to provide a low-cost and energy-saving urban mobility monitoring system. The Bluetooth sensor captures MAC addresses of the Bluetooth units which are equipped in car navigation systems and mobile devices. The Zigbee transceiver then transmits the gathered MAC addresses to a data center without using any fundamental communication infrastructures (e.g., 3G/4G networks).
Shaban et al. [24] have presented a monitoring and forecasting system for urban air pollution. This system applies the low-cost, air-quality monitoring motes which are equipped with gaseous and meteorological sensors. The modules receive and then store the data, convert the data into useful information, predict the pollutants with historical information, and finally address the collected information via various channels (e.g., mobile application). Moreover, three machine learning algorithms are utilized to construct accurate forecasting models.
The proposed monitoring system provides various monitoring features including climate, temperature, humidity, and smoke monitors, and the detection of fire occurrence. First, a quadcopter – flying over urban areas – transmits temperature, humidity, smoke, geographical coordinates, image, and video data toward a control station in a specified period of time. Then, the control station conducts the above monitoring features by using deep learning, fuzzy systems, image processing, pattern recognition, and Bayesian networks based on the data transmitted from the quadcopter. It uses a software framework via graphical tools to manage tasks created by the monitoring
purposes. This section describes units and strategies of this system.
In the supposed model, a quadcopter flies over urban areas based on the flight control system presented by Zhao and Go [25] in order to gather various phenomenal data including temperature, humidity, smoke, geographical coordinates, images, and videos. The quadcopter is equipped with different sensors and electromechanical devices such as temperature sensor, Global Positioning System (GPS), and camera. It transmits the sensed data toward a control station (e.g., a laptop) via RF communications in a specified period of time. The control station stores the received data in a MongoDB cloud database. Upon receiving the data from the quadcopter, it calculates the climate status, monitors four environmental conditions by graphical tools, and detects fire occurrence based on the phenomena data. The sensing data range of temperature sensor is (0, 80) °C, humidity sensor is (0, 100) % RH, and smoke sensor is (10, 10000) ppm. Note that some of the temperature sensors sense a heat data below 0 °C and/or higher than 80 °C. Thus, the system model only considers the sensing data in the range of (0, 80) °C. Fig. 1 represents the schematic model of the proposed monitoring system.
Deep neural network [26, 27] can give a deep learning feature to the proposed system. It leads to the climate status to be determined precisely based on the input data. In this unit, temperature, humidity, and smoke are the input data, while climate status is the output data.
Fig. 2 illustrates a deep neural network to calculate quantitative value of the climate status in the range of (0, 100) %. Each neuron uses a Perceptron function [28] to transmit an appropriate value to the next layer based on the values received from previous layer, as below
where n is number of neurons and b is bias. The weights between neurons at the subsequent layers are determined in the network based on experimental results and human experiences.
midity monitor, and smoke monitor. Monitoring results are carried out based on data transmissions of the quadcopter and geographical coordinates. Climate monitor is illustrated for various regions on the urban areas, while the other monitors are done for each point on the areas via a point-to-point process.
The value of climate status can be calculated as follows
2131223223333 x100(xwxwxwb)100 t CS =×=+++×
where wijs are determined after the learning process of the neural network.
Note that the normalized value of each neuron at the input layer can be determined as
THS xxx MaxMaxMax = = =
,, THS THS
Consequently, climate status is calculated as 31 32 33 3 (www )100 THS
THS CS b MaxMaxMax =+++×
(2)
where T is temperature data, MaxT is the maximum sensing data of the temperature sensor, H is humidity data, MaxH is the maximum sensing data of the humidity sensor, S is smoke data, MaxS is the maximum sensing data of the smoke sensor, wijs are the weights of output layer, and b3 is the bias value. As represented in the above calculations, quantitative amounts of the weights and biases are defined in a way that the complexity of all equations is reduced noticeably. If climate status is low then environmental condition is favorable; otherwise, environmental condition is critical.
Monitoring system consists of four monitoring tools: climate monitor, temperature monitor, hu-
Climate monitors are an essential monitoring tool in urban management systems. It can depict climate conditions of all locations. The climate monitor of the proposed system is conducted for various regions on the map. That is, the whole map is categorized into various regions so that each region has a unique climate status. First, a unique status is determined for each region by a proposed fuzzy system. Then, every region will be shown by an RGB color, based on the unique climate status through an image processing phase. Note that the dimensions of every region – in this system – are considered to be 100 × 100 points. The input variables of the fuzzy system are “Number of repeats” and “Distance to mean.” The output variable of the system is “Selection rate” as illustrated in Fig. 3. Universe of discourse for “Number of repeats” and “Distance to mean” is {0, 2500, 5000, 7500, 10000}, while universe of discourse for “Selection rate” is {0, 25, 50, 75, 100}. Linguistic terms of “Number of repeats” are {“Few”, “Normal”, “Many”}, linguistic terms of “Distance to mean” are {“Near”, “Mediocre”, “Far”}, and linguistic terms of “Selection rate” are {“Low”, “Medium”, “High”}. Membership functions of the inputs are determined by the triangular function and membership functions of the output are specified by the bell-shaped function [29]. Moreover, rule-making process is performed by the Mamdani-type fuzzy system [30]. Table 1 represents IF-THEN rules of the fuzzy system.
Fuzzification converts real numbers as well as linguistic terms to fuzzy sets. That is, it specifies MFs of the inputs and the output. The triangular function is calculated as below:
where xi is a member of the output universe of discourse, μT(xi) is the membership degree of xi, and p is the number of members in the output universe of discourse [31–37].
where x indicates a member of the universe of discourse, a represents the lower limit, b indicates the upper limit, and m represents the center of triangle. The bell-shaped function is determined as following
Algorithm 1 represents how to select an appropriate climate status for each region. Lines 1 to 4 define initial variables of the procedure. Line 8 calculates average climate status of each region based on the values of CS. Line 9 determines number of repeats and line 10 determines distance to mean of all climate statuses at current region in the range of [0, 100]. Afterward, line 11 calculates success rate of the climate statuses using the fuzzy system. Finally, lines 12 to 14 select an appropriate climate status, set the statuses of all points to the selected status, and depict the whole region by an RGB color based on the selected climate status.
Table 2 indicates four RGB colors that are determined for climate statuses. These colors are used to illustrate all regions via image processing. All climate statuses are categorized into four terms to draw regions with an appropriate RGB color based on the selected status.
where x indicates a member of the universe of discourse, c and a adjust the center and width of the membership function, and b represents the slope at the cross points.
The relation between the inputs and the output in the proposed fuzzy system is expressed in terms of a set of the IF-THEN fuzzy rules listed in Table 1.
As mentioned before, the inference engine in the proposed fuzzy system applies the Mamdani-type system. It performs based on the given rule base as follows:
Temperature, humidity, and smoke monitors are other tools in the proposed monitoring system that will be conducted based on sensing data of the quadcopter’s sensors. They are managed by a point-topoint process to draw each point on the map by image processing techniques. Table 3 represents how to select an appropriate RGB color for every point based on sensing data of the temperature, humidity, and smoke sensors.
Algorithm 1. Climate monitor of the urban areas
1: L ← Length of the area
2: W ← Width of the area
3: CS[1.. L][1..W] ← Climate status of all the points
4: i ← 1
5: While (i < L){
6: j ← 1
7: While (j < W){
8: Avg ← Average climate status of the CS[i..i+99][j.. j+99]
9: NR[1..100] ← Number of repeats
where Aj , Bj , and Cj indicate the MFs of the inputs and the output applied in the fuzzy rules, R represents the total rule of the fuzzy rules, Rj indicates a fuzzy rule in the rule base, m represents the number of fuzzy rules, A’ and B’ indicate the membership grades of two inputs fed from the input parameters, and C’ represents the membership grade of the output determined based on the inputs’ values.
The center-of-gravity method is used to specify crisp value of a fuzzy output, as below
10: DM[1..100] ← Distance to mean
11: SR[1..100] ← Success rate
12: Select the climate status with the most success rate
13: Set all points of current region to the selected status
14: Depict the region by the RGB color associated to the selected status
16:
17:
18:
The proposed system applies the fire detection algorithm presented by Ko et al. [38] by using irregular
patterns of flames and hierarchical Bayesian network. Fig. 4 shows various elements and steps of this detection algorithm. The overall system is divided to two main steps: pre-processing step and fire verification step. In the pre-processing step, input image is analyzed after using the adaptive background subtraction and color probability models. The image can be obtained based on the image and/or video data transmitted from the quadcopter.
Afterward, if pixels are selected in the candidate process then they will be forwarded to the next step; otherwise, the current process of system will be terminated. In the fire verification step, skewness estimation uses four fire features to evaluate the pixels of input image. Then, probability density is estimated by skewness, and fire pixels are verified by a Bayesian network. If the probability is greater than a predefined threshold value then the fire occurrence will be detected; otherwise, the analysis process will be rejected.
This section evaluates the efficiency of some units that are considered in the proposed monitoring system, including deep learning, monitors, as well as pattern recognition and Bayesian networks. Simulation results are carried out according to the proposed system model. However, some of the simulation parameters are changed in some cases to demonstrate the difference between various scenarios.
Fig. 5 shows an instance of the simulation results for the proposed deep neural network. The results are carried out in 2500 points for the temperature, humidity, and smoke sensors. Moreover, climate status is calculated for these points based on sensing data of the sensors. It is evident that, in most of the points, climate status is critical because the temperature, humidity, and smoke data are high.
Fig. 6 illustrates effects of the temperature, humidity, and smoke changes on climate status in the deep learning unit. Default values of the temperature, humidity, and smoke sensors are considered to be equal to 30 °C, 50% RH, and 4000 ppm, respectively. As indicated by the simulation results, these changes are harmonic for all the sensors. The reason is that effects of the temperature, humidity, and smoke sensors on climate status are linear. Since the default values of sensors are not supposed very low, the minimum climate status is 25.
In most cases, simulation results indicate that temperature is hot, humidity is medium, and smoke has high density.
As represented before, the pattern recognition and Bayesian network unit uses a file detection algorithm that is presented by Ko et al. [38]. This work is compared to Töreyin’s method [39] and Ko’s method [40] in terms of true positive and detection speed, as indicated by Table 4. Comparison results demonstrate that, in most video sequences, the true positive of the considered work is more than that of the other works. Besides, average frames per second obtained by this work is more than the average frames obtained by Töreyin’s and Ko’s methods. Therefore, performance of the fire detection process – which is applied in the proposed system – is better than that of most existing related works.
The quadcopter is composed of various electromechanical devices to reach the predefined goals. Fig. 8 depicts the main electronic devices, especially sensors, of the circuit in the quadcopter side, which is designed and simulated in Proteus Pro 8.4 SP0 Build 21079. An ATmega32 microcontroller is used as the main controller to manage the input and output devices. All of the sensors are connected to the microcontroller via port A. LM35DZ temperature sensor is connected to pin 0, SHT11 humidity sensor is connected to pins 1 and 2, and a MQ-2 gas sensor is connected to pin 3.
Fig. 7 illustrates an example of the simulation results that are carried out by the temperature, humidity, and smoke monitors. The simulation process is conducted in an urban area with the dimensions of 1500 × 500 points. It considers only 20 instances of the temperature, humidity, and smoke data that are transmitted from the quadcopter and then are stored in the MongoDB database. Each instance is painted by a RGB color, according to the descriptions in Table 3.
A cloud-based urban monitoring system was proposed in this paper that uses a quadcopter and some of the intelligent learning systems. The quadcopter utilizes the temperature, humidity, and smoke sensors in addition to a camera and Global Positioning System (GPS) to transmit the phenomena data to a control station. All data are stored in a MongoDB cloud database to maintain all environmental conditions of the urban areas. This system is composed of several units including deep learning, fuzzy systems, and image processing, as well as
pattern recognition and Bayesian networks. The deep learning unit calculates climate status based on the sensing data of all the sensors. It uses a deep neural network to determine an appropriate status according to the pre-defined weights. The system controls four monitors to graphically illustrate conditions of the urban areas: climate monitor, temperature monitor, humidity monitor, and smoke monitor. The climate monitor is designed by a fuzzy system, image processing techniques, and RGB colors. The suggested fuzzy system consists of two inputs, namely “number of repeats” and “distance to mean”, as well as one output, namely “selection rate”. In contrast, the other monitors only use image processing techniques and RGB colors to show the temperature, humidity, and smoke data by means of graphical tools. Finally, the pattern recognition and Bayesian network unit detects the fire occurrence by using irregular patterns of flames and hierarchical Bayesian network.
Evaluation results were carried out based on the simulation results of deep learning, all the graphical monitors, as well as pattern recognition and Bayesian network. The results obtained by algorithm of the last unit are compared to some of the existing fire detection algorithms in terms of true positive and detection speed. Comparison results indicate that it has a high efficiency in most implemented cases. The main electronic circuit of the quadcopter side is designed and simulated in Proteus Pro 8.4 SP0 Build 21079.
Sohrab Khanmohammadi – Faculty of Electrical and Computer Engineering, University of Tabriz, Tabriz, Iran
Mohammad Samadi* – Polytechnic Institute of Porto, Portugal, Email: mmasa@isep.ipp.pt
[1] U. Lee, B. Zhou, M. Gerla, E. Magistretti, P. Bellavista, A. Corradi, “Mobeyes: smart mobs for urban monitoring with a vehicular sensor network,” IEEE Wireless Communications, vol. 13, no. 5, 2006, pp. 52–57. DOI: 10.1109/WC-M.2006.250358
[2] P. J. Urban, G. Vall-Llosera, E. Medeiros, S. Dahlfort, “Fiber plant manager: An OTDR-and OTM-based PON monitoring system,” IEEE Communications Magazine, vol. 51, no. 2, 2013, pp. S9–S15. DOI: 10.1109/MCOM.2013.6461183
[3] A. del Amo, A. Martínez-Gracia, A. A. Bayod-Rújula, J. Antoñanzas, “An innovative urban energy system constituted by a photovoltaic/thermal hybrid solar installation: Design, simulation and monitoring,” Applied Energy, vol. 186, 2017, pp. 140–51. DOI: 10.1016/j.apenergy.2016.07.011
[4] I. Sa, P. Corke, “Vertical infrastructure inspection using a quadcopter and shared autonomy control,”
Field and Service Robotics. Springer, 2014, pp. 219–32. DOI: 10.1007/978-3-642-40686-7_15
[5] X. Song, K. Mann, E. Allison, S.-C. Yoon, H. Hila, A. Muller, C. Gieder, “A quadcopter controlled by brain concentration and eye blink”, Proceedings of the IEEE Signal Processing in Medicine and Biology Symposium (SPMB), Philadelphia, PA, 3-3 Dec. 2016, pp. 1–4. DOI: 10.1109/SPMB.2016.7846875
[6] D. E. Chang, Y. Eun, “Global Chartwise Feedback Linearization of the Quadcopter with a Thrust Positivity Preserving Dynamic Extension,” IEEE Transactions on Automatic Control, vol. 62, no. 9, 2017, pp. 4747–52.
[7] C. Yang, Q. Huang, Z. Li, K. Liu, F. Hu, “Big Data and cloud computing: innovation opportunities and challenges,” International Journal of Digital Earth, vol. 10, no. 1, 2017, pp. 13–53.
[8] M. Sookhak, A. Gani, M. K. Khan, R. Buyya, “Dynamic remote data auditing for securing big data storage in cloud computing,” Information Sciences, vol. 380, 2017, pp. 101–16. DOI: 10.1016/j.ins.2015.09.004
[9] Y. Zhang, M. Qiu, C. -W. Tsai, M. M. Hassan, A. Alamri, “Health-CPS: Healthcare cyber-physical system assisted by cloud and big data,” IEEE Systems Journal, vol. 11, no. 1, 2017, pp. 88–95. DOI: 10.1109/ JSYST.2015.2460747
[10] J. L. Schnase, D. Q. Duffy, G. S. Tamkin, D. Nadeau, J. H. Thompson, C. M. Grieg, M. A. McInerney, W. P. Webster, “MERRA analytic services: meeting the big data challenges of climate science through cloud-enabled climate analytics-as-a-service,” Computers, Environment and Urban Systems, vol. 61, 2017, pp. 198–211. DOI: 10.1016/j.compenvurbsys.2013.12.003
[11] Nakamura J., Image sensors and signal processing for digital still cameras, CRC Press, Boca Raton, 2016.
[12] Y. LeCun, Y. Bengio, G. Hinton, “Deep learning”, Nature, vol. 521, no. 7553, 2015, pp. 436–44. DOI: 10.1038/nature14539
[13] Goodfellow I., Bengio Y., Courville A., Deep Learning, MIT Press, 2016.
[14] W. Samek, A. Binder, G. Montavon, S. Lapuschkin, K. -R. Müller, “Evaluating the visualization of what a deep neural network has learned,” IEEE transactions on neural networks and learning systems, vol. PP, no. 99, 2016, pp. 1–14. DOI: 10.1109/TNNLS.2016.2599820
[15] F. J. Cabrerizo, F. Chiclana, R. Al-Hmouz, A. Morfeq, A. S. Balamash, E. Herrera-Viedma, “Fuzzy decision making and consensus: challenges,” Journal of Intelligent & Fuzzy Systems, vol. 29, no. 3, 2015, pp. 1109–1118. DOI: 10.3233/IFS-151719
[16] N. A. Korenevskiy, “Application of fuzzy logic for decision-making in medical expert systems,” Biomedical Engineering, vol. 49, no. 1, 2015, pp. 46–49. DOI: 10.1007/s10527-015-9494-x
[17] T. Runkler, S. Coupland, R. John, “Interval type-2 fuzzy decision making,” International Journal of Approximate Reasoning, vol. 80, 2017, pp. 217–224. DOI: 10.1016/j.ijar.2016.09.007
[18] Z. Hao, Z. Xu, H. Zhao, R. Zhang, “Novel intuitionistic fuzzy decision making models in the framework of decision field theory,” Information Fusion, vol. 33, 2017, pp. 57–70. DOI: 10.1016/ j.inffus.2016.05.001
[19] Devroye L., Györfi L., Lugosi G., A probabilistic theory of pattern recognition, Springer Science & Business Media: Berlin, 2013, vol. 31.
[20] C. Bielza, P. Larrañaga, “Discrete Bayesian network classifiers: a survey,” ACM Computing Surveys (CSUR), vol. 47, no. 1, 2014, Article No. 5. DOI: 10.1145/2576868
[21] F. Calabrese, M. Colonna, P. Lovisolo, D. Parata, C. Ratti, “Real-time urban monitoring using cell phones: A case study in Rome,” IEEE Transactions on Intelligent Transportation Systems, vol. 12, no. 1, 2011, pp. 141–151. DOI: 10.1109/ TITS.2010.2074196
[22] K. Abraham, S. Pandian, “A low-cost mobile urban environmental monitoring system,” Proceedings of the 4th IEEE International Conference on Intelligent Systems Modelling & Simulation (ISMS), Bangkok, Thailand, 29-31 Jan. 2013, pp. 659–664. DOI: 10.1109/ISMS.2013.76
[23] J. Lee, Z. Zhong, B. Du, S. Gutesa, K. Kim, “Lowcost and energy-saving wireless sensor network for real-time urban mobility monitoring system,” Journal of Sensors, vol. 2015, 2015, pp. 1–8. DOI: 10.1155/2015/685786
[24] K. B. Shaban, A. Kadri, E. Rezk, “Urban air pollution monitoring system with forecasting models,” IEEE Sensors Journal, vol. 16, no. 8, 2016, pp. 2598–2606. DOI: 10.1109/JSEN.2016.2514378
[25] W. Zhao, T. H. Go, “Quadcopter formation flight control combining MPC and robust feedback linearization,” Journal of the Franklin Institute, vol. 351, no. 3, 2014, pp. 1335–1355. DOI: 10.1016/j.jfranklin.2013.10.021
[26] J. Schmidhuber, “Deep learning in neural networks: An overview,” Neural Networks, vol. 61, 2015, pp. 85–117. DOI: 10.1016/j.neunet.2014.09.003
[27] M. Havaei, A. Davy, D. Warde-Farley, A. Biard, A. Courville, Y. Bengio, C. Pal, P.-M. Jodoin, H. Larochelle, “Brain tumor segmentation with deep neu-
ral networks,” Medical Image Analysis, vol. 35, 2017, pp. 18–31. DOI: 10.1016/j.media.2016.05.004
[28] C.-C. Hsu, K. -S. Wang, H.-Y. Chung, S.-H. Chang, “A study of visual behavior of multidimensional scaling for kernel perceptron algorithm,” Neural Computing and Applications, vol. 26, no. 3, 2015, pp. 679–691. DOI: 10.1007/s00521-014-1746-2
[29] Ross T. J., Fuzzy logic with engineering applications, John Wiley & Sons, 2009.
[30] M. Cococcioni, L. Foschini, B. Lazzerini, F. Marcelloni, “Complexity reduction of Mamdani Fuzzy Systems through multi-valued logic minimization,” Proceedings of the IEEE International Conference on Systems, Man and Cybernetics, Singapore, 12–15 Oct. 2008, pp. 1782–1787. DOI: 10.1109/ ICSMC.2008.4811547
[31] M. S. Gharajeh, S. Khanmohammadi, “Dispatching Rescue and Support Teams to Events Using Ad Hoc Networks and Fuzzy Decision Making in Rescue Applications,” Journal of Control and Systems Engineering, vol. 3, no. 1, 2015, pp. 35–50.
DOI:10.18005/JCSE0301003
[32] M. S. Gharajeh, S. Khanmohammadi, “DFRTP: Dynamic 3D Fuzzy Routing Based on Traffic Probability in Wireless Sensor Networks,” IET Wireless Sensor Systems, vol. 6, no. 6, 2016, pp. 211–219.
DOI: 10.1049/iet-wss.2015.0008
[33] S. Khanmohammadi, M. S. Gharajeh, “A Routing Protocol for Data Transferring in Wireless Sensor Networks Using Predictive Fuzzy Inference System and Neural Node,” Ad Hoc & Sensor Wireless Networks, vol. 38, no. 1–4, 2017, pp. 103–124.
[34] M. S. Gharajeh, “FSB-System: A Detection System for Fire, Suffocation, and Burn Based on Fuzzy Decision Making, MCDM, and RGB Model in Wireless Sensor Networks,” Wireless Personal Communications, vol. 105, no. 4, 2019, pp. 1171–1213.
DOI: 10.1007/s11277-019-06141-3
[35] M. S. Gharajeh, “Implementation of an Autonomous Intelligent Mobile Robot for Climate Purposes,” International Journal of Ad Hoc and Ubiquitous Computing, vol. 31, no. 3, 2019, pp. 200–218.
DOI: 10.1504/IJAHUC.2019.10022345
[36] M. S. Gharajeh, “A Knowledge and Intelligence-based Strategy for Resource Discovery on IaaS Cloud Systems,” International Journal of Grid and Utility Computing, vol. 12, no. 2, 2021, pp. 205–221. DOI: 10.1504/IJGUC.2021.114819
[37] M. S. Gharajeh, H. B. Jond, “Speed Control for Leader-Follower Robot Formation Using Fuzzy System and Supervised Machine Learning,” Sensors, vol. 21, no. 10, 2021, Article ID 3433. DOI: 10.3390/ s21103433
[38] B. C. Ko, K.-H. Cheong, J.-Y. Nam, “Early fire detection algorithm based on irregular patterns of flames and hierarchical Bayesian Networks,” Fire Safety Journal, vol. 45, no. 4, 2010, pp. 262–270. DOI: 10.1016/j.firesaf.2010.04.001
[39] B. U. Töreyin, Y. Dedeoğlu, U. Güdükbay, A. E. Cetin, “Computer vision based method for real-time fire and flame detection,” Pattern Recognition Letters, vol. 27, no. 1, 2006, pp. 49–58. DOI: 10.1016/j.patrec.2005.06.015
[40] B. C. Ko, K.-H. Cheong, J.-Y. Nam, “Fire detection based on vision sensor and support vector machines,” Fire Safety Journal, vol. 44, no. 3, 2009, pp. 322–329. DOI: 10.1016/j.firesaf.2008.07.006
Submitted: 21st July 2021; accepted 22nd February 2022
H. Suhaimi, N. N. Aziz, E. N. Mior Ibrahim, W. A. R. Wan Mohd IsaDOI: 10.14313/JAMRIS/2-2022/12
Abstract:
In alignment with smart city initiatives, Malaysia is shifting its educational landscape to a smart learning environment. The Ministry of Education (MoE) has made History a mandatory subject for passing the Malaysian Certificate of Education to grow awareness and instil patriotism among Malaysian students. However, History has been known as one of the difficult subjects to study for many students. On the other hand, the Malaysian Government Education Blueprint 2013-2025 seeks to “leverage ICT scale up quality learning” across the country. Therefore, this study aims to identify the factors that influence the intention to use Augmented Reality (AR) for mobile learning in learning History subject among secondary school students in Malaysia. Quantitative approach has been chosen as the research method for this study. A direct survey was conducted on 400 secondary school students in one of the smart cities in Malaysia as the target respondents. The collected data are analysed through descriptive statistics and Multiple Linear Regression analysis by using Statistical Package for the Social Sciences. Based on the results, the identified factors that influence the intention to use AR for mobile learning in learning History subject are Gender, Perceived Usefulness, Perceived Enjoyment, and Attitude Towards Use. The identified factors can be a good reference for schools and teachers to strategize their teaching and learning methods in pertaining to History subject among secondary school students in Malaysia. Future studies may include the study of various types of schools in Malaysia and explore more moderating effects of demographic factors.
Keywords: Smart City Education, Mobile Learning, Augmented Reality, History, Technology Acceptance Model
With the integration of various digital technologies into the learning and teaching process, the demand for smart learning has grown steadily in recent years, especially in smart city scenarios. Citizens’ engagement with smart city ecosystems in various ways using smartphones and mobile devices has paved a new way for a better learning experience in a smart learning environment [1].
Advanced technology in education should be applied at the school level so that the learning process will become more interactive especially for a factual and informative subject such as History. In addition, effective utilization of technology can develop the interest in learning among students in the classroom while making the teaching and learning process more interactive and meaningful. Thus, technology in education plays a significant role to assist the student to understand the subject better to increase the number of students to pass History subject in order to obtain the Malaysian Certificate of Education.
The Malaysian Government Education Blueprint 2013-2025 roadmap seeks to “leverage ICT to scale up quality learning” across the country. The importance of improving the curriculum in order to keep up with emerging changes has therefore been recognized and is being introduced as one of the 11 adjustments in the roadmap of pre-school and post-secondary education [2].
Mobile learning is considered as a part of a new educational environment introduced by affordable and portable supporting technologies. Mobile learning enhances ubiquitous learning experiences anytime and anywhere with the aid of mobile devices such as tablets, smartphones, PDA, and so on. Nowadays, mobile learning is projected to be used in both formal and informal learning in educational institutions [3]. In addition, mobile learning approach supports Goal 4 of Sustainable Development Goal (SDG) which is focusing on Quality of Education [4].
Furthermore, according to Gartner [5], the Top 10 Strategic Technology trends for 2019 are autonomous things, augmented analytics, AI-driven development, digital twins, empowered edge, immersive experience, blockchain, smart spaces, digital privacy and ethics, and quantum computing. Strategic technology trends can be defined as disruptive technology that can give an impact on the emerging state for the next five years. AR is one of the immersive technologies which can change the interaction between users with the world. Thus, it is hoped that AR would be an appropriate technology that will help students in enhancing the experience and interactivity in learning History subject.
A study conducted by Mohamed & Zali, in their research on enhancing essay abilities on History subject, recognized some challenges students had
with the topic [6]. In their research, it was found that students are lack interest in reading, have difficulty in memorizing a number of facts, have no interest in the topic, incapacity to understand certain topics and inefficient teaching methods by the teachers. One of the reasons that contribute to the challenges above is due to the huge number of factual data packed in textbooks [7].
In addition, a study by Magro [8] stated History subject was regarded by students as less interactive and demotivating. This is probably because the teaching process is separated from the reality and experience of the students. In fact, the SPM Subject Grade Point Average (SGPA) of History subject decline from 5.23 in 2017 to 4.98 in 2018. Literature analysis by Yilmaz [9] focusing on trends of AR in education between the year 2016 to 2017 shows that AR technology was mostly introduced in primary and graduate education. In addition, science education is the researchers’ most explored field of education. Therefore, it can be concluded that there is still a lack of studies and attention to the application of AR technology in secondary school and History subject. A feasibility study has been conducted on the readiness of M-Learning AR in learning History [10].
On the other hand, Technology Acceptance Model (TAM) is the most valid and robust model developed by Davis [11]. TAM perceives the user in determining behaviour of people in utilizing technology. Besides, the ability to predict people’s acceptance on technology by measuring their intentions, ability to explain their intentions in terms of their attitudes towards use, perceived usefulness, perceived ease of use, and external variables.
Despite promising features of mobile learning and AR, there is still a lack of implementation acceptance of these technologies at schools in Malaysia. Therefore, this study aims to identify the factors that influence the intention to use AR for mobile learning in learning History subject among secondary school students in Malaysia.
The Malaysian government Education Blueprint 2013-2025 roadmap seeks to “leverage ICT scale up quality learning” across Malaysia (Malaysia Ministry of Education, 2012) [2]. The significance of improving the curriculum in order to keep up with evolving trends has therefore been recognized and is being implemented as one of the 11 revisions to the strategic plan for Pre-school and Post-Secondary Education. In order to achieve Shift 7 which is to leverage ICT to scale up quality learning across the country, mobile learning plays an important role in this paradigm. AR technology has great potential to provide a better teaching and learning experience in challenging subjects such as History.
Based on preliminary study, most teachers and students are lack of awareness and understanding of AR mobile learning technology. This statement has been supported by the interview sessions with three History teachers and six students from selected
secondary schools. According to the teachers, the exposure of mobile learning through AR technology is still not exposed enough among Malaysian teachers, especially among senior teachers. Most of them still prefer to teach in a traditional way by using a whiteboard, books, and hands-on. Even though History subject was introduced at the school approximately three decades ago, the teaching methods can still be seen as traditional approach which depends entirely on the textbook. On the other hand, History subject is one of challenging subject to teach and learn since it requires a lot of memorizations of factual and dates with events happened in the past. As for the students, they also stated that they are not aware of mobile learning and AR technology since lack exposure in their learning process at the school.
Even though there is a number of mobile learning applications available in the market, these applications still do not get enough attention from both teachers and students. Features such as animation, audio 3D graphics, and other media content can provide many remarkable benefits for the students which can make learning History subject more interactive.
In addition, nowadays, the majority of the students own smartphones which can make it easier for them to get access to these applications on their devices.
However, there is still a lack of AR technology exposure and implementation in mobile learning for History subject. Therefore, there is a need to identify the influence factors of interactive mobile learning based on AR to assist teachers and students to enhance the learning experience through the new promising technology.
IEEE IoT Initiative’s Smart Cities Working Group defines a Smart City as an urban area that uses technological or non-technological services or products that can enhance the social and ethical well-being of its citizens, provide quality, performance, and interactivity of urban services to reduce costs and resource consumption, and increase contact between citizens and government [12].
With the integration of various digital technologies into the learning and teaching process, the demand for smart learning has grown steadily in recent years, especially in smart city scenarios. As the need for life-long learning has become more important in the digital age, smart learning environments in cities should be equipped to meet people’s demands. Furthermore, smart learning represents one of the key applications of smart cities [13]. Taking into consideration of the significant roles that education and learning play in the development of sustainability of a city, smart learning environments could be seen as a response to the needs of the new knowledge society [14]. By utilizing current advanced technology, smart education enables the process of teaching and learning to become more effective, efficient, flexible,
and comfortable [15]. Pallavi stated in his study that Smart Education can lead to a smart city [16].
In this technology era, smartphones play a significant role in the current education system. The educational applications for mobile devices have become an essential tool to provide accessibility, user-friendly, and efficient teaching and learning processes. It provides students with numerous opportunities to expand their use of Information and Communication Technologies [17].
Existing mobile learning definitions vary depending on the context that the authors and their studies focus on. Some literature focuses on technological aspects [18] and there are also broader definitions that include the role of the student [19] in the context of mobile learning as in the case of Petrova and Li which define mobile learning as “an omnipresent learning activity that occurs by person-to-person interaction using a mobile device.” The researchers discuss the technical and accessibility aspect, and three basic components of mobile learning which are software, application, and pedagogical architecture [20]. Mobile learning was developed from distance learning (d-learning) and electronic learning (e-learning) methods [21, 22, 23]. It is a learning continuum that provides users with flexibility. The word “learning” meant mobility because mobile learning should take place everywhere and at any time [10]. According to Shuib [24], mobile learning methods have in fact been practiced in Europe and the United States education systems.
According to Adkins [25], mobile learning has spread across the globe primarily due to the launch of dozens of successful Mobile Learning value-added service products sold directly to consumers. As of 2016, development in educational technology is heavily focused on four forms of learning goods: simulation-based, game-based, cognitive, and mobile learning. The market is flooded with highly advanced, low-cost products.
Augmented reality (AR) refers to a simple combination of real and virtual (computer-generated) worlds. Given a real subject, captured on video or camera, the
technology ‘augments’ that real world image with extra layers of digital information” [26].
Augmented Reality (AR) is a technology that allows computer-generated sensory input like images, video, or even sound to exactly overlay physical objects in real-time. On the other hand, virtual reality technology enabling the user to immerse them in the created virtual environment. AR allows users to interact and view 2-Dimensional or 3-Dimensional data with the real environment. Besides, AR is placed in between the real-world and the virtual. Both have the same environment namely “mixed reality”. Where, mixed reality shows the real environment shown in integrates digital information [27].
As shown in Figure 1, a real environment that is the reality that can be observed when looking at the real-world with the virtual environment is a scale of Milgram’s Reality-Virtuality Continuum [28]. Space real environment and virtual environment called mixed reality (MR) in the space continuum [29]. The Continuum MR easy to determine as the environment in which the real-world and the virtual world are combined together. Augmented reality and augmented virtually are the two main elements that support MR. As shown in Figure 1 AR is a combination of a real object with a small amount of data the virtual while AV is a virtual element that contains more digital data written in a virtual environment.
AR can be described as a condition where the user can see a mixture of objects in the virtual and realworld in real-time. Many researchers describe AR as a general system that incorporates data such as photographs and video streaming [30].
Although AR appears to have the potential for mobile learning, there are some challenges that hinder the educational content provider from including AR in the learning context. This may be attributed to the need for software storage and computing processing power that goes beyond the limitations of mobile devices. In contrast, the high cost of server-based solutions and relatively low connectivity networks linking mobile devices to databases are other factors that delay the introduction of AR [31].
AR implementation is rapidly increased in alignment with technology advancement. For instance,
the current version of mobile operating systems is equipped with features that can provide AR mapping tools to provide graphical information to users. Additionally, images have a more significant memory effect than text and can, therefore, facilitate the preservation of details by laying additional images and facts about the AR world [27].
The Theory Acceptance Model (TAM) is a model developed by Davis to predict in a workplace environment the acceptance of new technology [11]. Acceptance prediction and assessment are based on Perceived Usefulness (PU) and Perceived Ease of Use (PEoU) towards the system or technology. TAM is the most influential model used to study the new technology’s acceptance in various contexts. TAM also is one of the most well-known and well-developed models in the examination of the usage and acceptance of IT technology and related devices [32]. It provides a conceptual framework that is built on theories of social psychology. It also suggests the relationship between psychological aspects, attitude, intention, and behaviour to explain IT technology user acceptance.
As shown in Figure 2, the original Technology Acceptance Model (TAM) consists of 6 variables. These variables are related and may affect the actual system’s acceptance. Davis also explained that Perceived Usefulness is defined as the degree to which a user believes their job performance could be enhanced by using specific IT technology. The variable can be categorized into two types which are constructs variables and measured variables. Construct variables consist of three variables which are Perceived Usefulness, Perceived Ease of Use, and Attitudes Toward Use. The measured variable consists of other three variables which are External Variables, Intention to Use, and Actual System Usage. External variables are a
self-measure such as years of experience, job category, or familiarity with the technology. Intention To Use refers to the number of times the respondent’s intent to use the technology and lastly, Actual System Usage which is referred to the actual times the system usage by the users.
The aim of this study is to identify the factors that influence the intention to use AR for mobile learning in learning History subject among secondary school students in Malaysia. Based on the identified factors, the proposed recommendations to increase the acceptance of AR for mobile learning in learning History is discussed in this study. A direct survey was conducted with 400 students from a secondary school in one of the smart cities in Malaysia as the target respondents.
A demonstration video on how AR works in the learning process was demonstrated to the students before they completed the survey. From the video, the students could see interactive components such as 3D models, animation, graphics, sound, and video that create an immersive experience, which comprise the AR application. Based on observations made while the demonstration video was being played, they expressed their curiosity and desire to try and use it in the future for their learning process.
A set of questionnaires has been distributed to form four students from a selected school. Figure 3 shows the research model that has been adapted and modified for this study based on the Technology Acceptance Model (TAM).
Intention to Use depends on the independent variables, which are Perceived Usefulness, Perceived Ease of Use, Perceived Enjoyment, Attitude Towards Use, and Gender.
Perceived Usefulness in this study focuses on the implementation of AR-based mobile learning where it can improve the user’s efficiency. Perceived Ease of Use refers to the student’s belief that mobile learning does not need any effort to conduct or use it. Perceived Enjoyment refers to how AR-based mobile learning is considered enjoyable by the students. Attitude Towards Use refers to the students’ behaviour either positive or negative behaviour towards using MAR.
The aim of this study is to identify the factors that influence the intention to use AR for mobile learning in learning History subject among secondary school students in Malaysia. Based on the identified factors, the study proposed few recommendations to increase the acceptance of AR for mobile learning in learning History. A direct survey was conducted with 400 students from a secondary school in one of the smart cities in Malaysia as the target respondents. A set of five-point Likert-scale closed-ended questionnaires was used as an instrument for data collection and among Form Four students from selected secondary school in one of the smart cities in Malaysia as target respondents.
From 400 respondents, only 391 respondents have given complete feedback on the questionnaire survey. This section will discuss the results and findings of this study.
Table 1 shows the respondent’s gender where 61.9% of respondents are male and 38.1% are female.
The collected data were analysed using Pearson’s correlation coefficient is to determine the relationship between two independent variables and the dependent variable. The dependent variable is Intention to Use (ITU) while the independent variables are Gender, Perceived Usefulness (PU), Perceived Ease of Use (PEOU), Perceived Enjoyment (PEN), and Attitude towards Use (ATU). According to Cohen [33], to determine whether the relationship strength is weak, moderate, or strong it can be referred to its r value. If r < 0.30 or r < –0.30, the relationship is weak. If 0.30 > r <0.50 or –0.30 > r < –0.50, the relationship is moderate. If r > –0.50 or r >–0.50, the relationship is strong.
The result of the analysis reveals significant differences between the difference in Gender and ITU with r = –0.274 and ρ = 0.000. Besides, the results in determining the relationship between the PEOU and ITU also show a positive strong correlation with r = 0.616 and ρ = 0.000. The results between PEOU and ITU also indicated a strong relationship with r = 0.600 and ρ = 0.000. In addition, based on the results, PEN is independent of ITU with r = 0.748, ρ = 0.000. The strongest relationships of study are the relationships between ATU and ITU with r = 0.833 and ρ = 0.000. As a summary, the r value for all constructs (Gender, PU, PEOU, PEN, ATU) has a strong relationship as shown in Table 2.
MLR is the statistical procedure to predict the values of a response (dependent) variable from a collection of the predictor (independent) variable values [34]. MLR is used to identify the factors that influence intention to use AR for mobile learning in learning History subject among secondary schools in Malaysia.
According to Table 3, the model summary adjusted R² is 0.732. This indicates that 73% of study factors influence ITU explained in this model while 27% is influenced by other factors that may contribute to the intention to use mobile learning based on AR. According to Maxwell (2010), this is a large effect on the model.
(ρ =.000). From the ρ -value this study found that PEOU is the only factor that does not have a significance towards intention to use.
Table 4 tabulated the results of multiple regression analyses for the research model. To find the value of y (dependent variable), the unstandardized B value of independent variables was calculated. The equation is y = X_1 β_1 + X_1 β_2 + …X_κ β_κ + ε. Therefore, the predicted value of y=0.911.
This section discusses the findings from the analysis result. This study aims to identify the factors that influence the intention to use AR for mobile learning in learning History subject among secondary school students in Malaysia. A direct survey was conducted from a secondary school in one of the smart cities in Malaysia as the target respondents. This study focuses on student’s intention to use AR in mobile learning on History subject.
Based on the analysis result, most of the null hypotheses failed to reject and only one null hypothesis is rejected.
Table 5 shows the summary of hypothesis testing for this study. Hypothesis H01, H02, Hα3, H04, and H05 was supported, while hypothesis Hα1, Hα2, H03, Hα4, Hα5 was not supported.
Figure 4 shows the result of the research model. The figure indicates the significance levels of every factor towards intention to use mobile learning based on AR. The results show ρ -value of gender (ρ =.000), PU (ρ =.019), PEOU (ρ =.880), PEN (ρ =.023), and ATU
This study focuses on five independent variables, which are Perceived Usefulness, Perceived Ease of Use, Perceived Enjoyment, Attitude Towards Use, and Gender. According to the MLR results, there was a highly significant effect of gender (β=-0.227, ρ= .000) showing that gender gives a big impact on the intention of using AR for mobile learning. Furthermore, Perceived Usefulness also gives a significant effect as (β=-0.96, ρ = .019) on an intention to use showing that students feel the use of AR in mobile learning is helpful for their learning process. This result is in line with the findings by Ibáñez et al. [35] and Cabero Al-menara et al. [36] who found that there is a positive effect from PU on an intention to use mobile learning.
Furthermore, based on the findings, it was also found that there was a significant impact of PEN
No Hypothesis Result
H01 Gender will significantly influence the Intention to Use (ITU) of mobile learning based on AR Supported
Hα1 Gender not significantly influence the Intention to Use (ITU) of mobile learning based on AR Not supported
H02 Perceived Usefulness (PU) will significantly influence the Intention to Use (ITU) of mobile learning based on AR Supported
Hα2 Perceived Usefulness (PU) not significantly influence the Intention to Use (ITU) of mobile learning based on AR Not Supported
H03 Perceived Ease of Use (PEOU) will significantly influence the Intention to Use (ITU) of mobile learning based on AR Not supported
Hα3 Perceived Ease of Use (PEOU) not significantly influence the Intention to Use (ITU) of mobile learning based on AR Supported
H04 Perceived in Enjoyment (PEN) will significantly influence the Intention to Use (ITU) of mobile learning based on AR Supported
Hα4 Perceived in Enjoyment (PEN) not significantly influence the Intention to Use (ITU) of mobile learning based on AR Not Supported
H05 Attitude towards Use (ATU) will significantly affect the Intention to Use (ITU) of mobile learning based on AR Supported
Hα5 Attitude towards Use (ATU) not significantly affect the Intention to Use (ITU) of mobile learning based on AR Not Supported
(β=0.151, ρ = .023) on an intention to use AR for mobile learning. It shows that students find this kind of technology is enjoyable and can enhance their feelings to enjoy their learning process with fun. This finding was consistent with the findings of Ibáñez et al., Cabero Al-menara et al. and Poong et al. who found that perceived enjoyment had a positive impact on intention to use [35, 36, 37]
According to the findings, ATU also was found as a factor that has high significance on an intention to use as the value was (β=0.778, ρ = .000). The result indicates that the student has a positive attitude towards the intention to use mobile learning based on AR for their learning process. This finding was consistent with studies conducted by Ibáñez et al. [33] and Cabero Al-menara et al. [34] that found that there is a positive effect from Attitude Towards Use on an intention to use mobile learning. Based on these findings, Perceived Ease of Use is the only factor that does not have significance on an intention to use as the value was (β=-0.06, ρ = .880).
The first objective of this research is to identify the factors that influence the intention to use AR for mobile learning in learning History subject among secondary school students in Malaysia. According to the analysis result, four from five factors have been identified as the influence factors of the intention to use AR for mobile learning in learning History subject among secondary schools in Malaysia.
Based on the MLR analysis results, this study found that Gender and Attitude Towards Use are strong significant factors towards intention to use AR for mobile learning as both of them have .000 ρ-value, while the other two Perceived Usefulness and Perceived Enjoyment also have a positive significance that contributes to the intention to use R for mobile learning.
Figure 5 illustrates the factors that influence the intention to use AR for mobile learning in learning
History subject among secondary school in Malaysia. Based on the analysis result, there are four factors that influence the intention to use AR for mobile learning in learning History subject among secondary school in Malaysia. The four factors are Gender, Perceived Usefulness, Perceived Enjoyment, and Attitude Towards Use. The results also show that gender and Attitude Towards Use show a high significance towards intention to use AR for mobile learning which is (ρ =.000). According to the result, it shows gender has a high significance towards Intention to Use, which means that gender plays a big role as a factor.
Fig. 5. Factors that Influence Intention to Use AR for Mobile Learning in Learning History Subject among Secondary School in Malaysia
5.2. Recommendation to Increase Influence
Factors on Intention to use AR for mobile learning in learning History Subject among Secondary School in Malaysia
Based on the results of the analysis, there are four factors that influence the intention to use AR for mobile learning in learning History subject among secondary school in Malaysia. The four factors are Gender, Perceived Usefulness, Perceived Enjoyment, and Attitude towards Use. Gender gives a strong significance towards intention to use AR for mobile learning. This indicates that the gender-based digital divide between the intention to use mobile learning based on AR within the secondary school context is still big. In order to ensure that both genders play the same role equally, awareness programs about mobile learning should be organized by the school in order to attract students’ interest in using mobile learning as a part of their learning process.
Some recommendations could be suggested to increase the factor Perceived Usefulness in using interactive mobile learning based on AR. Perceived usefulness is people’s belief in using a system or technology can increase their job performance. Thus, Mobile Augmented Reality applications should come out with great content, detailed information, accessibility, and speed, as well as availability since they believe that it can improve their performance and understanding in the learning process [38].
Furthermore, Perceived Enjoyment is also a significant factor towards intention to utilize on intention to use AR for mobile learning. Perceived Enjoyment is how the system or technology is considered enjoyable by itself. In order to increase this influence factor, the application should be developed by having more interactive features in order to make the application is fun to use by the students. Elements such as audio, animation, graphics, and any media content can help to increase students’ interactivity in the learning process [39]. By having more interactive Mobile Aug-
mented Reality, the students will be more engaged in using the application in their learning process.
The last influence factor is Attitude towards Use, which is having highly significant towards intention to use. Attitude towards use is people’s behaviour either positive or negative behaviour towards utilizing a system or technology.
Another approach to make students having a positive attitude in using Mobile Augmented Reality towards their learning style is that both teachers and education institutions must encourage the use of Mobile Augmented Reality in the teaching process so that students can have a positive attitude towards using such educational application. By introducing Mobile Augmented Reality application with good features of AR for mobile learning applications such as providing good navigation, increase the usability of the application, enhance user experience, and taking into consideration of Human Computer Interaction (HCI) principles, it can increase student’s satisfaction on using applications and will develop a positive attitude towards Mobile Augmented Reality [40].
The aim of this study is to identify the factors that influence the intention to use AR for mobile learning in learning History subject among secondary school students in Malaysia. Based on the analysis result, the factors that influence the intention to use AR for mobile learning in learning History subject among secondary school students in Malaysia are Gender, perceived usefulness, perceived enjoyment, and attitude towards use.
The identified factors can be a good reference for schools and teachers to strategize their teaching and learning methods in pertaining to History subject among secondary school students in Malaysia. Future studies may include the study of various types of schools in Malaysia and explore more moderating effects of demographic factors.
In conclusion, this study contributes towards a better insight for education institutions in Malaysia towards the development of a smart learning environment. The identified factors can be a good reference for schools and teachers to strategize their teaching and learning methods in pertaining to History subject among secondary school students in Malaysia. The use of AR technology in mobile learning can provide many benefits in enhancing learning experiences for better educational purposes in the future. Future studies may include the study of various types of schools in Malaysia and explore more moderating effects of demographic factors.
H. Suhaimi – Faculty of Computer and Mathematical Sciences, UniversitiTeknologi MARA (UiTM), 40450, Shah Alam, Selangor, Malaysia.
N. N. Aziz* – The Nielsen Company Sdn Bhd, 46100, Petaling Jaya, Selangor, Malaysia, azizn3255@gmail. com
E. N. Mior Ibrahim – Faculty of Computer and Mathematical Sciences, UniversitiTeknologi MARA (UiTM), 40450, Shah Alam, Selangor, Malaysia.
W. A. R. Wan Mohd Isa – Faculty of Computer and Mathematical Sciences, UniversitiTeknologi MARA (UiTM), 40450, Shah Alam, Selangor, Malaysia.
*Corresponding author
[1] Nikolov R, Shoikova E, Krumova M, Kovatcheva E, Dimitrov V, Shikalanov A. Learning in a smart city environment. Journal of Communication and Computer. 2016, 13(7): 338–50.
[2] Ministry of Education. (2012). Preliminary Malaysia Education Blueprint 2013–2025 Available from http://www.moe.gov.my/userfiles/file/ PPP/Preliminary-Blueprint-Eng.pdf
[3] Chang W H, Liu Y C, Huang T H. Perceptions of learning effectiveness in M-learning: scale development and student awareness. Journal of Computer Assisted Learning. 2017, 33(5): 461–72.
[4] SDG. (2016). Goal 4: Quality Education. Retrieved September 1, 2019, from https://www. undp.org/content/undp/en/home/sustainable-development-goals/goal-4-quality-education.html
[5] Gartner. (2019). Gartner Top 10 Strategic Technology Trends for 2019. Retrieved June 12, 2019, from https://www.gartner.com/smarterwithgartner/gartner-top-10-strategic-technology-trends-for-2019/.
[6] Mohamad R N, Md Z. Zali. Increasing the skill of answer esey questions for History paper 2 SPM. In Proceeding Education Research Seminar IPBA 2005, (pp. 106–113).
[7] Ahmad A R, Awang M M. KURIKULUM STANDARD SEKOLAH RENDAH (KSSR) MATA PELAJARAN SEJARAH: PERLAKSANAANDAN CABARAN. In Proceeding 7th International Seminar on Regional Education 2016, 1: 435–445.
[8] Magro G, De Carvalho J R, Marcelino M J. Improving History Learning through Cultural Heritage, Local History and Technology. International Association for the Development of the Information Society; 2014.
[9] Yilmaz RM. Augmented reality trends in education between 2016 and 2017 years. State of the
art virtual reality and augmented reality knowhow. 2018, 81: 97.
[10] Taharim NF, Lokman AM, Hanesh A, Aziz AA. Feasibility study on the readiness, suitability and acceptance of M-learning AR in learning history. In AIP Conference Proceedings 2016 Feb 1 (Vol. 1705, No. 1, p. 020009). AIP Publishing LLC.
[11] Davis FD, Bagozzi RP, Warshaw PR. Extrinsic and intrinsic motivation to use computers in the workplace 1. Journal of applied social psychology. 1992, 22(14): 1111–32.
[12] Hammons R, et. al. Smart Cities. IEEE Internet of Things Magazine 2019, 2. 8–9.
[13] Liu D, Huang R, Wosinski M. Contexts of smart learning environments. In Smart Learning in Smart Cities 2017, (pp. 15–29). Springer, Singapore.
[14] Liu D, Huang R, Wosinski M. Smart learning in smart cities. Springer Singapore; 2017.
[15] Zhu Z T, Yu M H, Riezebos P. A research framework of smart education. Smart learning environments. 2016, 3(1): 1–7.
[16] Pallavi P, & Madhurank K. Smart education leads to a smart city. International Journal of Advance Research in Science and Engineering 2017, 6(1).
[17] Stylianidis P. Mobile learning: open topics, concept and design of a learning framework. In 2015 International Conference on Interactive Mobile Communication Technologies and Learning (IMCL) 2015, (pp. 421–424). IEEE.
[18] Quinn C. mLearning: Mobile, wireless, in-yourpocket learning. LiNE Zine. 2000, 2006: 1–2.
[19] O’Malley C., Vavoula G., Glew J. P., Taylor J., Sharples M., Lefrere P., Lonsdale P., Naismith L., Waycott J., Guidelines for learning/teaching/tutoring in a mobile environment.
[20] Sánchez Prieto J. C., Olmos Migueláñez S., García-Peñalvo F. J., Understanding mobile learning: devices, pedagogical implications and research lines.
[21] Brown T H. Towards a model for m-learning in Africa. International Journal on E-learning. 2005; 4(3): 299–315.
[22] Keegan D. The incorporation of mobile learning into mainstream education and training. In World Conference on Mobile Learning, Cape Town 2005, (Vol. 11).
[23] Taharim N. F., Lokman A. M., Isa W. A., Noor N. L., A relationship model of playful interaction, interaction design, kansei engineering and mobile usa-
bility in mobile learning. In 2013 IEEE conference on open systems (ICOS) 2013, (pp. 22–26). IEEE.
[24] Shuib A. S. Rekabentuk Kurikulum M.-Pembelajaran Sekolah Menengah: Teknik Delphi. In Proceedings of Regional Conference on Knowledge Integration in Information and Communication Technology 2010, (pp. 652–665).
[25] Adkins S. S. The 2016-2021 worldwide selfpaced eLearning market: The global eLearning market is in steep decline. Ambient Insight. 2016.
[26] Maxwell K. Augmented Reality also AG. In: Macmillan Dictionary 2010.
[27] Jamali S., Shiratuddin M. F., Wong K., An overview of mobile-augmented reality in higher education. International Journal on Recent Trends In Engineering & Technology. 2014, 11(1): 229–38.
[28] Milgram P., Kishino F., A taxonomy of mixed reality visual displays. IEICE TRANSACTIONS on Information and Systems. 1994, 77(12): 1321–9.
[29] Nincarean D., Alia M.B., Halim N.D., Rahman M.H., Mobile augmented reality: The potential for education. Procedia-social and behavioral sciences 2013, 103: 657–64.
[30] Cubillo J., Martín S., Castro M., Diaz G., Colmenar A., Botički I., A learning environment for augmented reality mobile learning. In 2014 IEEE Frontiers in Education Conference (FIE) Proceedings 2014, (pp. 1–8). IEEE.
[31] Gotow J. B., Zienkiewicz K., White J., Schmidt D. C., Addressing challenges with augmented reality applications on smartphones. In International Conference on Mobile Wireless Middleware, Operating Systems, and Applications 2010, (pp. 129–143). Springer, Berlin, Heidelberg.
[32] Park E., del Pobil A.P., Technology acceptance model for the use of tablet PCs. Wireless personal communications. 2013, 73(4): 1561–72.
[33] Cohen J., Statistical power analysis for the behavioral sciences. Academic press; 2013.
[34] Sinharay S., An overview of statistics in education.
[35] Ibáñez M. B., Di Serio A., Villarán D., Delgado-Kloos C., The acceptance of learning augmented reality environments: A case study. In 2016 IEEE 16th International Conference on Advanced Learning Technologies (ICALT) 2016, (pp. 307–311). IEEE.
[36] Cabero-Almenara J., Fernández-Batanero J.M., Barroso-Osuna J., Adoption of augmented reality technology by university students. Heliyon. 2019, 5(5): e01597.
[37] Poong Y. S., Yamaguchi S., Takada J. I., Investigating the drivers of mobile learning acceptance among young adults in the World Heritage town of Luang Prabang, Laos. Information Development. 2017, 33(1): 57–71.
[38] Zawaideh F., The Effect of Mobile Learning on the Development of the Students’ Learning Behaviors and Performance at Jordanian University”, International Journal of Business and Management Invention 2017, 6: 1–7.
[39] Almara’beh H., Amer E. F., Sulieman A., The effectiveness of multimedia learning tools in education. International Journal. 2015, 5(12).
[40] Crearie L., Human computer interaction (HCI) factors in technology enhanced learning. In Proceedings of the International Conference on Information Communication Technologies in Education 2013, (pp. 99–108).
Submitted:8th September2021;accepted:24th March2022
DOI:10.14313/JAMRIS/2‐2022/13
Abstract:
Therapiddevelopmentofroboticsinvolveshuman‐robot interaction(HRI).Itisanecessarytoassessusersatis‐factiontodevelopHRIeffectively.Thus,HRIcallsforin‐terdisciplinaryresearch,includingpsychologicalinstru‐mentssuchassurveyquestionnairedesign.Here,wepre‐sentafactoranalysisofaPolishversionoftheGodspeed Questionnaire(GSQ)usedtomeasureusersatisfaction. Thequestionnairewasadministeredto195participants. Then,factoranalysisoftheGSQwasperformed.Finally, reliabilityanalysisofthePolishversionoftheGSQwas done.Theadaptedversionofthesurveywascharacte‐rizedbyafour‐factorstructure,i.e.,anthropomorphism, perceivedintelligence,likeability,andperceivedsafety, withgoodpsychometricproperties.
Keywords: human‐robotinteraction(HRI),Godspeed questionnaire,UserExperience,perceptionoftherobot
Overthelast20years,oneobservetheevolu‑ tioninroboticsdevelopmenttowardsinteractionwith humans.Avarietyofrobotsperformtheirtasksby sharingspacewithhumansorworkingforthem,often engagingthemselvesininteractionswithhumansto accomplishtheirtasks.Assistive,medical,social,and entertainmentrobotsarethemostprominentexam‑ plesofthistechnologicaltrend,de�inedbythedom‑ ainofsocialrobotics.Thistendencycanbeobser‑ vedwhenanalyzingtheaimsandscopesofseveral recentEUfundedprojects,e.g.,FELICE,RAMCIP,Re‑ MeDi,DREAM,andLIREC[28–32].
Human‑robotinteraction(HRI)stillisanopenre‑ searchprobleminallfundamentalroboticdimensi‑ ons,includingperception,reasoning,oracting[26]. Thecurrentstateoftheartinscienceandtechnology showsintensivedevelopmentinbuildingrobotsca‑ pableofcooperationandsocialinteractionswithhu‑ mansatasatisfactorylevelinselectedareasoflife.It alsostillexempli�iesthematuringstagesoftechno‑ logicaladvancesofsocialrobots.Eachnewrobotic designinthecaseofhuman‑robotinteractionneeds evaluationsbasedonuni�iedandstandardizedinstru‑ mentssothatitcanbeimproved.
Attheleveloftechnicalspeci�icationandevalua‑ tionofHRI,psychologicalstudieshavebeguntoplay afundamentalroleinunderstandingtheimpactofso‑ cialrobotsonhumans.Therehavealsobeenseveral reportsfromthe�ieldofHRIpublishedinPoland(for instance,seeJAMRISarticles,e.g.:[18],[21]).Thepsy‑
chologyandengineeringcommunitieshaverealized thatHRIpresentsspeci�icchallengesregardingmea‑ suringrobotsfunctioningascooperatingpartnersfor humans.Evaluationsofindustrialrobotsmainlydeal withtheirperformanceandeffectivenessinhuman environments[6, 7]andcanbeappliedpreciselygi‑ venthetechnologicalconstraintsofrobotictechno‑ logy.However,effectiveinteractionsbetweenhumans andsocialrobotsalsoinvolvepsychologicalvariables, includingusersatisfaction[7].
IntheHRI�ield,thequalityofaproductisde�i‑ nedinISO9241normsbytwofactors:usabilityand userexperience(UX).Theformerisde�inedas,�the extenttowhichaproductorservicecanbeusedby speci�ieduserstoachievespeci�iedgoalsoneffective‑ ness,ef�iciency,andsatisfactioninaspeci�iedcontext ofuse�.Thelattercanbede�inedasusers’judgment ofaproductarisingfromtheirexperienceoftheinte‑ ractionandthequalitiesoftheproduct,intermsofthe effectiveuseoftheproductanduser’spleasure[24]. Inroboticsystems,UXevaluationmethods,especially forthosewithsharedattentioncapability,arenotap‑ parent.Inthecaseofcomputersystems,toevaluate UX,wecanuseaquestionnaire[16],inwhichparti‑ cipantsanswerquestionsregardingtheirexperience andsatisfactionfromworkingwiththesystem.The mostpopularquestionnairesintheareaofHCIareSUS (SystemUsabilityScale)andUMUX(UsabilityMetric forUserExperience)[17].Theyenablesubjectiveas‑ sessmentofusabilityanduserexperienceusingitems rankedontheLikertscale.
Thecommercialpotentialformeasuringusersa‑ tisfactionofagivenproduct,suchasthesocialro‑ bot,shouldalsonotbeoverlooked.Manypsycholo‑ gicalandmarketingstudiesaimatassessingtheaf‑ fectivestatesthatappearintheuser’smanifestedbe‑ haviorsorientedtowardstheproduct.Suchaffective factorsincludeattitudestowardtheproduct[14]and usersatisfaction[15].Thesevariableslargelydeter‑ minewhethertheuserdecidestobuythegoods,so theyshapethecommercialpotentialofagivenpro‑ duct[14].
Steinfeldandcolleagues[23]haveproposedthe ideaofintroducingastandardizedsetofHRImeasu‑ rementsthatconsiderusersatisfaction.Psychological measuresofusersatisfactioncanbedoneinseveral ways.Forinstance,onecanobserveauser’sbehavior foralongertimetoseehowtheuserengagesinthe interactionwiththesocialrobot.Behavior‑basedmea‑ surementsbasedonlong‑termobservationoftheuser oftenusevideorecordings[8].Long‑termobservation
ofHRImaybeproblematic,astheremaybemental statesunderlyingbehaviorsirrelevanttousersatis‑ faction.Forexample,theusermaybeinvolvedinthe interactionwiththerobotbecauseoffeelingsofsatis‑ factionduetoothercompetingcognitiveactivitieslin‑ kedtotheinteraction.
Othermeasuresofusersatisfactionarebasedon psychophysiologicalsignalslinkedwithheartactivity orelectricalskinconductivity.However,suchmea‑ surementsfaceinterpretationalissueswhenmaking cleardistinctionsbetweeninternalstates[20],e.g., euphoriaandangermayresultinthesamesignals fromthebody.Thethirdstandardmeasureofusersa‑ tisfactionisapsychologicalquestionnaire,whichisa seriesofquestionsaskedtouserstogainrelevantin‑ formationaboutagiventopic.Thistypeofmeasure‑ menthasprosandcons.Ononehand,thequestion‑ naireenablesquickmeasurement,isrelativelyeasyto apply,andalsoischaracterizedbysuf�icientprecision [10].Ontheotherhand,itmaybeinaccuratebecause oftheuser’stendencytogivebiasedanswers,i.e.ans‑ wersthattheyfeelaresociallyacceptable.
AvalidatedquestionnairemeasureofHRIisthe GodspeedQuestionnaire(GSQ),whichisavailableina varietyoflanguagesincludingEnglish,German,Spa‑ nish,Chinese,Japanese,French,Greek,Arabic,and Dutch[27].TheGQShasbeeneffectivelyusedforeva‑ luatingmanytypesofrobots[7, 27].TheGQShasbeen used,forexample,toassessamedicalrobot[3]that autonomouslymovesthroughcorridorstypicallyused byhumans.TheoriginalGSQversionproposesme‑ asuringusersatisfactionbyevaluatinguser’feelings andperceptionstowardsdistinctfeaturesofthero‑ bot:anthropomorphism,animacy,likeability,percei‑ vedintelligence,andperceivedsafety[7].Inparticu‑ lar,thefactorofanthropomorphismdescribestheat‑ tributionalprocessesofassigninghumancharacteris‑ ticstononhumanobjects,e.g.,robots,computers,or animals.Thesubscaleofanimacymeasurespercep‑ tionofthedegreetowhichmovingobjectsthatcan bedistinguishedasbeingalive.Thegradientof“being alive”distinguisheshumansfrommachines.Thesubs‑ caleoflikeabilitydescribespositivefeelingsandemo‑ tionsforothersorproducingpositiveevaluationsof theperceivedobject.Thefactorofintelligencerefers totherobots’abilitytoexpressintelligentandhuman‑ likebehavior.Finallyperceivedsafetymeasuresfee‑ lingsofdangerandcomfortwheninteractingwiththe socialrobot,indicatinguserwillingnesstoacceptaso‑ cialrobotasapartner.AccordingtoBartnecketal.[7], this�ive‑factorstructureofGSQhasprovenremarka‑ blyeffectiveinHRIresearch.Theoriginalfactorstruc‑ tureofGQSmaybesubjecttochangewhenadapting thispsychologicalmeasureintootherculturalandso‑ cialconditions.Thisstudyexaminethefactorstruc‑ tureofthePolishversionoftheGQSquestionnaireand itspsychometricparameters.
Thedevelopmentofanyrobotintendedtointeract withtheenvironmentnotonlymechanicallybutalso socially,requiresmeaningfulandobjectiveevaluation. Evaluationresultsarecrucialforimprovingandtu‑
ningtheultimateversionoftherobot.Here,weconsi‑ dertheGQSquestionnaireasatoolusedatacertain stageoftheintelligentroboticsystemdevelopment processratherthanameasurementtoolforgaininga pieceofnewknowledgeinHRI.Themeta‑analysisby WeissandBartneckreportedthatTheGodspeedQue‑ stionnaireSeriesarethemost‑publishedHRImeasu‑ rementininterdisciplinaryorcomputerandrobotic sciencejournals[27].
Onehundredandninety‑�ivepersons(110 woman and 85 men),agedbetween 18 and 58 (mean =26 44, SD =8 19),participatedinthestudy.Thepartici‑ pantswereundergraduatestudentsfromtheWrocław branchofUniversityofSocialSciencesandHumani‑ ties,WroclawUniversityofScienceandTechnology andUniversityofZielonaGóra.Allparticipantstook partinthisstudyafter�illingouttheinformedconsent forms.ThestudywasconductedfollowingtheDecla‑ rationofHelsinki.TheEthicsCommitteeattheInsti‑ tuteofPsychology,SWPSUniversityofSocialSciences andHumanitiesapprovedtheprotocol.
WeusedthePolishversionoftheGodspeedQuesti‑ onnaire,whichwasadaptedfrom�ivequestionnaires byBartnecketal.[7]thatmeasuredhumanpercep‑ tionsofdifferentrobotfeatures.Thetoolismadeof 24 itemsforming�ivesubscalesofusersatisfactionin HRI:anthropomorphism,animacy,likeability,percei‑ vedintelligence,andperceivedsafety.Eachiteminclu‑ desstatementsthatareassessedbyparticipantsona 7‑pointLikert‑typescalefrom 1 (totallydisagree)to 7 (totallyagree).TheauthorshavemadethePolishver‑ sionoftheGodspeedQuestionnaireavailableforpu‑ blicusefreeofcharge(seeAppendix).
Thestudyusedashortvideopresentingamo‑ ving,humanoidrobotNAO.Thevideowastheresult ofcollaborationbetweentheDepartmentofCyber‑ neticsandRoboticsandtheDepartmentofCompu‑ terScienceattheWrocławUniversityofScienceand Technology.Itpresentedtherobotplayinganinte‑ ractivegamewithanadult.Afterthevideopresen‑ tation,participantswereaskedto�illoutthePolish versionoftheGodspeedQuestionnairetoassesstheir perceptionsofanthropomorphism,animacy,likeabi‑ lity,perceivedintelligence,andperceivedsafetyofthe robot.
Toidentifyspeci�icconceptsoftheGodspeedin‑ ventory,aPrincipalComponentAnalysis(PCA)was conducted.Accordingtotheassumptionthatthefac‑ torsconstitutedifferentialscales,aVarimaxrotation methodwasperformed,whichisanorthogonalrota‑ tionmethodassumingthatthefactorsintheanalysis areuncorrelated.
TheanalysisestablishedfourGodspeedscales witheigenvalues > 1 0 (13 26, 2 16, 1 30,and 1 10, respectively).Additionally,aninspectionofthescree plotindicatedafour‑factorstructure.Inthenextstep, weusedPCAcombinedwithaVarimaxrotationmet‑ hodalongwithKaisernormalizationtoidentifyafour‑ factorsolutionofthescale.
Thefactorloadingsforthefour‑factorsolutionare presentedinTable 1.Thissolutionindicatedthatthe �irstscaleincluded 10 items,thesecondandthirdsca‑ leswerecomposedof 5 items,andthefourthscalehad only 3 items(oneitemisthereversescorestatement). Weincludedstatementswithfactorialloadingsabove thevalue 0 4 [11].Someoftheseitemsmetthecriteria fortheinclusionofseveralfactorsatthesametime.Fi‑ nally,theseitemswereincludedinthescalewithhig‑ herfactorialloadings.Allitemsmetthecriteriaforin‑ clusionintooneoftheselectedfactors.Thetotalvari‑ anceofthefour‑factorsolutionwas 74 24%,yieldinga valueof 27.29%forthe�irstfactor, 19.88%forthese‑ condfactor, 18.91%forthethirdfactorand 8.16%for thefourthfactor.
Thescalelabelsweretermedasfollows:Scale1–Anthropomorphism,2– PerceivedIntelligence,3– Li‑ keability,and4– PerceivedSafety.Scale1consisted ofelevenitemsconcerningtheattributionofahuman form,humancharacteristics,orhumanbehavior(e.g., Conscious vs Unconscious).Inthisfactor,allitems fromtheoriginalversionwereincluded.However,the statementsintheoriginalversionofGSQcomposing thescaleofanimacy(e.g.,dead vs. alive;stagnant vs. lively)formedthe�irstscaleinthePolishversion.Fac‑ tor2included�iveitemsregardingtheperceptionof therobotasintelligent,whichdependsonitscompe‑ tence(e.g.,incompetent vs. competent).Thethirddis‑ tinguishedconceptislabeledaslikeability(e.g.,un‑ pleasant vs. pleasant),andconsiderspositive�irstim‑ pressionsoftheobject,oftenleadingtomorepositive evaluationsofthatobject.Thelastfactorwasformed bythethreeitemsoftheperceivedsafetyscale,which referstotheuser’sperceptionofthelevelofdanger wheninteractingwitharobotandtheuser’slevelof comfortduringtheinteraction(e.g.agitated vs. calm).
Inthenextstep,weassessedthereliabilityofthe GodspeedscalebycalculatingtheCronbach’salphare‑ liabilitycoef�icientsforeachfactor.Weobtainedthe followingvaluesoftheCronbach’salphas:
1) Anthropomorphism α =0.947;
2) PerceivedIntelligence α =0.932;
3) Likeability α =0 927;
4) PerceivedSafety α =0 536
Thereliabilityofthe�irstthreescaleswasvery high,indicatingthehomogeneousstructureofthepar‑ ticularsub‑scales.Thealphavalueofperceivedsafety isbelow 0 7,andhenceweconcludedthatthelikeabi‑ litysubscalehadinsuf�icientinternalconsistencyre‑ liability.Forthatreason,weanalyzedthereliability afterremovingselecteditems.Thisproceduresigni�i‑ cantlyimprovedtheperceivedsafetyreliabilityafter deletingitem3:Quiescent vs. Surprised(Cronbach’s α =0 840).Thisitemhasbeenremovedfromthe scale.
SeveralHRIstudies[23]indicatetheimportanceof standardizingmethodsformeasuringHRI.Aninter‑
disciplinaryapproachtoadequatelymeasureHRIta‑ kesintoaccountusersatisfactionandrelevantpsycho‑ logicalaspectsofinteractionwiththesocialrobot[7]. Nowadays,standardizedassessmentsofuser’psycho‑ logyinHRIusessurveyingresultsfromastandardized GSQ[7].
ThisworkexaminetheadaptationofthePolish versionoftheGSQ.WeadaptedtheGSQtoPolishcultu‑ ralsettingsbyperformingfactoranalysis.Theanalysis revealedthefour‑factorstructureofusersatisfaction inhandlingHRI.Thefactorsincludedanthropomor‑ phism,perceivedintelligence,likeability,andpercei‑ vedsafety.Theresultingdiscrepanciesbetweenthe originalversionandthePolishversionarenothing unusualsincetheconceptofusersatisfactionisacom‑ plexphenomenonandmaybemodi�ied[7].Neverthe‑ less,oneshouldbearinmindthatadaptationofthe psychologicalquestionnaireforagivenculturemay substantiallychangeitsfactorstructure[10].Itturned outthattheanimacysubscalevanishedfromthePo‑ lishversion.Thissubscalemostlikelyconfusedthere‑ spondentsregardinganimacyandanthropomorphism aspreviouslyindicatedbyBartnecketal.[7],which hadsuggestedthatanthropomorphicobjectsmaybe alsoperceivedasanimatedandviceversa.InthePo‑ lishversion,mostitemspertainingtoanimacywere movedtotheanthropomorphismsubscale.
Goodpsychometricpropertiesofthesubscales characterizedthePolishadaptationoftheGSQ.The Cronbach’salphareliabilitycoef�icientswereanthro‑ pomorphism α =0 947;perceivedintelligence α = 0.932;likeability α =0.927;andperceivedsafety.Af‑ terremovingselecteditems,weendedupwiththe value 0 840.Moreover,lowerreliabilityofthesafety subscalewasdetectedbeforeremovingtheitemsfrom thefullversion.Thiseffectisinlinewithpreviousstu‑ dies[27]whichreporteddif�icultieswiththissubscale becauseofthesmallnumberofsafetyitems.Another questionnairemeasureofHRIproposedbyNomura andcolleagues[19]includedsafetywithmoreitems andhadbetterreliability.
AsmentionedintheIntroduction,currentdeve‑ lopmentinsocialroboticsstronglyfocusesoncoope‑ rationandinteractionwithhumans.Thisimpliesthat psychologicalresearchshouldbeappliedindesigning modernroboticprojects,inparticular,atthestageof systemevaluation.Thefollowingselectedworkscan serveasexamples[1, 2, 4, 5, 9, 12, 13, 25].Theseworks areaboutsocialrobotics,theexceptionbeingArentet al.[1].ThestudypresentsthedesignofthemedicalRe‑ MeDirobotthatwasevaluatedusingtheGQS.Undoub‑ tedly,thepresentproposalofthePolishadaptationof theGQSwillcontributetoadvancing�ieldsofsocial roboticsandHuman‑RobotInteractionsinPolandand willexpandabatteryofPolishquestionnairesaddres‑ singHRImeasurements,initiatedbyPochwatkoand colleagues,who�irsthadmadeavailableHRImeasure‑ mentsuchasNARS‑PL(PolishVersionoftheNegative AttitudeTowardRobotsScale)fortheJAMRIScommu‑ nity[22].
Table1. PrincipalComponentAnalysisandfactorloadingoftheGodspeeditems(N =195)forfour‐factorsolution. Note:Theitemsincludedintheparticularsubscalesarepresentedinbold.Thevaluesabove 0 3 wereestablishedas inclusioncriteria.
ThePolishversionoftheGSQ,whichisavailablefor publicusefreeofcharge,isincludedinTable 2.
RemigiuszSzczepanowski –DepartmentofCom‑ puterScienceandSystemsEngineering,Wroclaw UniversityofScienceandTechnology,Poland, e‑mail:remigiusz.szczepanowski@pwr.edu.pl,www: www.pwr.edu.pl.
TomaszNiemiec –1 InstituteofPsychology,WSB UniversityinTorun,Torun&Wrocław,Poland;Uni‑ versityofLowerSilesia,Wrocław,Poland,e‑mail: tomasz.niemiec@dsw.edu.pl,www: 1 www.wsb.pl, 2 www.dsw.edu.pl.
EwelinaCichoń –1 InstituteofPsychology,WSB UniversityinTorun,Torun&Wrocław,Poland;Uni‑ versityofLowerSilesia,Wrocław,Poland,e‑mail: ewelina.cichon@dsw.edu.pl,www: 1 www.wsb.pl, 2 www.dsw.edu.pl.
KrzysztofArent –DepartmentofCyberneticsandRo‑ botics,WroclawUniversityofScienceandTechnology, Poland,e‑mail:krzysztof.arent@pwr.edu.pl,www: www.pwr.edu.pl.
MarcinFlorkowski –InstituteofPsychology, FacultyofEducation,PsychologyandSocio‑ logy,UniversityofZielonaGóra,Poland,e‑mail: m.�lorkowski@wpps.uz.zgora.pl,www:psycholo‑ gia.wpps.uz.zgora.pl.
JanuszSobecki∗ –DepartmentofComputerScience
andSystemsEngineering,WroclawUniversity ofScienceandTechnology,Poland,e‑mail:ja‑ nusz.sobecki@pwr.edu.pl,www:www.pwr.edu.pl.
∗ Correspondingauthor
ACKNOWLEDGEMENTS
WethankMałgorzataGakis,aformerPhDstudent,for collectingthesurveydatafromundergraduatestu‑ dentsattheUniversityofSocialSciencesandHuma‑ nities.
TheauthorswishtothankDr.ChristophBartneck foragreeingtotranslateandpublishthePolishversion oftheGodspeedQuestionnaire.
[1] K.Arent,M.Cholewiński,ŁukaszChojnacki, W.Domski,M.Drwiȩga,J.Jakubiak,M.Ja‑ niak,B.Kreczmer,A.Kurnicki,B.Stańczyk,and D.Szczȩśniak‑Stańczyk,“SelectedTopicsinDe‑ signandApplicationofaRobotforRemoteMedi‑ calExaminationwiththeUseofUltrasonography andAscultationfromthePerspectiveoftheRE‑ MEDIProject”, JournalofAutomation,MobileRo‑ boticsandIntelligentSystems,vol.11,no.2,2017, 82–94,10.14313/JAMRIS_2‑2017/20.
[2] K.Arent,M.Gakis,J.Sobecki,andR.Szczepa‑ nowski,“TowardEmotionRecognitionEmbo‑ diedinSocialRobots:ImplementationofLa‑ banMovementAnalysisintoNAORobot”, Jour‑
nalofAutomation,MobileRoboticsandIn‑ telligentSystems,vol.11,no.4,2017,3–7, 10.14313/JAMRIS_4‑2017/31.
[3] K.Arent,J.Jakubiak,M.Drwiȩga,M.Cholewiń‑ ski,G.Stollnberger,M.Giuliani,M.Tscheligi, D.Szczȩśniak‑Stańczyk,M.Janowski,W.Brzo‑ zowski,andA.Wysokiński,“Controlofmobile robotforremotemedicalexamination:Design conceptsandusers’feedbackfromexperimental studies”.In: 20169thInternationalConference onHumanSystemInteractions(HSI),vol..,2016, 76–82,10.1109/HSI.2016.7529612.
[4] K.Arent,J.Kruk‑Lasocka,T.Niemiec,andR.Sz‑ czepanowski,“Socialrobotindiagnosisofau‑ tismamongpreschoolchildren”.In: 201924th InternationalConferenceonMethodsandModels inAutomationandRobotics(MMAR),vol..,2019, 652–656,10.1109/MMAR.2019.8864666.
[5] K.Arent,M.Wnuk,andM.Kabała,“Towardsthe TherapeuticSphericalRobot:Design,Program‑ mingandControl”.In: EighthInternationalIFAC SymposiumonRobotControl.Proceedings,vol.8, 2006.
[6] C.Bartneck,T.Belpaeme,F.Eyssel,T.Kanda, M.Keijsers,andS.S�abanović, Human‑RobotIn‑ teraction:AnIntroduction,CambridgeUniversity Press,2020,10.1017/9781108676649.
[7] C.Bartneck,D.Kulić,E.Croft,andS.Zoghbi, “Measurementinstrumentsfortheanthropo‑ morphism,animacy,likeability,perceivedintel‑ ligence,andperceivedsafetyofrobots”, Inter‑ nationalJournalofSocialRobotics,vol.1,no.1, 2009,71–81,10.1007/s12369‑008‑0001‑3.
[8] C.BartneckandM.Rauterberg,“HCIReality‑ an’UnrealTournament’?”, Int.J.Hum.‑ Comput.Stud.,vol.65,no.8,2007,737–743, 10.1016/j.ijhcs.2007.03.003.
[9] R.Budziński,J.Kȩdzierski,andB.Weselak. Pro‑ blemyrobotyki.MateriałyXIKrajowejKonferen‑ cjiRobotyki.PraceNaukowe,Elektronika:z.175, tom1,chapterSocialrobotheadSamuel‑con‑ struction�inPolish�.��icynaWydawniczaPoli‑ technikiWarszawskiej,2010.ISSN:0137‑2343.
[10] D.A.Dillman. TheLogicandPsychologyofCon‑ structingQuestionnaires,chapterchapter9.Rou‑ tledge,2008.
[11] A.Field, DiscoveringStatisticsUsingIBMSPSS Statistics,SagePublicationsLtd.,2013.
[12] J.Kȩdzierski,P.Kaczmarek,M.Dziergwa,and K.Tchoń,“DesignforaRoboticCompanion”, In‑ ternationalJournalofHumanoidRobotics,vol.12, no.01,2015.
[13]
J.Kȩdzierski,R.Muszynski,C.Zoll,A.Oleksy,and M.Frontkiewicz,“EMYS—EmotiveHeadofaSo‑ cialRobot”, InternationalJournalofSocialRobo‑ tics,vol.5,2013,237–249.
Human‑RobotInteraction,vol..,NewYork,NY, USA,2006,33–40,10.1145/1121241.1121249.
[24] A.Sutcliffe.“DesigningforUserEngagement: AestheticandAttractiveUserInterfaces”.In: SynthesisLecturesonHuman‑CenteredInforma‑ tics,volume2,1–55.SpringerCham,2009.
[14]
[15]
D.T.Kenrick,S.L.Neuberg,andR.B.Cialdini, So‑ cialpsychology:Unravelingthemystery(3rded.), PearsonEducationNewZealand,2005.
Y.‑F.Kuo,C.‑M.Wu,andW.‑J.Deng,“Therelati‑ onshipsamongservicequality,perceivedvalue, customersatisfaction,andpost‑purchaseinten‑ tioninmobilevalue‑addedservices”, Computers inHumanBehavior,vol.25,no.4,2009,887–896, https://doi.org/10.1016/j.chb.2009.03.003,In‑ cludingtheSpecialIssue:TheUseofSupportDe‑ vicesinElectronicLearningEnvironments.
[25] K.SydorandK.Arent. Problemyrobotyki.Materi‑ ałyXIKrajowejKonferencjiRobotyki.PraceNau‑ kowe,Elektronika:z.175,tom1,chapterFROGIT: asociallyinteractiverobot(inPolish).O�icyna WydawniczaPolitechnikiWarszawskiej,2010. ISSN:0137‑2343.
[26] R.Szczepanowski,E.Cichoń,K.Arent,J.So‑ becki,P.Styrkowiec,M.Florkowski,and M.Gakis,“Educationbiasesperceptionof socialrobots”, EuropeanReviewofApplied Psychology,vol.70,no.2,2020,100521, https://doi.org/10.1016/j.erap.2020.100521.
[16]
J.R.Lewis,“IBMComputerUsabilitySatisfaction Questionnaires:PsychometricEvaluationand InstructionsforUse”, InternationalJournalof Human‑ComputerInteraction,vol..,1995,57–78.
[27] A.WeissandC.Bartneck,“MetaAnalysisofthe UsageoftheGodspeedQuestionnaireSeries”. In: 201524thIEEEInternationalSymposiumon RobotandHumanInteractiveCommunication (RO‑MAN),vol..,2015,381–388,10.1109/RO‑ MAN.2015.7333568.
[17]
J.R.Lewis,B.S.Utesch,andD.E.Maher. UMUX‑ LITE:WhenThere’sNoTimefortheSUS,2099–2102.AssociationforComputingMachinery, NewYork,NY,USA,2013.
[18] A.Moussaoui,A.Pruski,andB.Cherki,“Inte‑ ractionmanagementbetweensocialangentsand human”, JournalofAutomation,MobileRobotics andIntelligentSystems,vol.5,no.1,2013,8–17.
[19] T.Nomura,T.Kanda,andT.Suzuki,“Experimen‑ talinvestigationintoin�luenceofnegativeattitu‑ destowardrobotsonhuman–robotinteraction”, AI&Society,vol.20,2006,138––150.
[20] K.Oatley,D.Keltner,andJ.M.Jenkins, Under‑ standingEmotions,2ndEdition,Wiley‑Blackwel, 2006.
[21] N.Piçarra,J.‑C.Giger,G.Pochwatko,andJ.Moża‑ ryn,“Designingsocialrobotsforinteractionat work:Socio‑cognitivefactorsunderlyinginten‑ tiontoworkwithsocialrobots”, JournalofAuto‑ mation,MobileRoboticsandIntelligentSystems, vol.10,no.4,2016,17–26,10.14313/JAMRI_4‑ 2016/28.
[22] G.Pochwatko,J.‑C.Giger,M.Różańska‑Walczuk, J.S�widrak,K.Kukie�ka,J.Możaryn,andN.Piçarra, “PolishVersionoftheNegativeAttitudeToward RobotsScale(NARS‑PL)”, JournalofAutomation, MobileRoboticsandIntelligentSystems,vol.9,no. 3,2015,65–72,10.14313/JAMRIS_2‑2015/25.
[23] A.Steinfeld,T.Fong,D.Kaber,M.Lewis,J.Scholtz, A.Schultz,andM.Goodrich,“CommonMetrics forHuman‑RobotInteraction”.In: Proceedings ofthe1stACMSIGCHI/SIGARTConferenceon
[28] “DREAM‑DevelopmentofRobot‑Enhanced therapyforchildrenwithAutisMspectrum disorders”.Projectwebsite.
[29] “FELICE‑FlexibleAssemblyManufacturingwith Human‑RobotCollaborationandDigitalTwin Models”.Projectwebsite.
[30] “LIREC‑LIvingwithRobotsandintEractive Companions”.Projectwebsite.
[31] “RAMCIP‑RoboticAssistantforMCIPatientsat home”.Projectwebsite.
[32] “ReMeDi‑RemoteMedicalDiagnostician”.Pro‑ jectwebsite.
Submitted: 3rd February 2022; accepted 12th April 2022
S. Ramalingam, S. Rasool MohideenDOI: 10.14313/JAMRIS/2-2022/14
Abstract:
In this research, a model of a robotic manipulator flexible structure and an equation of motion for controller design is planned. The structural material chosen for the robot structure was a hybrid composite. A comparison study was carried out for the aluminium 6082 alloy for the flexible manipulator arm application. Vibration behavior and control implementation was analyzed by adding joint flexibility in the system. Using a simulation algorithm, the system parameter calculation is carried out through MATLAB software for vibration amplitude, transient period, steady-state error, and settling time of flexible robotic arm system. In a systematized motion equation, flexible robotic deflections are organized via the assumed mode (AM) and Lagrange techniques (LT). The graph analysis of hybrid composite and AL6082 materials with high stiffness coefficients is plotted. These obtained values from the plot are utilized for Linear Quadratic Regulator (LQR) controller design. The LQR output facts for both aluminium structural robotic arm and composite material robotic arms are established.
Keywords: Composite material, Al6082 alloy, Simulation analysis, Vibration amplitude, LQR controller.
Nomenclatures:
EBBT – Euler-Bernoulli Beams Theory
TBT – Timoshenko Beam Theory
AMM – Assumed Mode Method
FEM – Finite Element Method
LPM – Lumped Parameter Model
HDPE – High Density Polyethylene
MATLAB – Math’s Laboratory
AL6082 – Aluminium 6082 alloy
LE – Lagrangian Equation
LQR – Linear Quadratic Regulator Control
FRP – Fiber Reinforced Polymer
The structural part of the flexible link element of a robotic manipulator with a flexible joint has the ability to reach position accuracy in an obstruction environment of industrial production or fabrication. The flexible material for the robotic link application is an alternative to giant manipulators: that is, a manipulator
which has oversize, posture, short range of work envelope with low payload lifting capacity is called a giant manipulator. Such a giant manipulator consumes vast energy, requires more task completion time, and has a problematic move-in work envelope. To overcome the issue a flexible material for the robotic link manipulator is introduced. It is lightweight, has less power absorption, has a quick processing time, fewer issues on mass moment inertia, and safe operation for the manipulator.
The main drawbacks encountered in the flexible links are residual vibration in the flexible structure. Kalyoncu [1] devised a revolving prismatic joint attached to the single-link manipulator with sliding robot arm movement and payload at the end portion of the link, an investigation of which leads to the mathematical derivation and the numerical output of a lightweight robot link manipulator with the addition of rotary-prismatic joint movement action.
Ahmad et al. [2] presented a single-link manipulator dynamic analysis which includes damping and hub inertia. The finite element method was used for system performance. Korayem et al. [3] developed modeling of a robotic manipulator with one link for analyses. The impact of beam length and shear deformation is considered using two-beam theories such as Euler-Bernoulli Beams Theory (EBBT) and Timoshenko Beam Theory (TBT). The above methods were established for performance analysis. The EBBT yields good results.
Rishi Raj et al. [4] have discussed the importance of using a digital prototype over a normal prototype for fast-growing industrial automation. At present, the industries are mechanized to meet customer demands and satisfaction. Industrial robots are engaged to perform tasks quickly and accurately. To increase the production rate, lightweight manipulators with composite materials as flexible links are developed. The composite material has the advantage of good mechanical strength, plenty of design option flexibility, comfort in the manufacturing process, good corrosion resistance, impact resistance, and outstanding fatigue strength. Due to the light weight, the moving arms experience very large elastic deformations, and stresses are formed in the loading condition of the manipulator.
The payload and weight of a robotic manipulator ratio for an existing mobile manipulator is starting from 1/10th up to 1/30th. The size of a robotic arm
with the overweight of the end effector of existing industrial robots and their lifting capacity are considered. This ratio is very low when compared to normal human workers: that is, the ratio is 1/3 to 1/5 for an individual person and sometimes more, depending upon the working environment. The increase of manipulator weight takes more time to move from initial point to final position, and the actuator size is also increased. To eliminate the above issue, the first thing is to design changes followed by new materials for robotic arms. A new type of composite material is to be developed and replaced for existing robots as per the normal human capacity ratio of 1/3. This is achieved through lightweight composite material and smart materials for the flexible robotic arm. These lightweight material numerical analysis are required for the standby of rigid and massive robotic manipulators [5]. A literature survey of dynamic modeling of the flexible single arm is discussed and approaches are listed, and also categorized as (a) Assumed Mode (AM) or Shape Function (SF) technique, (b) Finite Element (FE) analysis, (c) Elements of Lumped Mass Models and (d) Other technology studies. A single flexible or AM model with distributed parameters is included in the review robot modeling work by Dwivedy et al., [6-7] and work elaborates the control techniques of joint flexible manipulator robots.
From the information and numerical data collected from research existing work, it is clearly understood that to replace the slow speed robots with higher speed robots one must introduce low density, high mechanical strength arms with homogeneous flexibility. This is a tough task in the field of flexible robotic arm modeling and controls the approach to composite material flexible joint robotic arms. The prime investigation is to compare the steady-state of conventional and composite material flexible robotic arm behaviors.
In this research, to study the model equation of the flexible robotic manipulator arm, the design of a modern controller to reduce vibration and improve performance of a modern controller LQR is to be tested through a simulation tool on a single-link flexible manipulator of both composite and conventional materials. Numerical outputs of hybrid composite and aluminium material robotic arms are to be compared, correlated, and validated The MATLAB software tool is used for simulation output. The graphs were obtained for analysis. The additional goal is to calculate the end effector coordinates of a hybrid composite flexible one-link robotic arm and compare it with the conventional material.
The flexible material and its properties are significant for robotic link applications. The analysis of material suitability for the manipulator’s arm is inevitable for replacement giant robotic arms. The reduction of weight and mechanical properties are examined for robotic link material selection. At present, for robotic chasing, a high-density polyethylene (HDPE) material
is used which is not suitable for high-temperature environmental conditions and high payload carry tasks. The alternate is aluminum: it has light weight and is corrosion-resistant. But aluminium creates vibration when in operating conditions. Therefore, another alternate solution is to introduce new soft materials as a link as they have the benefit of higher speed, increased area mobility, more efficiency in terms of less energy consumption, and high payload carrying capacity. The use of composite material for a robot manipulator has good mechanical properties, combining two or more fiber materials. It aims to achieve the chosen physical properties, design flexibility, ease of fabrication, light weight, corrosion resistance, impact-resistance, excellent fatigue strength [8], and other desirable characteristics. The applications of robot links are a concern; varieties of fiber materials are used as links, such as carbon material fiber, boron material fiber, ceramic, glass fiber, and aramid fibers.
The robotic link parameters of aluminiun 6082 alloy material and composite material are calculated and listed in Table1. The composite lightweight material is made up of carbon, fiberglass fiber, and Kevlar fiber [9]. Using volume and weight ratio relation, the weight of material and thickness of composite materials are determined. The composite material is taken for simulation study and their parameters are given in Table 1. Both the materials were considered for the same cross-sectional area (A) and length (L) for parameter calculation to analyze the behavior of the materials. The link has also varied the mass as per material density concerned for same length.
Robotic arm kinematics deals with the analytical study of the motion of a robot arm w.r.t a fixed reference coordinate system as a function of time. Without regard to the forces or moments that cause the motion. The dynamics robotic arm deals the relation between robot arm motion and forces and moments acting on the robot. The system has pinned-free flexible link as a two degree of freedom (Dof) model.
The composite material manipulator as well as the aluminium 6082 alloy material links are considered
as the pinned-free flexible link as a 2Dof model, attached with the circular hub. The circular disc-type hub is moulded with the shaft of a rotating actuator. The rotating robotic link is a bend in a curved form freely in the two-dimensional horizontal plane due to flexibility, but rigid in a vertical position and also in torsion. The robotic arm length, width, and thickness of flexible links are hypothetical to be constant for both materials. The density of both the materials is varying. The robotic arm deformation due to shear as well as inertia due to revolving of the link is neglected as per the TBT theory [10]. The methodology flow chart is shown in Fig. 1. The schematic diagram of a robotic manipulator arrangement is given in Fig. 2.
To find the equations of motion of link, initially, the energies connected with systems are calculated. These are kinetic energy (KE), potential energy (PE), and dissipated energies [11]. The kinetic energy of the rotary hub, flexible arm, and tip-attached payload is calculated by considering small curved deflection and small angular velocity. The actuator input force τ (t) is used to calculate the nonconservative work (W) of a system, as
= (2)
The extended Hamilton’s principle is adopted. In this equation, only two modes have been taken for modeling by null rotary inertia and null shear deformation (Meirovitch, 1970). [12] To substitute the value u (x, t) in equation (1) and after simplifying, the motion equation of flexible manipulator with corresponding initial and boundary conditions are given by,
(3) where (EI) and (ρ) are the rigidity and density of the link material. The equation (3) is the “fourth-order partial differential equation” (PDE) which gives the motion equation of a flexible robotic arm dynamic model.
The solution of y (x, t) in the dynamic model of an equation of motion of manipulator as per of assumed mode method is given as,
1 (,)()() n ii i yxtxqt φ =
= ∑ (4)
where, i = 1, 2, …n, and y (x, t) is the total deflection. The displacement ϕ(x) along the length of the robotic manipulator is called mode shape or shape function and qi(t) is time arbitrary constant. It is derived by taking the y (x, t) value from equation (4) and substituting it in equation (1). After mathematical manipulation, it gives two expressions of order, ordinary differential equations. The first 4th order equation is given as, 4 4 4 (,) ()0 i ii dxt x dx φ βφ += (5)
The payload is attached at the tip of the robotic link and input torque force is given to the rotary hub of the link by an actuator. The ‘θ (t)’ is angular displacement and ‘u (x, t)’ is the flexible deflection of the link. Finally, the total displacement of link ‘y (x, t)’ is calculated. It has two functions: rigid-body rotation ‘θ (t)’ and link curved deflection ‘u (x, t)’ given in eq. (1),
Where i β is constant. For simplification the following quantities are used in the equations for numerical analysis,
where (λ) is system parameter, (Ih) and (Ib) are the hub inertia and link inertia of the system. (kc) is joint stiffness coefficient, (M) is mass of the manipulator link and ‘ε’ is the ratio between the hub and link in-
ertias which determines the vibration frequencies of the manipulator. The ‘η’ represents the ratio between payload inertia and link inertia, which is also indicative of vibration frequencies of the flexible manipulator. The equation (5) is fourth order ODE which has a general solution in the form (Ramalingam et al., 2019) as given below,
()sinsinh coscosh iiiii iiii
xAxBx CxDx φββ ββ =+ ++ (7)
To calculate the natural frequency and mode shape, the λi value satisfies the boundary conditions (BC) of the undriven flexible link manipulator. The above quantities are determined with corresponding coefficient values Ai, Bi, Ci, and Di from equation (7) using orthogonality property and boundary conditions of the systems.
The derived equations of motion based on the Lagrange principle are transformed in a state-space form [13-14] and [15] and the output as given in matrix form using state-space model as XAXBu • =+ and output as yCX = + D. The matrixes A, B, C and D are given below as,
The ‘λi’ values from Table 2 are used to calculate the natural frequency and damped natural frequency of the aluminium 6082 alloy robotic manipulator system. The obtained frequencies are shown in Table 4 and also noticed from the table that the frequencies are increased due to high flexibility in the joint. The damped natural frequencies are shown in Table 5.
(8)
(9)
The AL 6082 alloy material is considered as case-1 for robotic structural study. It is generally used as a structural component and the same is used for robotic flexible structure software simulation.
The general system characteristic equation is used to find the parameter λi (i = 0 to 4) for 1st mode, 2nd mode, and 3rd mode vibration frequencies, damped natural frequencies, mode shape constants, mode shape, tip position, deflection for conventional material aluminium for further analysis. The rigid and flexible joint system mode values λ0 to λ3 and λ4 for the payload case and the no-load case is calculated. The values are listed in Table 2. It is observed from Table 2 that the rigid mode is absent for the payload and noload case of a flexible system.
The general characteristic equation of a single-arm robotic manipulator system is resolved for (λ). The obtained values of (λ) are shown in Table 2, which are used for calculating the first mode, second mode, third mode, and fourth mode. The frequency and damped natural frequencies are determined using (λ) values from Table 2. Table 6 and Table 7 show the frequencies and damped natural frequencies for a hybrid composite material system.
The system mode shape constants are calculated using (λi) values for rigid, flexible, and high flexibility joint manipulators. To find Ai, Bi, Ci from the above equation (7) by assuming Di =1, the calculated values are shown in Table 8. These constants are used for both cases of conventional and hybrid composite materials.
Table
‘λi’ (i = 0 to 4)
The step input signal is given to the aluminium 6082 alloy robotic link manipulator and simulation results for three cases as rigid joint (kc = 0), flexible joint (kc = 1), and more flexible joint (kc = 5) are obtained [16]. The output graphs of aluminium alloy 6082 are given in Figs. 3–13.
The amplitudes of varying joint stiffness coefficient plots are shown in Figs. 3–6. The amplitudes of the highly flexible joint case of the first mode are 0.355m, the second mode is 0.495m. The system mode shapes are shown in Figs.7–8 and also observed from the plots that the node points are increased by increasing the mode shapes. Fig. 9 gives the maximum deflection of the aluminium link manipulator: the value is 0.456 m noticed from the plot when the stiffness is constant. The tip position of the flexible system measured from the initial coordinate of the system is shown in Fig. 11. The hub angle rotations of the system are shown in Fig. 12. The flexibility shown in Fig. 13.
The step input signal is given to the hybrid composite manipulator system and simulation output for three cases such as rigid joint (kc = 0), flexible joint, and highly flexible joint (kc = 5) are plotted. The output graphs are shown in Figs. 14–24. The numerical values for the composite material flexible links with different joint flexibilities are calculated.
The amplitude of highly flexible joints for composite material as a case for the first mode is noted as 4x10-14 m. The system mode shapes are shown in Figs.14–17 and also observed from the plots (Figs. 14–17) that the single node point is obtained by increasing the mode shapes.
Figs.18–19 gives the maximum deflection of composite link manipulator, and value is 0.46 m noticed from the plot when the stiffness is constant. The position of the end link of a system is measured from the fixed coordinate as shown in Fig. 22. The hub angle rotations of the system are shown in Fig. 23.
The proposed modern control system is activated and simulated through MATLAB, and consists of aluminium alloy robotic arm. Outcomes are shown in Fig. 25.
The input signal is given to the aluminium alloy 6082 structural robotic manipulators; the output response of the LQR control system [17–18], and [19] are observed. The hub angle and link deflection are not smooth at 0.6 sec. and velocity and acceleration also vary in the system up to a time period of 0.6 sec.
The modern controller technique of LQR used in the system and unit input torque to the controller. This is applied and simulation is carried out through appropriate software tools [20]. The output plot is shown in Fig. 26. The control scheme characteristic performance calculation of the hybrid composite material is interpolated. The hub angle rotation is settled smoothly at 0.4 sec and flexible arm deflection is a maximum of 0.15 m observed and settled at 0.6 sec. The system hub velocity varies initially and settles at 0.6 sec. Link velocity of the composite material is settled to the setpoint quickly without overshoot up to time t = 0.6 sec. This is shown in Fig. 21b. The output responses of LQR compared to other controllers yields better results in settling time: 0.1sec, steady-state error is 0.31% and the maximum overshoot is 5%.
(Al6082) and in case-2 analysis for hybrid polymer composite material was done. By considering these materials, the state space solution was obtained.
The composite material single link robot manipulator was used for simulation analysis. It was observed that the composite material link, soft and lightweight, took less power utilization. This was shown in Table 1. The dynamic responses of the flexible manipulators, such as natural frequencies, damped natural frequencies, are calculated as shown in Table 4 to Table 7 and tip deflection for conventional and composite materials has been carried out with flexibility conditions. The results are shown.
The vibration analysis of the system through MATLAB simulation has been conducted by using conventional and hybrid composite laminates; system output is shown in Table 4 and Table 6. The single-link manipulator under step input torque is simulated. The model numerical analysis of the robotic arm is carried out for different joint stiffness coefficients. In addition, lightweight hybrid composite has a quick response while in operating conditions. The materials such as aluminum and hybrid composite are analyzed. This analysis shows the total displacement history and variation of the hub rotation angle of the lightweight hybrid composite robotic link, which is less than that of aluminum materials. The hub angle for the composite link flexible robot manipulator is settled at 0.4 sec. But the 6082-aluminium alloy takes a long time to settle: more than 0.6 sec. The deflection of a lightweight composite material robotic arm is settled without any difficulty at 0.6 sec. But in the case of aluminium 6082 alloy there delay in settling. The velocity of a deflected link at 1.2 sec is smooth. But in the case of aluminium this varies at 0.6 seconds.
The researchers, Hafiz Muhammad Wahaj Aziz, et al. [21] investigated different control systems. The output values of the first-order controller and a super twisting side mode (SMC) controller was listed in Table 9.
The common mathematical equation for flexible robot manipulator was derived. This was commonly used for conventional material Al6082 alloy and hybrid polymer composite flexible link manipulators based on the AM method, Hamilton principle, using Lagrange presented. In case-1, analysis was conducted for aluminium alloy
The researchers, Waqar Alam et al., [22] were studied using the PID controller and the output responses controller as shown in Fig. 27. From the figure, it is observed that the system settled at desired output with a delay of 0.4363 seconds along with the settling period of time 2 sec.
The classic PID and modern controllers such as State Feedback and LQR were used in the system by adding step input to the controllers, and output responses are given in Table 10. From this Table 10, the LQR controller yields good results without overshooting. The settling time for the system is noted as 0.1sec.
S. Ramalingam – AMET University, Department of Mechanical Engineering, Chennai, India, email: sengalaniramalingam@gmail.com.
S. Rasool Mohideen* – School of Mechanical Science, BSA Crescent Institute of Science and Technology, Chennai, India-48, email: dean.sms@crescent.education
* Corresponding author
[1] M. Kalyoncu, “Mathematical modeling and dynamic response of a multi straight-line path tracing flexible robot manipulator with rotating-prismatic joint”, Applied mathematical modeling, vol. 32, no. 6, 2008, pp. 1087–98. DOI: 10.1016/ j.apm.2007.02.032
[2] M. A Ahmad, M. A Zawawi, “Effect of beam’s length on the dynamic modeling of flexible manipulator system”, 11th International Conference on Computer Modeling and Simulation, IEEE, 2009, pp. 356–61. DOI: 10.1109/UKSIM.2009.59
[3] M. H. Korayam, A. M. Shafei, S. F. Dehkordi, “Dynamic effect of beam’s length and beam’s theory on the flexible manipulator system”, International Research Journal of Applied and Basic Sciences, vol. 3, no. 7, 2012, pp. 1527–34, http:// www. irjabs.com.
By connecting the earlier author’s work, namely Hafiz Muhammad Wahaj Aziz, et al. (2016) and Waqar Alam, et al. (2018, results observed from the LQR controller simulation are found to be better.
The LQR control system is implemented to control vibration for the composite material link and AL6082 alloy link and compared. It is observed that the total deflection of the composite flexible link is reduced by 41%. But the oscillatory behavior is amplified to 40% higher than the conventional robotic arm. To suppress the vibration amplitude, a controller design is a must for composite material robotic arms. The arm endpoint elastic deflection for the hybrid composite material link is achieved at 5% less. The simulation output of LQR yields better results in settling time: 0.1sec. The steady-state error is 0.31% and the maximum overshoot of 5% is determined. The experimental development for validation of the system is in progress.
The authors wish to thank the B. S. A. Crescent Institute of Science and Technology, India, Chennai, which supported us to carry out the work under the No. 2021PDF04.
[4] Rishi Raj, Prabhat Kumar Sinha, Earnest Vinay Prakash, “Modeling, simulation and analysis of cantilever beam of different material by finite element method, Ansys & MATLAB”, International Journal of Engineering Research and General Science, vol. 3, no. 3, May-June, 2015, p. 89.
[5] E. Pereira, J. Becedas, I. Payo, F. Ramos, F., V. Feliu, V, “Control of Flexible Manipulators”, Theory and Practice, Universidad de Costilla-La Mancha, ETS Ingenious Industrials, Ciudad Real, Spain, 2010, pp. 278–9.
[6] S. K. Dwivedy, Peter Eberhard, “Dynamic analysis of flexible manipulators, a literature review,” Mechanism and Machine Theory, vol. 7, no. 41, 2006, pp. 749–77. DOI: 10.1016/j.mechmachtheory.2006.01.014
[7] S. Ozgoli, H. D. Taghirad, “A Survey on the control of flexible joint robots”, (1986-2001), Asian Journal of Control, vol. 8, 2006, pp. 1–15. DOI:10.1111/j.1934-6093. 2006.tb00285.x
[8] O.P. Kanna, “Material science and metallurgy,” Dhanpat Rai Publication, Third Edition, 2009, pp. 23.1–23.31.
[9] S Ramalingam, S. Rasool Mohideen, et al., “Hybrid polymer composite material for robotic manipulator subject to single link flexibility,” International Journal of Ambient Energy, 2019. DOI: 10.1080/01430750.2018.1557551
[10] R. Roy, CARIG, Jr., “Structural dynamics and introduction to computer methods,” John Wiley, 1981, p. 202. asmedigitalcollection.asme.org.
[11] S. Ramalingam, S. Rasool Mohideen, “Numerical Analysis of Robotic Manipulator Subject to Mechanical Flexibility by Lagrangian Method”, Proc. Natl. Acad. Sci., India, Sect. A: Phys. Sci., 2019. doi. org/10.1007/s 40010-019-00619-2
[12] S. Ramalingam, S. Rasool Mohideen, S., “Advanced structural fibre material for single link robotic manipulator simulation analysis with flexibility”, Journal of Automation, Mobile Robotics and Intelligent Systems, 2019, vol. 13, no. 4, 2019, pp. 38–46. DOI: 10.14313/JAMRIS/4-2019/36
[13] M.O. Tokhi, et al, “Flexible robot manipulators, modeling simulation and control”, IET, Control Engineering Series, vol. 68, 2008, pp. 23–33. ISBN:9780-86341-488-0.
[14] Katsuhiko Ogata., Modern control engineering, PHI publication, Fifth Edition, 2010, pp. 159–225.
[15] S. Ramalingam, S. Rasool Mohideen, P.S. Sridhar, “Composite material robot manipulator with joint flexibility-Mode and mode shape simulation,” International Journal of Recent Technology and Engineering, 2019, vol. 8, 4: pp. 902–909, DOI:10.35940/ ijrte. D7513.118419
[16] M. Joel Esposito, et. al, “Role of a MATLAB real-time hardware interface within a system modeling course”, Proceedings of the 2004 American Society for Engineering Education Annual Conference & Exposition, 2004, P.5.
[17] S. Ramalingam, S. Rasool Mohideen, S. Sounder Rajan, P.S. Sridhar, “Experimental of hybrid polymer composite material robotic single link flexible manipulator”, IJITEE, vol.9, no. 3, 2020, pp. 488–494. DOI: 10.35940/ijitee.C8461.019320
[18] Kuldeep Jayaswal, D. K. Palwalia, Suboth Kumar, “Analysis of robust control method for the flexible manipulator in reliable operation of medical robots during COVID-19 pandemic,” Microsystem Technologies, vol. 27, 2021, pp. 212, 116. DOI: 10.1007/ s00542-020-05028-9
[19] Dipendra Subedi, Ilya Tyapin, Geir Hovland, “Review on modeling and control of flexible link manipulators,” Modelling, Identification and Control, vol. 41, no. 3, 2020, pp. 141–63.
[20] S. Ramalingam, “Dynamic modeling, simulation and analysis of single link robotic manipulator with flexibility”, PhD thesis, June 2019, pp. 1034.
[21] Hafiz Muhammad Wahaj Aziz, Jamshed Iqbal, “Flexible Joint Robotic Manipulator: Modeling and Design of Robust Control Law” IEEE, 978-15090-4059-9/16, pp. 63–68, 2016. DOI: 10.1109/ ICRAI.2016.7791230
[22] Waqar Alam, Nihad Ali, Hafiz Muhammad Wahaj Aziz, Jamshed Iqbal, “Control of flexible joint robotic manipulator: design and prototyping” IEEE, 2018, 978-1-5386-0922-4/18.
Submitted: 8th February 2022; accepted 17th March 2022
Sheenal Malviya, Sourabh Dave, Kailash Chandra Bandhu, Ratnesh Litoriya*DOI: 10.14313/JAMRIS/2-2022/15
Abstract:
Cloud computing has emerged as a significant technology domain, primarily due to the emergence of big data, machine learning, and quantum computing applications. While earlier, cloud computing services were focused mainly on providing storage and some infrastructures/ platforms for applications, the need to advance computational power analysis of massive datasets. It has made cloud computing almost inevitable from most client-based applications, mobile applications, or web applications. The allied challenge to protect data shared from and to cloud-based platforms has cropped up with the necessity to access public clouds. While conventional cryptographic algorithms have been used for securing and authenticating cloud data, advancements in cryptanalysis and access to faster computation have led to possible threats to the traditional security of cloud mechanisms. This has led to extensive research in homomorphic encryption pertaining to cloud security. In this paper, a security mechanism is designed targeted towards dynamic groups using public clouds. Cloud security mechanisms generally face a significant challenge in terms of overhead, throughput, and execution time to encrypt data from dynamic groups with frequent member addition and removal. A two-stage homomorphic encryption process is proposed for data security in this paper. The performance of the proposed system is evaluated in terms of the salient cryptographic metrics, which are the avalanche effect, throughput, and execution time. A comparative analysis with conventional cryptographic algorithms shows that the proposed system outperforms them regarding the cryptographic performance metrics.
Keywords — Cloud Computing, Cloud Data Security, Dynamic Groups, Homomorphic Encryption, Chaotic Network, Throughput, Avalanche Effect.
Cloud computing has revolutionized the way computing has been performed conventionally. The emergence of cloud computing has allowed applications with constrained computational and memory resources to access platforms with highly high computational and storage prowess [1]. Using cloud-based services, users can harness the power of sophisticated
hardware or software through their remote machines. A typical cloud platform interacting with a remote client is depicted in figure 1.
In figure 1, multiple applications are being run by the cloud platform, accessible by the client (often termed as host) through the virtual machine (VM). The VM emulates a virtual interface between the host operating system and the cloud platform. If user groups are accessing the cloud platform, there is generally a group admin to oversee the group members [2]-[3]. The group members may have the authority to access, manipulate or upload data. Moreover, the groups are generally dynamic due to group members’ frequent addition and deletion. In general, several cloud service providers may or may not have access to the internal functionality of the cloud architecture, which often leads to loophole cloud security that employ conventional cryptographic techniques for cloud security.
Cloud-based security groups describe areas where different security measures can be applied. Correctly implemented cloud-based security groups help limit unlawful access to IT resources in the event of a security infringe. The motivation behind this proposed work is to provide better security mechanism for the public cloud, which is an essential requirement in today’s scenario. Cryptography is derived from the Greek word Krypto’s, which means hidden. It is the practice and study of secure communications techniques that enables only the sender and intended recipient of a data/message to view its contents. Secure Communication is observed as a scenario where the data or message shared amid two parties can’t be accessed by an adversary or hacker. The core principals of modern cryptographic algorithms are Data Integrity, Authentication, Data Confidentiality and Non-repudiation. Cryptographic algorithms are classified into two major categories: Symmetric Key Cryptography and Asymmetric Key Cryptography (popularly known as public key cryptography).
Generally, the authentication of group users is accomplished based on a group key sharing mechanism. The second issue to be addressed is the design of security mechanisms that can upload data to the cloudbased server in less time and high security to operate between the remote client and the server seamlessly. This work proposes a key generation approach to authenticate users in the group, and homographic encryption algorithms are employed to encrypt data to the cloud server.
The organization of this paper is as follows: Sect. 2 presents the system model for dynamic groups; Sect. 3 describes the proposed approach for homomorphic encryption. This section elaborates additive and multiplicative homomorphic encryption technique. The simulation setup and results are discussed in Sect. 4. The last part is Sect. 5, which concludes the research work done and experiences gained. The declarations are also mentioned in the last
Workgroups are generally dynamic, with members changing and/or members migrating to other groups [3]. Hence it is necessary to authenticate the members joining a group that has access to the cloud platform having the following accesses:
1) Fetching data
2) Manipulating data
3) Uploading data
The dynamic nature of such groups leads to the chance of impersonation, eavesdropping, and man-inthe-middle attack. With networks migrating from the wired to the wireless realm, authentication has become a severe challenge. Most of the intrusions generally occur at this level due to the ease of breakthrough into the network. However, the key should be renewed intermittently to decrease the chances of fraudulent intercepts. This is generally implemented based on a public key infrastructure (PKI) system to authenticate
users. The PKI infrastructure is usually preferred due to its relatively low complexity and ease of use. In the proposed architecture, the PKI-based authentication mechanism has the admin portal (AP), user portal (UP), and Cryptographic Server (CS) [4]. The admin portal oversees the functioning of user portals and grants access to files. The user portals can upload, download or manipulate data files [5]-[6]. Blockchain technology is also found to be a trustworthy implementation for securing transactions in many domains [24-30]. Song Li et al. [31] proposed a public auditing scheme with the blockchain technology to resist the malicious auditors. The user portals of each of the entities have unique login credentials and are authenticated using the key. The encryption is, however, done solely by the cryptographic server.
Homomorphic encryption has gained popularity due to its ability to allow computations directly on the ciphertext without the mandatory necessity of decrypting the plain text in the first place. This approach has two significant benefits [2]:
1) Enhanced Security: Since data processing can be done directly on the ciphertext, data hiding, manipulations, and multi-layer security can be accomplished relatively quickly compared to conventional encryption.
2) Lesser Execution Time: Since the need to decrypt the actual data is thwarted, execution time plummets.
Homomorphic encryption is generally categorized as additive homomorphic encryption and multiplicative homomorphic encryption.
A. Additive Homomorphic Encryption:
In this case, two large primes, p and q are selected, which yield an integer n given by:
Next, a key generator G is designed such that:
Here,
�+ is the positive set of integers.
Typically, n and G are public, while the values of p & q are private.
Let a plain text (p) and key (k) yield a ciphertext (c) given by:
Here,
E is the encryption algorithm. f stands for a function of. r is a chosen random number.
Then, the following relations hold true:
��(��,��) = �������� ������(�� 2) (4)
��(��1,��1)��(��2,��2) = ����1��1�� ����2��2�� �������� 2 (5)
Or ��(��1,��1)��(��2,��2) = ����1+��2��1��2�� �������� 2 (6)
Or ��(��1,��1)��(��2,��2) = ��(��1 + ��2,��1��2) (7)
Here,
p1 and p2 are two distinct plain texts. r1 and r2 are the two distinct random numbers chosen.
Equation (7) reveals that the separate plain texts can be obtained as a sum of the individual plain texts with the same key (k).
In this case, the following relations hold true:
��(��1)��(��2) = ��1����2��������(�� ) (8)
Or (��1��2)��������(�� ) = ��(��1��2) (9)
Equation (9) reveals that the separate plain texts can be obtained as a product of the individual plain texts with the same key (k).
Here a and b are chosen such that ���� ≡ 1
In Figure 2 the input is taken as a text as well as an image. After this a hash key is generated using MD5 and Flag Value and the text input is encrypted using AES. The image input is converted through Fresnel Transform into Homomorphic form and homomorphic Substitution algorithm. As an output encrypted text and image is generated.
The algorithm for proposed approach is used as mentioned below:
t as well as an image. After this a hash key is generated using MD5 and Flag Value and the text input is encrypted using AES. The image input is converted through Fresnel encrypted text
The algorithm for proposed approach is used as mentioned below:
Algorithm 1: Fresnel Transform, Key Generation and Encryption
Input: Plaintext (PT), Flag Value (f) and Image (I)
Compute Key:
Encryption: )
Fresnel Transform (Converting Images to Video Frames into Homomorphic Forms):
Homomorphic Substitution:
The proposed approach is an amalgamation of the Message Digest 5 (MD5) and the Advanced Encryption Standard (AES) algorithms [7]. The key is generated using the relation: (10) (11)
The proposed approach is an amalgamation of the Message Digest 5 (MD5) and the Advanced Encryption Standard (AES) algorithms [7]. The key is generated using the relation:
5
Here,
���� & ���� are chosen byte file and attribute file, respectively.
S is used to store the chosen byte file and attribute file. f is the flag value.
������ℎ����ℎ is the hash key at stage 1.
���������� �� is the encryption key of stage 2.
The first stage of encryption is done based on the AES algorithm given by:
�� ) (13)
Here, CT is Cyphertext. PT is Plaintext.
The homomorphic substitution for the second stage is done using logistic maps based on a chaotic network given by:
The fundamental idea behind the working of the algorithms is implementing chaos and making it infeasible for attackers to decrypt the data through brute force [18]. This is possible if the encryption mechanism is one way (trapdoor). It is easy to compute in one direction but infeasible to compute its inverse by applying brute force to cryptanalysis [20]. The one-way function (trapdoor) for encrypting the data is expressed as:
: Easy to Compute.
: Infeasible to Compute by Brute Force.
Algorithm 2: Encryption for Image or Video Data
Input:
// Where g denotes the function describing the dependence of the original image of
Key Generation is Based as a Function Given By:
// Here, represents the encryption key generation algorithm.
The Encrypted Image is Generated Based on a Random and Adaptive Key and Image Parameters Given By:
The image degradation function is denoted as
The image restoration function is denoted as .
The relation between and is Given By:
between the image and the Fresnel plane. also given by the convolution integral of the image I(x1,y1) and the term propagator function (p) [15]. transform can thus be computed as:
For Text Data, the Plain Text X is a Function of Only One Independent Variable to Argument, Given By:
convolution operation convolution operator. the convolution of any two functions g and h is given by: variable.
Without loss of generality, the convolution of any two functions g and h is given by:
The evaluation of the proposed system is based on the following metrics [21], [22]: (22)
Here, �� is called the translator variable.
lization of Fresnel Transform is given in figure 3. The actual computational equivalent is shown in figure 4 [16]. While the Fresnel Transform can be computed directly hardware, the Fresnel Transform is calculated indirectly using the modified version of the computationally less complex from a hardware point of view [17]
The physical visualization of Fresnel Transform is given in figure 3. The actual computational equivalent of the Fresnel Transform is shown in figure 4 [16]. While the Fresnel Transform can be computed directly but is complex on hardware, the Fresnel Transform is calculated indirectly using the modified version of the Fourier Method, which is computationally less complex from a hardware point of view [17].
Here, n is the number of bits. is the actual plain text. is the deciphered plain text after decryption.
SIMULATION RESULTS AND DISCUSSIONS
The simulation set up is presented through figure 6, which clearly shows that the user uploads the file to cryptography server and the server in turn encrypt the file using secure key and send back to user as well as upload it on cloud storage. Other users can also download the file with the help of secure key, provided to
eneration is Based as a Function Given By:
// Here, represents the encryption key generation algorithm.
eneration is Based as a Function Given By:
The Encrypted Image is Generated Based on a Random and Adaptive Key and Image Parameters Given By:
// Here, represents the encryption key generation algorithm. ncrypted Image is Generated Based on a Random and Adaptive Key and Image Parameters Given y:
// Here, represents the encryption key generation algorithm.
ncrypted Image is Generated Based on a Random and Adaptive Key and Image Parameters Given By:
The image degradation function is denoted as .
image degradation function is denoted as image restoration function is denoted as relation between and is Given By:
The image restoration function is denoted as . The relation between and is Given By:
The fundamental idea behind the working of the algorithms is implementing chaos and making it infeasible for attackers to decrypt the data through brute force [18]. This is possible if the encryption mechanism is one way (trapdoor). It is easy to compute in one direction but infeasible to compute its inverse by applying brute force to cryptanalysis [20]. The one-way function (trapdoor) for encrypting the data is expressed as:
image degradation function is denoted as image restoration function is denoted as relation between and is Given By:
�� = ��(��) : Easy to Compute
For Text Data, the Plain Text X is a Function of Only One Independent Variable to Argument, Given By:
The obtained results are presented in this section along with the values of the performance metrics: The implementation of the chaotic network can be visualized using the Mandelbrot set, which exhibits a random chaotic nature. This complies with the oneway (trapdoor) function requirements in which the one-way transform computation is feasible, but the inverse calculation is infeasible using brute force. The generator set of the chaotic network (often termed as the Mandelbrot set) is depicted in figure 10.
Text Data, the Plain Text X is a Function of Only One Independent Variable to Argument, Given By:
�� = ��–1(�� ) : Infeasible to Compute by Brute Force
Text Data, the Plain Text X is a Function of Only One Independent Variable to Argument, Given By:
D Evaluation Parameters
Evaluation Parameters
The evaluation of the proposed system is based on the following metrics [21], [22]:
The evaluation of the proposed system is based on the following metrics [21], [22]:
Evaluation Parameters
evaluation of the proposed system is based on the following metrics [21], [22]:
evaluation of the proposed system is based on the following metrics [21], [22]:
Here, n is the number of bits. is the actual plain text. is the deciphered plain text after decryption.
Here,
number of bits.
number of bits. actual plain text. deciphered plain text after decryption.
n is the number of bits.
the actual plain text.
Pia is the actual plain text.
IMULATION RESULTS AND DISCUSSIONS
the deciphered plain text after decryption.
Pid is the deciphered plain text after decryption.
The simulation set up is presented through figure 6, which clearly shows that the user uploads the file to cryptography server and the server in turn encrypt the file using secure key and send back to user as well as upload it on cloud storage. Other users can also download the file with the help of secure key, provided to
Figure 7 depicts the plain text (image data), which is a standard test image (cameraman.jpg), its encrypted counterpart. For simulation, standard .jpg images with attributes of has been chosen. The encrypted image is shown to hide the original information in the image. Moreover, data can also be hidden in the encrypted image’s least significant bits (LSB) locations. The data can be later extracted directly from the encrypted version of the image without the hard necessity to decrypt the image in the first place due to the homomorphic nature of the encryption. The essential facts about this approach are:
SIMULATION RESULTS AND DISCUSSIONS
simulation set up is presented through figure 6, which clearly shows that the user uploads the file to cryptography server and the server in turn encrypt the file using secure key and send back to user as well as on cloud storage. Other users can also download the file with the help of secure key, provided to
1) Only the reflectance co-efficient is encrypted.
simulation set up is presented through figure 6, which clearly shows that the user uploads the file to cryptography server and the server in turn encrypt the file using secure key and send back to user as well as it on cloud storage. Other users can also download the file with the help of secure key, provided to
The simulation set up is presented through figure 6, which clearly shows that the user uploads the file to cryptography server and the server in turn encrypt the file using secure key and send back to user as well as upload it on cloud storage. Other users can also download the file with the help of secure key, provided to them securely. The cryptographic algorithm is implemented using Dotnet Framework on cryptographic server and user account details are stored on Microsoft SQL Server Database and Encrypted Image is uploaded on Microsoft Azure Cloud Platform.
2) The reflectance co-efficient is again split into the Most Significant Bits (MSBs) and Least Significant Bits (LSBs).
3) The illumination co-efficient can be hidden in the LSB locations of the encrypted reflectance component.
4) Other secret images or data can also be hidden in the LSB locations to implement data hiding within the encrypted image.
5) The essence of data hiding in LSB locations makes the hidden data extremely imperceptible, thereby giving no clue to attackers about hidden data.
6) The major benefit of the techniques is the extraction of hidden data directly from the encrypted domain, not needing the decryption of the cover image.
Thus, the proposed approach supports data hiding.
The performance of the proposed system is evaluated in terms of the throughput, execution time, and the avalanche effect. Multiple runs of the proposed algorithm for varying data sizes have been depicted in figure 8.
While throughput is a measure of the speed of processing of the algorithm, the avalanche effect is a measure of the randomness of the algorithm. High throughput and avalanche effect values indicate that the proposed algorithm is fast and highly random. The MSE obtained is 1.08. The mean square error is significant for image data since the decrypted image shows slight variations or errors compared to the original plain text data. Due to positive and negative polarities of errors, it is customary to compute the mean square error.
Figures 9 and 10 depict the throughput and avalanche effect obtained by the proposed algorithm. A comparative analysis has been made w.r.t. Data Encryption Standard (DES), AES and Blowfish algorithms. It can be seen from the comparative analysis that the proposed approach outperforms the existing conventional techniques in terms of the avalanche effect and throughput.
It can be observed that the proposed algorithm outperforms the existing approaches in terms of the throughput and avalanche effect criteria. Increased avalanche effect implies that the algorithm is more sensitive to changes in initial conditions, i.e., the plain text data. The increased throughput suggests that the proposed algorithm is NOT computationally complex to be implemented practically on hardware, making it suitable for a wide range of applications on the cloud. High-speed broadband internet access and the ubiquity of mobile devices make cloud computing a viable option for many domains everywhere. several factors are driving cloud adoption. The proposed technique will benefit the cloud environment directly or indirectly in multiple ways. The most important benefit is the safety and security of the cloud. The proposed security mechanism enables the dynamic groups of the public cloud to exchange the data efficiently and securely. Through the two-stage homomorphic encryption technique, the transactions within the cloud will become seamless and secure.
The proposed work presents data security mechanisms for cloud environments accessed by dynamic groups. The security mechanism developed is a twoway approach in which the key is generated using the MD5 algorithm while the ciphertext is generated using the key and the AES algorithm. Homomorphic encryption is then implemented using the chaotic logistic map. The Mandelbrot set obtained also shown in the figure. The performance of the proposed algorithm is evaluated in terms of the avalanche effect, throughput, and execution time. The proposed approach is novel in terms of the efficient utilization of MD5 and AES to provide additional security for cloud environments. Also the work carried out in this paper contributes to the existing body of literature in the field of security and privacy. The obtained results indicate the superiority of the proposed algorithm terms of throughput, which is significantly high (137) and avalanche effects (122) as compare to the exist-
ing cryptographic techniques. The measures taken are very crucial and represent the inference metrics of speed and randomness of an algorithm. Thus, it can be concluded that the proposed approach is an effective technique for securing cloud data, especially for public clouds.
Funding: Not applicable
Conflicts of interest/Competing interests: The authors declare that there is no conflict of interest.
Availability of data and material: Data sharing not applicable to this article as no datasets were generated or analyzed during the current study.
Authors’ contributions: Sheenal Malviya, Sourabh Dave contributed to the design and implementation of the research. Kailash Chandra Bandhu and Ratnesh Litoriya performed the analysis of the results and to the writing of the manuscript. All authors discussed the results and contributed in the manuscript preparation.
Sheenal Malviya – Medi-Caps University, Indore, (M.P.) India, email: malviyasheenal7@gmail.com
Sourabh Dave – Medi-Caps University, Indore, (M.P.) India, email: sourabh.dave@gmail.com
Kailash Chandra Bandh – Medi-Caps University, Indore, (M.P.) India, email: kailashchandra.bandhu@ gmail.com
Ratnesh Litoriya* – Medi-Caps University, Indore, (M.P.) India, email: litoriya.ratnesh@gmail.com
[1] M. Sookhak, A. Gani, K. M. Khan, and R. Buyya, “Dynamic remote data auditing for securing big data storage in cloud computing,” Information Sciences, Vol. 380, pp. 101–116, 2017.
[2] P. A. Pimpalkar and H. A. Hingoliwala, “A Secure Cloud Storage System with Secure Data Forwarding,” International Journal of Scientific & Engineering Research, Vol. 4, Issue 6, pp. 3002–3010, 2013
[3] H.T.Wu, Y.M.Cheung, Z.Yang, S.Tang, “A high-capacity reversible data hiding method for homomorphic encrypted images,” Journal of Visual Communication and Image Representation, Elsevier 2019, Vol. 62, pp. 87–96.
[4] A. Bakhshandeh, Z. Eslami “An authenticated image encryption scheme based on chaotic maps
and memory cellular automata”, Journal of Optics and Lasers in Engineering, Elsevier 2013, Vol. 51, Issue 6, pp. 665–673.
[5] M. Rani, V. Kumar, “Superior mandelbrot set”, Journal of Korea Society of Mathematical Education, 2004, Vol. 8, Issue 4, pp. 279–291.
[6] M. Tebaa, S. El Hajji, A. El Ghazi, “Homomorphic encryption applied to the cloud computing security”, Proceedings of the World Congress on Engineering, 2012 Vol. 1, pp. 1–4.
[7] M.P. Babitha, K.R.R. Babu, “Secure cloud storage using AES encryption”, Proceedings in 2016 International Conference on Automatic Control and Dynamic Optimization Techniques (ICACDOT), pp. 859–864.
[8] E. Tanyildizi and F. Özkaynak, “A New Chaotic S-Box Generation Method Using Parameter Optimization of One Dimensional Chaotic Maps,” in IEEE Access, vol. 7, pp. 117829–117838, 2019.
[9] M. Alloghani, M.M. Alani, D. Al-Jumeily, T. Baker, “A systematic review on the status and progress of homomorphic encryption technologies”, Journal of Information Security and Applications, Elsevier 2019, Volume 48, 102362.
[10] L. Liu, F. Nie, A. William, Z. Li, T. Zhang, and B. C. Lovell, “Multi-Modal Joint Clustering With Application for Unsupervised Attribute Discovery,” in IEEE Transactions on Image Processing 2018, vol. 27, no. 9, pp. 4345–4356.
[11] F. Wang, J. Wang, R. Ni, Z. Zhu, and Y. Hu, “Resolution Adaptative Network for Cryptanalysis of Asymmetric Cryptosystems,” in IEEE Access, vol. 8, pp. 187419–187430, 2020.
[12] Z. Shao, X. Liu, Q. Yao, N. Qi, Y. Shang, J. Zhang, “multiple-image encryption based on chaotic phase mask and equal modulus decomposition in quaternion gyrator domain”, Signal Processing and Communication, Elsevier 2020, Volume 80, 115662.
[13] G. Luan, A. Li, D. Zhang, and D. Wang, “Asymmetric Image Encryption and Authentication Based on Equal Modulus Decomposition in the Fresnel Transform Domain,” in IEEE Photonics Journal, vol. 11, no. 1, pp. 1–7, Feb. 2019.
[14] Y. Kim, M. Sim, I. Moon, and B. Javidi, “Secure Random Phase Key Exchange Schemes for Image Cryptography,” in IEEE Internet of Things Journal, vol. 6, no. 6, pp. 10855–10861.
[15] D. Blinder, C. Schretter, H. Ottevaere, A. Munteanu, and P. Schelkens, “Unitary Transforms Using Time-Frequency Warping for Digital Holograms of Deep Scenes,” in IEEE Transactions on Compu-
tational Imaging, vol. 4, no. 2, pp. 206–218, June 2018.
[16] M. Khurana and H. Singh, “Asymmetric optical image encryption using random Hilbert mask based on fast Walsh Hadamard transform,” 2017 International Conference on Computing and Communication Technologies for Smart Nation (IC3TSN), 2017, pp. 374–377.
[17] L. Zhao, J. T. Sheridan, and J. J. Healy, “Unitary Algorithm for Nonseparable Linear Canonical Transforms Applied to Iterative Phase Retrieval,” in IEEE Signal Processing Letters, vol. 24, no. 6, pp. 814–817, June 2017.
[18] P.S. Goswami, T. Chakraborty, “Design of a Quantum One-Way Trapdoor Function”, Emerging Technology in Modelling and Graphics, Springer 2020, vol. 937, pp. 547–555.
[19] N. Döttling, S. Garg, Y. Ishai, G. Malavolta, T. Mour, “Trapdoor hash functions and their applications”, Advances in Cryptology, Springer 2019. Lecture Notes in Computer Science, vol. 11694, pp. 3–31.
[20] G. Luan, Z. Chen, C. Huang, “Silhouette-free multiple-image encryption using coherent superposition and Fresnel transform”, Optic, Elsevier 2020, Vol. 224, 165498.
[21] N. Shimbre, P. Deshpande, “Enhancing distributed data storage security for cloud computing using TPA and AES algorithm”, Proceedings in 2015 International Conference on Computing Communication Control and Automation, pp. 35–39.
[22] L. Coppolino, S. D’Antonio, G. Mazzeo, “Cloud security: Emerging threats and current solutions”, Journal of Computers and Electrical Engineering”, Elsevier 2017, Vol. 59, pp. 126–140.
[23] L. M. Bernardo and O. D. D. Soares, “Fractional Fourier transforms and optical systems,” Optics Communications, Vol. 110, no. 5–6, pp. 517–522, 1994.
[24] P. Pandey and R. Litoriya, “Securing E-health networks from counterfeit medicine penetration using Blockchain,” Wireless Personal Communications, Vol. 117, issue 1, pp. 7–25, 2020.
[25] P. Prateek and L. Ratnesh, “Promoting Trustless Computation through Blockchain Technology,” National Academy Science Letters, Vol. 44, pp. 225–231, 2020.
[26] P. Prateek and L. Ratnesh, “Securing and authenticating healthcare records through blockchain technology,” Cryptologia, Vol. 44, no. 4, pp. 341–356, 2020.
[27] P. Pandey and R. Litoriya, “Implementing healthcare services on a large scale: challenges and remedies based on blockchain technology,” Health Policy and Technology, Vol. 9, no. 1, pp. 69–78, Jan. 2020.
[28] S. Soner, R. Litoriya, and P. Pandey, “Exploring Blockchain and Smart Contract Technology for Reliable and Secure Land Registration and Record Management,” Wireless Personal Communications, Vol. 121, Issue 1, pp. 2495–2509 Aug. 2021.
[29] P. Pandey and R. Litoriya, “Ensuring elderly well being during COVID-19 by using IoT,” Disaster Medicine and Public Health Preparedness, pp. 1–10, Oct. 2020.
[30] P. Pandey and R. Litoriya, “Technology intervention for preventing COVID-19 outbreak,” Information Technology & People, vol. 34, no. 4, pp. 1233–1251, May 2021.
[31] Song Li, Jian Liu, Guannan Yang, Jinguang Han, “A Blockchain-Based Public Auditing Scheme for Cloud Storage Environment without Trusted Auditors”, Wireless Communications and Mobile Computing, vol. 2020, Article ID 8841711, 13 pages, 2020. https://doi.org/10.1155/2020/8841711
Submitted: 26th April 2022; accepted 28th July 2022
DOI: 10.14313/JAMRIS/2-2022/16
Abstract:
This paper presents a method for automatically measuring plants’ heights in indoor hydroponic plantations using the OpenCV library and the Python programming language. Using the elaborated algorithm and Raspberry Pi-driven system with an external camera, the growth process of multiple pak choi cabbages (Brassica rapa L. subsp. Chinensis) was observed. The main aim and novelty of the presented research is the elaborated algorithm, which allows for observing the plants’ height in hydroponic stations, where reflective foil is used. Based on the pictures of the hydroponic plantation, the bases of the plants, their reflections, and plants themselves were separated. Finally, the algorithm was used for estimating the plants’ heights. The achieved results were then compared to the results obtained manually. With the help of a ML (Machine Learning) approach, the algorithm will be used in future research to optimize the plants’ growth in indoor hydroponic plantations.
Keywords: Hydroponics, Image Analysis, Automatics, Mechatronic Systems, OpenCV, Phenotyping
As noted by the second United Nations Sustainable Development Goal (UNSDG) – Zero Hunger – the need for improving the agricultural productivity of small-scale food producers, together with the diversification of the food-producing systems, is one of the key problems of today [1]. Increasing the volume of crops grown in urbanized areas – especially domestically – is one of the ways to increase the availability of high-quality food in regions where the amount of space that could be used for growing plants is limited [2]. Hydroponic plantations may partially solve these problems. In hydroponic growing, plants can be grown indoors, within stacked stations, and even without access to natural light. Hydroponics reduces land requirements for crops by 75% or more, and reduces water usage by 90%, compared to the traditional methods of growing plants [3]. At the same time, hydroponics can provide a yield over ten times greater than that of conventional equivalent methods [4]. On the other hand, the greatest challenge for hydroponics is its energy consumption, especially in enclosed systems with artificial light sources. Thus,
there is a strong demand for the implementation of renewable energy sources in such applications [5]. Still, especially in regions suffering from hunger, i.e., East Africa, hydroponics is a promising technology for increasing food security. Implementation of this technology has the most potential in areas where water and fertilizer availability is limited, as well as in urbanized areas. Hydroponics also reduces negative impact on the environment compared to other methods, since it does not require pesticides and does not affect soil degradation [3, 5].
It should be underlined that this technology also shortens the supply chain and lowers the cost of healthy food, reducing transportation-related pollution [6].
With the fast development of digital technologies, the aforementioned hydroponic systems can be easily automated. Such technologies are getting cheaper and thus more affordable for domestic or SME (Small and Medium Enterprises) usage. The most promising digital technologies are image analysis and machine learning, which can be implemented alongside automation. It is even predicted that agriculture would benefit the most from digital technology implementation. Just a few applications of this technology include phenotyping, disease analysis, estimating crop yield, optimizing water usage, fertilizing, etc. [7–11].
From both food security and agricultural industry interest perspectives, one of the most important factors of crop production is cost-effectiveness, which limits both crop availability and profitability. Especially in systems based on artificial light usage, the longer a plant needs to grow, the more energy it consumes, increasing the overall cost. It also limits the production potential by occupying the system for an extended time.
In addition, the horticultural industry looks for cost reduction not only to grow food but also medicinal cannabis, the demands on light of which can be even seven times larger than in the case of crops. There are multiple studies that elaborate on various photosynthesis mechanisms in order to increase the overall cost-effectiveness and the plants’ growth rate [12–15].
The presented research’s main goal is to propose an automatic plant assessment method for indoor grow systems that could be used, e.g., for growth rate optimization. In the elaborated case, a method based on the application of Python and OpenCV is proposed, and
its implementation in a hydroponic system is shown. In chapter two, a short state-of-the-art of image processing and corresponding software tools are presented. Examples of the usage of such programs for hydroponics and crop cultivation are shown, pointing out the main difficulties in implementing these as universal tools. In the next chapter, the OpenCV library is described along with the performed experiment description, which refers to automatic height measurements of the plants grown in a hydroponic system. The goal was to extract the usable parameter for future plants’ growth optimization. The fourth chapter contains information about the software and hardware used in the setup. Chapter five contains details concerning the elaborated method’s algorithms. The sixth chapter compares results obtained with the elaborated method with those obtained with manual measurements. The last chapter contains a summary.
light as possible and reduce the same light dispersion. The drawbacks of such a solution, in the case of image analysis, are the plants’ reflections. In order to use such tools, a user would need to take the plant out of the plantation to take pictures. From the automation point of view, such a procedure is unwanted and may set the whole point under question.
Other solutions that allow multi-plant analysis, such as PlantCV, rely on setting up special markers to separate the plants’ areas and/or are based on a pattern [16]. Such an approach is hard to implement as a universal solution, requires additional space, and may cause problems when trying to implement such a solution for automatic analysis of plants, e.g., their height assessment. Since this also requires a plain background, the possibility for application for stations with reflective foil is thus limited.
Hydroponics is a method of growing plants in a liquid solution instead of soil. Most basic techniques use a simple mixture of tap water and organic fertilizers. However, these methods tend to result in lesser efficiency in growing crops. More advanced methods involve the usage of a mixture of demineralized water, synthetic fertilizers, carbon dioxide dosing, and pH balancing. In addition, some systems use reverse osmosis to reduce the amount of water waste.
As mentioned, hydroponics can be run with or without access to natural sunlight. In this case, advanced grow light systems are being used. By limiting the light spectrum to the absorption spectra of the plants’ dyes, it is possible to achieve cost-efficient light sources, which can even increase the plants’ growth rate in comparison to plants grown under natural sunlight. Additionally, due to the low power consumption of LED light sources, it is possible to use renewable energy sources with them, such as solar panels.
Machine learning techniques and digital image analysis are beneficial in automatic hydroponics applications. For example, there are a number of commercial and free software libraries supporting both digital image analysis and machine learning. Combining both can help to classify or extract key parameters of plants from digital images. The current paper focuses on digital image analysis, while in future research, it is planned to combine both.
Currently, the most popular free software for digital image analysis and recognition of plants are OpenCV [16], SimpleCV [17], BoofCV [18], PlantCV [19], PyCV [20], etc. Although multiple image-processing tools can be used for plant and crop analysis, a vast majority of them have some limitations. The first one, which highly reduces the possible implementations, is the requirement of providing a plain background for photographing plants [21–23]. This impediment almost excludes the possibility of application of such tools to indoor hydroponic stations, where, very often, a reflective foil is used. Such a foil is commonly used to get as much
Multiple studies elaborate on the problem of phenotyping using machine vision. However, this mostly refers to outdoor plantations, where the UAV (Unmanned Aerial Vehicle) usage is being considered and implemented [24–25]. Other methods that try to focus on the height assessments in indoor plantations report the usage of the RGBD (Red Green Blue Depth) sensors [26]. Despite very interesting results, there is a considerable lack of verification methods for these obtained values, which were estimated instead of measured. Due to this fact, there is no actual information about the accuracy of such methods, and the report about the results is questionable.
The following paper refers to the application of one of the most advanced free software libraries for image analysis, which is OpenCV [16]. It was assumed that the above library could be used for image-processing and thus optimization of crops’ growth rate in domestic, indoor hydroponic plantations. OpenCV contains API (Application Programming Interfaces) for different programming languages like C++, Python, Java, and Matlab [27].
The presented research uses the OpenCV library to analyze the commercially available hydroponic station by using an external camera. The above experimental setup was used for investigating pak choi cabbage (Brassica rapa L. subsp. Chinensis) growth. In the research, we focused on the problem of measuring the plants’ height in an environment that could cause issues such as reflections with the image processing. The main problem was distinguishing between the real plant and its counterpart or even counterparts, as there could be more than one reflection in the background.
The performed experiments’ primary motivation was to implement image recognition techniques to provide a simple parameter (plants’ height) that could be used for the optimization of the crops’ growth rate in hydroponic plantations. The elaborated algorithm introduces novelty by focusing on applications in which the environment’s background is not plain and where reflective foil
is being used. The test experiments were based on a commercially available hydroponic station and were allowed to gather referential data that could be used in the future experiments, which plan to focus on using machine learning algorithms in order to maximize the plants’ growth rate in hydroponic plantations.
The experimental setup consisted of the following hardware and software:
• commercial hydroponic system “Green Farm,”
• OpenCV library,
• single board computer Raspberry Pi version 3B running under Raspbian OS,
• OV5647 5Mpx camera.
A diagram of the applied system is presented in Figure 1.
In the research, the commercial Green Farm hydroponic station was used, which is presented in the figure 3. The device provided a simple set of sensors, such as a real-time clock, temperature sensor, and analog water level meter. These helped to provide essential and sustainable growth of the plants in a controlled hydroponic environment.
The decision to use the commercially available hydroponic system was chosen in order to implement automatic height measurements in a way that could be replicated in domestic applications. The Green Farm hydroponic station provided basic solutions for controlling the plants’ environment and allowed them to obtain sustainable growth. It was modified with a reflective foil to reproduce these conditions in enclosed stations.
The Raspberry Pi allows remote measurements without the need for a stationary PC, which is also a good choice in the case of domestic applications. It is one of the most popular SBC (Single-Board Computers), as it is both cheap and effective. The built-in port CSI (Camera Serial Interface) allows for easy implementation of external cameras. The Raspbian OS with implemented support for Python language allows for the use of OpenCV without any flaws.
The OV5657 camera is one of the most popular, cheap camera modules for Raspberry Pi and Arduino (ArduCam). The provided resolution of 5 Mpx and 72.4° camera angle provided good quality pictures for future processing.
The actual experimental setup is presented in Figure 2.
To be able to reproduce the setup in most indoor hydroponic systems, it was decided to set up an OV5647 camera, together with Raspberry Pi, outside of the system. This decision made the setup easily reproducible in most commercial hydroponic stations, simply by placing the camera outside of the setup and – if needed – opening the grow box without taking the plants outside of the station.
However, some more advanced hydroponic plantations use special grow boxes and reflective foil, such as mylar, to increase the amount of light delivered. It must be emphasized that putting the camera inside of the station’s tank would be more accurate. However, this would limit the number of cases in which the method could be applied and would require additional modifications to the already-made stations. The reason for choosing this solution was to find out whether OpenCV is a capable tool for collecting data for optimization of the plants’ growth rate, even in the case of commercially available hydroponic plantations that do not include machine learning technologies. To increase the challenges of plant detection and make the case more difficult and universal for indoor plantations, we added plain reflective foil on the investigated hydroponic station walls. Such a solution can simulate both mylar and cheap foil, which are often used in cheap and homemade stations. In order to
take pictures of the plants, small windows were cut from the foil.
For image processing, the OpenCV library and Python programming language were used. The advantages of the OpenCV library are advanced image processing methods and an available API for Python language, which combines the best qualities of OpenCV C++ API with Python.
The design and application of the algorithm consisted of the following stages:
• finding the bases of the plants, separating the areas containing plants from the rest of the image using the OpenCV library,
• finding the plants within the specified areas,
• obtaining their tops’ Y position and calculating the height.
The features of the OpenCV library that were used in the experiment, together with their functionality, are given in Table 1. Since OpenCV hardly relies on the NumPy library for providing compatibility and calculations, the functions used from the NumPy library for Python are given in the table 2.
The core of the system was a Raspberry Pi, with an OV5647 camera connected to it. The decision to use a Raspberry Pi was made due to the low cost and simplicity of programming, using the external camera, and later processing of the images. Due to the implemented solutions for remote access, by using SSH (Secure Shell), VPN (Virtual Private Network), and/ or VNC (Virtual Network Computing), remote control and access were possible.
The camera module that was used – OV5657 – provided a resolution of 5 Mpx and a 72.4° camera angle, which resulted in reasonable resolution and quality of the pictures for future processing. The pictures of the hydroponic station containing three plants were taken once a day. These pictures were then used for processing in order to extract the values of the plants’ height.
Function Description
array creating arrays
zeros creating arrays filled with zeros where returning the chosen element from the arrays depending on the condition
vstack stacking the arrays in sequence vertically
The elaborated image recognition algorithm consisted of the following steps:
• removing white and gray areas,
• removing the background with absdiff function,
• HSV (Hue Saturation Value) and RGB (Red Green Blue) filtering,
• separating images with the plants,
• finding contours,
• checking the size criteria,
• calculating the distance between the reference starting points and the plants’ tops.
The first issues we encountered were with black, white, and gray-colored areas. These are some of the common colors of tanks, pipes, housing, baskets for plants, etc. The easiest way to remove these areas from the pictures in OpenCV is with simple image-processing, such as removing any pixels between a specific threshold. In this case, the threshold was implemented in the RGB color space with the values of R, G, and B between 162 and 255 for each channel.
Function Description
Imread; Imwrite reading and saving the image files
The second way of dealing with the background was to use the differences between the captured images. In some cases, such a solution can easily detect the plants by using the picture of a plantation without plants as the reference image. However, in the investigated hydroponic station, the water level is not constant, and the plants’ elevation varies from one picture to another, which is crucial for height assessment. Thus, such a method is not always viable. However, it is a good practice to remove the background, even when the light conditions slightly vary. Therefore, it was decided that we would implement the differential method by comparing the picture taken on the first day of the experiment with those taken on successive days. The following steps are shown in Figure 4
bitwise_not;
bitwise_and bitwise operations on pictures
absdiff finding the differences between images
gaussianBlur blurring the masks
threshold creating a mask of specific values
Dilate morphological expansion of the masked areas
cvtColor converting color spaces (RGB, HSV, etc.)
convexHull Finding the convex hull of a 2D point
findContours finding the contours in the image
inRange checking if array elements are placed between the elements of two other arrays
The next step was to remove from the picture other objects/artifacts aside from plants. An excellent solution to this problem is to use color filtering. In the presented case, since there is a lot of noise coming from the background, station interior, and reflections, it was decided to use double-step filtering – both in the RGB and the HSV color spaces. It was noticed that RGB is great for filtering the wider ranges of color, but using the HSV color space helps to filter out narrow ranges of color. The lower RGB ranges for both the plants and bases detection were 190, 160, and 125, respectively, while for the top range, the value of 255 was used for each channel. In the elaborated case, the filtering had to be very strict due to extreme reflection conditions. This filtering resulted in the deconstruction of the plants’ shapes. However, to avoid detecting the reflections, this was a necessary step. To deal with the deconstruction of the plant images, the analysis of the image was split into multiple phases. In the first stage, the images of the plants were cropped basing on the contours of the bases of the plants, which in the early stages of the growing process are easier to distinguish. The values of HSV filters for this step were adjusted to detect the surrounding rockwool.
Multiple other approaches could also be implemented. When using small inserts made of foam, the
HSV filter rules could be adjusted, or this step could even be replaced with the detection of small plants in the early stages of growth. However, it was found that this way creates the most repetitiveness in the measurements.
For the case of HSV filtering, the cv2.dilate() function was used. After this step, all the aforementioned filters were combined using the cv2.bitwise_and() function on the source images, using the filtered images as the masks. The lower values of HSV filtering for the bases were set to 26, 91, and 118, respectively, while the top of the range was set to H = 33, S = 113, and V = 223. For the plants, the lower HSV threshold was set to 37, 65, 95, respectively, and 58, 250, 255 for the top values. This step is illustrated in Figure 5a.
For detecting the contours, both for the bases and plants, all the external contours on the picture were detected using the findContours() function. An array filled with zeros, called cont_status, was created afterward. Then, iterating over all of the contours, the distances between them were checked. If the criteria were met, the cont_dist variable for the contours was set to True, and the contours were connected together, creating multiple bigger, continuous shapes. The same was done with the sizes of evaluated contours and the cont_area variable. In this case, the distances and the sizes of the contours were investigated. After this process, all the connected contours were put into a separate cont_connected list.
As the last step for both of the stages, the cont_connected contours were checked again against another size criterion. This was done by iterating over all of the contours in the cont_connected list and checking if their size was bigger than the min_shown_area variable. All the contours that met the criteria were put into the cont_final list. For the iterations made for plant analysis, another cont_connected_copy list was made. The reason for that was an error that occurred when trying to process the same list with cv2.ContourArea() function multiple times with the OpenCV library version 4.4.0.44 that was used.
For most extreme cases, in which none of the final contours would meet the size criteria, a loop that decreased the value of the size threshold was implemented. This safety feature allowed us to get at least partial contours when the reflections did not allow for obtaining the full contours of the plants.
After obtaining the list of final contours, by subtracting the top Y-position value obtained, the height of the plants was calculated. This whole process is illustrated in Figure 5b.
As a result of the image processing, a series of continuous measurements of the three plants’ heights was estimated. The process took 15 days, resulting in 3 reference pictures of the plants and 42 separate images that were processed to estimate the plants’ heights each day.
The results obtained by the algorithm were calculated in millimeters using the plantations’ height as a reference. This is represented by the following formula:
shown in Figure 6. The values were fitted with an exponential function, the parameters of which are shown in Table 3. The confidence band of 99.7% was then calculated:
where hi is the i-plant’s height and n is the total number of plants grown.
where: href is a reference height object (the hydroponic station’s height) and hplant is the plant’s height. From all the measurements, the mean of plants’ height for each day was calculated using formula 2. The results of numerical assessments (hassessed) are
To check if the results obtained by the algorithm were valid, the mean calculated height values were compared to the results of the manual height assessment, and Er (Relative Mean Error) values were calculated for each measurement point. Manual measurements were conducted by measuring the pixel distance between the plants’ rockwool base and the top of the plants, and calculating the distance using the reference distance of a ruler and the hydroponic station’s height. Then, the results were confirmed by measuring the plants with a ruler. Er values were then approximated with a linear curve, and a 99.7% confidence band was calculated, which corresponds to the 3sigma standard. Both means, together with 99.7% confidence bands, were calculated according to the equation 3 and are shown in Figure 7.
(1)
(3) (3)
where Cb is the confidence band, ŷ is the actual measured value at xp, α is the confidence level, and tα/2 is the standard error of prediction for the value.
Additionally, the relative error Er is shown in Figure 8. Note that the plants’ mean height is very precisely calculated in the vast majority of the cases, showing that the elaborated algorithm allowed for achieving reliable measurements of the plants’ height.
Despite the reflections and uneven background, the measurements were performed with very satisfying results. Parts of the source pictures with the overlaid result images from the first, fifth, and fourteenth day of the experiment are shown respectively in Figures 9–11. In the first days, after growing their stems, the plants were observed to expand their leaves, which was the dominant process affecting the plants’ height up to the fifth day. From this point onward, the plants continued to grow their leaves. On the eighth day, plants spread out, and increased the number of, their leaves, which caused the observed height to drop on the ninth day. From this point, an uninterrupted height increase was observed.
It is worth noting that due to the heliotropism and the growth mechanisms of the plants, on different day cycles, the plants’ height varies. In the first stages of growth, the plant is looking for a light source, expanding its stem. Then, the process of expanding the leaves begins, which often cannot be held up by the thin stem. That is the reason why the B and C plants’ height in the Figure 10 is lower than on the first day (Figure 9). In addition, the plants’ height and the leaves’ shape may vary during the light cycle.
The obtained results prove the accuracy of the method, which was confirmed by the manual assessments. The maximum relative error was captured at 10%, which translated to 7 mm. The mean absolute error value was 2 mm.
With the exception of the measurement on the eighth day of the experiment, all the measurements fit into the 99.7% confidence bands of exponential approximation of the height vs. time function. This suggests that another mechanism was dominant in this growth phase, which was explained in previous paragraphs. However, the obtained results proved the usefulness of the method for automating plants’ height assessments. Additionally, it should be underlined that the plant’s height was assessed according to the evaluated rockwool bases’ positions
the measured top positions of
The elaborated algorithm will be used in the future research as a base for an autonomic hydroponic plantation, supported by machine learning techniques, that will fully control and optimize plants’ growth rate with light spectra adjustments, dosage of fertilizers, water, pH control, etc.
The goal of the research was to design a universal tool that would allow for automatic height assessments of the plants in hydroponic plantations.
According to the brief state-of-the-art, it was shown that most of the tools available for computer-vision-based automatic height assessments are problematic for indoor hydroponic plantations, where multiple plants are being grown, especially when a reflective foil is used.
Based on the elaborated hardware and software setup, an algorithm for OpenCV Python’s library was proposed and explained. This algorithm was then used to process images of the Green Farm hydroponic indoor plantation, which was modified by using a reflective foil, where three pak choi cabbages were being grown. Based on 15 source images that were captured over 14 days, 42 result images, together with the numerically assessed plants’ heights, were prepared by the algorithm.
The results allowed for calculations of the mean plants’ height values, which were compared to the values obtained with manual measurements. For both numerically assessed and manually measured values, an approximation was made, together with 3sigma confidence bands. These values were then compared, and the relative error between them was calculated. The results have shown that the elaborated algorithm
has very good precision and that it can be used as a viable tool for automatic height assessments in indoor hydroponic plantations with multiple plants, even when reflective foil is used.
The obtained results will be used as a base for future experiments that will take place in order to optimize the plants’ growth rate in a fully-automatic, machine-learning-driven hydroponic system supported by a number of detectors, sensors, and actuators.
Sławomir Krzysztof Pietrzykowski* – Faculty of Microsystem Electronics and Photonics, Wroclaw University of Science and Technology, Janiszewskiego Street 11/17, 50-372 Wroclaw, Poland, e-mail: slawomir.pietrzykowski@pwr.edu.pl.
Artur Wymysłowski – Faculty of Microsystem Electronics and Photonics, Wroclaw University of Science and Technology, Janiszewskiego Street 11/17, 50-372 Wroclaw, Poland, e-mail: artur.wymyslowski@pwr.edu.pl.
*Corresponding author
[1] United Nations, “2030 Agenda for Sustainable Development”, available from: https://www. un.org/ga/search/view_doc.asp?symbol=A/ RES/70/1&Lang=E, accessed: 2022-06-21
[2] Food and Agriculture Organization of the United Nations, “Farming in urban areas can boost food security”, 2005. Available from: https:// reliefweb.int/report/world/farming-urban-areas-can-boost-food-security
[3] Barbosa, Guilherme Lages et al. “Comparison of Land, Water, and Energy Requirements of Lettuce Grown Using Hydroponic vs. Conventional Agricultural Methods”, International journal of environmental research and public health vol. 12, no. 6, 2015, 6879–6891. .DOI:10.3390/ ijerph120606879
[4] Stefan P. et al. “A comparative cost-effectiveness analysis in different tested aquaponic systems”, Agriculture and Agricultural Science Procedia, vol. 10, 2016, 555–565. DOI: 10.1016/j.aaspro.2016.09.034.
[5] Margaret S. et al. “Building sustainable societies trough vertical soilless farming: A cost-effectiveness analysis on a small-scale non-greenhouse hydroponic system”, Sustainable Cities and Society, vol. 83, 2022, 101882. DOI: 10.1016/ j.scs.2022.103923.
[6] A. Malik et al. “A review on the science of growing crops without soil (soilless culture) – a nov-
el alternative for growing crops”, International Journal of Agriculture and Crop Sciences, vol. 7, 2014, 833–842.
[7] X. E. Pantazi et al. “Detection of biotic and abiotic stresses in crops by using hierarchical self-organizing classifiers”, Precision agriculture, vol. 18 no. 3, 2017, 383–393. DOI:10.1007/s11119-0179507-8.
[8] S. Amatya et al. “Detection of cherry tree branches with full foliage in planar architecture for automated sweet-cherry harvesting”, Biosystems Engineering, vol. 146, 2016, 3–15. DOI: 10.1016/j.biosystemseng.2015.10.003.
[9] M. Ebrahimi et al. “Vision-based pest detection based on SVM classification method”, Computers and Electronics in Agriculture vol. 137, 2017, 52–58 DOI: 10.1016/j.compag.2017.03.016.
[10] X.-E. Pantazi et al. “Active learning system for weed species recognition based on hyperspectral sensing”, Biosystems Engineering, vol. 146, 2016, 193–202. DOI: 10.1016/j.biosystemseng.2016.01.014.
[11] G. L. Grinblat et al. “Deep learning for plant identification using vein morphological patterns”, Computers and Electronics in Agriculture, vol. 127, 2016, 418–424. DOI: 10.1016/j.compag.2016.07.003.
[12] F. M. Westmoreland et al. “Cannabis lighting: Decreasing blue photon fraction increases yield but efficacy is more important for cost effective production of cannabinoids”, PLOS ONE, vol. 16, no. 3, 2021. DOI: 10.1371/journal. pone.0248988
[13] V. Rodriguez-Morrison, “Cannabis Yield, Potency, and Leaf Photosynthesis Respond Differently to Increasing Light Levels in an Indoor Environment”, Frontiers in Plant Science, vol. 12, 2021. DOI: 10.3389/fpls.2021.646020
[14] M. W. Jenkins, “Cannabis sativa L. Response to Narrow Bandwidth UV and the Combination of Blue and Red Light during the Final Stages of Flowering on Leaf Level Gas-Exchange Parameters, Secondary Metabolite Production, and Yield”, Agricultural Sciences vol. 12, no. 12, 2021, 1414–1432. DOI: 10.4236/as.2021.1212090
[15] M. Moher et al. “High Light Intensities Can Be Used to Grow Healthy and Robust Cannabis Plants During the Vegetative Stage of Indoor Production”, Preprints (2021). DOI: 0.20944/ preprints202104.0417.v1
[16] I. Culjak et al. “A brief introduction to OpenCV”, 2012 Proceedings of the 35th International Convention MIPRO, 2012, 1725–1730.
[17] SimpleCV, http://www.simplecv.org, accessed: 2021-02-07.
[18] BoofCV, http://boofcv.org/, accessed: 2021-0207.
[19] M. Gehan (Dong) et al. “PlantCV v2.0: Image analysis software for high-throughput plant phenotyping”. PeerJ, vol. 5, 2017. DOI: 10.7287/peerj.preprints.3225.
[20] M.-T. Pham, T.-J. Cham, “Online learning asymmetric boosted classifiers for object detection”, 2007 IEEE Conference on Computer Vision and Pattern, Minneapolis, USA, 2007, 1–8. DOI: 10.1109/CVPR.2007.383083.
[21] A. K. Hase et al. “Detection, categorization and suggestion to cure infected plants of tomato and grapes by using OpenCV framework for Android environment”, 2017 2nd International Conference for Convergence in Technology (I2CT), 2017, 956–959. DOI: 10.1109/ I2CT.2017.8226270.
[22] I. Suryawibawa et al. “Herbs recognition based on android using OpenCV, International Journal of Image, Graphics and Signal Processing”, vol. 7, 2015, 1–7. DOI: 10.5815/ijigsp.2015.02.01.
[23] Y. Chen et al. “Research on pest image processing method based on android thermal infrared lens”, IFAC-PapersOnLine, vol. 51, no. 17, 2018, 173–178.DOI: 10.1016/j.ifacol.2018.08.083.
[24] P. Hu et al. “Estimation of plant height using a high throughput phenotyping platform based on unmanned aerial vehicle and self-calibration: Example for sorghum breeding”, European Journal of Agronomy, vol. 95, 2018, 24–32. DOI: 10.1016/ j.eja.2018.02.004
[25] MA Hassan et al. “Accuracy assessment of plant height using an unmanned aerial vehicle for quantitative genomic analysis in bread wheat”, Plant Methods vol. 15, 2019, 15–37. DOI: 10.1186/s13007-019-0419-7
[26] B. Franchetti et al. “Vision Based Modeling of Plants Phenotyping in Vertical Farming under Artificial Lighting”, Sensors, vol. 19, 2019. DOI: 10.3390/s19204378
[27] OpenCV – about, https://opencv.org/about/, accessed: 2021-02-07.
Submitted: 9th February 2022; accepted 3rd May 2022
Boppuru Rudra Prathap, Kukatlapalli Pradeep Kumar, Javid Hussain, Cherukuri Ravindranath ChowdaryDOI: 10.14313/JAMRIS/2-2022/17
Abstract
The number of automobiles on the road grows in lockstep with the advancement of vehicle manufacturing. Road accidents appear to be on the rise, owing to this growing proliferation of vehicles. Accidents frequently occur in our daily lives, and are the top ten causes of mortality from injuries globally. It is now an important component of the worldwide public health burden. Every year, an estimated 1.2 million people are killed in car accidents. Driver drowsiness and weariness are major contributors to traffic accidents this study relies on computer software and photographs, as well as a Convolutional Neural Network (CNN), to assess whether a motorist is tired. The Driver Drowsiness System is built on the MultiLayer Feed-Forward Network concept CNN was created using around 7,000 photos of eyes in both sleepiness and non-drowsiness phases with various face layouts. These photos were divided into two datasets: training (80% of the images) and testing (20% of the images). For training purposes, the pictures in the training dataset are fed into the network. To decrease information loss as much as feasible, backpropagation techniques and optimizers are applied. We developed an algorithm to calculate ROI as well as track and evaluate motor and visual impacts.
Keywords: Artificial Intelligence, Machine Learning, Drowsiness Detection, Image Processing, Convolutional Neural Networks, AI Visuals
Road accidents are the top cause of death from injuries worldwide. They now form an essential part of the global burden of illness. Every year, 1.2 million people are killed in accidents, and an estimated 50 million are injured. Developing nations bear the burden, reporting 85% of all yearly deaths and 90% of life-threatening injuries because of road damage. Road injuries mainly affect men (73% of total) between the ages of 15 and 44; this burden creates significant economic imbalance.
The number of automobiles on the road grows with the advancement of car manufacturing, and the number of incidents looks to be gradually increasing, owing to the rapid proliferation of automobiles [1]. People get hurt in our everyday lives. The Driving Discovery System is built using concepts based on non-disruptive
machine learning. The system uses a small security camera that monitors the driver’s face and eyes. A driver’s warning [2] is given when fatigue is detected. This report explains how to find whether the eyes are open or closed. The algorithm developed differs from other papers. This system works using the application details gained from the grayscale image to find the edges of the eyes. The magnitude of changes in the position of the eyes determines the opening or closing of the eyes. Blindfolds are used to cover a large distance. When the driver’s eyes remain closed for five consecutive frames, the system concludes the driver has fallen asleep and sounds an alert to wake them up. Working in typical illuminated conditions, the device can also identify when the eyes are not focused.
Personal recognition procedures use a wide range of stimulants, obtained from many senses, if not all, including sound, touch, etc. For the most part, conditions and context information are used, as an example, to play a vital role in facial recognition in how to go and find. It is useless to try and try to improve the system with current technology, which will copy the unique face’s ability to identify people. However, as a brain, it has its limits in the number of people it can “remember” accurately. The primary benefit of a computer program is its ability to process large numbers of facial images. For the most part, photo applications have only present in the form of one or more views of two-dimensional data, so that input to a face recognition algorithm is only visual. For this, books updated in this field are limited.
Drowsiness in humans [3] is defined as when they are almost asleep or in a dormant state. It is a sign that they cannot stay awake or that they need to sleep [4]. Fatigue is another cause of drowsiness that is frequently and is defined as a weary physical condition, resulting in a state of mental and physical tiredness. Both drowsiness and fatigue happen as a result of overall physical tiredness. The level of weariness may also be a metric for attentiveness or observance. The condition of alertness is defined as the absence of sleepiness, and the state of being observant is one of being keenly focused on something [5].
A detailed review of relevant literature in psychophysics and neuroscience follows. We describe the applicable requirements for the face recognition system
[6] Facial recognition has been previously regarded as a digital process that differs from other awareness activities. Proof of dedicated presence in the face-toface program comes from several sources [7]:
1. Faces are easier for humans to remember than anything else when presented directly standing.
2. Prosopagnosia patients may not be able to remember a familiar face, but often they might not have deep agnosia. They use voice, hair color, dress code, etc., to remember people. It should be kept in mind that patients with prosopagnosia can see whether the given object has a face or not, but have difficulty remembering the face.
The differences in facial expressions and object detection can be summed up according to strong notions: (1) the effects of composition, (2) technology, (3) the differences discussed, (4) comparisons of polarity and lighting direction, (5) metric diversity, (6) Depth of field (related in the case of variability in machine recognition systems), and (7) rotation on the plane / shifted surface real-time eye, gaze, and face pose tracking system for driver attentiveness monitoring proposed by Qiang and Yang [8] and Chauhan et al. [9] introduced a face recognition system which would use IoT, the cloud, and deep learning. The authors used a cloud environment, which is a tree-based profound foundation for programmed facial recognition. Pillai et al. [10] proposed a model on frame diminution techniques for speed detection of vehicles. With a stationary camera, the author used the VDFT background subtraction approach to estimate vehicle speed. This is a recursive technique for calculating the rate of movement that involves obtaining the moving object’s area moving property and comparing it to the calibrated speed. The Support Vector Machine (SVM) [11] is used to improve eye recognition. In Principal Component Analysis (PCA) and Logical Analysis of Data (LAD), statistical characteristics like sparseness and kurtosis are employed to identify eye state. Crime analysis and prediction using data from road accidents has been done by Prathap and Ramesha et al. [12].
Fatigue is an important issue to consider while monitoring traffic accidents, as studied by Hamada et al. [13], Barr et al. [14], Eriksson and Papanikolopoulos [15], Eskandarian and Mortazavi [16], and Grace et al. [17]. Around 20% of all traffic accidents are caused by driver weariness while on the road. When driving while tired, the driver’s performance deteriorates, resulting in road accidents.
Several vehicle accidents happen due to driver inattention [18]. Detecting driver drowsiness and warning the motorist before an accident happens is unquestionably advantageous and could save lives. The most significant cause of road accidents is driver weariness. Detecting and preventing driver weariness is crucial to avoiding accidents and can be accomplished with new technology. Shahverdy et al. [19] presented a vision-based Intelligent Transportation Systems technique for detecting tired drivers and distractions by monitoring the system. A unique eye-detecting algorithm is utilized in this system, which integrates adaptive convolutional networks, dynamic boosting,
and blob recognition with eye verification. It cuts down on processing time considerably. According to Valsan, Mathai, and Babu [20], the central feature of Driver Sleepiness Detection is obtaining an image of the driver’s face, analyzing the image obtained, and estimating the drowsiness level, SVM (Support Vector Machine) is a sort of machine learning technique that employs. To achieve this, we need proper hardware. The research looks at the Raspberry Pi 3 Model B as a primary computer. The Raspberry Pi camera was chosen as the visual device. It is simple hardware to work with when used in tandem with well-defined software. As a result, software plays a vital role in the machine. The researchers have used OpenCV as an image and video library in their study.
Baek et al. [21] proposed an algorithm for detecting faces using a classifier known as Adaboost MCT, and showed how facial landmark detection works using an LBF regressor. Also, the running is swift and accurate on the embedded camera, as shown in the flow chart diagram of the drowsiness detection algorithm. The image is received from the camera after the video query. Next, it is preprocessed to remove the noise using conducted surveys to comprehend the needs and prerequisites of the general population. To do so, they went through different sites and applications and looked for the fundamental data. They made an audit that helped us get new thoughts and make different arrangements for our task based on these data. We determined demand for such an application and thought we had made some headway in this area.
Based on the above literature, the driver drowsiness detection system is based on eye closedness detection. The system uses the mathematical formula for finding the closedness of the eye. The cascades are used to detect the face and eyes in the represented video. The eye closedness is noticed by drawing the landmarks over the edges and peaks of the eye. These landmarks or points represent the condition of the eye and the driver’s state. The total combination of these points is defined as a Region of Interest (ROI). Once these edges and the peak points align horizontally, the algorithm predicts the result as drowsiness by giving an alert notification. Many of these are entirely embedded systems using Raspberry Pi and some other modules.
The driver drowsiness system works over the MultiLayer Feed Forward Network concept, especially on Convolutional Neural Networks (CNN). CNN is developed with the help of around 7000 images of eyes with different facial architecture in both drowsy and non-drowsy states. These images are split into training (80% of images) and testing (20% of images) datasets. The images under the training dataset are given as the network’s input for training purposes.
A backpropagation algorithm and optimizer are used, which reduce data loss as much as possible. Figure 1 shows the system architecture of the driver drowsiness system. This facial preprocessing identifies the images from the camera and gives the input to
network model for classifying the state of the driver, which is using a CNN classifier.
connected by arrows. In Figure 2, new images are given as an input to adjust brightness and contrast, where the face will be detected first. Based on the research, the main focus in detecting drowsiness is identifying the eye movement in the face detection. Based on the eye movement, it will give an alarm.
A system architecture shows the structural and behavioral view of the whole system. An architecture design is a formal design representing a system in such a way that it clearly shows the structures and behaviors of the system. Figure 1 shows that the system architecture contains system components and the sub-systems developed, working together to implement the overall system functioning. The architecture of this system consists of 5 parts. They are listed as (1) Driver, (2) Camera, (3) Facial Processing, (4) Network Model, and (5) Alert System. The driver interacts with the camera to capture the video. This video captured by the camera is given as input for the facial processing. It detects the Region of Interest (ROI) and transfers it to the network model. The network model calculates the driver’s drowsiness level with the help of output given by the facial processing step. Based on the result produced by the network model, an alert system notifies the driver if they are drowsy.
Figure 2 depicts the flow of events in a driver drowsiness system. In addition to representing a workflow, a flowchart may be a diagrammatic depiction of an algorithm, such as a step-by-step approach for accomplishing a job. The flowchart illustrates several kinds of boxes and the order in which they are
Figure 3 shows the architecture’s data flow diagram (DFD) (usually an information system). Here we also get the info about various aspects of an entity, like outputs and inputs of the process. A data-flow diagram does not have any control flow; there are no decision rules or loops in it.
The whole system is comprised of various smaller modules or components or working groups which are described as follows: (1) Video Capturing and Image Processing, (2) Video Capturing, and (3) Processing video. A detailed explanation of each module is as follows.
The video captured by the camera is to be refined, and faces are to be detected from them. Once seen, these faces are refined to provide information about the eyes. These eyes are to be transferred to network model to get classified. Several steps are to be followed in video capturing and image processing.
The video is captured with the help of the camera of a laptop. These videos will be sent to the preprocessing stage to find the region of interest or ROI.
Once the camera begins to capture video, the video is translated into frames, which are used as input for the processing software. This processing occurs in the background so that users cannot see the processing steps. From Figure 4, RGB frames are converted to grayscale (which comprises the range between black to white on the scale of 255 to 0) for easier detection of the facial landmarks.
The ROI from the frames captured is sent as input to the network for classification purposes. The network that mainly works over extensive dimensional data such as images is known as a convolutional neural network or CNN, and is built over around 7000 images of different facial architectures. There are several steps involved in the construction of this CNN.
The ROI transferred from module-1 (Video Capturing and Image Processing) is of high dimensionality. To reduce dimensionality, this data is transformed to grayscale, normalized in the range of 0 to 1. This preprocessed data is sent into the CNN model. The input is scaled to 32x32 dimensions.
Figure 5 shows the highlights to detect the region of interest (ROI). Once the ROI is detected, it is sent to the model for classification. Based on the results gathered from the model and the score value (effect of drowsiness), the system alerts the user. Once the conversion is completed, face and eye cascades are considered to detect face and eyes over frames. The edges are calculated using the Edge Gradient function given above. Using this function, the edges of the face are determined. Once the boundaries are determined, the face is detected, and using the cascades, the ROI (eyes region) is detected and transferred to the neural network for classification.
Figure 6 shows the CNN Model. The CNN contains 3 layers. These are (1) input layer, (2) hidden layer and (3) output layer. The input layer includes 32 input nodes in total. Input is passed to these nodes. Every node of this layer is connected to each node of hidden layers. The data is transferred from the input node to the hidden node. The processed data from the hidden node are transferred to the output nodes. The output of the input nodes is the same as the input of the input nodes, but in the hidden nodes, the input is the summation of the product of the previous node taking into consideration the weight of the connection. Along with that, a bias is added to the value to compensate for the loss of transmission. The output of the hidden layers is charged using the activation function. This output is the input for the next or succeeding hidden layers or for the output layer. There are different activation functions in use. We have used specific tasks for this system.
The primary process is optimizing the neural network that is to be done for the accuracy maintenance of the network. The optimization algorithm improves the network’s accuracy and decreases the network’s error loss. This optimization step is responsible for the backpropagation process. Different optimizing algorithms like Gradient Descent, SGD, Adam, etc. We have used the Adam optimizer, which provides the most accurate results for its users. Neural networks for image processing are the most complex and large-build algorithm. To recover this situation dropout function is used. The dropout function is used to remove the not-used or unwanted nodes from the neural network. This function reduces the execution time in the training process by temporarily disabling the nodes. To minimize the processing step-over, the images’ max pool step is performed. Max pool is the process of compressing the image without losing the contents. This process is done by reducing the size of the matrix by replacing the considerable matrix with a smaller one. This reduces the size of the image through which the processing of the image is reduced along with the reduction of the execution time.
Once the model is built, and optimization is done, the main step that needs to be done is training and testing. The training dataset is given as input for the network for training purposes, and the network is trained here. In the process of training, the network model dropout step is performed with a constraint. The trained network obtained is tested with the testing dataset to determine the accuracy of the network. The accuracy of the model is increased at every epoch with a reduction in data loss.
Based on the output received by the model and the core value of the state, the model alerts the user if the state is drowsiness.
The proposed research experiment is initially carried out by taking an input of a video stream with the camera. As the video stream is given as input, each frame is separated by initiating two threads running parallel. Figure 7 shows the working model of the proposed work. The input video streams will be converted into frames. The frames will be divided into thread1 and thread2, as explained in 3.2.1 and 3.2.2. Figure 8 explains the inception layer with filter concentration combined with the CNN algorithm. The proposed model works with a combination of the CNN model and Euclidian distance model to get an accurate result for the drowsiness identification of the driver.
In thread 1, the separated frame is given to the Haar cascade detector for detection and segmentation. Once the detection is done, the cropped image is sent to the Inception V3 model. The Inception V3 model is trained with 7000 images of eyes closed and open and has a training accuracy of 95%. T Inception V3 model classifies the
cropped image with real-time prediction for eyes closed or open.
In thread 2, the separated frame is given to a pertained eye key point detector. The key point detector maps the face with a key point and allocates variables to each of the eye key points. With the assigned key points to the upper and lower edges of the eyes, we calculate the Euclidian distance formula to evaluate the distance between every two points. The eye key point is given by using Euclidian distance.
In this research, a module has been introduced to minimize the number of accidents due to driver fatigue, which is why this method significantly focuses on increased safety. This research also deals with automatically detecting driver drowsiness using AI and visuals associated with CNN. The CNN method, combined with Euclidian distance, helps in identifying driver drowsiness with more accuracy related to the proposed system. This works over the concept of Multi-Layer Feed-Forward Network, especially on Convolutional Neural Networks (CNN) and Euclidian distance, giving more accurate results.
In mathematics, Euclidian distance is the length of a line segment between two particular points. Cartesian coordinates of the points help in calculating the distance using a simple Pythagorean theorem. Higher dimension calculations in computing and optimization use the square of the Euclidian distance.
The basic formula is as follows:
d = √[(x2 – x1)2 + (y 2 – y 1)2] (1)
where (x1,y1) is the coordinate associated with the first point, (x2,y2) is the coordinate associated with the second point, and ‘d’ is the Euclidian distance.
Figure 9 gives a simple understanding of distance calculation between two points using the Pythagorean theorem, leading to the formation of the equation (1).
This simple concept of the distance-making formula is used in our work in combination with the CNN. This provides better accuracy in forming the results in the context of driver drowsiness. The same is applied in the software code of the AI-based application that has been developed.
The sole objective of this system is to classify and notify the user of their drowsiness state. The working of our algorithm step-by-step process is as follows:
Step 1. Photo is taken from the camera as input. For accessing the webcam, we create an endless loop that holds each frame. We use CV2.capturevideo() to access the camera to capture images. Then we have CAP.READ(), which will set the captions, read the images frame by frame, and save them.
Step 2. Creating the region of interest (ROI): Our detection algorithm is based on OpenCV, so we need to convert the obtained color image to grayscale. We do not require color details to get the items. We use a Haar classifier to find the face. This sets our face partition = CV2. Cascade-Classifier (‘here we add the path to our XML file’). After that, we made the discovery with the help of faces = face. Multiscaledetect(gray), which returns the coordinates (x,y), along with the height and width of the object. Now we can trim the face and create the border-box for each one.
Step 3. Capture the eyes within the ROI and feed it to the divider: The same facial recognition process is made to capture the eyes. Initially, we place the cascade separator with the eye on one and then the other, and then find the eye using left eye = eye:multiscaledetect(gray). Now we are left with the extraction of optical data from the whole image. This can be done by removing the border of the eye and then removing the image from the frame.
Step 4. The separator will classify opened and closed eyes. We use CNN classification to predict eye conditions and give our image to the model because the model requires the right proportions to start with.
First, we convert the color image to gray using reye = cv2:cvtColor(reye;cv2:COLORBGR2GRAY), Step 5. Count Points for Checking Consciousness: Points are basically the amount we are going to use
to find out how long a person closes their eyes. When both the eyes are in closed state, we will continue to raise points and reduce points when the eyes open. We draw the effect on the screen using the cv2.putText () function to show the real-time statistics.
The following figures provide an explanation of the training the network and classifying the results.
Figure 7ab shows the GUI launching the camera. Clicking the “Okay” button launches the camera and the classification process. Once the camera is launched, the classification process is started, and it is shown at the bottom-left corner of the camera interface. “Open” and “closed” are the two classified outputs of the system. Based on the time of the “Closed” output, the alert system interacts with the driver. Here we considered 5 cycles of epoch as hyper parameters, as shown in Figure 7b.
Figure 8a shows the drivers’ eyes as “closed” and the scores are given: 167, 154, and 154. Hence, the alarm has to be invoked. Figure 8b the driver’s eyes and face is not being recognized at all, so the eyes are considered to be “closed” and the scores are given as 302, 207, and 249. Hence, the alarm has to be invoked. The eyes in Figure 8c are slightly closed so it is detected as “closed”, and the scores are given as 40, 83, and 325 (since a complete eye is not recognized). In this case, the alarm system will turn on.
Figure 9a shows the driver’s eyes completely shut, so it is detected as “closed.” The score is increased to 53, and the alarm is triggered. Figure 9b shows the driver is not looking towards the road or camera at all, so it is detected as “closed” and the score increases to 116, and we have another alarm notification.
Figure 10 shows a simulation of driver drowsiness using Convolutional Neural Networks combined with Euclidian distances using the algorithm explained in Figures 7–9. Figure 10a shows an active driver simulation, and 10e shows a complete drowsiness alert.
Drowsy driving is becoming more of a concern throughout the world. It poses a risk and a serious dilemma for both the individual and the nation. According to a study [22], drowsy driving is responsible for 20% of all crashes. As a result, developing a system for detecting driver drowsiness is not only justified but also essential. The first proposed system is used to find the fatigue of the driver based on eye detection. The videos are captured through a camera or web camera for offline detection of the driver, and also through an Android mobile phone used for real-time detection of the fatigue of the driver. In every situation, a new invention or innovation has both positive and negative sides, but we as humans need to improve the world towards using the positive side and ignoring the negative side of the outcome. With our drowsiness models, we were able to reach a prediction accuracy of 97.65% by considering 1232 images belonging to 2 classes (Open & Closed). Combining the eye key point calculation and Inception V3
prediction, the evaluation score is calculated. With the help of the score, the drowsiness is measured and the driver is alerted with a buzzing sound. However, working with Euclidian distance in connection with the Convolutional Neural Network helped us understand how to obtain more accurate results for the proposed work in this specific context. This aids better safety and offers a newer dimension in mitigating the number of accidents.
In the near future, there is a wide potential scope for AI, and the key for humans will be to maintain and balance its rise. Some people can still believe that developing AI can lead to the destruction of the human race, but it is far from our sight and time, and also near impossible. As part of the future extension of research, to reduce hardware expenses, we can implement this project in mobile app form. We could also add some additional features like speed control in case of drowsiness along with an alarm system.
Boppuru Rudra Prathap*- Department of Computer Science and Engineering, CHRIST (Deemed to be University), Bangalore-560074, Karnataka, India, E-Mail: boppuru.prathap@christuniversity.in.
Kukatlapalli Pradeep Kumar- Department of Computer Science and Engineering, CHRIST (Deemed to be University), Bangalore-560074, Karnataka, India.
Javid Hussain- Department of Computer Science and Engineering, CHRIST (Deemed to be University), Bangalore-560074, Karnataka, India.
Cherukuri Ravindranath Chowdary- Department of Computer Science and Engineering, CHRIST (Deemed to be University), Bangalore-560074, Karnataka, India E-Mail: boppuru.prathap@christuniversity.in.
[1] P. Inthanon and S. Mungsing, “Detection of Drowsiness from Facial Images in Real-Time Video Media using Nvidia Jetson Nano,” 2020 17th International Conf. Electrical Engineering/Electronics, Computer, Telecommunications and Information Technology (ECTI-CON), 2020, pp. 246–249, doi: 10.1109/ECTI-CON49241.2020.9158235.
[2] A. Dasgupta, D. Rahman and A. Routray, “A Smartphone-Based Drowsiness Detection and Warning System for Automotive Drivers,” IEEE Transactions on Intelligent Transportation Systems, vol. 20, no. 11, 2019, pp. 4045–4054, doi: 10.1109/TITS.2018.2879609.
[3] M. Ramzan et al., “A Survey on State-of-the-Art Drowsiness Detection Techniques,” IEEE Access, vol. 7, 2019 pp. 61904–61919, doi: 10.1109/ACCESS.2019.2914373.
[4] K.G. Seifert, T. Jan and T. Karnahl, “Don’t Sleep and Drive – VW’s Fatigue Detection Technology,” Proc. 19th International Technical Conf. Enhanced Safety of Vehicles (ESV), 2005.
[5] R.J. Sternberg, Cognitive Psychology, Cengage Learning Press, 2012.
[6] I. Biederman and P. Kalocsai, “Neural and Psychophysical Analysis of Object and Face Recognition.” Face Recognition, Springer, 1998, pp. 3–25.
[7] A. Ellis and R.M. Grieger, Handbook of Rational-Emotive Therapy, Vol. 2, Springer, 1986.
[8] J. Qiang and X. Yang, “Real-time eye, gaze, and face pose tracking for monitoring driver vigilance,” International Journal of Real-Time Imaging, vol. 8, no. 5, 2002, pp. 357–377, doi: 10.1006/rtim.2002.0279.
[9] D. Chauhan et al. “An effective face recognition system based on Cloud-based IoT with a deep learning model.” Microprocessors and Microsystems, vol. 81, 2021, pp. 103726.
[10] V.J. Pillai et al., “Fixed Angle Video Frame Diminution Technique for Vehicle Speed Detection,” Annals of the Romanian Society for Cell Biology, vol. 25, no. 2, 2021, pp. 3204–3210.
[11] S. Hu and G. Zheng, “Driver drowsiness detection with eyelid related parameters by Support Vector Machine,” Expert Systems with Applications, vol. 36, no. 4, 2009, pp. 7651–7658, doi: 10.1016/ j.eswa.2008.09.030.
[12] B.R. Prathap and K. Ramesha. “Spatio-Temporal Crime Analysis Using KDE and ARIMA Models in the Indian Context.” International Journal of Digital Crime and Forensics (IJDCF), vol. 12, no. 4, 2020, pp. 1–19, doi: 10.4018/IJDCF.2020100101.
[13] T. Hamada et al., “Detecting method for Driver’s drowsiness applicable to Individual Features,” IEEE Proc. Intelligent Transportation Systems, vol. 2, 2003, pp. 1405–1410, doi: 10.1109/ ITSC.2003.1252715.
[14] L. Barr et al., “A review and evaluation of emerging driver fatigue detection, measures and technologies,” A Report of U.S. Department of Transportation, 2009.
[15] M. Eriksson and N.P. Papanikolopoulos, “Eyetracking for detection of driver fatigue,” IEEE Proc. Intelligent Transport Systems, 1999, pp. 314–318, doi: 10.1109/ITSC.1997.660494.
[16] A. Eskandarian and A. Mortazavi, “Evaluation of a smart algorithm for commercial vehicle driver drowsiness detection,” IEEE Intelligent Vehi-
cles Symposium (IV’07), Istanbul, Turkey, 2007, pp. 553–559, doi: 10.1109/IVS.2007.4290173.
[17] R. Grace et al., “A Drowsy Driver Detection System for Heavy Vehicles,” Digital Avionics Systems Conference, 1998. Proceedings, 17th DASC. The AIAA/IEEE/SAE, vol. 2, 1998, pp. 50–70, doi: 10.1109/DASC.1998.739878.
[18] M.T. De Mello et al., “Sleep disorders as a Cause of Motor Vehicle Collisions.” International Journal of Preventive Medicine, vol. 4, no. 3, 2003, pp. 246–257.
[19] M. Shahverdy et al., “Driver Behavior Detection and Classification Using Deep Convolutional Neural Networks,” Expert Systems with Applications, vol. 149, 2020, pp. 113240, doi.org/10.1016/ j.eswa.2020.113240.
[20] V.A. Valsan, P.P. Mathai and I. Babu, “Monitoring Driver’s Drowsiness Status at Night Based on Computer Vision,” 2021 International Conference on Computing, Communication, and Intelligent Systems (ICCCIS), 989-993 (2021). doi: 10.1109/ICCCIS51004.2021.9397180.
[21] J.W. Baek et al., “Real-time Drowsiness Detection Algorithm for Driver State Monitoring Systems,” 2018 Tenth International Conf. Ubiquitous and Future Networks (ICUFN), 2018, pp. 73–75, doi: 10.1109/ICUFN.2018.8436988.
[22] Rivelli, Elizabeth. “Drowsy Driving 2021 Facts and Statistics | Bankrate.” Drowsy Driving 2021 Facts & Statistics | Bankrate, www.bankrate. com/insurance/car/drowsy-driving-statistics. Accessed 27 Jan. 2023.
Submitted:14th February2022;accepted:23th May2022
RudyHanindito,AdhaImamCahyadi,PraptoNugrohoDOI:10.14313/JAMRIS/2‐2022/18
Abstract:
Formationcontrolisanimportantpartofanysystem thatutilizesmultiplemobileagentstoachieveitsparti‐culargoals.Oneofthoseapplicationsisthemobilewi‐relessnetworksensor.Thisfieldhasbecomeincreasingly morepopularinrecenttimesduetotheadvancementof technology,especiallyinthefieldsofminiaturizationand telecommunications.Themainproblemofthisresearch istherelativelyuntestedsensingcapabilityofamobile wirelesssensornetworkinanoperatingareathathasdis‐tributedand/ormultiplelocationsofhighinterest.The purposeofthisresearchistodiscoverthecompatibility ofamultiple‐agentcoveragecontrolsystemwithseveral examplesofinterestfunctionsthathavemultipleand/or distributedpointsofglobalmaximumvalueinorderto exploremorethoroughlytheperformanceofagivensy‐steminavaryingenvironments.
Keywords: MobileWirelessSensorNetwork,Coverage Control,InterestFunction
Amobilewirelesssensornetworkisasystemthat employsmultiplemobilesensorstoachieveaparticu‑ largoal.Inorderforamobilewirelesssensornetwork systemtobefunctional,itmusthaveatleasttwopa‑ rameters:asensingfunctionandaninterestfunction. Asensingfunctionisarepresentationofhowwellan agentdoesitsjobs,whileaninterestfunctionisare‑ presentationofhowimportantsensingthelocation is.Thecombinationofboththesensingandinterest functioniscalledtheobjectivefunction,anditisused tomeasurethesensingperformanceofthemobilewi‑ relesssensornetwork.Oneadvantageofusingmobile wirelesssensornetworkisthattheyaremobile,which meansthatthesystemscanmovefromplacetoplace. Theyutilizemultipleagents,soifoneoftheagentsis down,thewholesystemdoesnotentirelyfail.Mobile wirelesssensornetworkshavemanypotentialappli‑ cations,e.g.tooperateinplacestoodangerousforhu‑ manstoaccess,suchasanareastruckbydisaster;or toodif�iculttoaccess,suchasanundergroundcavesy‑ stem.
Amobilesensornetworkcanbeprogrammedto operateintwodifferentways.The�irstwayistopro‑ gramittomaintaintheformation(i.e.theposition oftheagentsrelativetoeachother�whilesacri�icing overallcoveragecapability.Thesecondwayistoop‑ timizethesensingcapabilitywhilesacri�icingthefor‑ mationoftheagents.Thisresearchisconcernedwith
thesecondwayofoperatingthemobilewirelesssen‑ sornetwork.Coveragecontrolisanimportantpart ofanysystemthatutilizesmultiplemobileagentto achieveitsparticulargoals.Oneofthoseapplications isthemobilewirelessnetworksensor.Thisisa�ield thathasbecomeincreasinglypopularinrecenttimes duetoadvancementoftechnology,especiallyinthe �ieldsofminiaturizationandtelecommunications.
The�ieldofcoveragecontrolstartedtogainpopu‑ larityin2002withthereleaseofthepaperfromCor‑ tés,Martı́nez,Karatas,andBullo[3].Afterthatpoint, morepapersaboutcoveragecontrolwerepublished. Thosepapershavevaryingfociofresearch,suchas: moreef�icientalgorithms[7, 9, 11–13, 16, 18, 19],co‑ veragecontrolalgorithmwithnon‑idealconditions [4, 17],coveragecontrolalgorithmforacomplex�ield ofoperations[1, 2, 5, 8, 14, 15],anditsapplicationusing realhardware[6].Therehavenotbeenanystudies thatexaminetheperformanceofamobilewireless sensornetworksystemsthatoperatesinanareathat hasmultiplepointsofhighinterest.Thelackofper‑ formancedatainthisparticularsetuppresentsacon‑ siderablegapofknowledgeinthis�ield,sincethereis ahighpossibilitythatareal‑lifesystemwouldbere‑ quiredtobeabletohandlesuchascenario.
Themainprobleminthisresearchistherelati‑ velyuntestedsensingcapabilityofamobilewireless sensornetworksysteminanoperatingareathathas distributedand/ormultiplelocationsofhighinterest. Whilepreviousresearcheshavedealtwiththepro‑ blemofamobilesensornetworksystemoperatingin avariousoperatingareaslikeinpreviouslymentioned studies[1, 2, 5, 8, 14, 15],theperformanceofamo‑ bilewirelesssystemnetworksysteminanoperating areathathasdistributedand/ormultiplelocationsof highinterestisrelativelyunknown.Themainreason fortheimportanceofthisproblemisthathavingamo‑ bilewirelesssensornetworksystemthatcouldope‑ rateinanoperating�ieldthathasamultipleand/or distributedlocationsofhighinterestcouldoffermore �lexibilityinthereal‑lifeapplicationofsaidsystem. Whileintheoriginalresearchitwasnevermentioned thatthissystemcannotperformwellinanoperating �ieldthathasdistributedand/ormultiplelocationsof highinterest,theperformanceinsaidoperating�ield isrelativelyunknown.Thepurposeofthisresearchis todiscoverthecompatibilityofamultiple‑agentco‑ veragecontrolsystemunderseveralexamplesofin‑ terestfunctionsthathavemultipleand/ordistributed pointsofglobalmaximumvalue,inordertoexplore morethoroughlytheperformanceofagivensystemin
avaryingenvironments.Basedonthepreviouslymen‑ tionedpurpose,thisresearchaimstocontributebygi‑ vingseveralsamplesofbehaviorofamobilewireless sensornetworksystemundertheaconditiontode‑ terminethecompatibilityofanexistingcoveragecon‑ trolalgorithm.Theproposedmethodtoachievethe objectiveofthisresearchistoempiricallyformulate severalnewexamplesofinterestfunctionswithmul‑ tipleand/ordistributedpointsofhighinterestusing relativelysimplemathematicalexpressionsinorderto minimizemodi�icationstotheexistingalgorithm,and thentorunasimulationofamobilewirelesssensor networksystemwithexistingalgorithmsinsaidinte‑ restfunctions.Itishypothesizedthatwhileusingthe samealgorithm,theswarmwillbeabletoconvergeon thosemultipleinterestpointsalbeitwithreducedper‑ formance,suchasincreasedtimeuntilconvergence, non‑optimalsteadystatecoverage,ortakingawin‑ dingpathintheearlystagesofthedeployment.
Theinterestfunctionrepresentstheimportanceof acertainlocationinsideanoperatingarea.Itcanbe expressedas ϕ(q ): R2 → R,where q isanarbi‑ trarypointintheoperating�ield.Inthiscase,thein‑ terestfunctiontakesthe2Dpositionofapointand assignssaidpointavalueofimportance.Higherva‑ luecouldmeanhigherimportanceorhigherprobabi‑ lityofanoccurrenceofanobjectoraneventofinte‑ rest.Sensingfunctionrepresentsthecapabilityofan agenttoperformitstask.Thisfunctiontakesthepo‑ sitionandsensingcapability,inthiscaserepresented bythedistanceofapointintheoperating�ieldfrom thesensor,astheinputs,andgivesitssensingrelia‑ bilityastheoutput.Thisfunctioncanbeexpressedas f (pi ,q ): R2 × R2 → R,where pi isthe2Dposition ofthei‑thagentintheoperating�ieldand q isanarbi‑ trarypointinthe2Doperating�ield.Inthiscase,the reliabilityiscalculatedfromthe2Dsensingmodelof thesensorandthe2Dpositioninsidetheoperating area.
Coveragecontrolalgorithmhandlesthepositio‑ ningoftheagentsofamobilewirelesssensornetwork systemtoassurethateachagentbegivenanoptimum positiontoprovideitsmaximumpossiblecontribu‑ tion.Theoverallreliabilityofamobilewirelesssensor networksystemtakesaccountofallofitsagents’in‑ dividualreliability,whichheavilyrelyonthesensing function,andtheobjectivefunctionoftheoperating
�ield.�iththosefactorsaccountedfor,theoverallre‑ liability R(p1 ,...,pn ) canbeexpressedas
where f (q,pi ) relatestothesensingfunctionofi–thagent, ϕ(q ) referstotheinterestfunction,and Wi indicatesthesensingregionofthei–thagent.
Forthisstudy,theLloyd‑Maxalgorithmwillbe used[10].Thisalgorithmwasoriginallyusedinthe �ieldofsignalandtelecommunicationstooptimize quantizationinpulse‑codemodulation,butitisalso applicableinthe�ieldofcoveragecontrol.Inthecase oftheLloyd‑Maxalgorithmforcoveragecontrol,the sensingregionofanagentistheVoronoiregionofthe sensor,sincethisalgorithmworksundertheassump‑ tionthatonlythebestsensingvalueisusedforeach locationinsidewasoperating�ield.TheVoronoiregion ofanagentcanbeexpressedas
wherethepositionsofallNagentsactasthegenerator pointsfortheVoronoidecomposition.
Inordertoachieveagents’optimalposition,theva‑ lueof R(pi ) hastobeminimized.UsingtheLloyd‑Max algorithmtosolvethislocationaloptimizationpro‑ blem,theoptimalpositionofeveryagentscanbecal‑ culated.Then,accordingto[3],withagents’sensing functionof
thepositionoftheagentsisgovernedbytheequation
with pi denotingthepositionofthei‑thagent,and MWi and CWi calculatedusing
where Wi istheVoronoiregionofi‑thagent.Thisalgo‑ rithmcanbeinterpretedasthenextoptimalposition foreveryagentanditscentroidofthecurrentweig‑ htedVoronoiregion,therefore,asteadystatecanbe achievedifallagentsareintheirrespectiveoptimal positions.
3.1.ExperimentPreparation
Theentiretyoftheresearchprocessisdoneusing asoftwaresimulation.Thisisdonebecauseoftherela‑ tivelyuntestednatureofthetopicofthisresearch.Due tothecurrentnationalhealthandsafetyprotocols, saidguidelinerenderstheaccesstoseveralcompu‑ tersinlaboratoryunavailablewouldbeutilizableot‑ herwise.Therefore,sincetheexperimentwillbecon‑ ductedinonedevicewithrelativelyweakercompu‑ tingpower,severalsimulationlimitationswillalsobe imposedonthisresearch.
Thisresearchbeginswithaliteraturereviewto �indouttheunderlyingmechanismsofamobilewi‑ relesssensornetworksystem.Thenextstepistofor‑ mulatecandidatesofseveralinterestfunctionsthat willbetestedwithexistingcontrolalgorithm.Afterse‑ veralpromisingnewinterestfunctionshavebeenfor‑ mulated,thenextstepistochecktheircompatibility withtheexistingcontrolalgorithm.Thecompatibility ofsaidinterestfunctionsandthecontrolalgorithm willbemeasuredbymonitoringthevalueoftheover‑ allsensingreliability R(p1 ,...,pn ) overtime.
Inordertothoroughlyexploretheideaofinterest functionsthathavemultipleand/ordistributedpoints ofhighinterest,itisimprobabletoonlyhaveonenew
interestfunctionthatiscapableofbeinganexample ofmultitudeofpossibilitiesofcon�igurations.�ence, severalnewinterestfunctionswillbeproposed.These interestfunctions,asperthegoalofthisresearch,have morethanonepointofglobalmaximumvaluethat willbedistributedindifferingmanners,contraryto theirclassicalcounterpartswhichonlyhaveone.Ot‑ hernecessarypropertiesarethelimitationstobere‑ spected,namely,theboundariesoftheoperating�ield andthevalueof ϕ(q ).Theoperating�ieldmusthavea setboundaries,inordertobeabletobedecomposed intoseveralVoronoiregionswith�initesize,and ϕ(q ) isasemi‑de�initepositivefunction.
Forthisresearch,inordertoeaseanalysisofthere‑ sultsofthecompatibilitytest,alloftheinterestfuncti‑ onsinthisresearcharenormalizedtohavethetheva‑ lueof ϕ(q ) between0and1,andwitharectangular operatingareaboundedat 5 ≤ q ≤ 5.Fourinte‑ restfunctionswereformulatedempirically,eachwith itsownuniquedistributionofareaswithhighinterest, namely:
Fig.2. Experimentresultfortheclassiccase.Lefttorightcolumnsare”random”,”circle”,and”grid”startingconfiguration respectively.Toprowarethegraphsofvaluesofoverallsensingreliabilityovertime.Bottomrowareimagesofthe agents’trajectoryovertime.Blackcirclesdenoteinitialpositions.Blackcrossesdenotefinalpositions.Redlinesshowthe movementofagentsfrominitialtofinalpositions.Bluelinesshowtheborderofeachagents’Voronoiregionintheirfinal positions.Thecontouroftheinterestfunctionisalsoshown
Forthesakeofeaseinassociatingtheinterest functionswiththeirresultsinthispaperlater,these interestfunctionswillbenon‑formallycalled“pla‑ teau”,“basin”,“ridge”,and“valley”forequations(8) to(11)respectively.A3Dgraphicalrepresentationof thosefourinterestfunctionscanbefoundinFigure1 onthepreviouspage.
4.1.BaselinePerformanceFormulation
Tomeasurethecompatibilityofthenewinterest functionswiththeexistingcontrolalgorithm,twopa‑ rameterswillbeconsidered.The�irstistheoverall sensingreliabilityofthemobilewirelessnetworksy‑ stemovertime,tocheckitsgeneralperformance,and thesecondistheindividualagent’spositionovertime, tocheckforinef�icienciesofagents’movement,ifany. Sincethefocusofthisresearchistoseetheeffectsof thedifferentinterestfunctions,otherparametersare madetobeassimilaraspossiblebetweenoneinterest functionandanother.Foreveryinterestfunction,all fournewandoneold,therewillbe100agents,having sensingfunctionof(4),andthecalculationswillbe runfor100timesteps.Threeinitialcon�igurationsare usedforthestartingpositions,namely:“random”,“ci‑ rcle”,and“grid”.“�andom”con�igurationspreads100 agentsinarandompositionwithuniformdistribu‑ tion.Simulationsusingthisinitialcon�igurationswill beexecutedtentimesinordertogiveabetterpicture
oftheperformanceunderarandomstartinglocation foreachagent,andtoreducethevariancethatcould ariseduetotherandomnatureofthisstartingcon�i‑ guration.“�ircle”con�igurationplaces100agentsina circlearoundtheorigin,whilethe“grid”startingcon‑ �igurationspreads100agentsevenlyinaten‑by‑ten gridinsidetheoperatingarea.
Inordertobeabletomeasurecompatibility,aclas‑ sicinterestfunctionisalsoputintotheexperiment,in thiscase
ϕ(q )=1 qx 2 + qy 2 50 (12)
inordertocomplywiththelimitationspreviouslysta‑ ted.Theperformanceofthecontrolalgorithmunder thisinterestfunctionistobemadeabaselineper‑ formancewhichtheperformanceofthenewinterest functionsarecomparedagainst.
4.2.SensingReliabilityAnalysis
Fromthedatashownin�igures2.a,2.b,and2.cfor aclassicexampleandFigure3forthenewcases,it canbeobservedthatinbotholdandnewcases,the systemoperatesinasimilarfashion.Itstartsatare‑ lativelyhighvalue,thendecreasesovertime,bothre‑ spectivetoitsinterestfunction.Anotherinteresting observationisthatthevalueof R(pi ) isdecreasing inanexponential‑likebehaviorunderthe”random” and”circle”startingcon�igurations,andalmostline‑ arlyunder”grid”startingcon�iguration,regardlessof
R(p1 ,...,pn ) againsttimeforfournewcasesandthreestartingconfiguration.Topto bottomrowsarefor”random”,”circle”,and”grid”startingconfigurationrespectively.Lefttorightcolumnsarefor ”plateau”,”basin”,”ridge”,and”valley”interestfunctionsrespectively
Fig.3.
Tab.1. Overallsensingreliabilityforallcombinationsofinterestfunctionsandstartingconfigurations.Bothvaluesfor initialconditionandon100thtimesteparegiven
10 4 ,which,forall intentsandpurposes,cansafelyberoundeddowntozero
Fig.4. Agents’trajectorythroughtimeforfournewcasesandthreestartingconfigurations.Toptobottomrowsarefor ”random”,”circle”,and”grid”startingconfigurations,respectively.Lefttorightcolumnsarefor”plateau”,”basin”,”ridge”, and”valley”interestfunctions,respectively.Blackcirclesdenoteinitialpositions.Blackcrossesdenotefinalpositions. Redlinesshowthemovementofagentsfrominitialtofinalpositions.Bluelinesshowtheborderofeachagent’sVoronoi regionintheirfinalpositions.Thecontouroftheinterestfunctionisalsoshown
theinterestfunction.Itisalsoanobservationofinte‑ restthat�inalvalueofthesensingreliabilityisdepen‑ dentonthestartingpositionoftheagents.Thisim‑ pliesthatitispossibleforasystemwithworseinitial coveragebutmorefavorableagentsspreadtooutper‑ formanothersystemwithbetterinitialcoveragebut lessfavorablespreadatthe100thtimestep.Asum‑ maryofsensingreliabilityovertimethatcontainsnu‑ mericalvalueof R(pi ) atinitialand100thtimestepfor mostoftheexperimentcanbefoundinTable1onthe previouspage.
Fromtheexampleresultofagents’trajectoryin�i‑ gure4,itcanbeseenthatseveralagentsthatstarted inareaswithlowimportancemoveforanegligibledis‑ tanceordonotmoveatall,resultinginasuboptimal utilizationoftheresourcesgiven.Thisphenomenon isveryrareintheclassicexample,asshownin�igu‑ res2.d,2.e,and2.f.Thereasonfortheoccurrenceof thisphenomenoncanbenarroweddownbyexami‑ ningboththeequationthatgovernsthemovementof theagentandthevaluesofallvariablesinvolvedin thecalculation.Inthesecases,foragentsthathavethe entiretyofVoronoiregionattheminimumvalue,said agents’valueof MW becomesverysmallorzero.Since p =2MWi (CWi pi ),ifthevalueof MW isverysmall orzero,thevalueof p willalsobeverysmallorzero,re‑ sultinginnegligibleornomovementofsaidagent.In ordertopreventthisphenomenonfromhappening,a positiveoffsettotheentiretyofinterestfunctioncan beadded.Asummaryoftheagents’movement,which containsminimum,maximum,andaveragedistance traveled,canbefoundinTable2onthepreviouspage.
Basedontheresultofthesimulations,asample ofperformanceofamobilewirelesssensornetwork systeminanoperatingareawhichhasdistributed and/ormultiplelocationsofhighinteresthasbeen successfullyobtained.Besidethat,severalother�in‑ dingscanbeobserved.
Underthenewinterestfunctions,itcanbeobser‑ vedintheexperimentresultsthatthealgorithmisstill abletoperformitsdutyofmaximizingoverallsensing reliabilitybyminimizingthelocationaloptimization function,asseeninFigure 3.Figure 4 showstheinitial positionoftheagents,the�inalpositionoftheagents afterthe100‑timestepslimit,thepathtakenbyeach agents,thecontouroftheinterestfunction,andthe Voronoiregiondecompositionoftheoperating�ield basedonthe�inalpositionoftheagents.Notethatthe �inalpositionachievedinthisresearchisnotthemost optimalpositionpossibleforthegivenagents’starting positionsandinterestfunctions,sincetheexperiment ranforonly100timestepsduetocomputingpower andtimeconstraints.
Anothernoteworthy�indingisthatitispossible tomodelarelativelycomplexinterestfunctionsusing arelativelysimplemathematicalexpressionswhile maintainingcompatibilitywiththeexistingcontrolal‑
gorithm.This�indingisconsiderednoteworthysince thisopensthepossibilityofusingofmorecomplexor arbitraryinterestfunctions.
Forfurtherresearch,similarresearchwithexten‑ dednumberoftimestepscanbeperformedinorderto beabletoanalyzethealgorithm’ssteadystateperfor‑ mance.Anotherpossibilityistousedifferentsensing functionstoexaminetheperformanceunderasimilar interestfunction.Moreover,anapplicationusingphy‑ sicalagentsinacomparablesetupcouldbeconducted tohaveabetterunderstandingofitsreallifecapabili‑ ties.
RudyHanindito∗ –UniversitasGadjahMada, Yogyakarta,Indonesia,e‑mail:rudyhanin‑ dito@mail.ugm.ac.id.
AdhaImamCahyadi –UniversitasGadjahMada,Yo‑ gyakarta,Indonesia,e‑mail:adha.imam@ugm.ac.id. PraptoNugroho –UniversitasGadjahMada,Yogya‑ karta,Indonesia,e‑mail:tatok@ugm.ac.id.
∗ Correspondingauthor
Authorswouldliketothankallfamilies,colleagues, andfriendsfortheirmoral,knowledge,andmaterial supportincompletingthisresearch.
[1] T.M.ChengandA.V.Savkin,“Decentralized controlofamobilesensornetworkfordeploy‑ mentincorridorcoverage”.In: Proceedings ofthe48hIEEEConferenceonDecisionand Control(CDC)heldjointlywith200928thChi‑ neseControlConference,2009,pp.7897–7902, 10.1109/CDC.2009.5400065.
[2] T.M.ChengandA.V.Savkin,“Decentralized coordinatedcontrolofavehiclenetwork fordeploymentinsweepcoverage”.In: 2009IEEEInternationalConferenceonCon‑ trolandAutomation,2009,pp.275–279, 10.1109/ICCA.2009.5410535.
[3] J.Cortes,S.Martinez,T.Karatas,andF.Bullo, “Coveragecontrolformobilesensingnet‑ works”.In: Proceedings2002IEEEInternati‑ onalConferenceonRoboticsandAutomation (Cat.No.02CH37292),vol.2,Washington,DC, USA,2002,pp.1327–1332,10.1109/RO‑ BOT.2002.1014727.
[4] P.DamesandV.Kumar,“AutonomousLocali‑ zationofanUnknownNumberofTargetsWit‑ houtDataAssociationUsingTeamsofMobile Sensors”.In: IEEETransactionsonAutomation ScienceandEngineering,vol.12,no.3,2015,pp. 850–864,10.1109/TASE.2015.2425212.
[5] T.Elmokadem,“Distributedcoveragecontrolfor roboticsensornetworksin3dsensing�ields:
Barrierandsweepingproblems”.In: 2019Chi‑ neseControlConference(CCC),2019,pp.6088–6093,10.23919/ChiCC.2019.8865256.
[6] X.Han,L.Ma,andK.Feng,“Distributedcoverage controlofnetworkedheterogeneousrobots”.In: 20176thDataDrivenControlandLearningSys‑ tems(DDCLS),Chongqing,China,2017,pp.286–291,10.1109/DDCLS.2017.8068084.
[7] I.I.HusseinandD.M.Stipanovic,“Effective CoverageControlforMobileSensorNet‑ worksWithGuaranteedCollisionAvoidance”. In: IEEETransactionsonControlSystems Technology,vol.15,no.4,2007,pp.642–657, 10.1109/TCST.2007.899155.
[8] X.Jing,C.Fei,andX.Linying,“Event‑triggeredco‑ veragecontrolforcontinuous‑timemulti‑agent systems”.In: 201534thChineseControlConfe‑ rence(CCC),Hangzhou,China,2015,pp.1303–1308,10.1109/ChiCC.2015.7259822.
[9] M.Khaledyan,A.P.Vinod,M.Oishi,andJ.A. Richards,“OptimalCoverageControland StochasticMulti‑TargetTracking”.In: 2019 IEEE58thConferenceonDecisionandControl (CDC),Nice,France,2019,pp.2467–2472, 10.1109/CDC40024.2019.9029307.
[10] S.Lloyd,“Leastsquaresquantizationin PCM”.In: IEEETransactionsonInformation Theory,vol.28,no.2,1982,pp.129–137, 10.1109/TIT.1982.1056489.
[11] C.Y.T.Ma,D.K.Y.Yau,N.K.Yip,N.S.V.Rao, andJ.Chen,“StochasticSteepestDescentOp‑ timizationofMultiple‑ObjectiveMobileSensor Coverage”.In: IEEETransactionsonVehicular Technology,vol.61,no.4,2012,pp.1810–1822, 10.1109/TVT.2012.2189591.
[12] Y.NakaiandH.Ichihara,“Coveragecontrol ofmobilerobotswithadaptivegradient”.In: 201756thAnnualConferenceoftheSociety ofInstrumentandControlEngineersofJa‑ pan(SICE),Kanazawa,2017,pp.1016–1017, 10.23919/SICE.2017.8105446.
[13] M.Naruse,K.Sekiguchi,andK.Nonaka,“Co‑ verageControlforMulti‑CopterwithAvoidance ofLocalOptimumandCollisionUsingChangeof theDistributionDensityMap”.In: 201857thAn‑ nualConferenceoftheSocietyofInstrumentand ControlEngineersofJapan(SICE),Nara,2018,pp. 1116–1121,10.23919/SICE.2018.8492606.
[14] M.Santos,S.Mayya,G.Notomista,andM.Eger‑ stedt,“DecentralizedMinimum‑EnergyCo‑ verageControlforTime‑VaryingDensity Functions”.In: 2019InternationalSymposium onMulti‑RobotandMulti‑AgentSystems(MRS), NewBrunswick,NJ,USA,2019,pp.155–161, 10.1109/MRS.2019.8901076.
[15] A.ShamshirgaranandF.Abdollahi,“Dynamicco‑ veragecontrolviaunderactuatedautonomous
underwatervehiclesinunknown3Denviron‑ ment”.In: 20164thInternationalConfe‑ renceonControl,Instrumentation,andAutoma‑ tion(ICCIA),Qazvin,Iran,2016,pp.333–338, 10.1109/ICCIAutom.2016.7483184.
[16] R.Wang,W.Wan,X.Ma,andY.Li,“Cloud model‑basedcontrolstrategyonclustercom‑ municationcoverageforwirelesssensornet‑ works”.In: 20102ndInternationalAsiaConfe‑ renceonInformaticsinControl,Automationand Robotics(CAR2010),vol.1,2010,pp.307–310, 10.1109/CAR.2010.5456840.
[17] Y.WangandI.I.Hussein,“AwarenessCoverage ControlOverLarge‑ScaleDomainsWithInter‑ mittentCommunications”.In: IEEETransacti‑ onsonAutomaticControl,vol.55,no.8,2010,pp. 1850–1859,10.1109/TAC.2010.2042346.
[18] J.Xiao,L.Sun,andS.Zhang,“Distanceop‑ timizationbasedcoveragecontrolalgo‑ rithminmobilesensornetwork”.In: 2008 IEEEInternationalConferenceonSystems, ManandCybernetics,2008,pp.3321–3325, 10.1109/ICSMC.2008.4811809.
[19] K.YangandX.Wang,“Team‑BasedBlanketCo‑ verageControlforHeterogeneousMulti‑agent Systems”.In: 202039thChineseControlConfe‑ rence(CCC),Shenyang,China,2020,pp.759–764, 10.23919/CCC50068.2020.9189045.