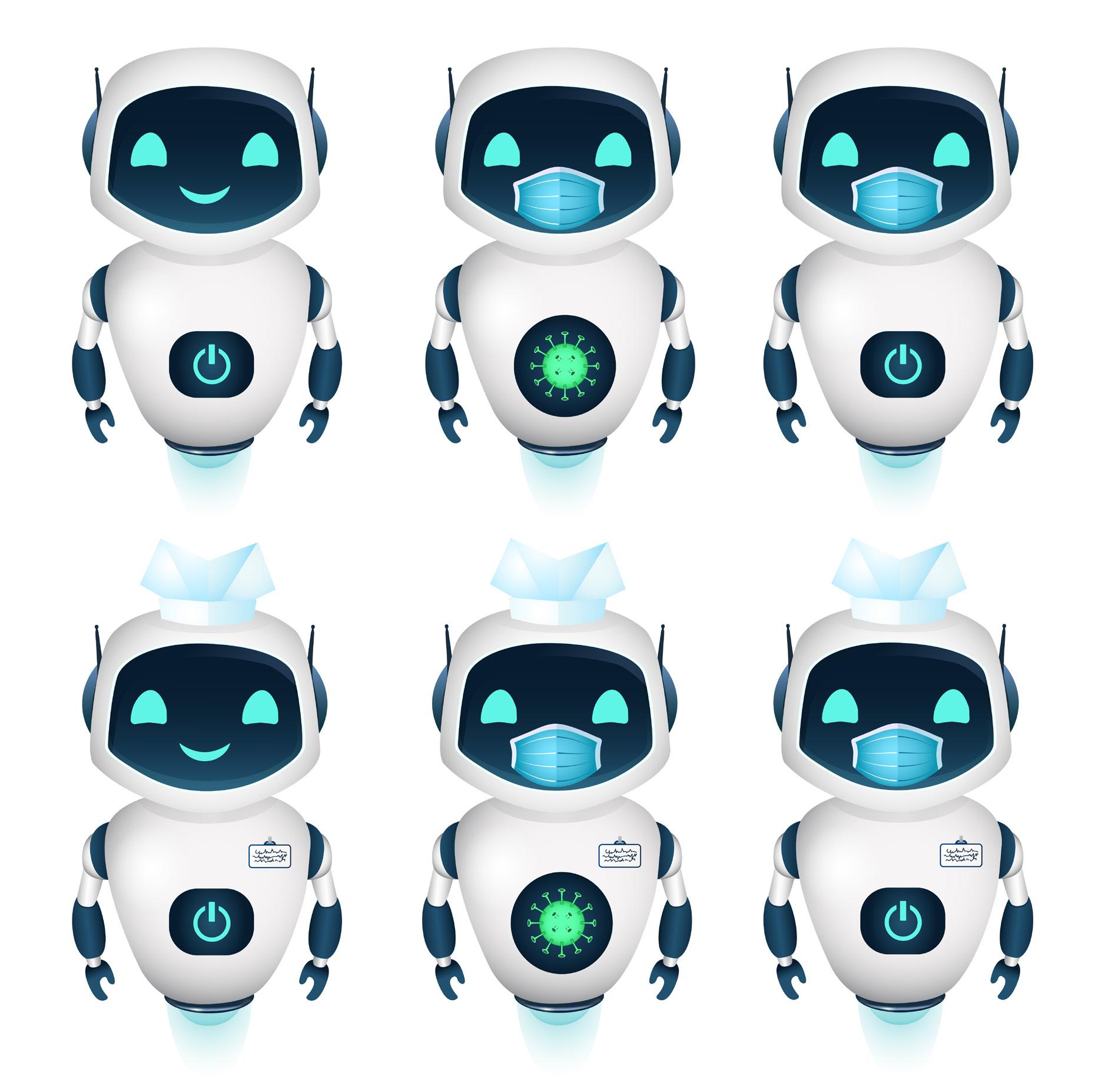
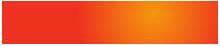
Fundamentals of
Fundamentals of
A peer-reviewed quarterly focusing on new achievements in the following fields:
Mechatronic systems in robotics
Applied automatics
Sensors and
Janusz Kacprzyk (Polish Academy of Sciences, Łukasiewicz-PIAP, Poland)
Dimitar Filev (Research & Advenced Engineering, Ford Motor Company, USA)
Kaoru Hirota (Japan Society for the Promotion of Science, Beijing Office)
Witold Pedrycz (ECERF, University of Alberta, Canada)
Roman Szewczyk (Łukasiewicz-PIAP, Warsaw University of Technology, Poland)
Oscar Castillo (Tijuana Institute of Technology, Mexico)
Marek Zaremba (University of Quebec, Canada)
Katarzyna Rzeplinska-Rykała, e-mail: office@jamris.org (Łukasiewicz-PIAP, Poland)
Piotr Skrzypczynski (Poznan University of Technology, Poland)
Statistical Editor
Małgorzata Kaliczynska (Łukasiewicz-PIAP, Poland)´
Editorial Board:
Chairman – Janusz Kacprzyk (Polish Academy of Sciences, Łukasiewicz-PIAP, Poland)
Plamen Angelov (Lancaster University, UK)
Adam Borkowski (Polish Academy of Sciences, Poland)
Wolfgang Borutzky (Fachhochschule Bonn-Rhein-Sieg, Germany)
Bice Cavallo (University of Naples Federico II, Italy)
Chin Chen Chang (Feng Chia University, Taiwan)
Jorge Manuel Miranda Dias (University of Coimbra, Portugal)
Andries Engelbrecht (University of Pretoria, Republic of South Africa)
Pablo Estévez (University of Chile)
Bogdan Gabrys (Bournemouth University, UK)
Fernando Gomide (University of Campinas, Brazil)
Aboul Ella Hassanien (Cairo University, Egypt)
Joachim Hertzberg (Osnabrück University, Germany)
Evangelos V. Hristoforou (National Technical University of Athens, Greece)
Ryszard Jachowicz (Warsaw University of Technology, Poland)
Tadeusz Kaczorek (Białystok University of Technology, Poland)
Nikola Kasabov (Auckland University of Technology, New Zealand)
Marian P. Kazmierkowski (Warsaw University of Technology, Poland)
Laszlo T. Kóczy (Szechenyi Istvan University, Gyor and Budapest University of Technology and Economics, Hungary)
Józef Korbicz (University of Zielona Góra, Poland)
Krzysztof Kozłowski (Poznan University of Technology, Poland)
Eckart Kramer (Fachhochschule Eberswalde, Germany)
Rudolf Kruse (Otto-von-Guericke-Universität, Germany)
Ching-Teng Lin (National Chiao-Tung University, Taiwan)
Piotr Kulczycki (AGH University of Science and Technology, Poland)
Andrew Kusiak (University of Iowa, USA)
Mobile robots control
Data transmission
Distributed systems
Biomechatronics
PanDawer, www.pandawer.pl
Piotr Ryszawa (Łukasiewicz-PIAP, Poland)
ŁUKASIEWICZ Research Network
Navigation
Mobile computing
– Industrial Research Institute for Automation and Measurements PIAP Al. Jerozolimskie 202, 02-486 Warsaw, Poland (www.jamris.org) tel. +48-22-8740109, e-mail: office@jamris.org
The reference version of the journal is e-version. Printed in 100 copies. Articles are reviewed, excluding advertisements and descriptions of products. If in doubt about the proper edition of contributions, for copyright and reprint permissions please contact the Executive Editor.
Mark Last (Ben-Gurion University, Israel)
Anthony Maciejewski (Colorado State University, USA)
Krzysztof Malinowski (Warsaw University of Technology, Poland)
Andrzej Masłowski (Warsaw University of Technology, Poland)
Patricia Melin (Tijuana Institute of Technology, Mexico)
Fazel Naghdy (University of Wollongong, Australia)
Zbigniew Nahorski (Polish Academy of Sciences, Poland)
Nadia Nedjah (State University of Rio de Janeiro, Brazil)
Dmitry A. Novikov (Institute of Control Sciences, Russian Academy of Sciences, Russia)
Duc Truong Pham (Birmingham University, UK)
Lech Polkowski (University of Warmia and Mazury, Poland)
Alain Pruski (University of Metz, France)
Rita Ribeiro (UNINOVA, Instituto de Desenvolvimento de Novas Tecnologias, Portugal)
Imre Rudas (Óbuda University, Hungary)
Leszek Rutkowski (Czestochowa University of Technology, Poland)
Alessandro Saffiotti (Örebro University, Sweden)
Klaus Schilling (Julius-Maximilians-University Wuerzburg, Germany)
Vassil Sgurev (Bulgarian Academy of Sciences, Department of Intelligent Systems, Bulgaria)
Helena Szczerbicka (Leibniz Universität, Germany)
Ryszard Tadeusiewicz (AGH University of Science and Technology, Poland)
Stanisław Tarasiewicz (University of Laval, Canada)
Piotr Tatjewski (Warsaw University of Technology, Poland)
Rene Wamkeue (University of Quebec, Canada)
Janusz Zalewski (Florida Gulf Coast University, USA)
Teresa Zielinska (Warsaw University of Technology, Poland)
Volume 15, N° 4, 2021
DOI: 10.14313/JAMRIS/4-2021
3
An AI & ML Based Detection & Identification in Remote Imagery: State-of-the-Art
Hina Hashmi, Rakesh Dwivedi, Anil Kumar
DOI: 10.14313/JAMRIS/4-2021/22
Trajectory Planning for Narrow Environments That Require Changes of Driving Directions
Jörg Roth
DOI: 10.14313/JAMRIS/4-2021/23
30
Experimental Research of Veles Planetary Rover Performing Simple Construction Tasks
Maciej Trojnacki, Przemysław Brzęczkowski, Dominik Kleszczyński
DOI: 10.14313/JAMRIS/4-2021/24
Development of Grinding and Polishing Technology for Stainless Steel With a Robot Manipulator
Jinsiang Shaw, Yu-Jia Fang
DOI: 10.14313/JAMRIS/4-2021/25
44
Development of Smart Sorting Machine Using Artificial Intelligence for Chili Fertigation Industries
M. F. Abdul Aziz, W. M. Bukhari, M. N. Sukhaimie, T.A. Izzuddin, M.A. Norasikin, A. F. A. Rasid, N. F. Bazilah
DOI: 10.14313/JAMRIS/4-2021/26
The Identification Method of the Sources of Radiated Electromagnetic Disturbances on the Basis of Measurements in the Near-Field Zone
Krzysztof Trzcinka
DOI: 10.14313/JAMRIS/4-2021/27
Resource Optimisation in Cloud Computing: Comparative Study of Algorithms Applied to Recommendations in a Big Data Analysis Architecture
Aristide Ndayikengurukiye, Abderrahmane Ez-Zahout, Akou Aboubakr, Youssef Charkaoui, Omary Fouzia
DOI: 10.14313/JAMRIS/4-2021/28
Simple and Flexible Way to Integrate Heterogeneous Information Systems and Their Services into the World Data System
Grzegorz Nowakowski, Sergii Telenyk, Kostiantyn Yefremov, Volodymyr Khmeliuk
DOI: 10.14313/JAMRIS/4-2021/29
Submitted: 8th October 2021; accepted: 8th February 2022
DOI: 10.14313/JAMRIS/4-2021/22
Remotely sensed images and their allied areas of ap plication have been the charm for a long time among researchers. Remote imagery has a vast area in which it is serving and achieving milestones. From the past, after the advent of AL, ML, and DL-based computing, remote imagery is related techniques for processing and ana lyzing are continuously growing and offering countless services like traffic surveillance, earth observation, land surveying, and other agricultural areas. As Artificial intel ligence has become the charm of researchers, machine learning and deep learning have been proven as the most commonly used and highly effective techniques for object detection. AI & ML-based object segmentation & detection makes this area hot and fond to the research ers again with the opportunities of enhanced accuracy in the same. Several researchers have been proposed their works in the form of research papers to highlight the effectiveness of using remotely sensed imagery for commercial purposes. In this article, we have discussed the concept of remote imagery with some preprocess ing techniques to extract hidden and fruitful information from them. Deep learning techniques applied by various researchers along with object detection, object recog nition are also discussed here. This literature survey is also included a chronological review of work done re lated to detection and recognition using deep learning techniques.
Keywords: Convolutional Neural Network, Remote Sensed Imagery, Object Detection, Artificial Intelligence, Feature Extraction, Deep Learning, Machine Learning
In this continuously growing world in the direction and advancement of technology, remote imagery is serving the world in multiple facets. Data nowadays is collected and stored in digital formats that make interpretation and analysis possible on that. Remote sensing images, images are collected through various satellites, Aerial photography, Lidar, Landsat, Spy Sat ellites, and sentinel images. Remote imagery is nowa days recorded in digital form so that analysis can be done on them for extracting some hidden information from them. But the limitation with the remote imag ery is that the images collected from the satellites are not always up to the mark. It usually contains some kind of noise, unclear visuals, and mixed color chan
nels. So, it becomes necessary to process that data before applying any processing on them [31]. Digital image processing involves many procedures and func tions to format and correct the data for segmentation and classification. With the help of those processes and techniques, the data can be used refined and it can be used for various commercial purposes like Earth observation, weather forecasting, Forestry, Ag ricultural usage, Surface changes, and the analysis of Bio-diversity. Remote applications are also useful for interpreting the road conditions in rural areas, iden tifying crop conditions, etc. For a long time, remote sensing images are being processed and analyzed with the help of deep learning techniques that is part of neural networks. Although, before the development of deep learning techniques, remote sensing imagery was being observed with the help of a support vector machine (SVM) and various ensemble classifiers like Random forest for change detection or image classifi cation. SVM got high demand and attention due to its capability of dealing with high and multi-dimensional data with a limited amount of training data [2].
In the recent era, the emerging trends in DL have renewed the interest of the remote community for neural networks. From the near 2014, the whole re mote sensing community has diverted its attention toward DL as DL techniques and algorithms have re corded its achievements in many image analysis tasks like Land cover Land use observations, object detec tion, scene classification, etc [30] [14] [36] [52] [35] [53] [54]. Going through the vast literature regarding DL is showing that DL is having general approaches related to the development of basic deep learning algorithms [55] and the detailed reviews for vari ous emerging and advanced fields like medical im age recognition, speech recognition techniques [56]. Some studies are showing evidence of DL in remote sensing applications [4]. The literature survey by [57] has focused on the applications of DL in remote sensing images classification for major observations. [15] has performed a complete broad review specif ically focusing on related uncommon sub-areas of remote data application areas like 3-d modeling. DL algorithms and techniques are having diverse sub-do main within the remote sensing field and the applica tion areas are continuously increasing in getting the more quantitative and systematic analysis of the data [32]. The motivation of this study is to draft a com prehensive review of DL algorithms in various areas and sub-areas of remote sensing applications that include object detection, image segmentation, scene
classification, image registration, image fusion, etc in images as well as videos [33]. By going through a vast review in the area of object detection in remote imag es through deep learning techniques, we have sum marized the findings in various research articles. At last, a critical summary is included followed by major gaps and challenges found.
Remotely sensed images are not simple images, they contain multiple formats and resolution chal lenges. They can be single channel or multi-band images having variations in their resolutions too. The spatial resolution of remote imagery is the most important aspect that is directly related to the accuracy of objects. To generate land cover maps for various reasons like environment planning, change detection, transport, and traffic planning temporal resolution is used. Medium resolution re mote sensed imagery is used for data integration, analysis of urban areas, also to differentiate various zones like residential, industrial, and commercial. By reviewing a huge number of databases related to various articles on remote sensing data, features, and parameters, the information is summarized and shown in Table 1.
Tab. 1. Attributes used for Remote Sensing and DL Attributes Categories
Remote Sensing Data Hyper-spectral, Lidar, SAR, etc.
Target Study Area Urban, Agriculture, Rural, Water DL Model CNN, RNN, AE, DBN, other
Target Scene Classification, Image Fusion, Object Detection, Segmentation, LULC Classification, and other
Processing Parameters Object, Pixel Samples for Training Value
Accuracy Value Study Site Value
Paper Category Conference, Journal Image Resolution Value (high resolution, coarse, moderate)
Satellite data includes various other resolutions and types of images. Spatial Resolution is to mea sure the closed lines in an image. Spatial resolution is dependent on the device from which the image is captured. It is not only to measure the capacity of ppi (pixel per unit). The spatial resolution of any image decides its quality in the form of clar ity. It generally refers to the count of independent pixels per unit in any image. Spatial resolution is limited by aberrations, diffraction, imperfect focus, and atmospheric distortion. Figure 1 (a), 1(b), 1(c) is showing the difference between multiple range spatial resolutions.
Spectral Resolution is to resolve spectral features as separate components, spectral resolution is used. Color images involve multiple and distinguished light effects on different spectra as Fig. 2 is showing. Multi
Fig. 1.(a) Spatial Resolution of 1 meter (b) 10 meter (c) 30 meter
A temporal resolution is a measurement unit of any area concerning time as a movie and high-speed cam eras can capture the scenes at many different points in time. Time resolution that a general movie camera captures generally at a rate of 24-48 frames/ second. Although, high-speed cameras can capture the scenes at a very high speed up to 50-300 frames/ second or even more. Radio-metric Resolution is to determine the fine representation and differentiation among intensity, radiometric resolution is used. It is gener ally represented as the number of bits or levels. For ex- digital image is having 256 levels i.e. 28 bits. The reflected intensity will be better and finer than most as the bits are higher. While working practically, noise levels are used to limit radiometric resolutions in stead of bits representation. Multi-Spectral Resolution is performed on the multi-spectral image the image data is captured across the range of electromagnetic spectrum at a specific wavelength. The wavelength can be captured with the help of supported devices that can separate to capture or detect by filters some times beyond the visibility range of light like- ultravi olet and infrared. Hyperspectral Resolution is gener ally applied on hyperspectral images is also a kind of multi-spectral image that captures the image data at several different wavelengths of the electromagnetic spectrum. To extract the data for each pixel in an im age for detection of objects, material identification, hyperspectral images are used.
As remote sensing imagery is having various reso lutions and dimensions of the data, rectification, and restoration of data became the important aspect for getting desired information or extracting hidden data by analyzing the image. The pre-processing operation is generally applied to correct and refine platform or sensor-specific radiometric data. It also includes the geometric distortion of the data. For eliminat ing scene illusions, sensor noise, etc. these types of pre-processing are required for remote imagery. Var
band images can resolve finer differences of wave lengths or spectrum by storing or measuring common RGB images.ious pre-processing methods are used to rectify the data collected by the sensor are included further. Each of these methods is different by their working nature or by having a different sensor or platform used for data acquisition.
Radiometric Corrections. Correction of data that includes the issues related to unwanted sensor data, irregularities of sensor data is the prime function of radiometric corrections. After the corrections, the data is converted to accurately measure the reflected light by the sensor.
Contrast Enhancement. It involves increasing in contrast value among background and target. An im age histogram is a basic concept to understand con trast enhancement.
Geometric Corrections. For correcting the ge ometric distortion that occurs because of Earth-sen sor geometrical variations and to convert that into real-world earth-surface coordinates, geometric cor rections are used. Distortion can arise due to reasons that include altitude variations, the velocity of the sensor platform, earth convexity, atmospheric diver sion, length of the displaced object.
Spatial Filtering. To reduce the smaller details in an image, Low pass spatial filters are designed to focus more on homogeneous large pixels of the same tone. This makes the smooth appearance of an image. These kinds of spatial filters are very much useful for reducing random noise from the image. Median and Average filters are an example of low pass spatial fitters [34]. On contrary, High pass filters work just opposite the operation that a low pass filter does. It operates to sharpen the fine details in the image to fine-tune the appearance. Some filters like edge or di rectional detection filters are used to identify the field boundaries or roads in an image.
Band Rationing. One of the most commonly ap plied transformations is spectral rationing or band rationing. It serves to highlight and focus the spectral variations of surface covers.
Piecewise Linear Stretch for contrast enhance ment. To utilize the complete range of value in bright ness component, the maximum and minimum param eters of data allocated to new applied data. For e.g.: an image is having a minimum brightness value of 45 and a maximum of 205. If that image (Fig. 3) is rep resented without enhancement, the values from 0 to 44 and the values from 206 up to 255 will not be dis played. By stretching the values from 0 to 45 and up to 205, the important features can be accessed.
Deep learning is a subset of Artificial intelligence that helps in creating automated applications and services for performing analytical physical tasks without human involvement. Now deep learning is behind each everyday product including digital as sistants, self-driven cars, credit card fraud detection, and many more emerging techniques. Deep learning involves a neural network with a minimum of three layers. This network is developed to simulate human behavior through machines by learning them with a huge amount of data. Deep learning is an organized structure of multiple hidden layers. A single layer in a neural network is capable of predicting results; still, additional layers can be used for enhancing accuracy and efficiency [35].
Recent work in this field is showing that deep learning has achieved a lot in the field of replicating human behavior either in a simple task or complex operations like object detection, and image classifica tion [36]. If comparing deep learning with other tra ditional approaches it is performing outstandingly in result predictions with good accuracy. Deep learning was firstly introduced in the 1980s and it has become the most emerging technology for serving the world in various domains. It requires a large amount of la beled data with the highest computational power to train the model to get more accurate results. Deep learning models learn features directly from data other outside feature extraction techniques are not required while working with a deep neural network. Deep learning can be classified into various catego ries like Supervised and Unsupervised. Feature ex traction is one of the most important aspects of deep learning that uses an algorithm to construct meaning ful features automatically for training, understanding, or learning.
From 1943 till now deep learning is being used and improving its applications day by day. Image process ing through deep learning can be tracked since early 1943. In [58] created a neural network like the hu man brain by using a combination of algorithms and threshold logic. In [59], researchers have developed a continuous backpropagation model by improvising the basic neural networks. In 1962, a simple neural network model for image classification using the ba sic chain rule is developed. In 1965, a deep learning model for group data handling is developed. During the 1970s, the very first AI winter came into existence that uses some AI techniques for basic image process ing. In 1973, Neocognitron that was an artificial neu ral network that used multilayered and hierarchical designs is created. The proposed system was able to recognize visual patterns through the computer. In [50] demonstrated backpropagation by combining CNN along with backpropagation for reading hand written digits through the computer. In 1991, a mod el is created to identify the problems related to van ishing gradient. In [60] a paper is presented on deep belief networks for learning the images in a faster manner. For speech recognition, deep learning algo rithms are developed. In 2009, ImageNet is launched to serve deep learning researchers. In 2012, AlexNet
Fig. 3. Piecewise Linear Contrast Stretchis developed which is constructed with multiple GPUs concept. In [61] Generative Adversarial Networks is developed to enhance deep learning abilities in sci ence, art, and fashion.
Over recent years, Computer Vision tasks, such as ob ject detection, image classification especially in digi tal images and videos grasp a lot of attention from researchers. Computer vision is a part of Artificial Intelligence that makes the computer enables every thing that a human mind can perform from simple calculations to analyzing or reading minds [3]. Object detection is a technology related to Computer Vision that directly deals with the detection of objects from various classes [37]. Among all the problems and challenges available in image recognition, object de tection is the major challenge to solve with the help of the Computer. Object detection is an emerging tech nology nowadays as it consists of various applications in various domains like Face Recognition, Video- Sur veillance, Crowd Counting, Transport Management, Image annotation, Video co-segmentation, tracking the locations of a ball during a cricket match, etc. Figure 4 is showing the milestones achieved in the field of object detection through traditional and deep learning methods.
Computer vision in favor of AI is touching the benchmarks in the world of the computer by increas ing its computational power and results in calculation abilities with more accuracy and reliabilities. In [37] highlighted and focused on the role of computer vi sion in his work. Several deep learning methods are adopted to develop deep learning models for remote sensing images; some of them include transfer learn ing, learning rate decay, training from scratch, and dropout. Nowadays Artificial Intelligence and the various ways of computing of achieving the same are also in practice and evaluation. So, the researchers are looking the AI ways like Machine Learning and Deep Learning to attain new and amazing results in remote sensing imagery. Hence, during this study, we will be exploring various facets of remote sensing imagery, advanced artificial intelligence-based machine learn ing, and deep learning techniques for remote sensing imagery segmentation, object detection, and classifi cation.
In [38] presented various examples of measuring space clustering processes for a range of three mul tispectral images captured over Ariz, Phoenix. In [51], NASA proposed the study at the Centre for Research, the University of Kansas with the cooperation and support of government research agencies and other universities have shown the applicability of satellite imagery in various fields within agriculture, ocean ography, and earth sciences. This paper shows how characteristics and features of radar are used to get geo-science information.
In [39] [40], the authors described a process for spatial registration of multi-temporal and digital multi-spectral imagery. Experimental results are also defined here as the result of correlation analysis be
tween digital satellite photographs and multi-spectral imagery. The registration process of space photogra phy and multi-spectral airborne line-scanner digital imagery is also described here.
In [41], authors attempted to identify between welters of results to get unbeatable achievements also liked hurdles, also to assess the contribution to get expected image-processing operations in exper imental and operational usage of the upcoming tor rent of raw data.
Recent trends in deep learning techniques are emerging with powerful methods for automated sys tems that involve automatic feature learning through raw data. Most particularly, these methods achieved benchmarks in object detection; this area has become the most interesting area for new researchers [24].
Along with computer vision is touching the trends of the computer world in computational pow er with a tremendous speed, and result producing capacities with more reliability than human minds. In [37] highlighted the role of vision tasks with the help of certain projects to prove the tested approach for various research works like virtual skinning, vir tual painting, human-computer interaction, depth recovery, etc.
In [42] detected motion of objects using cellular neu ral network. In [43] provided a model to detect and focus objects in an unfocused background. In [45] de veloped an object-oriented based segmentation algo rithm for edge extraction. In [46] developed a model to detect patterns in spectrograms using cellular neu ral network. In [47] developed a robust model based on deep learning methods to detect pedestrian using low- and high-level features. In [48] developed a mod el using CNN for logo detection of vehicles. In [53] pro vided their review for object detection algorithms for optical remote sensing imagery. They focused to re view the brief history of available literature for deep learning techniques and the datasets for the detection of objects from remote imagery. Table 2 is represent ing the work (done in the period 2012-19) by many researchers on various datasets and extracted results for object detection in earth observation [2]. The re view is given for dataset with the category of images, image count including width and the detected objects annotation style. A number of methods are developed for detection and recognition of objects. Many de tector algorithms are proposed like VJ (Viola Jones),
similar
figure 6 (c). The difference is in range, the range is here is [ 1, 1]. The benefit of using the tanh function is that here negative numbers are mapped as strongly negative and zero values mapped near zero.
ure 6 (c). The difference is in range, the range is here [ 1, 1]. The benefit of using the tanh function is that here negative numbers are mapped as strongly negative and zero values mapped near zero.
Hyperbolic Tangent (tanh) function It is very much similar to the sigmoid function as it can be seen in figure 6 (c). The difference is in range, the range is here is [ 1, 1]. The benefit of using the tanh function is that here negative numbers are mapped as strongly negative and zero values mapped near zero.
is that here negative numbers are mapped as strongly negative and zero values mapped near zero.
(6)
(6)
Dropout Layer. This layer is used to help in over-fit ting issues also improves the network’s performance. The dropout layer can be applied in any of the layers.
(6)
(a) (b) (c) (6) (a) (b)
Fig. 6. Representation of various activation functions: a ReLU b Sigmoid, c tanh
. 6. Representation of various activation functions: a ReLU b Sigmoid, c tanh
(c)
Fig. 6. Representation of various activation functions: a- ReLU b- Sigmoid, c- tanh
Fig 6 Representation of various activation functions: a ReLU b Sigmoid, c tanh
Normalization Layers. To implement inhibition paradigms, the normalization layer is used for the observation of the biological brain [62].
Normalization Layers. To implement inhibition paradigms, the normalization layer is used for the observation of the biological brain [62].
Normalization Layers. To implement inhibition paradigms, the normalization layer is used for the observation of the biological brain [62].
Normalization Layers. To implement inhibition par adigms, the normalization layer is used for the obser vation of the biological brain [62].
Pooling Layer. After each successive convolution layer, the pooling layer is presented that can reduce input layer size via some non linear functions. They also help in reducing the computational and parametric amount in the network. It also helps to control over fitting [63]. Figure 7 is representing the pooling operation with [2 x 2] filter. Following are some main pooling operations: Max pooling. The maximum value is calculated for each input patch. It preserves the maximum value of each stride while sliding over the feature map. Its mathematical representation is as follows
Pooling Layer After each successive convolution layer, the pooling layer is presented that can reduce input layer size via some non linear functions. They also help in reducing the computational and parametric amount in the network. It also helps to control over fitting [63]. Figure 7 is representing the pooling operation with [2 x 2] filter. Following are some main pooling operations: Max pooling. The maximum value is calculated for each input patch. It preserves the maximum value of each stride while sliding over the feature map. Its mathematical representation is as follows
Pooling Layer After each successive convolution layer, the pooling layer is presented that can reduce input layer size via some non linear functions. They also help in reducing the computational and parametric amount in the network. It also helps to control over fitting [63]. Figure 7 is representing the pooling operation with [2 x 2] filter. Following are some main pooling operations: Max pooling. The maximum value is calculated for each input patch. It preserves the maximum value of each stride while sliding over the feature map. Its mathematical representation is as follows
Pooling Layer. After each successive convolution layer, the pooling layer is presented that can reduce input layer size via some non-linear functions. They also help in reducing the computational and para metric amount in the network. It also helps to control over-fitting [63]. Figure 7 is representing the pooling operation with [2 x 2] filter. Following are some main pooling operations:
Max pooling. The maximum value is calculated for each input patch. It preserves the maximum value of each stride while sliding over the feature map. Its mathematical representation is as follows
(7)
(7)
Average pooling For each input, it calculates the average value. This layer divides the input various pooling regions by computing their average values.
Average pooling For each input, it calculates the average value. This layer divides the input various pooling regions by computing their average values.
(7)
Fully Connected Layer. At this layer, the final out put is represented in the form of a 2-D array by con nected with a fully connected layer. By the result of the Convolution process, this layer classifies an image into various classes. The activation function at the last layer computes the probability of results belonging to each class [16]. Commonly, for multi-class classifica tion, the softmax activation function is used having a range of probability between [0, 1] with a sum equal to 1. The fully connected layer is situated at the last layer in a neural network. The fully connected layer is having the neurons that have a full connection to all the neurons of previous layers [63]. The calculations of this connection can be evaluated by matrix multi plication function later followed by bias offset [62].
The remainder of this paper is being organized as section-2 is showing the various existing pre-trained models on ImageNet and CIFAR-10 datasets, section-3 is giving the inceptive contributions at beginning of re mote senses image processing, section-4 is the detailed literature review as a tabulated formation categorized by various technological benchmarks used, section-5 shows the challenges mapping with reviewed litera ture and section 6 involves the complete summary.
(7)
Average pooling. For each input, it calculates the av erage value. This layer divides the input various pool ing regions by computing their average values.
Average pooling. For each input, it calculates the average value. This layer divides the input various pooling regions by computing their average values.
(a) (b) (c) (8)
= (8)
= (8)
Fig. 7. Operation of Pooling using 2 x 2 filters with stride of two
Deep neural networks, such as Convolutional neural networks [64], Recurrent neural networks [65], Graph neural networks [66], Attention neural networks [67], have been applied for various AI tasks at a wide range. The pre-trained models can be considered as the models that are developed based on any exist ing techniques (As mentioned various deep learning techniques). The models that have been already got trained on some dataset like ImageNet, CifarNet-10 are known as pre-trained models. Nowadays pretrained models are widely used for image classifica tion and object detection. Table 3, 4are showing the summary and comparison of various pre-trained models trained on ImageNet and CIFAR-10 dataset respectively.
Tab. 3. Various pre-trained models trained on ImageNet dataset
Model Depth Size (MB) Parame ters (Million) Top-1 Accuracy Top-5 Accura cy
Xception 81 88 22.9M 79.0% 94.5%
VGG16 16 528 138.4M 71.3% 90.1%
VGG19 19 549 143.7M 71.3% 90.0%
ResNet50 107 98 25.6M 74.9% 92.1%
Inceptionv3 189 92 23.9M 77.9% 93.7%
DenseNet201 402 80 20.2M 77.3% 93.6%
EfficientNetB0 132 29 5.3M 77.1% 93.3%
Tab. 4. Various pre-trained models trained on CIFAR-10
Model Depth Size (MB)
Parameters (Million)
Top-1 Accuracy Top-5 Accuracy
DenseNet121 242 33 8.1M 75.0% 92.3%
EfficientNetV2L 479 52 1.19M 85.7% 97.5%
MUXNet-m 289 62 2.1M 98.0% 98.3%
AutoFormer-S 384 384 81 23M 99.1% 99.2%
In [6] authors presented a model for the interpreta tion and evaluation of scenes for image analysis.
In paper [5] authors proposed a blackboard mod el as a control structure for the detection of objects in aerial images.
In [7] researchers focused their research on feature extraction in SAR images as this process has a higher level of complexity due to the level of noise and quality issues in these images. This paper implemented an au tomated algorithm for feature extraction of large-scale objects from SAR imagery. For the experiments, images of the Ottawa area are used through SAR imagery.
In [8] authors proposed a model to detect a cover change in the forest on Landsat data.
In [9] authors developed a model for the detection of manmade objects like airports, bridges, industries, etc.
In [10] authors proposed a classification and detec tion model named CADCM to target hidden objects in hyperspectral imagery. The process is accomplished in three phases. Initially, a band selection process is ap plied, next band rationing is done and finally, automatic target detection is achieved. Results show the targets hidden by natural background, shades, or objects can be detected finely.
In [11] two matrices namely object space and im age space are used to refer and monitor the existing monocular building detection system with the help of 83 images collected by 18 different sites. By the anal ysis, the effects of image inscrutability along with ob jects complexity are examined. Edge fragmentation is also shown here in this research. The usage of rigorous photogrammetric space modeling is also demonstrat ed.
In [12] a review article is presented to reduce cloud impacts by analyzing various existing algorithms.
In [13] an automatic approach for building foot print extraction and its 3-D reconstruction from the imagery of airborne light and ranging (LIDAR) data is represented. Initially, a digital surface model (DSM) is generated to extract objects higher than the ground surface. To separate a building from other objects, ge ometric characteristics such as size, height, and shape are used. Extracted building footprints are simplified for better quality using an orthogonal algorithm. Roofs are identified by information like ridgelines and slopes. Finally, an accuracy assessment is conducted by com paring the results with manually digitized building ref erence data.
In [14] authors represented the image analysis method for the extraction of building features. They have used three consecutive steps to accomplish this
task. Initially, the supervised neural network is inputted by RGB multi-band images for roof identification. Next, spatial details are extracted through a hybrid approach of edge and region segmentation. Lastly, the extracted information is used to refine the results.
In [15] authors proposed a building extraction method. GIS data is used as an input in this method. A segmentation algorithm is used to extract the fea tures of the building. GIS data is used to provide prior building knowledge. Data pre-processing, Object seg mentation, and result post-processing are the three steps used in this method. Experimental results are also included in this to showcase the efficiency of the algorithms.
In [17] a two-step model method for tree detection is implemented including segmentation followed by classification is proposed here. The results presented show the effectiveness of the approach.
In [18] authors detected bridges in multispectral remote images through their developed model. The multi-seed supervised classification technique is used to classify the multispectral image into eight land-cov er types. A knowledge-based approach is used that find out the spatial arrangement of the bridge and its sur roundings. Testing is done on the IRS-1C/1-D satellite has a spatial resolution of 23.5m.
In [19] authors investigated ship detection in Ter raSAR-X (TSX) ScanSAR images (19-m resolution). Kol mogorov-Smirnov test is applied for the verification of the goodness of fit for the K-distribution to TSX images. A target detection algorithm is developed and also ver ified.
In [20] amorphous-shaped objects are detected by their developed model. The model is showing the re sults of experiments achieving high accuracy rates.
In [21] an automatic content-based analysis is pre sented to detect arbitrary objects in aerial imagery. In this, the two-stage training model using a convolution al neural network is implemented also verified over remote imagery. Model is tested for accuracy using UC Merced data set with an accuracy of 98.6%.
In [22] a deep CNN model is invented with en hanced functionality for feature extraction along with region classification and region proposal. Their method is based on ResNets that consists of multiple sub-networks (Object detection and Object proposal). To enhance feature map resolution, the output gener ated by multiple-scale layers is combined. VHR-10 da taset is used to train the model in the proposed work.
In [23] two algorithms are studied (Edgeboxes and Selective Search) for object detection. The evaluation is also performed on both algorithms using high-reso lution remote imagery. Algorithms are tested and eval uated through the NWPU-VHR-10 class data set. For performance measurement, execution time and recall rate are used as performance parameters. By the statis tical results, authors proved that EdgeBoxes algorithm is showing optimal results over Selective Search in re call rate.
In [25] a feature fusion method is proposed for extracting the fine-grained features from multiple layers of the remotely sensed image as those images have dense features concerning intra-class differenc
es, high inter-class similarities, and multidirectional objects. They have initially used ResNet50 for extract ing the features from multiple layers then the channel attention method is used to enhance the features. As a result, cross-layer bilinear pooling and feature con nection are used for fusion. After extracting the fea tures through ResNet50, squeezing-and- excitation (SE) method is used in its advanced version. Along with this, the traditional activation functions are replaced with the HandTan function which is simpler and more effective than traditional activation functions. The deep learning framework PyTorch is used to build the train ing model. The comparison is shown in their research with the existing models.
In [27] a branch regression framework is presented in a dual-mode based on remote image observations in detecting targets. This model can independently pre dict various variables and orientations effectively. To deal with multi-level features through spatial pooling, an advanced smart feature is added to the research. This is an example of an advanced, accurate model that is able in doing parallel operations of localization and classification.
In 2020, Yang et al. [28] suggested a novel cloud detection neural network with an encoder-decoder structure, called CDnetV2 as a sequence of work in the detection of Cloud. If Channel Attention Fusion Model (CAFM), Spatial Attention Fusion Model (SAFM), and Channel Attention Refinement Model (CARM). Pro posed HFFM is used to extract the linguistic high-level information from HLSIGFs. Here, some experimental results on the ZY-3 satellite thumbnail data set show that the proposed CDnetV2 is achieving exact and ac curate results and it is outperforming several state-ofthe-art methods.
In [29] authors proposed a novel approach to cope with such kinds of variant labels, i.e., class attention module and decomposition-fusion strategy. A class attention module is created to generate multiple class attention modules. Salient detection is proposed which
breaks down semantic segmentation into multi-class major detection and then combines them to produce a semantic map. Some experiments have been done on US3D Dataset. The imbalance label problem is also resolved with more accuracy than the previously avail able approach.
In [26] PTAN is developed including three-stage strategies for object detection in HD images. The model is achieving a mAP value of 0.7958. On the NWPU VHR10 dataset, PTAN is achieving a mAP value of 0.9187.
In [4] authors proposed an up-sampling, down-sam pling feature pyramid for obtaining the richer context information by bi-directionally involving shallow and deep features, and skipping connections. Experiments are done on DIOR, NWPUVHR-10, and on the self-as sembled datasets SDOTA, SDD to show the excellent performance of the proposed method by comparing it with other detectors. The proposed method is achiev ing 74.3% mAP on the public DIOR dataset.
In [1] authors proposed EFPN to detect small ob jects like plants, small buildings, etc. It is created to im prove feature extraction capabilities.
In [3] authors recommended a dynamic curriculum procedure that can learn the object detectors with the help of training images.
Table-5 is showing the major findings by thoroughly reviewing many articles and research papers on ob ject detection using deep learning and machine learn ing. From the past till now many researchers have presented their research work and ideas for feature extraction [7] and object detection including various kinds of objects in remotely sensed images [5] like buildings [13], trees, plants [1], roads, commercial ar eas, water, quality of soil, ships [4], oil slicks, bridges, industries, airports [9], shadow detection [10] and cloud detection [3].
NWPU
grained
Model is providing various accuracy rations in variant models. Need to create a hybrid model with more accuracy.
More
[49]
object relationship reasoning CNN (ORRCNN)
Aerial Image data set (AID), UC Merced Land-Use data set, and WHU-RS19 data set
[69]
Deep learning algorithms on NVIDIA DGX-1 supercomputer
[22]
[21]
[51]
[35]
[26]
Pre-trained dataset of SpaceNetfine-tuned on planet database.
An enhanced deep CNN based VHR-10 data set
Two-stage training model using convolutional neural network
accuracy for multiband data
Time Complexity & Efficiency
Need more prior information and parameters of the geometrical shape for template designing
For the training of CNN, a huge set of training data along with more computation powers is needed
substantial number of densely packed objects
UCMerced Dataset Arbitrary objects
Need to be more enhanced
Sometimes outliers are involved in predictions
AASM Open access satellite images Ability and Efficiency Sensitive to shape and viewpoint change
Convolutional capsule network
hierarchical bilinear pooling (HBP) with hierarchical attention and bilinear fusion net HABFNet
Open access Remote imagery fine grained features from multiple layers
1. UC Merced dataset released in 2010
2.AID dataset released in 2017
3.NWPU-RESISC45 dataset released in 2017
Accuracy with fine grained features
Unable in detection for robust dataset
Limit number images are used for the testing and training.
[68] RTANet Publicly available open dataset Speed
[24] optical remote sensing video (ORSV)
[23]
[27]
Selective Search and EdgeBoxes
compatibility loss clustering method (CLCM)
ORSV images motion-drive
Prediction accuracy is limited in number of images given for testing
Need to be changed in terms of methodological view
NWPU VHR-10 Involves high recall rate, faster Class imbalance issues occurring
Benchmark Technique: R-CNN Deep Learning
1. DOTA 2.UCAS-AOD 3.NWPU VHR-10 4.RSOD-Dataset
[59]
R-CNN algorithm with dialed convolution
[49] CFEM, A context-based feature enhancement module
[25]
[29]
PTAN (A patch-based threestage aggregation network)
Class attention module with multi-class segmentation network
HRSC2016 dataset
Accuracy & efficiencyMore layers can be added to enhance the accuracy of prediction.
Accuracy with respect to its feature extraction
ISPRS Vaihingen data setSpeed & Accuracy
Computationally expensive
Traditional Neural Network approach is using, need to be more enhance with respect to methodology
1.DOTA 2.NWPU VHR-10
US3D Dataset
Accuracy & efficiency Need to enhance the performance
Accuracy & efficiencyResults need to be improved on various parameters
Benchmark Technique: Machine Learning
[5]
[6]
Blackboard model Expert systems for image processing knowledge representationReal time detection is not possible
Multi Expert System for Scene Interpretation and Evaluation (MESSIE)
[7]
[8]
automated algorithm for feature extraction
A correlation mechanism for bi-temporal band pairs
Suburban images Class of an object from general structure
Images of Ottawa area are used through SAR imagery
Detection of homogeneous areas
Multi-temporal Landsat TM data water reflectance
Multiple spatial scales and aspect ratio
Limited data is used
Traditional approach is used
[9]
A multi-valued recognition system
IRS satellite Imagery
[10] CADCM HYDICE Hyperspectral digital imagery
[11]
[12]
[13]
[15]
[36]
Object space and image space-based matrices
An automatic recognition system
83 images of 18 sites
Multiple class selection
Hidden targets in hyperspectral images
Not showing more accuracy for multiple sub classes data
Only working for hidden targets, also including shadows
Performance evaluation Limited data is tested
Various open access databases minimizing the deleterious effects of cloud
digital surface model (DSM) Imagery of airborne light and ranging (LIDAR)
A segmentation algorithm GIS data
[16]
[17]
[18]
[19]
[20]
[55]
Not tested on a valid dataset
building footprint extraction Not able to fine-grained the results due to limited classes used
efficiency of the algorithms Need to enhance the performance
SE-MGMM synthetic aperture radar images change detection System is not obtaining a good accuracy for large amount of data or on live images.
Multistage model for road detection SPOT multispectral images of district near Hongqiao Airport improved detection probability
Model is showing multiple areas of Class imbalance
Two-step approach LIDAR aerial image Segmentation using weighted features multiple scales are needed to be applied
A model to detect bridges over water bodies
IRS-1C/1-D satellite images of 23.5.
Kolmogorov-Smirnov TerraSAR-X (TSX) ScanSAR images (19-m resolution)
neighborhood model hypothesis imagery and DIRSIG
An algorithm for building shadow detection panchromatic satellite imagery
The goal of object detection is to achieve the highest accuracy with efficiency by developing an automated robust detection algorithm. By critically reviewing the existing work done in this field it is analyzed that two major challenges still exists in finding or detec tion of objects in remote sensing images. We have di vided the found research gap in two categories, accu racy and efficiency. Accuracy can affect due to various reasons like class imbalance, captured image condi tion or environment, image noise, dual priorities, etc. Table 6 is showing the complete mapping of found re search gap or challenges with the reviewed literature in this paper. By critically reviewing many articles it is analyzed that there is still a gap exists in finding out the optimized solution in object detection in remote sensed images.
A Internal class imbalance. One of the major chal lenges that a model faces while dealing with real ob
multispectral imagery Dual priorities
Ship Detection
Various overfitting problems involved
Amorphously shaped objects Need to be more enhanced in terms of accuracy
Shadow detection More classes and sub-classes can be included
jects like shapes, sizes, colors, or directions of objects is class imbalance. This issue can occur due to the mentioned reasons a model could not be able to de tect the same objects having a different shape, color, or pose in multiple images [3][7][13][21][35][26].
B. Imagery conditions and unconstrained en vironments. Factors that include lighting, occlusion, weather conditions, viewpoint, object physical loca tion, shadow clutter, blur, motion, etc. [8] [11] [41] [22] [9].
C. Imaging noise. Imaging noise is one of the challenges in achieving high accuracy. Also factors like compression noise, low-resolution images, and filter distortions [47] [35] [17] [43] [20].
Low computational devices like mobile have low memory and less computational speed; it is a bottle neck in detecting objects with high efficiency [39].
Millions of unstructured and structured real-world existing object categories for distinguishing are
a challenge for the detector [19] [23] [36]. Some times image data especially remote images are hav ing a large size this became a challenging situation for object detectors. To find some unseen objects is also a challenge [44] [33].
Yao et al. [3] Ionescu et al. [7], Haithcoat et al. [13], Sevo et al. [21], Yu et al. [35], Guo et al. [26]
Coppin et al. [8], Shufelt et al. [11], Nagy et al. [41], Deng et al. [22], Mandal et al. [9]
Takarli et al. [47], Yu et al. [35], Secord et al. [17], Yang et al. [43], Grant et al. [20]
Anuta et al. [39], Paes et al. [19], Farooq et al. [23], Xue et al. [36], Tolluoglu et al. [44], Kumar et al. [33]
[1] Q. Yao, X. Hu and H. Lei, “Multiscale Convolu tional Neural Networks for Geospatial Object Detection in VHR Satellite Images”, IEEE Geo science and Remote Sensing Letters, vol. 18, no. 1, 2021, 23–27, 10.1109/LGRS.2020.2967819.
[2] G. Mountrakis, J. Im and C. Ogole, “Support vec tor machines in remote sensing: A review”, ISPRS Journal of Photogrammetry and Remote Sensing, vol. 66, no. 3, 2011, 247–259, 10.1016/j.isprsj prs.2010.11.001.
[3] X. Yao, X. Feng, J. Han, G. Cheng and L. Guo, “Au tomatic Weakly Supervised Object Detection From High Spatial Resolution Remote Sensing Images via Dynamic Curriculum Learning”, IEEE Transactions on Geoscience and Remote Sen sing, vol. 59, no. 1, 2021, 675–685, 10.1109/ TGRS.2020.2991407.
This paper is mainly focusing on a thorough, com prehensive, and chronological study of existing work done in the field of AI including machine learning and deep learning. This paper is performing the role of a pathway to all new researchers who are wishing to work in this field. This review paper is not only fo cusing on the published papers on object detection in remote imagery. It also involves the review of the papers those are having a modern approach of deep learning like CNN [2] [3] [4], R-CNN [6] [9] [11] [12] [15] and CornerNet [32] [35], etc. The paper is prov ing a complete outlook on existing ML and DL frame works, and the existing datasets used in object detec tion. Some problems and challenges in computing vision are also discussed here like crowd detection, color imbalance, live detection, etc. This paper is re viewing some most relatable topics like Ship detec tion, building detection, geospatial object detection, cloud detection, small-scale objects with the highest resolution in remote images. Some papers which in volve transfer learning concepts are also reviewed in this literature review.
Hina Hashmi* – CCSIT, Teerthanker Mahaveer Uni versity, Moradabad, India, e-mail: hinahashmi170@ gmail.com,
Rakesh Dwivedi – CCSIT, Teerthanker Mahaveer Uni versity, Moradabad, India,
Anil Kumar – Indian Institute of Remote Sensing, Dehradun, India.
* Corresponding author
[4] L. Li, Z. Zhou, B. Wang, L. Miao and H. Zong, “A No vel CNN-Based Method for Accurate Ship Detec tion in HR Optical Remote Sensing Images via Rotated Bounding Box”, IEEE Transactions on Geoscience and Remote Sensing, vol. 59, no. 1, 2021, 686–699, 10.1109/TGRS.2020.2995477.
[5] T. Matsuyama, “Knowledge-Based Aerial Image Understanding Systems and Expert Systems for Image Processing”, IEEE Transactions on Geo science and Remote Sensing, vol. GE-25, no. 3, 1987, 305–316, 10.1109/TGRS.1987.289802.
[6] P. Garnesson, G. Giraudon and P. Montesinos, “An image analysis, application for aerial ima gery interpretation”. In: Proc. 10th International Conference on Pattern Recognition, vol. 1, 1990, 210–212, 10.1109/ICPR.1990.118094.
[7] D. Ionescu and G. Geling, “Automatic detection of large object features from SAR data”. In: Proc. IGARSS ‘93 - IEEE International Geoscience and Remote Sensing Symposium, 1993, 1225–1227, 10.1109/IGARSS.1993.322663.
[8] P. R. Coppin and M. E. Bauer, “Processing of multitemporal Landsat TM imagery to opti mize extraction of forest cover change featu res”, IEEE Transactions on Geoscience and Re mote Sensing, vol. 32, no. 4, 1994, 918–927, 10.1109/36.298020.
[9] D. P. Mandal, C. A. Murthy and S. K. Pal, “Analysis of IRS imagery for detecting man-made objects with a multivalued recognition system”, IEEE Transactions on Systems, Man, and CyberneticsPart A: Systems and Humans, vol. 26, no. 2, 1996, 241–247, 10.1109/3468.485750.
[10] Hsuan Ren and Chein-I Chang, “A computer -aided detection and classification method for concealed targets in hyperspectral imagery”. In:
IGARSS ‘98. Sensing and Managing the Environ ment. 1998 IEEE International Geoscience and Remote Sensing. Symposium Proceedings. 1998, 1016–1018, 10.1109/IGARSS.1998.699658.
[11] J. A. Shufelt, “Performance evaluation and analy sis of monocular building extraction from aerial imagery”, IEEE Transactions on Pattern Analysis and Machine Intelligence, vol. 21, no. 4, 1999, 311–326, 10.1109/34.761262.
[12] J. G. Shanks and B. V. Shetler, “Confronting clo uds: detection, remediation and simulation ap proaches for hyperspectral remote sensing sys tems”. In: Proc. 29th Applied Imagery Pattern Recognition Workshop, 2000, 25–31, 10.1109/ AIPRW.2000.953599.
[13] T. L. Haithcoat, W. Song and J. D. Hipple, “Buil ding footprint extraction and 3-D reconstruc tion from LIDAR data”. In: IEEE/ISPRS Joint Workshop on Remote Sensing and Data Fusion over Urban Areas, 2001, 74–78, 10.1109/ DFUA.2001.985730.
[14] K. Chen and R. Blong, “Extracting building fe atures from high resolution aerial imagery for natural hazards risk assessment”. In: IEEE In ternational Geoscience and Remote Sensing Symposium, vol. 4, 2002, 2039–2041, 10.1109/ IGARSS.2002.1026437.
[15] J. Duan, V. Prinet and H. Lu, “Building extraction in urban areas from satellite images using GIS data as prior information”. In: Proc. IEEE Inter national Geoscience and Remote Sensing Sym posium, 2004. IGARSS ‘04., vol. 7, 2004, 4762–4764, 10.1109/IGARSS.2004.1370223.
[16] Yan Dongmei, Zhao Zhongming and Chen Zhong, “A fused road detection approach in high re solution multi-spectrum remote sensing ima gery”. In: Proc. 2005 IEEE International Geo science and Remote Sensing Symposium, 2005. IGARSS ‘05., vol. 3, 2005, 1557–1560, 10.1109/ IGARSS.2005.1526290.
[17] J. Secord and A. Zakhor, “Tree Detection in Urban Regions Using Aerial Lidar and Image Data”, IEEE Geoscience and Remote Sensing Letters, vol. 4, no. 2, 2007, 196–200, 10.1109/ LGRS.2006.888107.
[18] D. Chaudhuri and A. Samal, “An Automatic Brid ge Detection Technique for Multispectral Ima ges”, IEEE Transactions on Geoscience and Re mote Sensing, vol. 46, no. 9, 2008, 2720–2727, 10.1109/TGRS.2008.923631.
[19] R. L. Paes, J. A. Lorenzzetti and D. F. M. Gherar di, “Ship Detection Using TerraSAR-X Images in the Campos Basin (Brazil)”, IEEE Geoscience and
Remote Sensing Letters, vol. 7, no. 3, 2010, 545–548, 10.1109/LGRS.2010.2041322.
[20] C. S. Grant, T. K. Moon, J. H. Gunther, M. R. Stites and G. P. Williams, “Detection of Amorphously Shaped Objects Using Spatial Information Detec tion Enhancement (SIDE)”, IEEE Journal of Se lected Topics in Applied Earth Observations and Remote Sensing, vol. 5, no. 2, 2012, 478–487, 10.1109/JSTARS.2012.2186284.
[21] I. Sevo and A. Avramovic, “Convolutional Neu ral Network Based Automatic Object Detection on Aerial Images”, IEEE Geoscience and Remote Sensing Letters, vol. 13, no. 5, 2016, 740–744, 10.1109/LGRS.2016.2542358.
[22] Z. Deng, L. Lei, H. Sun, H. Zou, S. Zhou and J. Zhao, “An enhanced deep convolutional neural ne twork for densely packed objects detection in remote sensing images”. In: 2017 Internatio nal Workshop on Remote Sensing with Intel ligent Processing (RSIP), 2017, 1–4, 10.1109/ RSIP.2017.7958800.
[23] A. Farooq, J. Hu and X. Jia, “Efficient object pro posals extraction for target detection in VHR remote sensing images”. In: 2017 IEEE Inter national Geoscience and Remote Sensing Sym posium (IGARSS), 2017, 3337–3340, 10.1109/ IGARSS.2017.8127712.
[24] Y. Li, L. Jiao, X. Tang, X. Zhang, W. Zhang and L. Gao, “Weak Moving Object Detection In Opti cal Remote Sensing Video With Motion-Drive Fusion Network”. In: IGARSS 2019 - 2019 IEEE International Geoscience and Remote Sen sing Symposium, 2019, 5476–5479, 10.1109/ IGARSS.2019.8900412.
[25] B. Sui, M. Xu and F. Gao, “Patch-Based Three-Sta ge Aggregation Network for Object Detection in High Resolution Remote Sensing Images”, IEEE Access, vol. 8, 2020, 184934–184944, 10.1109/ ACCESS.2020.3027044.
[26] J. Guo, J. Yang, H. Yue, H. Tan, C. Hou and K. Li, “CDnetV2: CNN-Based Cloud Detection for Re mote Sensing Imagery With Cloud-Snow Coexi stence”, IEEE Transactions on Geoscience and Remote Sensing, vol. 59, no. 1, 2021, 700–713, 10.1109/TGRS.2020.2991398.
[27] Y. Han, S. Ma, Y. Xu, L. He, S. Li and M. Zhu, “Ef fective Complex Airport Object Detection in Re mote Sensing Images Based on Improved End -to-End Convolutional Neural Network”, IEEE Access, vol. 8, 2020, 172652–172663, 10.1109/ ACCESS.2020.3021895.
[28] J. Yang, J. Guo, H. Yue, Z. Liu, H. Hu and K. Li, “CDnet: CNN-Based Cloud Detection for Remo te Sensing Imagery”, IEEE Transactions on Geo
science and Remote Sensing, vol. 57, no. 8, 2019, 6195–6211, 10.1109/TGRS.2019.2904868.
[29] Z. Rao, M. He and Y. Dai, “Class Attention Network for Semantic Segmentation of Remote Sensing Images”, 2020 Asia-Pacific Signal and Informa tion Processing Association Annual Summit and Conference (APSIPA ASC), 2020, 150–155.
[30] P. Sharma, N. Ding, S. Goodman and R. Soricut, “Conceptual Captions: A Cleaned, Hypernymed, Image Alt-text Dataset for Automatic Image Cap tioning”. In: Proceedings of the 56th Annual Me eting of the Association for Computational Lingu istics (Volume 1: Long Papers), 2018, 2556–2565, 10.18653/v1/P18-1238.
[31] A. Sisodia, Swati and H. Hashmi, “Incorporation of Non-Fictional Applications in Wireless Sen sor Networks”, International Journal of Inno vative Technology and Exploring Engineering, vol. 9, no. 11, 2020, 42–49, 10.35940/ijitee. K7673.0991120.
[32] H. Hashmi, R. K. Dwivedi and A. Kumar, “Identifi cation of Objects using AI & ML Approaches: Sta te-of-the-Art”. In: 2021 10th International Con ference on System Modeling & Advancement in Research Trends (SMART), 2021, 1–5, 10.1109/ SMART52563.2021.9676273.
[33] A. Kumar, H. Hashmi, S. A. Khan and S. Kazim Naqvi, “SSE: A Smart Framework for Live Vi deo Streaming based Alerting System”. In: 2021 10th International Conference on Sys tem Modeling & Advancement in Research Trends (SMART), 2021, 193–197, 10.1109/ SMART52563.2021.9675306.
[34] S. Belongie, J. Malik and J. Puzicha, “Shape mat ching and object recognition using shape conte xts”, IEEE Transactions on Pattern Analysis and Machine Intelligence, vol. 24, no. 4, 2002, 509–522, 10.1109/34.993558.
[35] D. Yu, H. Guo, Q. Xu, J. Lu, C. Zhao and Y. Lin, “Hie rarchical Attention and Bilinear Fusion for Re mote Sensing Image Scene Classification”, IEEE Journal of Selected Topics in Applied Earth Ob servations and Remote Sensing, vol. 13, 2020, 6372–6383, 10.1109/JSTARS.2020.3030257.
[36] D. Xue, T. Lei, X. Jia, X. Wang, T. Chen and A. K. Nan di, “Unsupervised Change Detection Using Mul tiscale and Multiresolution Gaussian-Mixture -Model Guided by Saliency Enhancement”, IEEE Journal of Selected Topics in Applied Earth Ob servations and Remote Sensing, vol. 14, 2021, 1796–1809, 10.1109/JSTARS.2020.3046838.
[37] P. Peer and B. Batagelj, “Computer vision in con temporary art (introduction to the special ses
sion)”, 2008 50th International Symposium EL MAR, vol. 2, 2008, 471–474.
[38] R. M. Haralick and G. L. Kelly, “Pattern recognition with measurement space and spatial clustering for multiple images”, Proc. of the IEEE, vol. 57, no. 4, 1969, 654–665, 10.1109/PROC.1969.7020.
[39] P. E. Anuta, “Spatial Registration of Multispectral and Multitemporal Digital Imagery Using Fast Fourier Transform Techniques”, IEEE Trans actions on Geoscience Electronics, vol. 8, no. 4, 1970, 353–368, 10.1109/TGE.1970.271435.
[40] W. P. Waite and H. C. MacDonald, ““Vegeta tion Penetration” with K-Band Imaging Ra dars”, IEEE Transactions on Geoscience Elec tronics, vol. 9, no. 3, 1971, 147–155, 10.1109/ TGE.1971.271487.
[41] G. Nagy, “Digital image-processing activities in remote sensing for earth resources”, Proc. of the IEEE, vol. 60, no. 10, 1972, 1177–1200, 10.1109/ PROC.1972.8879.
[42] T. Roska, T. Boros, P. Thiran and L. O. Chua, “De tecting simple motion using cellular neural ne tworks”. In: IEEE International Workshop on Cellular Neural Networks and their Applications, 1990, 127–138, 10.1109/CNNA.1990.207516.
[43] Xiou-Ping Yang, Tao Yang and Lin-Bao Yang, “Extracting focused object from defocused background using cellular neural networks”. In: Proc. of the Third IEEE International Workshop on Cellular Neural Networks and Their Appli cations (CNNA-94), 1994, 451–455, 10.1109/ CNNA.1994.381633.
[44] O. Tolluoglu, O. M. Ucan, S. Kent and S. Kargin, “Edge detection in noise mounted picture”. In: Proc. of the IEEE 12th Signal Processing and Communications Applications Conference,, 2004, 335–338, 10.1109/SIU.2004.1338328.
[45] G. Grassi, E. Di Sciascio, A. L. Grieco and P. Vec chio, “A New Object-Oriented Segmentation Algorithm based on CNNs - Part I: Edge Extrac tion”. In: 2005 9th International Workshop on Cellular Neural Networks and Their Applications, 2005, 158–161, 10.1109/CNNA.2005.1543185.
[46] K. Slot, P. Korbel, M. Gozdzik and H. Kim, “Pat tern detection in spectrograms by means of Cellular Neural Networks”. In: 2006 10th Inter national Workshop on Cellular Neural Networks and Their Applications, 2006, 1–6, 10.1109/ CNNA.2006.341632.
[47] F. Takarli, A. Aghagolzadeh and H. Seyedarabi, “Robust pedestrian detection using low level and high level features”. In: 2013 21st Iranian Conference on Electrical Engineering (ICEE), 2013, 1–6, 10.1109/IranianCEE.2013.6599574.
[48] W. Thubsaeng, A. Kawewong and K. Patanukhom, “Vehicle logo detection using convolutional neu ral network and pyramid of histogram of orien ted gradients”. In: 2014 11th International Joint Conference on Computer Science and Software Engineering (JCSSE), 2014, 34–39, 10.1109/JCS SE.2014.6841838.
[49] Z. Li, Q. Wu, B. Cheng, L. Cao and H. Yang, “Re mote Sensing Image Scene Classification Based on Object Relationship Reasoning CNN”, IEEE Geoscience and Remote Sensing Letters, vol. 19, 2022, 1–5, 10.1109/LGRS.2020.3017542.
[50] Y. LeCun, L. Bottou, Y. Bengio and P. Haffner, “Gradient-Based Learning Applied to Document Recognition”, Proc. of the IEEE, vol. 86, 1998, 2278–2324, 10.1109/5.726791.
[51] A. Femin and K. S. Biju, “Accurate Detection of Buildings from Satellite Images using CNN”. In: 2020 International Conference on Elec trical, Communication, and Computer Engi neering (ICECCE), 2020, 1-5, 10.1109/ICEC CE49384.2020.9179232.
[52] D. Marmanis, J. D. Wegner, S. Galliani, K. Schin dler, M. Datcu and U. Stilla, “Semantic Segmen tation of Aerial Images With an Ensemble of CNNs”, ISPRS Annals of Photogrammetry, Re mote Sensing and Spatial Information Sciences, vol. III-3, 2016, 473–480, 10.5194/isprsannals -III-3-473-2016.
[53] L. Cheng, K. E. Trenberth, M. D. Palmer, J. Zhu and J. P. Abraham, “Observed and simulated full -depth ocean heat-content changes for 1970–2005”, Ocean Science, vol. 12, no. 4, 2016, 925–935, 10.5194/os-12-925-2016.
[54] N. Kussul, M. Lavreniuk, S. Skakun and A. She lestov, “Deep Learning Classification of Land Cover and Crop Types Using Remote Sensing Data”, IEEE Geoscience and Remote Sensing Letters, vol. 14, no. 5, 2017, 778–782, 10.1109/ LGRS.2017.2681128.
[55] M. I. Elbakary and K. M. Iftekharuddin, “Shadow Detection of Man-Made Buildings in High-Re solution Panchromatic Satellite Images”, IEEE Transactions on Geoscience and Remote Sen sing, vol. 52, no. 9, 2014, 5374–5386, 10.1109/ TGRS.2013.2288500.
[56] X. Zhang, S. Noda, R. Himeno and H. Liu, “Car diovascular disease-induced thermal responses during passive heat stress: an integrated com putational study: An integrated bioheat transfer model”, International Journal for Numerical Me thods in Biomedical Engineering, vol. 32, no. 11, 2016, 5374–5386, 10.1002/cnm.2768.
[57] L. Liu, W. Ouyang, X. Wang, P. Fieguth, J. Chen, X. Liu and M. Pietikäinen, “Deep Learning for Ge neric Object Detection: A Survey”, International Journal of Computer Vision, vol. 128, no. 2, 2020, 261–318, 10.1007/s11263-019-01247-4.
[58] W. S. McCulloch and W. Pitts, “A Logical Calculus of the Ideas Immanent in Nervous Activity”, The Bulletin of Mathematical Biophysics, vol. 5, no. 4, 1943, 115–133, 10.1007/BF02478259.
[59] Y.-C. Hsieh, C.-L. Chin, C.-S. Wei, I.-M. Chen, P.-Y. Yeh and R.-J. Tseng, “Combining VGG16, Mask R-CNN and Inception V3 to identify the benign and ma lignant of breast microcalcification clusters”. In: 2020 International Conference on Fuzzy Theory and Its Applications (iFUZZY), 2020, 2022–0104, 10.1109/iFUZZY50310.2020.9297809.
[60] G. E. Hinton, S. Osindero and Y.-W. Teh, “A Fast Learning Algorithm for Deep Belief Nets”, Neural Computation, vol. 18, no. 7, 2006, 1527–1554, 10.1162/neco.2006.18.7.1527.
[61] V. Dumoulin, I. Goodfellow, A. Courville and Y. Bengio, “On the Challenges of Physical Imple mentations of RBMs”, Proceedings of the AAAI Conference on Artificial Intelligence, vol. 28, no. 1, 2014, 10.1609/aaai.v28i1.8924.
[62]
O. Russakovsky, J. Deng, H. Su, J. Krause, S. Sa theesh, S. Ma, Z. Huang, A. Karpathy, A. Khosla, M. Bernstein, A. C. Berg and L. Fei-Fei, “ImageNet Large Scale Visual Recognition Challenge”, Inter national Journal of Computer Vision, vol. 115, no. 3, 2015, 211–252, 10.1007/s11263-0150816-y.
[63] G. M. Castelluccio and D. L. McDowell, “Micro structure and mesh sensitivities of mesoscale surrogate driving force measures for transgra nular fatigue cracks in polycrystals”, Materials Science and Engineering: A, vol. 639, 2015, 626–639, 10.1016/j.msea.2015.05.048.
[64] A. Krizhevsky, I. Sutskever and G. E. Hinton, “Ima geNet classification with deep convolutional neural networks”, Communications of the ACM, vol. 60, no. 6, 2017, 84–90, 10.1145/3065386.
[65] L. Tian and A. Noore, “Software Reliability Pre diction Using Recurrent Neural Network with Bayesian Regularization”, International Journal of Neural Systems, vol. 14, no. 3, 2004, 165–174, 10.1142/S0129065704001966.
[66] A. Benamira, B. Devillers, E. Lesot, A. K. Ray, M. Saadi and F. D. Malliaros, “Semi-Supervised Learning and Graph Neural Networks for Fake News Detection”. In: Proceedings of the 2019 IEEE/ACM International Conference on Advan ces in Social Networks Analysis and Mining, 2019, 568–569, 10.1145/3341161.3342958.
[67] X. Zhou, M. W. Koch and M. W. Roberts, “A Se lective Attention Neural Network for Invariant Recognition of Distorted Objects”. In: IJCNN -91-Seattle International Joint Conference on Neural Networks, vol. ii, 1991, 10.1109/ IJCNN.1991.155543.
[68] L. Fu, D. Zhang and Q. Ye, “Recurrent Thrifty At tention Network for Remote Sensing Scene Re cognition”, IEEE Transactions on Geoscience and Remote Sensing, vol. 59, no. 10, 2021, 8257–8268, 10.1109/TGRS.2020.3042507.
[69] R. Larionov, V. Khryashchev and V. Pavlov, “Se paration of Closely Located Buildings on Aerial Images Using U-Net Neural Network”. In: 2020 26th Conference of Open Innovations Asso ciation (FRUCT), 2020, 256–261, 10.23919/ FRUCT48808.2020.9087365.
Submitted: 19th August 2021; accepted: 8th February 2022
DOI: 10.14313/JAMRIS/4-2021/23
In the area of mobile robotics, trajectory planning is the task to find a sequence of primitive trajectories that con nect two configurations, whereas non-holonomic con straints, obstacles and driving costs have to be consid ered. In this paper, we present an approach that is able to handle situations that require changes of driving di rections. In such situations, optimal trajectory sequences contain costly turning maneuvers – sometimes not even on the direct path between start and target. These situ ations are difficult for most optimization approaches as the robot partly has to drive paths with higher cost values that seem to be disadvantageous. We discuss the problem in depth and provide a solution that is based on maneuvers, partial backdriving and free-place discovery. We applied the approach on top of our Viterbi-based tra jectory planner.
Keywords: Mobile Robots, Navigation, Trajectory Plan ning, Complex Turning Situations
Trajectory planning is a fundamental function of a mobile robot. When executing tasks such as trans porting items, the robot has to drive trajectories that meet certain measures of optimality. Corresponding cost functions consider driving time, energy con sumption, mechanical wear or buffer distance to ob stacles. A planning from the current pose to a target pose takes into account an obstacle map and creates a sequence of primitive movement commands such as driving arcs or straight, whereas the resulting se quence of trajectories minimizes the given cost func tion. Approaches that solve this problem often have two phases:
• A route planner tries to find a line string in the workspace with minimal costs that does not cut obstacles, with respect to the robot’s driving width,
• A trajectory planner in the configuration space takes the route points from the former phase, but also considers non-holonomic constraints such as minimal curve angles or driving orientations. For route planning, there exist a variety of efficient solutions, most base on A*, where the workspace may be modelled by, e.g., grids or visibility graphs. The two-phase planning makes the problem manageable even for complex or large-scale environments as the
pure workspace planning only operates on few di mensions. The second phase is much harder, because non-holonomic constraints make it difficult to use straight-forward optimization techniques. Note that other approaches, such as probabilistic path planning may compute a solution in a single phase. We discuss this in the next section.
Even though effective for many situations, some times the two-phase approach fails. This is because the workspace planning sometimes suggests a route that cannot adequately be mapped into the configu ration space. In this paper, we consider robots that are not able to directly turn in place. If, e.g., a car-like robot has to change its driving direction (fore to back wards or vice versa), turning maneuvers are required. If starts or targets are in a narrow area, we have to plan trajectories where the locations of turns are not obvious. E.g., think of a charging station that requires the robot to drive backwards into a parking bay.
Fig. 1 illustrates such a situation: the robot should only slightly move, but should turn by 180°. A pure workspace planning that ignores the orientation com putes a short direct route. However, if the hallway is too narrow, the robot first has to drive a costly route out side the narrow area to perform a turning maneuver.
Typical planning approaches have difficulties to find such paths. These situations can be found in everyday’s life, e.g., when we want to park a car in a narrow parking bay or when we want to reverse the driving direction but have to consider constraints giv en by the road geometry.
This paper proposes an approach to efficiently compute paths with up two turning maneuvers. Note that for typical robots, a maximum of two changes of driving directions are sufficient. The key idea: we
Start Targetsuggest an iterative planning that tries to optimize a sequence of maneuvers. As we keep multiple inter mediate maneuvers per iteration, required turning maneuvers with higher local costs are also consid ered. To deal with paths that have to be extended to a position away from the direct connection (such as in Fig. 1), we suggest an extension of A* to find ap propriate free places. We finally model all algorithmic components by a multi-strategy planning that pro vides a single software interface for planning tasks, meanwhile automatically applies the least complex approach for a specific scenario.
Early work investigated shortest paths for vehicles that are able to drive straight forward and circular curves [3, 5, 17] or integrated additional primitive trajectories [2, 22]. Even though a combination of such primitive trajectories may appear as turning ma neuvers, turning situations are not explicitly consid ered. Moreover, only path lengths, but not the costs for driving direction changes were examined.
Further work looked at longer paths that go through an environment of obstacles. As the variation space of possible trajectory sequences gets very large, probabilistic approaches were suitable to find at least a suboptimal solution [10, 12, 13]. They randomly connect configurations by primitive trajectories, cre ate a connection graph, then find an actual path. Some work also used potential fields [1] or visibility graphs [15]. With the help of geometric route planners, the overall problem of trajectory planning can be reduced. In [11], the route planning step and a local trajectory planning step were recursively applied.
[16] introduced the state lattice idea, which is a discrete graph embedded into the continuous state space. Vertices represent states that reside on a hyper dimensional grid, whereas edges join states by trajec tories that satisfy the robot’s motion constraints. The original approach was based on equivalence classes for all trajectories that connect two states and per formed inverse trajectory generation to compute the result trajectory. [7] introduced a two-step approach, with coarse planning of states based on Dynamic Pro gramming, and a fine trajectory planning that con nected the formerly generated states.
Random sampling can also be used to improve gen erated trajectories. E.g., CHOMP [27] used functional gradient techniques based on Hamiltonian Monte Carlo to iteratively improve the quality of an initial trajectory. The approach in [14] represented the continuous-time trajectory as a sample from a Gaussian process gen erated by a linear time-varying stochastic differential equation. Then gradient-based optimization technique optimized trajectories with respect to a cost function.
Special planning situations such as driving in the parking bay may explicitly be integrated into the plan ning component. Approaches such as [25, 26] were suitable in the area of autonomous driving, where tasks such as parking cars often occur. For this, the planning components identify the respective pattern
and integrate a pre-defined movement. It is difficult to extend such an approach to arbitrary situations.
Probabilistic path planning based on RRT [12] created numerous random configurations and tried to connect them to a tree of valid trajectories. Ad vanced variations such as RRT* [9] introduced the property of asymptotic optimality – if we spend more runtime, the solutions get better and converge to op timal paths. This means, probabilistic approaches are in principle suitable situations that require changes of driving directions. However, they have to face two problems: first, the integration of new configurations into a tree of configurations only considers local op timality. The respective connections try to optimize the costs for the small part of the tree that covers the closer area of the new configuration. It is not probable that costly turning maneuvers or driving to free plac es are thus considered in the first place. As a result, even though an approach is asymptotically optimal, adequate routes are considered very late. The second problem: in a small scale, we still have to introduce turning maneuvers to connect new configurations. In RRT or RRT*, this problem was abstracted away by procedures called steer or rewire, however must be actually implemented to also work for complex turn ing situations.
Our goal is to create a deterministic planning ap proach based on a two-phase planning [19].
We start with the architecture of the motion plan ning and execution (Fig. 2). The Navigation compo nent provides a point-to-point route planning in the workspace. The Trajectory Planning computes a driv able sequence of trajectories between configurations and considers non-holonomic constraints. The Eval uator computes costs of paths based on the obstacle map and the desired cost properties – a certain robot application may plug-in its own Evaluator into the system. Cost values may take into account the path length, expected energy consumption or the amount backwards driving. Also, the distance to obstacles could be considered, if, e.g., we want the robot to keep a safety distance where possible.
The lower components are not focus of this paper: The Trajectory Regulation permanently tries to hold the planned trajectories, even if the position drifts off due to slippage. Simultaneous Localization and Map ping (SLAM) constantly observes the environment and computes the most probable own position and location of obstacles by motion feedback and sensors (e.g., Lidar or camera). The current error-corrected configuration is passed to all planning components. Observed and error-corrected obstacle positions are stored in the Obstacle Map. The Motion System finally is able to execute and supervise driving commands.
We assume the robot drives in the plane in a work space W with positions (x, y). The configuration space C covers an additional dimension for the orienta tion angle, i.e., a certain configuration is defined by (x, y, θ). The goal is to find a collision-free sequence of trajectories that connects two configurations, mean while minimizes the costs.
This problem has many degrees of freedom. Every two positions can be connected by an infinite number of trajectories and the problem gets worse for larger environments with obstacles. We thus introduce the following concepts:
• A route planner that solely operates on workspace W computes a sequence of collision-free lines of sight (with respect to the robot’s width) that minimize the costs.
• As the route planning only computes route points in W, we have to specify additional variables in C (here orientation θ). From the infinite assignments, we only consider a small finite set.
• From the possible set of trajectories between two route points, we only consider a small set of maneuvers. Maneuvers are trajectories, for which we know formulas that derive the respective geometric parameters.
• Even though these concepts reduce the problem space to a finite set of variations, this set is by far too large for complete checks. We thus apply a Viterbi-like approach that significantly reduces the number of checked variations. We carefully separated the cost function from all planning components. We assume, we are able to as sign costs to any route or trajectory sequence accord ing to two rules: First, we must be able to define a to tal order on costs. This is required, as we iteratively compare routes or trajectory sequences and identify the ‘best’ one from a set of candidates. Second, a colli sion with obstacles has to result in infinite costs.
The basic movement capabilities of a robot are de fined by a set of primitive trajectories. The respective set can vary between different robots. E.g., the Carbot [20] is able to execute the following primitive trajec tories:
• L(): linear (straight) driving over a distance of (that may be negative for backward);
• A(, r): drive a circular arc with radius r (sign distinguishes left/right) over a distance of (that
may be negative for backward);
• C(, κs, κt): clothoid over a distance of with given start and target curvatures.
We are able to map primitive trajectories directly to driving commands that are natively executed by the robot’s motion subsystem.
Implicitly, primitive trajectories specify functions that map configurations cs to ct. Due to non-holonomic constraints, for given cs, ct∈C there is usually no prim itive trajectory that maps cs to ct. At this point, we in troduce maneuvers. Maneuvers are small sequences of primitive trajectories (usually 2 to 5 elements) that are able to map given cs, ct∈C. More specifically:
• A maneuver is defined by a sequence of primitive trajectories (e.g., denoted ALA or AA) and further constraints. Constraints may relate or restrict geometric parameters.
• For given cs, ct∈C there exist formulas that specify the geometric parameters of the involved primitive trajectories, e.g., for L, A and C, r for A, κs, κt for C.
• Sometimes, the respective equations are underdetermined. As a result, multiple maneuvers of a certain type (sometimes an infinite number) map cs to ct. Thus, we need further parameters, we call free parameters to get a unique maneuver.
Until now, we identified about thirty maneuvers of which Fig. 3 shows six. We assigned names that illustrate the maneuvers’ shape, e.g., the J-Arc drives a path that looks like the letter ‘J’. The Dubins-Arcs correspond to the combination of three arcs of Dubins original approach [3].
Let Π denote the set of maneuver types relevant for a certain scenario or application. Note that Π can eas ily be extended or reduced to reflect to robot’s driv ing capabilities. E.g., a certain robot may not support clothoids. In this case, we could remove all maneuvers that contain a C primitive from Π
Let p1=(x1, y1)…pn=(xn, yn) denote a route found by the route planning component for a start (p1, θ1) and target (pn, θn). Our problem is to find a sequence of
maneuvers that connects start, target and all route points p2,... p
in-between.
Of the infinite number of intermediate orienta tions and maneuver parameters we define a finite set of promising candidates. This obviously leads to sub-optimal results. In reality, however, it does not significantly affect the overall costs. Let Oi denote all orientation candidates for a route point i≥2. We sug gest variations of angles from the previous and to the next route point (Fig. 4).
For a new route step pi+1, we again have to check multiple orientation angles of Oi+1. For this, we try to extend all trajectory sequences in S by maneuvers. I.e., we compute all possible maneuver types in Π with possible parameters to get to (pi+1, θi+1,j). We store the optimal trajectory sequences to each of the orienta tion angles in a new list S’ that forms the S for the next iteration.
In the last step we have On={θn}, i.e., we only have to check the single target angle. Thus, if there is at least one trajectory sequence found, we get |S|=1 and the optimal sequence is the single element of S.
For free parameters we distinguish the small set of variations for arc senses (e.g., two for J-Bow) and the infinite set for arc radii (e.g., for Wing-Arc). For the first type we are able to iterate through all vari ations. For the second type, we select a small set of candidates, similar to orientation angles. We suggest {
We want to extend the current two-phase approach to also support situations that require changes of driving directions. The maneuver-based trajec tory planning is a solid foundation to integrate new mechanisms for required paths. Our approach can be summarized as follows:
• We integrate new turning maneuvers, required to change the driving direction.
• We extend the Viterbi-based optimization to find one or two backdriving sequences, if required.
min}, where
min is the minimal curve ra dius. Let params(M) denote the set of parameters for a maneuver type M∈Π. For maneuver types with no free parameters, we define params(M)={∅}, whereas ∅ is an empty parameter setting.
Even though we now have a finite set of variations Π × Oi × params(M) for a single route step, the total number still is too large for a complete check. To give an impression: for 5 route points we get a total num ber of 20 million, for 20 route points 2⋅1037, for an average of 20 maneuvers and 5 intermediate angles. Obviously, we need an approach that computes a re sult without iterating through all permutations.
Our approach [19, 21] is inspired by the Viterbi algorithm [23] that tries to find the most likely path through hidden states. Our adaption looks for a se quence of maneuvers/orientations/free parameters that connects them with minimal costs.
A Viterbi-like approach is suitable, because opti mal paths have a characteristic: the interference be tween two primitive trajectories in that path depends on their distance. If they are close, a change of one usually also causes a change of the other. This is be cause a certain maneuver influences its end-orienta tion and thus the start-orientation of the next maneu ver. If they are far, we may change one trajectory of the sequence, without affecting the other. Viterbi reflects this characteristic, as it checks all combinations of neighbouring maneuvers to get the optimum.
Fig. 5 illustrates the idea. Starting with (p1, θ1) it iteratively finds optimal maneuvers to (pi, θij). For the multiple intermediate angles Oi={θi1, θi2, …}, we keep optimal trajectory sequences to each of these angles in a list S. Because the number of trajectories in S only depends on the last route point (and in particular not on the route before), the runtime and memory usage is of O(n).
• We integrate a mechanism to find free places for turning maneuvers, when start and target are only connected by narrow paths.
• The new mechanisms are integrated by a software structure that supports multi-strategy planning. Note that, whenever backdriving is required, a max imum of two backdriving sequences is sufficient for op timal paths. We come back to this point in section 4.2.
The trajectory planner prefers forward driving as long as possible, if the cost function indicates this. Sensors that map the environment or prevent collisions often point in driving direction. Thus, it is reasonable to re ward forward driving. A change of driving direction requires stopping the motors that interrupts a smooth driving. A typical cost function penalizes a switch of forward to backwards driving and vice versa.
However, the change of driving directions is not always avoidable. As a first goal, we have to introduce new maneuvers that change between driving forward and backwards and vice versa. In daily life we are fa miliar with parking or turning maneuvers in the con text of car driving that have a similar objective. For our planning approach, we identified three turning maneuvers (Fig. 6):
• One-Bow-Turn (LAL): an arc (usually with a larger turning angle) is embedded into two linear trajectories. The change of driving direction is at start or end of the arc.
• Two-Bow-Turn (AAL): two arcs with turning angle nearly 90° are connected by a change of driving direction. The linear trajectory is required to reach any target position.
Start Start
• Parking (LAA): After a linear trajectory we have a change of drive direction. Two arcs (left/right or right/left) are then driven to reach the target position.
All these maneuvers have the single arc radius or the two arc radii as free parameters. For Two-BowTurn and Parking we require the same radius for both arcs to get a unique maneuver. Fig. 7 illustrates the constructions. We require the following geometric computations:
• (A) Shift the straight line through the robot’s pose by r to left or right.
• (B) Creation of a circle (left or right) with radius r that touches the robot’s position and has the robot’s orientation as tangent.
• (C) Intersection of two straight lines.
• (D) Intersection of straight line and circle. For the Bow-Turn we apply projections (A) to start and target pose. The intersection of these (C) creates the arc centre. Note that of the four variations (start or target, left or right) of the (A) projections, two do
not change the driving direction. Of the remaining two, we chose the one with least costs.
For the Two-Bow-Turn we create the start arc using (B). We then intersect (D) a circle with radius 2r and the arc centre with the target straight line projection (A). The intersection is the second arc’s centre. For Parking we apply the reverse construction: the start straight line projection (A) is intersected (D) with the target circle (B).
One Bow Turn r (A) (B) (C) (D)
For Two-Bow-Turn and Parking we have four varia tions: left/right of straight line projection and left/ right of the created start or target circle. Of these, two do not change the driving direction in the required manner. Of the remaining two, we chose the one with least costs. Of course, all constructions (A) to (D) have to be mapped to formulas; fortunately, all have closed solu tions (linear or quadratic).
The next question is how to integrate the turning ma neuvers into paths. In the following, we assume that forward driving is preferred over driving backwards. We further assume, if a robot is able to drive forward though an environment, it may also be able to drive backwards on the same trajectory. This means, the robot’s geometry (e.g., concerning clearance width) is not different for the two driving directions. This is true for most robots.
Fig. 8a) shows, how turning maneuvers can be in tegrated into a trajectory sequence for different cases of start and target directions.
• The trajectory sequence can entirely be driven forward.
• The trajectory sequence can entirely be driven backwards – this may be a result of short route or no place for a turning maneuver.
• The trajectory sequence contains a single turning maneuver. This happens, if start and target can
One Bow Turn Start Two Bow Turn Parking Linear Arc fore back fore back Start Start Start Start Target Two Bow Turn Parking Startonly be accessed by a certain driving direction, and this is different for start and target.
• The trajectory sequence contains two turning maneuvers. This happens, if the start can only be left and target can only be reached by backwards driving. Moreover, there may be place for two turning maneuvers and the costs of forward driving pays off.
On the first glance, there may be more than two turning maneuvers. However, as a consequence of our assumptions, we get a maximum two: if we had three or more, we can always remove a sequence with an even number that start with driving backwards (Fig. 8b).
The most complex situation is presented in Fig. 9. Here, we want to switch to forward driving as soon as possible, i.e., as far as there is a place for the turning maneuver. In addition, we want to switch to backdriv ing as late as possible.
Our approach of Viterbi-optimization is able to create the respective trajectory sequence, if we add one modification: in addition to the orientation candi dates (Fig. 4) we consider the reverse angles (i.e., the angles plus 180°). This modification creates sufficient candidates to integrate one or two turning maneu vers, if required.
We have to justify, why this modification in rela tion with the Viterbi approach creates the turning maneuvers at optimal places. Remember that the Viterbi approach iteratively goes through the work space positions, meanwhile keeps optimal trajectory sequences to all configurations at the last considered position. I.e., at each last point, we know how to reach it in forward and backwards orientation (and further graded angles in-between). We have to consider two effects: 1) the switch to forward driving (if it is re quired in the sequence) is as early as possible, and 2)
1) This effect occurs, if the environment prohibits forward driving when leaving the starting area. This means, forward driving configurations are considered in one step, but no single maneuver is able to reach it, as long as the robot is in the narrow area. But as soon as possible, the respective turning maneuver is taken into account. In the following iterations, both driving directions are expanded simultaneously, however at a certain point, forward driving causes least costs.
2) Shortly before entering a narrow target area, multiple orientations are considered, but even backdriving orientations may be reached with least costs, if all orientations before were forward orientations. When entering a narrow area, both driving directions still are considered simultaneously. However, if the final target can only be an extension of the backdriving variation, the last possible position for changing the direction is planned.
As we can see here, we still are able to create the trajectory sequence step by step in a greedy manner without loosing optimality. As a last point, we have to discuss a more technical problem. Usually, the naviga tion tries to produce as few as possible route points,
a) Cases for turning maneuvers b) Replacement of multiple turning maneuvers the switch to backwards driving (if it is required in the sequence) is as late as possible. Route Line String Final Trajectory Sequence Configuration Space Alternatives Viterbi Optimiziation Viterbi Optimiziation Viterbi Optimiziation Start Fig. 9. Planning of Backdriving with Viterbi-Optimization Start Target Startas the number influences the computation speed of the second planning phase. This may cause large distances between route points, if, e.g., the robot is able to drive straight in a hallway. The problem: the change of forward and backwards driving can only be planned at a route point computed by the navigation component. Thus, on large route sections, the planner often does not have a chance to identify an appropri ate position. To solve this, we have to artificially inte grate route points, if a distance exceeds a certain lim it. For this, we can simply cut a large section linearly.
The approach until now is able to plan trajectory sequences with partial backwards driving on direct routes. However, there exist scenarios where the workspace route cannot be extended to the configu ration space (Fig. 10).
This problem occurs, when a turning maneuver is required, but no route point offers enough space for its integration. This may require arbitrary long de tours far away from the direct route: imagine a maze where the robot first has to drive outside to find an appropriate place for a turning maneuver.
An observation: the maximum of turning maneu vers outside the workspace route is one. This is be cause the situation only occurs, when both start and target are inside the same narrow area and a single turn outside this area is sufficient. Thus, we can formu late a solution as follows: find a turning position that • offers sufficient space for a turning maneuver and • generates minimal route costs for the sum of start to turning position and turning position to target. Here, we do not want to iterate through all places, meanwhile calling the route planning two times. Our approach is based on the idea presented in [18] and extends the A* route planning approach [8].
A* Point to Point Planning. To understand our approach, we first have to briefly describe the tradi tional route planning based on A*. First, we have to map the environment on a graph with nodes qi. This requires a kind of discretization. There exist several methods to do this, e.g., using a grid [4], Voronoi re gions [6] or visibility graphs [24].
In a second step we have to assign cost values to edges between connected nodes, denoted c(qi, qj).
We furthermore have to provide a lower cost limit estimation between two nodes, denoted h(qi, qj). Let further c*(qi, qj) denote the costs of the optimal route. Our goal is now to compute c*(start, target) and the respective sequence of nodes.
The actual route computation by A* iteratively assigns three ‘states’ to the node: not_visited means, there is no knowledge how to reach it from the start; open means, we know at least one route from the start; closed means: we are sure how the reach a node from the start by an optimal route. Obviously, we ter minate, when the target has been closed. To control the state changes, we need an array g[qi] that is
• ∞, if qi is not_visited,
• c*(start, qi), if qi is closed,
• not less than c*(start, qi), if qi is open
Let further denote f[qi]=g[qi]+h(qi, target). A* is based on a key observation: for the qi with the lowest f[qi], we get g[qi]=c*(start, qi). As a result, we can as sign the closed state to this node. In addition, we iter ate through all neighbours qj of qi and check, if qj can be reached with least costs going over qi. We further assign the open state to all non-closed neighbours of qi. Finally, every node gets a backlink entry that points to the neighbour over which a node is accessed on an optimal route from the start.
To quickly find the qi with lowest f[qi], we need an efficient structure, e.g., the priority queue, that is based on types of ordering the open nodes.
Routes Through Free Places. We now want to route over a free place, sufficiently large to enable a turning maneuver. More formally, we search a free place node I that minimizes
As an additional property, the resulting costs must be below a certain cost limit, relative to the direct path. This is useful as we may consider any path that re quires too much driving costs as failure and report this to the application. The developer thus defines a factor v and we only consider I with
c*(start, I)+c*(I, target) ≤ v opt (2)
where opt=c*(start, target) denotes the optimal route costs. This means, the set Φ of all nodes that are can didates as free place is
Φ(start, target, v) = {I | c*(start, I)+c*(I, target) ≤ v⋅opt} (3)
The remaining section describes, how we efficiently compute Φ and thus implicitly the routes from start to I and from I to target. A first observation: from c*(start, I)+c*(I, target) ≤ v opt (4) and f[qi]=g[qi]+h(qi, target)=c*(start, qi)+h(qi, target) ≤c*(start, qi)+c*(qi, target) (5)
which is true, because h(qi, target)≤c*(qi, target), fol lows f[qi]≤v⋅opt (6)
As the f-value of the next node in the open list cannot get smaller, we can stop, when we poll a node with an f-value larger than v opt. Because we do not know opt from the beginning, we first expand nodes (accord ing to A* [8]) until we polled the target node. Then, we visit more nodes, as far as we get the first node with f>v opt. As a consequence, the field goes beyond the target. This is reasonable, as those nodes are also candidates for I. Algorithm 1 sums up these consider ations and shows, how to compute the start field. To
clearly distinguish the respective structures, we apply an index s to all start field arrays.
In a second round, we have to generate a target field with index t (Algorithm 2). The approach is simi lar to Algorithm 1, but in order to apply the appropri ate ordering of route nodes, we have to incorporate these changes:
• The first open node is the target
• Whenever we expand a node qi, we check the distance from the neighbour qj, i.e., c(qj, qi,), not to the neighbour.
• The estimation h is computed from start to the respective node.
• The sequence of backlink entries points to the target not to the start
In addition, we can directly use the opt value of the start field. Moreover, the target field generation can benefit from a much better estimation h compared to the start field (see (*) in Algorithm 2): we set h=gs[qj] whenever gs[qj]≥0. This does not change the result, but significantly reduces the number of visited nodes for the target field, thus significantly improves the runtime. Using the gs for h does not only provide an estimation, but returns the real costs, thus is the best considerable estimation.
The start and target field generation produce gs[qi] =c*(start, qi) for all qi with states[qi]=closed and gt[qi]= c*(qi, target) for all qi with statet[qi]=closed. As a consequence, we are now able to provide an effi cient formula for Φ:
Φ(start, target, v) = {qi | states[qi]=statet[qi]=closed and gs[qi]+gt[qi] ≤ v opt} (7)
This approach is by far more efficient than an ap proach that iteratively computes two optimal routes for every node I in the graph.
}
fnew
if states[qj]=not_visited
In order to find an appropriate turning place, we need to evaluate the property of ‘free space’ for a node. As the trajectory planning is an additional step, we do not know the actual required space to drive a turning maneuver. However, we can find an appropriate simplification that can be evaluated in workspace. E.g., we can estimate the clearance space required by a turning maneuver through a node us ing simulations. We furthermore are able to decide, whether a node has sufficient distance to the closest obstacle. This is required anyway to create an initial graph, as a node represents a single point in work space, but the robot has a certain geometric extent. To sum up, we are able to efficiently decide, if a certain node has sufficient distance for a turning maneuver. Let free(qj) denote this property. We now can put all together. We compute
Iopt = arg min (gs[I]+gt[I]) I∈ Φ,free(I) (8)
For this, we first compute Φ with formula (7) sort ed by gs[qi]+gt[qi]. We then iterate though Φ starting with the smallest value. For each we test free(qj) until we get a hit.
Until now, we considered different approaches to get from start to target, namely
• workspace route planning: with or without routing over free places, assigning different values for v • trajectory planning: with or without reverse angle candidates, different sets of maneuvers, with or without turning maneuvers.
This produces a large set of possible settings to com pute a trajectory sequence. Because more complex approaches such as finding a free place request more
fnew
fs[
gs[
start
is less costly backlinks[
than the formerly stored route states[qj]
open; openList.add(qj
// if already added, update f
} while not openList.isEmpty(); return failure; // no route at all from start to target
) gt[target]
target_field(start, target,
0; ft[target]
0; statet[target]
open; openList.add(target); // add target to open for all qi
target { // initialize fields gt[qi
1; ft[
0; statet[qi
not_visited; }
do { // Note: opt is known from start field qi
openList.poll(); // get open qi with minimal ft[qi] if ft[qi]>
opt // this cannot be an I return success; // going over I is too costly: finish statet[qi
closed; for all neighbours qj of
if statet[qj
// Note: driving direction is qj
// expand node gnew
if gs
else
closed
Note: costs from qj
(*) estimation start to
available
the real costs, from start field,
start
if statet[
not available: compute
yourself fnew
not_visited
Note:
fnew
estimates start
target is less costly backlinkt
gt
than the formerly stored route statet[
open
openList.add(
// if already added, update f
} while not openList.isEmpty(); return failure; // no route at all from start to target
First, we consider a pair of workspace route planning and trajectory planning as a single planner component. This takes start and tar
runtime, we do not want to start with such an ap proach. In real scenarios, most of the planning tasks are still of a simple type. Thus, we should not spend too much planning time for these.
We want to support developers of corresponding applications to express planning tasks. A developer could define a list of rules, e.g.:
• If a planning without free places and without reverse angle candidates is successful, take the respective result.
• If not, consider reverse angle candidates.
• If this still is not successful, additionally integrate turning maneuvers.
• If this still is not successful, try the route planning through a free place in combination with reverse angle candidates and turning maneuvers.
We get even more variations, if we deal with different route planning approaches (e.g., grid, Voronoi regions or visibility graphs), different safety distances to ob stacles, or different candidates for desired curve radii.
To gain control over the multitude of possibilities, we suggest a software architecture that allows the devel oper to express rules to combine them. Our approach is based on two key ideas:
• First, we consider a pair of workspace route planning and trajectory planning as a single planner component. This takes start and target configurations (Fig. 11 left).
• Second, we hierarchically build new planning components that internally contain own planners, but their interface still appears as a single planner (Fig. 11 right).
Encapsulating the two planning phases by a single component allows us even to integrate alternative ap proaches for single-step planning such as probabilis tic trajectory planning [9, 12].
A developer may make a choice based on available computational resources and runtime restrictions. The best-costs component requires to run all inner planning components, but this can be parallelized, if the runtime system supports it. The first-success component safes computational power, if there is a high probability for successful inner planning com ponents that are checked first. However, the final re sult may be sub-optimal.
We implemented the approach on our Carbot robot (Fig. 12). It has a size of 35 cm × 40 cm × 27 cm and a weight of 4.9 kg. It is able to run with a speed of 31 cm/s. The wheel configuration allows to indepen dently steer two wheels. For arcs, the different num bers of revolutions of the powered front wheels as well as the steering angles of the steered rear wheels are adapted to follow the respective curve geometry.
A rotating Lidar device (Slamtec RPLidar A2M4) on top is used for world modelling. It scans the 360° degrees in 0.9° steps, i.e., produces 400 distance points per rotation. The sample frequency is 4000 measurements per seconds, i.e., 100 ms for every scan of 360°. The range is 0.15 to 6.00 m with a reso lution of smaller than 0.5 mm.
The hierarchical components are not restricted to two levels – there can be any deep nesting. A Selec tor controls, which inner planning components may be executed and how multiple inner results are com bined to a single result. Until now, we developed two hierarchical components:
• Best-costs: all inner components execute the planning tasks and the inner result with best costs is returned as the component’s total result.
• First-success: the inner planning components are executed one after the other in a given order and the first successful planning is returned as the component’s total result.
We run the experiments in our simulation envi ronment that simulates the robot on hardware-level [20]. It is very close to the real robot. E.g., the same binary code runs on the real robot and simulator. Mo tors and sensors are simulated on low levels. E.g., the simulated and real motors have the same I2C com mand interface. Typical sensor errors and physical effects such as slippage can be applied.
We used the simulator to create situations where complex turning situations occur. Without the simu lator it would be very difficult to create environments that systematically challenge the mechanisms pre sented above.
Fig. 13 shows characteristic environments. Situ ation a) is the most simple: as the required turning maneuver can be applied at the penultimate route section, the Viterbi approach checks a change of driv ing direction without any further mechanisms.
Situations b) and c) are more complex: a Viter bi-optimization that only considers forward driving would fail, as we need to drive backwards for a longer time to get into the parking position. Thus, we addi tionally require the reverse angle candidates. Note that for the entire driving in the narrow hallway, for ward as well as backwards driving is considered as suitable direction. Only at the end, the forward driv ing candidate (and thus the corresponding trajectory sequence in the hallway) is removed and solely the presented sequences in the figure remain.
In situation d) it pays off to integrate two changes of directions. This is because, we have two places that support the turning maneuvers and their distance is large enough. Note that sequences with two turning maneuvers are only planned, if the cost function pe nalizes backwards driving, otherwise entirely driving backwards would be a more suitable solution.
Situations e) and f) require free-place turnings. In e), as a first try, the direct (workspace) connection is preferred over the large route around the mid square. However, any trajectory planning based on the direct connection failed, as not any turning maneuver can be
integrated. Our multi-strategy planning then tries the free-place planning. The nearest free place is the area on the left. The corresponding two routes, one from start to free place and one from free place to target now can easily be planned. Situation f) is the same as situation c) apart from the start position. In f) the ro bot first has to fully drive outside the long hallway to integrate the turning maneuver. This situation should illustrate that in worst case, a free turning place can be far away from start and target.
In these situations, the robot already knows all involved obstacles. Either the robot has investigated the map before or an obstacle map was given. In many scenarios, however, the robot has to find a path even though the map is unknown or only partly known. In such scenarios, we have two options: either the robot first has to explore the environment or the robot ap plies an iterative routing approach. The next exper iment illustrates the latter case. The robot should leave a maze that is unknown at the beginning. Dur ing driving, the Lidar scanner discovers new obstacles that are included into the robot’s map.
The iterative routing approach works as follows: a trajectory sequence is planned on the partly known map, whereas undiscovered areas are for the moment considered as obstacle-free. As a result, large parts of the route go straight through currently undiscovered obstacles.
While driving, the robot permanently checks, if the formerly planned trajectories now go though newly discovered obstacles. Corresponding collisions re quire a new planning. To reduce the number of plan ning calls, we wait, until the distance between robot and collision are below a given threshold.
Fig. 14 shows some snapshots when the robot searches a way out of a narrow maze. We can easily see straight trajectories going through unknown are as. We also see the integration of turning maneuvers. This, e.g., occurs, when the robot drives into a dead end, drives backwards when leaving the dead-end and wants to continue driving forward.
Note that a route with two turning maneuvers and a route through a free place only rarely occur in the situation of iterative routing. This is because the map in the area of the target is unknown and thus all for ward trajectories first can be planned without collid ing with obstacles.
Complex turning situations that require changes of driving directions are crucial. They may lead to lengthy paths, long planning time, or even worse, a complete failure of the planning component. We suggested a solution built upon our maneuver-based planning with Viterbi-optimization.
We integrate new turning maneuvers, extend the optimization approach to create up to two backdriv ing sequences and are able to find free places for turning maneuvers. A software structure supports multi-strategy planning. With this, the planning com ponent is able to react to the degree of complexity: for simple situations (still the majority), a simple and quick planning strategy still is sufficient. Only if this fails, more complex strategies are activated. The ap plication developer can pre-define the adaptive ac tivation of strategies in a fine-granular manner. We successfully implemented the approach on our Carbot platform.
AUTHOR Jörg Roth – Faculty of Computer Science, Nuremberg Institute of Technology, Nuremberg, Germany, e-mail: joerg.roth@th-nuernberg.de.
[1] L. Barraquand, B. Langlois and J. -C. Latombe, “Numerical potential field techniques for ro bot path planning”, IEEE Trans. on Syst., Man., and Cybern., vol. 22, no. 2, 1992, 224–241, 10.1109/21.148426.
[2] J. D. Boissonnat, A. Cerezo and J. Leblond, “A note on shortest paths in the plane subject to a con straint on the derivative of the curvature”, Re search Report 2160, Inst. Nat. de Recherche en Informatique et an Automatique, 1994.
[3] X. N. Bui, J. D. Boissonnat, P. Soueres and J. P. Lau mond, “Shortest Path Synthesis for Dubins Non -Holonomic Robot”. In: IEEE Conf. on Robotics and Automation, San Diego, CA, USA, 1994, 2–7, 10.1109/ROBOT.1994.351019.
[4] M. Čikeš, M. Đakulović and I. Petrović, “The path planning algorithms for a mobile robot based on the occupancy grid map of the environment – A comparative study”. In: XXIII Intern. Sym posium on Information, Communication and Automation Technologies, Sarajevo, 2011, 1–8, 10.1109/ICAT.2011.6102088.
[5] L. E. Dubins, “On curves of minimal length with a constraint on average curvature and with pre scribed initial and terminal positions and tan gents”, American Journal of Mathematics, vol. 79, no. 3, 1957, 497–516, 10.2307/2372560.
Fig. 14. Leaving a Maze[6] S. Garrido and L. Moreno, “Mobile Robot Path Planning using Voronoi Diagram and Fast Mar ching”, Robotics, Automation, and Control in Industrial and Service, 2015, 10.4018/978-14666-8693-9.
[7] T. Gu and J. M. Dolan, “On-Road Motion Planning for Autonomous Vehicles”. In: Su, CY., Rakheja, S., Liu, H. (eds) Intelligent Robotics and Appli cations. ICIRA, 2012, 588–597, 10.1007/978-3642-33503-7_57.
[8] P. E. Hart, N. J. Nilsson and B. Raphael, “A Formal Basis for the Heuristic Determination of Mini mum Cost Paths”, IEEE Transactions on Systems Science and Cybernetics, no. 2, 1968, 100–107, 10.1109/TSSC.1968.300136.
[9] S. Karaman and E. Frazzoli, “Incremental sam pling-based algorithms for optimal motion plan ning”, Robotics Science and Systems, no. VI 104.2, 2010, 10.48550/arXiv.1005.0416.
[10] S. Karaman, M. R. Walter, A. Perez, E. Frazzoli and S. Teller, “Anytime Motion Planning using the RRT*”. In: 2011 IEEE International Conference on Robotics and Automation, 2011, 4307–4313, 10.1109/ICRA.2011.5980479.
[11] J.-P. Laumond, P. E. Jacobs, M. Taïx and R. M. Mur ray, “A Motion Planner for Nonholonomic Mo bile Robots”, IEEE Transactions on Robotics and Automation, vol. 10, no. 5, 1994, 577–593, 10.1109/70.326564.
[12] S. M. LaValle, “Rapidly-exploring random trees: A new tool for path planning”, Technical Report 98-11, Computer Science Dept., Iowa State Uni versity, 1998.
[13] S. M. LaValle and J. J. Kuffner, “Randomized ki nodynamic planning”, International Journal of Robotics Research, vol. 20, no. 5, 2001, 378–400, 10.1177/02783640122067453.
[14] M. Mukadam, X. Yan and B. Boots, “Gaussian Process Motion Planning”. In: 2016 IEEE In ternational Conference on Robotics and Au tomation (ICRA), 2016, 9–15, 10.1109/ ICRA.2016.7487091.
[15] V. F. Muñoz and A. Ollero, “Smooth Trajectory Planning Method for Mobile Robots”. In: Proc. of the Congress on Comp. Engineering in System Ap plications, Lille, France, 1995, 700–705.
[16] M. Pitvoraiko and A. Kelly, “Efficient constrained path planning via search in state lattices”. In: Proceedings of 8th International Symposium on Artificial Intelligence, Robotics and Automation in Space (iSAIRAS ‘05), 2005.
[17] J. A. Reeds and L. A. Shepp, “Optimal paths for a car that goes both forwards and backwards”,
Pacific Journal of Mathematics, no. 145, 1990, 367–393.
[18] J. Roth, “Efficient Computation of Bypass Are as”. In: Progress in Location-Based Services 2016, Proc. of the 13th Intern. Symposium on Location -Based Services, Vienna, Austria, 2016, 193–210, 10.1007/978-3-319-47289-8_10.
[19] J. Roth, “A Viterbi-like Approach for Trajectory Planning with Different Maneuvers”. In: Intern. Conf. on Intelligent Autonomous Systems 15, 2018, Baden-Baden (Germany), 2018, 10.1007/9783-030-01370-7_1.
[20] J. Roth, “Robots in the Classroom – Mobile Robot Projects in Academic Teaching”. In: Innovations for Community Services. I4CS 2019., vol. CCIS 14041, 2019, 10.1007/978-3-030-22482-0_4.
[21] J. Roth, “Continuous-Curvature Trajectory Plan ning”, Journal of Automation, Mobile Robotics and Intelligent Systems, vol. 15, no. 1, 2021, 9–23, 10.14313/JAMRIS/1-2021/2.
[22] A. Scheuer and T. Fraichard, “Continuous -Curvature Path Planning for Car-Like Ve hicles”. In: Proceedings of the 1997 IEEE/RSJ International Conference on Intelligent Ro bot and Systems. Innovative Robotics for Real -World Applications. IROS ‘97 , 1997, 10.1109/ IROS.1997.655130.
[23] A. Viterbi, “Error bounds for convolutional co des and an asymptotically optimum decoding algorithm”, IEEE Transactions on Information Theory, vol. 13, no. 2, 1967, 260–269, 10.1109/ TIT.1967.1054010.
[24] Y. You, C. Cai and Y. Wu, “3D Visibility Graph ba sed Motion Planning and Control”. In: ICRAI ‘19: Proceedings of the 2019 5th International Con ference on Robotics and Artificial Intelligence, 2019, 10.1145/3373724.3373735.
[25] J. Zhang, Z. Shi, X. Yang and J. Zhao, “Trajectory planning and tracking control for autonomous parallel parking of a non-holonomic vehicle”, Me asurement and Control, vol. 53, no. 9-10, 2020, 1800–1816, 10.1177/0020294020944961.
[26] Z. Zhang, S. Lu, L. Xie, H. Su, D. Li, Q. Wang and W. Xu, “A guaranteed collision-free trajecto ry planning method for autonomous parking”, IET Intelligent Transport Systems, vol. 15, no. 2, 2021, 331–343, 10.1049/itr2.12028.
[27] M. Zucker, N. Ratliff, A. Dragan, M. Pivtora iko, M. Klingensmith, C. Dellin, J. A. Bagnell and S. Srinivasa, “CHOMP: Covariant Hamilto nian Optimization for Motion Planning”, Inter national Journal of Robotics Research, 2013, 10.1177/0278364913488805.
Submitted: 8th December 2021; accepted: 8th February 2022
Maciej Trojnacki, Przemysław Brzęczkowski, Dominik KleszczyńskiDOI: 10.14313/JAMRIS/4-2021/24
The paper is concerned with the problem of experimental research of Veles planetary rover performing simple con struction tasks. The current state of the art in planetary rovers and their research in construction tasks are dis cussed. The Veles rover solution designed for construc tion tasks and experimental testbed are described. The experimental testbed included a test room with Moon regolith analogue. Experimental research concerning rover mobility and manipulation tasks were carried out. Experimental research consisted of various scenarios, in cluding clearing an area that removes boulders and lev elling the soil. The complementary scenario for the area preparation was to exchange the tools of the manipula tor. In this case, the gripper and the shovel were used as end-effectors for moving objects, both structured or in the form of regolith. Results of selected experimental research are presented and discussed. Finally, directions of future works of the rover are pointed out.
Keywords: planetary rover, mobility, construction tasks, manipulation tasks, in-situ re-source utilization, experi mental research.
Extraterrestrial, hypothetical life has been fascinating to the human race for hundreds of years. This prompt ed humanity to start traveling to foreign planets and moons of the Solar System. As for the study of extra terrestrial life, planetary rovers play a key role.
Planetary rovers are unmanned ground vehicles (UGVs) intended to move across the surface of plan ets, asteroids or moons, in the area close to the land ing spot. Their task is exploration and research, e.g., analysis of climate or soil samples. They can be both teleoperated and with various degrees of autonomy. For safety reasons, rovers usually have low maximum speed. In addition, higher speeds cause more slip and larger bulldozing of wheels on the soft ground [2, 20].
Historically, the first rovers were Lunokhods, which studied the surface of the Moon in the early 70s within the framework of the Soviet space program Luna. They were teleoperated vehicles operated by the crew located on Earth. Un-manned missions to Mars are very popular. The first rover, which in 1971 arrived safely on that planet, was a Soviet Prop-M, but contact with it was lost in several seconds. In 1997, NASA Mars Pathfinder lander with Sojourner rov
er landed successfully on Mars. In turn, since 2003 NASA Mars Exploration Rover program has been carried out, consisting of twin rovers Spirit and Op portunity. The purpose of the rovers is to explore the planet mainly for geological and climatic conditions. In assumption, the mission would determine whether there has been water on Mars and whether there have been conditions for life. It can be noticed that in sub sequent missions, the rovers are getting bigger and bigger and have a larger and larger mass.
Recent solutions of rovers include the ExoMars rover by ESA [24] and Curiosity designed by NASA [23], which found pieces of evidence for the presence of liquid water on the Martian surface.
Modern rovers for planets’ exploration have a high number of motors for driving and steering the wheels. Therefore, they are classified as highly overactuated vehicles. They usually are 6-wheeled with all-wheel independent drive and with at least outer wheels steered. As a result, they have good maneuverability, stability of motion and mobility on various terrain. Due to low speed of motion and small slips of wheels, they also have a good dead reckoning. [19]
When it comes to mobile robots for construction works in terrestrial conditions, there are few such solutions. Examples of commercially available robots are e.g., the HadrianX bricklaying machine [25] and the Husky A200, which is designed for autonomous logistic tasks [26]. However, many examples of solu tions are in the research phase, including prototyp ical JA-WA technology for automatic concrete laying of composite walls [22] and mobile robots dedicated for habitable house construction by 3D printing [18].
On the other hand, rovers for construction works are currently in the concept or research phase. It is usu ally assumed that they work based on local resources, i.e., they implement the In-Situ Resource Utilization (ISRU) concept. These kinds of solutions are aimed primarily at building habitats.
Construction robots can carry out works relat ed to the movement of the mobile platform, such as grading, manipulation activities, such as digging, and including the simultaneous movement of the mobile platform and manipulation, such as bricklaying. How ever, automated execution of this type of works by mo bile robots is a very complex issue. It includes, among others, the problem of planning and controlling the movement of a mobile platform, a manipulator or both of them, localization and environment mapping, as well as identification of objects in surroundings based on machine vision.
From the point of view of implementing the move ment of the rover’s mobile platform on loose ground, typical for Mars or Moon, its mobility is of decisive im portance. Mobility can be defined as a robot’s ability to move with desired parameters of motion in defined environment conditions, with limitations of the robot itself taken into account [15].
Other properties of the rover’s mobile platform, discussed in [19] and important from the point of view of construction works, are maneuverability, sta bility of motion and dead reckoning. The maneuver ability is the robot’s ability to change its direction of motion. The stability of motion is understood as robot resistance to the unevenness of the ground during its movement. Finally, the dead reckoning of a vehicle is the ability to estimate its location based on estimat ed speed, direction and time of travel with respect to a previous estimate [6].
The basis for research of rovers’ mobility is terra mechanics [2], which originator is Polish researcher M.G. Bekker, who was the main designer of the Lunar Roving Vehicle used in Apollo 15-17 missions on the Moon. The results of re-search in this area can also be found in works [1, 7, 9, 10, 12, 21].
Regarding rovers’ motion control, this issue is the subject of, among others, work [9]. These kinds of solutions are based on the theory for mobile robots known, for example, from [6, 16]. For the rovers, an important additional issue is the modularity and re configurability, as well as their ability to function in case of partial failure. Examples of solutions in modu larity and reconfigurability include NASA Athlete, SMC Rover system [11] and the modular robotic concepts for planetary surface excavators [8]. In turn, examples of works in the area of analysis of the possibility of op eration of mobile robots after partial dam-age are [4], where the damage of environmental sensors is taken into account, and [17], in which the wheel failure is analyzed.
In turn, rover manipulation tasks are the subject of works [13, 5, 3]. The paper [13] concerns complex manipulation tasks, including moving the payload from the lander onto the rover, picking up the pay load from the ground and approaching the ground with a shovel. The article [5] presents a whole-body Cartesian impedance controller for a planetary rover equipped with a robotic arm. The MSc thesis [3] anal yses robust visual servo control and tracking for the manipulator of a planetary exploration rover, which was finally evaluated in a simulation environment.
This article was prepared in the scope of the PROACT project, which concerns the problem of cooper ation between several robots. It focused on multi-ro bot system architecture, task allocation by mutual negotiation, cooperative planning and task execution, cooperative simultaneous localization and mapping, cooperative manipulation as well as robot hardware adaptations. [14, 28]
This work concerns experimental research of a planetary rover performing construction tasks based on In-Situ Resource Utilisation (ISRU). ISRU assumes, among others, the construction task using the materials available on the moons or planets. It
includes a scenario, the goal of which is to clear an area removing boulders and levelling the soil. This requires identifying the debris from the selected area that needs to be graded to achieve a levelled surface. The complementary objective for area preparation, analyzed in this paper, is to exchange tools for the ma nipulator end-effector. [14, 28]
This allows for implementing a wide range of tasks related to the construction of habitats.
For experimental research purposes, the Veles plan etary rover was prepared. Veles includes a highly off-road-capable mobile platform with high tow ing capabilities and the ability to carry a heavy pay load. Veles platform is based on IBIS mobile robot by ŁUKASIEWICZ PIAP Institute, which was adopted for the PRO-ACT project purposes. The mechanical de sign of the rover and its implementation are shown in Fig. 1.
Fig. 1. Veles planetary rover: a – mechanical design, b – implementation [28]
The robot has no steered road wheels; therefore, it is more similar to the solutions used on Earth than rovers for planetary exploration. This is due to a dif ferent robot application and the assumption that it is dedicated to working in a familiar environment and performing simple construction works. It can there
fore move at a higher velocity, and the supervision of its operation can be local. For this reason, it may be a simpler and therefore cheaper solution.
The Veles rover consists of a mobile platform with six non-steered and independently driven wheels, an optional grader blade mounted in front of the mo bile platform, a seven DoF manipulator and a set of end-effectors including a shovel and gripper.
The so-called EBOX was placed in the rear part of the rover for powering the robotic arm, all sensors and computer subsystems (see Fig. 1a).
The EBOX houses the following electronic compo nents:
• Board Computer (OBC),
• Motion Control CPU (MCCPU),
• Robot Arm Controller (RAC),
• Power Supply Management computer (PSU),
• Instrument Control Unit (ICU),
• Network equipment, consisting of:
o Network switch,
o Wireless router,
o Mesh Radio communication system,
• Ultrasonic locator device.
On the robot were also mounted sensors, includ ing:
1. DMU30-01-0100 Inertial Measurement Unit (IMU) by Silicon Systems,
2. ZED stereoscopic camera,
3. Velodyne Puck VLP16 LiDAR,
4. Basler acA2040-25gm wrist camera,
5. Force/Torque sensor based on CL16 3F3M sensors by ZEPWN.
The locations of these sensors on the rover are il lustrated in Fig. 1a using above mentioned numbers. The sensors no. 1-3 are crucial for SLAM algorithms and autonomous control. The wrist camera mounted near the gripper allows accurate pose estimation of manipulated objects. The arm is equipped with a force and torque sensor and HOTDOCK interface enabling connection with end-effectors. [28]
The HOTDOCK is a standard interface developed by Space Applications Services NV/SA (SpaceApps). The HOTDOCK is dedicated for robotic manipulation providing an androgynous coupling to transfer me chanical loads, electrical power, data and (optionally) thermal loads through a single interface. [27]
In PRO-ACT project the HOTDOCK was used, among others, to enable tool exchange of the rover’s manipulator. The main benefits of the HOTDOCK in clude: large misalignment tolerance in translation and rotation, optimal form-fit geometry for self-guid ance, embedded sensors for automatic alignment and connection status, high mechanical load capabilities, mechanical robustness of the external shape, update and integration of control and communication elec tronics, symmetric design for optimal robotic manip ulator alignment and natural protection against dust, at least in connected mode. In Fig. 2 are shown two versions of HOTDOCK: active (a) and passive (b) as well as two Veles end-effectors: shovel (c) and grip per (d), attached to passive HOTDOCK interfaces. [28]
The most important rover’s parameters are:
• mass: 240 / 370 kg (without / with robotic arm and EBOX, respectively),
• maximum velocity: 5 km/h,
• maximum towing force by the robotic arm: 200 N. In turn, gripper performance parameters are as follows:
• maximum gripping force: 475 N,
• closing/opening time: 56,5 s,
• payload maximum weight: 20 kg,
• stroke per jaw: 100 mm,
• gripper mass: 5 kg.
In this work, for experimental research, the tests room with Moon regolith analogue was used.
Test room dimensions were 6 m × 6 m and about 2,5 m high. All the walls were covered with a black photographic background. The floor was divided into two sections: the work area for the test team, com puters and supplementary hardware, and the test area covered with regolith analogue. The work area had dimensions of 3,5 m x 1,5 m. To simulate regolith, 3 tons of clear and dry silicon sand was used (1,5 tons of 0,1 mm to 0,5 mm grains and 1,5 tons of 0,2 mm to 0,8 mm grains). [28]
A number of supplementary mockups were pre pared and used in test demonstration scenarios, as shown in Fig. 1b. Mockups were boxes covered with black foil with a marker. One of them was equipped with a simple handle, whilst the others with passive HOTDOCK. [28]
The test room shown in Fig. 3 was equipped with Marvelmind Indoor Navigation System, which is an
Fig. 2. Veles HOTDOCK interfaces and end-effectors: a – active HOTDOCK, b – passive HOTDOCK, c – shovel (left), d – gripper (right) [28]ultrasound system for robot position estimation provided by SpaceApps. It included static beacons mounted on walls and a mobile beacon attached to Veles rover. The accuracy of the pose estimation ac cording to manufacturer is 2 cm. Thus, this allowed an acceptable localization solution since the wheel odometry suffered a very high slip in the fine sand, and the Visual Odometry was not always reliable due to the features-poor terrain. This was because the ro bot’s environment was too homogeneous and did not contain enough individual features to enable explicit and precise visual localization. [28]
9. Moving the arm to the gripper docking pose,
10. De-latching the gripper,
11. Moving the arm to the shovel docking pose,
12. Latching of the shovel using HOTDOCK.
The goal of the first task was to verify the rover ability of grading for specific grading blade height. Grading was performed in teleoperation mode, con trolled by an operator, and in automatic mode, in which the rover moves based on a set of waypoints. In automatic mode, the rover guidance system got way points for grading and commanded the robot to reach the desired waypoints.
The example realization of the grading task is shown in Fig. 4. This task includes pose estimation and digital elevation model generation. Veles rover successfully carried out the desired task. However, grading via teleoperation was complex due to the low adhesion of regolith analogue. Sandhill visibility on records was also limited due to lightning conditions. Grading was carried out on the basis of the elevation map, determined according to LiDAR data, until the surface uniformity was obtained.
When it comes to pose estimation, it was stable, shown no drift, and correctly located the rover with respect to the map reference frame. The digital ele vation model developed by SpaceApps was consistent with the rover’s surroundings and was updated as the grading takes place. The elevation map representa tion was accurate and consistent taking into account the estimated pose and the input point clouds. [28]
This paper aims to present results of experimental re search of the Veles rover performing simple construc tion tasks. As an example of this kind of task, among others, the scenario including tasks of grading and tool exchange was analyzed. This scenario validated two rover activities, i.e. motion of the mobile platform as well as end-effector change and manipulation ca pabilities. The project also investigated six other sce narios not described in this article, including coopera tive mapping, cooperative unloading and assembling modules, cooperative virtual manipulation and trans port, cooperative transport (with object) as well as remote gantry deployment. [28]
In this scenario, the Veles rover was equipped with a grading blade installed directly on the platform at a specific height coming from the grading tests. In addition, the end-effector interface was mounted on the manipulator tip, and the end-effector holster was prepared to carry two different end-effectors (gripper and shovel).
The whole scenario included the following steps:
1. Moving via teleoperation and setting grading blade height,
2. Digital Elevation Map generation before grading,
3. Offline determination of waypoints for Rover Guidance to follow for grading,
4. Executing grading process,
5. Post-grading Digital Elevation Map generation and repetition of grading, if necessary,
6. Moving the arm to the gripper docking pose,
7. Latching of the gripper using HOTDOCK,
8. Executing manipulation task using gripper,
Fig. 5 illustrates the digital elevation model before (left) and after (right) the grading operation.
The robotic arm movement was verified during the second part of the scenario, which covers end-ef fector change and manipulation capabilities. Position matched the goal within range, and the HOTDOCK was aligned to perform de-latching and latching with the gripper and shovel.
Fig. 3. Two static beacons (on walls) and a mobile beacon (on Veles rover) used within the Ultrasound Location System [28] Fig. 4. Veles rover performing grading task [28]Fig. 6 illustrates the steps taken to demonstrate the tool exchange. The sequence shows the HOT DOCK’s de-latching to let go of the gripper, the arm’s movement towards the second tool (shovel) and the latching of the second tool using HOTDOCK. Video presenting PRO-ACT project concept and above-described results of experimental research are available on [29].
In the present work, the problem of performing sim ple construction tasks by planetary rover was ana lyzed. The rover executed two kinds of tasks: 1. grad ing, including motion of the mobile platform, and 2. end-effector change and manipulation using a robotic arm.
The most important conclusions of the work are summarized below.
• The robot successfully performed both grading and manipulation tasks.
• Grading via teleoperation was difficult due to the low adhesion of the regolith analogue. In this case, automation of motion control allows for stabilization of motion.
• The kinematic structure of the rover’s chassis provides high stability in longitudinal movement. Still, it is characterized by large dead reckoning during turning or rotating in place.
• Thanks to a large number of degrees of freedom, the manipulator allows for the implementation of complex manipulation tasks. However, this is an overactuated solution; therefore, its automatic control is complex.
Directions of future works can cover, among others:
• Realization of cooperative manipulation and transport tasks by two robots.
• Executing other typical construction tasks like digging, rock crushing, bricklaying etc. necessary for building habitats.
This project has received funding from the European Union’s HORIZON 2020 research and innovation pro gramme under a grant agreement No 821903.
Maciej Trojnacki* – PIAP Space Sp. z o.o., Al. Jerozolimskie 202, 02-486 Warsaw, Poland, e-mail: maciej.trojnacki@piap-space.com
Fig. 5. Digital elevation model of the terrain before (a) and after (b) the grading operation [28] Fig. 6. Veles rover executing tool exchange task [28]Przemysław Brzęczkowski – PIAP Space Sp. z o.o., Al. Jerozolimskie 202, 02-486 Warsaw, Poland, e-mail: przemyslaw.brzeczkowski@piap-space.com
Dominik Kleszczyński – PIAP Space Sp. z o.o., Al. Jerozolimskie 202, 02-486 Warsaw, Poland, e-mail: dominik.kleszczynski@piap-space.com
[1] A. Lopez Arreguin, S. Montenegro and E. Dilger, “Towards in-situ characterization of regolith strength by inverse terramechanics and machi ne learning: A survey and applications to pla netary rovers”, Planetary and Space Science, vol. 204, 2021, 10.1016/j.pss.2021.105271.
[2] M. G. Bekker, Off-the-road Locomotion: Research and Development in Terramechanics, University of Michigan Press, 1960.
[3] L. M. Bielenberg, “Robust Visual Servo Control and Tracking for the Manipulator of a Planeta ry Exploration Rover”, Master Thesis, Technical University Munich, 2021.
[4] P. Bigaj, J. Bartoszek and M. Trojnacki, “Sensitivi ty Analysis of Semiautonomy Algorithm of Mobi le Robot to Environmental Sensors’ Failure - Si mulation Research and Experimental Tests”. In: 28th International Symposium on Automation and Robotics in Construction, Seoul, Korea, 795800, 10.22260/ISARC2011/0148.
[5] K. Bussmann, A. Dietrich and C. Ott, “Whole-Bo dy Impedance Control for a Planetary Rover with Robotic Arm: Theory, Control Design, and Expe rimental Validation”. In: 2018 IEEE International Conference on Robotics and Automation (ICRA), 2018, 910–917, 10.1109/ICRA.2018.8460533.
[6] P. Corke, Robotics, Vision and Control, Springer International Publishing, 2017.
[7] M. Heverly, J. Matthews, J. Lin, D. Fuller, M. Ma imone, J. Biesiadecki and J. Leichty, “Traver se Performance Characterization for the Mars Science Laboratory Rover”, Journal of Field Ro botics, vol. 30, no. 6, 2013, 835–846, 10.1002/ rob.21481.
[8] A. S. Howe, B. H. Wilcox, H. Nayar, R. P. Muel ler and J. M. Schuler, “Maintenance-optimi zed Modular Robotic Concepts for Planetary Surface ISRU Excavators”. In: 2020 IEEE Ae rospace Conference, 2020, 1–15, 10.1109/ AERO47225.2020.9172688.
[9] K. Iagnemma and S. Dubowsky, Mobile Robots in Rough Terrain: Estimation, Motion Planning,
and Control with Application to Planetary Rovers, Springer Berlin Heidelberg, 2004.
[10] G. Ishigami, A. Miwa, K. Nagatani and K. Yoshi da, “Terramechanics-based model for steering maneuver of planetary exploration rovers on lo ose soil”, Journal of Field Robotics, vol. 24, no. 3, 2007, 233–250, 10.1002/rob.20187.
[11] A. Kawakami, A. Torii, K. Motomura and S. Hi rose, “SMC Rover: Planetary Rover with Trans formable Wheels”. In: B. Siciliano and P. Dario (eds.), Experimental Robotics VIII, vol. 5, 2003, 498–506, 10.1007/3-540-36268-1_45.
[12] T. Kobayashi, Y. Fujiwara, J. Yamakawa, N. Yasufu ku and K. Omine, “Mobility performance of a ri gid wheel in low gravity environments”, Journal of Terramechanics, vol. 47, no. 4, 2010, 261–274, 10.1016/j.jterra.2009.12.001.
[13] P. Lehner, S. Brunner, A. Domel, H. Gmeiner, S. Riedel, B. Vodermayer and A. Wedler, “Mobi le manipulation for planetary exploration”. In: 2018 IEEE Aerospace Conference, 2018, 1–11, 10.1109/AERO.2018.8396726.
[14] L. Lopes, S. Govindaraj, W. Brinkmann, S. Lacro ix, J. Stelmachowski, F. Colmenero, J. Purnell, K. Picton and N. Aouf, “Analogue lunar research for commercial exploitation of in-situ resour ces and planetary exploration - Applications in the PRO-ACT project”. In: EGU General Assembly Conference Abstracts, 2021, 1–11, 10.5194/egu sphere-egu21-9180.
[15] P. Sandin, Robot Mechanisms and Mechanical De vices Illustrated, McGraw-Hill/TAB Electronics, 2003.
[16] R. Siegwart, I. R. Nourbakhsh and D. Scaramuzza, Introduction to Autonomous Mobile Robots, MIT Press, 2011.
[17] A. J. Stimpson, M. B. Tucker, M. Ono, A. Steffy and M. L. Cummings, “Modeling risk perception for mars rover supervisory control: Before and after wheel damage”. In: 2017 IEEE Aerospace Confe rence, 2017, 1–8, 10.1109/AERO.2017.7943871.
[18] K. Subrin, T. Bressac, S. Garnier, A. Ambiehl, E. Paquet and B. Furet, “Improvement of the mo bile robot location dedicated for habitable house construction by 3D printing”, IFAC-PapersOnLi ne, vol. 51, 2018, 716–721.
[19] M. Trojnacki and P. Dąbek, “Mechanical Proper ties of Modern Wheeled Mobile Robots”, Journal of Automation, Mobile Robotics and Intelligent Systems, vol. 13, no. 3, 2019, 3–13, 10.14313/ JAMRIS/3-2019/21.
[20] M. Trojnacki and P. Dąbek, “Studies of dynamics of a lightweight wheeled mobile robot during
longitudinal motion on soft ground”, Mechanics Research Communications, vol. 82, 2017, 36–42, 10.1016/j.mechrescom.2016.11.001.
[21] Z. Wang, H. Yang, L. Ding, B. Yuan, F. Lv, H. Gao and Z. Deng, “Wheels’ performance of Mars exploration rovers: Experimental study from the perspective of terramechanics and structural mechanics”, Journal of Terramechanics, vol. 92, 2020, 23–42, 10.1016/j.jterra.2020.09.003.
[22] A. Więckowski, ““JA-WA” – A wall construction system using unilateral material application with a mobile robot”, Automation in Construction, vol. 83, 2017, 19–28, https://doi.org/10.1016/ j.autcon.2017.02.005.
[23] “Curiosity – NASA’s Mars Exploration Program”, National Aeronautics and Space Administra tion, https://mars.nasa.gov/msl/, Accessed on: 2022-08-30.
[24] “ESA – Robotic Exploration of Mars”, European Space Agency, https://exploration.esa.int/web/ mars/, accessed on: 2022-08-30.
[25] “Hadrian X® – Outdoor Construction and Bric klaying Robot from FBR”, https://www.fbr.com. au/view/hadrian-x/, accessed on: 2022-08-30.
[26] “A mobile robot for construction sites”, https:// www.electricmotorengineering.com/a-mobi le-robot-for-construction-sites/, accessed on: 2022-08-30.
[27] “Mating/Demating Device - HOTDOCK”, https:// www.spaceapplications.com/products/mating -demating-device-hotdock, accessed on: 202208-30.
[28] “Planetary RObots Deployed for Assembly and Construction Tasks – PRO-ACT”, project docu mentation (2021).
[29] “PRO-ACT: Power of Robots Collaborating in Space Applications”, https://www.youtube. com/watch?v=eVK68jOOuk4, accessed on: 2022-08-30.
Submitted: 11th October 2021; accepted: 8th February 2022
DOI: 10.14313/JAMRIS/4-2021/25
In traditional industries, manual grinding and polish ing technologies are still used predominantly. However, these procedures have the following limitations: exces sive processing time, labor consumption, and product quality not guaranteed. To address the aforementioned limitations, this study utilizes the good adaptability of a robotic arm to develop a tool-holding grinding and pol ishing system with force control mechanisms. Specifically, off-the-shelf handheld grinder is selected and attached to the robotic arm by considering the size, weight, and processing cost of the stainless steel parts. In addition, for contact machining, the robotic arm is equipped with a force/torque sensor to ensure that the system is active compliant. According to the experimental results, the de veloped system can reduce the surface roughness of 304 stainless steel to 0.47 µm for flat surface and 0.76 µm for circular surface. Moreover, the processing trajectory is programmed in the CAD/CAM software simulation environment, which can lead to good results in collision detection and arm posture establishment.
Keywords: Active compliant, hybrid position/force con trol, robot manipulator, surface machining, surface roughness
The world is facing many problems, including the effects of an aging society and declining birthrate in some countries. According to the statistics by the United Nations, the global fertility rate continues to fall, and the labor force is gradually aging. This will significantly affect national competitiveness. It is of utmost important for the government and enterprises to find ways to replace labor after the supply of labor has declined. Many companies started introducing robotic arms to replace manpower. Currently, the in dustry uses robotic arms for automated operations. Since the first robotic arm was invented by Joseph Engelberger in 1959, robots have been used in appli cations ranging from manufacturing of electronics to agriculture, medical industry, and even service sec tors. Therefore, it is possible to use robotic arms in any operation. To satisfy human needs and environ mental restrictions, the design of intelligent robotic arms has been considered as a new topic of research.
For typical contact processing tasks, such as grind ing and polishing, several steps require experienced
operators. During the welding and polishing for met al processing, the time for grinding and polishing is nearly four times the welding time. However, this time-consuming and labor-intensive processing pro cedure can be replaced by utilizing the high-efficiency robot arm. Hence, it has become a popular research topic. Modern robotic polishing systems are divided into hand-holding tools and handheld workpieces. The former is used for large workpieces, and the lat ter is suitable for smaller objects [1]. In the studies on modern robotic arm polishing, most of the robotic arms are used for clamping workpieces that should be ground and polished. For example, Zhu et al. [2] pro posed a combination of a force model and abrasive belt grinding force to evaluate the surface roughness of a workpiece, and Ma et al. [3] performed polishing with a constant force in a self-designed abrasive belt grinding system. The major limitation of this system is that once the weight or size of the workpiece ex ceeds the range of the robotic arm, the workpiece can not be clamped. Therefore, in the polishing process of large workpieces, the hand-holding polishing tool is optimal. Furthermore, the research of this robotic polishing system is based on the combination and de sign of the end effector of the robotic arm and grind ing tool [4-6]. In addition, it is worth mentioning that an active contact flange (ACF) based on active compli ant technology and powered pneumatically has been available in the market for robotic grinding and pol ishing application [7]. The cost is yet expensive.
In this study, a cost-effective robotic polishing sys tem equipped with a grinding and polishing module and a force sensor is proposed. Furthermore, experi ments are conducted on 304 stainless steel, which is commonly used in the industry. The grinding experi ments in this study are classified into two types. The first type of experiments involve the position control of the robotic arm according to the path planned by RoboDK (a robot simulation software). The other type of experiments involve a hybrid position/force control that combines the planning path and force control.
In this section, the experimental architecture includ ing hardware and computer software are described.
Hardware in this robotic polishing system mainly in cludes a robotic arm, a force sensor, and a grinding
module. The industrial robotic arm (Stäubli TX60L) has six degrees of freedom which exhibits a high de gree of flexibility, solid structure, and special reduc tion gear system. The main task of the robotic arm in volves accepting the instructions provided by the host computer and conducting grinding and polishing on the workpiece. Second, the force sensor (ATI Axia80 EtherCAT F/T sensor) plays the role of calculating the grinding force in this robotic polishing system. The maximum force that this sensor can measure is 500 N and the torque is 20 Nm. The application level of the force sensor is extremely wide, including robotic arm loading work, contact force feedback, and constant force work. Finally, to reduce cost, a self-designed tool holder by 3D printing and the off-the-shelf handheld grinder are combined into a grinding module. The grinding module performs functions including cut ting, grinding, and polishing solely by changing the granularity of the grinding wheel. Figure 1 shows the hardware architecture used in this study.
All software algorithms in this robotic polishing sys tem are executed in a host computer. The program ming language in host computer is C#. Tasks of the host computer involve sending commands via Eth ernet to the robot controller for moving the robotic arm, reading data of the force sensor via EtherCAT, monitoring the postures of the robot arm during op eration, and generating polishing path using RoboDK package. Note that combination of TwinCAT (the Win dows Control and Automation Technology, a C# proj ect) and EtherCAT is used as an easy-to-configure au tomated system. Figure 2 depicts the communication protocol used in this study.
This section describes the force control strategy of the system for grinding and polishing tasks. Most robotic arms, either industrial or collaborative, are typically used for repetitive and time-consuming ac tions, such as pick-and-place, locking, and assembly. The common point of these actions is that they use a pure position-control architecture to perform tasks. However, under pure position control, irrespective of the force applied in the working environment, the ro botic arm will move to the position based on the co ordinate point provided by the operator. This control method may generate contact forces, such as those for mechanical processing, which often lead to the exces sive force, and thereby causing damage to the robotic arm or processed workpieces. Therefore, the ability of the robotic arm to comply with external forces is an extremely important issue. To solve the aforemen tioned problems, the force control strategy can enable the robot to interact with the force it experiences dur ing operation, such as imparting the same force to an object, or adjusting when encountering geometrical differences in assembly tasks. Force control exhibits evident certain advantages in terms of safety consid erations or adapting to the environment. Generally, control strategies are divided into two categories: in direct force control strategies and direct force control strategies.
Force control of robotic systems usually uses force/ torque sensors to process the external forces mea sured in the environment. However, indirect force
Fig. 1. Robotic polishing system: 1 robot arm, 2 force sensor, 3 grinding modulecontrol does not require force/torque sensors. Under this control strategy, when the robot’s current posi tion coordinates deviate too far from the target posi tion, a force is exerted to control it. Therefore, there is no clear closed-loop feedback control, which implies that the control mechanism cannot handle the large external force due to the trajectory deviation, and the flexibility is relatively poor when compared to that of the direct force control. Indirect force control in volves two control strategies: impedance control and admittance control. Among the strategies, the spring damping system is the most accurate representation of impedance control. The contact force and arm mo tion are used as input and output, respectively. This implies that the impedance mechanism controls the robot, and the external force generated by the envi ronment ensures compliance of the movement pro cess.
When compared with indirect force control, the direct force control strategy employs a force/torque sensor that senses external force, and the measured force is fed back to the robot for path trajectory correction to ensure compliance of the end effector of the robotic arm [8]. In this control strategy, trajectories of force and motion are considered for robot control and can be further matched with indirect force control. For robots it is often required to maintain external forces within a certain range. Hybrid position/force control is a common direct force control strategy. This control strategy involves simultaneous control of the force and movement of the end effector of the robotic arm. To perform hybrid position/force control on the ro bot, a surface is created first. Then, position control is performed in the tangential direction of the surface and force control is performed along the normal di rection of the surface. The force and position are con trolled in two directions to form a hybrid position/ force control strategy. When the robot starts per forming work, it searches for the contact force on the unconstrained axis, and it only moves along this axis until it generates contact force with the surface of the object. Throughout the process, the force/torque sen sor sends the force data back to the controller. Once contact is realized, a constant force is applied to the constrained axis for control, and the force is always maintained when the programmed trajectory is exe cuted. Hence, this ensures that the position of the end effector of the robotic arm and force are controlled in a closed loop.
In this study, a proportional-derivative (PD) con troller is used for force control. Thus, the force of grinding and polishing can be maintained via a PD controller. In this experiment, the force control is applied to the position path of the robotic arm, and Cartesian coordinates of the robotic arm are adjusted according to the contact force. This ensures that the position changes and continuously caters to the force value to obtain a constant force effect. The PD formula designed in this study is shown as follows
where Zn+1 denotes the new coordinate position of the robotic arm in the surface normal direction, Zn denotes the current coordinate position, Fe(n) denotes the error between the desired force value Fd (gravity compensation is included) and the current force val ue, and Fe(n–1) represents the last error value. Figure 3 shows a force comparison with and without force control. The figure shows that in the contact force ex periment, position control directly through the path does not have the ability to adjust the position. Hence, this leads to a larger force deviation. However, the force that is obtained by adjusting the position via the PD controller is controlled within the desired value.
This section describes the machining process of ro botic arm grinding and polishing and the machining path for different shapes of workpieces.
The workpiece used in this study is 304 stainless steel. This kind of stainless steel has wide applications and is used mainly for food-grade utensils, containers, and furniture. The surface of stainless steel is usually stained due to chemical and electrochemical corro sions. Additionally, weld beads and scratches also af fect the surface of the workpiece. Therefore, grinding and polishing using grinding wheels with a variety of particle sizes and cloth wheels is proposed to regain excellent stainless steel surfaces. To date, this type of mechanical processing method still exists in major factories and is done mostly by skilled workers. How ever, stainless steel exhibits high toughness and thus is not easy to grind. In this study, by consulting skilled workers in this field, the hands-on experience by the authors, and also by referring to modern grinding and polishing technology principles [9, 10], a 6-steps ma chining process is proposed. First, grinding wheels made by polyvinyl alcohol (PVA) with grit size of 60 and 120 are used for rough grinding. Then grinding wheels with grit size of 240 and 320 are followed for fine grinding. Furthermore, a grinding wheel with grit
size of 400 is employed for final grinding. Lastly, cloth wheel with polishing wax is applied in the final step for the polishing process. Based on this processing sequence, the surface of stainless steel can reach the #300 grade as per the Japanese standard, namely a smooth and mirror-grade surface.
After the process design is completed, the follow ing rules of thumb are advised for robotic arm grind ing and polishing stainless steel:
1. During grinding and polishing processes, the total material removal should not exceed 0.3 mm in thick to the maximum possible extent. Essentially, within this range, the workpiece will not be affected.
2. When performing rough grinding, the feed rate must be greater than that of fine grinding.
3. The number of repetitions of each process needs to be reduced. Grinding and polishing are techniques for removing material. Repeating too many times will excessively increase the amount of material removed.
4. The angle of the grinder with respect to surface tangent during grinding varies across individuals. Typically, it is in the range of 30°–45°. However, approximately 5° and up is sufficient for robotic arm grinding and polishing.
5. The grinding wheel with high grit size wears faster than the one with low grit size, so care must be taken for the latter grinding processes.
6. As far as the grinding and polishing process is concerned, the correct method involves holding the grinder to move forward for a certain distance after the grinding wheel touches the workpiece and then pulling it up. It is important not to move grinder back and forth because it can easily result in uneven surfaces.
In the study, stainless steel workpieces are divided into flat workpieces and curved workpieces. Both of which require path planning using RoboDK package as described in the next subsection.
The grinding path of a flat workpiece is relatively sim ple. We use a robotic arm equipped with a grinder and choose the grinding surface of the grinding wheel as the TCP (tool center point) position. A grinding area with a width of 42.55 mm and length of 100 mm is considered based on the TCP coordinates. The grind ing path is a straight line divided into 20 points, and the tool orientation remains unchanged along the path. In grinding and polishing operations, the robotic arm moves forward in a straight line throughout the entire process and is pulled up at the end in a manner similar to a skilled worker. The rough grinding and fine grinding process are performed 1–2 times, and the polishing process is performed 5–6 times.
When grinding a curved workpiece with a diam eter of 212.30 mm, the grinding wheel moves along the surface for an arc length of 237.92 mm. Along the curved path, the number of points is 96 and the X-axis and Z-axis of the tool coordinate change continuous ly so that Z-axis always keeps normal to the surface. The grinding and polishing operations for the curved
workpiece is similar to that for the flat workpiece as described in the previous paragraph. Furthermore, it is necessary to focus on collision detection and path generation due to the large curvature of the work piece. A schematic diagram of path planning using RoboDK package is shown in Figure 4.
The study is divided into two experimental methods, namely position control [11] and hybrid position/ force control [12, 13], and two types of workpiece, flat and curved workpieces. First, in the position control experiment, the robotic arm directly uses the path planned by the RoboDK package and the converted coordinate position for the experiment. Simultaneously, the force sensor is turned on and is responsible for monitoring the force value during pure position control. Second, in the experiment of hybrid position/force control, force sensor is em ployed for adjusting the grinding path in Z direction through the PD controller in (1). During the grind ing and polishing process, the coordinate position of the end effector of the robot arm is updated, and the robot attempts to maintain the grinding force as con stant. In terms of parameter settings, the rotation speed of the grinder is fixed at 12000 rpm and the feed rate of the robotic arm is set to 25 mm/s. More over, the surface roughness is related to the grinding force, and excessive force can easily lead to poor sur face quality. After a few tries in the study, the results indicated that when the applied force exceeded 30 N, the cloth wheel responsible for the polishing task was easily burnt and the stainless-steel surface was overheated and oxidized. Thus, the desired applied force for grinding and polishing is set to 10 N in the experiment. The robot arm grinding and polishing processes are shown in Figure 5.
The experimental results of the study were summa rized into two parts, namely the mean absolute error (MAE in %) of the grinding and polishing force of each process
Tab. 1. MAE error between the actual grinding force and desired force
Experiment Process Position control (%) Hybrid position/ force control (%)
#60 29.3 11 #120 37.73 12.11 #240 97.99 20.44 #320 153.8 22.45 #400 197.9 14.07
Polishing 42.63 15.09
Tab. 2. Surface roughness of stainless steel of each process
Experiment Process Position control (µm) Hybrid position/ force control (µm)
#60 2.27 2.10 #120 1.66 1.60 #240 1.26 1.19 #320 0.95 0.80 #400 0.80 0.66 Polishing 0.68 0.47
and the value of the surface roughness (arithmetic mean deviation Sa) [14] of stainless steel after each process is completed. To measure surface roughness, a 3D surface profiler (Keyence VR 3000) with a highmagnification lens (40x) was used to evaluate the machining quality for each process. In the results of the flat workpiece, given that the position control ex periment was not aided by force control, a large gap existed between the grinding and polishing force and the desired value. In the hybrid position/force control experiment, force control was added to the original path such that the force response was improved dur ing flat grinding, and the force error was significantly reduced. In other words, the robotic arm attempted to process the workpiece surface with the desired grinding force. The MAE of machining force for each process using PVA sponge wheel for grinding (granu larity: 60–400) and cloth wheel for polishing of the two experiments was listed in Table 1. Large force deviation using only position control was apparently reduced by applying hybrid position/force control in each process. Improved surface roughness for each corresponding process was listed in Table 2, and the finished workpiece surfaces were shown in Figure 6 with clearly seen mirror effect on both surfaces. For flat workpiece, surface roughness has been reduced from 3.04 µm before grinding to final 0.68 µm and 0.47 µm respectively by position control and hybrid position/force control. Approximate 30.88% im provement in surface roughness was achieved by hy brid position/force control over pure position control.
Fig. 6. Finished flat workpieces: position control (top) and hybrid position/force control (bottom)
For curved workpiece, because the surface was al ready smooth in the beginning thus only the last 3 steps of fine grinding (using grinder with grit size 320 and 400) and polishing (by cloth wheel) were conducted. MAE errors of the grinding and polish ing force of each process for the two control methods were compared in Table 3, where in general hybrid position/force control outperformed the position control. Improved surface roughness for each cor responding process was shown in Table 4, where in the final polishing task approximate 32.14% improve ment (from 1.12 µm to 0.76 µm) was obtained by the hybrid position/force control over pure position con trol. The finished workpiece surfaces were illustrated in Figure 7. Indeed, the curved workpiece surface was smoother and brighter by the hybrid position/force control.
Tab. 3. MAE error between the actual grinding force and desired force
Experiment Process Position control (%) Hybrid position/ force control (%)
#320 75 23.7
#400 51.45 29.8
Polishing 19.7 20
Tab. 4. Surface roughness of stainless steel of each process
Experiment Process Position control (µm) Hybrid position/ force control (µm)
#320 1.76 1.01
#400 1.25 0.96
Polishing 1.12 0.76
In this study, a robotic arm and two experimental methods were used to realize the automatic grind ing and polishing of stainless steel. Furthermore, a 6-steps machining process for polishing stainless steel along with some practical rules of thumb was proposed. Grinding and polishing with pure position control is simple to implement, however it could have untouched areas on the workpiece in the planned path due to deformation and/or wear of the grind ing wheel, especially for the curved workpiece. For example, the last step of polishing curved workpiece in Table 3 has 19.7% MAE in the polishing force for position control which is comparable to 20% MAE for hybrid position/force control. But position control gives worse surface roughness 1.12 µm as compared to 0.76 µm by the hybrid position/force control as shown in Table 4, this is because almost half of the polishing path is untouched by the cloth wheel using pure control method (during this period the zero pol ishing force is excluded in the calculation of MAE).
By using a force/torque sensor in the robot arm, the developed hybrid position/force control method was excellent in terms of the consistency of machining force and the surface quality of the finished workpiece. Therefore, problems such as uneven applied force by either human operator or pure position control, man ufacturing and/or positioning errors in the workpiece, and deformation and/or wear of the grinding wheel can be alleviated to certain extent by adding force control in the machining process. The flat and curved stainless steel in the experiments were surface finished to reach respective 0.47 µm and 0.76 µm in surface roughness by the proposed machining procedures with hybrid position/force control. According to DIN standard for surface quality of stainless steel [15], the results in this study reached 1J-2J grade in the category of Mechani cally Polished & Brushed Stainless Steel Finishes, with which grade the stainless steel is usually used in fur niture, elevator door, and upholstery accessories. For comparison with a skilled worker, the surface rough ness obtained by a skilled worker basically can reach about 0.4 µm, which is not far from what this paper can achieve. However, a skilled worker is hard to find and expensive to hire, and requires years of training but has a limited working hours per day. The technique devel oped in this paper can help save training costs and ex cessive labor expenses.
Fig. 7. Finished curved workpieces: position control (top) and hybrid position/force control (bottom)It is noted that the developed polishing technique can also be applied to a workpiece with both flat and curved surfaces, since the employed RoboDK soft ware can deal with straight and curved path. In addi tion, the desired applied force was set to 10 N for both flat and curved surfaces in the experiment, hence the control algorithm can be used throughout the entire object without difficulty.
Jinsiang Shaw* – National Taipei University of Tech nology, Taiwan, e-mail: jshaw@ntut.edu.tw.
Yu-Jia Fang – National Taipei University of Technol ogy, Taiwan, e-mail: high1204br520@gmail.com.
* Corresponding author
[1] J. Li, T. Zhang, X. Liu, Y. Guan and D. Wang, “A Su rvey of Robotic Polishing”. In: 2018 IEEE In ternational Conference on Robotics and Biomi metics (ROBIO), 2018, 2125–2132, 10.1109/ ROBIO.2018.8664890.
[2] D. Zhu, X. Xu, Z. Yang, K. Zhuang, S. Yan and H. Ding, “Analysis and assessment of robotic belt grinding mechanisms by force modeling and force control experiments”, Tribology Interna tional, vol. 120, 2018, 93–98, 10.1016/j.tribo int.2017.12.043.
[3] K. Ma, X. Wang and D. Shen, “Design and Expe riment of Robotic Belt Grinding System with Constant Grinding Force”. In: 2018 25th Interna tional Conference on Mechatronics and Machine Vision in Practice (M2VIP), 2018, 1–6, 10.1109/ M2VIP.2018.8600899.
[4] M. Jinno, F. Ozaki, T. Yoshimi, K. Tatsuno, M. Taka hashi, M. Kanda, Y. Tamada and S. Nagataki, “De velopment of a force controlled robot for grin ding, chamfering and polishing”. In: Proceedings of 1995 IEEE International Conference on Robo tics and Automation, vol. 2, 1995, 1455–1460, 10.1109/ROBOT.1995.525481.
[5] M. A. Elbestawi, K. M. Yuen, A. K. Srivastava and H. Dai, “Adaptive Force Control for Robotic Disk Grinding”, CIRP Annals, vol. 40, no. 1, 1991, 391–394, 10.1016/S0007-8506(07)62014-9.
[6] J. A. Dieste, A. Fernández, D. Roba, B. Gonzalvo and P. Lucas, “Automatic Grinding and Poli shing Using Spherical Robot”, Procedia Engine ering, vol. 63, 2013, 938–946, 10.1016/j.pro eng.2013.08.221.
[7] “Active contact flange”, FerRobotics Compliant Robot Technology GmbH. https://www.ferrobo tics.com/en/services/products/active-contact -flange/. Accessed on: 2022-08-30.
[8] B. Siciliano and L. Villani, Robot Force Control, Springer US, 1999.
[9] W. B. Rowe, Principles of modern grinding tech nology, William Andrew, 2014.
[10] N. Kurihara, S. Yamazaki, T. Yoshimi, T. Eguchi and H. Murakami, “The proposal of automatic task parameter setting system for polishing robot”. In: 2015 12th International Conference on Ubi quitous Robots and Ambient Intelligence (URAI), 2015, 479–481, 10.1109/URAI.2015.7358808.
[11] J. Kim, W. Lee, H. Yang and Y. Lee, “Real-time monitoring and control system of an industrial robot with 6 degrees of freedom for grinding and polishing of aspherical mirror”. In: 2018 International Conference on Electronics, Infor mation, and Communication (ICEIC), 2018, 1–4, 10.23919/ELINFOCOM.2018.8330691.
[12] P. G. M. Cáceres. Grinding Force Control of the Cutting Edge of a Blade by a Robot Manipulator, Master’s Thesis, National Taipei University of Technology, Taipei, Taiwan, 2019, https://hdl. handle.net/11296/wnu34a.
[13] M. Fazeli and M. J. Sadigh, “Adaptive hybrid po sition/force control for grinding applications”. In: 2012 IEEE International Conference on Cyber Technology in Automation, Control, and Intelli gent Systems (CYBER), 2012, 297–302, 10.1109/ CYBER.2012.6392569.
[14] E. Jansons, J. Lungevics and K. A. Gross, “Surface roughness measure that best correlates to ease of sliding”. In: 15th International Scientific Confe rence, Engineering for Rural Development, 2016.
[15] “Stainless Steel Finishes Explained – EN & ASTM,” (2019), Andreas Velling, https://frac tory.com/stainless-steel-finishes-en-astm/. Ac cessed on: 2022-08-30.
Submitted: 17th November 2021; accepted: 8th February 2022
M. F. Abdul Aziz, W. M. Bukhari, M. N. Sukhaimie, T.A. Izzuddin, M.A. Norasikin, A. F. A. Rasid, N. F. Bazilah
DOI: 10.14313/JAMRIS/4-2021/26
This paper presents an automation process is a need in the agricultural industry specifically chili crops, that im plemented image processing techniques and classifica tion of chili crops usually based on their color, shape, and texture. The goal of this study was to develop a portable sorting machine that will be able to segregate chili based on their color by using Artificial Neural Network (ANN) and to analyze the performance by using the Plot Confu sion method. A sample of ten green chili images and ten red chili images was trained by using Learning Algorithm in MATLAB program that included a feature extraction process and tested by comparing the performance with a larger dataset, which are 40 samples of chili images. The trained network from 20 samples produced an over all accuracy of 80 percent and above, while the trained network from 40 samples produced an overall accuracy of 85 percent. These results indicate the importance of further study as the design of the smart sorting machine was general enough to be used in the agricultural indus try that requires a high volume of chili crops and with other differentiating features to be processed at the same time. Improvements can be made to the sorting system but will come at a higher price.
Keywords: Precision Agriculture, Artificial Neural Net work, Smart Fertigation
A sorting process that is automated with the use of a control system will not only make the process simple and precise but also reliable to be used as a machine [1]. This is because an automatic sorting machine has the purpose to replace the basic function of the human vision, thinking, and actuate for sorting operation [2]. It has many possible uses in the food processing in dustry especially fruits and vegetable products such as chili to be sorted based on their differentiating fea tures such as color, shape, and texture [3]. However, to automatically inspect and classify the chili accurately and effectively, the normal use of simple controllers and sensors without the ability to learn and predict the outcome will not be effective enough to handle the required task [4].
One way to automatically classify chili and to achieve an accurate result is to use artificial intelli
gence with the help of machine vision [5], [6], [7]. Chili can be classified based on their color by using Artificial Neural Network (ANN) and the image captured simply by using a smartphone camera [8]. Other studies also decided to use ANN as the fruits and vegetable classi fier having a variety of colors [9], [10], [11]. However, past studies only focus on the segregation process of dried red chili and the maturity level of the chili. Few studies have been made for the classification of fresh chili and in real-time application [12], [13].
This study presents a design for the classification of fresh chili based on color for real-time application and implementation of the system by using a sorting machine. By using the image processing technique for the preparation of feature extraction, the data can be trained using ANN in MATLAB and tested. The cho sen method for this study proven in two ways, using plot confusion to confirm the accuracy of the trained network and also by analyzing the plot receiver oper ating characteristic curve to confirm the performance of the trained network.
A system for sorting most common usage is to classify a batch of objects such as chili based on the desired condition. This can be various types of sorting vari ables such as sort based on color, shape, size or even defect feature.
A previous study designed a strategy to sort objects at high speed by using a Delta robot [14]. The design included a vision module using a CCD camera for grabbing the image of the objects to be processed and object tracking purposes by using a servo motor that sends the position pulse data to the system. The sec ond module was motion control that controlled the speed of the conveyor for 400 millimeters per second and 120 sorting tasks per minute of the Delta robot. The proposed strategy worked efficiently for only two different pieces of the object.
To segregate objects efficiently especially for small size objects such as rice grain or chili, a vibrating could also be used. However, from the previous studies, the sorting process was only able to separate unwanted products from the rest without any other differentiat ing features [15,16]. A system with multiple features to differentiate will be a bit complex. This is shown in
a study, where a system designed to sort sweet tam arind into three different classes of size using three pneumatic segregator and defective factor to the end line of the product stream [17]. The important feature that this study was missing was the color trait for the product.
There are various methods for controlling a sorting machine system [18, 19, 20, 21]. The most common basic controller for this purpose can be by using AR DUINO controller, Peripheral Interface Controller (PIC) microcontroller, Raspberry Pi, or even Program mable Logic Controller (PLC). ARDUINO has been used for many various purposes such as robotic con test implementation, robotic devices control system with the implementation of Pulse Width Modulation (PWM), and complicated tasks such as controlling various types of sensors monitoring and vision mod ules [22], [23], [24]. For automation of sorting system, ARDUINO controller has been used to control three conveyor system that consists of dc motor, stepper motor and servo motor [25]. Besides that, another study suggested that a sorting system based on col or by using the ARDUINO microcontroller will prove high efficiency with low cost [26].
Since digital image can be represented in matrix form, therefore MATLAB should be ideal for image process ing as MATLAB has the computing ability of matrixoriented operations. There is four basic image type that MATLAB support which are index image, gray image, RGB image and binary image [27,28]. The most common digital image operations that can be run by toolboxes in MATLAB including Morphological operation, Histogram equalization, Discrete Fourier and Cosine Transform (DFT and DCT), Image Denois ing Filters and lastly Edge Detection operation which include Sobel operator and Prewitt operator. The pur pose of the process of image processing is to prepare the digital image for further processing or to extract the valuable feature from the original image. Fig. 1 shows the block diagram of a digital image process system.
derstanding the natural language, and also heuristic classification [30, 31]. Machine learning which is one of the branches of AI had already grown quickly in almost all technical fields that utilized the usage of computer science and statistics for commercial use as well as in industries [32]. The most common four types of learning in this field are Supervised Learning, Unsupervised Learning, Semi-Supervised Learning, and Reinforcement Learning. The categories which fall upon this field are as shown in Fig. 2.
Artificial intelligence (AI) is a field in computer sci ence for making a machine with the intelligence that has the computational ability in a similar way of human brain works. Some of the applications of AI include computer vision, speech recognition, un
Supervised Learning is the process in which the learning process takes the inputs and the expected outputs to be considered in the calculation and ana lyzing process. Then, the desired output can be ob tained. Therefore, the accuracy of the calculated out put will improve and becomes closer to the desired output. The purpose of Supervised Learning is to conclude the function or mapping of training data in a labeled format [34]. The system will be given an input data of vector x which is the training data and also de sired output labeled as y which is a function of input data that is yet to be determined. An output vector y is a description of each respective input example from input vector x. By combining these two labeled data, a training model can be formed [35].
The process of labeling output vector y is to be done manually for each training example present in the training data. The implementation of Semi-Su pervised Learning is used normally when the labeled data points are of a limited amount while there are a lot of unlabeled data points in the datasets. Both la beled and unlabeled data points will be used by the system to generate a better learning model. Rein forcement Learning is used when less information is available to the model by trial and error to determine the output that gives higher rewards to the system [36]. The Unsupervised Learning will have no known output for the model, only provided with the inputs
labeled as vector x. The algorithm will then calculates and makes an analysis based on the patterns in train ing data to predict the output. The categories under this type of learning are clustering, dimensionally re duction, and anomaly detection.
Unsupervised Learning will have no known output for the model, only provided with the inputs labeled as vector x. The algorithm will then calculates and makes an analysis based on the patterns in training data to predict the output. The categories under this type of learning are clustering, dimensionally reduction, and anomaly detection.
There are different types of sorting mechanisms as previously discussed. There is a sorting mechanism that used pneumatic or hydraulic to segregate the products, using Delta Robot, sorting tray using step per or a servo motor, and also multiple air blowers as in industrial applications. For this system, a step per motor was chosen as it can be moved precisely and accurately based on the design requirement. A 12V stepper motor will be driven by a motor driver ULN 2003 that is normally used as a driver circuit for relays, LED lighting and stepper motor [37, 38]. The microcontroller, ARDUINO Uno was to control both the power window and stepper motor It will receive its supply from a portable laptop that was set up to include a MATLAB program for image processing. A Camera Module OV7670 VGA that interfaced with the ARDUINO was used to capture the chili image. A setup for the MATLAB program will then processed the im age retrieved from the saved folder of the image cap tured using the VGA camera [39, 40, 41].
There are different types of sorting mechanisms as previously discussed. There is a sorting mechanism that used pneumatic or hydraulic to segregate the products, using Delta Robot, sorting tray using stepper or a servo motor, and also multiple air blowers as in industrial applications. For this system, a stepper motor was chosen as it can be moved precisely and accurately based on the design requirement. A 12V stepper motor will be driven by a motor driver ULN 2003 that is normally used as a driver circuit for relays, LED lighting and stepper motor [37, 38]. The microcontroller, ARDUINO Uno was to control both the power window and stepper motor It will receive its supply from a portable laptop that was set up to include a MATLAB program for image processing. A Camera Module OV7670 VGA that interfaced with the ARDUINO was used to capture the chili image. A setup for the MATLAB program will then processed the image retrieved from the saved folder of the image captured using the VGA camera [39, 40, 41].
Classification of chilies can be implemented by using an appropriately designed pathway. Fig. 3 con tained the information for the process of classifica tion of chili.
Classification of chilies can be implemented by using an appropriately designed pathway. Fig. 3 contained the information for the process of classification of chili.
To simplify the design process, a block diagram that presents the overall system was developed as shown in Fig. 5. It can be seen that ARDUINO will act as the main controller for the task. A camera module acted as the sensor for this operation. MATLAB soft ware that included ARDUINO Toolboxes was pro grammed to process the image and classify the chili. The stepper motor will move the sorting tray of the chili based on their color by using the signal received from ARDUINO.
To simplify the design process, a block diagram that presents the overall system was developed as shown in Fig. 5. It can be seen that ARDUINO will act as the main controller for the task. A camera module acted as the sensor for this operation. MATLAB software that included ARDUINO Toolboxes was programmed to process the image and classify the chili. The stepper motor will move the sorting tray of the chili based on their color by using the signal received from ARDUINO.
For automation of sorting system, ARDUINO con troller has been used to control three conveyor sys tem that consists of dc motor, stepper motor and servo motor. Besides that, a sorting system based on color by using the ARDUINO microcontroller will prove high efficiency with low cost. Fig. 4 showed the flowchart of the sorting mechanism process.
For automation of sorting system, ARDUINO controller has been used to control three conveyor system that consists of dc motor, stepper motor and servo motor Besides that, a sorting system based on color by using the ARDUINO microcontroller will prove high efficiency with low cost. Fig. 4 showed the flowchart of the sorting mechanism process.
Image pre processing is a crucial process as it can aid in providing more detailed information for the feature extraction process. This process helps in the improvements of the image.
Image pre-processing is a crucial process as it can aid in providing more detailed information for the feature extraction process. This process helps in the improve ments of the image.
Images of chili will be used as the input data for this project. The images were taken from the real-world image of the chili samples from the conveyor band by using the VGA camera module. The image of the chili will then be used as the training dataset and testing dataset. The chili images taken will then be cropped into the region of interest (ROI).
Images of chili will be used as the input data for th is project. The images were taken from the real world image of the chili samples from the conveyor band by using the VGA camera module. The image of the chili will then be used as the training dataset and testing dataset. The chili images taken will then be cropped into the region of interest (ROI).
ImageThe MATLAB program that was chosen for this proj ect was pattern recognition. It can be done manually by training the data using a pattern recognition tool in MATLAB or by including the parameters of the pro gram in the coding. The architecture of ANN is such that the number of input features for each data point has the same number of nodes of the input layer. The hidden layer of the architecture or the number of nodes is set to ten hidden layers as it is user-defined. The number of nodes in the output layer corresponds to the number of classes (targets), which were also pre-defined in the MATLAB coding. For this project, the number of targeted outputs was set for two class es, red and green chili. The block diagram of the net work is shown in Fig. 7.
Generally, the error reduces after more epochs of training, especially the train data will keep on de creasing until validation data hit the best perfor mance, meaning reaching the lowest possible Mean Square Error (MSE). However, the validation data might start to increase after reaching the best perfor mance, indicating that the network starts overfitting the training data. In the default setup, the network model will stop training the data after producing six consecutive increases or validation errors after reach ing the lowest MSE, represented by the green line. The best performance was taken from the epoch with the lowest validation error.
The performance of the trained network for 20 samples of chili images was 0.232 at epoch 8 as shown in Fig. 8. After hitting the best performance, the threeline which are train, validation, and test data start to pass away from the dotted line (best performance line), indicating that divergence has occurred. After retraining the network several times, this divergence still occurred during the process, it showed that the performance of the model network did not work well with the number of chili images samples that have been chosen.
The best validation performance of the trained net work for 40 samples of chili images was 0.042121 at epoch 20 as shown in Fig. 9. After hitting the best per formance, the three-line which are train, validation, and test data tend to maintain the line near the dotted line (best performance line), indicating that conver gence has occurred. After retraining the network sev eral times, this convergence still occurred during the process, it showed that the performance of the model network did work well with the number of chili im ages samples that have been chosen.
The accuracy of the ANN classifier when the network was trained with 40 samples of chili images was better than when using 20 samples. This shows that a higher number of samples used for training the network has an effect on the efficiency in classifying the chili. In addition, it also predicts more cases correctly even though the number of samples was higher. Hence, the model network was more reliable to be used for
though the number of samples was higher. Hence, the model network was more reliable to be used for further application such as for the use in a real time situation. Besides, the time taken for both network model situations to be trained were about the same with only a difference of 0.01 second. The comparison for the performance of the model network was shown in Fig. 10.
further application such as for the use in a real-time situation. Besides, the time taken for both network model situations to be trained were about the same with only a difference of 0.01 second. The comparison for the performance of the model network was shown in Fig. 10.
Model Network with 40 Samples
Model Network with 40 Samples2
chili images were good. The model network should be tested with a larger dataset to improve the effectiveness and reliability of the classifier. Therefore, these findings showed that the overall accuracy of the network for 40 samples was 85%, which was better than the network trained with 20 samples that had an overall accuracy of 80%. By using a larger dataset that used 40 samples of chili images instead of 20 samples, the classifier will also have a higher number of samples for testing and validation of the model network. Hence, the trained network is more reliable for further application as more data were being trained while maintaining the performance. T
Accuracy
In conclusion, a deep understanding of the processes of constructing a sorting conveyor belt has been provid ed. For the chili classification, the program was designed by using MATLAB software. The program included the image pre-processing, the feature extraction process, and the ANN model network as a pattern recognition classification. The techniques and methods proposed in this study need to be improved as the performance of the classifier can still be enhanced. For future research, the classification of the chili images can be done by us ing other types of classifiers such as Support Vector Ma chines (SVM), or other branches of machine learning such as clustering by using K-means value. Based on the results, the feature extraction methods could also be im proved to achieve a higher percentage of accuracy. The color of the chili images could also be extracted by using CIE lab color spaces, or other spaces such as HSV and HSI. By measuring these color indexes and their statisti cal value, a more accurate threshold can be established. The performance of the classifier also being affected by the quality of the camera that has been used. Thus, by using a higher-quality camera, a clearer chili image can be obtained for processing and color extraction. Lastly, the Gray Level Co-occurrence (GLCM) and Regionprops can be used to determine the shape, texture, and size of the chili. Therefore, the chili can be classified into much more classes depending on the application.
The process of constructing a sorting conveyor in this study takes place from scratch and by using inexpensive materials Furthermore, the important steps in building a classification model network using ANN have been elaborated in this thesis. Therefore, it is clear that image pre processing is a crucial step for further processes to take place in classifying the chili. Proper image processing methods will help to perform a feature extraction process on the image for the classification. Color analysis on the chili image was done to extract the color of the chili by measuring the components of the RGB mean value. These results of extracted features are then being fed as the input for the ANN classifiers. The performance of the trained model network is then compared for a model network using 20 samples of chili images and 40 samples of chili images to find the optimum parameter that affects the overall efficiency in classification.
The process of constructing a sorting conveyor in this study takes place from scratch and by using inexpen sive materials Furthermore, the important steps in building a classification model network using ANN have been elaborated in this thesis. Therefore, it is clear that image pre-processing is a crucial step for further processes to take place in classifying the chili. Proper image processing methods will help to per form a feature extraction process on the image for the classification. Color analysis on the chili image was done to extract the color of the chili by measuring the components of the RGB mean value. These results of extracted features are then being fed as the input for the ANN classifiers. The performance of the trained model network is then compared for a model network using 20 samples of chili images and 40 samples of chili images to find the optimum parameter that af fects the overall efficiency in classification.
Based on the result, both performances of the model network when using 20 samples and 40 sam ples of chili images were good. The model network should be tested with a larger dataset to improve the effectiveness and reliability of the classifier. There fore, these findings showed that the overall accuracy of the network for 40 samples was 85%, which was better than the network trained with 20 samples that had an overall accuracy of 80%. By using a larger da taset that used 40 samples of chili images instead of 20 samples, the classifier will also have a higher num ber of samples for testing and validation of the model network. Hence, the trained network is more relia ble for further application as more data were being trained while maintaining the performance. T
In conclusion, a deep understanding of the processes of constructing a sorting conveyor belt has been provided. For the chili classification, the program was designed by using MATLAB software. The program included the image pre processing, the feature extraction process, and the ANN model network as a pattern recognition classification. The techniques and methods proposed in this study need to be improved as the performance of the classifier can still be enhanced. For future research, the classification of the chili images can be done by using other types of classifiers such as Support Vector Machines (SVM), or other branches of machine learning such as clustering by using K means value. Based on the results, the feature extraction methods could also be improved to achieve a higher percentage of accuracy. The color of the chili images could also be extracted by using CIE lab color spaces, or other spaces such as HSV and H SI. By measuring these color indexes and their statistical value, a more accurate threshold can be established. The performance of the classifier also being affected by the quality of the camera that has been used. Thus, by using a higher quality camera, a clearer chili image can be obtained for processing and color extraction. Lastly, the Gray Level Co occurrence (GLCM) and Regionprops can be used to determine the shape, texture, and size of the chili. Therefore, the chili can be classified into much more classes depending on the application.
The authors would like to thank for the financial sup ports from the Universiti Teknikal Malaysia Melaka (UTeM) under the Center of Research and Innova tion Management (CRIM). This project is also linked with the chili fertigation industry based in Alor Gajah Melaka. The short-term grant number for the project is PJP/2020/FKE/PP/S01747.
M. F. Abdul Aziz – Universiti Teknikal Malaysia Mela ka, Hang Tuah Jaya, 76100 Durian Tunggal, Melaka, Malaysia,
The authors would like to thank for the financial supports from the Universiti Teknikal Malaysia Melaka (UTeM) under the Center of Research and Innovation Management (CRIM). This project is also linked with the chili fertigation industry based in Alor Gajah Melaka. The short term grant number for the project is PJP/2020/FKE/PP/S01747.
W. M. Bukhari* – Universiti Teknikal Malaysia Mela ka, Hang Tuah Jaya, 76100 Durian Tunggal, Melaka, Malaysia, e-mail: bukhari@utem.edu.my,
M. N. Sukhaimie – Melor Agricare PLT, MK 18 Kg Padang, 78000 Alor Gajah Melaka, Malaysia,
T.A. Izzuddin – Universiti Teknikal Malaysia Melaka, Hang Tuah Jaya, 76100 Durian Tunggal, Melaka, Ma laysia,
M.A. Norasikin – Universiti Teknikal Malaysia Mela ka, Hang Tuah Jaya, 76100 Durian Tunggal, Melaka, Malaysia,
A. F. A. Rasid – Universiti Teknikal Malaysia Melaka, Hang Tuah Jaya, 76100 Durian Tunggal, Melaka, Ma laysia,
N. F. Bazilah – Universiti Teknikal Malaysia Melaka, Hang Tuah Jaya, 76100 Durian Tunggal, Melaka, Ma laysia,
*Corresponding author
[1] M. W. Khaing, A. M. Win and D. T. Aye, “Automatic Sorting Machine”, International Journal of Scien ce and Engineering Applications, vol. 7, no. 8, 2018, 138–142.
[2] J. M. Low, W. S. Maughan, S. C. Bee and M. J. Ho neywood, “5 - Sorting by colour in the food in dustry”. In: E. Kress-Rogers and C. J. B. Brime low (eds.), Instrumentation and Sensors for the Food Industry (Second Edition), 2001, 117–136, 10.1533/9781855736481.1.117.
[3] J. A. Kodagali and S. Balaji, “Computer Vision and Image Analysis based Techniques for Automatic Characterization of Fruits A Review”, Interna tional Journal of Computer Applications, vol. 50, no. 6, 2012, 6–12, 10.5120/7773-0856.
[4] S. A. Bini, “Artificial Intelligence, Machine Lear ning, Deep Learning, and Cognitive Computing: What Do These Terms Mean and How Will They Impact Health Care?”, The Journal of Arthropla sty, vol. 33, no. 8, 2018, 2358–2361, 10.1016/ j.arth.2018.02.067.
[5] O. Cruz-Domínguez, J. L. Carrera-Escobedo, C. H. Guzmán-Valdivia, A. Ortiz-Rivera, M. García -Ruiz, H. A. Durán-Muñoz, C. A. Vidales-Basurto and V. M. Castaño, “A novel method for dried chili pepper classification using artificial intelli gence”, Journal of Agriculture and Food Research, vol. 3, 2021, 10.1016/j.jafr.2021.100099.
[6] V. Kakani, V. H. Nguyen, B. P. Kumar, H. Kim and V. R. Pasupuleti, “A critical review on computer vision and artificial intelligence in food indu stry”, Journal of Agriculture and Food Research, vol. 2, 2020, 10.1016/j.jafr.2020.100033.
[7] M. M. Sofu, O. Er, M. C. Kayacan and B. Cetişli, “De sign of an automatic apple sorting system using machine vision”, Computers and Electronics in Agriculture, vol. 127, 2016, 395–405, 10.1016/j. compag.2016.06.030.
[8] N. Khuriyati, D. A. Nugroho and N. A. Wicakso no, “Quality assessment of chilies (Capsicum annuum L.) by using a smartphone camera”. In: IOP Conference Series: Earth and Environ mental Science, vol. 425, 2020, 10.1088/17551315/425/1/012040.
[9] H. J. G. Opeña and J. P. T. Yusiong, “Automated Tomato Maturity Grading Using ABC-Trained Artificial Neural Networks”, Malaysian Journal of Computer Science, vol. 30, no. 1, 2017, 12–26, 10.22452/mjcs.vol30no1.2.
[10] M. R. Fiona, S. Thomas, I. J. Maria and B. Hannah, “Identification Of Ripe And Unripe Citrus Fru its Using Artificial Neural Network”. In: Journal of Physics: Conference Series, vol. 1362, 2019, 10.1088/1742-6596/1362/1/012033.
[11] F. M. A. Mazen and A. A. Nashat, “Ripeness Clas sification of Bananas Using an Artificial Neu ral Network”, Arabian Journal for Science and Engineering, vol. 44, no. 8, 2019, 6901–6910, 10.1007/s13369-018-03695-5.
[12] M. Ataş, Y. Yardimci and A. Temizel, “A new ap proach to aflatoxin detection in chili pepper by machine vision”, Computers and Electronics in Agriculture, vol. 87, 2012, 129–141, 10.1016/ j.compag.2012.06.001.
[13] W. H. M. Saad, S. A. A. Karim, M. S. J. A. Razak, S. A. Radzi and Z. M. Yussof, “Classification and detection of chili and its flower using deep le arning approach”. In: Journal of Physics: Confe rence Series, vol. 1502, 2020, 10.1088/17426596/1502/1/012055.
[14] W. Zhang, J. Mei and Y. Ding, “Design and De velopment of a High Speed Sorting System Ba sed on Machine Vision Guiding”, Physics Pro cedia, vol. 25, 2012, 1955–1965, 10.1016/ j.phpro.2012.03.335.
[15] K. Akila, B. Sabitha, K. Balamurugan, K. Balaji and T. Ashwin Gourav, “Mechatronics System Design for Automated Chilli Segregation”, International Journal of Innovative Technology and Exploring Engineering, vol. 8, no. 8S, 2019, 546–550.
[16] J. Camacho, R. Lewis and R. S. Dwyer-Joyce, “Wear of a chute in a rice sorting machine”, Wear, vol. 263, no. 1, 2007, 65–73, 10.1016/ j.wear.2006.11.052.
[17] B. Jarimopas and N. Jaisin, “An experimental ma chine vision system for sorting sweet tamarind”, Journal of Food Engineering, vol. 89, no. 3, 2008, 291–297, 10.1016/j.jfoodeng.2008.05.007.
[18] S. Sheth, R. Kher and P. Dudhat, “Automatic Sor ting System Using Machine vision”. In: Multi Di sciplinary International Symposium on Control, Automation & Robotics, 2010.
[19] C. Kunhimohammed, K. Muhammed Saifudeen, S. Sahna, M. Gokul and S. U. Abdulla, “Automa ted Color Sorting Machine Using TCS230 Colour Sensor and PIC Microcontroller”, International
Journal of Research and Innovations in Science & Technology, vol. 2, no. 2, 2015.
[20] K. Kumar and S. Kayalvizhi, “Real Time Industrial Colour Shape and Size Detection System Using Single Board”, International Journal of Science, Engineering and Technology Research (IJSETR), vol. 4, no. 3, 2015.
[21] J. Sobota, R. PiŜl, P. Balda and M. Schlegel, “Ra spberry Pi and Arduino boards in control edu cation”, IFAC Proceedings Volumes, vol. 46, no. 17, 2013, 7–12, 10.3182/20130828-3-UK2039.00003.
[22] S. Silva, D. Duarte, R. Barradas, S. Soares, A. Va lente and M. J. C. S. Reis, “Arduino recursive back tracking implementation, for a robotic contest”. In: Human-Centric Robotics, 2017, 169–178, 10.1142/9789813231047_0023.
[23] A. D. Salman and M. A. Abdelaziz, “Mobile Ro bot Monitoring System based on IoT”, Journal of Xi’An University of Architecture & Technolo gy, vol. 12, no. 3, 2020, 5438–5447, 10.37896/ JXAT12.03/501.
[24] K. Nosirov, S. Begmatov, M. Arabboev, T. Kuch korov, J. C. Chedjou, K. Kyamakya, P. De Silva and K. Abhiram, “The Greenhouse Control Ba sed-Vision and Sensors”. In: Developments of Artificial Intelligence Technologies in Compu tation and Robotics, vol. 12, 2020, 1514–1523, 10.1142/9789811223334_0181.
[25] B. B. Krishnan, P. A. M. Kottalil, A. Anto and B. Alex, “Automatic Sorting Machine”, Journal for Research, vol. 2, no. 4, 2016, 66–70.
[26] V. Chakole, P. Ilamkar, R. Gajbhiye and S. Nagrale, “Oranges Sorting Using Arduino Microcontroller (A Review)”, International Research Journal of Engineering and Technology (IRJET), vol. 6, no. 2, 2019, 1800–1802.
[27] X. Meng, “Digital Image Processing Technology Based on MATLAB”. In: Proceedings of the 4th In ternational Conference on Virtual Reality, 2018, 79–82, 10.1145/3198910.3234654.
[28] B. Siemiątkowska and K. Gromada, “A New Ap proach to the Histogram-Based Segmentation of Synthetic Aperture Radar Images”, Journal of Au tomation, Mobile Robotics and Intelligent Systems, 2021, 39–42, 10.14313/JAMRIS/1-2021/5.
[29] A. D. M. Africa and J. S. Velasco, “Development of a Urine Strip Analyzer Using Artificial Neural Network Using an Android Phone”, ARPN Jour nal of Engineering and Applied Sciences, vol. 12, no. 6, 2017, 1706–1713.
[30] J. McCarthy, “What Is Artificial Intelligence?” http://jmc.stanford.edu/articles/whatisai.html, 2007. Accessed on: 2022-08-30.
[31] T. Gevorgyan, “Adoption and inclusion of Artifi cial Intelligence in digitalization strategies of or ganizations,” Master Thesis, Aalborg University, Copenhagen, 2019.
[32] M. I. Jordan and T. M. Mitchell, “Machine learning: Trends, perspectives, and prospects”, Science, vol. 349, no. 6245, 2015, 255–260, 10.1126/ science.aaa8415.
[33] A. Moubayed, M. Injadat, A. B. Nassif, H. Lutfiyya and A. Shami, “E-Learning: Challenges and Rese arch Opportunities Using Machine Learning & Data Analytics”, IEEE Access, vol. 6, 2018, 39117–39138, 10.1109/ACCESS.2018.2851790.
[34] “Use of Decision Trees and Random Forest in Machine Learning an Insight into Supervised Le arning for Classification Problems,” TechVidvan, https://techvidvan.com/tutorials/supervised -learning/. Accessed on: 2022-08-30.
[35] S. Luthra, “Machine Learning: An Automated Le arning Approach”, International Journal of Com puter Engineering and Applications, vol. 12, no. 1, 2018, 156–161.
[36] A. Mehta, “An Ultimate Guide to Understanding Supervised Learning”, https://www.digitalvi dya.com/blog/supervised-learning/. Accessed on: 2022-08-30.
[37] “12V 28BYJ-48 Stepper Motor + ULN2003 Dri ver Board,” Cytron, https://my.cytron.io/p-12v28byj-48-stepper-motor-plus-uln2003-driverboard?r=1&gclid=EAIaIQobChMIq5OnjtCd7gIV Cx4rCh3DQAM3EAQYAyABEgKR5PD_BwE. Ac cessed on: 2022-08-30.
[38] “In-Depth: Control 28BYJ-48 Stepper Motor with ULN2003 Driver & Arduino,” LastMinute Engineers.com, https://lastminuteengineers. com/28byj48-stepper-motor-arduino-tutorial/. Accessed on: 2022-08-30.
[39] “OV7670 VGA Camera Module,” Cytron, https:// my.cytron.io/p-ov7670-vga-camera-module?r= 1&gclid=EAIaIQobChMIsNmo0MWd7gIVyH4rC h2vewohEAQYAyABEgLijfD_BwE. Accessed on: 2022-08-30.
[40] R. Pelayo, “Arduino Camera (OV7670) Tutorial,” https://www.teachmemicro.com/arduino-came ra-ov7670-tutorial/. Accessed on: 2022-08-30.
[41] C. Sirawattananon, N. Muangnak and W. Pukdee, “Designing of IoT-based Smart Waste Sorting
System with Image-based Deep Learning Appli cations”. In: 2021 18th International Conference on Electrical Engineering/Electronics, Computer, Telecommunications and Information Technolo gy (ECTI-CON), 2021, 383–387, 10.1109/ECTI -CON51831.2021.9454826.
Submitted: 27th August 2021; accepted: 28th December 2021
DOI: 10.14313/JAMRIS/4-2021/27
The paper presents results of tests of a simple device, consisting in the location of a source of disturbance emis sion by means of measurements within the near field with manual positioning of the measuring probe. The effectiveness of the source of disturbance location was finally verified by a positive result of the radiated emis sion tests in the EMC laboratory using a standardised method.
Keywords: Electromagnetic compatibility, EMC, near electromagnetic field, radiated emission, near-field probes, sources of disturbance
The ensuring of electromagnetic compatibility (EMC) of the equipment under test (EUT) means that this equipment is immune to electromagnetic disturbances existing in the environment of its operation [1, 2, 3] and can properly operate there, and that the EUT does not generate and emit to this environment electromag netic disturbances [1, 4, 5] at such levels, that could disturb proper operation of other equipment working in the surroundings. The emission of electromagnetic disturbances from the EUT may be intended, i.e., may be an element necessary to ensure its functionality. Such emission exists e.g., in the case of equipment with radio communication, such as a mobile phone, where the transmitted radio waves are an intended effect, necessary to ensure wireless communication. Instead, unintended emission of radiated electromagnetic dis turbances originates as a side effect of the equipment operation (radiating cables, printed circuit tracks, or conducting elements of the housing).
The level of disturbances generated and emitted from the EUT, conducted and radiated, cannot exceed the limits, which are specified in standards applicable to the tested EUT and its operation environment [1, 4, 5], or in very strict requirements for military equip ment [6]. The electromagnetic compatibility plays a big role in the initial stage of the lifecycle, from the development to deployment of electric and electronic devices, because each EUT prior to starting the pro duction should positively pass EMC tests, confirming the compliance with requirements of the EMC direc tive [1]. Without a positive result of tests, the equip ment cannot be placed on the market.
It seldom happens now that even a simple elec tric device would not comprise active semiconductor components and microprocessor circuits in its struc ture, which e.g., take care of proper execution of the programmed functions. In addition, an increasingly great degree of the equipment complication and pro gressing miniaturisation cause that the ensuring of electromagnetic compatibility EMC for the planned device becomes one of more difficult and important tasks for the designer.
Nowadays, it is rare that even a simple electrical device does not have active semiconductor elements and microprocessor systems in its structure, which include they watch over the correct implementation of the programmed functions. In addition, the increas ing complexity of devices and the progressive minia turization make ensuring the EMC electromagnetic compatibility for the designed device one of the more difficult and important tasks for the designer. There fore, we are looking for effective methods of testing the emission of radiated disturbances with the use of relatively inexpensive equipment.
It should be emphasised, that the limits of emis sion levels specified in standards [4, 6] are very strict. Therefore, issues with excessive emission radiated during tests in the EMC laboratory using standardised methods occur so often, especially if from the moment of device prototype designing till the tests the ensur ing of EMC was neglected by the engineer due to time and budget limitations. It also happens that, despite a serious attitude to the ensuring of EMC for the new de vices under development, from the moment of project implementation till the first prototype assembling (ap plication of design recommendations, performance of verification measurements at various project stages), after conducting tests in the EMC laboratory, it turns out that it is necessary to introduce costly changes in the device, mainly due to the excessive radiated emis sion. High purchase costs of professional testing instru ments and limited availability of EMC laboratories as well as high costs of testing cause that the first tests of radiated emission by means of standardised methods are most frequently carried out only on a ready proto type of the device. Major problems occur then, threat ening the entire project success. The problems may consist in e.g., design assumptions mistakes, errors in the electronic system, errors in the housing shielding: metal elements of the housing ‘by chance’ become an tennas, also gaps in the shielded housing can take the role of antennas (radiators) [7, 8].
design assumptions mistakes, errors in the electronic system, errors in the housing shielding: metal elements of the housing ‘by chance’ become antennas, also gaps in the shielded housing can take the role of antennas (radiators) [7, 8].
design assumptions mistakes, errors in the electronic system, errors in the housing shielding: metal elements of the housing ‘by chance’ become antennas, also gaps in the shielded housing can take the role of antennas (radiators) [7, 8].
near electromagnetic field exists at a small distance from the EUT (close to radiating elements in the EUT).
near electromagnetic field exists at a small distance from the EUT (close to radiating elements in the EUT).
In EMC laboratories the emission of radiated electro magnetic disturbances is most frequently measured in SAC (Semi Anechoic Chamber) type chambers by means of an antenna and disturbance receiver, and it is expressed as an amplitude spectrum of electric field E in dBµV/m units, in a selected frequency range. During such measurements the antenna, according to document [9], may be situated at a distance of 3, 5, or 10 m from the tested equipment, depending on the chamber sizes. As a result of such measurements an amplitude spectrum of the electric field is ob tained, determined as the level of radiated emission, and it is referred to emission limits specified in the standards for the assessment of tested equipment conformity with the standard. The obtained results of radiated emission measurements, in the form of an amplitude spectrum graph within the selected frequency range, provide engineers with general in formation, whether the EUT meets the requirements of the standard, or whether the standard limits have been exceeded. If the radiated emission limits are ex ceeded, the information is available on: the level of individual spectrum components, for which frequen cy ranges the excesses have occurred, and for which orientation of the measuring antenna in space (azi muth, polarisation). The information obtained from such measurements is usually not sufficient to find and remove the main reason for exceeding the limits of radiated emission, although it is useful for further analyses and measurements. Therefore, methods for testing the emission of radiated disturbances are necessary, which allow to illustrate in space the dis tribution of electromagnetic field intensity around the tested EUT. The identified highest values of com ponent amplitudes of the spectrum prove a close lo cation of the disturbance source in the EUT, which is the information required to introduce dedicated and right design changes, which should result in the reduction of the radiated emission level, generated by the tested equipment.
In EMC laboratories the emission of radiated electromagnetic disturbances is most frequently measured in SAC (Semi Anechoic Chamber) type chambers by means of an antenna and disturbance receiver, and it is expressed as an amplitude spectrum of electric field E in dBµV/m units, in a selected frequency range. During such measurements the antenna, according to document [9], may be situated at a distance of 3, 5, or 10 m from the tested equipment, depending on the chamber sizes. As a result of such measurements an amplitude spectrum of the electric field is obtained, determined as the level of radiated emission, and it is referred to emission limits specified in the standards for the assessment of tested equipment conformity with the standard. The obtained results of radiated emission measurements, in the form of an amplitude spectrum graph within the selected frequency range, provide engineers with general information, whether the EUT meets the requirements of the standard, or whether the standard limits have been exceeded. If the radiated emission limits are exceeded, the information is available on: the level of individual spectrum components, for which frequency ranges the excesses have occurred, and for which orientation of the measuring antenna in space (azimuth, polarisation). The information obtained from such measurements is usually not sufficient to find and remove the main reason for exceeding the limits of radiated emission, although it is useful for further analyses and measurements. Therefore, methods for testing the emission of radiated disturbances are necessary, which allow to illustrate in space the distribution of electromagnetic field intensity around the tested EUT. The identified highest values of component amplitudes of the spectrum prove a close location of the disturbance source in the EUT, which is the information required to introduce dedicated and right design changes, which should result in the reduction of the radiated emission level, generated by the tested equipment.
In EMC laboratories the emission of radiated electromagnetic disturbances is most frequently measured in SAC (Semi Anechoic Chamber) type chambers by means of an antenna and disturbance receiver, and it is expressed as an amplitude spectrum of electric field E in dBµV/m units, in a selected frequency range. During such measurements the antenna, according to document [9], may be situated at a distance of 3, 5, or 10 m from the tested equipment, depending on the chamber sizes. As a result of such measurements an amplitude spectrum of the electric field is obtained, determined as the level of radiated emission, and it is referred to emission limits specified in the standards for the assessment of tested equipment conformity with the standard. The obtained results of radiated emission measurements, in the form of an amplitude spectrum graph within the selected frequency range, provide engineers with general information, whether the EUT meets the requirements of the standard, or whether the standard limits have been exceeded. If the radiated emission limits are exceeded, the information is available on: the level of individual spectrum components, for which frequency ranges the excesses have occurred, and for which orientation of the measuring antenna in space (azimuth, polarisation). The information obtained from such measurements is usually not sufficient to find and remove the main reason for exceeding the limits of radiated emission, although it is useful for further analyses and measurements. Therefore, methods for testing the emission of radiated disturbances are necessary, which allow to illustrate in space the distribution of electromagnetic field intensity around the tested EUT. The identified highest values of component amplitudes of the spectrum prove a close location of the disturbance source in the EUT, which is the information required to introduce dedicated and right design changes, which should result in the reduction of the radiated emission level, generated by the tested equipment.
Methods for measurements of electromagnetic field intensity, carried out in the near zone, feature such properties; depending on the nature of disturbance source and selected probe they allow to measure the electric component of field, E, or magnetic component of field, H. A near electromagnetic field exists at a small distance from the transmitting antenna. In the case of emission radiated from the EUT, that means that the
Methods for measurements of electromagnetic field intensity, carried out in the near zone, feature such properties; depending on the nature of disturbance source and selected probe they allow to measure the electric component of field, E, or magnetic component of field, H. A near electromagnetic field exists at a small distance from the transmitting antenna. In the case of emission radiated from the EUT, that means that the
Methods for measurements of electromagnetic field intensity, carried out in the near zone, feature such properties; depending on the nature of distur bance source and selected probe they allow to meas ure the electric component of field, E, or magnetic component of field, H. A near electromagnetic field exists at a small distance from the transmitting anten na. In the case of emission radiated from the EUT, that means that the near electromagnetic field exists at a small distance from the EUT (close to radiating ele ments in the EUT).
Fig. 1. Nature of electromagnetic field and the distance from the disturbance source [10]
Fig. 1. Nature of electromagnetic field and the distance from the disturbance source [10]
Fig. 1. Nature of electromagnetic field and the distance from the disturbance source [10]
Fig. 1 presents a graph of wave impedance chang es versus the distance from the disturbance emission source [10], which illustrates changes of the nature of electromagnetic field with increasing distance from the radiated emission source. Simplifying, the zone of near electromagnetic field is situated at a distance from the source, smaller than r, where:
Fig. 1 presents a graph of wave impedance changes versus the distance from the disturbance emission source [10], which illustrates changes of the nature of electromagnetic field with increasing distance from the radiated emission source. Simplifying, the z one of near electromagnetic field is situated at a distance from the source, smaller than r, where:
Fig. 1 presents a graph of wave impedance changes versus the distance from the disturbance emission source [10], which illustrates changes of the nature of electromagnetic field with increasing distance from the radiated emission source. Simplifying, the z one of near electromagnetic field is situated at a distance from the source, smaller than r, where:
���� = ���� 2���� (1)
���� = ���� 2���� (1)
���� = 300 ���� (2)
λ wavelength (m); f frequency (MHz).
���� = 300 ���� (2)
λ wavelength (m); f frequency (MHz).
λ – wavelength (m); f – frequency (MHz).
Around the point r = λ/2π there is a transition area between the near and far field, referred to as the Fresnel zone [11]. In Fig. 1 one can notice, that the field intensity in the near zone goes down with increasing distance from the source, inversely proportionally to the square or third power of the distance, depending on the type of source. In the far field zone the field intensity diminishes inversely proportionally to the distance. In the near field zone the electric or magnetic field becomes dominating. If the field source has low impedance, then in the near zone the magnetic component prevails, while at high impedance of the source the electric component prevails. In the measurement methods in the near electric or magnetic field, the measured quantity is the voltage or current of disturbance induced in the measuring probes of the near field E and H (E electric field, H magnetic field), which in the spectrum analyser are converted into an amplitude spectrum.
(2)
Around the point r = λ/2π there is a transition area between the near and far field, referred to as the Fresnel zone [11]. In Fig. 1 one can notice, that the field intensity in the near zone goes down with increasing distance from the source, inversely proportionally to the square or third power of the distance, depending on the type of source. In the far field zone the field intensity diminishes inversely proportionally to the distance. In the near field zone the electric or magnetic field becomes dominating. If the field source has low impedance, then in the near zone the magnetic component prevails, while at high impedance of the source the electric component prevails. In the measurement methods in the near electric or magnetic field, the measured quantity is the voltage or current of disturbance induced in the measuring probes of the near field E and H (E electric field, H magnetic field), which in the spectrum analyser are converted into an amplitude spectrum.
Around the point r = λ/2π there is a transition area between the near and far field, referred to as the Fresnel zone [11]. In Fig. 1 one can notice, that the field intensity in the near zone goes down with in creasing distance from the source, inversely propor tionally to the square or third power of the distance, depending on the type of source. In the far-field zone the field intensity diminishes inversely proportionally to the distance. In the near-field zone the electric or magnetic field becomes dominating. If the field source has low impedance, then in the near zone the magnet ic component prevails, while at high impedance of the source the electric component prevails. In the meas urement methods in the near electric or magnetic field, the measured quantity is the voltage or current of disturbance induced in the measuring probes of the near field E and H (E – electric field, H – magnetic field), which in the spectrum analyser are converted into an amplitude spectrum.
This paper is aimed at presentation of a location method for radiated disturbance sources in the EUT, with manual positioning of the measuring probe in the near field zone, using an example of a simple tested device EUT, for which negative results of radiated emission measurements in the EMC laboratory have
This paper is aimed at presentation of a location method for radiated disturbance sources in the EUT, with manual positioning of the measuring probe in the near field zone, using an example of a simple tested device EUT, for which negative results of radiated emission measurements in the EMC laboratory have (1)
This paper is aimed at presentation of a location method for radiated disturbance sources in the EUT, with manual positioning of the measuring probe in the near-field zone, using an example of a simple test ed device EUT, for which negative results of radiated
emission measurements in the EMC laboratory have been obtained. The disturbance source within the tested EUT was searched for by carrying out meas urements of electric field E intensity by an E field probe or measurements of magnetic field H intensity by means of an H field probe. The probe is positioned manually in space around the tested EUT. The posi tions of probe arrangement in space are determined manually, measurements of E or H field intensity are made then by a fixed probe. The effectiveness of this location method of a disturbance source, described further on, has been confirmed by the obtained posi tive result of radiated emission tests in the EMC labo ratory. This method, despite some limitations, in cer tain cases may be used as one of engineering tools to locate disturbance sources in the tested prototypes of equipment.
Because, in view of frequently occurring problems with excessive emission of electromagnetic distur bances radiated from equipment prototypes, such measurement methods and techniques are being sought, which would be a tool supporting designer engineers. The tool, which will allow to evaluate the emission radiated from the equipment prototype possibly at any stage of product (equipment) devel opment, i.e., single printed circuit boards (PCB), as sembled models without housing, a prototype in the target housing, as well as the tool, which will facilitate resolving problems identified during the first emis sion tests in the EMC laboratory. For PCBs there are already commercially available tools at an acceptable price, but for finished prototypes, which dimensions exceed the size of a cube with a 40 cm side, there are no effective tools and methods, apart from those standardised, used in EMC laboratories. Hence fur ther on in the paper the properties of a method with manual positioning of the measuring probe have been presented, using a practical example.
Methods for measurements of electromagnetic field intensity in the near zone are used for preliminary verification of the equipment condition from the point of view of level of radiated electromagnetic emission and/or location of disturbance sources. The emission of radiated electromagnetic disturbances is understood as the measured level of electric E com ponent of electromagnetic field intensity, expressed in dBµV/m units. In the case of measuring the mag netic component H of the electromagnetic field, it is converted into the electric component for consistency of units and to facilitate the analysis of signal levels comparison. Measurement methods for the electro magnetic field intensity in the near zone are most frequently used after a previously obtained negative result of tests in the EMC laboratory by a standardised method. Measurement methods in the near zone al low to identify pretty effectively sources of unwanted emission [12, 13, 14], but for simple equipment, with
small dimensions, or printed circuit boards PCB. Mea surements in the near zone may be carried out using a spectrum analyser and a set of near-field probes, moving the probes manually or automatically, using commercially available scanners for EMC tests.
An instrument of the Detectus company is one of com mercial solutions for EMC scanners (Fig. 2), which au tomatically carries out amplitude measurements of spectrum components of electromagnetic field inten sity, using a near-field probe moved automatically at a short distance from the tested object. This solution allows to measure the electromagnetic field intensity from components, cables, PCBs, and entire products in a measuring area of up to 600x400x400mm. The system consists of a numerically controlled robot, moving the measuring probe of E or H field in 3 axes: X-Y-Z. The system is equipped with a set of near-field probes, a spectrum analyser, and a PC with special ised software managing the entire measuring process. During the test the near-field probe is moved by a ro botic arm in the selected range (the measuring grid is defined by the user) above the tested object. At the end of measurement, the user obtains a measurement result in the form of a 3D graph of component ampli tudes of the spectrum of electromagnetic field inten sity, imposed on the tested object, which facilitates the analysis of results. The measurement time with the EMC scanner, depending on the frequency range, selected measuring grid, and the object dimensions, can last even 24 hours.
Fig. 2. EMC scanner – a Detectus RSE series. Source: https://astat-emc.pl/produkty/ urzadzenia-pomiarowe/skaner-emc/seria-rse/
Another type of an EMC scanner is the solution of fered by the Canadian company EMScan, which of fers a family of near-field scanners named EMxpert (Fig. 3). An example of scanner contains a fixed matrix of 1218 magnetic field sensors. The solution is dedi cated for measurements in the near field of electro magnetic field intensity from a PCB with maximum dimensions of 31.6×21.8 cm, with resolution of 3.75 mm, and features a high speed of carried out mea surements. Paper [15] presents studies using such a
scanner to locate a source of unwanted emission (dis turbance source) in a prototype of equipment from the automotive sector. A printed circuit board PCB of a DC-DC converter was the tested equipment. Finally, the effectiveness of disturbance source location and the effectiveness of introduced design changes was verified by the measurement in an SAC type chamber by means of a standardised method (acc. to CISPR 25 standard); a good correlation of results was obtained.
es is verified by the next measurements of emission radiated in the near zone at the same positions of the measuring probe. The assessment of effectiveness consists in the comparison of measurement results obtained prior to changes and after the change im plementation. The result is satisfactory, if levels of spectrum components of the electromagnetic field intensity decreased after the implementation of de sign changes, otherwise the measurements should be continued and the introduced design changes verified until the required effect.
The described method of testing, albeit very time-consuming and requiring a complicated analysis of results, in certain cases gives good results. The ef fectiveness of this method depends on the structured and meticulous attitude to the performance of meas urements and analyses, i.e., on the experience of the person carrying out measurements. An example of effective identification, by means of this method, of emission sources of radiated disturbances is present ed in results of the research further on in this paper.
Fig. 3. EMC scanner – an EMScan EMxpert EHX. Source: https://www.emcfastpass.com
Because of greater affordability of measuring sets, a manual method for probe moving is most frequently used to identify sources of radiated emission. Also, in this solution a spectrum analyser with a set of nearfield probes is used. A measuring probe is moved manually above the equipment under test. In this way amplitudes of spectrum components are mea sured for the signal detected for various areas of the tested equipment. The obtained results of measure ments are presented as spectrum characteristics of the measured value in the selected frequency range, for each position of the measuring probe in relation to the tested equipment. A separate characteristic of the emission spectrum is obtained for each position of the probe. For a large number of measuring probe positions a large number of graphs is obtained, result ing in a time-consuming and complicated analysis. On this basis engineers try to identify circuits, fragments, and elements of the equipment housing, which are unwanted sources of electromagnetic radiation, plac es of maximum levels of signal measured in selected frequency ranges.
The basic drawback of this solution consists in a complicated and time-consuming analysis of results for a large number of measuring points. Moreover, the measurement with a manually moved probe brings about inaccuracies resulting from the need to keep a fixed distance between the probe and the measured element, which is very important for the assessment of the measurement uncertainty. Assuming that sources of unwanted radiated emission have been identified, design changes should be introduced (sys tem changes, shielding, filtering, etc.), to reduce the level of disturbances emitted from the equipment. In the next stage the effectiveness of introduced chang
Standardised methods are known for measurements of electromagnetic radiated emission, carried out in the near-field zone. The document IEC TS 619673:2014 [16] is an example, defining assessment methods for the electric and magnetic component of electromagnetic field in the near zone for integrated circuits installed on any printed circuit board, PCB. The method allows to map E or H components of the near electromagnetic field in a frequency range of up to 6 GHz. The resolution of measurements depends on dimensions of the measuring probe and the precision of the probe positioning system. Paper [17] presents results of studies on the correlation between the re sults obtained by the method presented in the docu ment IEC TS 61967-3:2014 [16] and the results of studies obtained with the use of a TEM chamber acc. to IEC 61967-2 [18].
Other widely known methods, based on measure ments carried out in the zone of near electromagnetic field, used by engineers to resolve problems with exces sive radiated emission or for preliminary assessment of equipment prototypes [11], are not standardised but were published in many scientific papers [11, 12, 1921]. Paper [11] presents general principles of emission measurements performance in the near-field zone and the usability of such measurements in the engineer ing practice. This method in the closest way describes the aspects of the method with manual positioning of probes and is similar to the method described in this paper. Instead, paper [12] presents a method for radi ated emission measurement in the near zone, using a robotic arm positioning the probe in space around the tested equipment in a repeatable way. These measure ments were used to evaluate the shielding. In turn, the method from paper [21] applies to measurements of emission in the near zone, but in relation to integrat
ed circuits. Methods, which are based on the measure ment of electromagnetic field intensity by means of a spectrum analyser with a set of near-field probes and software for the acquisition and visualisation of meas urement data, supported additionally with a system for the measuring probe positioning against the tested equipment, are most frequently used.
The knowledge of fundamental principles of measurements for the emission radiated in the zone of near electromagnetic field is necessary to interpret the obtained results properly. Because the results ob tained in the near field to a large extent depend on the geometry of emission sources and its properties (domination of E or H field) [11]. The complexity of such measurements performance and interpretation of results causes that there are numerous research studies in order to search for effective methods of radiated emission measurements, carried out in the zone of near electromagnetic fields. These studies fo cus on the identification (location) of unwanted emis sion sources [11, 12, 14, 22], and on conversion of results obtained in the near zone into results of emis sion measurements in the far zone, to enable prelim inary assessment of conformity based on the level of electromagnetic emission radiated in the near zone [13], using the emission limits specified in standards and referring to measurements in the far zone.
For example, in paper [12], the radiated emission in the near field was investigated by conducting meas urements using a robot and a magnetic field probe. On this basis a radiation model of the disturbance source has been developed, which was used to predict the ef fectiveness of shielding of equipment enclosures. Con siderations included the method of measuring probe calibration and the influence of the entire measur ing path (antenna coefficient of the probe, amplifier gain, cables attenuation) on the measurement results. The radiated emission by the tested equipment was modelled based on measurements of the near field, in which the radiation sources were presented by means of equivalent magnetic dipoles.
One of literature examples is the study, in which, based on results of measurements obtained by means of a near-field scanner and equivalent measurements of the far field obtained in a semi-anechoic chamber, a statistic relationship between the intensity of mag netic field in the near field and electric field intensity in the far zone was achieved [13]. Based on statistic measurements the limit for the tested EUT, deter mined in the standards for the far field, was convert ed into an equivalent limit in the near field. For such approach statistical measurements were performed for 14 multi-layer PCBs, in which the oscillator fre quencies were 10, 25, and 50 MHz. It was found, that the method features a potential as a tool for prelim inary EUT assessment in terms of radiated emission requirements. Phase measurements were not used in the research, which resulted in under- or over-esti mation of the emission amplitude at certain frequen cies. The author indicated the need for further studies for a larger number of PCB samples, as well as using the phase measurements in research.
Other literature examples, where the issue of the effect of estimation or prediction of measurement re sults of the emission radiated in the near field on the emission radiated in the far field is considered [20, 21, 23, 24], apply mainly to small and simple devices or their fragments (printed circuit boards, radiating integrated circuits, radiating tracks). However, there are no studies, which would try to estimate the results of emission radiated in the near field onto results in the far field, referred to entire and also large and com plex EUTs, closed in shielded casings. Partial issues are considered in the mentioned scientific papers. For example, for the case of a radiating micro-strip line there are attempts to predict the radiated emission in the far field based on emission measurements in the near field [20], and to develop numerical mod els allowing to calculate components of the far-field emission [23]. Another example is the research, re lated to the estimation of measurement results from the near field onto the far field for integrated circuits [21]. Instead, the approach presented in [13] utilises statistical relationships between the emission in the near and far field with regard to the testing of printed circuit boards PCB. There is also a study [24], in which a method based on modelling of disturbance sources by means of an array of dipoles, using the regularisa tion techniques, was applied to develop models used for conversion of results of emission measurements from the near field into the far field. The actual meas urement results of radiated emission, obtained in the near field and in the far field for integrated circuits, were used to develop this method.
The most recent study [14], related to the meas uring systems operating also based on a near-field scanner principle, is directed towards increasing the precision (resolution) of the spatial distribution rep resentation of the near field, for a more precise loca tion of disturbance emission sources in PCB circuits of high packing density.
Apart from methods of scanning in the near elec tromagnetic field, other location techniques for dis turbance sources comprise so-called emission source microscopy (ESM) techniques, in which electromag netic disturbance sources are located via the meas urement of the amplitude and phase of electromag netic field intensity in the far-field zone, at a distance from the equipment determined as a few wavelengths [25, 26]. These methods are fit for location of electro magnetic disturbance sources in complex and large devices, but the authors emphasise, that they still re quire further research.
The research were aimed at identification of radiated emission sources in the equipment based on mea surement results of electric field intensity in the near zone, with the use of manual positioning of the probe. The subject of the research consists of a control and measuring device, used to control parameters of an insulated supply network. Because of the confiden tial nature of results of the research, carried out for
a commercial customer, the actual view of the device and its full name cannot be used in the description of this study. The EUT-1 name will be used.
a)
b)
Fig. 4. a) EUT-1 test object – external view of the housing; b) EUT-1 test – a view of PCB, schematic representation of the source of unwanted radiated emission and of the measuring probe positions (1, 2, 3)
Tests of radiated emission with a standardised meth od in the laboratory in the SAC chamber, at a distance of 3 m from the antenna to the EUT-1, provided the obtaining of radiated disturbance emission in the range of 30–1000 MHz (Fig. 5 and 6). The results were negative, i.e. the limit of radiated emission has been exceeded at both polarisations of the measuring antenna (vertical and horizontal). The negative test results presented in Figs. 5 and 6 are related to mea surements of the emission only for the vertical polari sation of the antenna, because the exceeding of limits was the highest in this configuration.
Fig. 6. Measurement results for emission of radiated disturbance of the EUT-1 object, in the range of 230 –1000 MHz, at the vertical polarisation of the antenna. The limit of PN-EN 55011 standard was exceeded highest for class B at frequencies: 500 MHz and 600 MHz
The analysis of the obtained results has shown, that the exceeding of the limit was observed at the fol lowing frequencies:
• Fig. 5, the range of 30-230 MHz: 50 MHz, 100 MHz, 150 MHz, and 200 MHz;
• Fig. 6, the range of 230-1000 MHz: 500 MHz and 600 MHz; high levels, which did not exceed the limit, were observed at the following frequencies: 250 MHz, 300 MHz, 350 MHz, and 400 MHz.
Based on the list of frequencies, at which high am plitude levels of electric field intensity, resulting in ex ceeding the standard limit, have been observed, it is easy to notice, that the frequency of f0 = 50 MHz was the common divisor, the next cases of limit exceeding are multiplicities of the frequency f0, they are har monics of 50 MHz frequency.
5.2. Measurements in the Near-Field Zone –a Method for the Source of Disturbance Emission Location with Manual Positioning of the Probe
Fig. 7 shows a block diagram of the algorithm pre senting the method for disturbance sources location based on measurements in the near-field zone. This is a general method for proceeding, which was used to locate a disturbance source in the tested equipment after obtaining negative results of radiated emission tests by means of the standardised method in the EMC laboratory. Following the algorithm, the source of dis turbance was then eliminated and the effectiveness of source location and its elimination was confirmed by measurements in the near-field zone and by labo ratory measurements by means of the standardised method.
Fig. 5. Measurement results for emission of radiated disturbance of the EUT-1 object, in the range of 30 –230 MHz, at the vertical polarisation of the antenna. The limit of PN-EN 55011 standard was exceeded highest for class B at frequencies: 100 MHz, 150 MHz, and also 200 MHz and 225 MHz
Fig. 7. Block diagram of the algorithm for location of disturbance emission, taking into account the process of disturbance sources elimination through the introduction of design changes
A trial to locate the source of unwanted emission, applying the method of measurements with the use of a spectrum analyser and a set of near-field probes, with the use of the algorithm from Fig. 7, comprised the following sequence of actions:
1. The configuration of the EUT-1 device was reproduced on a test station, like during the tests in the EMC laboratory (in accordance with the standards), maintaining the same mode of operation and arrangement in space.
2. Preliminary experimental measurements were performed in the near-field zone, aimed at choosing the probe type and selection of measurement places, guided by the best sensitivity of the measuring path.
The method to choose a near-field probe: Based on preliminary experimental measurements, with the working EUT-1 device, it was found that the best effects (the best sensitivity) resulted from the application of an E electric field probe, ball type. Hence further measurements were carried out using this probe.
The selection of measurement places (selection of probe positions grid in relation to the EUT-1): For the EUT-1 device, due to its small dimensions (165 × 90 × 65) mm excluding cables, based on the experimental measurements a decision was made to perform measurements at a distance of 5 cm at three points above the device, as shown in Fig. 9. During the preliminary experimental measurements, it was found, that it is relatively easy to find such a location of the measuring
probe in relation to the tested equipment, in which there is the maximum level of the measured signal (maximum emission) for the frequency of 150 MHz (frequency at which during the tests in the laboratory the emission limit was maximally exceeded – Fig. 5). Hence it was decided to record results of measurements for further analyses only for three locations of the probe close to the EUT-1. If it is not possible to clearly identify the place with the highest level of disturbance emission, the measuring grid should be compressed.
Configuration of the measuring instruments: A PMM 9010 measuring receiver, with a module of PMM 9030 extensions, with a set of near-field probes, and a pre-amplifier and a computer with the software for data acquisition, were used for measurements. During the measurements performance the probe was positioned in space in relation to the tested equipment by means of a stand, made of a non-conducting material. Measurements were carried out by means of a peak value detector (PEAK), at the RBW filter set at 120 kHz (settings like during the measurement in the EMC laboratory, in accordance with the standard); the shape of the spectrum graph was then similar to that obtained by the standardised method during the laboratory measurements, which facilitated the analysis.
3. The selected sphere-type probe of electric field E was used to measure the disturbance emission background on the test station.
4. The EUT-1 device was started and reference measurements were performed in the near the device, in accordance with the determined grid of probe positions (Fig. 4). During the emission measurement at each position the probe was set at a distance of 5 cm from the top point of the housing. An example of measurement results for position 3 of the measuring probe is presented in Fig. 8.
5. The results of measurements recorded in the near zone for 3 determined probe positions were analysed (amplitude spectra were compared for frequencies of the maximum levels occurrence). Figs. 9, 10 and Table 2 present the specification of results. (At this stage, if analyses do not indicate clearly the location of the disturbance source, the grid of measuring probe positions should be compressed).
6. The analysis of recorded results has shown, that the place marked with number 3 in Fig. 4 was the area of the device, at which the maximum amplitude of the radiated emission spectrum was observed. Based on the results of measurements a hypothesis was suggested, that the element or fragment of the circuit, operating at the frequency of 50 MHz, was the main basic source of emission, because the observed high levels of the measured signal were multiplicities of this frequency. The maximum signal amplitudes were registered for 150 MHz and 500 MHz (these are also multiplicities of 50 MHz), hence the frequency
of 50 MHz was chosen for the further analysis, as well as two frequencies, for which the maximum signal amplitude was measured, i.e., 150 MHz and 500 MHz.
For the transparency of the described methodology, further analyses were limited to the frequency range of 30 MHz – 500 MHz, despite the fact that the measurements were carried out in a wider range up to 1 GHz, excluding the (88-110) MHz band, due to a high level of environment disturbances background, caused by the existence of radio station transmitters. Attention should be drawn to the fact, that high emission levels at frequencies above 500 MHz also existed at multiplicities of 50 MHz frequency, but to simplify the analysis, for the needs of this paper, the results from this frequency band were not analysed.
7. The identified area (measurement point 3), with the highest levels of the amplitude of spectrum components of the measured signal at 150 MHz and 500 MHz, was subject to analyses of: the entire device design from the mechanical and electrical point of view, diagrams of electric circuits and tracks in the area of measuring point 3.
Fig. 8. Results of the reference measurement of disturbance emission using a probe of near field E for position 3 of the probe (immediately after testing in the EMC laboratory, without introduction of design changes)
As a result of analyses and consultations with the device designer a hypothesis was suggested, that the 50 MHz oscillator was the source of emission, connected e.g., to the microprocessor system and circuits responsible for ensuring communication via the Ethernet interface. In the tested version of the device this interface was not used or software supported. The device expansion with the Ethernet interface was planned by the producer in an unspecified future, to cut the design costs of a new device version. Hence it has turned out that at the moment of testing the elements, being the source of excessive radiated emission, could have been removed via unsoldering, without a loss of device functionality. The 50 MHz oscillator (Fig. 4b) was removed, and the oscillator tracks were short-circuited with a resistor to the system ground.
Fig. 9. Recorded characteristics of the intensity spectrum of electric field in the near zone for 3 fixed positions of the probe (3rd harmonic of the oscillator frequency has been marked)
suggested hypothesis was confirmed, related to the frequency of the disturbance source; the source of excessive radiated emission was successfully eliminated. As a result, the device was corrected to the situation of conformity with the standard requirement, which has been finally confirmed in the EMC laboratory. Figs. 12 and 13 present the results.
[dBµV/m]
8. The effect of introduced changes was confirmed by the measurement of radiated emission at the same place and for the same settings and EUT-1 configuration, and the measuring instruments at the measuring point (location) 3. Based on the comparison of levels of component amplitudes of the measured signal spectrum (Fig. 11), the suggested hypothesis was confirmed, related to the frequency of the disturbance source; the source of excessive radiated emission was successfully eliminated. As a result, the device was corrected to the situation of conformity with the standard requirement, which has been finally confirmed in the EMC laboratory. Figs. 12 and 13 present the results.
Fig. 8. Results of the reference measurement of disturbance emission using a probe of near field E for position 3 of the probe (immediately after testing in the EMC laboratory, without introduction of design changes)
40 80 120
Probe
2
Fig. 10. Comparison of intensity amplitudes of electric field of emitted disturbances for each of three positions of the measuring probe in relation to the EUT 1
Fig. 10. Comparison of intensity amplitudes of electric field of emitted disturbances for each of three positions of the measuring probe in relation to the EUT-1
Tab. 1 Comparison of measurement results for the electric field intensity, for each of three positions of the measuring probe 105.1 99.83 104.61 90.53 100.37 120.07 89.98 99.51 111.94
Intensity amplitude of electric field
105.1 99.83 104.61
Tab. 1. Comparison of measurement results for the electric field intensity, for each of three positions of the measuring probe
Specification of results
a) b)
Frequencies [MHz]
Intensity amplitude of electric field [dBµV/m]
Point 1 Point 2 Point 3
50 105.1 99.83 104.61
150 90.53 100.37 120.07
500 89.08 99.51 111.94
Fig. 12. Results of measurements of radiated disturbance emission in the far zone of the EUT-1 object, in the range of 30–230 MHz, at the vertical polarisation of the antenna – a positive result
Fig. 11. Results of measurements of radiated disturbance emission in the near zone for the measuring point 3 before design changes and after the changes introduction: a) spectrum within the 30-300 MHz range; b) spectrum for a frequency of 150 MHz
Fig. 13. Results of measurements of radiated disturbance emission in the far zone of the EUT-1 object, in the range of 230–1000 MHz, at the vertical polarisation of the antenna – a positive result
The location method for the source of radiated distur bances, applied in the paper, provided good results; its effectiveness was confirmed by experimental tests. The duration of disturbance source location was relatively short, the entire process took a few hours. However, it is necessary to emphasise, that the analysed case was simple, the tested device was small, featuring a simple structure, with one source of unwanted emission. The difference in the intensity amplitude of elec tric field at a frequency of 150 MHz before and after the introduction of design changes was 42.5 dBµV/m for measurements performed in the near-field zone, while for measurements carried out in the labora tory in the far zone, the difference was similar, i.e. 39.5 dBµV/m (Table 2). Measurements in the near zone were performed above the housing at the place, where the disturbance source was identified.
Tab. 2. Assessment of introduced changes effectiveness, based on the measurement of electric field intensity for a frequency of 150 MHz
Method of emission measurements
Intensity amplitude of electric field for 150 MHz before introduction of changes
[dBµV/m]
Intensity amplitude of electric field for 150 MHz after introduction of design changes (source removal) [dBµV/m]
Difference in the intensity amplitude of electric field before and after introduction of changes for 150 MHz [dBµV/m]
In the near-field zone (Results based on Fig. 11 b).) 120.1 77.6 42.5
Standardised in the EMC laboratory (Results based on Fig. 5 and 12) 56.5 17 39.5
In this method it is very important to prepare a plan of studies: configuration determination of the station, instruments, and the tested equipment, and then it is important to meticulously observe the made assumptions, because measurements of the near field give only relative results, which may be compared with each other, provided that they are performed at the same settings and conditions before and after the introduction of changes in the EUT [11].
In the measurements of radiated electromagnet ic emission in the near-field zone a low level of the electromagnetic background is important in the sur roundings of the place of studies, to be capable of ef fectively carry out measurements and rightly locate the source of disturbances in the tested equipment. Hence it is best to perform the measurements in a shielded room.
The described method of studies is fit for location of disturbance sources in simple and uncomplicat ed equipment. It does not have limitations in terms of the equipment dimensions, but it has also many drawbacks, such as:
time-consuming analysis of the results of studies, because for each measuring point at least one graph is obtained; the more configuration cases – the more graphs, hence the analysis of results becomes complicated, it is required to strictly stick to the test plan and configuration settings, to ensure repeatability, orientation of the probe in space is changed manually, hence the imprecision of the probe orientation in space can result in measurement errors and the distortion of the image of disturbance emission from the device.
Summarising, the location methods for distur bance sources known from the literature, using meas urements in the near-field zone for printed circuit boards PCB [13, 14, 15, 17, 20, 23] and for integrated circuits [21], are a good approach leading to the de sign compliance with the requirements of standards, and later on to a smooth passing through the stage of EMC tests in the laboratory. However, in the case of a more complex equipment, e.g. a mobile robot or an other large-size piece of equipment, which contains many various PCBs, cabling etc., this approach does not guarantee success. Even if all PCBs in the process of designing were verified by scanning methods in the near field, to locate and eliminate all disturbance sources, their integration into one multi-function equipment does not give certainty of obtaining a pos itive results of radiated emission tests in the laborato ry. The more complicated the equipment is, the higher is the risk of radiating from the equipment of distur bances with overly high levels, and the identification of such sources is more difficult.
Considering the current state of knowledge and technology, we are short of methods for disturbance sources location and visualisation for the equipment of larger sizes, and featuring a complicated structure (irregular shapes). So it seems, that the construction of a test station for measurements of radiated emis
sion in the near-field zone, with the idea of operation similar to EMC scanners positioning the measur ing probe in 3 perpendicular axes, but intended for equipment of larger sizes, is a right attitude. However, the issue of optimisation in terms of measurements duration remains to be resolved, as well as selection or development of dedicated measuring probes of the near field, to ensure that the process of disturbance sources location and assessment of the radiated emis sion level, if any, is as effective as possible. Further re search will be carried out in this direction.
This article was written in cooperation with the Fac ulty of Electrical Engineering of the Białystok Univer sity of Technology, Poland, as part of the implementa tion doctorate.
AUTHOR
Krzysztof Trzcinka – Department of Automation and Robotics, ŁUKASIEWICZ Research Network – Indus trial Research Institute for Automation and Measure ments PIAP, Warsaw, Poland, e-mail: krzysztof.trzcin ka@piap.lukasiewicz.gov.pl.
[1] “Directive 2014/30/EU of the European Parlia ment and of the Council of 26 February 2014 on the harmonisation of the laws of the Member Sta tes relating to electromagnetic compatibility (re cast)”, https://eur-lex.europa.eu/legal-content/ EN/TXT/PDF/?uri=CELEX:32014L0030&rid=4. Accessed on: 2022-08-30.
[2] “EN IEC 61000-6-1:2019
Electromagnetic com patibility (EMC) – Part 6-1: Generic standards – Immunity standard for residential, commer cial and light-industrial environments,“ https:// standards.iteh.ai/catalog/standards/clc/1a6d 24ca-4ba7-4d0d-a66f-c3d1f226ca0b/en -iec-61000-6-1-2019 Accessed on: 2022-08-30.
[3]
[4]
“EN IEC 61000-6-2:2019
Electromagnetic com patibility (EMC) – Part 6-2: Generic standards –Immunity standard for industrial environment,“ https://standards.iteh.ai/catalog/standards/ clc/6cfd8fa2-dfaf-459d-8d98-814154d1bfde/en -iec-61000-6-2-2019 Accessed on: 2022-08-30.
“EN IEC 61000-6-3:2021
Electromagnetic com patibility (EMC) – Part 6-3: Generic standards –Emission standard for equipment in residential environments,“ https://standards.iteh.ai/cata log/standards/clc/a145bf06-c5b5-4e0d-9af3 -dd51c414b7f9/en-iec-61000-6-3-2021 Acces sed on: 2022-08-30.
[5] “EN IEC 61000-6-4:2019 Electromagnetic com patibility (EMC) – Part 6-4: Generic standards –Emission standard for industrial environments,“ https://standards.iteh.ai/catalog/standards/ clc/70229e6a-bc18-4d7d-81f7-96babbfea9ec/ en-iec-61000-6-4-2019 Accessed on: 2022-08-30.
[6] “NO-06-A200:2012 Kompatybilność elektroma gnetyczna. Poziomy dopuszczalne emisji ubocz nych i odporności na narażenia elektromagne tyczne”. (in Polish)
[7] H. W. Ott, Noise Reduction Techniques in Elec tronic Systems, 2nd Edition, Wiley-Interscience, 1988.
[8] D. J. Bem, Anteny i rozchodzenie się fal radiowych, WNT Warszawa, 1973. (in Polish)
[9] “CISPR 16-2-3:2016 Specification for radio di sturbance and immunity measuring apparatus and methods – Part 2-3: Methods of measure ment of disturbances and immunity – Radiated disturbance measurements,“ https://standards. iteh.ai/catalog/standards/iec/645f733a-84a9 -4ec8-988b-7f1852e118da/cispr-16-2-3-2016 Accessed on: 2022-08-30.
[10] T. MacNamara, Handbook of Antennas for EMC, 2nd ed., Artech House, 2018.
[11] V. Kraz, “Near-Field Methods of Locating EMI So urces,” https://www.ramayes.com/download/ OnFILTER/OnFILTER-Near-Field-Methods-ofLocating-EMI-Sources.pdf, 1995. Accessed on: 2022-08-30.
[12] F. Benyoubi, M. Feliachi, Y. Le Bihan, M. Ben setti and L. Pichon, “Implementation of tools for electromagnetic compatibility studies in the near field”. In: 2016 International Confe rence on Electrical Sciences and Technologies in Maghreb (CISTEM), 2016, 1–6, 10.1109/CI STEM.2016.8066810.
[13] K.-Y. See, N. Fang, L.-B. Wang, W. Soh, T. Svimo nishvili, M. Oswal, W.-Y. Chang and W.-J. Koh, “An Empirical Approach to Develop Near-Field Li mit for Radiated-Emission Compliance Check”, IEEE Transactions on Electromagnetic Compa tibility, vol. 56, no. 3, 2014, 691–698, 10.1109/ TEMC.2014.2302003.
[14] J. Wang, Z. Yan, C. Fu, Z. Ma and J. Liu, “Near-Field Precision Measurement System of High-Density Integrated Module”, IEEE Transactions on Instru mentation and Measurement, vol. 70, 2021, 1–9, 10.1109/TIM.2021.3078000.
[15] A.-M. Silaghi, R.-A. Aipu, A. De Sabata and P.-M. Ni colae, “Near-field scan technique for reducing ra diated emissions in automotive EMC”. In: 2018 IEEE International Symposium on Electromagnetic Com
patibility and 2018 IEEE Asia-Pacific Symposium on Electromagnetic Compatibility (EMC/APEMC), 2018, 831–836, 10.1109/ISEMC.2018.8393897.
[16] “IEC TS 61967-3:2014 Integrated circuits – Me asurement of electromagnetic emissions – Part 3: Measurement of radiated emissions – Surface scan method,“ https://standards.iteh.ai/cata log/standards/iec/806cb255-99c1-4aca-ac84 -8db6623bf6e0/iec-ts-61967-3-2014 Accessed on: 2022-08-30.
[17] G.-Y. Cho, J. Jin, H.-B. Park, H. H. Park and C. Hwang, “Assessment of Integrated Circuits Emissions With an Equivalent Dipole-Moment Method”, IEEE Transactions on Electromagne tic Compatibility, vol. 59, no. 2, 2017, 633–638, 10.1109/TEMC.2016.2633332.
[18] “EN 61967-2:2005 Integrated circuits – Me asurement of electromagnetic emissions, 150 kHz to 1 GHz – Part 2: Measurement of radia ted emissions – TEM cell and wideband TEM cell method,“ https://standards.iteh.ai/catalog/ standards/clc/41042894-a36d-4123-b1cd1e85986b06ee/en-61967-2-2005 Accessed on: 2022-08-30.
[19] W. Liu, Z. Yan, J. Wang, Z. Ning and Z. Min, “Ultra wideband Real-Time Monitoring System Based on Electro-Optical Under-Sampling and Data Acquisition for Near-Field Measurement”, IEEE Transactions on Instrumentation and Measure ment, vol. 69, no. 9, 2020, 6603–6612, 10.1109/ TIM.2020.2968755.
[20] H. Weng, D. G. Beetner and R. E. DuBroff, “Pre diction of Radiated Emissions Using Near-Field Measurements”, IEEE Transactions on Electro magnetic Compatibility, vol. 53, no. 4, 2011, 891–899, 10.1109/TEMC.2011.2141998.
[21] Z. Yu, J. A. Mix, S. Sajuyigbe, K. P. Slattery and J. Fan, “An Improved Dipole-Moment Model Ba sed on Near-Field Scanning for Characterizing Near-Field Coupling and Far-Field Radiation From an IC”, IEEE Transactions on Electromagne tic Compatibility, vol. 55, no. 1, 2013, 97–108, 10.1109/TEMC.2012.2207726.
[22] R. Zaridze, V. Tabatadze, I. Petoev, D. Kakulia and T. Tchabukiani, “Emission source localization using the method of auxiliary sources”. In: 2016 International Symposium on Electromagnetic Compatibility – EMC EUROPE, 2016, 829–834, 10.1109/EMCEurope.2016.7739167.
[23] W. Liu, Z. Yan and Z. Min, “The Far-field Estima tion for Microstrip Line Based on Near-field Scan ning”. In: 2018 12th International Symposium on Antennas, Propagation and EM Theory (ISAPE), 2018, 1–4, 10.1109/ISAPE.2018.8634064.
[24] J. Wang, Z. Yan, W. Zhang, T. Kang and M. Zhang, “An effective method of near to far field transfor mation based on dipoles model”. In: 2015 Asia -Pacific Microwave Conference (APMC), vol. 3, 2015, 1–3, 10.1109/APMC.2015.7413331.
[25] S. Yong, S. Yang, L. Zhang, X. Chen, D. J. Pomme renke and V. Khilkevich, “Passive Intermodu lation Source Localization Based on Emission Source Microscopy”, IEEE Transactions on Elec tromagnetic Compatibility, vol. 62, no. 1, 2020, 266–271, 10.1109/TEMC.2019.2938634.
[26] L. Zhang, V. V. Khilkevich, X. Jiao, X. Li, S. Toor, A. U. Bhobe, K. Koo, D. Pommerenke and J. L. Drewniak, “Sparse Emission Source Mi croscopy for Rapid Emission Source Imaging”, IEEE Transactions on Electromagnetic Compa tibility, vol. 59, no. 2, 2017, 729–738, 10.1109/ TEMC.2016.2639526.
Submitted: 18th April 2021; accepted: 9th February 2022
Aristide Ndayikengurukiye, Abderrahmane Ez-Zahout, Akou Aboubakr, Youssef Charkaoui, Omary Fouzia
DOI: 10.14313/JAMRIS/4-2021/28
Recommender systems (RS) have emerged as a means of providing relevant content to users, whether in social networking, health, education, or elections. Further more, with the rapid development of cloud computing, Big Data, and the Internet of Things (IoT), the component of all this is that elections are controlled by open and accountable, neutral, and autonomous election man agement bodies. The use of technology in voting pro cedures can make them faster, more efficient, and less susceptible to security breaches. Technology can ensure the security of every vote, better and faster automatic counting and tallying, and much greater accuracy. The election data were combined by different websites and applications. In addition, it was interpreted using many recommendation algorithms such as Machine Learning Algorithms, Vector Representation Algorithms, Latent Factor Model Algorithms, and Neighbourhood Meth ods and shared with the election management bodies to provide appropriate recommendations. In this paper, we conduct a comparative study of the algorithms ap plied in the recommendations of Big Data architectures. The results show us that the K-NN model works best with an accuracy of 96%. In addition, we provided the best recommendation system is the hybrid recommendation combined by content-based filtering and collaborative filtering uses similarities between users and items.
Keywords: Cloud computing, Big Data, IoT, Recommend er system and KNN algorithm
Nowadays, many countries are trying to put in place laws to make it easier for the public to express their opinion on a given issue and especially to make a choice of their leaders through voting. The conven tional procedure is manual or paper voting which sometimes does not facilitate counting and is not convenient for voters which sometimes decreases the voting rate. With the evolution of information and communication technologies, electronic voting has become a necessity to overcome these problems and facilitate voting for any geographical area to partici pate.
This technological evolution has generated an ex plosion of a large mass of digital data, which makes it imperative to find solutions to enable adequate
storage and analysis, thus giving rise to the concept of Big Data. Indeed, Big Data requires enormous storage and processing capacities that conventional methods cannot offer. To promote the deployment of Big Data comes the concept of Cloud Computing which allows access and use of computer resources such as storage and network on demand. In other words, it provides users with computing services that allow them to save and manipulate data, install models and access mul tiple resources on the web, according to their needs. One of the most advantageous features of the cloud is its elasticity, as it allows computing capacity to be adapted to demand and only the necessary resourc es to be allocated [1]: The most popular cloud-based services include Amazon web services, SAP (System Applications and Products), Oracle, Cloudera, Data bricks, etc.
With this rapid increase in the volume of digital data on websites and applications worldwide has led to the problem of data overload which makes it dif ficult to extract relevant information. As a response to this problem, optimisation algorithms and recom mender systems have been proposed and these var ious services mentioned above serve as a platform for the development and evaluation of recommender systems.
When search engines are not sufficient to process this large amount of online information, recommend er systems try to guess what information or goods and services we really need. Any recommender sys tem needs to know a user profile that, for example, contains the user’s preferences. This profile can be ac quired implicitly by analyzing the user’s past behav ior, or explicitly by asking the user about their pref erences. In this case, recommender systems that take into account a community of users are often called collaborative approaches [2], while those based on the description of articles are called content-based approaches [3]. The hybrid approach [4] is a combi nation of two different methodologies or systems that aims to create a new model and is strongly rooted in information retrieval and information filtering. User preferences and article descriptions are somehow compatible so that it is possible to compare them and find an article that matches the user’s preferences.
One of the central issues in recommender systems is how to model and then compare user preferences and item descriptions in a flexible and natural way. Obviously, user preferences, as well as item descrip tions, can be imprecise, incomplete, subjective, and of
different importance. The first approach is based on the use of the fuzzy set theory adopted by Yager [5]. Another approach, which uses fuzzy clustering as a tool for a recommendation, was presented in [6].
Throughout political elections, voters have to make a decision about who to vote for. This decision has become particularly difficult in recent times when much more attention is paid to personalities, images, and campaign events than to party manifestos and policy issues. In addition, various applications have been created to help voters easily pick their favorite candidates. The general idea of these systems is to put x on the symbols of the two candidates. Finally, these choices are compared to select the candidates that are accessible.
In this paper, we present an overview of the differ ent algorithms used in recommender systems and the different tools used in Big Data analysis. We also make a comparative study of some of these algorithms in recommender systems applied to a vote.
The rest of this article is organized as follows. Sec tion 2 presents the different tools used in Big Data analysis and a brief overview of recommender sys tems. Section 3 presents some of the work done on this topic. Section 4 presents the methodology used in our work. Section 5 presents the results obtained in this article. We end with a conclusion.
HDFS. Hadoop Distributed File System (HDFS) is an architecture that consists of NameNode which is a server that regulates file access by clients and man ages the file system namespace. HDFS allows user data to be stored in files through a file namespace. In HDFS the opening, closing, and renaming of files and directories are operations performed by the Na meNode and also determines the mapping of blocks to Datanodes [4].
HDFS has the ability to scale to applications with datasets ranging in size from a few gigabytes to a few terabytes. It can also scale to hundreds of nodes in a single cluster and can provide high bandwidth for ag gregated data [7].
Spark. In advanced data analysis in Big Data, Spark is the most widely used in-memory distributed process ing framework. The reasons for its success include its Application Programming Interface (API) and a set of features that allow querying large amounts of data us ing Structured Query Language (SQL) to distill com plex Machine Learning (ML) models using the most popular algorithms. Spark also reduces the execu tion time of an application by reducing the number of read/write operations on the disk [5].
Despite these advantages, it can mask some of the problems caused by a distributed code execution mechanism, making testing inefficient. Creating Dock ers containers is the most efficient way to create a test environment that resembles the cluster environment. The advantage of this approach is that applications can be tested on different versions of the Framework by simply changing some parameters in the configu ration file [6].
Another advantage is that it is designed to be run on different types of clusters. Cluster managers such as Yet Another Resource Negotiator(YARN), the Ha doop Platform Resource Manager, Mesos, or Kuber netes are supported by a spark [4]. The figure shows the architecture of HDFS [3].
MapReduce. The huge amount of data provides com panies with many opportunities. One of the biggest problems is to be able to process it efficiently on tradi tional systems and therefore to turn to new solutions specifically designed for this purpose.
MapReduce makes data processing easier. It breaks down massive volumes of data into smaller chunks. These pieces of data are then processed in parallel on hadoop servers. Afterward, it sends the consolidated result to the application [8].
With the evolution of technology and especially the advent of the web, the amount of data to be exploited and processed has become very large, making it dif ficult to search and find. One of the computer tech niques that has been developed to extract relevant information is recommendation systems. They allow the user to be guided through the data in order to find the most relevant data.
In RS, the usefulness of the item is usually repre sented by a score that indicates how a user liked a par ticular item. The problem that arises is to solve the esti mation of scores for items that have not been evaluated by the user. Whenever it is possible to estimate scores for items that have not yet been evaluated, users are recommended items with a higher estimated score [9].
Fig. 1. Architecture of HDFSmeasuring the rather than formula ���������������� (6)
weight: systems achieve better filtering and technique studied in education[15], much of systems or was mainly that uses source for a system from the their mobile and builds a objects. These personalized authors used their work, works on users, objects, device that is needs and the users, characteristics of users selecting in [19] these services they are recommendations, analysis using allowed them services relationships
in [19]. proposed a recommendation system for these services to help different cloud users better find what they are looking for. To generate reliable recommen dations, they adopted the formal fuzzy concept analy sis using the lattice representation. This method al lowed them to transform the repository of different cloud services into a set of small clusters, where re lationships between high-quality services and highquality services are highlighted.
between high quality services and high quality services are highlighted.
The process of designing the recommendation system with the progression of Internet of Things (IoT), this case of cloud computing is evolving rapidly using clus ters. He gives elasticity to users of associated informa tion technology (IT) services to save and manage data, install models and easily provide recommendations as and when the best needs. On the other hand, we have proposed a hybrid recommendation approach to the context to get the candidates are selected.
The process of designing the recommendation system with the progression of Internet of Things (IoT), this case of cloud computing is evolving rapidly using clusters. He gives elasticity to users of associated information technology (IT) services to save and manage data, install models and easily provide recommendations as and when the best needs. On the other hand, we have proposed a hybrid recommendation approach to the context to get the candidates are selected.
Our approach combines two different methods: content filtering and collaborative filtering. Each method was adapted to a stage-specific to the voter. First, the approach contained attempts to create a profile used to predict ratings on unseen items. Sec ondly, the collaborative approach uses the products using user notes (explicit or implicit) from the given history. It works in a database of user preferences for the items (Items) that are similar. Finally, we propose a more efficient recommendation system that is based on the hybrid recommendation to put to eliminate the inconvenience in our born gift. Fig. 3. shows the meth odology of our work.
Our approach combines two different methods: content filtering and collaborative filtering. Each method was adapted to a stage specific to the voter. First, the approach contained attempts to create a profile used to predict ratings on unseen items. Secondly, the collaborative approach uses the products using user notes (explicit or implicit) from the given history. It works in a database of user preferences for the items (Items) that are similar. Finally, we propose a more efficient recommendation system that is based on the hybrid recommendation to put to eliminate the inconvenience in our born gift. Fig. 3. shows the methodology of our work.
Furthermore, we have implemented fourteen al gorithms, but the best respectively of them are: K-NN, K-NN with GridSearchCV, SVM, and Decision Tree (DT) Classifier of precisions: 96%, 96%, 92% and 89%, such that the procedures of each algorithm is written:
Furthermore, we have implemented fourteen algorithms, but the best respectively of them are: K NN, K NN with GridSearchCV, SVM, and Decision Tree (DT) Classifier of precisions: 96%, 96%, 92% and 89%, such that the procedures of each algorithm is written:
Algorithm SVM. SVM (Support Vector Machine) is supervised learning model with associated learning algorithms that analyze data for classification and re gression analysis.
Algorithm: SVM
Input: Determine the various training and test data Output: Determine the calculate accuracy
Select the optimal value of cost and gamma for SVM While (stopping condition is not met) do
1. Implement SVM train step for each data point. 2. Implement SVM classify for testing data point. End while
Algorithm Decision Tree Classifier. The decision tree algorithm belongs to the family of supervised machine learning algorithms
Algorithm: Decision Tree
Input: S, where S= set of classified instances Output: Decision Tree Require: S
, num_attibutes > 0
Algorithm K NN. The K Nearest Neighbors (K NN) algorithm is a machine learning algorithm that belongs to the class of simple and easy to implement supervised learning algorithms that can be used to solve classification problems and regression.
Algorithm K-NN. The K-Nearest Neighbors (K-NN) algorithm is a machine learning algorithm that be longs to the class of simple and easy to implement su pervised learning algorithms that can be used to solve classification problems and regression.
Input: the training set D, test objet x, category label set C.
Procedure Build Tree
Repeat
splitA
Entropy(Attributes)
Output: the category c(x) of test object x, c(x) belongs to C.
1. Begin
Input: the training set D, test objet x, category label set C. Output: the category c(x) of test object x, c(x) belongs to C. 1. Begin 2. For each y belongs to D calculate the distance D(y,x) between y and z. end for
2. For each y belongs to D calculate the distance D(y,x) between y and z. end for
3. Select the subset N from the data set D, the N contains k training samples which are the k narest neighbors of the test sample x.
3. Select the subset N from the data set D, the N contains k training samples which are the k narest neighbors of the test sample x.
End
End for
Partition(S,splitA)
Until all partitions processed
End procedure
4. Calculate the category of x:
4. Calculate the category of x:
5. End
End
We evaluated and compared the performance of sev eral recommendation algorithms. We conducted an experimental study on Databricks and Jupyter note book, a publicly available election dataset, to analyse
Based on the results, we can clearly observe that the K-NN and SGDC GridSearchCV outperformed all the other classifiers. In the future, we will use those classifiers in our smart Recommender system, where personalized recommendations would be generated to each user based on his condition voting or not voting.
Tab. 1. Comparison between the algorithms by the metrics: precision, recall, F1-score, and accuracy
Algorithm Precision Recall Fi-score Accuracy
Rocchio’s 0.77 0.56 0.66 0.66
KNN 0.96 0.96 0.96 0.96
that admits colossal values in terms of errors, which induces slope one represents the best algorithm that better explains our variable which moreover pre sents an MSE of 0.59<1, RMSE of 0.768<1, and MAE= 0.760<1.
The rest of the curve is made up of the precision and recall values for the threshold values which are between 0 and 1. Our goal is to make the curve as close as (1,1), which means a good precision and a good recall which is in the first curve of Fig. 4. in class 11 of area 87%.
SGDC GridSearch
CV 0.96 0.96 0.96 0.96
DTC 0.88 0.89 0.88 0.89
SVM 0.85 0.92 0.89 0.92
Tab. 2. This table shows the comparison between the algorithms by the metrics: MSE, RMSE, MAE, and AUC
Algorithm MSE RMSE MAE AUC
Rocchio’s 22.893 4.784 2.17 0.96
KNN 1871268587.5 43258.16 565.19 1.0
SGDC GridSearch
CV 195255897.58 12460.17 429.92 1.0
Naïve Bayes 231533.567 481.17 16.638 0.99
DTC 231514.49 481.15 15.831 0.89
SVM 0.85 0.92 0.89 0.92
LR 1.0 1.0 1.0 1.0
ANN 0.991 0.9995 0.01 0.5
Tab. 3. This table shows the comparison between the algorithms by the metrics: MSE, RMSE, MAE, and MAE
Algorithm MSE RMSE MAE
Slope One 0.59 0.768 0.760
NMF 40110374.3 2002.608221 2002.56
SVD 4010369.24 2002.608225 2002.56
SVDpp 231533.567 481.17 16.638
According to Table 3, the slope one algorithm has the lowest errors in MSE, RMSE and MAE compared to all other algorithms followed by the SVDpp algo rithm. It can be seen that the SVD algorithm is the one
In the Second curve Fig. 5., when 0.5 < AUC <1, there is a good chance that the classifier is able to distinguish positive class values from negative class values. This is because the classifier is able to detect more numbers of true positives and true negatives than false negatives and false positives. In our case, we have a multi-class binary classification problem using the technique One vs All. For this, the best class of ROC is Class 8 of the 96% area.
In Fig. 6., we have AUC = 1, then the classifier is able to distinguish perfectly all the positive and neg ative class points correctly. If, however, the AUC had been 0, then the classifier would have predicted all the negatives as positive and all the positives as neg ative.
In Fig. 8., we have AUC = 1, then the classifier is able to distinguish perfectly all the positive and negative class points correctly. If, however, the AUC had been 0, then the classifier would have predict ed all negatives as positives and all positives as neg atives.
In Fig. 7, we have AUC = 1, then the classifier is able to distinguish perfectly all the positive and negative class points correctly. If, however, the AUC had been 0, then the classifier would have predicted all negatives as positives and all positives as negatives.
Fig. 9. shows that we can clearly see that the train ing score is always around the maximum of 1.0 and that the validation score, could be increased with more training samples of 0.88.
In Fig. 10., we can see clearly that the training score is still around the maximum of score 0.92 and the validation score could be increased with more training samples of the maximum score is 0.92.
Fig. 11. clearly shows that the training score is al ways around the maximum of 0.0 and that the valida tion score could be lowered with more training sam ples.
In Fig. 13., we have AUC = 0.5, then the classifier is not able to distinguish between the Positive and Negative class points. This means that the classifier predicts a random class or a constant class for all data points.
In Fig. 12., we use this curve to select the optimal number of clusters by fitting the model with a range of values for K in the K-means algorithm. We have shown a line drawn between SSE (Sum of Squared Errors) and the number of clusters to find the point representing the “elbow point” (this is a point after which the SSE or inertia starts to decrease linearly). The point on the SES / Inertia graph where the SES or inertia starts to decrease linearly is the elbow point. In this Fig. 12., it can be noted that it is at the number of clusters = 6 that the SSE starts to decrease linearly.
Fig. 14., shows that the training score is still around the maximum score of 1.0 and the validation score could be increased with more training samples of the maximum score is 1.0.
In Fig. 15, we get the index of the first five prod ucts correlated with the product Id chosen by the user, then we see the ratings that the user could give to these 5 most correlated products by made.
The growth of IoT devices generating a large amount of data has made cloud computing an indispensable technology to facilitate data storage, processing, anal ysis and easy access. As the usage is diversified, it is very important that it is always in an optimal way. Sev eral methods such as recommender systems can be used to improve its use. Recommender systems are known to provide relevant suggestions to a user based on his preferences or needs. However, with the rapid progress of technology. Therefore, current recom mender systems rely on the Internet of Things (IoT) to achieve certain goals. In addition, there is too much information available on the Internet that needs to be analyzed or calculated for recommendation purposes. However, with the increase in the volume of data, the system performance gradually degrades. Therefore, a distributed environment such as the cloud is needed to perform and store all the computations on a single system. Based on this study, we observe that all the above areas are interdependent.
In this work, we have a comparative study on the performance of fourteen models applied to the elec tion dataset among others: Rocchio, Latent Semantic Analysis, K-Nearest Neighbors, Naï�ve Bayes, Decision Tree Classifier, Support Vector Machine, Linear Re gression, K-means, Slope One, Non-matrix Factoriza tion, Singular Decomposition Vector, Co-clustering, Pearson Correlation and Artificial Neural Network. The evaluation of the algorithms is based on preci sion, recall, f1-score, accuracy, RMSE, MSE, MAE and area under the curve. The results, shows that K-NN and SGDC GridSearchCV perform best in terms of all evaluation parameters.
Aristide Ndayikengurukiye* – Mohammed V Univer sity, Rabat, Morocco. e-mail: lemure.dede@gmail.com.
Abderrahmane Ez-Zahout – Mohammed V Universi ty, Rabat, Morocco. e-mail: abderrahmane.ezzahout@ um5.ac.ma.
Akou Aboubakr – Mohammed V University, Rabat, Morocco. e-mail: aboubakar_aakaou@um5.ac.ma.
Youssef Charkaoui – Mohammed V University, Ra bat, Morocco. e-mail: youssef_charkaoui@um5.ac.ma.
Omary Fouzia – Mohammed V University, Rabat, Mo rocco.
* Corresponding author
REFERENCES
[1] R. M. Frey, R. Xu and A. Ilic, “A Novel Recommen der System in IoT”. In: 2015 5th International Conference on the Internet of Things (IOT 2015), 2015, 10.3929/ETHZ-A-010561395.
[2] L. Mathiassen, “Collaborative practice research”, Information Technology & People, vol. 15, no. 4, 2002, 321–345, 10.1108/09593840210453115.
[3] R. P. Adhikary, “Effectiveness of Content–based Instruction in Teaching Reading”, Theory and Practice in Language Studies, vol. 10, no. 5, 2020, 10.17507/tpls.1005.04.
[4] S. Sharma, A. Sharma, Y. Sharma and M. Bhatia, “Recommender system using hybrid approach”. In: 2016 International Conference on Computing, Communication and Automation (ICCCA), 2016, 219–223, 10.1109/CCAA.2016.7813722.
[5] H. J. Zimmermann, “Fuzzy set theory”, Wiley In terdisciplinary Reviews: Computational Statistics, vol. 2, no. 3, 2010, 317–332, 10.1002/wics.82.
[6] A. Gosain and S. Dahiya, “Performance Analysis of Various Fuzzy Clustering Algorithms: A Re view”, Procedia Computer Science, vol. 79, 2016, 100–111, 10.1016/j.procs.2016.03.014.
[7] T. Di Noia, R. Mirizzi, V. C. Ostuni and D. Romito, “Exploiting the web of data in model-based re commender systems”. In: Proceedings of the sixth ACM conference on Recommender systems - RecSys ‘12, 2012, 253–256, 10.1145/2365952.2366007.
[8] A. A. Abdallat, A. I. Alahmad, D. A. A. Amimi and J. A. AlWidian, “Hadoop MapReduce Job Schedu ling Algorithms Survey and Use Cases”, Modern Applied Science, vol. 13, no. 7, 2019, 10.5539/ mas.v13n7p38.
Fig. 15. Results of the hybrid approach[9] M. Uta, A. Felfernig, V.-M. Le, A. Popescu, T. N. T. Tran and D. Helic, “Evaluating recommender systems in feature model configuration”. In: Pro ceedings of the 25th ACM International Systems and Software Product Line Conference – Volume A, 2021, 58–63, 10.1145/3461001.3471144.
[10] N. Bhalse and R. Thakur, “Algorithm for movie recommendation system using collaborative filtering”, Materials Today: Proceedings, 2021, 58–63, 10.1016/j.matpr.2021.01.235.
[11] K. Inagaki, S. Takaki, Y. Honda, M. Inoue, N. Mori, H. Kawakami, Y. Kawakami, M. Kawakami, H. Okamoto, K. Tuji, M. Tuge and K. Chayama, “A case of hepatitis E virus infection in a patient with primary biliary cholangitis”, Acta Hepato logica Japonica, vol. 58, no. 3, 2017, 183–190, 10.2957/kanzo.58.183.
[12] M. Pazzani and D. Billsus, “Learning and Revi sing User Profiles: The Identification of Intere sting Web Sites”, Machine Learning, vol. 27, no. 3, 1997, 313–331, 10.1023/A:1007369909943.
[13] H. Christian, M. P. Agus and D. Suhartono, “Single Document Automatic Text Summarization using Term Frequency-Inverse Document Frequen cy (TF-IDF)”, ComTech: Computer, Mathematics and Engineering Applications, vol. 7, no. 4, 2016, 285–294, 10.21512/comtech.v7i4.3746.
[14] R. Madani, A. Ez-Zahout and A. Idrissi, “An Overview of Recommender Systems in the Context of Smart Cities”. In: 2020 5th Inter national Conference on Cloud Computing and Artificial Intelligence: Technologies and Appli cations (CloudTech), 2020, 1–9, 10.1109/Cloud Tech49835.2020.9365877.
[15] V. Vaidhehi and R. Suchithra, “A Systematic Re view of Recommender Systems in Education”, International Journal of Engineering & Technolo gy, vol. 7, no. 3.4, 2018, 188–191, 10.14419/ijet. v7i3.4.16771.
[16] Y. Ge, S. Zhao, H. Zhou, C. Pei, F. Sun, W. Ou and Y. Zhang, “Understanding Echo Chambers in E-commerce Recommender Systems”. In: Proceedings of the 43rd International ACM SI GIR Conference on Research and Development in Information Retrieval, 2020, 2261–2270, 10.1145/3397271.3401431.
[17] A. Felfernig, V.-M. Le, A. Popescu, M. Uta, T. N. T. Tran and M. Atas, “An Overview of Re commender Systems and Machine Learning in Feature Modeling and Configuration”. In: 15th International Working Conference on Variability Modelling of Software-Intensive Systems, 2021, 1–8, 10.1145/3442391.3442408.
[18] X. Zheng, L. D. Xu and S. Chai, “QoS Recommenda tion in Cloud Services”, IEEE Access, vol. 5, 2017, 5171–5177, 10.1109/ACCESS.2017.2695657.
[19] Y. R. Shrestha and Y. Yang, “Fairness in Algori thmic Decision-Making: Applications in Multi -Winner Voting, Machine Learning, and Recom mender Systems”, Algorithms, vol. 12, no. 9, 2019, 10.3390/a12090199.
[20] I. Palomares, C. Porcel, L. Pizzato, I. Guy and E. Herrera-Viedma, “Reciprocal Recommender Systems: Analysis of state-of-art literature, chal lenges and opportunities towards social recom mendation”, Information Fusion, vol. 69, 2021, 103–127, 10.1016/j.inffus.2020.12.001.
[21] D. S. Tarragó, C. Cornelis, R. Bello and F. Herre ra, “A multi-instance learning wrapper based on the Rocchio classifier for web index recommen dation”, Knowledge-Based Systems, vol. 59, 2014, 173–181, 10.1016/j.knosys.2014.01.008.
[22] R. M. Suleman and I. Korkontzelos, “Extending latent semantic analysis to manage its syntac tic blindness”, Expert Systems with Applications, vol. 165, 2021, 10.1016/j.eswa.2020.114130.
[23] R. J. Kuo, C.-K. Chen and S.-H. Keng, “Application of hybrid metaheuristic with perturbation-ba sed K-nearest neighbors algorithm and densest imputation to collaborative filtering in recom mender systems”, Information Sciences, vol. 575, 2021, 90–115, 10.1016/j.ins.2021.06.026.
[24] S. Renjith, A. Sreekumar and M. Jathavedan, “An extensive study on the evolution of context-awa re personalized travel recommender systems”, Information Processing & Management, vol. 57, no. 1, 2020, 10.1016/j.ipm.2019.102078.
[25] R. Panigrahi and S. Borah, “Rank Allocation to J48 Group of Decision Tree Classifiers using Bi nary and Multiclass Intrusion Detection Data sets”, Procedia Computer Science, vol. 132, 2018, 323–332, 10.1016/j.procs.2018.05.186.
[26] J. Hamidzadeh, E. Rezaeenik and M. Moradi, “Predicting users’ preferences by Fuzzy Rough Set Quarter-Sphere Support Vector Machine”, Applied Soft Computing, vol. 112, 2021, 10.1016/ j.asoc.2021.107740.
[27] K. Luo, S. Sanner, G. Wu, H. Li and H. Yang, “Latent Linear Critiquing for Conversational Recommender Systems”. In: Proceedings of The Web Conference 2020, 2020, 2535–2541, 10.1145/3366423.3380003.
[28] A. Yassine, L. Mohamed and M. Al Achhab, “Intelligent recommender system based on unsupervised machine learning and demogra
phic attributes”, Simulation Modelling Practice and Theory, vol. 107, 2021, 10.1016/j.sim pat.2020.102198.
[29] Q.-X. Wang, X. Luo, Y. Li, X.-Y. Shi, L. Gu and M.-S. Shang, “Incremental Slope-one recommen ders”, Neurocomputing, vol. 272, 2018, 606–618, 10.1016/j.neucom.2017.07.033.
[30] T. V. R. Himabindu, V. Padmanabhan and A. K. Pujari, “Conformal matrix factorization based recommender system”, Information Sciences, vol. 467, 2018, 685–707, 10.1016/ j.ins.2018.04.004.
[31] A. L. Vizine Pereira and E. R. Hruschka, “Simulta neous co-clustering and learning to address the cold start problem in recommender systems”, Knowledge-Based Systems, vol. 82, 2015, 11–19, 10.1016/j.knosys.2015.02.016.
[32] F. Maazouzi, H. Zarzour and Y. Jararweh, “An Effective Recommender System Based on Clu stering Technique for TED Talks”, International Journal of Information Technology and Web En gineering (IJITWE), vol. 15, no. 1, 2020, 35–51, 10.4018/IJITWE.2020010103.
[33] C. Djellali and M. Adda, “A New Hybrid Deep Learning Model based-Recommender System using Artificial Neural Network and Hidden Mar kov Model”, Procedia Computer Science, vol. 175, 2020, 214–220, 10.1016/j.procs.2020.07.032.
Submitted: 8th October 2021; accepted: 17th March 2022
Grzegorz Nowakowski, Sergii Telenyk, Kostiantyn Yefremov, Volodymyr KhmeliukDOI: 10.14313/JAMRIS/4-2021/29
Abstract:
The approach to applications integration for World Data Center (WDC) interdisciplinary scientific investigations is developed in the article. The integration is based on mathematical logic and artificial intelligence. Key ele ments of the approach – a multilevel system architec ture, formal logical system, implementation – are based on intelligent agents interaction. The formal logical sys tem is proposed. The inference method and mechanism of solution tree recovery are elaborated. The implemen tation of application integration for interdisciplinary sci entific research is based on a stack of modern protocols, enabling communication of business processes over the transport layer of the OSI model. Application integration is also based on coordinated models of business process es, for which an integrated set of business applications are designed and realized.
Keywords: research; application integration; business processes; mathematical logic; formal logic; inference mechanisms; multi-agent systems; protocols; software agents
In view of the globalization of the economy and social life, National Science must integrate into the world and European organizations that promote the consolida tion of research and consequently the development of scientific activity [27]. This is a very important pro cess since there is an urgent need for interdisciplinary research, primarily for the assurance of sustainable development globally and regionally [26]. However, effective implementation of interdisciplinary research requires the creation of appropriate conditions for in formation exchange in the process of solving scientific problems. The scientific and technical progress that in its time facilitated the creation of information and communication technologies (ICT) nowadays benefits greatly from them. They are developing rapidly, cover ing new spheres of human activity and enhancing per formance. Yet only field specialists can use ICT in a ra tional way, whereas the need for efficient information exchange within interdisciplinary research can only be met through rational ICT application by specialists with deep knowledge in their areas of expertise [19].
The development of the information technologies (IT) domain that is experiencing qualitative changes
related to ICT strengthening has created conditions for distributed computation and the efficient use of information and other resources. Consolidation of resources and the introduction of virtualization tech nologies are contributing to the process of substitut ing local solutions with distributed ones that allow the comprehensive use of all computing powers and data storage systems linked into a global network, thus granting access to accumulated information resourc es. The service approach formed on the basis of com munication services has spread to the infrastructure, software development tools, and applications. The emergence of a wide range of new types of services, especially content-based ones, has led to the conver gence of services and the formation of a generalized concept of information and communication services (ICS). The number of ICS providers has grown rapid ly and convergent providers have emerged. The wide functionality, high quality, and moderate price of new services rendered by providers allow businesses to abandon the development of in-house or IT infra structure and to use a wide variety of available ICS for component-based design of their information and telecommunication systems (S).
However, unified access to services is becoming a condition for the efficient use of the advantages of dis tributed systems and the possibilities of service-ori ented technologies. At the same time, the formation of a new democratic IT environment, in which even small businesses can render services, has naturally been accompanied by the use of various tools and ac cess technologies [19]. Therefore, historically the IT environment is heterogeneous, hence user access to its resources is to some extent complicated or at least inconvenient.
The same situation is characteristic of scientif ic activity in particular. For example, the World Data Centers (WDC) system created in 1956 under the aegis of the International Council for Science (ICSU) ensures collection, storage, circulation, and analysis of data obtained in various science areas [22]. Dur ing its existence, the WDC system has accumulated a lot of data and applications that may be used to solve the challenging problems of social development. They are one of the most powerful information resources used by hundreds of thousands of scientists, and the demand for it is increasing in proportion to the need for interdisciplinary research related to sustainable development, the solution of urgent environmental protection issues, etc. However, problems related to
the incompatibility of legacy applications caused by architecture differences, the variety of data presenta tion formats, and other factors prevent the effective use of such WDC resources.
Promising architectural solutions are being devel oped and gradually implemented in the ICT field, such as Next Generation Network (NGN) and Next Gener ation Service Overlay Network (NGSON) [24], that ensure the interaction of various transport layer tech nologies. Yet there is a need for a comprehensive in tegration of resources from various sources, and ICT developers’ efforts should seek to enable scientists working in various domains to use the accumulated resources based on their areas of expertise, and not on the IT particularities. The sources, comprehensive access to which it is reasonable to ensure, include da tabases [16], websites and portals, various legacy file management systems, and data repositories struc tured according to various models.
Nowadays there exist more than 50 WDCs that for more than 50 years have created a system for data accumulation, analysis, processing, and international exchange. WDCs’ powerful data storage systems re tain huge volumes of astronomical, geophysical and other scientific data. Certainly, from the point of view of scientists working on various resource-intensive problems, it is important to have access not to a large disordered system of possibilities, but to an integral complex of data and application sources intended to meet their specific needs, with a user-friendly inter face that does not require any special IT knowledge. Nevertheless, the system for WDC data accumulation, analysis, processing, and international exchange does not provide such access. Furthermore, it was not de signed for the growing level of scientific society’s re quirements and is not versatile enough to be used in interdisciplinary research. Therefore, a new interdis ciplinary structure was created in 2008 – the World Data System (WDS) – to develop and implement a new coordinated global approach to scientific data, which guarantees omni-purpose equal access to quality data for research, education, and decision making. The new structure will have to solve the accumulated tasks, pri marily the unification of formats and data transfer pro tocols, assurance of convenient access to data, and the organization of scientific data quality control [27].
The related difficulties are emerging because the existing WDCs are accumulating and providing heter ogeneous data determined by the specificity of a cor responding science area and country. The possibilities of telecommunication systems are constantly growing, as well as the possibilities of modern computers and data storage, thus making way for new distributed computation technologies – grid systems and cloudbased computation. Integrating formerly independent systems for the accumulation, storage, and processing of WDC data on a new advanced integration basis will allow a considerable enhancement of their overall effi ciency. The creation of such a system will provide sci entists with convenient centralized access to formerly separate resources, facilitating and quickening scientif ic and research activity around the world.
To implement such integration solutions that sup port the continuous lifecycle of research data, it is proposed to apply the well-proven Continuous Inte gration (CI) and Delivery (CD) software development practices.) [28], [29], [30]. Continuous Integration and Continuous Delivery are often quoted as the es sential ingredients of successful DevOps. DevOps is a software development approach which bridges the gap between development and operations teams by automating build, test and deployment of appli cations. It is implemented using the CI/CD pipeline. DevOps lifecycle contains six phases: Plan, Build, Con tinuous Integration, Deploy, Operate and Continuous Feedback. Throughout each phase, teams collaborate and communicate to maintain alignment, velocity, and quality.
CI/CD are required for software development us ing an Agile methodology, which recommends using automated testing to fast debug working software. Automated testing gives stakeholders access to newly created features and provides fast feedback.
The use of CI/CD practices requires the fulfill ment of a set of basic requirements for a develop ment project.
In particular, the source code and everything needed to build and test the project should be stored in a repository of the version control system, and operations for copying from the repository, building and testing the whole project should be automated and easily called from external programs. The GitLab environment allows to manage project repositories, document functionality and results of improvements and tests, as well as track errors and work with the CI/CD pipeline [31], [32].
In this paper, the authors would primarily like to focus on the consideration of urgent among the WDC prob lems requiring efficient solutions is that of integra tion. Presently, numerous WDCs use legacy technolo gies based on program systems with rigid structures, whereas newer solutions have for a long time been based on client- server and web-oriented technologies. The service approach to data processing and analy sis has not spread to the required extent. Sometimes connection to geoinformatics systems and even to basic public services such as Google Maps is unavail able. Combined with the lack of a unified standard, this fact shows that the need for data sources integration, including applications integration and databases [16] integration, may be considered sufficiently substanti ated. Therewith, the formation of a modern IT environ ment envisages the following integration aspects:
• technology: integrated data and services should be based on a single stack of interaction protocols, using common data transfer formats in order to render the integration
• possible;
• content: a single data description format in the semantic structure of data sources should be sup ported;
• functionality: the use of different services in the single structure to solve user-defined problems.
An overview of existing integration solutions in all the mentioned aspects, starting with the integration technology aspect has been done. The existing solutions are powered by technologies for the creation, function ing, and development of distributed systems. Notwith standing the differences of various technologies for the creation of distributed service-oriented systems, the overall principles and theoretical and methodological approaches are always similar. Independent services should be registered, described, and provided with the possibility to communicate transparently with clients and with each other. Furthermore, networking inter action requires us to determine protocols for all levels of the OSI model. To do so, the corporate ITS standard structure with services intellectualization operations may be used, as suggested in [15], taking into account international, state, and branch standards, and corpo rate documents, first of all [4]. The structure consists of two parts, where the first covers the traditional four levels of standards of the TCP/IP protocols stack, and the second covers the user applications in accordance with the ITS class, destination, and services intellectu alization operations. Fig. 1 presents an example of the corporate standard structure.
For convenience, the figure presents the levels of the international standard for interaction of open OSI systems and their correlation with the corre sponding levels of the TCP/IP protocols stack. The ITS classification used is proposed in [15]. It allows the systematization of various ICS by the level of us ers’ requirements and queries, practical needs, and professional training, with no limitations imposed on the ITSs’ functionality, attributes, or operations. The changes introduced apply to the first part of the structure and to the detailing of protocols that power the intellectualized interaction of applications in the course of solving users’ more complex problems.
The services registry is maintained by the Uni versal Description, Discovery and Integration (UDDI) technology [6] that allows both people and client-pro grams to publish information on services and search for a required service.
The Web Service Description Language (WSDL) that, according to the W3C definition, constitutes an XML format for the description of networking servic es as a set of operations working with document- or procedure-oriented information through messages [11], is used for the unified description of services, which allows them to be used independently from the programming language. WSDL documents, by virtually creating a unified layer that allows the use of services created on the basis of various platforms, describes the service interface, URL, communication mechanisms “understood” by the service, methods provided by it with corresponding parameters (type, name, location of the service Listener), and the ser vice messages structure. Let us mention that the ma jority of platforms support the formation of WSDL descriptions of services, enabling developers to work with services as simple classes.
To implement the key part of the interaction -mes sages exchange – one of the widespread technologies may be used, and the selection should be determined by compliance with the requirements of the system for data accumulation, processing, and exchange:
• SOAP: a user-friendly technology that is easy to use with the Business Process Execution Language (BPEL), which ensures interaction of distributed systems irrespective of the object model, operating system, or programming language. Data is trans ferred as special-format XML documents [5];
• CORBA: a mechanism created to support the de velopment and deployment of complex objectoriented applied systems, which is used for the in tegration of isolated systems, allowing programs that are developed in different programming lan
Fig. 1. Stack of services interaction protocolsguages and working in different network nodes to interact with the same ease as if they were in the address space of a single process. Such interaction is ensured through unified construction of their interfaces using a special-purpose declarative Interface Definition Language (IDL). At the same time, the interface and the description thereof do not depend on the operating systems or the pro cessor architecture either [1];
• REST (Representational State Transfer) is a style of software architecture for distributed systems, which is used to create web services. A global URL identifier unambiguously identifies each unit of information in an unvarying format. Data is trans ferred without any layers [17];
• WCF (Windows Communication Foundation) is Microsoft’s platform designed to create applica tions that exchange data through the network and are independent from style and protocol [7].
Although nowadays the most acceptable choice seems to be SOAP, based on which the interaction presented in Figure 1 is performed, all the technolo gies allow the integration of services of various origin [9,10].
To take into consideration service-based, pro cess-functional, and component-based approaches to the design and maintenance of users’ solutions, the following well-known architectural means of organ izing shared functionality are used:
Web Service Choreography is the approach that determines the protocols of web services’ interaction to perform a single global task by performing parts thereof. The role assumed by the service determines its model of exchanging messages with other servic es. This method shows high efficiency for small tasks, but with increased complexity of tasks the number of services involved grows rapidly, solutions become too massive, and the efficiency drops quickly [13].
Service orchestration is the approach that uses an orchestrator service to associate services into a single multifunctional business service. The role of orchestrator services may be performed by agents introduced into the system, which allows its hierar chical structure to be maintained, thus combining the advantages of orchestration and multi-agent systems (MAS). To perform orchestration, a special-purpose language was developed to describe the business processes and the protocols of their interaction –BPEL. This language, sometimes called the orches trating language, provides an orchestrator process (service) to coordinate the work of services subor dinated to it. The coordinated interaction is enabled through messages exchange between web services. The problem lies in the fact that, in order to carry out long-term business processes with complex struc ture, not only messages themselves are important, but also the exchange sequence, account of state, re sults, time and other aspects of business processes.
If the work of basic web services is organized by a program agent as a higher-level orchestrator ser vice, we get the management mechanism at the web services level, whereas organizational aspects (mes
saging sequence, account of load, etc.) will be dealt with by higher-level agents. As a rule, SOAP is used along with BPEL to implement messages exchange, although there are alternatives [2].
The scientific community is already using systems based on the decomposition of the overall problem (flow) into a sequence of individual subtasks (sub flows), the so-called workflow system. The effective interdisciplinary workflow environments worth men tioning are Triana Workflows and Kepler. A research er user may edit the task-performing scripts, using system automation means, which renders it versatile and widens its application sphere. Those systems’ ar chitecture consists of the following levels:
1) client (allows a user to quickly develop new work flows using the graphic script editor);
2) middleware (its flow server ensures workflows performance, authorization and coordination with the heterogeneous resources involved);
3) resource (provides resources to perform separate subflows).
A workflow constitutes a separate ready-to-use component. Components may be parts of other com ponents. Due to rigid typification (internal formats determined for flows and components) and the for mation of metadata for each component, which de scribe the types of their input and output data, flows and components are verified to make sure their input and output data are compatible. These systems were widespread due to their easy-to-use graphic user in terface, extensive library of standard components de veloped for various disciplines, effective script perfor mance manager, and means of monitoring.
Taverna Workflow Management System, a sys tem for the development, design, and performance of complex workflows, which allows the use of different services such as WSDL, BioMoby, SoapLab and other types to be coordinated, has also been widely used lately [8].
Alas, such systems are incompatible at the levels of both the performance manager and the description of flows and components. Furthermore, they lack an intellectual constituent, or such a constituent is not sufficiently developed, and therefore any working se quence of services needs to be built up manually or edited, which substantially limits these systems’ ver satility and application sphere.
Based on the abovementioned technologies, a few solutions have been worked out, which may be used to create applied systems for the accumulation, pro cessing, and exchange of scientific data in different ar eas. The best known among them are the ESIMO and GEOSS systems. They are quite widespread, although they have a number of draw- backs in terms of pro cessing heterogeneous information:
• difficulties in compiling the association of hetero geneous types of data from different sources in one query;
• difficulties in enhancing the functionality and de gree of automation, caused by drawbacks in these systems’ architecture, which does not envisage the possibility of forming a single network;
• no unified metadata model for all the metadata objects;
• no unified standard for data presentation and pro cessing;
• users need special expertise, knowledge, and skills to form a query.
Based on this overview, we can draw a substan tiated conclusion regarding the possibility of using the developed approaches, models, technologies, and means for integration of applications, primarily:
• schemes for the implementation of applications interaction based on the stack of protocols UDDI, SOAP (or REST), BPEL, WSDL, XML above the transport level;
• technology of WCF type;
• approach to the integration of services through an orchestrator service into a single multifunctional business service.
Nonetheless, for user-formulated tasks, the level of automation of services interaction schemes should be enhanced. Ultimately, this is a question of devel oping efficient models and methods of applications integration, based on which a new state-of-the-art ap plications integration system may be created, capable of providing users with the abovementioned advan tages.
Secondly, let us dwell briefly on the content aspect. As a rule, data integration in information systems is viewed as the provision of a single unified interface to access a certain number of generally heterogene ous independent data sources [3,14]. For a user, in formation re- sources from all the integrated sources should be presented as a new single source, and the system providing such possibilities is called the data integration system [12]. There are several data inte gration approaches, some of which are successfully implemented and quite widespread in real systems. The analytical review thereof is presented in [12,20]. The data space concept viewed as a new abstraction of data management has been steadily changing its positions lately. In Ukraine and Poland, it was devel oped in works by N. Shakhovska, for instance [21].
Finally, let us move on to the third aspect of inte gration. The view on integration as the interaction of applications is not as widespread, yet is extreme ly important in the con- text of resolving the com prehensive integration issue in the new IT environ ment. It was mostly formed within the community of specialists in programming automation, notably in relation to the implementation of promising tech nologies for industrial software production, such as “component-based programming”. In terms of coun teracting program systems complexity, the focus was transferred from developing versatile, adjustable, and scalable structures, such as application packages, to developing means for the interaction of independent program systems.
The specific systematic research of the applica tion integration began at the turn of the millennium, when the issue of the integration of numerous busi ness applications, primarily of CRM, ERP, SSM class es, etc., abruptly emerged. Under the new economic
conditions, business charges IT with new tasks, the fulfillment of which through the purchase of new and the modernization of available business applications does not ensure a return on investment any more. At that very moment, the need arose to build enterprises’ information systems based on the integrated complex of business applications, and the first attempts were made at the formalized description of business appli cations and their interaction with the aim of reaching the business divisions’ objectives. Thus emerged the Component Object Model (COM) and Common Object Request Broker Architecture (CORBA) technologies that ensured programs interaction on a single com puter, and subsequently such possibilities were ap plied to the interaction of program systems located on different computers. The abovementioned possi bilities implemented in the Distributed COM (DCOM), Electronic Access Interchange (EAI), and CORBA technologies ensured independent program systems integration to solve users’ problems that are more complex.
Another solution that is important for applica tions integration was the Service-oriented Architec ture (SOA), whose emergence gradually, through the integration of multi-bases and federated databases, large data storages, and various repositories, promot ed the transition of the integration concept onto web applications. Nonetheless, a total renunciation by IT departments of solving efficacy issues through the in tegration of business applications into the existing IT infrastructure with minimal losses only became pos sible after the 2008 crisis, with the emergence and implementation of cloud-based computation technol ogies. At that very moment, there arose the obvious necessity to transparently see the IT infrastructure of an enterprise’s entire information system and the means of its architecting, operation, and develop ment, with an account of the new possibilities of the IT environment that was being intensely formed on the basis of consolidation, virtualization, and mul ti-services.
The industry leaders proposed a solution based on integration platforms ensuring complete function ality through advanced means for scaling and adjust ment. However, such a centralized approach requires a respective IT infrastructure and not every enter prise can afford this. Furthermore, it entails a certain dependence of the enterprise on companies produc ing such integration solutions.
Therefore, the majority of enterprises are more interested in the integration approach related to the wide use of business applications by various develop ers through proxies, such as Message Oriented Mid dleware (MOM) programs, combined with modern approaches to cost-cutting in IT-infrastructure own ership, primarily the means of using legacy applica tions. Another obstacle in the way of implementing the decentralized approach is differences in the ar chitectural components of business applications by different producers, notably in the models of data and business processes, technologies of designing the program system and its basic elements (DBMS serv
ers and applications), component cooperation means, users’ access, etc.
Cloud-based computation allows the provision of software services of a determined quality for a fair price, thus justifying the practicability of software (SaaS), infrastructure (IaaS) and platform (PaaS) ser vices [25]. Nowadays, this activity may be viewed as a theoretical and methodological basis for the migration of the service approach to information systems crea tion and the wide introduction of component-based development thereof.
In order for legacy applications to become part of a service-based system, their data models and business processes must be in a certain way coordinated with those supported by the new systems, and interaction with the program system’s basic elements must be ensured. Therefore, data integration in information systems and integration as the coordination of appli cations have to be combined. The necessity arises to develop a technical solution that will allow:
• creating mechanisms for efficient information re sources management;
• widening areas of protocols standardization and data exchange formats based on the interaction of commodity systems;
• creating cross-platform applications, integrating data and applications;
• facilitating systems deployment and development by concealing software and hardware internal de tails.
To create solutions that provide the above possi bilities, it is required to:
1) develop formal models and methods to supply metadata in order to describe sources, their func tionality, and particularities of access;
2) develop models and methods for the automated design and implementation of unified-architec ture applications that are capable of efficient in teraction in order to reach the objectives of inter disciplinary research, and on the basis thereof to create platform solutions according to the PaaS model;
3) develop models, methods, and means to imple ment applications interaction at the semantic level;
4) develop models and methods to facilitate users’ queries for required data using the terms from topical area(s) of research that are convenient for them;
5) bring the IT infrastructure of the WDC system into compliance with the needs through consolidation, virtualization, and multi-services powered by cloud-based computation models and technolo gies;
6) develop models, methods, and means for efficient administration of the IT infrastructure of the WDC system, capable of ensuring the determined level of information services quality. One of the most crucial issues is that of develop ing mathematical models, methods, and means for the integration of various applications, both legacy ones based on traditional technologies and client-server
and web-oriented technologies. The article propos es an approach to applications integration using the mathematical logic instrument and artificial intelli gence theory for interdisciplinary research through the example of the WDC system functioning. The specificity of the applications integration for interdis ciplinary research is that the coordination of business processes models is not required because it is, in fact, substituted with schemes for performing users’ tasks. In general, the applications integration is performed on the basis of coordinated business processes mod els, and the integrated complex of business applica tions is intended to support them.
Mathematical models and methods as the basis for a holistic solution should be created to power data stor age and processing centres, which will provide users with versatile possibilities at the level of information integration and servers availability. The mentioned models and methods should be devoid of the draw backs characteristic of the algorithmic approach, related to the need to reprogram data processing al gorithms upon the emergence of new data types and changes in the implemented algorithms or the emer gence of new ones. At the data processing level, such a solution should ensure [18,22]:
• computations distribution and the use of remote hardware resources;
• versatility and adaptation to the system load;
• system usage space;
• data supply by remote client or service;
• availability of intellectual data processing means directly to the end user with no special knowledge or skills;
• fast and simple integration of data and applica tions of various global information systems.
The principal tenet for the creation of a distributed system is the method of organizing services interac tion. Of the two known main ways of services inter action – orchestration and choreography – the more efficient for the WDC systems is the former. Indeed, orchestration that aggregates basic services into hi erarchically integrated systems, subordinating them to administrators – “orchestrator” services – allows services to be unified by attributes that are conve nient to WDC (science area, functionality, regional location, etc.), and to provide orchestrator services with powers in accordance with the international data exchange policies. Thus, it is possible to line up a simple and effective system, in which the search for the necessary services or the construction of their composition for the complex queries inherent in in terdisciplinary research will not require a lot of time, as every Orchestrator Service has information on the functionality of inferior services, and in addition they
can coordinate their possibilities in the process of the planning and execution of users’ queries. Plugging new services into the system will not present a par ticular problem either, as doing so will only require the service description to be laid down, entered into the registry, and assigned to a certain orchestrator service [18,22].
Such an approach will work when users know ex actly their information needs and have corresponding knowledge and skills to compile chains of queries to the known orchestrators. What is more important is that the approach will provide the opportunity to work with the system for users who cannot initiate services, by determining the sequence of their work and speci fying execution and interaction parameters. Users only have to know how to formulate their needs in terms of a particular subject domain. In this case, the associa tion of services and the organization of their cooper ation require an intellectual constituent. For this pur pose, intellectual agents are used, which constitute the system core, implementing its functioning logic, which promotes the formation of queries, plans their execu tion, and organizes basic services interaction.
The introduction of intellectual agents as orches trator services into the hierarchical structure forms a two-tier system; the bottom level consists of ser vices performing basic tasks, the top level consists of intellectual agents that orchestrate basic services. By using the registries of subordinated basic services and their functionality and by interacting with each other, interconnected intellectual agents implement methods of logical inference. The inference result is the composition of basic agents’ operations that allow user-defined tasks to be performed. This operations composition, or proof, is transferred to the lower lev el, where the operations necessary to solve the user’s task are performed. At this point, control is handed over to the lower-level agents that only return the fi nal result to be sent to the user.
The user’s query itself is performed upon forma tion of the proof containing references to one or sev eral pointers to data sources and IDs of methods that the system services should apply to the data. Some queries do not require pre-processing of data, since the execution of services methods is sufficient upon receipt of information from the data source. Others require a certain sequence of prior data operations, and as a result, the execution of other services meth ods. The necessary connections are described in the axioms entered into the knowledge base upon reg istration of respective services in the system. These operations yield the final product of the system – data that are the solution to the user’s problem. Since the system operation is determined by the user’s query, the system interface should help them make a query in familiar terms.
The proposed solution uses UDDI to create the ser vice registries and WSDL for the unified description thereof. The key part – messaging – is implemented using SOAP, and services orchestration – using BPEL. Agents are described by means of JADE. The system components interaction scheme in presented in Fig. 2.
The description of the interface (WSDL) of every low-level service registered in the system and of re lated axioms is input into the system knowledge base and directly into the knowledge base of the Controller Agent to which the new service will be subordinated. The Controller Agent is selected in accordance with the policies adopted in the WDC system, for example, by the criteria of the geographical location of the de ployed agent, in order to minimize data exchange.
At the agent level, the orchestrator agent (or sever al), having received the user’s query, interrogates the agents in order to search for the necessary services and available resources. The user’s query is processed taking into account the services of the system, their functionality (described by the axioms of the system), and the inference rules defined by logical formalism. The resulting proof is transferred to the lower level to be implemented.
At the services level, each agent invokes the re quired services from its set and sends processed data according to the action chains. This process is carried out in accordance with the task solution tree recon structed by the solution tree reconstruction mecha nism based on the proof.
To realize this interaction of services in the WDC system, the most appropriate is the logical approach, which allows both the level of the abovementioned re quirements to be reached and the drawbacks of a tra ditional algorithmic approach to be eliminated. Indeed, it is only required to develop the inference method and the solution tree reconstruction mechanism that will implement the query formation processes, plan their execution, reconstruct and implement the task solu tion scheme. The logical approach is the most appro priate one to create and describe these constituents. The logical approach implementation requires one to:
• describe the existing applications and their func tional capacities in the formal language;
• formulate the inference rules;
• determine the inference method;
• develop an algorithm of the user’s query execution tree reconstruction based on the proof;
• implement these methods and mechanisms in the agents of the system.
The principal tenet for the creation of a distributed system is the method of organizing services interac tion [18,22,23].
Let us describe the formalism upon which the pro gram system that will ensure solution of the formulat ed problem will be built. We will take the first order clausal logic for a base and describe the formal system language in accordance with the structural elements determined in [27].
Symbols: service: (, ), [, ], {, }, :, <, >, ,; constant:
1. individual, of primary types (int, real, char, bool) –1 1a , 1 2a , …, 2 1a , 2 2a , ... where each constant k ai pertains to type (primary type) k; structural type (construct) – с1, с2, ...; procedural type (method) –d1, d2, ...; objective type (problem, entity, relation) — е1, e2, ...;
2. functional i-place, for individuals of type k –1 1h , 1 2h , …, 2 1h , 2 2h , ...;
3. predicate i-place, for individuals of type k –1 1A , 1 2A , …, 2 1A , 2 2A , ... (this class includes taxonomic, rela tional and other predicates, as well as traditional relations, at least equality = and order ≥); variable: for individuals of type k –1 1x , 1 2x , …, 2 1x , 2 2x , , where every variable k xi pertains to type k; logical: ¬∧∨←∃∀⇔ ,,,,,,
Individual terms of type k:
1. each individual constant k ai of type k is an indi vidual term of type k
2. each free variable k xi for individuals of type k is an individual term of type k;
3. if jhi is a certain functional constant for individu als of type k and τ1, …, τj are terms for individuals of type k, then jhi (τ1, …, τj) is an individual term of type k;
4. there are no other individual terms of type k
The terms obtained by applying construction rules 1 or 2 of the definition will be called primary, and all the others—complex.
1. if jAi is a predicate constant for individuals and τ1, …, τj are terms for them, then jAi (τ1, …, τj) is the atomic formula for individuals;
2. the atomic formula for individuals is the formula for them;
3. there are no other formulas for individuals.
Hereinafter we consider that the system contains an omni-purpose transformer of formulas into the traditional for the clausal form (we are talking about the Horn clauses) view with a single → symbol, atom ic formulas to its left and right, and an implicit quanti fier ∀
Specifiers are constructions of type τ1, where τ1 is a term for an individual object. The construct spec ifiers are: if e i e ee 1 are individual terms of type entity, and r i r ee ...1 are individual terms of type relation, and Aj 1 ( 11 1 aaj )… jAi ( k j k aa 1 ) are atomic formulas for the individuals of primary types, and τ is an individual term of type construct, then τ: ( ,1 e i e ee , ,1 r i r ee , Aj 1 ( 11 1 ... aaj )… jAi ( k j k aa 1 )) is a construct specifier.
4. there are no other construct specifiers.
Preconditions:
1) if c1 is a specifier of construct, and П is a sequence of atomic formulas, then <τ1:(c1,П)> is the elemen tary precondition;
2) elementary precondition – precondition;
3) if <τ1> is a precondition, and τ2 is an elementary precondition, then < τ1, τ2> is the precondition;
4) there are no other preconditions.
Post-conditions:
1) if τ1 is a construct specifier, and П is a sequence of atomic formulas, then <τ1:(c1,П)> is the elemen tary post-condition;
2) elementary post-condition – post-condition;
3) if <τ1> is a post-condition, and а τ2 is an elemen tary post-condition, then < τ1, τ2> is the post-con dition;
4) there are no other post-conditions.
Specifiers of methods:
1) if τ is an individual term of type method, and <τ1> is a precondition, and < τ2> is a post-condition, then τ: (<τ1>, < τ2>) is a method specifier;
2) the arity by constructs of method precondition must not be lower than the arity by constructs of method post-condition;
3) there are no other method specifiers.
1) if <τ1> is a precondition, and <τ2> is a post-con dition, and τ is a term for an individual object of type Problem, then τ: << τ1>,< τ2>> is the problem specifier;
2) there are no other problem specifiers.
Clause is an expression of type П →Λ, where П is a sequence of atomic formulas; Λ is a single atomic for mula. Depending on their type, atomic formulas will be distributed into the following clauses:
1) individual (contains only atomic formulas for indi viduals);
2) typified (contains only atomic formulas for types);
3) general type (contains atomic formulas for indi viduals and types).
The system’s knowledge base consists of the on tology of axioms depicting methods of system servic es and the ontology of data sources described in the OWL language based on RDF. The knowledge base also includes the inference rules necessary to obtain
the desired result when it is required to combine methods of a single service or of different services [18, 22, 23].
Inference rules:
1) If d1: (<τ1>,<τ2>) and d2: (<τ3>,<τ1>) then d1: (<d2>,<τ2>)
2) If d1: (<τ1>,<τ2>) and d2: (<τ3∧τ4>,<τ1>) then d1: (<d2>,<τ2>)
3) If d1: (<τ1∧τ2>,<τ3>) and d2: (<τ4>,<τ1>) and d3: (<τ5>,<τ2>) then d1: (<d2∧ d3>,<τ3>)
4) If d1: (<τ1>,<τ2>) and d2: (<τ3∨τ4>,<τ1>) then d1: (<d2>,<τ2>)
5) If d1: (<τ2∧τ2>,<τ1>) then d1: (<τ2>,<τ1>)
6) If d1: (<τ1,τ2>,<τ3>) and d2: (<τ4>,<τ1>) and d3: (<τ5>,<τ2>) then d1: (<d2, d3>,<τ2>)
7) If d1: (<τ1>,< τ2,τ3>) and d2: (<τ2>,<τ4>) and d3: (<τ3>,<τ5>) then d2: (<d1>,<τ4>) and d3: (<d1>, <τ5>)
We use the method proposed in [27] based on analogy and types of assertions. Its idea is to build a proof in abstract space and subsequently use it to control the inference process in the initial solution search space. This will increase the inference effectiveness by cut ting off most of the unpromising proof branches in the initial space.
Hereinafter a multiset of literals (atomic formulas) will be called a multiclause (m-clause), and literal L will be recorded in the m-clause as many times as it is repeated. An ordinary clause will be considered as an m-clause in which every literal’s multiplicity is 1. The operations ∪ (unification), ∩ (intersection), — (difference), (concatenation) and relation ⊆ (occur rence) for multisets are naturally performed.
Suppose A1∈C1, A2∈C2 and α1, α2 are such substi tutions that some literal L on the right side of А1 and on the left side of А2, A1α1={L}, A2α2={L}, and if |Ai|>1, i = 1, 2, the corresponding substitution αi is the most common unifier of literals from А1. Then the clause obtained from the unification of clauses C1α1 and C2α2 by removing L of the left and right parts is called the m-resolvent of m-clauses С1 and С2 Suppose all the m-clauses in the original set S are ordered, and С1, С2 are two of them. Suppose A1∈C1, A2∈C2 and α1, α2 are such substitutions that for some literal L on the right side of А1 and on the left side of А2, A1α1={L}, A2α2={L}, and if |Ai|>1, i = 1, 2, the corresponding substitution αi is the most common unifier of the literals from Ai. Then the clause obtained from the unification of clauses C1α1 and C2α2 by removing L of the right part and the last L of the left part and by eliminating any non-underlined literal that is not followed by any oth er literal, is called the ordered linear m-resolvent of ordered m-clauses С1 and С2. If the last literal on the left side of the ordered m-clause is unified with the underlined literal on the right side of the same clause, the ordered linear m-resolvent can be obtained by re moving the last literal from the left part of the ordered m-clause, i.e., m-clause reduction
The next pair whose first component is a set of proof vertices and the second is a set of triples of verti ces (to select components, a pair of the function-selec tors s-N(Tm) and s-M(Tm) will be respectively used) is called the m-resolution proof and denoted Tm. Each vertex of the m-resolution proof is characterized by a mark and the depth in the proof, so that if n∈s-N(Tm), then s-L(n) is the mark of vertex n and s-D(n) is its proof depth. If <n1, n2, n3> ∈ s-M(Tm), then s-L(n3) is the m-re solvent of s-L(n1) and s-L(n2). Each triple of such a form is called an m-resolution. If n∈s-N(Tm) and vertex n is not the third component of any of the triples from s-M(Tm), then n is called the initial vertex of proof Tm. The mark of such vertex is the initial m-clause. If n∈sN(Tm) and vertex n is neither the first nor the second component of any of the triples from s-M(Tm), then n is called the terminal vertex of proof and its mark is called the terminal m-clause. In general, Tm must satisfy the following restriction: if < n1, n2, n3> ∈ s-M(Tm), then < n2, n1, n3> ∈ s-M(Tm). The depth (in the proof) of each vertex n, n∈s-N(Tm), by definition:
1) s-D(n) = 0 for any initial vertex п;
2) s-D(n) = 1 + min{max{s-D(n1), s-D(n2)}|<n1, n2, n> ∈ s-M(Tm)} for any non-initial vertex п.
If the marks of the initial vertices Тт belong to the set of m-clauses S, then m-resolution proof Тт is called the proof from S. С is inferred from S if Tm is a proof from S, while C is the mark of one of the Tm vertices.
Suppose the value of function Result() is defined for Tm and is equal to С, if terminal clause С of proof Tm is the only one, i.e. Result(Tm) = C. Suppose Tm1 and Tm2 are m-resolution proofs. Proof Tm1 is called the sub-proof of Тт2 (and denoted Тт1 ⊆ Tт2), if the fol lowing conditions are fulfilled: s-N(Tm1) ⊆ s-N(Tm2) and s-M(Tm1) ⊆ s-M(Tm2). Moreover, if all the initial vertices of Тт1 are the initial vertices of Тт2, then Тт1 is called the initial sub-proof of Тт2
Suppose Тт is the m-resolution proof from S and all its m-clauses are ordered. If for any triple < n1, n2, n3> from s-M(Tm) s-L(n3) is the ordered linear m-re solvent of s-L(n1) and s-L(n2), then Тт is called the or dered linear proof from S. Then, by the m-resolution proof definition, the following condition must be satis fied: max{s-D(n1), s-D(n2)} = s-D(n3) – 1.
In addition to the general properties of m-resolu tion proofs, the ordered linear proofs have their own features. For example, for the ordered linear m-resolu tion proof the following conditions are fair:
1) if < n1, n2, n3> ⊆ s-M(Tm), then < n2, n1, n3> ∉ s-M(Tm);
2) for each non-initial vertex п3 with proof depth r there exists a triple < n1, n2, n3>, in which n1 has the proof depth r 1, and n2 corresponds to the initial vertex or is absent;
3) Тт contains a single terminal clause.
To manage an ordered linear proof, we will use the typification abstraction proposed in [26]. Suppose f is such mapping from the set of m-clauses into the set of m-clauses that:
1) if m-clause С3 is the m-resolvent of m-clauses C1 and С2, while D3∈f(C3), then there exist such
D1∈f(C1) and D2∈f(C2) that the result of the sub stitution of some m-resolvent D1 and D2 belongs to D3;
2) f(∅)=(∅);
3) if the result of some substitution of m-clause С1 belongs to m-clause С2, then for any abstraction D2 for С2 there exists such abstraction D1 for C1 that the result of D1 substitution belongs to D2 Such mapping is called f m-abstraction mapping, while any D from f(С) is called m-abstraction. The typ ification mapping is understood as a certain mapping ϕ from a set of literals into a set of literals that reflects each atomic formula into the formula whose terms have the type closest to the basic types in the hierar chy. Work [8] determines that typification mapping φ is m-mapping.
Work [14] determines that typification mapping φ is m-mapping. A relationship between the ordered lin ear proofs Тт and Uт is called the relationship of im provement (and denoted Тт→fUт), where f is m-ab straction mapping, if the vertices of proofs Тт and Uт are in such a relationship of accordance R that:
1) the following conditions are fulfilled simultane ously: ∀n(n∈s–N(Tm)) ∃n'(n'∈s–N(Um))(nRn') and ∀n(n∈s–N(Um)) ∃n'(n'∈s–N(Tm))(nRn');
2) if nRn', where n∈s–N(Tm), n'∈s–N(Um), then п and n' may be initial or terminal vertices only simulta neously;
3) for terminal vertices Тт and Uт, relationship R is a one-to-one relationship;
4) if <n1, n2, n3> ∈ s-М(Тт), <n'1, n'2, n'3> ∈ s-M(Uт) and n3Rn'3, then n1Rn'1 and n2Rn'2;
5) if п and n' are initial vertices of proofs Тт and Uт respectively, and nRn', then s-L(n') ∈ f(s-L(n));
6) if п and n' are non-initial vertices of proofs Тт and Uт respectively, and nRn', then example s-L(n') be longs to f(s-L(n)).
So the ordered linear proofs have the property of minimality, i.e. there is exactly one terminal vertex, and if triple <n1, n2, n3> belongs to the minimal or dered linear deduction, then no other triple can con tain vertex n3 as the third component.
On this property of the ordered linear proofs, the authors have built the inference mechanisms implemented by agents. Once a user has formulated the problem, the system builds a proof – in fact, by checking the possibility of performing the query with existing resources. This task is performed by Orches trator Agents as an important part of the system’s intellectual kernel. With access to the list of services, their descriptions and axioms that specify services application capabilities, these agents initiate the in ference process. The inference process constructs a chain of services capable of providing the user with the desired result, starting with obtaining data from relevant sources.
The ordered linear m-resolution proof is speci fied by a pair of vertices sets V = {k1, k2, k3 ... kn} and
vertices triples T{<k1, k2, k3>, <k3, k4, k5> ... <kn–2, kn–1, kn>}, where ki is the vertices of the tree, kn is the ter minal vertex, the result of the algorithm execution. The proof is formed based on the post-condition of the formulated problem as the initial proof vertices, and the compatible axiom from the knowledge base as the lateral vertex. The axiom is compatible if the se quence of atomic formulas matching the given proof vertex or any part thereof is the axiom post-condition. The third vertex of the triple is obtained by applica tion of the axiom to the post-condition formula, tak ing account of the inference rules. The third vertex of the first triple becomes the first vertex of the second triple, and the process is repeated until the terminal vertex that corresponds to the formulated problem precondition is reached. If the atomic formula corre sponding to the third vertex of the triple can be re duced by using one of the inference rules, it is reduced in the following triple that will only have two vertices – the first corresponding to the formula to be reduced, and the second corresponding to the reduced formu la. To search for compatible axioms, the constructs of the currently processed post-condition are compared to the constructs of the ontology axioms. Thus, the set of axioms that describe services processing the ob jects of the desired type and format is selected from the ontology. The inference mechanism deducts and selects axioms only in this set. If, while searching for axioms for another vertex, several compatible axioms are found in the knowledge base, each of these axi oms is used for further construction of their “parallel” versions of the proof. As a result, the inference mecha nism can form several proof sets with various lengths and complexity, depending on the number of axioms in the knowledge base and combinations of their ap plication to each vertex.
After the inference mechanism’s work is over, the proof with the fewest vertices triples and the fewest applied axioms is selected from among the set of ob tained proofs. Longer, cyclic, or dead-end proofs are discarded.
This set of formulas and clauses, corresponding to the proof triples vertices and reflecting the applica tion of axioms and the rules of their construction, is the result of the inference mechanism work.
The inference mechanism work algorithm is pre sented in Figure 3.
The example of inference mechanism work and solu tion tree reconstruction for problem Р of type problem
P: <<A>,<K>>, where А and K are a precondition and a post-condition is presented in Figure 4.
Proof, a set of vertices triples: <A,A
A>, <A
dence of edges to pairs of vertices. The scheme deter mines the sequence and the correspondence accord ing to the data of actions to be performed by the data processing system’s executive mechanisms to obtain the result desired by the user [18,22].
Algorithm for vertex scheme reconstruction was presented on Figure 5.
To obtain from the proof generated by the inference mechanism a functional sequence of actions to be used by our system, taking account of services fea tures and nature, the solution scheme reconstruction mechanism should be initiated [18,22].
The solution scheme constitutes a connected directed graph with no oriented cycles with parallel directed paths from the root to the vertices; it has three types of vertices, and is specified by triple G=<V,E,Θ>, where V=V1∪V2∪V3, V1 is a set of method vertices, V2 is a set of precondition vertices and post-condition vertices, V3 is a set of data vertices in which data is merged or split; Е is a set of edges; Θ is a subset of Cartesian product Е×V×V, which determines the correspon
Example of the solution tree reconstruction mecha nism work for problem. Tree reconstruction by triples:
Fig. 4. The example of inference mechanism work and solution tree reconstructionThe reconstructed solution tree is fed to the actuator input. The implementation of a particular method specified in the solution tree is represented by a construct that describes the input data (entities, connections, relations between them), method preconditions, method post conditions, and output data. The solution tree branches downstream of the data splitting vertex can be executed in parallel until they reach the data merging vertex, where, after completing all the parallel branches involved in merging, they continue to be executed consecutively. The work algorithm of the solution tree reconstruction mechanism is presented in Figure 6.
The reconstructed solution tree is fed to the actu ator input. The implementation of a particular meth od specified in the solution tree is represented by a construct that describes the input data (entities, con nections, relations between them), method precondi tions, method post-conditions, and output data. The solution tree branches downstream of the data-split ting vertex can be executed in parallel until they reach the data-merging vertex, where, after completing all the parallel branches involved in merging, they con tinue to be executed consecutively. The work algo rithm of the solution tree reconstruction mechanism is presented in Figure 6.
The determined sequence of services performance:
S1 (<A>, <B>)
S2 (<A>, <C>)
S5 (<C∧B>, <E>)
S7 (<B>, <D>)
S6 (<E∧C>, <F>)
S9 (<F∧D>, <K>)
The life safety component formula: ������������ = √∑ ����ℎ���������������� ����3 0 3 , where the threat value is normalized by formulas (2) or (3). The purpose of indicating critical values of threat indicators is to determine the priority in consideration thereof in the decision making process on the level of a single region and the entire country in order to mitigate the impact of threats on sustainable development.
The purpose of indicating critical values of threat indicators is to determine the priority in considera tion thereof in the decision-making process on the level of a single region and the entire country in order to mitigate the impact of threats on sustainable devel opment.
Suppose that for every administrative unit i=1, in = there is a set of values 〈xi,1,xi,2,...,xi,m〉 of indicators Xj,j= ,1,j Xjm = , which characterize the negative impact of certain phenomena on the sustainable development processes in the economic, social, and ecological spheres. Such indicators, whose essence and compo sition are determined by experts, will be called threat indicators.
Suppose that for every administrative unit 1, in = there is a set of values ,1,2, ,,..., iiim xxx of indicators ,1,j Xjm = , which characterize the negative impact of certain phenomena on the sustainable development processes in the economic, social, and ecological spheres. Such indicators, whose essence and composition are determined by experts, will be called threat indicators.
Given the content of the critical values indication problem, the following characteristic function must be determined:
Given the content of the critical values indication problem, the following characteristic function must be determined: ���� (��������,���� ) = {0, �������� �������������������� �������� ���� �������� ������������ �������������������������������� 1, �������� ��������ℎ������������������������
where 1,,1, injm ==
where 1,,1, injm == .
It is clear that the determination of function () xij must be based upon certain criteria that take into consideration exceeding by , xij a hazardous limit, the relative position of region i in the indicators rating Xj compiled for the comparison groups and for the entire country, and the degree of
It is clear that the determination of function Ψ(x i,j) must be based upon certain criteria that take into consideration exceeding by xi,j a hazardous limit, the relative position of region i in the indicators rating Xj compiled for the comparison groups and for the en tire country, and the degree of “hazard” of value xi,j in comparison to values of other indicators for region i.
To account for the relative position of the region in the entire country’s ratings, the following criterion is used:
1 1 axij b ij Re =+
if higher values of indicator Xj correspond to a higher impact of the respective threat on the sustainable de velopment, and: 1
The determined sequence of services performance:
S1 (< A >, < B >)
S2 (< A >, < C >)
S5 (< C ∧ B >, < E >)
S7 (< B >, < D >)
S6 (< E ∧ C >, < F >)
S9 (< F ∧ D >, < K >)
algorithm of the solution tree reconstruction mechanism
if lower values of indicator Xj correspond to a higher impact. In formulas (2)-(3), parameters a and b are calculated by the following formulas:
This section is not mandatory, but can be added to the manuscript if the discussion is unusually long or com plex.
tree reconstruction mechanism reconstruction by triples:
The approach efficiency on the real scientific task of calculating the component of life safety and indi cating critical values of threat indicators for analyzing the sustainable development of the regions of Ukraine has been demonstrated.
This section is not mandatory, but can be added to the manuscript if the discussion is unusually long or complex.
Criterion Ri,j is a dimensionless number that as sumes values within [0,1]. Values of around 0.5 corre spond to average values of Xj in the selection, and val ues higher than 0.75 correspond to values that exceed the average ones by more than a standard deviation. In this case, the characteristic function (1) taking ac count of one criterion Ri,j may be expressed as follows:
The approach efficiency on the real scientific task of calculating the component of life safety and indicating critical values of threat indicators for analyzing the sustainable development of the regions of Ukraine has been demonstrated.
The life safety component formula:
The life safety component formula: ������������ = √∑ ����ℎ���������������� ����3 0 3 , where the threat value is normalized by formulas (2) or (3).
The purpose of indicating critical values of threat indicators is to determine the priority in consideration thereof in the decision making process on the level of a single region and the entire country in order to mitigate the impact of threats on sustainable development. , where the threat value is normalized by formulas (2) or (3).
Criterion Pi,j that takes into account a region’s rel ative position in the comparison group may be calcu lated by formulas (2)-(3) taking account of the fact
Fig. 6. The example of the solution tree reconstruction mechanism work for problemthat parameters a and b are calculated by formula (4) independently for each comparison group.
Criteria Ri,j and Pi,j are dimensionless numbers and are of similar nature and can therefore be aggregated through a weighted sum:
Ki,j=wRRi,j+wPPi,j; wR+wP=1,
where weighting factors wR and wP are determined by experts.
Thus, for region i=1, in = we have a set 〈Ki,1,Ki,2,...,Ki,m〉 of values of the aggregated criterion that accounts for a region’s relative position in ratings compiled for comparison groups and for the entire country. Now, among values Ki,j,j= , ,1,ij Kjm = , the worst must be deter mined, which may also be performed by formula (2): 1 , 1 aKij b
Ie =+
where parameters a and b are calculated in selection Ki,j,j=, ,1,ij Kjm =
Axiom 1: (<Di,j>,<Ri,j>)
Axiom 6: (<Pi,j>,<P'i,j>)
Reduction by rule 5: Di,j,Pi,j,Pi,j⇔Di,j,Pi,j
Axiom 2: (<Di,j>,<Pi,j>)
Reduction by rule 5: Di,j,Di,j⇔Di,j
The proof constitutes a set of vertices triples: <D; D, D>, <D, D; (<D>,<P>); D, P>, <D, P; D, P, P>, <D, P, P; (<P>,<P’>); D, P, P’>, <D, P, P’; (<D>,<R>); R, P, P’>, <R, P, P’; R, R, P, P’>, < R, R, P, P’; (<R>,<R’>); R, R’, P, P’>, < R, R’, P, P’, R, R’, P, P’, P>, < R, R’, P, P’, P; R, R’, P, P’, R, P>, < R, R’, P, P’, R, P; (<R, P>, <K>); R, R’, P, P’, K>, < R, R’, P, P’, K; (<K>,<I>); R, R’, P, P’, I>, < R, R’, P, P’, I; R, R’, P, P’, I, I>, < R, R’, P, P’, I, I; (<I>,<I’>); R, R’, P, P’, I, I’>
Ψ=
For values of criterion Ii,j the same remarks apply as for criterion Ri,j. Therefore, characteristic function (1) may be expressed as follows: , , , 0,0,75; () 1,0,75. ij Iij ij
Thus, values ΨI(xi,j) correspond to the highest prior ity of attention to be paid to the value of indicator Xj in the administrative decision-making process on the level of single region i.
Let us pass to the example. Task: to calculate the indication of critical values of threat indicators for the entire country, in compari son with the group of a macro-district, and regarding a specific region.
Given: Xi,j – a set of threat indicators values
Problem: <<Xi,j>,<Ri,j,R'i,j,Pi,j,P'i,j,Ii,j,I'i,j>> where R'i,j,P'i,j, I'i,j are criteria that underwent indication.
Axioms:
Linear normalizing for the entire country: (<Di,j>,<Ri,j>)
Linear normalizing for a macro-district: (<Di,j>,<Pi,j>)
Aggregation of criteria Ri,j, Pi,j: (<Ri,j,Pi,j>,<Ki,j>)
Linear normalizing for a specific region: (<Ki,j>,<Ii,j>)
Indication of critical threats values for the country: (<Ri,j>,<R'i,j>)
Indication of critical threats values for a macro-dis trict: (<Pi,j>,<P'i,j>)
Indication of critical threats values for a region: (<Ii,j>,<I'i,j>)
Search for a solution:
Axiom 7: (<Ii,j>,<I'i,j>)
Reduction by rule 5: Ri,j,R'i,j,Pi,j,P'i,j,Ii,j,Ii,j⇔Ri,j,R'i,j,Pi,j,P'i,j,Ii,j
Axiom 4: (<Ki,j>,<Ii,j>)
Axiom 3: (<Ri,j,Pi,j>,<Ki,j>)
Reduction by rule 5: Ri,j,R'i,j,Pi,j,P'i,j,Ri,j,Pi,j⇔Ri,j,R'i,j,Pi,j,P'i,j,Pi,j
Reduction by rule 5: Ri,j,R'i,j,Pi,j,P'i,j,Pi,j⇔Ri,j,R'i,j,Pi,j,P'i,j
Axiom 5: (<Ri,j>,<R'i,j>)
Reduction by rule 5: Ri,j,Ri,j,Pi,j,P'i,j⇔Ri,j,Pi,j,P'i,j
The execution sequence is found:
Axiom 2: (<Di,j>,<Pi,j>)
Axiom 6: (<Pi,j>,<P'i,j>)
Axiom 1: (<Di,j>,<Ri,j>)
Axiom 5: (<Ri,j>,<R'i,j>)
Axiom 3: (<Ri,j,Pi,j>,<Ki,j>)
Axiom 4: (<Ki,j>,<Ii,j>)
Axiom 7: (<Ii,j>,<I'i,j>)
Based on the analysis of existing data centres, their equipment and software, a high-quality solution is offered that provides a simple and flexible way to in tegrate heterogeneous information systems and their services into the World Data System. One of the key features of the proposed solution is the automation of algorithm construction of the actions sequence that executes users’ queries. A logical formalism has been created to describe this solution, and on its basis an inference method and a solution tree reconstruction mechanism have been developed.
The analysis of available technologies used for the implementation of distributed systems allowed the use of such a set of software solutions for practical implementation of this solution: UDDI for creating a registry of services entered into the system; WSDL for a unified description of services; SOAP for exchanging notifications between services; BPEL for the overall coordination of services. Intellectual agents can be implemented using JADE.
The implementation of the proposed solution will provide an opportunity to use all the integrated computing capacities and data storage systems of the World Data System in a comprehensive manner. Thus, users will be able to easily gain access to all the nec essary resources and services available to the system.
The research was conducted at the Faculty of Elec trical and Computer Engineering, Cracow University of Technology and was financially supported by the Ministry of Science and Higher Education, Republic of Poland (grant no. E-1/2022).
Grzegorz Nowakowski* – Faculty of Electrical and Computer Engineering, Cracow University of Technol ogy, Cracow, Poland, e-mail: gnowakowski@pk.edu.pl.
Sergii Telenyk – Faculty of Electrical and Computer Engineering, Cracow University of Technology, Cra cow, Poland, e-mail: stelenyk@pk.edu.pl.
Kostiantyn Yefremov – World Data Center for Geo informatics and Sustainable Development, Kyiv, Ukraine, e-mail: k.yefremov@wdc.org.ua.
Volodymyr Khmeliuk – National Technical Universi ty of Ukraine “Igor Sikorsky Kyiv Politechnic Institute” Kyiv, Ukraine, e-mail: hmelyuk@gmail.com.
* Corresponding author
[1] “About the Common Object Request Broker Ar chitecture Specification Version 3.4,” The Object Management Group (OMG), https://www.omg. org/spec/CORBA Accessed on: 2022-08-30.
[2] M. Juric, “BPEL and Java,” 2021, https://www. theserverside.com/news/1364554/BPEL-andJava Accessed on: 2022-08-30.
[3] “Data Integration Definition,” https://www. heavy.ai/technical-glossary/data-integration Accessed on: 2022-08-30.
[4] “About the OMG System Modeling Language Specification Version 1.6,” The Object Manage
ment Group (OMG), https://www.omg.org/ spec/SysML/About-SysML/ Accessed on: 202208-30.
[5] “SOAP Version 1.2 Part 1: Messaging Framework (Second Edition),” World Wide Web Consorti um (W3C), https://www.w3.org/TR/soap12part1/ Accessed on: 2022-08-30.
[6] “UDDI Version 3.0.2,” http://www.uddi.org/ pubs/uddi_v3.htm Accessed on: 2022-08-30.
[7] “Windows Communication Foundation Architec ture Overview,” Microsoft Corporation, http:// msdn.microsoft.com/en-us/library/ aa480210. aspx Accessed on: 2022-08-30.
[8] “Taverna – Apache Incubator,” https://incuba tor.apache.org/projects/taverna.html Accessed on: 2022-08-30.
[9] S. A. El-Seoud, H. F. El-Sofany, M. A. F. Abdelfattah and R. Mohamed, “Big Data and Cloud Comput ing: Trends and Challenges”, International Jour nal of Interactive Mobile Technologies, vol. 11, no. 2, 2017, 10.3991/ijim.v11i2.6561.
[10] S. Graham, Building Web services with Java: mak ing sense of XML, SOAP, WSDL, and UDDI, Sams Publishing, 2005.
[11] J. Greer, Web Services Description Language: 55 Most Asked Questions – What You Need To Know, Emereo Publishing, 2014.
[12] M. R. Kogalovsky and L. A. Kalinichenko,“Con ceptual and ontological modeling in informa tion systems”, Programming and Computer Soft ware, vol. 35, no. 5, 2009, 241–256, 10.1134/ S0361768809050016.
[13] J. Laznik, BPEL and Java Cookbook: Over 100 Rec ipes to Help You Enhance Your SOA Composite Ap plications with Java and BPEL, Packt Pub., 2013.
[14] A. Y. Levy, “Logic-Based Techniques in Data In tegration”. In: J. Minker (eds.), Logic-Based Arti ficial Intelligence, 2000, 575–595, 10.1007/9781-4615-1567-8_24.
[15] P. Maslianko, “Fundamentals of the Methodology of System Design of Information and Communi cation Systems”, Research Bulletin of the Nation al Technical University of Ukraine “Kyiv Polytech nic Institute”, vol. 6, 2007, 54–60.
[16] G. Nowakowski, “Open source relational da tabases and their capabilities in constructing a web-based system designed to support the functioning of a health clinic”, Czasopismo Tech niczne, vol. Automatyka Zeszyt 1-AC (2), 2013, 53–65.
[17]
G. Nowakowski, “Rest API safety assurance by means of HMAC mechanism”, Information Sys
tems in Management, vol. 5, no. 3, 2016, 358–369.
[18] G. Nowakowski, S. Telenyk, K. Yefremov and V. Khmeliuk, “The Approach to Applications Inte gration for World Data Center Interdisciplinary Scientific Investigations”. In: 2019 Federated Conference on Computer Science and Information Systems, 2019, 539–545, 10.15439/2019F71.
[19] S. Telenyk and O. Pavlov, “Algorithmization and IT in management,” Kyiv: Technics, 2002.
[20] N. Shakhovska, M. Medykovskyj, V. Lytvyn, “Da taspace Class Algebraic System for Modeling In tegrated Processes,” Journal of Applied Comput er Science, vol. 20, no. 1, 2012, 69–80, https:// it.p.lodz.pl/file.php/12/2012-1/JACS-1-2012Shakhovska.pdf Accessed on: 2022-09-11.
[21] N. Shakhovska, “Methods of Processing Data Us ing Consolidated Data Space”, Problems of Devel opment, National Academy of Sciences of Ukraine, Institute of Software of NAS of Ukraine, vol. 4, 2011, 72–84.
[22] S. Telenyk, G. Nowakowski, K. Yefremov and V. Khmeliuk, “Logics based application integration for interdisciplinary scientific investigations”. In: 2017 9th IEEE International Conference on Intelli gent Data Acquisition and Advanced Computing Sys tems: Technology and Applications (IDAACS), 2017, 1026–1031, 10.1109/IDAACS.2017.8095241.
[23] S. Telenyk, G. Nowakowski, E. Zharikov and J. Vovk, “Conceptual Foundations of the Use of Formal Models and Methods for the Rapid Cre ation of Web Applications”. In: 2019 10th IEEE International Conference on Intelligent Data Acquisition and Advanced Computing Systems: Technology and Applications (IDAACS), 2019, 512–518, 10.1109/IDAACS.2019.8924416.
[24] M. Ulema, B. Wu, J. Hwang, F. Lin and J.-H. Yi, “Next generation service overlay networks (NGSON)”, IEEE Communications Magazine, vol. 50, no. 1, 2012, 52–53, 10.1109/MCOM.2012.6122532.
[25] H. G. Miller and J. Veiga, “Cloud Computing: Will Commodity Services Benefit Users Long Term?”, IT Professional, vol. 11, no. 6, 2009, 57–59, 10.1109/MITP.2009.117.
[26] M. Zgurovsky, O. Akimova, A. Boldak, S. Voitko, O. Havrysh, O. Gluhanyk, I. Dzhygyrey, K. Yefre mov, A. Ishchenko, A. Kovalchuk, M. Kokorina, S. Lazareva, I. Makodym, T. Matorina, Y. Matsuki, A. Melnychenko, I. Pyshnohrayev, A. Prakhovnik, G. Statyukha and S. Tulchinska, “Part 2. Ukraine in Indicators of Sustainable Development (20112012)”. In: Analysis of Sustainable Development –Global and Regional Contexts, 2012.
[27] M. Z. Zgurovsky, A. D. Gvishiani, K. V. Yefremov and A. M. Pasichny, “Integration of the Ukrainian science into the world data system”, Cybernetics and Systems Analysis, vol. 46, no. 2, 2010, 211–219, 10.1007/s10559-010-9199-9.
[28] V. Ivanov and K. Smolander, “Implementation of a DevOps Pipeline for Serverless Applications”. In: M. Kuhrmann, K. Schneider, D. Pfahl, S. Amasa ki, M. Ciolkowski, R. Hebig, P. Tell, J. Klünder and S. Küpper (eds.), Product-Focused Software Pro cess Improvement. PROFES 2018, Lecture Notes in Computer Science, 2018, 48–64, 10.1007/9783-030-03673-7_4.
[29] C. Vassallo, S. Proksch, A. Jancso, H. C. Gall and M. Di Penta, “Configuration smells in continu ous delivery pipelines: a linter and a six-month study on GitLab”. In: Proceedings of the 28th ACM Joint Meeting on European Software Engineer ing Conference and Symposium on the Founda tions of Software Engineering, 2020, 327–337, 10.1145/3368089.3409709.
[30] N. Siegmund, N. Ruckel and J. Siegmund, “Di mensions of software configuration: on the configuration context in modern software de velopment”. In: Proceedings of the 28th ACM Joint Meeting on European Software Engineer ing Conference and Symposium on the Founda tions of Software Engineering, 2020, 338–349, 10.1145/3368089.3409675.
[31] S. P. Gilroy and B. A. Kaplan, “Furthering Open Science in Behavior Analysis: An Introduction and Tutorial for Using GitHub in Research”, Perspectives on Behavior Science, vol. 42, no. 3, 2019, 565–581, 10.1007/s40614-019-00202-5.
[32] M. Auch, M. Weber, P. Mandl and C. Wolff, “Sim ilarity-based analyses on software applications: A systematic literature review”, Journal of Sys tems and Software, vol. 168, 2020, 10.1016/ j.jss.2020.110669.
Publisher: Łukasiewicz
– Industrial Research Institute for Automation and Measurements PIAP