
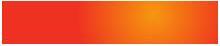

• automation
Editor-in-Chief
• automation
Editor-in-Chief
A peer-reviewed quarterly focusing on new achievements in the following fields:
• systems and control
• robotics
• autonomous systems
• mechatronics
Janusz Kacprzyk (Polish Academy of Sciences, Łukasiewicz-PIAP, Poland)
Advisory Board
• multiagent systems
• data sciences
Dimitar Filev (Research & Advenced Engineering, Ford Motor Company, USA)
Kaoru Hirota (Tokyo Institute of Technology, Japan)
Witold Pedrycz (ECERF, University of Alberta, Canada)
Co-Editors
Roman Szewczyk (Łukasiewicz-PIAP, Warsaw University of Technology, Poland)
Oscar Castillo (Tijuana Institute of Technology, Mexico)
Marek Zaremba (University of Quebec, Canada)
Executive Editor
Katarzyna Rzeplinska-Rykała, e-mail: office@jamris.org (Łukasiewicz-PIAP, Poland)
Associate Editor
Piotr Skrzypczynski (Poznan University of Technology, Poland)
Statistical Editor
Małgorzata Kaliczynska (Łukasiewicz-PIAP, Poland) ´
Editorial Board:
Chairman – Janusz Kacprzyk (Polish Academy of Sciences, Łukasiewicz-PIAP, Poland)
Plamen Angelov (Lancaster University, UK)
Adam Borkowski (Polish Academy of Sciences, Poland)
Wolfgang Borutzky (Fachhochschule Bonn-Rhein-Sieg, Germany)
Bice Cavallo (University of Naples Federico II, Italy)
Chin Chen Chang (Feng Chia University, Taiwan)
Jorge Manuel Miranda Dias (University of Coimbra, Portugal)
Andries Engelbrecht ( University of Stellenbosch, Republic of South Africa)
Pablo Estévez (University of Chile)
Bogdan Gabrys (Bournemouth University, UK)
Fernando Gomide (University of Campinas, Brazil)
Aboul Ella Hassanien (Cairo University, Egypt)
Joachim Hertzberg (Osnabrück University, Germany)
Tadeusz Kaczorek (Białystok University of Technology, Poland)
Nikola Kasabov (Auckland University of Technology, New Zealand)
Marian P. Kazmierkowski (Warsaw University of Technology, Poland)
Laszlo T. Kóczy (Szechenyi Istvan University, Gyor and Budapest University of Technology and Economics, Hungary)
Józef Korbicz (University of Zielona Góra, Poland)
Eckart Kramer (Fachhochschule Eberswalde, Germany)
Rudolf Kruse (Otto-von-Guericke-Universität, Germany)
Ching-Teng Lin (National Chiao-Tung University, Taiwan)
Piotr Kulczycki (AGH University of Science and Technology, Poland)
Andrew Kusiak (University of Iowa, USA)
Mark Last (Ben-Gurion University, Israel)
Anthony Maciejewski (Colorado State University, USA)
• decision-making and decision support •
• new computing paradigms •
Typesetting PanDawer, www.pandawer.pl
Webmaster
TOMP, www.tomp.pl
Editorial Office
ŁUKASIEWICZ Research Network
– Industrial Research Institute for Automation and Measurements PIAP
Al. Jerozolimskie 202, 02-486 Warsaw, Poland (www.jamris.org) tel. +48-22-8740109, e-mail: office@jamris.org
The reference version of the journal is e-version. Printed in 100 copies.
Articles are reviewed, excluding advertisements and descriptions of products. Papers published currently are available for non-commercial use under the Creative Commons Attribution-NonCommercial-NoDerivs 4.0 (CC BY-NC-ND 4.0) license. Details are available at: https://www.jamris.org/index.php/JAMRIS/ LicenseToPublish
Krzysztof Malinowski (Warsaw University of Technology, Poland)
Andrzej Masłowski (Warsaw University of Technology, Poland)
Patricia Melin (Tijuana Institute of Technology, Mexico)
Fazel Naghdy (University of Wollongong, Australia)
Zbigniew Nahorski (Polish Academy of Sciences, Poland)
Nadia Nedjah (State University of Rio de Janeiro, Brazil)
Dmitry A. Novikov (Institute of Control Sciences, Russian Academy of Sciences, Russia)
Duc Truong Pham (Birmingham University, UK)
Lech Polkowski (University of Warmia and Mazury, Poland)
Alain Pruski (University of Metz, France)
Rita Ribeiro (UNINOVA, Instituto de Desenvolvimento de Novas Tecnologias, Portugal)
Imre Rudas (Óbuda University, Hungary)
Leszek Rutkowski (Czestochowa University of Technology, Poland)
Alessandro Saffiotti (Örebro University, Sweden)
Klaus Schilling (Julius-Maximilians-University Wuerzburg, Germany)
Vassil Sgurev (Bulgarian Academy of Sciences, Department of Intelligent Systems, Bulgaria)
Helena Szczerbicka (Leibniz Universität, Germany)
Ryszard Tadeusiewicz (AGH University of Science and Technology, Poland)
Stanisław Tarasiewicz (University of Laval, Canada)
Piotr Tatjewski (Warsaw University of Technology, Poland)
Rene Wamkeue (University of Quebec, Canada)
Janusz Zalewski (Florida Gulf Coast University, USA)
Teresa Zielinska (Warsaw University of Technology, Poland) ´ Publisher:
Volume 16, N°1, 2022
DOI: 10.14313/JAMRIS/1-2022
Processing of LiDAR and IMU data for target detection and odometry of a mobile robot
Nicola Ivan Giannoccaro, Takeshi Nishida, Aimè LayEkuakille, Ramiro Velazquez, Paolo Visconti
DOI: 10.14313/JAMRIS/1-2022/1
Development of a modified ant colony algorithm for order scheduling
Igor Korobiichuk, Serhii Hrybkov, Olga Seidykh, Volodymyr Ovcharuk, Andrii Ovcharuk
DOI: 10.14313/JAMRIS/1-2022/6
Development of an Omnidirectional AGV by Applying ORB-SLAM for Navigation Under ROS Framework
Pan-Long Wu, Jyun-Jhen Li, Jin-Siang Shaw
DOI: 10.14313/JAMRIS/1-2022/2
Autonomous Anomaly Detection System for Crime Monitoring and Alert Generation
Jyoti Kukade, Swapnil Soner, Sagar Pandya
DOI: 10.14313/JAMRIS/1-2022/7
Selection of Manipulator Configuration for a Portable Robot for Special Tasks
Tomasz Krakówka, Andrzej Typiak, Maciej Cader
DOI: 10.14313/JAMRIS/1-2022/3
Migrating Monoliths to Microservices Integrating Robotic Process Automation into the Migration Approach
Burkhard Bamberger, Bastian Körber
DOI: 10.14313/JAMRIS/1-2022/8
A Scalable Tree Based Path Planning for a Service Robot
A. A. Nippun Kumaar, Sreeja Kochuvila, S. R. Nagaraja
DOI: 10.14313/JAMRIS/1-2022/4
Fuzzy Automatic Control of the Pyrolysis Process for the Municipal Solid Waste of Variable Composition
Oleksiy Kozlov, Yuriy Kondratenko, Hanna Lysiuk, Viktoriia Kryvda, Oksana Maksymova
DOI: 10.14313/JAMRIS/1-2022/9
Prototype and Design of Six-Axis Robotic Manipulator
Mateusz Pająk, Marcin Racław, Robert Piotrowski
DOI: 10.14313/JAMRIS/1-2022/5
Submitted: 10th June 2021; accepted: 2nd August 2022
Nicola Ivan Giannoccaro, Takeshi Nishida, Aimè Lay-Ekuakille, Ramiro Velazquez, Paolo ViscontiDOI: 10.14313/JAMRIS/1-2022/1
Abstract:
In this paper, the processing of the data of a 3D light detection and distance measurement (LiDAR) sensor mounted on a mobile robot is demonstrated, introducing an innovative methodology to manage the data and extract useful information. The LiDAR sensor is placed on a mobile robot which has a modular design that permits the easy change of the number of wheels, was designed to travel through several environments, and saves energy by changing the number and arrangement of the wheels in each environment. In addition, the robot can recognize landmarks in a structured environment by using a classification technique on each frame acquired by the LiDAR. Furthermore, considering the experimental tests, a new simple algorithm based on the LiDAR data processing together with the inertial data (IMU sensor) through a Kalman filter is proposed to characterize the robot’s pose by the surrounding environment with fixed landmarks. Finally, the limits of the proposed algorithm have been analyzed, highlighting new improvements in the future prospective development for permitting autonomous navigation and environment perception with a simple, modular, and low-cost device.
Keywords: mechatronics device, LiDAR sensor, measurement processing, 3D object recognition, robotic implementation.
LiDAR sensors have been firmly established as an essential component of many mapping, navigation, and localization applications for autonomous vehicles, as demonstrated by the increasing number of recent studies focused on the usage of LiDAR data for different applications. This popularity is mainly due to the improvements in LiDAR performance in terms of range detection, accuracy, power consumption, and physical features such as dimension and weight. This technology is considered to be state of the art, considering that only very recent contributions permit us to compare different algorithms, models, methodologies, and techniques for different applications of LiDAR in the autonomous vehicles. Goelles et al. present a systematic review on faults and suitable detection and recovery methods for automotive perception sensors with a focus on LiDAR to review the
state-of-the-art technology and identify promising research opportunities [1]. Raj et al. explore LiDAR scanning mechanisms employed in LiDAR technology from past research to current commercial products, revealing that electro-mechanical scanning is the most prominent technology in use today [2]. Another recent review provides a comprehensive survey of the Simultaneous Localization and Mapping (SLAM) used for many applications, including mobile robotics, self-driving cars, unmanned aerial vehicles, or autonomous underwater vehicles, focusing on solutions a sensor fusion between vision and LiDAR [3].
As demonstrated by recent reviews, applications of LiDAR are becoming significant, and it is important to acquire new ideas and algorithms related to the use of this data. Among the various fields of LiDAR application, mobile robots form an important category. Mobile robots often have to analyze the surrounding environment to get information about the presence of objects and the trajectory of the robot concerning landmarks or mobile targets. Often in applications, it is necessary to measure the environment in detail when it is difficult to identify the position by global navigation satellite system (GNSS) signals; in these cases, the generation of the three-dimensional digital map by LiDAR is a good solution, as shown in several papers [4-11]. Methods of measuring the surrounding environment using 2D LiDAR have been proposed for four-wheeled mobile robots [7-8], for an unmanned aerial vehicle (UAV) [7], and for mobile robots with a rocker-bogie mechanism [8]. Various techniques of processing and filtering the sensor’s data have also been studied and presented [12].
This research focuses on a simple, low-cost robot designed and introduced by Giannoccaro et al. in 2019, driven by ROS and realized with a modularized wheel and a frame for connecting them and for supporting a scanning LiDAR [13]. A Lidar Hokuyo 3D sensor, which has been increasingly used in recent years, especially for mapping and operations in the automation field, is installed on the robot case. Moreover, an inertial measurement unit (IMU sensor) is present on the robot, and supplies the three linear accelerations along the three axes [x, y, z] and the three angular accelerations along the same axes with a given sampling frequency.
The objectives of the research work are to provide an algorithm capable of estimating the odometry of the mobile robot with high reliability during the jour-
neys made. This practice is very important in the robotic field: in the literature, there are various SLAM algorithms and, more generally, algorithms aimed at reconstructing the vehicle’s trajectory. In most cases, IMU sensors are used alongside either GPS data or, more rarely, data provided by fixed cameras that inspect a certain control environment to obtain the input data of the algorithms. It is therefore clear that both methodologies have limits. In the first case, the method would not apply to indoor environments to be inspected due to the difficulty of the GPS signal to reach the robot, while in the second case, the area to be inspected should be equipped with element support, such as cameras or other types of sensors, binding the research to very limited environments. Preliminary results obtained by using only LiDAR data for the proposed robot are shown in a 2020 study by Giannoccaro et al. [14]. The algorithm presented here, on the other hand, uses the IMU sensor and the LiDAR sensor, with the latter being capable of providing the frames containing point cloud data with a defined sampling rate, thus characterizing the neighboring environment. This makes it more versatile in eliminating critical issues, with a similar methodology to that used for an array of ultrasonic sensors for scanning external environments, determining useful characteristics for the reconstruction of simple external reference surfaces [15-17]. The algorithm makes use of a linear Kalman filter, which evaluates the dynamic state of a system starting from measurements affected by noise. In the test proposed, in the Kalman filter, greater weight was given to the filter correction phase using the LiDAR observations compared to the prediction phase, because the IMU model installed on the robot was not suitable for estimating the installation due to its noise level, as demonstrated by the first preliminary experimental tests. With an opportune preliminary sensor accuracy evaluation, the statistical proposed algorithm could be adapted and generalized to every situation.
The use of the Kalman filter for improving the fusion of the data from LiDAR and other inertial sensors has recently become a highly used and investigated modality for locating and estimating the position of robots or mobile vehicles. Some contributions recently published show innovative procedures that use this strategy for specific applications. In one paper, the author proposes a method for autonomous cars localization based on the fusion of LiDAR and radar measurement data using the unscented Kalman filter for object detection; in this case, the LiDAR and rad fusion obtained by the Kalman filter provide pole-like static object pose estimations that are well suited to serve as landmarks for vehicle localization in urban environments [18]. In another paper, the authors introduce an odometry procedure based on the fusion of LiDAR feature points with IMU data using a tightly coupled, iterated, extended Kalman filter to allow robust navigation in UAV flight [19].
In a paper by Chauchat et al., the authors propose a localization algorithm based upon the Kalman filter to improve smoothing on the fusion of IMU and
LiDAR data successfully tested on an equipped car [20]. Muro et al. present a method for moving-object detection and tracking using a LiDAR mounted on a motorcycle and the distortion in the scanning LiDAR data is corrected by estimating the pose of the motorcycle in a period that the LiDAR scan period using information from an IMU via the extended Kalman filter [21]. Zhang et al. introduce a multi-level sensor data fusion method for real-time positioning and mapping [22]. In this method, coordinates are transformed based on the pre-integration results of IMU data in data pre-processing to register the laser point cloud. Features of the laser point cloud are sampled to reduce the computation of point cloud matching. Next, the robot pose is obtained by combining IMU and LiDAR observations with an unscented Kalman filter algorithm to improve the loop closure detection effect.
In another paper, the authors propose an adaptive pose fusion (APF) method to fuse the robot’s pose and use the optimized pose to construct an indoor map [23]. Firstly, the proposed method calculates the robot’s pose by the camera and inertial measurement unit (IMU), respectively. Then, the pose fusion method is adaptively selected according to the motion state of the robot. When the robot is in a static state, the proposed method directly uses the extended Kalman filter (EKF) method to fuse camera and IMU data, and when the robot is in a motive state, a weighted pose fusion method is used to fuse camera and IMU data. Next, the fusion-optimized pose is used to correct the distance and azimuth angle of the laser points obtained by LiDAR.
Morita et al. propose a robust model predictive control for the trajectory tracking of a small-scale autonomous bulldozer in the presence of perturbations; the pose estimation, for control feedback, is based on sensor data fusion performed by an extended Kalman filter, which processes inertial measurement unit (IMU) and light detection and ranging (LiDAR) measurements [24]. In another paper, the authors introduce a methodology for positioning and autonomous navigation of Unmanned Surface Vehicle based on two-dimensional lidar combined with IMU to perceive the surrounding environment, and the Extended Kalman Filter algorithm is used for the fusion of map matching data and IMU pre-integration data [25].
In this work, the use of the Kalman filter for combining IMU and LiDAR data is applied to a mobile robot specifically built with the characteristics of low cost, modularity, and simplicity, with the aim of using the detection of targets (fixed vertical flat planes), often available in real scenarios. For an indoor scenario, this could be the walls of rooms, or, for an outdoor scenario, the external walls of a building, a deposit, a house of tools, etc., for improving the estimation of the robot trajectory. The proposed procedure is simple, without relevant post-processing of the IMU and LiDAR data, and it uses the vertical planes to simplify the post-processing that requires only classical tools and a low computational effort also compatible with a low-cost solution such as the proposed one.
This paper will deal with the following topics: in Section 2, a description of the mobile robot and the considered LiDAR and IMU sensors; in Section 3, a strategy for a pre-processing of the LiDAR data; in Section 4, the innovative algorithm based on LiDAR and IMU data for odometry and target recognition; in Section 5, the results of experimental tests; and, finally, in Section 6, the conclusions of the work.
The mobile robot prototype used was entirely designed and built in the laboratories of the Kyushu Institute of Technology [13, 14]. It is shown in Figures 1 and 2, and it has the specific characteristic that its frame has been designed so that the number of drive wheels can be changed according to the environment in which the robot is travelling. Depending on the environment, the robot can be easily changed by the user to two energy-efficient wheel versions, six-wheel versions with high running ability against complicated terrain, or four-wheel versions with intermediate capabilities [13, 14]. The aim of this modularized, low-cost asset was to minimize the oscillations on the robot and minimize the errors of acquisition of sensors mounted on the robot during exploration in unstructured environments such as a forest environment [24]. The structure has an almost cubic, hollow case, which houses part of the electronic components for the user to control the robot, as well as the battery pack and a red safety button. In addition to the internal cavity, there is also a cavity on the upper part accessible by opening a hatch. This inlet is used mostly to lay the terminal with which to launch the various codes for the management of locomotion and data recording. The LiDAR sensor with which the robot is equipped is the Hokuyo XVT-35 LX 3D LiDAR sensor model [25]; it is installed in the front part of the case (Fig. 2) and housed in a structure designed to avoid any knocks and damage to the sensor during the robot’s motion [13, 14]. The solid angle that can be inspected with this LiDAR model is provided by a maximum horizontal angle of 210° and a maximum vertical angle of 35° (the central vertical axis is 15° over the horizon). Regarding the inertial measurement unit, the model MPU-6500 is installed on the robot [26]. The IMU sensor is fixed to the robot by bolting to avoid unwanted movements by the unit that would lead to incorrect measurements, and is located in the front of the case (Figure 2), near the LiDAR sensor and in an area as free from vibrations as possible.
The locomotion of the robot is due to two main circuits: a power supply circuit and a drive wheel control circuit. For the robot, each of the driving wheels is independently powered by a D.C. motor. Each D.C. motor is powered by a Cytron MDD10A board [27]. The MDD10A board may control two high-current D.C. motors, supplying up to 10A continuously. As for the control circuit, the Arduino MEGA electronic board is used. Each power supply board is capable of controlling two motors; therefore, a total of six PWM outputs are required for speed control and six digital outputs are required for direction control. The Arduino MEGA electronic board is used for the control circuit. A scheme of connection for the controller and motors is shown in Figure 3; the positive board terminal is connected to an emergency stop button referred to at the beginning of this chapter, also known as the “mushroom” button. During operation, the button maintains the continuity of the positive power cable, but, if pressed, immediately interrupts it, opening the power circuit so that the robot stops. Finally, the battery fitted to the prototype is a 24V 3800mAh Batteryspace Panasonic.
a)
To control the robot, one laptop computer with Intel Core m5 (1.10 GHz) and Ubuntu 14.04 LTS has been used, placing it at the top of the robot (as shown in Fig. 1 a) and connecting it to the boards and sensors through a USB cable. The robot operating system (ROS) [28] was adopted as middleware to manage the locomotion and control system and to acquire the data from the LiDAR and IMU sensors. The formats of the files containing IMU data and LiDAR data are different. For the former, a file in “.csv” format shows the linear acceleration data relating to the three axes in the first three columns and the angular velocity data relating to the same axes in the last three. For the latter, at first, a file with the “.bag” extension, which must be subsequently converted into as many “.pcd” files as the number of frames acquired. Each acquisition file includes a reference about the P.C. processor clock to synchronize the different files of the sensors. Finally, a joystick connected to the laptop through the USB gate has been considered to manually driving the robot during the experimental tests below shown.
The visualization of the LiDAR data scans may be carried out with various methodologies; one of the most common makes use of the A.R. Viz Virtual Studio software, with which it is possible to dynamically reproduce the captured frames over time [29]. This method has an immediate visual impact but is not functional for the purposes of this research work. Rather, it is necessary to thoroughly process the single frame and then, at a later stage, draw conclusions on the entire duration of the test. To do this, a first code was written in a Matlab environment [30] that performs the rotation of the image 180° concerning the x-axis of the robot (this operation is necessary as the LiDAR is mounted upside down due to the confirmation of the structure in which it is housed, see Figure 2) and the plotting of the resulting Point Cloud Data. Moreover, the first preliminary tests were carried out by moving the robot using a joystick and acquiring it from the Lidar. Some frames of the path in Figure 4 (which coincides with the x-axis of the chosen reference system, with the z-axis upward, and the y-axis completes the cartesian reference system) have been preliminarily analyzed, and one of them is shown in Fig. 5a. The image of a frame (PCD) shows many disturbances in the surrounding environment, including the ground reflection. To this end, a preprocessing denoising and filtering code has been used to eliminate the presence of the supporting ground and to reduce the noise and the disturbing elements in the following target recognition phase. This operation is also of fundamental importance, as the data initially have different outliers that could compromise subsequent processing operations. The effect of pre-processing is evident in Fig. 5 a) and b) where the same frame is shown before and after the pre-processing process: the underlying ground and many disturbances of the surrounding environment disappear, leaving only the tree and the boulders to the left and right sides of the robot.
b)
position of the landmarks selected as a reference. It is clear that both solutions have limitations; the use of a GPS is versatile and allows the scanning of very large areas without apparent significant problems, and this sensor is ideal for testing outdoor but could present several complications for indoor explorations due to the difficulty of receiving the signal from the satellite. On the other hand, even the use of laser sensors or fixed cameras for the recognition of features present in the environment is binding, as it would be necessary to equip the environment with certain equipment prior to the exploration phase, which could be difficult to implement in some cases. Furthermore, the inability of these devices to follow the robot’s motion limits the exploration area to the places where these elements are present.
The use of a LiDAR sensor to operate the robot observations partially solves the problems just outlined. The sensor is installed directly on the external structure of the robot, and this allows for greater flexibility in the choice of landmarks that may not even be selected upstream of the exploration. Furthermore, there is no difficulty related to the reception of external signals, and the post-processing operations can also be carried out offline for all applications that do not require the autonomous motion of the robot.
b)
4. Algorithm for robot odometry and target following based on LiDAR and IMU data
4. Algorithm for robot odometry and target following based on LiDAR and IMU data
The algorithm proposed here can be categorized under the category of Simultaneous Localization and Mapping (SLAM) algorithms, but with some substantial differences: SLAM algorithms aim to estimate the pose of the robot and to carry out the mapping of the environment starting from the checks and observations performed by the robot during exploration. Both types of input data are affected by statistical noise, and this makes it necessary to use statistical filters for the processing of probability densities. The algorithm proposed here considers the use of a linear Kalman filter for updating the iterative process for estimating the pose and from the map. The novelty proposed in this research work consists of the use of a LiDAR sensor to perform the “observations” of the surrounding environment; the most common odometric techniques make use of Dead Reckoning methodologies in which the inertial data are corrected, using a Kalman filter, using data acquired by GPS systems. Another technique is linked to the SLAM algorithms implemented, considering “controls” of an inertial nature and “observations” of the environment performed with laser sensors or fixed cameras that provide the
The algorithm proposed here can be categorized under the category of Simultaneous Localization and Mapping (SLAM) algorithms, but with some substantial differences: SLAM algorithms aim to estimate the pose of the robot and to carry out the mapping of the environment starting from the checks and observations performed by the robot during exploration. Both types of input data are affected by statistical noise, and this makes it necessary to use statistical filters for the processing of probability densities. The algorithm proposed here considers the use of a linear Kalman filter for updating the iterative process for estimating the pose and from the map. The novelty proposed in this research work consists of the use of a LiDAR sensor to perform the "observations" of the surrounding environment; the most common odometric techniques make use of Dead Reckoning methodologies in which the inertial data are corrected, using a Kalman filter, using data acquired by GPS systems. Another technique is linked to the SLAM algorithms implemented, considering "controls" of an inertial nature and "observations" of the environment performed with laser sensors or fixed cameras that provide the position of the landmarks selected as a reference. It is clear that both solutions have limitations; the use of a GPS is versatile and allows the scanning of very large areas without apparent significant problems, and this sensor is ideal for testing outdoor but could present several complications for indoor explorations due to the difficulty of receiving the signal from the satellite. On the other hand, even the use of laser sensors or fixed cameras for the recognition of features present in the environment is binding, as it would be necessary to equip the environment with certain equipment prior to the exploration phase, which could be difficult to implement in some cases. Furthermore, the inability of these devices to follow the robot's motion limits the exploration area to the places where these elements are present.
The algorithm proposed here can be categorized under the category of Simultaneous Localization and Mapping (SLAM) algorithms, but with some substantial differences: SLAM algorithms aim to estimate the pose of the robot and to carry out the mapping of the environment starting from the checks and observations performed by the robot during exploration. Both types of input data are affected by statistical noise, and this makes it necessary to use statistical filters for the processing of probability densities. The algorithm proposed here considers the use of a linear Kalman filter for updating the iterative process for estimating the pose and from the map. The novelty proposed in this research work consists of the use of a LiDAR sensor to perform the "observations" of the surrounding environment; the most common odometric techniques make use of Dead Reckoning methodologies in which the inertial data are corrected, using a Kalman filter, using data acquired by GPS systems. Another technique is linked to the SLAM algorithms implemented, considering "controls" of an inertial nature and "observations" of the environment performed with laser sensors or fixed cameras that provide the position of the landmarks selected as a reference. It is clear that both solutions have limitations; the use of a GPS is versatile and allows the scanning of very large areas without apparent significant problems, and this sensor is ideal for testing outdoor but could present several complications for indoor explorations due to the difficulty of receiving the signal from the satellite. On the other hand, even the use of laser sensors or fixed cameras for the recognition of features present in the environment is binding, as it would be necessary to equip the environment with certain equipment prior to the exploration phase, which could be difficult to implement in some cases. Furthermore, the inability of these devices to follow the robot's motion limits the exploration area to the places where these elements are present.
The algorithm proposed here can be categorized under the category of Simultaneous Localization and Mapping (SLAM) algorithms, but with some substantial differences: SLAM algorithms aim to estimate the pose of the robot and to carry out the mapping of the environment starting from the checks and observations performed by the robot during exploration. Both types of input data are affected by statistical noise, and this makes it necessary to use statistical filters for the processing of probability densities. The algorithm proposed here considers the use of a linear Kalman filter for updating the iterative process for estimating the pose and from the map. The novelty proposed in this research work consists of the use of a LiDAR sensor to perform the "observations" of the surrounding environment; the most common odometric techniques make use of Dead Reckoning methodologies in which the inertial data are corrected, using a Kalman filter, using data acquired by GPS systems. Another technique is linked to the SLAM algorithms implemented, considering "controls" of an inertial nature and "observations" of the environment performed with laser sensors or fixed cameras that provide the position of the landmarks selected as a reference. It is clear that both solutions have limitations; the use of a GPS is versatile and allows the scanning of very large areas without apparent significant problems, and this sensor is ideal for testing outdoor but could present several complications for indoor explorations due to the difficulty of receiving the signal from the satellite. On the other hand, even the use of laser sensors or fixed cameras for the recognition of features present in the environment is binding, as it would be necessary to equip the environment with certain equipment prior to the exploration phase, which could be difficult to implement in some cases. Furthermore, the inability of these devices to follow the robot's motion limits the exploration area to the places where these elements are present.
external structure of the robot, and this allows for greater flexibility in the choice of landmarks that may not even be selected upstream of the exploration. Furthermore, there is no difficulty related to the reception of external signals, and the post-processing operations can also be carried out offline for all applications that do not require the autonomous motion of the robot.
external structure of the robot, and this allows for greater flexibility in the choice of landmarks that may not even be selected upstream of the exploration. Furthermore, there is no difficulty related to the reception of external signals, and the post-processing operations can also be carried out offline for all applications that do not require the autonomous motion of the robot.
external structure of the robot, and this allows for greater flexibility in the choice of landmarks that may not even be selected upstream of the exploration. Furthermore, there is no difficulty related to the reception of external signals, and the post-processing operations can also be carried out offline for all applications that do not require the autonomous motion of the robot.
4.1. The kinematic model for robot controls using inertial measurements
4.1. The kinematic model for robot controls using inertial measurements
The controls on the installation of the robot can be carried out using inertial data acquired during the explorations by the IMU sensor that measures the triaxial linear and angular accelerations. A double integration operation must then be performed over time to obtain the three spatial coordinates that will identify the pose of the robot from the measured accelerations. The system and output equations written for a generic dynamic system in the state space form (1) can be particularized for the kinematic of the robot as expressed in (2) and (3), where x,y,z, vx,vy,vz, ax, ay, az, are the displacement, velocity and acceleration components, along with the xyz cartesian reference system.
The controls on the installation of the robot can be carried out using inertial data acquired during the explorations by the IMU sensor that measures the triaxial linear and angular accelerations. A double integration operation must then be performed over time to obtain the three spatial coordinates that will identify the pose of the robot from the measured accelerations. The system and output equations written for a generic dynamic system in the state space form (1) can be particularized for the kinematic of the robot as expressed in (2) and (3), where x,y,z, vx,vy,vz, ax, ay, az, are the displacement, velocity and acceleration components, along with the xyz cartesian reference system.
The controls on the installation of the robot can be carried out using inertial data acquired during the explorations by the IMU sensor that measures the triaxial linear and angular accelerations. A double integration operation must then be performed over time to obtain the three spatial coordinates that will identify the pose of the robot from the measured accelerations. The system and output equations written for a generic dynamic system in the state space form (1) can be particularized for the kinematic of the robot as expressed in (2) and (3), where x,y,z, vx,vy,vz, ax, ay, az, are the displacement, velocity and acceleration components, along with the xyz cartesian reference system.
The controls on the installation of the robot can be carried out using inertial data acquired during the explorations by the IMU sensor that measures the triaxial linear and angular accelerations. A double integration operation must then be performed over time to obtain the three spatial coordinates that will identify the pose of the robot from the measured accelerations. The system and output equations written for a generic dynamic system in the state space form (1) can be particularized for the kinematic of the robot as expressed in (2) and (3), where x,y,z, vx,vy,vz, ax, ay, az, are the displacement, velocity and acceleration components, along with the xyz cartesian reference system.
(1)
(2)
(3)
The model has then been discretized by using the forward Euler method, which is among the simplest, and provides a coherent model for sampling sufficiently high frequencies. Naming the sampling period Ts, the discretised kinematic model is expressed in (4) where [I+ATs] and B.T.s are expressed in (5) and (6), taking into account (2), (3).
The model has then been discretized by using the forward Euler method, which is among the simplest, and provides a coherent model for sampling sufficiently high frequencies. Naming the sampling period Ts, the discretised kinematic model is expressed in (4) where [I+ATs] and B.T.s are expressed in (5) and (6), taking into account (2), (3).
The model has then been discretized by using the forward Euler method, which is among the simplest, and provides a coherent model for sampling sufficiently high frequencies. Naming the sampling period Ts, the discretised kinematic model is expressed in (4) where [I+ATs] and B.T.s are expressed in (5) and (6), taking into account (2), (3).
The model has then been discretized by using the forward Euler method, which is among the simplest, and provides a coherent model for sampling suffi-
x(k+1)=[I+A Ts ]x(k)+BTs u(k) (4)
x(k+1)=[I+A Ts ]x(k)+BTs u(k) (4)
ciently high frequencies. Naming the sampling period Ts, the discretised kinematic model is expressed in (4) where [I+ATs] and B.T.s are expressed in (5) and (6), taking into account (2), (3). x(k+1)=[I+A
In this way, the matrices to implement the prediction phase of the Kalman filter through the realization of a kinematic model have been obtained.
In this way, the matrices to implement the prediction phase of the Kalman filter through the realization of a kinematic model have been obtained.
In this way, the matrices to implement the prediction phase of the Kalman filter through the realization of a kinematic model have been obtained.
The basic principle of the proposed algorithm is similar to that of robot observations in SLAM problems, but instead of evaluating the presence and position of landmarks through laser transducers, they are evaluated by applying a specific algorithm to each frame acquired during the exploration by the LiDAR sensor.
The basic principle of the proposed algorithm is similar to that of robot observations in SLAM problems, but instead of evaluating the presence and position of landmarks through laser transducers, they are evaluated by applying a specific algorithm to each frame acquired during the exploration by the LiDAR sensor.
The algorithm is mainly efficient for indoor tests, in which it is possible to identify some fixed reference structures concerning where to identify the installation of the robot. In particular, it is necessary to be in the presence of vertical flat walls, as is the case for any indoor test, to evaluate the intersection between two of them and consider this as a landmark to identify the spatial coordinates of the robot's center of gravity. The results that will be shown below refer to outdoor tests, although, as previously stated, the algorithm proposed for robot observations is more efficient for indoor environments, given the almost certain presence of vertical walls that can be taken as a reference. However, an environment of this type is preferred to have more space available for exploration while still having references available to use. The setting chosen for the explorations is one of the courtyards present in the Kyushu Institute of Technology depicted in Fig. 6a; Fig.6b shows an image from above of the courtyard explored. The two vertical walls are highlighted in red in Fig 6b; these walls, in most of the acquired experimental tests, have been taken as a reference for the odometric information of the robot acquired by LiDAR.
The algorithm is mainly efficient for indoor tests, in which it is possible to identify some fixed reference structures concerning where to identify the installation of the robot. In particular, it is necessary to be in the presence of vertical flat walls, as is the case for any indoor test, to evaluate the intersection between two of them and consider this as a landmark to identify the spatial coordinates of the robot's center of gravity. The results that will be shown below refer to outdoor tests, although, as previously stated, the algorithm proposed for robot observations is more efficient for indoor environments, given the almost certain presence of vertical walls that can be taken as a reference. However, an environment of this type is preferred to have more space available for exploration while still having references available to use. The setting chosen for the explorations is one of the courtyards present in the Kyushu Institute of Technology depicted in Fig. 6a; Fig.6b shows an image from above of the courtyard explored. The two vertical walls are highlighted in red in Fig 6b; these walls, in most of the acquired experimental tests, have been taken as a reference for the odometric information of the robot acquired by LiDAR.
The basic principle of the proposed algorithm is similar to that of robot observations in SLAM problems, but instead of evaluating the presence and position of landmarks through laser transducers, they are evaluated by applying a specific algorithm to each frame acquired during the exploration by the LiDAR sensor. The algorithm is mainly efficient for indoor tests, in which it is possible to identify some fixed reference structures concerning where to identify the installation of the robot. In particular, it is necessary to be in the presence of vertical flat walls, as is the case for any indoor test, to evaluate the intersection between two of them and consider this as a landmark to identify the spatial coordinates of the robot’s center of gravity. The results that will be shown below refer to outdoor tests, although, as previously stated, the algorithm proposed for robot observations is more efficient for indoor environments, given the almost certain presence of vertical walls that can be taken as a reference. However, an environment of this type is preferred to have more space available for exploration while still having references available to use. The setting chosen for the explorations is one of the courtyards present in the Kyushu Institute of Technology depicted in Fig. 6a; Fig.6b shows an image from above of the courtyard explored. The two vertical walls are highlighted in red in Fig 6b; these walls, in most of the acquired experimental tests, have been taken as a reference for the odometric information of the robot acquired by LiDAR.
It is possible to trace the coordinates of the landmark relative to the robot, since for each pcd frame, the origin of the Cartesian axes coincides with the center of gravity of the LiDAR, which is installed on the robot itself. For this reason, it is legitimate to consider, omitting a negligible error, the origin of the axes centered in the center of gravity of the rover. For each LiDAR-acquired frame, the algorithm before performs the pre-processing filtering described in Section 3, then also removes the points present in a sphere with the center at the origin of the axes and a radius of 1 meter. This operation is necessary since the LiDAR also “observes” some lateral protrusions of the robot that fall within the sensor's field of view. The postprocessing involves the recognition of two flat vertical walls possibly present in the pcd frame under examination. The function used is also, in this case, the “pcfitplane”, providing in input a maximum distance between inliers and fitting plane equal to 0.5 meters [30]. Since, after some tests, it was noticed how this algorithm was not robust and could not capture the plans of interest, a more restrictive condition was inserted: the plan fitting process is stopped only if the mean square error of the inliers points of the model is less than a certain threshold. After this, the algorithm became more robust. In some cases, it is possible to insert a further condition that provides for the orthogonality between the two flat walls (the typical situation for indoor environments) to further strengthen the algorithm. To divide the points, a clustering technique aimed at homogeneous grouping objects starting from a set of data has been used. Clustering techniques are based on measures relating to the similarity between the elements The discriminating variable for the grouping of the elements depends on the problem proposed; in the case of point cloud data, it is the distance between the points. In this work, the clustering has been carried out by using the “kmeans” function [15-17, 30]. The “kmeans” function takes as input the coordinates of the point cloud on which clustering will be performed and the desired number of partitions, while it outputs the indices of the points indicative of the assignment of points to one of the partitions, the coordinates of the centroids, and the distance of all points from each centroid. In this case, fixing the number of partitions
It is possible to trace the coordinates of the landmark relative to the robot, since for each pcd frame, the origin of the Cartesian axes coincides with the center of gravity of the LiDAR, which is installed on the robot itself. For this reason, it is legitimate to consider, omitting a negligible error, the origin of the axes centered in the center of gravity of the rover. For each LiDAR-acquired frame, the algorithm before performs the pre-processing filtering described in Section 3, then also removes the points present in a sphere with the center at the origin of the axes and a radius of 1 meter. This operation is necessary since the LiDAR also “observes” some lateral protrusions of the robot that fall within the sensor's field of view. The postprocessing involves the recognition of two flat vertical walls possibly present in the pcd frame under examination. The function used is also, in this case, the “pcfitplane”, providing in input a maximum distance between inliers and fitting plane equal to 0.5 meters [30]. Since, after some tests, it was noticed how this algorithm was not robust and could not capture the plans of interest, a more restrictive condition was inserted: the plan fitting process is stopped only if the mean square error of the inliers points of the model is less than a certain threshold. After this, the algorithm became more robust. In some cases, it is possible to insert a further condition that provides for the orthogonality between the two flat walls (the typical situation for indoor environments) to further strengthen the algorithm. To divide the points, a clustering technique aimed at homogeneous grouping objects starting from a set of data has been used. Clustering techniques are based on measures relating to the similarity between the elements The discriminating variable for the grouping of the elements depends on the problem proposed; in the case of point cloud data, it is the distance between the points. In this work, the clustering has been carried out by using the “kmeans” function [15-17, 30]. The “kmeans” function takes as input the coordinates of the point cloud on which clustering will be performed and the desired number of partitions, while it outputs the indices of the points indicative of the assignment of points to one of the partitions, the coordinates of the centroids, and the distance of all points from each centroid. In this case, fixing the number of partitions
It is possible to trace the coordinates of the landmark relative to the robot, since for each pcd frame, the origin of the Cartesian axes coincides with the center of gravity of the LiDAR, which is installed on the robot itself. For this reason, it is legitimate to consider, omitting a negligible error, the origin of the axes centered in the center of gravity of the rover. For each LiDAR-acquired frame, the algorithm before performs the pre-processing filtering described in Section 3, then also removes the points present in a sphere with the center at the origin of the axes and a radius of 1 meter. This operation is necessary since the LiDAR also “observes” some lateral protrusions of the robot that fall within the sensor’s field of view. The post-processing involves the recognition of two flat vertical walls possibly present in the pcd frame under examination. The function used is also, in this case, the “pcfitplane”, providing in input a maximum distance between inliers and fitting plane equal to 0.5 meters [30]. Since, after some tests, it was noticed how this algorithm was not robust and could not capture the plans of interest, a more restrictive condition was inserted: the plan fitting process is stopped only if the mean square error of the inliers points of the model is less than a certain threshold. After this, the algorithm became more robust. In some cases, it is possible to insert a further condition that provides for the orthogonality between the two flat walls (the typical situation for indoor environments) to further strengthen the algorithm. To divide the points, a clustering technique aimed at homogeneous grouping objects starting from a set of data has been used. Clustering techniques are based on measures relating to the similarity between the elements. The discriminating variable for the grouping of the elements depends on the problem proposed; in the case of point cloud data, it is the distance between the points. In this work, the clustering has been carried out by using the “kmeans” function [15-17, 30]. The “kmeans” function takes as input the coordinates of the point cloud on which clustering will be performed and the desired number of partitions, while it outputs the indices of the points indicative of the assignment of points to one of
the partitions, the coordinates of the centroids, and the distance of all points from each centroid. In this case, fixing the number of partitions at 3, it is possible to identify the two flat models and the robot feature, and it is possible to calculate the intersection point between these two floors and the fitting ground plane calculated in the pre-processing phase. An example of a frame processing procedure obtained while the robot moved in an indoor environment is shown in Figure 7a and 7b (first and after the preliminary processing), and the consequent walls and landmark detection is shown in Figures 8 and 9. In Figure 8 and, for more clearness Figure 9, the results of the proposed algorithm for one frame acquired by the LiDAR sensor mounted on the robot: the 3 clusters identified through the clustering technique used are indicated with Cluster 1,2 and 3 and their points have been differentiated with different symbols in Fig.7. The centroids of the 3 clusters are indicated with a square; by checking the alignment of the individuated clusters, the walls points are identified and the planes 1 and 2 are estimated by fitting the points. Finally, the landmark is evaluated by considering the intersection of the estimated planes at the level z=0.
a) b)
This procedure may be generalised considering a trajectory traveled by the robot during which the succession of frames relating to different positions are acquired and processed with the technique shown. The detection of the reference point allows the reconstruction of the position of the robot concerning the two walls identified in the sequence of frames during the movement. Taking into account the spatial coordinates of the robot in the previous step, it is possible to calculate the displacement considering the fixed reference in the various subsequent frames. It is therefore possible to structure a while loop that builds the position matrix containing the displacements, in meters, of the robot, starting from the initial position at step k = 1, at coordinates [0,0,0]. The output of the algorithm, therefore, consists of a matrix n x 3, where n is the number of analyzed frames, which expresses the odometry of the robot concerning the identified landmark, the intersection of the identified walls. However, this work aims to propose a methodology for integrating the information on the position of the robot obtained from the LiDAR, with those obtained from the inertial data (IMU) from the sensor mounted on the robot.
Considering the possibility of having odometric data simultaneously from LiDAR and IMU, it was decided to integrate the information using a discrete Kalman filter, in which the information of one of the sensors is considered an estimate of the position of the robot, while the information of the other constitutes the updated feedback. The prediction equation for the kinematic model expressed in (4)-(6) is shown in (7), where P(k) is the error covariance matrix at step k, and Q is the process noise covariance matrix. The correction equations are shown in (8), where R is the measurement noise covariance matrix, and C derives from the state model (1).
resolution and long-term tests preliminary experiments found the presence measurement reason, it was keeping the robot from the start the pre-processing algorithm calculates recorded in the These averages from the data test. The results below is an example
and
(8)
(7)
For the evaluation of the system noise related to the inertial sensor and more generally for the calibration of the sensors, the results of various tests performed with the robot completely stopped are evaluated. From the trends of the measured accelerations, it can be seen that all channels are affected by ample noise. In particular, the channel relating to the y axis is the one with a higher standard deviation of the data and , therefore, the loudest. The covariance matrices of process noise Q and measurement noise R used for the tests shown in the next paragraph are reported in (9) and (10) and take into account the characteristics of the two sensors’ calibration data.
(9)
(10)
(9) (10)
(9) (10)
Given the high noise encountered during the calibration phase in the inertial measurement unit (IMU), by providing these covariance matrices as input, it was decided to give greater weight to the corrections made with LiDAR measurements. The matrices must therefore be adapted and chosen based on the characteristics of the sensors available. The output provided by the Kalman filter consists of the laying of the robot as a function of time starting from the inertial controls of the robot, corrected by the observations made by the LiDAR with a frequency of about 5 Hz.
(9) (7) (8)
(10)
resolution and higher cost, the algorithm is valid for long-term tests and trajectories of any kind. The preliminary experiments with the robot stopped also found the presence of a signal offset for all three measurement channels of the inertial sensor. For this reason, it was decided to carry out all the tests by keeping the robot in stasis for the first 10 seconds from the start of data recording. Subsequently, during the pre-processing of the inertial data, the used algorithm calculates the average of the values recorded in the first 10 seconds by all 3 channels. These averages constitute the offset to be subtracted from the data collected for the entire duration of the test. The results of the experimental tests are similar; below is an example for demonstrating the efficiency of the proposed innovative approach. The proposed results refer to a test carried out in the Kyutech courtyard (shown in Figure 6), in which the robot, manually guided by a joystick, after the first 10 seconds in which it was stationary in the initial position, moved with a straightforward movement for 10 seconds, covering about 4 meters, and then stopped in the final position. For this test, the accelerometric data acquired by the inertial sensor IMU in the 3 directions x, y, and z are shown in Figure 10 a; their elaborations, using numerical integration and elimination of the offset, provide the displacement data shown in Figure 10b.
lates the average of the values recorded in the first 10 seconds by all 3 channels. These averages constitute the offset to be subtracted from the data collected for the entire duration of the test. The results of the experimental tests are similar; below is an example for demonstrating the efficiency of the proposed innovative approach. The proposed results refer to a test carried out in the Kyutech courtyard (shown in Figure 6), in which the robot, manually guided by a joystick, after the first 10 seconds in which it was stationary in the initial position, moved with a straightforward movement for 10 seconds, covering about 4 meters, and then stopped in the final position. For this test, the accelerometric data acquired by the inertial sensor IMU in the 3 directions x, y, and z are shown in Figure 10 a; their elaborations, using numerical integration and elimination of the offset, provide the displacement data shown in Figure 10b.
It is evident that the poor quality of the inertial sensor makes the data excessively noisy, so the trajectory determined on the 3 axes differs considerably from the actual behavior, in which the robot is stationary for the first 10 seconds and then moves about 4 meters in the x-direction for the next 10, stopping definitively at the 20th second of the test.
resolution and higher cost, the algorithm is valid for long-term tests and trajectories of any kind. The preliminary experiments with the robot stopped also found the presence of a signal offset for all three measurement channels of the inertial sensor. For this reason, it was decided to carry out all the tests by keeping the robot in stasis for the first 10 seconds from the start of data recording. Subsequently, during the pre-processing of the inertial data, the used algorithm calculates the average of the values recorded in the first 10 seconds by all 3 channels. These averages constitute the offset to be subtracted from the data collected for the entire duration of the test. The results of the experimental tests are similar; below is an example for demonstrating the efficiency of the proposed innovative approach. The proposed results refer to a test carried out in the Kyutech courtyard (shown in Figure 6), in which the robot, manually guided by a joystick, after the first 10 seconds in which it was stationary in the initial position, moved with a straightforward movement for 10 seconds, covering about 4 meters, and then stopped in the final position. For this test, the accelerometric data acquired by the inertial sensor IMU in the 3 directions x, y, and z are shown in Figure 10 a; their elaborations, using numerical integration and elimination of the offset, provide the displacement data shown in Figure 10b.
resolution and higher cost, the algorithm is valid for long-term tests and trajectories of any kind. The preliminary experiments with the robot stopped also found the presence of a signal offset for all three measurement channels of the inertial sensor. For this reason, it was decided to carry out all the tests by keeping the robot in stasis for the first 10 seconds from the start of data recording. Subsequently, during the pre-processing of the inertial data, the used algorithm calculates the average of the values recorded in the first 10 seconds by all 3 channels. These averages constitute the offset to be subtracted from the data collected for the entire duration of the test. The results of the experimental tests are similar; below is an example for demonstrating the efficiency of the proposed innovative approach. The proposed results refer to a test carried out in the Kyutech courtyard (shown in Figure 6), in which the robot, manually guided by a joystick, after the first 10 seconds in which it was stationary in the initial position, moved with a straightforward movement for 10 seconds, covering about 4 meters, and then stopped in the final position. For this test, the accelerometric data acquired by the inertial sensor IMU in the 3 directions x, y, and z are shown in Figure 10 a; their elaborations, using numerical integration and elimination of the offset, provide the displacement data shown in Figure 10b.
It is evident that the poor quality of the inertial sensor makes the data excessively noisy, so the trajectory determined on the 3 axes differs considerably from the actual behavior, in which the robot is stationary for the first 10 seconds and then moves about 4 meters in the x-direction for the next 10, stopping definitively at the 20th second of the test.
The results obtained using the proposed method of assessing the position are much more interesting and accurate, identifying, through the data obtained from LiDAR, a landmark at the intersection of identified reference walls. The LiDAR in the experimental tests automatically acquires with a frequency of 5 Hz, and all the frames are processed as shown in section 4.2, to evaluate, through the fixed position of the landmark, the position of the robot during the test.
It is evident that the poor quality of the inertial sensor makes the data excessively noisy, so the trajectory determined on the 3 axes differs considerably from the actual behavior, in which the robot is stationary for the first 10 seconds and then moves about 4 meters in the x-direction for the next 10, stopping definitively at the 20th second of the test.
The tests performed are of medium duration. The robot, driven manually by a joystick, performs simple longitudinal movements with limited rotations. The simplicity of the tests was imposed by the quality and resolution of the inertial sensor, which was found to be very noisy. Even if using sensors with higher
Given the high noise encountered during the calibration phase in the inertial measurement unit (IMU), by providing these covariance matrices as input, it was decided to give greater weight to the corrections made with LiDAR measurements. The matrices must therefore be adapted and chosen based on the characteristics of the sensors available. The output provided by the Kalman filter consists of the laying of the robot as a function of time starting from the inertial controls of the robot, corrected by the observations made by the LiDAR with a frequency of about 5 Hz.
Given the high noise encountered during the calibration phase in the inertial measurement unit (IMU), by providing these covariance matrices as input, it was decided to give greater weight to the corrections made with LiDAR measurements. The matrices must therefore be adapted and chosen based on the characteristics of the sensors available. The output provided by the Kalman filter consists of the laying of the robot as a function of time starting from the inertial controls of the robot, corrected by the observations made by the LiDAR with a frequency of about 5 Hz.
Given the high noise encountered during the calibration phase in the inertial measurement unit (IMU), by providing these covariance matrices as input, it was decided to give greater weight to the corrections made with LiDAR measurements. The matrices must therefore be adapted and chosen based on the characteristics of the sensors available. The output provided by the Kalman filter consists of the laying of the robot as a function of time starting from the inertial controls of the robot, corrected by the observations made by the LiDAR with a frequency of about 5 Hz.
5. Results of experimental tests
5. Results of experimental tests
5. Results of experimental tests
The results obtained using the proposed method of assessing the position are much more interesting and accurate, identifying, through the data obtained from LiDAR, a landmark at the intersection of identified reference walls. The LiDAR in the experimental tests automatically acquires with a frequency of 5 Hz, and all the frames are processed as shown in section 4.2, to evaluate, through the fixed position of the landmark, the position of the robot during the test.
a) b) Fig. 10. a) accelerations of the IMU sensor during the Test b) displacements integrating the IMU data
In this application, the odometric data obtained from the LiDAR (shown in Fig. 11) are, for all 3 coordinates x, y, and z, much closer to the real values. For this reason, the choice of the position data obtained from the LiDAR as reference (corrective) data for the Kalman filter was considered by choice of the covariance matrices R and Q (9-10), giving greater weight to the measurements made with the LiDAR. The output provided by the Kalman filter, as introduced in this work, consists of the laying of the robot as a function of time starting from the inertial controls of the robot, corrected by the observations made by the LiDAR with a frequency of about 5 Hz. The x and y coordinates estimated from the proposed approach for the considered experimental test are shown in Figure 12a and 12b, demonstrating how the Kalman filter can clean the noisy and inaccurate behavior of the IMU data. In Figures 13 and 14, a 3-dimensional view of the estimated trajectory of the robot by using the proposed procedure is shown. a) b)
Other experimental tests, in addition to the test whose results are shown in Figures 10–14, have been carried out by successfully estimating the trajectory with the proposed procedure. All the tests were similar to the proposed one, all conducted on a flat surface (the courtyard floor shown earlier), with simple trajectories, also including rotations, which are easy to compare with the estimated ones. From the experimental tests carried out and the results obtained we can conclude that the proposed methodology has been experimentally tested on simple paths with positive results. It can also be foreseen that, by appropriately managing the number of clusters to be identified and using more accurate inertial sensors, it is also possible to detect the position relative to detected objects, both stationary and in motion, and even to establish their trajectory and their movement concerning the robot in exploration.
Today, odometric techniques are of great importance in the world of robotics and automation; for this reason, an innovative algorithm has been proposed that can estimate the pose of the robot during inspection operations. This algorithm exploits the potential of the Kalman filter by combining inertial data with those of the LiDAR. In this paper, a modularized driving-wheels robot that is able to balance the mobile robot’s efficiency according to the environment has been considered together with an efficient algorithm using LiDAR data for robot self-localization and detection of mobile targets.
The developed robot, which has been constructed by combining modules, may travel through several environments with saving energy by changing the number and arrangement of the wheels according to the environment.
Experimental tests were carried out with the robot to inspect the environments and receive the data necessary for the estimate of the odometry. From the calibration tests, it was possible to note how the IMU model installed on the robot was not suitable for estimating the installation as it was too noisy. Besides, the double integration of inertial data caused the error to grow beyond an acceptable threshold. For these reasons, in the use of the Kalman filter, greater weight was given to the filter correction phase using the LiDAR observations compared to the prediction phase, because the IMU model installed on the robot was not suitable for estimating the installation due to the noise. With this foresight, the proposed algorithm can accurately estimate the trajectory of the rover.
The particularity of the proposed approach is related to the simplicity of the procedure, that uses known tools, so it could be easily implemented, and uses a specific target (flat vertical fixed planes) for simplifying the trajectory estimation. The experimental results shown in this paper demonstrate that, in compatible scenarios, the proposed algorithm can accurately estimate the trajectory of the rover, using a Kalman filter that can compensate for the difference in accuracy between the LiDAR and the IMU data, evaluated by an initial calibration.
Besides, a specific algorithm involving the use of a clustering technique for automatically analyzing the LiDAR data has been presented and tested, demonstrating that, in specific structure scenarios, the robot can self-localize its position compared to fixed landmarks.
The authors thank the students Alessio Monaco and Kakeru Yamashita for their precious work related to this research.
Nicola Ivan Giannoccaro* – Department of Innovation Engineering, University of Salento, Lecce, 73100, Italy, ivan.giannoccaro@unisalento.it.
Takeshi Nishida – Department of Control Engineering, Kyushu Institute of Technology, Kitakyushu, Japan, nishida@cntl.kyutech.ac.jp
Aimè Lay-Ekuakille – Department of Innovation Engineering, University of Salento, Lecce, 73100, Italy, aime.lay.ekuakille@unisalento.it
Ramiro Velazquez – Universidad Panamericana, Aguascalientes, Ags, 20290, MEXICO, rvelazquez@ up.edu.mx.
Paolo Visconti – Department of Innovation Engineering, University of Salento, Lecce, 73100, Italy, paolo. visconti@unisalento.it
*Corresponding author
[1] T. Goelles, B. Schlager, S. Muckenhuber, “Fault Detection, Isolation, Identification and Recovery (FDIIR) Methods for automotive perceptions sensors including a detailed literature survey for lidar”, Sensors, vol. 20, 3662, 2020, pp. 1–21.
[2] T. Raj, F. Hanim Hashim, A. Baseri Huddin, M. Faisal Ibrahim, A. Hussain, “A survey on LiDAR scanning mechanisms”, Electronics, vol. 741, 9, 2020, pp. 1–25.
[3] C. Debuenne, D. Vivet, “A review of visual-Lidar fusion based simultaneous localisation and mapping”, Sensors, vol. 20, 2068, 2020, pp. 1–20.
[4] P. Forsman, A. Halme, “3-D mapping of natural environments with trees by means of mobile perception”, IEEE Transactions on Robotics, vol. 21, 2005, pp. 482–490. 10.1109/TRO.2004.838003
[5] T. Tsubouchi, A. Asano, T. Mochizuki, S. Kondou, K.Shiozawa, M. Matsumoto, S. Tomimura, S. Nakanishi, A. Mochizuki, Y. Chiba, K. Sasaki, T. Hayami, “Forest 3D Mapping and Tree Sizes Measurement for Forest Management Based on Sensing Technology for Mobile Robots,” Berlin, Heidelberg: Springer Berlin Heidelberg, 2014, pp. 357–368.
[6] J. Billingsley, A. Visala, M. Dunn, “Robotics in agriculture and forestry” Springer handbook of robotics; Springer, 2008, pp. 1065–1077.
[7] X. Liang, P. Litkey, J. Hyyppa, H. Kaartinen, M. Vastaranta, M. Holopainen, “Automatic Stem Mapping Using Single-Scan Terrestrial Laser Scanning”, IEEE Transactions on Geoscience and Remote Sensing, vol. 50, 2012, pp. 661–670. 10.1109/TGRS.2011.2161613
[8] M. A. Juman, Y. W. Wong, R. K. Rajkumar, L. J. Goh, “A novel tree trunk detection method for oil-palm plantation navigation”, Computers and Electronics in Agriculture, vol. 128, 2016, pp. 172–180. 10.1016/j.compag.2016.09.002
[9] S. Li, H. Feng, K. Chen, K. Chen, J. Lin, L. Chou, “Auto-maps generation through self path generation in ROS based Robot Navigation”, Journal of Applied Science and Engineering, vol. 21, no. 3, 2018, pp. 351–360.
[10] M. Ocando, N. Certad, S. Alvarado, A. Terrones, ”Autonomous 2D SLAM and 3D mapping of an
environment using a single 2D Lidar and ROS”, Proc. of 2017 Latin American Robotics Symposium, 2017.
[11] Y. Wang, C. Peng, A. Ravankar, A. Ravankar, “A single LIDAR-Based feature fusion indoor localisation algorithm”, Sensors, vol. 1294, 2018, pp. 1–19.
[12] A. Lay-Ekuakille, A. Trotta, “Binomial Filtering to Improve Backscattered Lidar Signal Content”, Proc. of XVII IMEKO World Congress, June 22–27, 2003, Dubrovnik, Croatia.
[13] N. I. Giannoccaro, T. Nishida, “The Design, Fabrication and Preliminary Testing of a Variable Configuration Mobile Robot”, International Journal of Robotics and Automation Technology, 2019, 6, pp. 47–54.
[14] N.I. Giannoccaro, T. Nishida, “Analysis of the surrounding environment using an innovative algorithm based on lidar data on a modular mobile robot”, Journal of Automation, Mobile Robotics and Intelligent Systems, vol. 14, no. 4, 2020.
[15] N.I. Giannoccaro, L. Spedicato, C. Di Castri, ”A new strategy for spatial reconstruction of orthogonal planes using a rotating array of ultrasonic sensor” IEEE Sensors, vol. 12, no. 5, 2012, pp. 1307–1316.
[16] N.I. Giannoccaro, L. Spedicato, “Exploratory data analysis for robot perception of room environments by means of an in-air sonar scanner”, Ultrasonics, vol. 53, no. 6, 2013, pp. 1163–1173.
[17] N.I. Giannoccaro, L. Spedicato, L. Aiello, “Kernel PCA and approximate pre-images to extract the closest ultrasonic arc from the scanning of indoor specular environments”, Measurement: Journal of the International Measurement Confederation, vol. 58, no. 1, pp. 2014, 46–60.
[18] W. Farag, “Real-Time Autonomous Vehicle Localization Based on Particle and Unscented Kalman Filters”, Journal of Control, Automation and Electrical Systems, vol. 32, no. 2, 2021, pp. 309–325.
[19] W.Xu, F. Zhang, “FAST-LIO: A fast, robust, LiDAR-inertial odometry package by tightly-coupled iterated Kalman-filter”, IEEE Robotics and Automation Letters, vol. 6, no. 2, 2021, pp. 3317–3324.
[20] P. Chauchat, A. Burrau, S. Bonnabel, “Factor Graph-Based Smoothing without Matrix Inversion for Highly Precise Localization”, IEEE Trans. On Control System Technology, vol. 29, no. 3, 2021, pp. 1219–1232.
[21] S. Muro, I. Yoshida, M. Hashimoto, T. Takahashi, “Moving-object detection and tracking by scanning LiDAR mounted on motorcycle based
on dynamic background subtraction”, Artificial Life and Robotics, Vol. 26, no. 4, 2021, pp. 412–422.
[22] H. Zhang, N. Chen, Z. Dai, G. Fan.” A Multi-level Data Fusion Localization Algorithm for SLAM”, Jiqiren/Robot, vol. 43, no. 6, 2021, pp. 641–652.
[23] J. Zhang, L. Xu, C. Bao, “An Adaptive Pose Fusion Method for Indoor Map Construction”, International Journal of Geo-Information, vol. 10, 2021, pp. 1–22.
[24] M. Morita, T. Nishida, Y. Arita M., Shige-eda, E. di Maria, R. Gallone, N.I. Giannoccaro, “Development of Robot for 3D Measurement of Forest Environment”, Journal of Robotics and Mechatronics, vol. 30, 2018, pp. 145–154. 10.20965/ jrm.2018.p0145.
[25] https://hokuyo-usa.com/application/files/7815/9111/2405/YVT-35LX-FK_Specifications.pdf
[26] https://www.invensense.com/products/motion-tracking/6-axis/mpu-6500/
[27] https://www.cytron.io/p-10amp-5v-30v-dc-motor-driver-2-channels.
[28] M. Quigley, K. Conley, B. Gerkey, J. Faust, T. Foote, J. Leibs, R. Wheeler, A. Y. Ng, “ROS: an open-source Robot Operating System”, Proc. of ICRA workshop on open source software, Kobe, Japan, vol. 3, no. 5, 2009.
[29] https://www.vizrt.com/en/products/viz-virtual-studio
[30] Matlab, “Computer Vision Toolbox”, The Mathworks, 2018.
Submitted: 10th October 2021; accepted: 8th February 2022
Pan-Long Wu, Jyun-Jhen Li, Jin-Siang ShawDOI: 10.14313/JAMRIS/1-2022/2
Abstract:
This paper presents the development of an automated guided vehicle with omni-wheels for autonomous navigation under a robot operating system framework. Specifically, a laser rangefinder-constructed two-dimensional environment map is integrated with a three-dimensional point cloud map to achieve real-time robot positioning, using the oriented features from accelerated segment testing and a rotated binary robust independent elementary feature detector-simultaneous localization and mapping algorithm. In the path planning for autonomous navigation of the omnidirectional mobile robot, we applied the A* global path search algorithm, which uses a heuristic function to estimate the robot position difference and searches for the best direction. Moreover, we employed the time-elastic-band method for local path planning, which merges the time interval of two locations to realize time optimization for dynamic obstacle avoidance. The experimental results verified the effectiveness of the applied algorithms for the omni-wheeled mobile robot. Furthermore, the results showed a superior performance over the adaptive Monte Carlo localization for robot localization and dynamic window approach for local path planning.
Keywords: ROS, ORB-SLAM, Omnidirectional AGV, Autonomous Navigation
With the advancement of technology, the production process has gradually developed automation, which has improved the production efficiency and quality of products. Consequently, robots have become an indispensable part of industrial automation. Robot manipulators and mobile platforms are key elements in robot automation. To enable a mobile robot to navigate autonomously, it is first necessary for the robot to construct an environment map and locate itself in the map. For this, the simultaneous localization and mapping (SLAM) technique is applied, which can be divided into three-dimensional (3D) visual SLAM (VSLAM) and two-dimensional (2D) laser SLAM, based on the sensor types. Specifically, 3D SLAM techniques, such as oriented features from accelerated segment test and rotated binary robust independent elementary
feature detector-SLAM (ORB-SLAM), use visual scanning to match 3D point clouds [1, 2]. Gmapping, which was proposed by Grisetti et al. [3], is commonly adopted in 2D SLAM techniques. Path planning in robot navigation also needs to specify a goal on the map such that the robot will move forward along the specified path and simultaneously avoid obstacles. Currently, the most widely used algorithm for global path planning is the A* algorithm, which was proposed by Bostel and Sagar [4]. The dynamic window approach (DWA) or timed-elastic-band (TEB) method is employed as a local path planning algorithm for dynamic obstacle avoidance [5, 6]. Autonomous navigation for a mobile robot can be made possible only after the aforementioned processes have been completed.
Mobile robots may have different types of traction. The most common types are differential traction, tricycles, and omnidirectional. An omnidirectional mobile robot can rotate 360° and is capable of X-axis transverse motion, thereby offering a higher degree of freedom than a differential wheel robot [7, 8]. Although there is abundant research on the autonomous navigation of mobile robots with differential wheels using SLAM techniques under a robot operating system (ROS) framework [9, 10], studies on autonomous navigation for omnidirectional mobile robots are relatively rare [11]. In this study, we investigated autonomous SLAM navigation for an omni-wheeled mobile robot under an ROS framework. In particular, 3D ORB-SLAM for robot precision positioning, along with A* and TEB algorithms for path planning, was emphasised for better performance. To the best of the authors’ knowledge, this is the first application of this kind for an omnidirectional mobile robot in the literature. The experimental results were evaluated with those obtained by the well-known 2D localization methods of adaptive Monte Carlo localization (AMCL) [12] and encoder odometers [13].
To navigate an automated guided vehicle (AGV) smoothly to a desired location, an ROS navigation stack was employed. We set a target point within the map first. Then, a costmap was generated through a static map with inflation layer constructed around the boundary and obstacles. By applying a localization algorithm to locate the robot in the map, the goal could be reached with global path planning, while avoiding obstacles through local path planning.
The AGV used in this research is a three-wheeled omnidirectional robot, and the kinematic model [14, 15] and constructed robot are shown in Figure 1.
Equation 4 represents the kinematics of the AGV in its coordinate system. The AGV center point velocity AGV[,,] mm VVxyp w is the “cmd-vel” output from the move_base package, a path-planning package in ROS framework [16]. However, the actual speeds of the three omni-directional wheels detected by the attached encoders may deviate from the target speeds
V1, V2, and V3 in Equation 4 during the navigation task; therefore, a proportional-integral-derivative (PID) controller [17] is applied to each wheel’s motor to compensate for the error, thereby ensuring a precision AGV motion control. A corresponding AGV speed control node graph in ROS structure is configured to implement such a closed-loop motor control.
Several open-source SLAM algorithms exist in the ROS framework. Various 2D SLAM methods were compared, and the Gmapping algorithm was proven the best in constructing a corridor environment [18]. Moreover, the localization algorithm often used the AMCL and encoder odometer.
The center position and yaw angle of the AGV can be expressed as qw = (x,y,θ) in world coordinates. In encoder odometer localization, the omni-wheel speeds V1, V2, and V3 are detected first by
=×× 1,2,3 1001 2 VR PPRt π (5)
The angle between the three wheels of the AGV is 120°, forming an equilateral triangle; thus, the distances from the center of the robot to the three wheels are equal. V1, V2, and V3 are the linear speeds of the left, right, and rear wheels, respectively; L is the distance from the center of the vehicle to the center of the wheel; and φ (= 60°) is the angle between wheel 1 (wheel 2) and the X-axis of the robot coordinate system. Assume that the linear speed of the AGV at any moment is Vxm in the X-direction and V y m in the Y-direction (the heading direction), and wp is the angular speed of the AGV center point relative to the robot frame. The kinematics equations for each wheel can be derived as follows:
=−−+ 1 cossinmm VVVLxyp ϕϕw (1)
=−++ 2 cossinmm VVVLxyp ϕϕw (2)
=+ 3 m VVLxp w (3)
Subsequently, they can be written in a vector-matrix form as follows:
where PPR (= 500 in this study) is the number of pulses per revolution of the attached encoder, t is the time passed for every 100 pulses, and R is the radius of the wheel. After the actual wheel speeds are obtained from Equation 4, the X-axis speed, Y-axis speed, and angular speed of the vehicle relative to the robot frame can be computed as follows:
Then by integrating Equation 6 for a unit time, we obtain
where Ddx is the displacement in the X-axis, Ddy is the displacement in the Y-axis, and Dθx is the angular displacement per unit time of the vehicle in robot frame. Subsequently, the X-axis and Y-axis displacements of the AGV per unit time in world coordinate can be calculated as follows:
Finally, the robot center position and yaw angle are constantly updated as follows:
=+D=+D=+D xxxyyy θθθ (9)
The AMCL localization algorithm distributes particles uniformly on the map and simulates the movement of the robot through the particles. Assuming that the robot moves forward a unit distance, the particles also move the same way. The position of the particles in the environment was used to simulate the sensor information and compare it with the sensor information from the one placed on the AGV. Each particle is thus given a probability based on the comparison, and the algorithm redistributes the particles according to this probability. A particle with a higher probability will have more particles around it. In this iterative process, all the particles tend to converge to a location, and the position of the robot is identified accordingly.
In this experiment, visual SLAM used the ORB-SLAM algorithm, where a red-green-blue-depth camera was utilized to obtain abundant environmental information. The localization of ORB-SLAM is to use visual odometry to track unmapped areas and to match their feature points. The system sees robust to severe motion clutter, allows wide baseline loop closing and relocalization, and is capable of automatic map initialization. There are three main aspects to ORB-SLAM that were executed simultaneously: tracking, local mapping, and loop closing. After confirming the loop closing, the system enters the global bundle adjustment (BA) optimization to form a 3+1 parallel threading. In the tracking part, localization of the camera is completed according to the ORB features of the image, and the time needed for inserting a keyframe is determined as well. Local mapping is responsible for processing new keyframes, using local BA to the camera position, and then filtering the inserted keyframes and removing redundant keyframes. Loop closing is performed by searching for loops with every new keyframe to confirm whether or not a closed loop is formed, computing a similarity transformation that provides information about the drift errors accumulated in the loop and merging nearby duplicate points. Finally, the global BA optimizes the camera position and map point simultaneously to achieve the best results.
The ROS node graph of ORB-SLAM is shown in Figure 2. The /kinect2 node is responsible for describing the coordinate relationship between the base link of the AGV and the Kinect-v2 camera. Both the color image information (/image_colour_rect) and image depth information (/image_depth_rect) with quad high definition pixels are used as the two messages into the ORB-SLAM algorithm, from which the point cloud information of the map (/map_points), positioning information of the AGV (/pose), and result of the image processing (/image_topics) are calculated. Finally, the AGV position is inputted into the odometer to calculate the relative displacement between the
map and AGV, and the current coordinates of the map and AGV are updated accordingly.
Under the ROS 3D visualization tool (RVIZ), this experiment used the point cloud map constructed by ORB-SLAM and static map constructed by Gmapping, such that the two maps are overlapped through coordinate transformation, as shown in Figure 3. Specifically, the same origin was set for the two maps and their boundaries were made to overlap, such that the difference between 2D and 3D SLAM could be intuitively compared. The actual scene captured by the Kinect-v2 camera was shown in the lower left corner of Figure 3. The green points were the ORB features after image processing, and the blue points were obtained by visual odometry which were to match the ORB features to locate the AGV within the map in the localization mode.
The move_base in ROS is a package for path planning, which includes a global planner, local planner, global costmap, local costmap, and recovery behaviour node. The global costmap establishes a path plan in the entire area and generates an inflation layer on the boundary of the static map. In the local costmap, there is an inflation layer that is generated around obstacles near the AGV, limiting the accessible part of the entire area. When a kidnapped robot problem occurs, it triggers recovery behaviours: rereading the sensor messages to update the map, deleting the invalid obstacle layers, and planning the route again.
The two algorithms most used in the move_base package for global path planning are Dijkstra and A* [19], which are extremely similar. The only difference between the two is that A* attempts to find a better path using a heuristic function, while Dijkstra’s explores all possible paths. Therefore, we chose A* for the global path planning for efficiency concern. During navigation, if obstacles that did not exist at the time of mapping appear on the map, they cause a collision problem between the AGV and obstacles, which is solved by the local path planning. The DWA and TEB are the most commonly used methods in such cases. The DWA simulates the trajectory of the AGV, according to the sensors and odometry information, and scores the paths at each speed. The TEB optimizes the execution time of the A* trajectory, minimises the deviations of speed, acceleration, obstacle avoidance, etc., and finally optimizes the best local path. According to Rösmann et al. [20], it is known that the DWA slows down before obstacles to avoid collisions; however, the TEB method, having previously predicted the collisions, chooses an alternative route earlier. Therefore, TEB is the better choice for this study.
The experiments were conducted in part of the basement corridor of a campus building, which is a semiopen environment. A SICK LiDAR was employed to construct the corresponding 2D map using Gmapping SLAM algorithm, as shown in Figure 4 where the garden area is open to the sky. We designed two routes, and , for the experiment. We assume that the start point of the AGV is point O in front of our laboratory, and a waypoint is point A for avoiding the automatic path planning finding the shortest path through the garden; further, points B and C are the first and second target points, respectively.
scanning point from the RVIZ did not fit well the actual wall boundaries. Several reasons were accountable for this positioning error: this experiment relied only on the encoder and inertial measurement unit for the sensing information, and the error in computing travel distance (Equation 9) by integrating AGV velocity (Equation 7) became more significant as the travel distance increased. Furthermore, it was susceptible to interference from external factors such as excessive wheel friction due to AGV weight, slipping or motor idling due to the floor material used, and floor flatness. In summary, for this experiment, the mean absolute error (MAE) of positioning error from the goal was 57 cm, and MAE of the angle deviation from the target direction was approximately 5.4°.
The first experimental route was , which had a length of 25 m. In this route, the encoder odometer, AMCL, and ORB-SLAM were separately used to test the positioning accuracy 15 times. One of the navigation results of the encoder odometer is shown in Figure 5, where the green line was the LiDAR scanning points and the red line was the local costmap in the move_ base. The left figure showed clearly that the laser
In the AMCL experiment, sometimes the AGV could not obtain the current valid LiDAR sensor information because of the lack of apparent features on both sides of the corridor; therefore, it was possible to lose the location information. To address this, one can place mirrors on both sides of the corridor to increase the features to solve the problem. However, particle divergence did not occur very often in this experiment. For example, Figure 6 illustrated one of the successful experimental results: the AMCL particles converged in front of the AGV, and the positioning information during navigation was relatively accurate and stable. The right figure showed a matched laser scanning line with the wall in the X-axis of the word coordinate. The final MAE of positioning error was 34 cm, 40% less than the encoder odometer method, and the MAE of the angle deviation was 5.8°.
In ORB-SLAM localization, several key points were found for successful navigation: stable lighting condition; reduced angular speed about Z-axis, especially during the corner turning midway between Points O and A; and reduced weighting of the X-axis movement (transverse direction) in path planning. These
key points ensured accurate feature points extraction and matching without too much frame rate loss due to unnecessary rapid lens rotation. One of the experimental results was depicted in Figure 7, where the green scanning line overlapped with the wall closely. The final MAE of positioning error was 13 cm, 77% and 62% less than the respective encoder odometer method and AMCL, and MAE of the angle deviation was 6°.
call it was 34 cm and 5.8° in Path 1). Comparing results of Path 1 with those of Path 2 showed that the AMCL robot navigating to the elevator still was mainly affected by the long corridor, and the rich number of feature points from point B to point C (15 m long) contributed not much to the MAE. Consequently, the AMCL was verified again to be unsuitable for the long corridor environment. For ORB-SLAM navigation to the elevator, it was relatively more accurate than AMCL, as clearly shown in Figure 9 and Table 1; the MAE of positioning error was 17.7 cm (34.8 cm for AMCL), and MAE of the angle deviation was 7.3° (8.6 for AMCL). Note also that the advantage of ORBSLAM over AMCL in positioning error in Path 2 (49% less) was worse than that in Path 1 (62% less) due to another corner turning near Point B. Indeed, it was observed that recovery behaviour for robot re-localization occurred multiple times nearby Point B for corner turning for ORB-SLAM, leading to this reduction in the advantage of ORB-SLAM over AMCL.
The experimental route is , which has a length of 40 m. Note that Point C is located 2 m in front of an elevator, resulting in Path 2 simulating a robot navigation from the laboratory to an elevator. Based on the experiment results in Path 1, we knew that the deviation from the goal using the encoder odometer increased with distance and was the worst compared to the other two localization algorithms. Therefore, we applied AMCL and ORB-SLAM to navigate the robot 30 times for each method on this path. One of the experimental results is shown in Figure 8, where both methods successfully dispatched the robot to an elevator 40 m away and gave closely matched laser scanning lines with the front wall.
The test route was repeated 30 times for each localization method; the deviation error from the goal for each run in the X-Y plane is depicted in Figure 9, and the MAE of positioning error was summarized in Table 1. The MAE of positioning error for AMCL was 34.8 cm, and MAE of the angle deviation was 8.6° (re-
In this paper, a static map established with a 2D LiDAR sensor was integrated with a point cloud map constructed with an RGBD camera to increase robot localization accuracy. Moreover, the A* algorithm in
global path planning and TEB method in local path planning for dynamic obstacle avoidance were employed in a three-wheeled omnidirectional AGV for autonomous navigation. Experimental results showed that the 3D ORB-SLAM localization outperformed the 2D AMCL for 49% MAE reduction of positioning error in a test route from the laboratory to an elevator 40 m away.
To highlight the effectiveness of the TEB method over DWA in local path planning and maneuvering of the omni-wheel drive over differential-wheel drive, the authors also applied DWA with ORB-SLAM to a differential wheel robot for the same test route (OC) [21]. Unfortunately, the robot seldom succeeded in reaching the goal (Point C) right in front of the elevator, mainly due to localization divergence, especially near the two corner turnings. In summary, an omnidirectional mobile robot with TEB and ORB-SLAM can navigate autonomously and more precisely to an elevator 40 m distance away than other cases studied in this paper [21].
This study was supported by the National Taipei University of Technology – Nanjing University of Science and Technology Joint Research Program (NTUTNUST-108-01)
Pan-Long Wu – Nanjing University of Science and Technology, China, e-mail: plwu@njust.edu.cn.
Jyun-Jhen Li – National Taipei University of Technology, Taiwan, e-mail: dory110220@gmail.com.
Jinsiang Shaw* – National Taipei University of Technology, Taiwan, e-mail: jshaw@ntut.edu.tw
*Corresponding author
[1] R. Mur-Artal, J.M.M. Montiel and J.D. Tardós, “ORB-SLAM: A versatile and accurate monocular SLAM system”, IEEE Trans Robot, vol. 31, no. 5, 2015, pp. 1147–1163.
[2] R. Mur-Artal and J.D. Tardos, “ORB-SLAM2: An open-source SLAM system for monocular, stereo and RGB-D cameras”, IEEE Trans Robot, vol. 33, no. 5, 2017, pp. 1255–1262.
[3] G. Grisetti, C. Stachniss and W. Burgard, “Improved techniques for grid mapping with Rao-Blackwellized particle filters”, IEEE Trans Robot, vol. 23, no. 1, 2007, pp. 34–46.
[4] A.J. Bostel and V.K. Sagar, “Dynamic control systems for AGVs”, Comput Control Eng J, vol. 7, no. 4, 1996, pp. 169–176.
[5] D. Fox, W. Burgard and S. Thrun, “The dynamic window approach to collision avoidance”, IEEE Robot Autom Mag, vol. 4, no. 1, 1997, pp. 23–33.
[6] C. Rösmann, W. Feiten, T. Wösch, et al., “Efficient trajectory optimization using a sparse model”, European Conference on Mobile Robots, Barcelona, Spain, 25–27 September 2013, pp. 138-143.
[7] K.V. Ignatiev, M.M. Kopichev and A.V. Putov, “Autonomous omni-wheeled mobile robots”, 2nd International Conference on Industrial Engineering, Applications and Manufacturing, Chelyabinsk, Russia, 19–20 May 2016, pp. 1-4.
[8] S.A. Magalhães, A.P. Moreira and P. Costa, “Omnidirectional robot modeling and simulation”, IEEE International Conference on Autonomous Robot Systems and Competitions, Ponta Delgada, Portugal, 15–16 April 2020, pp. 251-256.
[9] J. Xin, X.L. Jiao, Y. Yang, et al., “Visual navigation for mobile robot with Kinect camera in dynamic environment”, 35th Chinese Control Conference, Chengdu, China, 27–29 July 2016, pp. 47574764.
[10] Z. Meng, C. Wang, Z. Han, et al., “Research on SLAM navigation of wheeled mobile robot based on ROS”, 5th International Conference on Automation, Control and Robotics Engineering, Dalian, China, 19–20 September 2020, pp. 110-116.
[11] Y. Feng, C. Ding, X. Li, et al., “Integrating Mecanum wheeled omni-directional mobile robots in ROS”, IEEE International Conference on Robotics and Biomimetics, Qingdao, China, 3–7 December 2016, pp. 643-648.
[12] B.A. Berg, Markov chain Monte Carlo simulations and their statistical analysis. Hackensack, New Jersey: World Scientific, 2004.
[13] R. Akkaya and F.A. Kazan, “A new method for angular speed measurement with absolute encoder”, Elektron Elektrotech, vol. 26, no. 1, 2020, pp. 18–22.
[14] Figure source: Open-Base. https://github.com/ GuiRitter/OpenBase
[15] J. Goncalves, J. Lima and P. Costa, “Real time tracking of an omnidirectional robot – An extended Kalman filter approach”, 5th International Conference on Informatics in Control, Automation and Robotics, Funchal, Portugal, 11–15 May 2008, pp. 5–10.
[16] Package source: move_base. http://wiki.ros. org/move_base
[17] J.G. Ziegler and N.B. Nichols, “Optimum settings for automatic controllers”, J Dyn Sys Meas Control, vol. 115, no. 2B, 1993, pp. 220–222.
[18] E.B. Olson, “Real-time correlative scan matching”, IEEE International Conference on Robotics and Automation, Kobe, Japan, 12–17 May 2009, pp. 4387-4393.
[19] M. Seder and I. Petrovic, “Dynamic window based approach to mobile robot motion control in the presence of moving obstacles”, IEEE International Conference on Robotics and Automation, Rome, Italy, 10–14 April 2007, pp. 1986-1991.
[20] C. Rösmann, F. Hoffmann and T. Bertram, “Planning of multiple robot trajectories in distinctive topologies”, European Conference on Mobile Robots, Lincoln, UK, 2–4 September 2015, pp. 1-6.
[21] P.L. Wu, Z.M. Zhang, C.J. Liew, et al., “Hybrid navigation of an autonomous mobile robot to depress an elevator button”, Journal of Automation, Mobile Robotics and Intelligent Systems, accepted, 2022.
Submitted: 22nd September 2021; accepted 8th February 2022
Tomasz Krakówka, Andrzej Typiak, Maciej CaderDOI: 10.14313/JAMRIS/1-2022/3
Abstract:
This paper presents a method of selection of configuration for manipulators of portable robots for special purposes. An analysis of tasks and related requirements for the functionality of the manipulator was presented on the example of the portable PIAP Patrol robot. From the set of robot tasks, the tasks that had the greatest impact on the manipulator parameters were selected. Both kinematic and static criteria were used as the basis for adopting the objective function. With the use of multi-criteria optimization tools, the manipulator configuration parameters were selected. Selected working capacities were maximized while ensuring that the imposed requirements for mass and kinematic limitations were met. The results of simulation tests were presented, and the scope of further work has been outlined.
Keywords: Field robotics, EOD robots, manipulators
1. Introduction
Among mobile robots dedicated to special applications, one can distinguish those that are man-packable, weighing up to 15 kg, and 2-man portable robots weighing up to 75 kg. [1] [2]. The users of portable robots indicate the mass and load to mass ratio as important features of the robot [3][4]. Striving to reduce the mass of the robot’s components must not adversely affect the operating capabilities and parameters. This article presents an analysis of the literature on the selection of manipulator parameters and presents a new approach to the selection of the robot manipulator configuration, in which the main criterion was the analysis of tasks performed by robots for special tasks. On the example of the portable PIAP Patrol robot, the analysis of tasks and related requirements for the functionality of the manipulator has been presented.
In the process of selecting the manipulator parameters, a model was used consisting of joints represented by point masses and connectors with a specific mass of an arm section of a unit length. The parameters of the joints were selected, indicating the distances between the axes – the lengths of the links and the sizes of the executive modules in the joints.
From the set of robot tasks, the characteristic tasks that have the greatest impact on the manipulator pa-
rameters were selected. The selection was made using both kinematic and static criteria.
This was the basis for adopting three objective functions: maximization of horizontal reach, maximization of lifting capacity at maximum reach, and maximization of load capacity at a certain minimum distance from the body of the mobile platform.
With the use of multi-criteria optimization tools, we carried out the selection of the manipulator configuration parameters, which leads to the maximization of selected working capacities while ensuring that the imposed requirements and mass and kinematic limitations are met. The results of simulation tests were presented and the scope of further work was outlined.
The process of selecting parameters for manipulators has been presented in many papers. Z. Du, Y. Xiao, and W. Dong carried out a multi-criteria optimization of the geometric parameters of the manipulator and gear parameters to minimize the mass-to-load ratio and to maximize the natural frequency of the structure [5]. The authors used the NSGA-II algorithm and checked the results in the ADAMS system. Thanks to optimization, they achieved a 10% mass reduction. H. Yin, S. Huang, M. He, and J. Li proposed a method of designing a manipulator in which the optimization of parameters for design and the selection of manipulator drives was performed [6]. The manipulator mass was minimized while maintaining the constraints resulting from the assumed robot dynamics. Parametric optimization was performed in the Ansys program and a manipulator model simulated in the ADAMS system was used for the selection of drives.
In another paper, C. Lanni, S. F. P. Saramago, and M. Ceccarelli formulated the task of manipulator design as a problem of optimization, in which the objective functions are minimization of the size of the manipulator and maximization of workspace volume [7]. The numerical solution of the optimization problem was solved by using two different numerical techniques: sequential quadratic programming and simulated annealing.
H. Lim, S. Hwang, K. Shin, C. Han and presented the procedure and the results of the multi-objective optimization for designing a seven-degree-of-freedom (7DOF) robot manipulator with higher global performance [8]. Global performance was defined
by the global conditioning index and the structural length index, which represents the ratio of the total link length of the robot manipulator to the volume of the reachable workspaces. Analysis of variance (ANOVA) was completed, both to analyze the effect of the link parameters on the performance of the robot manipulator, and to find which link parameter affects the performance of the robot manipulator. The results of the optimization of the prototype robot manipulator were presented.
Wang X. et al. optimized the serial manipulator by integrating topology optimization and parametric system optimization [9]. Scientists at first constructed the stiffness model of the manipulator, then determined typical load configurations and loads. On the part level, they performed topology optimization. On the system level, they performed parametric optimization, which was used to determine the mass division into different components.
Xu Q. et al optimized the link lengths of an anthropomorphic manipulator [10]. The authors defined a global comprehensive performance index considering manipulability, the Jacobian matrix condition number, and end stiffness of the manipulator. The index was used to measure performance during optimization.
Lim et al. optimized 7 DOF manipulators’ parameters using Genetic Algorithm and Modified Dynamic Conditioning Index as an objective function [11].
The conducted analysis of the literature shows that although the topic of selecting the parameters of manipulators was undertaken by many authors, the problem of selecting the configuration of manipulators in the context of mobile robotics for special tasks was not discussed in the available literature.
The specificity of mobile robots for special applications such as intelligence, surveillance, and reconnaissance; combat support; mine clearance; detection and neutralization of explosives; counteracting CBRN threats; transport; search and rescue; and firefighting requires an individual approach to their design, taking into consideration desired mobility and working capacity. According to technical report. [4], the robots carrying out the above-mentioned tasks should be capable of:
• detection of thin lines, antennas, cables, and wires, metals masked with ground, and explosives;
• observation of objects, both low-lying (e.g., under a car) as well as objects located high above the robot;
• picking up or removing objects with a manipulator;
• revealing objects in the ground;
• excavating items;
• checking passenger cars and trucks (chassis, interior, trunk);
• checking culverts and bridges;
• neutralization of IEDs by alternative methods. Each of these tasks is associated with specific requirements for both the platform and its equipment. The manipulator is the basic equipment of the robot, which is often jointed with the platform. In terms of
the manipulator’s working abilities, the following tasks should be considered:
• picking up and carrying objects, opening the door with a gripper;
• exposing, digging, and burying in the ground with the help of additional equipment;
• moving and dragging objects on the ground using a gripper or an arm;
• cutting wires, wires, rods, punching tires, breaking windows with the help of additional equipment mounted in the gripper;
• carrying out neutralization with a shotgun or pyrotechnic disruptor mounted on the manipulator (various mounting configurations);
• X-raying objects with the use of additional equipment mounted in the gripper;
• freezing objects with liquid nitrogen, using a lance fixed in the gripper;
• sampling and measurements with CBRN sensors mounted on the gripper or arm;
• placing charges with a gripper;
• conducting reconnaissance and observation of sensors installed on the manipulator.
The robots work in an unstructured environment, with limited situational awareness of the operators. As a result, the structure of the manipulator must also be resistant to such events as hitting obstacles, lifting the platform to the working position in the case of a rollover, or balancing and supporting the mobile platform while overcoming obstacles.
Backpack-class robots must be light enough for soldiers to carry them for extended periods, or, in the case of a portable class, to be carried by 2 people. This results in the requirements for the mass and dimensions of the robot in the transport position.
The basis for the reduction of the structure mass is the identification of loads to which the structure will be subject, as well as the definition of the critical load states that will be taken into account during the calculations. During operation, the manipulator of a mobile robot for special tasks is subject to complex loads:
• quasi-static loads resulting from lifting and shifting objects with a gripper or a working tool, such as lifting the maximum load to be lifted with the grapple from the ground, lifting the maximum load from ground level for the maximum reach of the arm, moving objects along the ground with the grapple by rotating the platform in place, loading of the manipulator through the thrust on, or pulling a stationary object with the robot’s drive system;
• loads from digging and burying in the ground;
• dynamic loads resulting from the movement of the mobile platform over obstacles, curbs, stairs, etc. It can also be a drop of a robot with a manipulator from a given height;
• vibrations resulting from the tread or segments of the track system of the mobile platform;
• dynamic loads from pyrotechnic ejectors or shotguns mounted on the manipulator’s arm;
• dynamic loads resulting from robot operator errors.
Some loads can be determined analytically, and some require measurements or simulations. Recoil tests during shots with a rifle or pyrotechnic disruptor have been carried out by many scientists and the results have been presented in several papers [1214]. The results of these experiments will be used at a later stage to model the stroke while firing a shotgun attached to the robot manipulator arm.
It is necessary to define what combinations of the above-mentioned loads are possible, and, on this basis, to select key load states. It should also be considered that preparing the structure for some hypothetically possible loads may be difficult and costly. An example of such a load may be a collision with the full speed of movement of the platform, a fully deployed side-facing robot manipulator, e.g., with a tree. Securing the structure for such an eventuality may be expensive and complicated. It may be necessary to assume that such a threat would be eliminated by appropriate training and, for example, a function of reduction of the robot’s speed in the configuration with an unfolded arm, implemented in software. Risk analysis would allow for the development of a structure tailored to the requirements which would not be oversized.
The aim of the work carried out at Łukasiewicz PIAP was to develop a method for selecting the parameters of the manipulator structure with the assumed maximum lifting capacity and range, ensuring that the mass limit and other requirements and limitations resulting from the tasks of mobile robots would be met. This task has been decomposed into the following subtasks:
1. Selection of parameters of drive modules and link lengths.
In the sub-task, the parameters of the drive modules and the arm lengths will be selected to obtain the most advantageous combination of parameters, considering the expected requirements.
2. Development of a family of reduced-mass drive modules
The structure of drive modules with a minimum mass for the loads resulting from the tasks should be developed for the components in the subtask. One of the possible methods is topological optimization.
3. Selection of actuator components with the best load-to-mass ratio.
This subtask should analyze the parameters of the components that can be used. The key is the selection of the last gear stage in the manipulator drive. The most commonly used gears with perpendicular axes are worm gears (e.g., MedEng, ECA, Łukasiewicz-PIAP) or spiroid gears (Teledyne Flir) with a high ratio. The design of the drive modules and the entire manipulator will depend on the type of gear.
At each stage of the above-mentioned subtasks, the requirements and limitations typical for robots
for special tasks are important. They affect working loads, working space, the need for electromagnetic shielding, environmental sealing, etc. As part of the article, the authors will focus on the selection of the manipulator parameters listed as the first subtask.
The PIAP Patrol robot can be used as an example of a portable robot. This robot is equipped with a manipulator (Fig. 1) with five rotational degrees of freedom. The first axis is vertically oriented, the second, third and fourth are parallel and horizontally directed, the fifth axis is responsible for the rotation of the gripper about its axis. The structure of the manipulator’s arms includes joints with actuator mechanisms and links, the length of which can be easily modified.
In the process of selecting the parameters of the manipulator, a model with a structure similar to that of the PIAP Patrol robot was used, consisting of joints represented by point masses and connectors with a specific mass of an arm section of a unit length (Fig. 2).
The parameters of the joints were selected, indicating the distances between the axes – the links lengths and the sizes of the actuator modules in the joints. For such a task, the manipulator configuration is described by the vector:
Man_config = [J1,J2,J3,J4,L1,L2,L3,L4] where:
J1, J2, J3, J4 – ID numbers of the actuator modules used in the manipulator’s joints
L1, L2, L3, L4 – distances between the joints
Actuator module sizes J1, J2, J3, and J4 are selected from the family of sets. This approach is justified, because when designing a transmission, it is often
not possible to choose the components freely, but the constructor must instead select components from the family of parts available from manufacturers. Such a limitation often results from the necessity to unify subassemblies and limit the assortment of various ordered and stored parts.
In the Man_config vector, the joint size is represented by the ID number of the selected actuator module for each joint. The joint is defined as a vector Joint = [J_ID,J_T,J_m] where:
J_ID – ID number of actuator module, J_T – designated torque of actuator, J_m – a mass of the actuator module
For the construction of the gearbox, the use of commercial components sets was assumed, and 13 sets were selected with parameters listed in Table 1.
From this set of tasks, the characteristic tasks that have the greatest impact on the parameters of the manipulator were selected. The criteria have been divided into kinematic and static. Kinematic criteria are responsible for ensuring the possibility of adopting the appropriate configuration for the planned tasks and they mainly affect the lengths of the links. Static criteria are responsible for ensuring that loads can be picked up in selected positions.
The following kinematic criteria were considered:
a) Maximizing the reach when reaching under the car (Fig. 4a).
b) Maximum horizontal reach (Fig. 4b).
c) Minimizing the dimensions of the robot in its folded position (Fig. 4c).
d) Maximize reach when reaching down below the ground level (Fig. 4d).
e) Maximum vertical reach for picking up a load (Fig. 4e).
The maximum torque values presented in Table 1 result from the torque limitation with the overload clutch. The mass of the joint presented in Table 1 was estimated based on the analysis of the existing examples of joints of the manipulators of mobile robots. Apart from the transmission components such as the worm and the worm wheel, the mass of the joints includes the body, shafts, bearings, seals, overload friction clutch, clutch, the motor with planetary gear, position sensors, and controllers. Figure 3 shows the points from which the relationship was determined, which approximates the mass of the joints depending on the maximum moment. The linear form of the regression function has been adopted.
When in tight spaces, it is advantageous when the gripper is short, so the length was fixed and excluded from the optimization process. The length was assumed based on the analysis of the existing structure. It was assumed that due to the necessity to maintain minimum dimensions during transport (Figure 4c), the height of the first manipulator member should be the minimum possible and was determined by the analysis of the existing structure. This ensures minimization of the folded robot’s height.
When working in tight spaces, it is advantageous when the gripper is short, so the length was fixed and excluded from the optimization process. The length was assumed based on the analysis of the existing structure. It was assumed that due to the necessity
(a) under-car inspection
(b) maximum horizontal reach
(c) transport
(e) maximum vertical reach
to maintain minimum dimensions during transport (Figure 4c), the first manipulator member should be the minimum height possible, which was determined by the analysis of the existing structure. This ensures minimization of the height of the folded robot.
As the waist and gripper lengths have been fixed, the arm and shoulder length of the manipulator have been further optimized.
This allowed us to reduce the criteria a) and e). Maximizations of the horizontal reach and maximization of vertical reach requirements coincided, because both meant that the longer the sum of L2 and L3 is, the better. These criteria were reduced to one criterion: the maximization of the horizontal range.
To be able to perform a visual inspection of the car chassis (requirement a), the arm-length condition has been added, to provide the possibility of lowering the shoulder joint above the ground. Case d) of the criteria was no longer considered because it coincided with cases b) and a).
The possibility of rotating the axis waist (the first–vertical axis) of the manipulator and the fifth axis responsible for the rotation of the gripper around its axis was not taken into account. In this case, the task of selecting parameters could be treated as two-dimensional.
Static criteria following objective functions were considered:
• maximization of lifting capacity at maximum reach;
• maximization of the load capacity when picking up the load at a certain minimum distance from the body of the mobile platform (Fig. 5).
Objective functions that are responsible for maximizing the robot’s lifting capacity in selected tasks were constructed from the conditions of ensuring the possibility of transferring the load for individual joints and the condition of maintaining the stability of the robot’s mobile platform. For example, for the fixed remaining parameters of the manipulator, Figures 6 and 7 show the lifting forces achieved by the manipulator at maximum reach depending on the length of the arm and shoulder, and the maximum lifting forces depending on the length of the arm and shoulder of the manipulator.
Observing the nature of the graphs, it can be noted that for different arm lengths, the maximum lifting force is limited by various restrictions. In Figure 7, it can be seen that for some configurations, the lifting force was zero. This is how the configurations were assessed in the case when the manipulator was too weak
to lift the object or with a given combination of parameters the manipulator could not reach the object. For the mentioned objective functions, a multi-criteria optimization of the parameters describing the configuration of the manipulator stored in the Man_ config vector was carried out, assuming additional constraints:
• the manipulator mass cannot exceed the assumed limit of 15 kg;
• the first joint actuator of the manipulator (with the vertical axis) must carry the load that results from moving along a ramp with a lateral inclination of 30 degrees with the manipulator fully extended, with the maximum load that can be transferred by the remaining degrees of freedom;
• the lengths of the links must allow for the configuration of the load to be lifted at a predetermined distance from the front of the mobile platform to a certain height. (The position of the robot’s manipulator during this task is shown in Fig. 5.);
• the lengths of the links allow taking a position that allows to inspect and pick up the load from under the car (Fig. 4a);
• the lengths of the links allow for inspection and taking the load through the passenger car window.
The multi-criteria optimization algorithm NSGA-II presented by K. Deb [15] as implemented in Matlab function gamultiopt [16] was used to select the manipulator parameters. The algorithm of the function is explained in Figure 9.
For the population size 2000, the algorithm after 206 generations achieved the calculation end criterion, which was to obtain the difference in the mean distance of the points belonging to the Pareto front below the assumed tolerance. The result obtained is a point cloud in 3-dimensional space that is difficult to visualize on paper. Figure 10 shows three different views of the same values of the fitness function for the final generation of the algorithm’s operation.
It can be seen that the results are divided into groups of points distributed over the surfaces, which is particularly visible in Figure 10b. Figures 11a and 11b show groups of fitness functions values in where the torques transmitted by the arm of the manipulator are highlighted in color.
Particular groups of solutions are located on surfaces that group solutions according to the selected gear of the elbow and shoulder.
In Figure 11, it can be seen that in the final generation of results of the manipulator configurations, there are 3 sizes of joints in the elbow and 4 sizes in the shoulder.
Selected configurations and values of the fitness functions for solutions from the Pareto front are shown in Table 2.
Figure 11b shows layers of points representing fitness functions values by the maximum torque capacity of the shoulder of the manipulator, which is coded by color. It can be noticed that there is a group of results that have constant lifting capacity at maximum reach. In this group of results, lifting capacity was limited by gripper torque.
It can be noticed that the best assessments of the fitness function of lifting capacity at the maximum reach are in the configurations in which the largest gears are in the shoulder joint, and the subsequent degrees of freedom are smaller and smaller.
For the fitness function responsible for maximizing the manipulator’s lifting capacity, the best scores were given to configurations in which the gears driving the arm were as large or larger than the gears driving the shoulder. In the positions that the manipulator had to take when lifting heavier loads from the ground, the upper arm joint was the most loaded. It can also be seen that the masses of the manipulators in the proposed resulting configurations used almost the entire mass limit.
This adopted simple model allows for the initial assumption of design parameters for the manipulator that meets the functional requirements and mass limitations. The adopted method of selecting parameters allowed for the maximum use of the available mass limit to improve the functionality of the manipulator. One of the solutions that can be considered is, for example, a manipulator ID 10 shown in Table 2. It allows to lift up to 14 kg at a maximum extension of 2 m from the vertical axis of the manipulator and can lift a maximum of 40 kg at a distance of 0.5 m from the front edge of the platform. When, for similar reach, maximum capacity is more important than the load capacity at the maximum reach, solution ID 11 should be considered. It allows for lifting a maximum mass of 56kg at the expense of decreased lifting capability at maximum reach to 11 kg. To be able to draw correct conclusions from the analysis, it is important to correctly estimate the masses and torques transmitted by the joints and arms. Further work will focus on refining the model and taking into account other loads specified in the work, such as loads resulting from the use of additional robot accessories. It is also planned to take into account the influence of the selection of parameters on the vibrations of the manipulator resulting from the operation of the robot’s drive system.
Tomasz Krakówka* – Department of Mobile Systems, Łukasiewicz PIAP, Warsaw, Poland, e-mail: tomasz.krakowka@piap.lukasiewicz.gov.pl.
Andrzej Typiak – Faculty of Mechanical Engineering, Military University of Technology, Warsaw, Poland, e-mail: andrzej.typiak@wat.edu.pl.
Maciej Cader – Deputy Director of Research, Łukasiewicz PIAP, Warsaw, Poland, e-mail: maciej.cader@ piap.lukasiewicz.gov.pl.
*Corresponding author
[1] M. Hinton, M. Zeher, M. Kozlowski, and M. Johannes, “Advanced explosive ordnance disposal robotic system (AEODRS): A common architecture revolution”, Johns Hopkins APL technical digest vol. 30, pp. 256–266, 2011.
[2] “Unmanned Ground Vehicle (UGV) Interoperability Profile (IOP)”, Robotic Systems, Joint Project Office, 2011.
[3] C. Lundberg, H. I. Christensen, and R. Reinhold, “Long-term study of a portable field robot in urban terrain”, J. Field Robot., vol. 24, no. 8–9, 2007, pp. 625–650. doi: 10.1002/rob.20214
[4] “Studium wykonalności projektu Programu Strategicznego na rzecz bezpieczeństwa and obronności państwa pt.: “Rodzina bezzałogowych platform lądowych (BPL) do zastosowań w systemach bezpieczeństwa and obronności państwa”. Wojskowa Akademia Techniczna, Warszawa, 2012.
[5] Z. Du, Y. Xiao, and W. Dong, “Method for optimizing manipulator’s geometrical parameters and selecting reducers”, J. Cent. South Univ., vol. 20, no. 5, 2013, pp. 1235–1244. doi: 10.1007/ s11771-013-1607-7
[6] H. Yin, S. Huang, M. He, and J. Li, “A unified design for lightweight robotic arms based on unified description of structure and drive trains”, Int. J. Adv. Robot. Syst., vol. 14, no. 4, 2017. doi: 10.1177/1729881417716383
[7] C. Lanni, S. F. P. Saramago, and M. Ceccarelli, “Optimal design of 3R manipulators by using classical techniques and simulated annealin”, J Braz. Soc. Mech. Sci., vol. 24, no. 4, 2002, pp. 293–301. doi: 10.1590/S0100-73862002000400007
[8] H. Lim, S. Hwang, K. Shin, C. Han, “Design Optimization of the Robot Manipulator Based on Global Performance Indices Using the Grey-based Taguchi Method”, IFAC Proc. Vol., vol. 43, no. 18, 2010, pp. 285–292. doi: 10.3182/20100913-3US-2015.00078
[9] X. Wang, D. Zhang, C. Zhao, P. Zhang, Y. Zhang, and Y. Cai, “Optimal design of lightweight serial robots by integrating topology optimization and parametric system optimization”, Mechanism and Machine Theory, vol. 132, 2019, pp. 48–65. doi: 10.1016/j.mechmachtheory.2018.10.015
[10] Q. Xu, Q. Zhan, and X. Tian, “Link Lengths Optimization Based on Multiple Performance Indexes of Anthropomorphic Manipulators”, IEEE Access, vol. 9, 2021 pp. 20089–20099. doi: 10.1109/ACCESS.2021.3054834
[11] S. Hwang, H. Kim, Y. Choi, K. Shin, and C. Han, “Design optimization method for 7 DOF robot manipulator using performance indices”, Int. J. Precis. Eng. Manuf., vol. 18, no. 3, 2017, pp. 293–299. doi: 10.1007/s12541-017-0037-0
[12] B. Canfield-Hershkowitz, T. Foster, and W. Meijer, “Rifle and Shotgun Recoil Test System”, 2013.
[13] B. A. Parate, S. Chandel, and H. Shekhar, “Estimation of Recoil Energy of Water-Jet Disruptor”, Probl. Mechatroniki Uzbroj. Lot. Inż. Bezpieczeństwa, vol. 11, no. 2, 2020, doi: 10.5604/01.3001.0014.1991
[14] M. Ceh and T. Josey, “Recoil Measurement of Improvised Explosive Device. Disruptors Lightweight AB Precision Ltd. – Pigstick and Hotrod”. DRDC – Suffield Research Centre, 2016. https://cradpdf.drdc-rddc.gc.ca/PDFS/unc262/ p805077_A1b.pdf
[15] K. Deb, S. Agrawal, A. Pratap, and T. Meyarivan, “A Fast Elitist Non-dominated Sorting Genetic Algorithm for Multi-objective Optimization: NSGA-II”, Parallel Problem Solving from Nature PPSN VI, Berlin, Heidelberg, pp. 849–858, 2000. doi: 10.1007/3-540-45356-3_83
[16] “Matlab documentation”, MathWorks, 2020, 1994.
Submitted:23rd November2021;accepted:8th February2022
DOI:10.14313/JAMRIS/1‐2022/4
Abstract:
Pathplanningplaysavitalroleinamobilerobotnavi‐gationsystem.Itessentiallygeneratestheshortesttra‐versablepathbetweentwogivenpoints.Therearemany pathplanningalgorithmsthathavebeenproposedbyre‐searchersallovertheworld;however,thereisverylittle workfocussingonpathplanningforaserviceenviron‐ment.Thegeneralassumptionisthateithertheenviron‐mentisfullyknownorunknown.Bothcaseswouldnot besuitableforaserviceenvironment.Afullyknownen‐vironmentwillrestrictfurtherexpansionintermsofthe numberofnavigationpointsandanunknownenviron‐mentwouldgiveaninefficientpath.Unlikeotherenvi‐ronments,serviceenvironmentshavecertainfactorsto beconsidered,likeuser‐friendliness,repeatability,sca‐lability,andportability,whichareveryessentialfora servicerobot.Inthispaper,asimple,efficient,robust, andenvironment‐independentpathplanningalgorithm foranindoormobileservicerobotispresented.Initially, therobotistrainedtonavigatetoallthepossibledesti‐nationssequentiallywithaminimaluserinterface,which willensurethattherobotknowspartialpathsintheen‐vironment.Withthetraineddata,thepathplanningal‐gorithmmapsallthelogicalpathsbetweenallthedes‐tinations,whichhelpsinautonomousnavigation.Theal‐gorithmisimplementedandtestedusinga2Dsimulator Player/Stage.Theproposedsystemistestedwithtwodif‐ferentserviceenvironmentlayoutsandprovedtohave featureslikescalability,trainability,accuracy,andrepe‐atability.Thealgorithmiscomparedwithvariousclassi‐calpathplanningalgorithmsandtheresultsshowthat theproposedpathplanningalgorithmisonparwiththe otheralgorithmsintermsofaccuracyandefficientpath generation.
Keywords: LearningfromDemonstration,pathmapping, pathplanning,navigationsystem,mobilerobot,service robot
Mobileservicerobotshavebeengettingpopular inthelastdecadeintheconsumerelectronics ield. Theyareusedinserviceenvironmentslikehomes,of‑ ices,hospitals,hotels,museums,etc.Themajorrea‑ sonforthispopularityisthattheyareoperatedau‑ tonomouslyandhelpuserswithday‑to‑daytasks.An inherentpartofanykind(wheeled,legged,airborne, etc.)ofamobileservicerobotisthenavigationsy‑ stem.Anavigationsystemisakeyelementinma‑ kingaservicerobotautonomous.Ithelpsthero‑
bottonavigatefromagivensourcetoadestination autonomously.Anavigationsystemiscomposedof subsystemslikelocalization,whichlocatestherobot intheenvironment,pathplanning,usedtoplanthe pathbetweentwopoints,obstacleavoidance,tona‑ vigateinadynamicenvironment,etc.Overall,auto‑ nomousservicerobotscanbeclassi iedintotwoty‑ pes:environment‑dependent,andenvironmentinde‑ pendent.Environment‑dependentrobotsuseonboard sensors(sensorsintherobot’schassis)andoff‑board sensors(sensorsplacedintheenvironment)fornavi‑ gation.Environmentindependentrobotsuseonlyon‑ boardsensorsfornavigationandthusenvironment portabilityispossible.
Theserviceenvironmentdemandsthatthero‑ bottraverseapathrepeatedly,increasingthenum‑ beroflocationsdrasticallyandthatevenanoviceuser shouldbeabletohandletherobotandmovefromone serviceenvironmenttoanotherwithoutanyexpert help.Inthiswork,apath‑planningarchitecturefora wheeledmobilerobotdeployedinaserviceenviron‑ mentwithalltheaforementionedkeyfactorsispro‑ posed.Theproposedalgorithmisgenericinnature, whichcaterstotheneedsofvariousserviceenviron‑ ments.Serviceenvironmentstargetedarethosewith largecarpetedarea,devoidofanykindofenvironment augmentation.Overallworkisdividedintotwopha‑ ses:theLearningfromDemonstration(LfD)phaseand thepathplanningphase.IntheLfDphase,therobotis taughtallthepossibledestinationssequentiallywith aminimaluserinterface,whichgivesapartialunder‑ standingofthepathsavailable.Inthepathplanning phase,acompletepathtreeisgeneratedbymapping allthepossiblelogicalpathsamongpossibledestinati‑ onsandnavigatingintheshortestpathpossibleauto‑ nomously.Theselogicalpathsaretotallybasedonthe learntpathsintheLfDphase.
Theremainderofthearticleisorganizedasfol‑ lows.Section2highlightsthestateofartintheLfD andpath‑planning ield.TheproposedLfDandpath‑ planningalgorithmaredescribedinsection3.Section 4presentstheresultandanalysis,andacomparison withvariousclassicalapproaches.Section5concludes thework.
ResearchonLfDinroboticsstartedintheearly 1970’sandhasgrownsigni icantlyduringthepastde‑ cade[19].ThemainaimforLfDistotrainarobot toperformataskratherthanprogrammingit.Main advantagesareuser‑friendlinessandenvironmental
portability.Toprogramarobot,anexpertisrequired, whereastoteacharobot,anoviceuserwillbeableto doit.Therobotcanbeportedtoanyenvironmentwith anoverheadofjusttrainingforthenewenvironment; thiswouldreducebothtimeandcost.Initially,thero‑ botismanuallytrainedbyahumantoperformatask. Trainingcanbedoneusingvarioustoolsliketeaching pendant[3]camera‑basedlearning,orimitationlear‑ ning[14].MostoftheinitialworkinroboticsLfDisfor industrialmanipulators[13,25].Forthepastdecade, LfDhasbeenusedinmobilerobotstoperformspeci ic navigationtasksliketraversingacorridororpassing adoorway[32,40].
Therobotlearnsbystoringtheon‑boardandoff‑ boardsensors’data,anditisusedtoperformthele‑ arnttaskautonomously.Therehavebeenalotofrese‑ archadvancementsonprocessingthelearntdataand usingitef icientlyinautonomousmode.In[8],anonli‑ nearregressionmodeltoidentifytherelationshipbet‑ weenonboardsensorsandactuatorswhiletrainingis proposed.Asetoflibrarytasksistaught,andtheseli‑ brarytasksareusedintheautonomousphasein[13]. Inarticle,[6],feedbackintermsoftheadviceoperator isgiventotherobotbytheuserbasedontasklearned. Thisallowsthetrainertocorrecttherobotinrealtime. Whileinalltheaforementionedliterature,thetrainer andtherobotareinthesameplace,in[36],aremote trainingalgorithmusingtheWebisproposed.Atour guiderobotin[2]istrainedtoallpossiblepathsbyfol‑ lowingatrainerandstorestheposesasrouteparame‑ ters.In[17],adifferentapproachoftrainingarobotin astaticenvironmentandexecutioninadynamicenvi‑ ronmentisproposed.
TheLfD ieldhasdevelopedrapidlysinceitsincep‑ tion,butthereisnoonegeneraltechniquethatcan beusedindifferentdomainsofrobotics.Mostappro‑ achesaretestedusingonlyasingledomainwithone orafewtasksonasingleroboticplatform.Thisisthe primaryreasonforthelackofcomparisonsbetween algorithms.MostoftheworkdoneinLfDisonindus‑ trialmanipulators.Itisnotpossibletousethesame techniqueinmobilerobots,asthelearningparame‑ tersandteachingapproachdifferforbothplatforms. ExistingLfDsystemsheavilydependuponof board sensorslikethecamera,wearablesensorsfortrainer, etc.,whichisnotsuitableforaserviceenvironment.
Pathplanningisanactiveresearchareawitha lotofscopeforadvancements.Earlierpathplanning algorithmshavebeenusedinthe ieldsofcompu‑ ternetworks,circuitboarddesign,etc.Latelythey havebeenextensivelyusedinthe ieldofmobilero‑ botics.Pathplanningisidentifyingtheshortestpath betweenagivensourceanddestination.Overallpath planningtechniquescanbecategorizedbasedonthe environmentalawarenessoftherobot,withknown, unknown,andpartiallyknownenvironments[31].
Themainprerequisiteforknown‑environmentpath planningisthattheenvironmentmaphastobepre‑ loaded,nodynamicchangesintheenvironmentare possible,andthatitwillplantheshortestpathavai‑ lable.Inunknown‑environmentpathplanning,thero‑
botperceivestheenvironmentusingsensorsanddeci‑ desupontheshortestpath,andthiscanworkindyna‑ micenvironments.Partiallyknownenvironmentpath planningisacombinationofboththeaforementioned types;thisworksinadynamicenvironment,andatthe sametimetheshortestpossiblepathisidenti ied.
Classicalheuristicsearchpathplanningalgo‑ rithmslikeDijkstra’s[11]andA*[21]arebasedon pathplanningwithknownenvironments.A*isbased onDijkstra’salgorithm,withamorefocussedsearch towardsoptimalstates.Thesealgorithmscaniden‑ tifyanoptimalpathbetweenthestartandendpoint. Whilethesealgorithmsworkwellinastaticknown environment,theyfallshortindynamicunknownen‑ vironmentswherethepathisplannedbasedonthe onboardsensorsinrealtime.Algorithmslikethemo‑ torschema‑basedapproach[4,23],potential ieldmet‑ hod[24],improvedpotential ieldmethod[27],arti i‑ cialpotential ieldmethod[43],modi ied lexiblevec‑ tor ieldmethod[22]areusedinsomeoftheearlier literatureforunknownenvironments.Techniqueslike D*[41]andFieldD*[15]belongtothiscategory.The D*algorithmisbasedontheA*algorithmbutwith dynamicreplanningcapability.Algorithmslikeconju‑ gategradientdescent,improvedpotential ield,orD* Lite[12,46,47]useknownpathplanningalgorithms likeA*deliberativeplanningtechniqueandusere‑ planninglocallyifrequired.
Sometechniquescan’tbecategorizedintooneof theaforementionedclasses.Visibilitybinarytree[39] isonesuchtechniquethatcanbeusedinknownor unknownenvironments.Thismethodcreatesabinary treeoffreespaceandobstaclestoplanthepathto‑ wardsthegoal.Someotherpopulartechniquesare coverage‑basedpathplanning[16]andrapidlyexplo‑ ringrandomtrees(RRT)[29].RRTgeneratespaths withlocalconstraintsusingprobability.Anotherwork [18]usesprobabilityforitsnavigationfunction,along withGaussianprobabilitydistributionforlocationsof obstaclesandtherobotintheenvironment.In[42] theproblemofconvergencetimeinacluttereden‑ vironmentbybi‑directionalRRT*andintelligentbi‑ directionalRRT*isdiscussed.Theauthorsproposea potentiallyguidedbidirectionaltree,whichimprove theconvergencerate.Apredictivealgorithmtoavoid dynamicandstaticobstaclesusingtherangesensors intherobotisdiscussedin[26].Thepredictivealgo‑ rithmspredictthevelocityvectorsofthedynamicob‑ staclesandplanacollision‑freepathtowardsthegoal. Article[7]discussesanonparametricmotioncontrol‑ lerusingGaussianprocessregressionfortrajectory prediction.Predictedtrajectories,alongwiththero‑ bot’slimitedperception,areusedforrobotmotion control.In[45],aSLAMalgorithmbasedonparticle il‑ teroptimizationisdiscussed.Imageprocessing‑based navigationtechniquesarealsoexploredextensively, andin[44],adynamiclandmarkidenti icationsystem usingthevisualservomethodisproposed.
Withthelatestadvancementsinsoftcomputing techniques,extensiveresearchonapplyingittorobo‑ ticpathplanningisbeingcarriedout.In[20],path
planninginapartiallyknownenvironmentispresen‑ ted.Initially,anof linepathplannerwillplanthepaths withinthegivenenvironment.Duringactualexecu‑ tion,theonlinepathplannerusesreactivepathplan‑ ningforunknownobstacles.Afuzzyinferencesy‑ stemisusedfortrajectorytracking.Adaptiveparti‑ cleswarmoptimizationtechniquesareusedforpath planningin[9].Objectivefunctionsarebasedonthe distancefromrobottogoalandfromrobottoobsta‑ cle.Article[38]usesthe ire lyalgorithmformobile robotnavigationinunknowndynamicenvironments. Hybridsoftcomputingapproacheshavebeengetting moretractionlately.[37]usesanadaptiveneuro‑fuzzy inferencesystemcontrollertogettherobotsteer‑ ingangledynamicallybasedontherobot’sforward obstaclesintheenvironment.Pathplanningusinga geneticalgorithmandadaptivefuzzy‑logiccontrolis proposedin[5].Ageneticalgorithmisusedtoge‑ neratethecollision‑freeinitialpath.Apiecewisecu‑ bictermiteinterpolatingpolynomialisusedtosmooth thegeneratedoptimalpath.Later,anadaptivefuzzy‑ logiccontrollerisusedtomaintaintherobotinthe desiredpath.In[1]afuzzylogiccontrollerisused fornavigationinanunknownenvironment.Laterthe controllerisoptimizedusinggeneticalgorithms,neu‑ ralnetworks,andparticleswarmoptimization.All theseoptimizationtechniqueswithamanuallycon‑ structedfuzzylogiccontrollerarecompared.Asur‑ veypaper[30]discusstheef iciencyofpathplanning techniquesusingfuzzy,neuralnetworks,geneticalgo‑ rithms,nature‑inspiredalgorithms,andhybridalgo‑ rithms,andconcludesthathybridalgorithmsarebet‑ ter.Acloud‑basedmapstorageapproachisdiscussed in[28].Inthispaper,theentireenvironment’sinfor‑ mationisstoredinthecloud.Thisishelpfuliftherobot workareaconsistsofmultiplebuildings/ loors.The robotcanaccesstheclouddatabyscanningspeci ic environmentaltagslikeARTagsandQRcodes.
ThisworkaimsatdevelopingageneralizedLfD techniquetotrainamobileservicerobottonavigate tocertainlocationsinanindoorserviceenvironment. Majorfeaturesofthealgorithmareitsportable,gene‑ ric,anduser‑friendlynature.Portabilitycanbeachie‑ vedbytrainingonlywiththeonboardsensorsavaila‑ bleontherobotandnotaugmentingtheenvironment. Thismakesthesystemindependentoftheenviron‑ ment.Thesystemwillbegenericifitcanbeusedin anymobilerobotplatforminanyindoorserviceen‑ vironmentwithminimalmodi ications.Asimple,in‑ tuitiveuserinterfacewithoutanywearabledevicefor trainingmakesituser‑friendlysothatevenanovice usercantraintherobot.
Theoverallworkisdividedintotwostages:le‑ arningfromdemonstrationstageandpath‑planning stage.IntheLfDstage,therobotistrainedtona‑ vigatetoallpossibledestinationssequentially.Trai‑ ningisstartedfromtherobot’shomepositiontodes‑ tination1,thendestination1todestination2,and soon.”Home”istherobot’sstartingpositioninthe
autonomousexecutionstage.TheproposedLfDalgo‑ rithmistheEnhancedEncoderBasedLfDalgorithm (EEBL),whichisbasedonauthor’spreviousworkon theEncoderBasedLfDalgorithm(EBL)[33–35].Some ofthekeyfeaturesofEEBLthatarenotinEBLin‑ cludetrainingtoallthedestinationssequentially,dy‑ namicorientationandpositionerrorcorrectionme‑ chanism,andidenti icationofreferencepointsinthe environment.EEBLhasatrainingphaseandautono‑ mousphase;duringthetrainingphasepathsarelear‑ nedandpathvariablesarestoredasapathmatrix.As destinationsaresequentiallytaught,notallthepossi‑ bleroutesarelearned.Withthelearnedpathsasseed paths,theproposedTreeBasedPlanner(TBP)maps, thepossiblelogicalpathsandatreeisformedwiththe homepositionastherootnodeandallthedestinations aschildnodes.TBPalgorithmgeneratesVirtualLand‑ Mark(VLM)basedonthetaughtpaths,whichwillact aswaypointfornavigation.VLMsaretheintermedi‑ atenodesinthetree.Givenasourceanddestination, TBPusesthetreetoplantheshortestroutetonavi‑ gateautonomously.Fig. 1 showsthe lowdiagramof theproposedsystem.
Ahexagon‑shapeddifferentialdrivemobilerobot, asshowninFig. 2,isusedfortesting.Therobot hasthreeonboardsensors:wheelencoders,proximity sensors,andposesensors.Fourproximitysensorsare placedstrategically,coveringtherobotwithoutany blindspots.
Thisalgorithmusesonboardsensorstolearn pathstaughtbythetrainer.Thetrainerusesasim‑ pleinterfacetomovetherobotfront,left,right,and back.Wheelencoderdataisusedtolearnthelinear andangulardistanceforapathandisstoredinama‑ trixcalledthepathmatrix.Whentherobotisnavi‑ gatingautonomouslyjustwiththedistanceinforma‑ tion,itispronetohavingerrorslikepositionerror
andorientationerror.Inordertoovercometheafo‑ rementionederrors,proximitysensorsandposesen‑ sorsareused.Proximitysensorsareusedtoidentify thereferencepointswhilelearningapath.Reference pointsare ixedpointsintheenvironmentlikewalls, corridors,etc.,andtheproximitytothemisusedto adjusttherobot’spositionduringautonomousnavi‑ gation.Whenareferencepointisidenti ied,proximity valuesarestoredinamatrixcalledthereferencema‑ trix.Posesensorsgivetheorientationangle θ withre‑ ferencetoY‑axis,asshowninFig.2oftherobot.While training,theposeoftherobotisupdatedinamatrix calledtheorientationmatrixaftereveryforwardmo‑ vement.Boththereferencematrixandorientationma‑ trixareusedtocorrectthepositionandorientationer‑ rors,respectively,duringautonomousnavigation.
TheEEBLalgorithmisdividedintotwophases:the trainingphaseandautonomousphase.Inthetraining phase,therobotistrainedtonavigateapath,usingan interfacetodirectittowardsthegoalpoint.Training isinitiatedfromthehomepositiontothe irstdestina‑ tionandcontinuedsequentiallyuntilthepathtothe lastdestinationistaught.Twopathvariablesarele‑ arnedduringthisphase:headingdirectionandlinear orangulardistance.Thesetwovariablesarestoredin apathmatrixasonwhentherobotisstoppedorhea‑ dingdirectionischangedduringtraining.Everyrowin thepathmatrixisthepathvariablesofasinglepath. Eventhoughthetrainingiscarriedoutsequentially, thepathmatrixisbuiltbasedonthehomepositionas asource.Thisisachievedbytakingapartialpathfrom thepreviousrowinthematrixasperuserinputand appendingthenewpathvariableslearnedforanew destinationtoit.Thisistomaintainuniformityand easeinbuildingapathtreewiththehomepositionas therootnode.
vement,backwardmovement,leftturn,andrightturn respectively. L/θ isthelineardistanceencodervalue iftheheadingdirectionisfront/backorangulardis‑ tanceencodervalue.‘D’and‘L/θ’arecalledapose pair,whichwilldeterminetheposeoftherobot’sstate. Here,‘n’isthetotalnumberofstates,therobotwill transitthrough,beforegettingtothecorresponding destination.Ifapathhasfewerposepairsthan‘n’,then zeroswillbepaddedtotheremainingposepairsto keepthematrixnotskewed.Allthepathsfromhome todifferentdestinationsarerowsoftheformedpath matrix.Anexamplepathwithitsarrayisdepictedin Fig.3with9posepairs.
Equation 1 isapatharrayforasinglepathwith pathvariablesDandL/ θ.Distheheadingdirection, whichisencodedas1,2,3and4forforwardmo‑
Thepathmatrixformedinthetrainingphaseis usedtonavigateautonomously.Unfortunately,this dataisnotenoughforarobottoreachadestination accuratelyandrepeatedly.Mobilerobotsareproneto errorsduetosensorerrors,motorspeedmismatch andwheelmisalignment,whichleadtoloweraccuracy andrepeatability.Twoerrorsareidenti ied:position errorandorientationerror.Ifpositionororientation changesinthesourceoralongthepath,thedestina‑ tionpointwillvaryrelativetotheerrorinpositionand orientation.Fig.4andFig.5depictascenariowhere therobot’sstartingposition/orientationischanged; theeffectisthesameifanerroroccursalongthepath. Asthepathdistanceincreases,theerroralsoincreases relatively.
PositionError Positionerroroccursifthereisadis‑ placementintherobot’sposition.Itiscorrectedby identifyingthereferencepointsintheenvironment. Referencepointsare ixedpointsintheenvironment likewallsandcorridors.Thesepointsareidenti ied usingtheonboardproximitysensoroftherobot.Inthe trainingphase,whentherobotismovingforward,it scansforreferencepointsusingitsleftandrightproxi‑ mitysensors.Alocationintheenvironmentisselected asareferencepointiftheproximityvalue(foreither leftorrightsensor)ismorethanthegiventhreshold valueandisconstantforagivensamplingdistance. Whenareferencepointisidenti ied,proximityvalues
(fortheleftandrightsensor)anditsdistancefromthe sourcelocationarestoredinamatrixcalledthere‑ ferencematrix.Similartothepathmatrix,eachrow ofthereferencematrixisforapath,andtherecanbe multiplereferencepointsinapath.Thereferencema‑ trixisupdatedinsyncwiththepathmatrix.Thepath matrixisupdatedtore lectareferencepointassoon asitisidenti ied.Equation2isareferencearrayfora pathwiththereferencepoint’m’.
where,
LSV=LeftSensorValue
RSV=RightSensorValue
OrientationError Theorientationofarobotcan change,mainlyduetohardwaremalfunctionslike wheelmisalignmentandwheelencodererror.This causesover‑turningorunder‑turningoftherobot, whichwillcauserelativechangeinthedestination coordinates.Itcanbecorrectedusingtheorientation informationoftherobot,whichisobtainedusinga posesensor.Theposesensorgivestheorientationan‑ gle θ withreferencetoY‑axis,asshowninFig. 2 of therobot.Inthetrainingphase,orientationinforma‑ tionisupdatedasposeangle α inamatrixcalledthe orientationmatrixatthebeginningofeachforward movement.Thismatrixisupdatedalongwiththepath matrixandreferencematrix.Equation 3 showsthe orientationarrayforasinglepath.
ErrorCorrection Letusconsidertheexamplepath showninFig.3,butwithwallsalongthepathasshown inFig. 6.Thegrayblocksarewallsandthereddots alongthewallsarethepointsidenti iedasreference pointsbythealgorithm.Inorderto indthereference pointtwofactorshavetobe ixed:proximitythreshold andsamplingdistance.Letusconsidertheproximity thresholdtobe0.5units(unitcanbeanystandardunit oflength)andsamplingdistanceas1unit.Assumethe gridsinthe igureare0.5x0.5unit.Basedonthese
twoparameters,thealgorithmhasidenti iedthreere‑ ferencepoints(R1,R2,andR3)alongtheforwardmo‑ vementpathoftherobot.Assoonasthereference pointisidenti ied,thepathmatrixisupdatedwiththe forwarddistancevalueuntilthatpointandthecorre‑ spondingsensorvalueisstoredinthereferencema‑ trix.Ifonlyonesensorvalueissatisfyingthecondition, thenanothersensorvalueisstoredasNil.Orientation arrayisupdatedwithposeangles α1, α2, α3, α4 and α5 atthebeginningoftheforwardmovementsL1,L3,L5, L7andL9,respectively.
Intheautonomousphase,aPIcontrolsystemis usedtoauto‑correcttherobot’spositionandorienta‑ tionerror.Theidenti iedreferencepointproximityva‑ luesandposeanglesareusedasthereferencevalues forpositionandorientationcorrection,respectively. Thecontrolequationisgiveninequation4.Kpvalueis assumedtobe1andKiisassumedtobe0.0001,which isobtainedbythetrialanderrormethod.
Y (t)= Kpe(t)+ Ki ∑ e(t) (4)
Algorithm1 EnhancedEncoderBasedLfDAlgorithm
1: Initializeon‑boardsensors
2: Initializepath_matrix,reference_matrix,orientai‑ ton_matrix
3: InitializepathcountP
4: CheckforuserinputD
5: if D==Forward then
6: while D==Forward do
7: Robot ← Moveforward
8: if ReferencePoint then
9: Reference_Matrix[P] ← (LSV,RSV)
10: Path_Matrix[P] ← (1,Encoder_Value)
11: endif
12: endwhile
13: Path_Matrix[P] ← (1,Encoder_Value)
14: Orientation_Matrix[P] ← α
15: elseif D==Backward then
16: while D==Backward do
17: Robot ← Movebackward
18: endwhile
where,
e(t)=(Current_value‑Reference_value)
e(t)‑Error
Y(t)‑Controlledvariable
Kp‑Proportionalconstant
Ki‑Integralconstant
Inthepositioncorrectionsystem,therobot’spo‑ sitioniscorrectedbycontrollingtheslidingvelocity usingY(t),andintheorientationcorrectionsystem, therobot’sangularvelocityiscontrolledusingY(t) tocorrecttheposeoftherobot.Algorithm 1 shows theEEBLalgorithmwithallpossiblerobotmovements anditsmatricesupdatingondifferentconditionsfora path.
Thetreebasedplannerisapathmappingandplan‑ ningalgorithmthatusesthelearnedpathmatrixas input.ThepathmatrixformedbytheEEBLalgorithm hasonepossibleroutetoalldestinationsfromhome; thisiscalledapartiallyknownpath.Butthisinforma‑ tionisnotenoughtoplanef icientpaths.Initiallyusing theTBPalgorithm,alltheotherpossiblelogicalpaths toallthedestinationsaremapped.Asanendresult ofpathmapping,apathtreeisformedwiththehome positionastherootnodeandallthedestinationsas childnodes.Pathmappingiscarriedoutbyidentifying VLMs,whichactaswaypointsinthelogicalpathscre‑ atedandessentiallymapstheshortestpossiblepaths.
VLMsareidenti iedbasedonthetaughtpaths.Anin‑ tuitivestrategyisusedtoidentifytheVLMsandallthe pointswheretherobottakesaturn(leftorright)are consideredtobeVLMs.VLMsaretheintermediateno‑ desinthetree.Later,givenasourceanddestination, TBP’spathplannerusesthepathtreetoplanef icient pathtonavigateautonomously.
Fig.7depictsascenariowithahomepositionand threedestinationsD1,D2andD3.Allthegrayblocks areobstacles,andthelinebetweenallthedestina‑ tionsarethepathsitistaughttonavigate.Thetrai‑
19: Path_Matrix[P] ← (2,Encoder_Value)
20: elseif D==Left then
21: while D==Left do
22: Robot ← SteerLeft
23: endwhile
24: Path_Matrix[P] ← (3,Encoder_Value)
25: elseif D==Right then
26: while D==Right do
27: Robot ← SteerRight
28: endwhile
29: Path_Matrix[P] ← (4,Encoder_Value)
30: else
31: P ← P++
32: endif
Algorithm3 PreprocessingPhase‑SubPathMatrix
Stage
1: intfcount,rcount,lcount;
2: for r =0 ; r<maxr ; r ++ do
3: if Path_Matrix[r][0]==1 then
4: for c =0 ; c<macc ; c ++ do
5: Path_Matrixf[fcount][c]=
6: Path_Matrix[r][c];
7: endfor
8: endif
9: SimilarlyforLeftandRightdirectioncreating Path_Matrixl&Path_Matrixrmatrix
10: endfor
Algorithm2 TBPAlgorithm‑PathMapping
1: PreprocessingPhase‑SortingStage
2: for ri =0 ; i<maxr ; ri ++ do
3: for rj = ri +1 ; rj<maxr ; rj ++ do
4: if Path_Matrix[ri][0]>Path_Matrix[rj][0] then
5: swap(ri,rj);
6: else
7: continue;
8: endif
9: endfor
10: endfor
ningsequencefollowedwasHome→D1,D1→D2,and D2→D3,respectively.Withthistraineddata,theTBP algorithmwillgenerateVLMsandformthepathtree.
TwoVLMsareidenti ied(blackdotsalongthepath inthe igure),namelyVLM1andVLM2.VLM1ischo‑ senbecausethereisaleftturninthepathtoreach D1.Likewise,VLM2ischosenbecausethereisaright turntoreachD2.The inalpathtreewithVLMsand DestinationisshowninFig. 8.Thehomepositionis therootnode,alltheVLMsareintermediatenodes, andallthedestinationsarechildnodes.Thisisater‑ narygraph,whereeverynodecanhaveamaximumof threechildnodes,namelycenterchild,leftchild,and rightchild.Center,leftandrightnodesarethesym‑ bolicrepresentationsofnavigationdirectionforthe parenttoreachthechildnode.Foraparentnodeto reachacenterchildnode,therobothastomovefor‑ ward,andleftandrightchildnodescanbereachedsi‑ milarly.Everynodecontainsinformationlikeforward distance,turningangle,referencepointdetails,pose angle,andchildnodesdetails.
TheTBLalgorithmhastwophases:thepathmap‑ pingandthepathplanningphase.Thepathmapping phasehastwostages:thepreprocessingstageandthe
Algorithm4 StateFormationPhase
1: fcount
2: if (fcount!=0) then
3: for r =0;r<maxr;r ++ do
4: for c =0;c<maxc & Path Matrixf [r][c]!=0; do
5: if Path_Matrixf[r][c]==1 then
6: create‑forward‑VLM;
7: endif
8: SimilarlyfforLeft‑VLM&Right‑VLM
9: c+=2;
10: endfor
11: create‑destination;
12: endfor
13: else
14: Exit;
15: endif
nodeformationstage.Thepreprocessingstagesplits thepathmatrixintothreematricesbasedontherea‑ chabledestinationbymovingforwardfromhome,mo‑ vingleftfromhome,andmovingrightfromhome.This wouldbetheinputforthenodeformationstage.In thisstage,VLMsandtheirconnectivitytohomeorany destinationisidenti ied.Finally,atreewiththehome asrootnodeisformed.Algorithms2,3and4showthe pathmappingphase.
Inthepathplanningphase,theformedtreeis usedtonavigatefromagivensourcetoadesti‑ nation.Inthisphase,twopathswillbeidenti ied fromhome→sourceandhome→destination.These twopathsaremergedonacommonVLMnodeasa waypoint;thiswouldgivetheshortestroutepossible. IfthereisnocommonVLM,thenhomeisthecommon nodethatwillactasthewaypoint,andthisisthelon‑ gestroutepossible.Algorithm5showsthepathplan‑ ningphase.
ConsiderthetreeinFig. 8.Ifapathhasto beplannedbetweenD3→D1,pathsfromhometo boththenodeshavetobeidenti ied.Theyare home→VLM1→D1andhome→VLM1→VLM2→D3. ThesetwopathshaveVLM1asacommonnode.
1: path1[m]= ind_path(hometosource)
2: path2[m]= ind_path(hometodestination)
3: for r =0;r<maxr;r ++ do
4: for c =0;c<maxc;c ++ do
5: if path1[r]==path2[c] then
6: break;
7: else
8: continue;
9: endif
10: endfor
11: endfor
12: merge_routes(r,c)
MergingthepathsasVLM1asthewaypointweget D3→VLM2→VLM1→D1,andthisistheshortestlogi‑ calpathpossible.Thelongestpathwithoutusingthis techniquewouldbeD3→VLM2→VLM1→home→D1, andthisgoesthroughthehome,whichisunnecessary.
Thepathgeneratedbythepathplanningphaseis passedtotheEEBLalgorithmautonomousphase.This phasenavigatestherobotinthedesiredpathtothe destinationautonomously,whilenavigation,position, andorientationcorrectionarecarriedoutifneces‑ sary.Thisreducestheerrorinreachingthedestina‑ tionaccurately.
Theproposedalgorithmsareimplementedinthe player/stage2Droboticsimulationplatform.Thesy‑ stemistestedintwodifferentenvironmentlayouts, houseandhospital,asshowninFig.9.Thehousela‑ youtisasmallerenvironment,with8destinations, andthehospitalisacomparativelybiggerandmore complexenvironment,with28destinations.
Initially,therobotisassignedahomepositionin theenvironmentandsequentialtrainingtonavigate towardallthedestinationsiscarriedoutusingthe EEBLalgorithm.Whilethepathsarebeinglearned,the robotalsoidenti iesvirtuallandmarks(VLM)andre‑ ferencepointsintheenvironment.Afterthetraining phase,theTBPalgorithmgeneratesatreewiththe homeastherootnode,VLMsasintermediatenodes, andallthedestinationsaschildnodes.
Fig.10depictsthepathsinthehouselayoutwith VLMsandreferencepoints,andFig. 11 showsthe treegeneratedforthepathslearnedwith4VLMsand 14nodes.Fig. 12 showsthepathslearnedinhos‑ pitallayoutwithVLMsandreferencepoints.Fig. 13 showsthetreeformedusingthehospitallayoutwith 24VLMsandatotalof52nodes.Comparedtothe houselayout,thislayoutis3.5timesbiggerinterms ofsize,numberofdestinations,andnumberofnodes inthetree.Thisprovesthatthealgorithmisscala‑ ble.Givenadestination,apathcanbeplannedusing thetreeandnavigatedtoreachthedestination.For example,considertherobot’scurrentpositiontobe inD1ofthehospitallayoutandthegivendestina‑
tiontobeD21.Inthiscase,therobotplansapath bytraversingthetreeand indingtheshortestpos‑ sibleroutefromsourcetodestination,whichwillbe
Themostcommonerrorsinamobilerobotnaviga‑ tionsystemarepositionerrorandorientationerror. Orientationerroriscorrectedbystoringtheposeva‑ lueoftherobotalongwithpathvariablesduringpath learning.Positionerrorscanbecorrectedbyidenti‑ fyingreferencepointsalongthepaths.Thesereferen‑ ceswillbeusedintheautonomousphasetocorrect positionerroralongthex‑axisoftherobot’scoordina‑ tes.Aclosed‑loopPIcontrolsystemisusedtocorrect theerrors.
Atestwasconductedtoprovetheef iciencyofthe errorcorrectioncontrolsystem,andtherepeatability oftheproposedsystem.Inordertotesttheerrorcor‑ rectionalgorithmef iciency,aGaussianerrorisadded intheforwardmovementoftherobot(toaddade‑ viationintheheadingdirection)andthewheelenco‑
deroftherobot(tointroduceerrorintheturningan‑ gle).Repeatabilitywasveri iedbyrunningarobotto andfroma ixedsourceanddestinationseveraltimes andcalculatingtherelativeaverageerrorofitsposi‑ tion.Ashadowrobotisaddedinthesimulationtorun withtheactualrobotbutwithoutgoingthroughany errorcorrectionalgorithm,toshowtheeffectiveness oftheproposederrorcorrectionalgorithm.Equation 5showstherobotpositionrepresentationinthesimu‑ lationenvironment.
Case1:HometoD3 Considerthehouselayoutwith thesourceashomeanddestinationasD3.Thispath wastraversed100timesbackandforthcontinuously withaGaussianerrorof µ=0and σ=1,whichwascho‑ senbytrialanderrormethod.Essentially,therobotre‑ acheshomeandD3,50timeseach.Positiondataforall thetrialsarecollected.Referenceposition(recorded inthetrainingphase),actualpositionwitherrorcor‑ rectionandactualpositionwithouterrorcorrection areplottedinFig.14.Fig.15showstheplotofthero‑ bot’sD3position.
Case2:D2toD5 Thispathismappedbymergingtwo pathsfromhome→D2andhome→D5.Thispathistra‑ versedbackandforth100timeswithGaussianerror of µ=0and σ=1.Therobot’spositionplotsinD2and D5after100runsareshowninFig.16andFig.17,re‑ spectively.
Case3:HometoD3withGausserror(0,2) Tovali‑ datetheef iciencyoftheerrorcorrectionalgorithm thesamepathascase1isexecutedwithadifferent Gaussianerrorparameter.Thecorrespondingplots areshowninFig.18andFig.19.
Relativeaveragepositionerroriscalculatedforall thecases.Equation6isusedtocalculatetheerror% withrespecttothereferenceposition.Table1shows therelativeaveragepositionerrorofthecasesdis‑ cussed.ItisveryevidentthattheXpositionandpose averagewitherrorcorrectionaremuchlessthanwit‑ houterrorcorrectionaverage.Eventhoughthemain objectiveofourerrorcorrectionalgorithmistoreduce Xpositionandposeangleerrors,toanextentitisin‑ directlycorrectingtheYpositionerror,too.Thiscan beobservedincase1:D3andcase3:D3scenarios.The overallaveragepositionalerrorbasedonallthethree casesisgiveninequation7
exactreplica(withthesamenumberofcells)ofthe hospitallayoutiscreatedintheJavasimulatorand iscomparedwiththeresultantpath.Twopathsare considered:Home→D28andD7→D28.Fig.20and21 showthepathgeneratedbytheproposedandother algorithmsfortwodifferentpaths.Thepathmapped bytheproposedalgorithmisgiveninequation 8.A quantitativecomparisonbasedonboththepathsis depictedintable2.Thepathlength(No.ofcells)map‑ pedbytheproposedalgorithmisonparwiththeot‑ heralgorithms,whereasthevisitedcellcountisvery smallcomparedtootheralgorithms.Thisconsidera‑ blyreducesthepathplanningtimeintheproposedal‑ gorithm.Hencetheproposedalgorithmisfasterwith ef icientpathmapping.
Theproposedalgorithmiscomparedwithbreadth irstsearch(BFS),Dijkstra’s,greedybest irstsearch (BBF),A*andrapidlyexploringrandomtrees(RRT) algorithms.Tocomparewiththeproposedalgorithm, aJava‑basedpathplanningsimulator[10]isused.An
5.Conclusion
Inthispaper,anovelpathplanningalgorithmba‑ sedonLearningfromDemonstrationandTreeBased Pathplanneralgorithmsisproposed.Themainfocusis onindoormobileservicerobots,whichcanbeusedin commercialanddomesticplaceswithoutanyenviron‑ mentaugmentation.Overall,twoalgorithms,theEn‑ hancedEncoderBasedLfD(EEBL)algorithmandTree BasedPathPlanner(TBP)algorithm,areproposed. Boththesealgorithmsworkincohesiontoachievethe desiredpathgivenasourceanddestination.Initially, EEBLisusedtolearnthepartialpathsintheenviron‑ ment.Later,TBPmapsallthelogicalpathswithvirtual landmarks(VLM)andreferencepoints.Thesystemis alsocapableofcorrectingpositional/orientationer‑ rorsthatoccurduetohardwareanomaliesinthero‑ bot.AsimplePIcontrolsystemisusedtocorrectthe errors.Theproposedsystemisimplementedandtes‑ tedinPlayer/Stage,a2Droboticsimulator.Theal‑ gorithmistestedintwodifferentenvironmentswit‑ houtanychangesinthealgorithm.Thismakesthepro‑ posedalgorithmenvironment‑independenti.e.porta‑ ble.Oneenvironmentis3.5timesbiggerthantheot‑ her,whichprovesthealgorithmisscalable.Inorder totesttheaccuracyandrepeatability,asinglepathis executedrepeatedlybyaddinggaussianerrorinthe robotmovement.Theoverallaveragedestinationpo‑ sitionaccuracywascalculatedtobe ±5%(bothX‑ positionandpose).Finally,thepathplannedfroma ixedsourceanddestinationbytheproposedalgo‑ rithmiscomparedwithsomeoftheclassicalandstate‑ of‑the‑artpathplanningtechniques.Theresultshows thattheproposedalgorithmhasfewervisitedcells
andisonparintermsofpathlength.Hencethepro‑ posedalgorithmprovestohavepropertieslikeporta‑ bility,scalability,repeatability,accuracy,shorterpath planningtime,andef icientpathgeneration.
A.A.NippunKumaar∗ –DepartmentofCom‑ puterScienceandEngineering,AmritaSchoolof Engineering,Bengaluru,AmritaVishwaVidyapeet‑ ham,India,e‑mail:nippun05@gmail.com,www: www.nippunkumaar.in.
SreejaKochuvila –DepartmentofElectronics andCommunicationEngineering,AmritaSchoolof Engineering,Bengaluru,AmritaVishwaVidyapeet‑ ham,India,e‑mail:k_sreeja@blr.amrita.edu,www: https://amrita.edu/faculty/k‑sreeja/.
S.R.Nagaraja –DepartmentofMechanical Engineering,AmritaSchoolofEngineering, Bengaluru,AmritaVishwaVidyapeetham,In‑ dia,e‑mail:sr_nagaraja@blr.amrita.edu,www: https://amrita.edu/faculty/sr‑nagaraja/.
∗Correspondingauthor
[1] M.Algabri,H.Mathkour,H.Ramdane,andM.Al‑ sulaiman,“Comparativestudyofsoftcompu‑ tingtechniquesformobilerobotnavigationin anunknownenvironment”, ComputersinHu‑ manBehavior,vol.50,2015,pp.42–56. https://doi.org/10.1016/j.chb.2015.03.062
[2] V.Alvarez‑Santos,A.Canedo‑Rodriguez,R.Igle‑ sias,X.Pardo,C.Regueiro,andM.Fernandez‑ Delgado,“Routelearningandreproductionin atour‑guiderobot”, RoboticsandAutonomous Systems,vol.63,Part2,2015,pp.206–213.
https://doi.org/10.1016/j.robot.2014.07.013
[3] B.D.Argall,S.Chernova,M.Veloso,andB.Brow‑ ning,“Asurveyofrobotlearningfromde‑ monstration”, RoboticsandAutonomousSys‑ tems,vol.57,no.5,2009,pp.469–483.
https://doi.org/10.1016/j.robot.2008.10.024
[4] R.Arkin,“Motorschema‑basedmobilerobotna‑ vigation”, InternationalJournalofRoboticsRese‑ arch,vol.8,1989,pp.92–112.
[5] A.Bakdi,A.Hentout,H.Boutami,A.Ma‑ oudj,O.Hachour,andB.Bouzouia,“Optimal pathplanningandexecutionformobilero‑
botsusinggeneticalgorithmandadaptive fuzzy‑logiccontrol”, RoboticsandAutono‑ mousSystems,vol.89,2017,pp.95–109. https://doi.org/10.1016/j.robot.2016.12.008
[6] D.A.Brenna,B.Brett,andM.V.Manuela,“Policy feedbackforthere inementoflearnedmotion controlonamobilerobot”, InternationalJournal ofSocialRobotics,vol.4,no.4,2012,pp.383–395. 10.1007/s12369‑012‑0156‑9
[7] S.Choi,E.Kim,K.Lee,andS.Oh,“Real‑time nonparametricreactivenavigationofmobilero‑ botsindynamicenvironments”, RoboticsandAu‑ tonomousSystems,vol.91,2017,pp.11–24. https://doi.org/10.1016/j.robot.2016.12.003
[8] K.Dermot,U.Nehmzow,andA.S.Billings.“To‑ wardsautomatedcodegenerationforautono‑ mousmobilerobots”,June2010.
[9] H.S.Dewang,P.K.Mohanty,andS.Kundu, “Arobustpathplanningformobilerobot usingsmartparticleswarmoptimization”, ProcediaComputerScience,InternationalCon‑ ferenceonRoboticsandSmartManufacturing (RoSMa2018),vol.133,2018,pp.290–297. https://doi.org/10.1016/j.procs.2018.07.036
[10] S.S.Dhanjal. PathPlanninginSingleandMulti‑ robotSystems.PhDthesis,BITS,Pilani,May2016.
[11] E.W.Dijkstra.“Anoteontwoproblems inconnexionwithgraphs”,December1959. 10.1145/3544585.3544600
[12] D.Dolgov,S.Thrun,M.Montemerlo,andJ.Diebel. “Practicalsearchtechniquesinpathplanningfor autonomousdriving”,Jan2008.
[13] S.DongandB.Williams,“Learningandrecogni‑ tionofhybridmanipulationmotionsinvariable environmentsusingprobabilistic lowtubes”, In‑ ternationalJournalofSocialRobotics,vol.4,no.4, 2012,pp.357–368.10.1007/s12369‑012‑0155‑ x
[14] M.Ehrenmann,O.Rogalla,R.Zöllner,andR.Dill‑ mann.“Teachingservicerobotscomplextasks: Programmingbydemostrationforworkshop andhouseholdenvironments”,2002.
[15] D.FergusonandA.T.Stentz.“The ieldd*al‑ gorithmforimprovedpathplanningandreplan‑ ninginuniformandnon‑uniformcostenviron‑ ments”,June2005.
[16] E.GalceranandM.Carreras,“Asur‑ veyoncoveragepathplanningforrobo‑ tics”, RoboticsandAutonomousSystems, vol.61,no.12,2013,pp.1258–1276. https://doi.org/10.1016/j.robot.2013.09.004
[17] A.M.E.GhalamzanandM.Ragaglia,“Ro‑ botlearningfromdemonstrations:Emu‑ lationlearninginenvironmentswith movingobstacles”, RoboticsandAutono‑ mousSystems,vol.101,2018,pp.45–56. https://doi.org/10.1016/j.robot.2017.12.001
[18] S.Hacohen,S.Shoval,andN.Shvalb,“Applying probabilitynavigationfunctionindynamicun‑ certainenvironments”, RoboticsandAutono‑ mousSystems,vol.87,2017,pp.237–246. https://doi.org/10.1016/j.robot.2016.10.010
[19] D.Halbert.“Programmingbyexample”.PhDthe‑ sis,UniversityofCalifornia,Berkeley,November 1984.
[20] M.HankandM.Haddad,“Ahybridapproach forautonomousnavigationofmobilerobotsin partially‑knownenvironments”, RoboticsandAu‑ tonomousSystems,vol.86,2016,pp.113–127. https://doi.org/10.1016/j.robot.2016.09.009
[21] P.Hart,N.Nilsson,andB.Rafael,“Aformalbasis fortheheuristicdeterminationofminimumcost paths”, IEEETransactionsonSystemsScienceand Cybernetics,vol.4,1968,pp.100–107.
[22] J.Hong,Y.Choi,andK.Park.“Mobilerobot navigationusingmodi ied lexiblevector ield approachwithlaserrange inderandIRsen‑ sor”,2007InternationalConferenceonControl, AutomationandSystems,2007,pp.721–726. 10.1109/ICCAS.2007.4406993
[23] R.Huq,G.K.Mann,andR.G.Gosine, “Mobilerobotnavigationusingmotor schemaandfuzzycontextdependentbe‑ haviormodulation”, AppliedSoftCompu‑ ting,vol.8,no.1,2008,pp.422–436. https://doi.org/10.1016/j.asoc.2007.02.006
[24] Y.K.HwangandN.Ahuja,“Apotential ieldap‑ proachtopathplanning”.In: IEEETransactionon RoboticsandAutomation,vol.8,1992,pp.23–32.
[25] R.Jäkel,S.R.Schmidt‑Rohr,S.W.Rühl,A.Kas‑ per,Z.Xue,andR.Dillmann,“Learningofplan‑ ningmodelsfordexterousmanipulationbased onhumandemonstrations”, InternationalJour‑ nalofSocialRobotics,vol.4,no.4,2012,pp.437–448,10.1007/s12369‑012‑0162‑y
[26] F.Kamil,T.S.Hong,W.Khaksar,M.Y.Moghra‑ biah,N.Zulki li,andS.A.Ahmad,“Newro‑ botnavigationalgorithmforarbitraryunknown dynamicenvironmentsbasedonfuturepre‑ dictionandprioritybehavior”, ExpertSystems withApplications,vol.86,2017,pp.274–291. https://doi.org/10.1016/j.eswa.2017.05.059
[27] J.Lee,Y.Nam,S.Hong,andW.Cho,“Newpo‑ tentialfunctionswithrandomforcealgorithms usingpotential ieldmethod”, JournalofIntelli‑ gent&RoboticSystems,vol.66,no.3,2012,pp. 303–319.10.1007/s10846‑011‑9595‑z
[28] R.Limosani,A.Manzi,L.Fiorini,F.Cavallo,and P.Dario,“Enablingglobalrobotnavigationbased onacloudroboticsapproach”, InternationalJour‑ nalofSocialRobotics,vol.8,no.3,2016,pp.371–380.10.1007/s12369‑016‑0349‑8
[29] S.M.Lavalle.“Rapidly‑exploringrandomtrees:A newtoolforpathplanning”,May1999.
[30] T.T.Mac,C.Copot,D.T.Tran,andR.D.Key‑ ser,“Heuristicapproachesinrobotpath planning:Asurvey”, RoboticsandAutono‑ mousSystems,vol.86,2016,pp.13–28. https://doi.org/10.1016/j.robot.2016.08.001
[31] D.Nakhaeinia,S.Tang,S.Noor,andO.Motlagh,“A reviewofcontrolarchitecturesforautonomous navigationofmobilerobots”, InternationalJour‑ nalofPhysicalSciences,vol.6,2011,pp.169–174.
[32] U.Nehmzow,O.Akanyeti,C.Weinrich,T.Kyria‑ cou,andS.A.Billings.“Programmingmobilero‑ botsbydemonstrationthroughsystemidenti i‑ cation”,EuropeanConferenceonMobileRobots, Freiburg,Germany,19Sep2007‑21Sep2007.
[33] A.A.NippunKumaarandT.S.B.Sudarshan,“Mo‑ bilerobotprogrammingbydemonstration”.In: 2011FourthInternationalConferenceonEmer‑ gingTrendsinEngineeringTechnology,vol.394, 2011,pp.206–209.
[34] A.A.NippunKumaarandT.S.B.Sudarshan,“Le‑ arningfromdemonstrationwithstatebasedob‑ stacleavoidanceformobileservicerobots”, App‑ liedMechanicsandMaterials,vol.394,2013,pp. 448–4556.
[35] A.NippunKumaarandT.Sudarshan,“Sensor counterapproachforamobilerobottonavi‑ gateapathusingprogrammingbydemonstra‑ tion”, ProcediaEngineering,InternationalCon‑ ferenceonCommunicationTechnologyandSy‑ stemDesign,vol.30,2012,pp.554–561. https://doi.org/10.1016/j.proeng.2012.01.898
[36] S.Osentoski,B.Pitzer,C.Crick,G.Jay,S.Dong, D.Grollman,H.B.Suay,andO.C.Jenkins, “Remoteroboticlaboratoriesforlearningfrom demonstration”, InternationalJournalofSocial Robotics,vol.4,no.4,2012,pp.449–461. 10.1007/s12369‑012‑0157‑8
[37] A.Pandey,S.Kumar,K.K.Pandey,andD.R.Parhi, “Mobilerobotnavigationinunknownstaticen‑ vironmentsusingan iscontroller”, Perspectives inScience,RecentTrendsinEngineeringand MaterialSciences,vol.8,2016,pp.421–423. https://doi.org/10.1016/j.pisc.2016.04.094
[38] B.Patle,A.Pandey,A.Jagadeesh,andD.Parhi, “Pathplanninginuncertainenvironment byusing ire lyalgorithm”, DefenceTechno‑ logy,vol.14,no.6,2018,pp.691–701. https://doi.org/10.1016/j.dt.2018.06.004
[39] A.T.Rashid,A.A.Ali,M.Frasca,andL.For‑ tuna,“Pathplanningwithobstacleavoi‑ dancebasedonvisibilitybinarytreealgo‑ rithm”, RoboticsandAutonomousSystems, vol.61,no.12,2013,pp.1440–1449. https://doi.org/10.1016/j.robot.2013.07.010
[40] I.R.Roberto,N.Ulrich,K.Theocharis,and B.Steve.“Modellingandcharacterisationofa mobilerobot’soperation”,September2006.
[41] A.Stentz.“Optimalandef icientpathplanning forpartially‑knownenvironments”,May1994.
[42] Z.Tahir,A.H.Qureshi,Y.Ayaz,andR.Na‑ waz,“Potentiallyguidedbidirectionalized rrt*forfastoptimalpathplanninginclut‑ teredenvironments”, RoboticsandAutono‑ mousSystems,vol.108,2018,pp.13–27. https://doi.org/10.1016/j.robot.2018.06.013
[43] P.Vadakkepat,T.H.Lee,andL.Xin.“Application ofevolutionaryarti icialpotential ieldinrobot soccersystem”,AnnualConferenceoftheNorth AmericanFuzzyInformationProcessingSociety, July2001.
[44] J.Wang,H.Kimura,andM.Sugisaka,“Intelli‑ gentcontrolforthevision‑basedindoornavi‑ gationofana‑lifemobilerobot”, Arti icialLife andRobotics,vol.8,no.1,2004,pp.29–33. 10.1007/s10015‑004‑0283‑y
[45] L.Wang,“Automaticcontrolofmobilerobotba‑ sedonautonomousnavigationalgorithm”, Arti‑ icialLifeandRobotics,vol.24,no.4,2019,pp. 494–498.10.1007/s10015‑019‑00542‑0
[46] L.Xu,L.G.Zhang,D.G.Chen,andY.Z.Chen, “Themobilerobotnavigationindynamicenvi‑ ronment”, 2007InternationalConferenceonMa‑ chineLearningandCybernetics,vol.1,2007,pp. 566–571.10.1109/ICMLC.2007.4370209
[47] D.Zhenjun,Q.Daokui,X.Fang,andX.Dianguo. “Ahybridapproachformobilerobotpathplan‑ ningindynamicenvironments”,2007IEEEInter‑ nationalConferenceonRoboticsandBiomime‑ tics(ROBIO),2007,pp.1058‑1063.10.1109/RO‑ BIO.2007.4522310
Submitted: 21st July 2021; accepted 22nd February 2022
Mateusz Pająk, Marcin Racław, Robert PiotrowskiDOI: 10.14313/JAMRIS/1-2022/5
Abstract:
The paper presents a design for a six-axis manipulator. The design consists of specially designed solutions for housing, planetary gearboxes, and electronics. The manipulator is controlled by a supervisory control system. The use of a series of measuring elements allows tracking of the current position of each axis. This can be used to create a cascade control loop with velocity and acceleration feed-forward. The implemented control algorithm together with the microcontroller software allows one to tune parameters of the controllers for both control loops: inner, related to the speed of the robot; outer, related to its position.
Keywords: industrial manipulators, servostepper motors, cascade control, stateful machine
The uninterrupted technological development in industrial branches that was initiated decades ago has replaced human participation in some hard and monotonous work with robot work. Initially, devices that were used made it only slightly easier to perform harder tasks. Most of them still required considerable human intervention. Their operation was limited to only simple movements such as lifting or moving elements.
Replacement of human participation in work that requires much effort and precision has opened opportunities for dynamic development of computer-controlled machines. More and more modern machines appeared, bringing with them the possibility of performing more and more complex tasks. This made it possible to partially eliminate the role of the human being as an unit of the lowest degree of production. The rapid increase in use of programmable machines allowed advanced units to enter production lines. The first industrial use of a robotic manipulator is credited to the automotive industry, in the case of General Motors. The robots were used to handle heavy metal parts. However, the real breakthrough in the design of manipulators was made in 1969: the Stanford arm manipulator with two rotary connections, prismatic and spherical. This design significantly increased handling capabilities of robots [1].
As a result of mass technological development, industrial robots, and with them various types of manipulators, have found their place in many industrial
branches. Currently, they can be found in the automotive industries as well as in food production. Due to their multi-tasking capabilities, they can be used in tasks that require use of high forces, for instance, transferring heavy materials, or exceptional precision, as in the case of precision soldering. Replacement of the actuator allows the manipulator to be adapted to the prevailing conditions or purpose, enabling it to perform a range of tasks such as welding, material processing, or assembling available parts together. However, despite their versatile uses, their biggest advantage is the ability to cooperate with people on production lines, enabling maximization of profits while reducing human effort.
The goal of this article is the physical representation of a miniature version of an industrial manipulator with an executive element. During the design process, it was decided to use a mechatronic approach using knowledge of mechanics, electronics, control engineering, and computer science. The work can be divided into two main stages: modeling and physical representation. The modeling process included the implementation of a three-dimensional model of the robot housing, a model of the control algorithm, and modeled electronic connections. The construction stage consists of the implementation of the housing, connection of actuators, implementation of control algorithms, and integration all of the above individual components. Due to the robot’s structure, it is considered as a dynamic, non-linear, and unstable system.
The design assumptions determined the dimensions of the robot, its load capacity, and its shape. The first and most important step in the construction process was to determine preliminary dimensions of particular components. Knowledge of such dimensions allowed for selection of the motors. During motor selections many parameters were taken into account, such as their type (stepper motor), winding, current value, load curve, and price. Finally, appropriate motors were selected using basic mechanical relationships related to the mass of elements and their arrangement. Their selection directly determined the final dimensions of the manipulator. The final version in the base position reached a height not exceeding 40 cm.
Arranging the arm in a horizontal position, the most critical in terms of load, allowed the manipulator to reach objects more than half a meter away, measured from the first axis of rotation.
generally found in the automotive industry. The initial stage of the project was to use so-called reverse engineering on motors, consisting in obtaining a three-dimensional model from existing elements. Their virtual version allowed the starting of the design stage of subsequent parts of the manipulator. The fundamental part of the model is the base with the axis 1 motor placed in it. It forms the basis of the whole robot. Its design divides it into two parts that can be easily connected. Appropriate holes have also been made to allow the device to be permanently attached at the workplace. The base model includes mounting holes for the base motor inside of it , which allows for connection of the motor shaft with gearing. It was decided to make custom planetary gearboxes due to the nonstandard dimensions of the collective elements. To obtain proper movement of joints, a special system that holds gearboxes with joints together was designed to integrate both the gearbox and a custom-designed ball bearing system into the chassis. Also, appropriate recesses were made to allow easy and effective placement of bearing balls around the ring gears of the gearbox. The combination of these two elements made i t possible to obtain a suitable gearbox ratio at the output , and to ensure its smooth course.
The purpose of the designed model was to make its functionality and appearance similar to robots generally found in the automotive industry. The initial stage of the project was to use so-called reverse engineering on motors, consisting in obtaining a three-dimensional model from existing elements. Their virtual version allowed the starting of the design stage of subsequent parts of the manipulator. The fundamental part of the model is the base with the axis 1 motor placed in it. It forms the basis of the whole robot. Its design divides it into two parts that can be easily connected. Appropriate holes have also been made to allow the device to be permanently attached at the workplace. The base model includes mounting holes for the base motor inside of it, which allows for connection of the motor shaft with gearing. It was decided to make custom planetary gearboxes due to the nonstandard dimensions of the collective elements. To obtain proper movement of joints, a special system that holds gearboxes with joints together was designed to integrate both the gearbox and a custom-designed ball bearing system into the chassis. Also, appropriate recesses were made to allow easy and effective placement of bearing balls around the ring gears of the gearbox. The combination of these two elements made it possible to obtain a suitable gearbox ratio at the output, and to ensure its smooth course.
ones. Bigger modules allowed more infill material inside the teeth which made for a stronger support structure. The smaller pieces would be more prone to deformation as the outer shell of 3D printed parts is processed more for quality rather than durability by most of the available 3D printing software. To achieve this task the correct selection of the number of teeth was required. For this purpose, the equation (1) describing the relationship between the number of teeth on planets (P1-P2), rings (R1-R2), and the sun (S1) in relation to the ratio (R) was applied. Due to the forces needed to move individual axles, it was decided to set the ratio at the level of 30–45: 1. This value of the gear ratio allowed for smooth movement with sufficient power needed to move. The limitations in the method of execution resulted in top-down ranges as to the number and size of teeth in gears. Using the intended number of teeth on one of the gears, the values of the others were found using the equation.
stronger support structure. The smaller be more prone to deformation as the printed parts is processed more for than durability by most of the available software. To achieve this task the correct the number of teeth was required. For the equation (1) describing the relationship the number of teeth on planets (P1-P2), and the sun (S1) in relation to the applied. Due to the forces needed to axles, it was decided to set the ratio at 45: 1. This value of the gear ratio allowed movement with sufficient power needed limitations in the method of execution down ranges as to the number and gears. Using the intended number of the gears, the values of the others were the equation.
(1)
(1)
The result was two transmission sizes with a ratio of 41:1 for the first axis motor and 32:1 for the 4–6 axis motors. Connecting the axle with custom planetary gears requires two levels defining the desired gear ratio. In the lower part, most of the elements are mounted together with planets with double number of teeth (Fig. 1). The upper part contained a ring necessary for the axis movement.
From the mechanical point of view, the biggest load is placed on the second axis. Because the dimensions of the motor of the second axis were increased, it was decided to make a custom mount for it, allowing it to be placed in the axis of rotation of the previous axis. Due to the high forces acting on this axle, a special, dedicated metal gearbox was purchased. To make sure that the plastic case of second axle will not break due to the big load that it is supposed to carry , it has been reinforced with three metal rods that were connected to the shaft of the motor. Their installation inside the housing significantly strengthened the entire structure, increasing the resistance to material destruction caused by the shear moment generated by the motor.
From the mechanical point of view, the biggest load is placed on the second axis. Because the dimensions of the motor of the second axis were increased, it was decided to make a custom mount for it, allowing it to be placed in the axis of rotation of the previous axis. Due to the high forces acting on this axle, a special, dedicated metal gearbox was purchased. To make sure that the plastic case of second axle will not break due to the big load that it is supposed to carry, it has been reinforced with three metal rods that were connected to the shaft of the motor. Their installation inside the housing significantly strengthened the entire structure, increasing the resistance to material destruction caused by the shear moment generated by the motor.
While selecting actuators, it was decided to use two stepper motors with pre-attached planetary metal gearboxes for axes two and three as plastic ones potentially could not handle required torques. Their ratio allows them to obtain the necessary moment for the appropriate axle. In order to reduce cost, other motors were connected to individual 3D printed gearboxes. The model of the manipulator was designed in the Autodesk Inventor 2019 environment [2] with functionality in mind. By using gears with larger teeth and modules, it was possible to achieve a better gear ratio with less mechanical wear than by using smaller
The result was two transmission sizes 41:1 for the first axis motor and 32:1 motors. Connecting the axle with custom gears requires two levels defining the ratio. In the lower part, most of the mounted together with planets with of teeth (Fig. 1). The upper part contained necessary for the axis movement.
In the project it was planned to obtain an independent executive element in the form of a gripper. The design of its individual components as a separate object aims to achieve relative modularity. The special design allows easy connection with the arm and gives the opportunity to replace it with another actuator if needed. The gripper has a single servo motor as its actuator. In the design process of the tool, accuracy and high precision were the most important aspects. The main components are the appropriate gears re-
sponsible for the transfer of force from the servo and gripping parts. Their specific shape allows for gripping both regular oval objects and irregular objects.
The elements of the manipulator were created by using 3D printing technology. The ability to print almost any shape allowed for effective production of individual housing parts. Proper stiffness and durability of elements was achieved by appropriate thickness of components and the use of appropriate material. Two types of material were used in the printing process: PLA (polylactic acid, polylactide) and PETG (polyethylene terephthalate). The first of them is used to make the entire housing and the gripper, as it is easier to print and postprocess. Due to the highest mechanical load, it was decided to use a filament with much higher strength (PETG) for the production of gears. Because of limited possibilities associated with the available print field, individual parts had to meet the requirements related to their size. As a result, a modular representation of the entire manipulator was obtained (Fig. 2). To facilitate the process of joining elements, the robot was assembled using bolts and nuts of various sizes.
municate with the master system. The heart of the system is the 32 bit STM32F103RBT6 microcontroller. The main selection criterion was the number of counters and the appropriate number of I/O. On the board, apart from the microcontroller, there are two voltage controllers: 3.3V and 5V. With those controllers, it is possible to power the logic with motor power supply (24V). The 3.3V voltage is used to power the microcontroller, A4988 controllers located on another module, encoders, and as a reference voltage for the limit switches located on the manipulator. 5V supplies the TB6600 controllers, which are controlled from the microcontroller through the 74HC245 IC which acts as a logic level converter. The role of the master device was played by a PC computer communicating with the motherboard through a USB / Serial converter.
The next module is the AM4096 magnetic encoder board. The selection criterion was very high resolution: 4096 pulses per revolution and numerous communication options. In addition to the encoder IC, the board only has filter capacitors and a four-pin connector. Ready encoder modules were mounted on the rear parts of the stepper motors. For communication with the motherboard, it was decided to use the I2C bus. Before using the encoders, they had to be configured; also, different I2C addresses had to be set. Configuration was done using an Arduino UNO board.
Control of stepper motors is done by using additional systems that act as controllers. In order to control the motors of the second and third axes, it was decided to use TB6600 controllers, which are able to supply current up to 4A per coil. Control of the remaining motors was achieved by means of popular A4988 controllers. Due to their physical structure, which prevents direct mounting on the base, adapters were made. Each of them has two controllers. The adapters have screw connectors connecting the motors with controllers and their power supply.
The motherboard was made by an external company. The remaining modules were made using the thermal transfer method [3].
Due to the large amount of electronics required to control the robot, it was decided to divide the electronic part into a set of dependent modules arranged in the robot base. The electronic part project was started by dividing the required electronics into modules and by planning the topology of connections between them. Finally, the electronics were divided into 6 modules, whose logic inputs / outputs were connected by ribbon cables using ribbon connectors.
The most important and the most complicated module is the motherboard. Its task is to coordinate the work of other modules, read the sensors, send control signals, perform control algorithms, and com-
A separate Atmega328p microcontroller in the form of an Arduino Nano was used to control the gripper, which communicates with the motherboard via second I2C bus. The use of an additional microcontroller gives the opportunity to change the type of tool, thereby increasing the number of potential applications of the robot and creating a multi-task device. The gripper is driven by the MG995 servo motor.
The manipulator control was made using a hierarchical layered structure (Fig. 3) [4]. The top layer is the PC, and the bottom was the control system. The master control system sets the current position of the manipulator, its movement, and control parameters. The manipulator’s control board only listens to the commands as a slave device and performs control algorithms. Position time series are generated in the robot microcontroller software.
The manipulator is controlled by a system of commands sent from the master device to the motherboard through the serial port. Commands are sent as frames of different lengths, in which the first byte specifies the function, then n bytes determines the function arguments, and the last two bytes act as a checksum. The commands are received by the command interpreter, which transmits the command to the lower layers of the system to initiate movement or change the parameters of the control system. The interpreter can be in one of two states: operating or configuration state. In operating state, the interpreter accepts two types of commands: those responsible for movement of the robot’s joints and those that are feed forwarded to the executive device, which is the gripper.
The configuration state allows tuning the parameters of the control system, including the parameters of P and PI controllers from the cascade control system, feed-forward gain amplification of speed and acceleration, and the low-pass filter that filters speed readings from the encoder. Configuration state also allows reading the motors step response, step responses of speed and position. After the command passes
through the interpreter, the system responds with information about how the command was interpreted. In the event of an error or a fatal error, the frame includes relevant information on the type of occurring error.
The control of a single servo stepper motor was implemented using a state machine diagram [5], in which each state corresponds to a single motor functionality. Transitions between individual states are shown in Figure 4. The state of control and holding position is the default state of the motor. There are also three additional states responsible for receiving step response of the motor and control system, homing and the state in which the motor has not been homed.
The initial state of the entire system is a no-reaction state, where it is impossible to perform any action except axis homing. This condition occurs after switching the power supply on or after performing a step test response. Homing was also based on a set of states and is done using the seek-and-feed method, similar to CNC (Computerized Numerical Control) machines. During homing, the axis moves quickly towards the limit switch (seek) and after encountering it moves away, then moves again towards it, but slower than before (feed). This procedure allows quick finding of the area where the limit switch is located and finding the exact position of this switch. When switch is found, the microcontroller resets the appropriate encoder register. To prevent contact vibration, the limit switches are equipped with additional debouncing capacitors. After homing, the motor enters the regulation state and maintains its position regardless of the forces and interferences. In order to maintain the set position, a cascade control system with an internal speed loop and an external loop from the position was designed (Figure 5). In addition, a feed-forward from speed and acceleration has been added to the control system [6]. The use of feed-forward improves the system’s dynamic properties and accelerates its response to setpoint change. In order to measure the speed, it was decided to differentiate the position from the encoder and pass it through a low-pass filter to eliminate high frequency noise [7].
While the encoder IC allows speed measurement, it has been shown that it is not suitable for the project because of too large measurement error and too large velocity oscillations. The control system uses nonlinear elements, improving the quality of regulation. The first one is saturation, which prevents the control signal from being too high. Saturation was placed at the output of both controllers of the cascade control system. The integrator in the velocity controller was equipped with an anti-windup filter. The last nonlinear component in the system is the dead zone, without which, due to the properties of the used motors, the axis would oscillate between two discrete steps.
The dead zone value was determined as +/- the value of one step after the transfer through the gearbox. Thanks to this operation, despite the occurring non-zero adjustment error resulting from the placement of the set point between two steps, the axis stably maintains the given position. During tests without a dead zone, it was shown that the control system on axes 2 and 3 is able to enter a state in which the system loses its stability.
From the regulation state, it is possible to home the manipulator again, or if it is in the configuration state, it is possible to obtain one of three step responses. Each step response sample is sent, as a standard functions response, and is secured by a checksum. After step response, it is possible to convert it to a CSV file, which allows further testing and simulation. Thanks to this it is possible to test the quality of control offline.
The operation of control algorithms should be controlled and protected against unexpected events. For this purpose, three independent protections were applied in the microcontroller’s program. For each of them, the manipulator will interrupt the currently performed operation. Also, with each attempt to control, it will respond with a critical error frame with a description of where the event occurred. The first critical event is the loss of connection with encoders.
The second critical event concerns the state of limit switches. Switches can be pressed only at appropriate times during homing. If a low state is detected at any other time, it may mean that the switches have worn debouncing or someone has broken safety rules and is ding the allowable movement area of the axis. The algorithm doesn’t allow such movement but it is possible that the controller parameters have been incorrectly selected and the system has too much overshoot. In this case prevented by safety
In order to test the finished device, a dedicated PC application was written. The application allows control of individual axes of the manipulator,
If any of the encoders on the I2C bus fail to respond after a few attempts, the manipulator will stop.
The second critical event concerns the state of limit switches. Switches can be pressed only at appropriate times during homing. If a low state is detected at any other time, it may mean that the switches have worn out, something has happened to the debouncing capacitors, or someone has broken safety rules and is in the presence of a working robot.
The last event is related to exceeding the allowable movement area of the axis. The algorithm doesn’t allow such movement but it is possible that the controller parameters have been incorrectly selected and the system has too much overshoot. In this case collision is possible and should be prevented by safety measures.
performance of homing procedure, updating the parameters of the control loop, and obtaining of step responses in jpg, png, bmp, pdf and csv formats. The state of each axis, its setpoint and process value , can be observed on graphs updated every 100ms or on displays.
In order to test the finished device, a dedicated PC application was written. The application allows control of individual axes of the manipulator, performance of homing procedure, updating the parameters of the control loop, and obtaining of step responses in jpg, png, bmp, pdf and csv formats. The state of each axis, its setpoint and process value, can be observed on graphs updated every 100ms or on displays.
It was decided to carry out the identification of the control system using the engineering method. Using the program described in section 4, the system’s step response was obtained. It was determined that the system is astatic. It was decided to pe rform the identification of the system using two methods. The first one was to find static gain, system delay, and time constant and approximate using (2).
It was decided to carry out the identification of the control system using the engineering method. Using the program described in section 4, the system’s step response was obtained. It was determined that the system is astatic. It was decided to perform the identification of the system using two methods. The first one was to find static gain, system delay, and time constant and approximate using (2).
For example, assuming T=1, a gain K=0.0385 of and delay of T0 ~ 0.03 were obtained for the motor of the first axis. Due to the very small delay value, it was decided to round the e -sT0 ) to 1 to simplify the model. The second method was to use the tfest function available in the Matlab environment. The result of using the function was a first order system with free coefficient close to zero which was ignored. After ignoring the free coefficient both transfer functions were similar. From this point, dynamics received from tfest function were used. Comparison of the real response with model’s response is shown in Figure 6.
The outer control loop uses a proportional controller. The gain of the controller was chosen experimentally. For this purpose a small value of gain such as 0.1 was set and was slowly increased until a satisfactory dynamic of the system without any overshoot was obtained. Values of the feed-forward were set like in [8]; however, it was determined that the gain value of the acceleration was too great and must have been significantly decreased in the real device. Finally, it was found that the object exhibits the best dynamic properties with a proportional gain of 1.5, velocity feedforward gain of 0.6, and acceleration feed-forward gain of 0.2. In addition, it has been shown that the axles transferring the largest moments (2 and 3) suffered very badly from the presence of acceleration’s feed-forward gain and in their case the acceleration gain was set to 0. The final response of the first axis is shown in Figure 8.
A PI controller was selected for the internal control loop. Tuning of the controller gains was carried out using the engineering method [9,10]. At the beginning, the proportional gain was increased to obtain satisfactory system stability, i.e. as fast as possible response of the system with low overshoot. After the appropriate value had been selected, it was reduced by half, and then the integrator’s gain was increased until system’s response was quick enough. Finally, the gain values were selected from the range of and . The dynamics of the internal loop of axis 1 is shown in Figure 7.
The research has shown that the robot’s movement is stable and there is no overshoot in position. The machine’s capacity was experimentally determined to be about 600 grams; however, the biggest limitation in capacity is the material that most of the manipulator is made of. In order to increase capacity, parts should be printed with more infill or should be redesigned. In addition, during tests of control algorithms it was shown that safety measures were working properly. A potential path for project development is to add a dedicated master device such as PC with Robot Operating System (ROS).
Mateusz Pająk – Gdańsk University of Technology, Faculty of Electrical and Control Engineering, Poland, Email: mpajak.main@gmail.com
Marcin Racław – Gdańsk University of Technology, Faculty of Electrical and Control Engineering, Poland, Email: mar.97.rac@gmail.com.
Robert Piotrowski* – Gdańsk University of Technology, Faculty of Electrical and Control Engineering, Poland, Email: robert.piotrowski@pg.edu.pl.
*Corresponding author
[1] L. Nocks, The Robot: The Life Story of a Technology, Johns Hopkins University Press, 2008, pp. 67–71.
[2] T. Sham, Autodesk Inventor Professional 2019 for Designers, 19th ed., CADCIM Technologies, 2018.
[3] L. Ball, “Make your own PC Boards,” Electronics Now, vol. 68, , 1997, pp. 48-51.
[4] Š. Kozák, “State-of-the-art in control engineering,” Journal of Electrical Systems and Information Technology, vol. 1, no. 1, 2014, pp. 7–8.
[5] M. L. Minsky, Finite-state Machines in Computation: Finite and infinite machines, Prentice Hall, 1967, pp. 11–31.
[6] M. Malek, P. Makys, M. Stulrajter, “Feedforward Control of Electrical Drives – Rules and Limits,” Power Engineering and Electrical Engineering, vol. 1, no. 1, 2011, pp. 35-42.
[7] A. Baehr, P. Mutschler, “Comparison of Speed Acquisition Methods based on Sinusoidal Encoder Signals,” J. Electr. Eng., vol. 2, no. 1, 2002, pp. 35–42.
[8] G. Ellis, “Feed-forward in position-velocity loops,” 20 Minute tune-up, Sept. 2000.
[9] J. G. Ziegler, N. B. Nichols, “Optimum Settings for Automatic Controllers,” ASME Trans., vol. 64, 1942, pp. 759–68.
[10] Ch. Grimholt, S. Skogestad, “Optimal PI and PID control of first-order plus delay processes and evaluation of the original and improved SIMC rules,” Journal of Process Control, vol. 70, 2018, pp. 36–46.
Submitted: 14th September 2021, Accepted 24th February 2022
Igor Korobiichuk, Serhii Hrybkov, Olga Seidykh, Volodymyr Ovcharuk, Andrii OvcharukDOI: 10.14313/JAMRIS/1-2022/6
Abstract:
This developed modified ant colony algorithm includes an additional improvement with local optimization methods, which reduces the time required to find a solution to the problem of optimization of combinatorial order sequence planning in a food enterprise. The planning problem requires consideration of a number of partial criteria, constraints, and an evaluation function to determine the effectiveness of the established version of the order fulfillment plan. The partial criteria used are: terms of storage of raw materials and finished products, possibilities of occurrence and processing of substandard products, terms of manufacturing orders, peculiarities of fulfillment of each individual order, peculiarities of use of technological equipment, expenses for storage and transportation of manufactured products to the end consumer, etc. The solution of such a problem is impossible using traditional methods. The proposed algorithm allows users to build and reconfigure plans, while reducing the time to find the optimum by almost 20% compared to other versions of algorithms.
Keywords: order fulfillment planning, modified ant colony algorithm, efficiency of the algorithms, optimization, food industry.
The food industry is one of the most strategic industries in any country, because it ensures the nation’s food independence. The food industry market is characterized by very high competition, in particular between domestic and foreign producers. In the face of fierce competition, food industry enterprises, which are trying to work on long-term contracts, face the acute task of keeping consumers, which is possible only by making quality products at a reasonable price and providing services for a short period of time. This requires the purchase and storage of raw materials, which have a limited period of use, to make products in compliance with all necessary technological requirements, and to ensure storage of manufactured products. Under these conditions, enterprise management must ensure flexible and responsive production with maximum consideration of external and internal factors and constraints in production planning and operational decision-making [1].
Most technological and production processes in the food industry are difficult to describe quantitatively, which, together with the multi-criteria nature of planning and control tasks, complicates the use of traditional deterministic mathematical methods to make appropriate decisions [2, 3]. This necessitates the use of new intelligent methods for solving management problems based on operational information from specialist experts regarding the prioritization of any alternatives in the decision-making process.
It is important to note that the industry problem of improving the efficiency of food enterprises requires a comprehensive solution, which includes a set of problem-oriented models, methods and algorithms, management technologies and appropriate information and software [4-6].
It should also be noted that very often, the process of planning the fulfillment of orders for the manufacture of products in food enterprises takes place under conditions of uncertainty and risk.
Estimation of efficiency of the established version of the plan requires consideration of external and internal influences.
For the criteria, which are estimated in monetary units, the convolution with the prioritization of each of them for each individual case is applied [7].
The total estimated function will be represented by the additive convolution of all criteria (1):
FF F 01 1 2
7 max, (1) where lg is the criterion importance ratio lg ∈ (0,1), Slg = 1;
F1 is the total maximum profit from fulfillment of orders for production for a given planning period;
F2 is the total amount of penalties for late fulfillment of the order for a given planning period;
F3 is the total amount of costs when fulfilling the order for a given planning period;
F4 is the total costs of maintenance and service of equipment not used in the given planning period;
F5 is the total costs for processing and disposal of substandard products or production waste in the fulfillment of all orders for a given planning period;
F6 is the total costs of storage and delivery of the end product to the final consumer for a given planning period;
F7 is the total storage costs of raw materials and supplies in the enterprise for a given planning period.
In addition, it is necessary to ensure the fulfillment of each order to a given moment of shipment, and to minimize downtime of the main equipment.
Most often, a manager should form an order fulfillment plan, taking into account all the operations of the technological process in the manufacture of products, from the supply of raw materials to the delivery of finished products to the end consumer. Depending on the situation and the type of enterprise, the manager can adjust the necessary set of partial criteria, as well as take into account the necessary features of the parameters of order fulfillment. The planning task can also arise in case of emergency situations, which require reconfiguration of the existing order fulfillment plan in order to minimize losses in case of downtime or repair of certain technological equipment. Such a problem is a complex combinatorial multi-criteria optimization problem. To solve such problems, it is reasonable to use modified heuristic and metaheuristic algorithms [7-9].
Thus, the actual scientific and applied problem is the development and use of modified algorithms and methods to ensure reduction of time for decision-making, reducing the impact of the human factor and reducing costs and time to fulfill orders for the manufacture of products by the food enterprise.
In a 2020 paper by Hrybkov et al., the information technology for solving the problem of planning the fulfillment of orders for manufacturing products in food enterprises in conditions of uncertainty and risk is proposed [7]. The information technology for solving the problem of order fulfillment planning is based on the method of data mining, modified multi-agent and genetic algorithms. A combined algorithm for solving the planning problem was developed to take into account the peculiarities of the subject domain. The paper does not consider other modifications of multi-agent algorithms, nor does it investigate modifications of the ant colony algorithm.
Another study by Hrybkov et al. and Kharkianen et al., the authors proposed a mathematical model of the problem of planning the fulfillment of contracts, and suggested the use of a modified ant colony algorithm [8, 9]. However, the mathematical model and the solution were directed to non-food industry enterprises.
In a paper by Senthilkumar et al., the authors proposed a hybrid planning algorithm based on the particle swarm algorithm and the ant colony algorithm. The proposed algorithm does not take into account the specifics of the food enterprise [10].
A 2013 paper aimed to solve the planning problem using the bee colony algorithm, and the local search is based on a greedy constructive-destructive procedure [11]. This approach cannot be applied when it is necessary to take into account the priorities of partial criteria.
The mathematical model given in a paper by LIn does not take into account all of the partial efficiency criteria for planning the fulfillment of orders, and
does not take into account the supply of the final product and its storage [12].
Another study considered the application of the ant colony algorithm to solve the problem of production planning in metallurgical plants, but this approach requires adaptation for food enterprises [13].
Another paper consolidated the approaches to production planning of various products [14]. The paper highlights the factors affecting the solution of the planning problem using classical optimization methods, but is not devoted to planning in the enterprises of the food industry.
A 2014 paper by Pintea is devoted to the application of solving planning problems using ant colony algorithms, but does not consider modifications of the ant colony algorithm [15].
Having analyzed the literature reviewed above, we may argue for the feasibility of creating and using a modified ant colony algorithm to solve the problem of planning the fulfillment of orders for the manufacture and delivery of products in food enterprises in a given time to minimize costs.
The main management task, which requires considering the task and constraints of all levels, is the operational adjustment of the production plan to fulfill orders. As a rule, the solution of such a task was reduced by decomposition to the required level. In this case, only the necessary criteria and functions with constraints were taken into account, but the connection of all impact criteria was lost.
The production plan is optimal if its fulfillment provides the maximum profit for a given period of time. The optimal plan of order fulfillment does not violate the overall strategic plan of the enterprise, minimizes variable costs, and allows the maximum use of production and technological equipment.
This problem belongs to the class of hierarchical multi-criteria optimization problems, including various partial criteria, which together influence the choice of the optimal solution and the use of which is determined by specific conditions [7-9].
Depending on the social and economic situation, as well as the characteristics of the manager responsible for drawing up an operational and calendar plan for order fulfillment, the problem can be solved in different ways [7, 8]:
• all the criteria are considered and ranked in the evaluation of the effectiveness of the operational plan of order fulfillment, or
• the task is simplified to the selection of certain partial criteria.
The complexity of solving such a problem increases with the number of orders, as well as with the stages of different execution options at different technological links of production.
The task can be simplified only at enterprises where the technological process of product manufacturing takes place at one automated technological complex having a continuous conveyor production cycle. An example would be pasta production, where a certain type of product is manufactured on a single automated production line.
It should be noted that some of the equipment used in domestic food enterprises requires additional adjustment efforts when introducing new types of products. In that case, the cost of adjusting and setting up the process equipment according to each product manufacturing process is added.
We propose a modified “ant colony” algorithm to solve the problem of order fulfillment planning in a food enterprise.
The “ant colony” algorithm is based on the principle of collective intelligence as exemplified by their behavior in finding optimal routes for finding food. In the task of making an operational plan for order fulfillment, the best plan, which is optimal according to the given criteria and constraints, acts as a food. Each version of the schedule corresponds to one ant, performing during the algorithm the construction of its own route, which corresponds to the sequence of order fulfillment. Among the obtained variants of alternative solutions, the best options are selected according to the value of the target function or given criteria. The information obtained is accumulated and used by the ants in the following iterations. Each ant performs its actions according to the rules of a probabilistic algorithm, and uses statistical information when choosing the next step, which reflects the previous history of the collective search, not only based on the target function [9, 13].
First, all orders are divided according to the possibility of their fulfillment on certain equipment, and operational planning for certain equipment and orders begins. A multilayer graph is taken as the basis, where each of the layers corresponds to a certain level of task decomposition.
The first level has representations of the order level, where the node denotes the order number; the edges have directions reflecting the sequence of order fulfillment, namely, the transition from one order to another. The start time of the next order is determined based on the completion of the first stage of the current order according to the technological path of production [9, 13].
The transition from the a and b vertices of the graph determines the time that must be spent to pass the technological operations of manufacturing products by order a without downtime and overlapping operations in the fulfillment of order b [8, 9, 13].
The second level has detailing, which displays all possible options for the fulfillment of orders received from the customer in the form of a graph, where we denote an edge — the department or technological line l, on which a certain technological operation j can be performed.
The node of the graph is the intermediate state in which the semi-finished product is at the transition between technological operations. The transition between the nodes of the graph determines the time Δtijl, required to pass the entire batch of semi-finished product for the implementation of the corresponding
i technological operation of the j step on the l equipment [9, 13].
The third level of decomposition reflects the ordering sequence graph for each l equipment.
The application of the modified “ant colony” algorithm determines, depending on the production and conditions that are determined by the decision maker, the search at different levels. In the simplest variant only the first level is used, which implies the use of only a complete automated line that performs all the technological operations for production [9, 13].
Due to the repeated iterative search, at each iteration, the best option among the current ones is selected and compared with the global value. There is also a control of the best sequence of order fulfillment for a given number of ants. In this case, each ant corresponds to one of the variants of the schedule of order fulfillment, which means that, when performing one iteration of the algorithm, it is this ant that forms it. Positive feedback is implemented as an imitation of the ant behavior, that is, “leave traces – move by traces.” The more traces are left on the path, the more ants will move along it [8, 9, 13, 17].
New traces appear on the path (corresponding to the order fulfillment sequence), attracting additional ants. The positive feedback is realized by the following stochastic rule: the probability of including an edge of the graph in the ant’s path is proportional to the number of pheromones on it. This rule ensures the randomness of the formation of new variants. The number of pheromones deposited by an ant on an edge of the graph is inversely proportional to the efficiency of the schedule. The more efficient the schedule is, the more pheromones will be deposited on the corresponding edges of the graph, and more ants will use them when synthesizing their routes. The pheromones deposited on the edges allow for efficient routes (execution sequences) to be stored in the ant’s global memory. Such routes can be improved in the subsequent iterations of the algorithm [8, 9, 18-20].
Using only positive feedback leads to prematurely finding a convergent solution when all ants move along the same suboptimal route. To avoid this, negative feedback (pheromone evaporation) is used. The evaporation time should not be too long, because the previous situation with finding a suboptimal route may occur. A very short evaporation time will lead to a loss of colony memory, thus not ensuring cooperative behavior of the ants.
Cooperativity is important for the algorithm: multiple identical ants simultaneously explore different points in the solution space and communicate their experiences through changes in the cells of the ant’s global memory. For each ant, the transition from node a to node b depends on three components: ant memory, visibility, and virtual pheromone trace. For the second level, a list of operations to be performed emerges [2, 3, 7, 10-14].
An ant’s memory is a list of orders already included in execution that should not be considered when scheduling. Using this list, an ant is guaranteed not to choose the same order. At each step, the memory of the
ant grows, and at the beginning of each new iteration of the algorithm the memory becomes empty. Let us designate through the Lta,i the list of orders already included in the schedule of i ant (Lta,i ∈ n, where n is all orders) after the inclusion of the a order, and the list to be included in the schedule is Lna,i (Lna,i ∈ n, where n is all orders). That is Lta,i ∪ Lna,i = n, herewith Lta,i ∩ Lna,i = 0. For the second and third levels of graph decomposition, the list of j stage number from the set of stages is taken into account j ∈ wi for i order, wi is the number of steps required for i order, as well as the l number of equipment from the set of equipment (j ∈ si) for i order, si is the amount of equipment involved to perform all stages in the manufacture of the i order [10, 13, 16].
Visibility is the value inverse of the evaluation of edges of graph a and b, namely ηa,b(ı) = 1/Da,b(ı), where Da,b(ı)t is the value of the graph edge estimation (depending on the level of management, decomposition can be represented in cost or time form) between vertices a and b (the more optimal the value, the more preferable it is to choose this transition) at iteration [8, 9, 13, 16-20].
The virtual pheromone trail on the edge (a, b) reflects the desire to visit vertex b from vertex a based on ant experience. Unlike visibility, the pheromone trail is a more global and dynamic information. It changes after each iteration of the algorithm, reflecting the experience accumulated by ants [16]. The amount of virtual pheromone on edge (a, b) at iteration, which leaves i insect, is denoted by ξa,b(ı,i). In the first iteration ı = 0 of the algorithm operation, the amount of pheromone for each i ant is taken equal to a small positive number 0 < ξ0,b(0,i) <1.
The transition probability of i ant to vertex b from vertex a at the ı iteration is determined by Formula (2) [10, 12-14].
(2) does not change over the course of the algorithm iteration, but in two different ants, the value of transition probability will differ, because they have different list of visited vertices [16-19].
where α is pheromone weight ratio, 0 ≤ α ≤ 1, which determines the relative importance of the mark intensity influence on the choice of the path. At α = 0 the shortest edge (according to the evaluation of the problem – time, cost) will be selected for the transition that corresponds to the greedy algorithm. At α = 1 the edge with the highest level of marks will be selected.
b is the visibility coefficient in route selection
0 ≤ b ≤ 1, which determines the relative importance of the influence of visibility on the choice of the path, when b = 0 only pheromone amplification will work, which will lead to finding a local suboptimal solution: α + b = 1, with the coefficient α determining the greediness of the algorithm, and b being hardiness.
Formula (2) defines only the probability of selection of the next order to include it in the execution schedule, which will correspond to the graph of selection of the next vertex. The value obtained by Formula
After completing the route, each ant deposits on each edge the number of pheromones that are included in its route and meet the schedule, which are calculated by Formula (3). ab i i i
i F Q S if ab Lt if ab Lt , , *, , ,,
1 0 0 , (3)
where a and b are the indices of the pair of nodes uniting the edge the agent passed; Lti(ı) is the formed route at the ı iteration of the i ant; Si(ı) is route length Lti(ı), expressed in time or cost; Q is the adjustable parameter approximating the optimal route, given or calculated by the previous iteration; and ′ F� is the evaluation of the route according to the selected partial criteria or evaluation function.
In the case that the number of pheromones deposited was not excessive, they are updated according to Formula (4), taking into account the pheromone level update coefficient 0 ≤ r ≤ 1, which determines its relative decrease over time.
ab ab ab i
n i *, 11 1 , (4)
where n is the number of ants, which corresponds to the number of orders, and r ∈ [0,1] is the pheromone evaporation coefficient.
To protect against prematurely finding a suboptimal solution, it is proposed to introduce restrictions on the concentration of pheromones on the edges Dξmin ≤ Dξa,b ≤ Dξmax.
The formula for updating the number of pheromones on graph edges turns from expression (3) to Formula (5) [2, 11-14].
ab ab ab best ab best Lt
* *
,, ,, ,
11 1 1 , (5)
where Ltbest(ı) is the best formed route on the ı iteration.
After each iteration of the algorithm, only one ant leaves a trail to choose from: with the best at the current iteration being Ltbest(ı) = Ltı_best(ı), and the best for all the time the algorithm works being Ltbest(ı) = = Ltgl_best(ı) It is reasonable in the first iterations to use Ltı_best(ı), and in the last iterations to use Ltgl_best(ı).
At each iteration, the amount of pheromone on each edge of the graph is adjusted according to Formula (6).
ab
ab ab ab ,
min, min ,min ,max max
, ,
if if ,, max, if ab
. (6)
Instead of the empirical rule of choosing the best ant, it is proposed to use Formula (7) based on the
Cauchy probability distribution law, which allows for a smooth transition from the technology of global search, at the initial steps, to the technology of selecting the best global search for the whole time of the algorithm at the final steps.
1. Selection of the planning period. According to a given period, orders are selected, which should be performed during this period on certain equipment.
2. Selection of the evaluation function and partial selection criteria for solving the problem.
3. Formation of a multilayer graph, describing each of the layers responsible for a particular level of decomposition of the problem.
where ımax is the selected number of generations of colony life.
Based on a comparison of their values, either the best ant for the entire time of the algorithm Ltgl_best(ı) or the best at the current iteration Ltı_best(ı) is selected. The diversification operations are used if no improvement in the global solution has occurred for every ımax/2 or ımax/10 iteration.
During the diversification operation, the amount of pheromone on all edges is re-initialized. The maximum pheromone value is determined using Formula (8) by assigning them a value inverse to the evaporation coefficient and multiplied by an estimate of the global optimum path [16-20].
4. Initialization of algorithm parameters α, b, r and Q
5. Selecting rules for calculating visibility parameters ηa,b and pheromone concentration ξa,b.
6. All orders are sorted by time parameter dti, for which the products of the i order must be produced. As a result we get a sorted order list Lna,i (Lna,i ∈ n, where N is all orders).
The minimum value of the pheromone concentration on the rib is calculated by Formula (9). = max
where n is the number of ants, which corresponds to the number of orders.
After each finding of the best solution the values of ξmax and ξmin should be listed by formulas (8) and (9), respectively [16].
When constructing a route, the selection of the transition from the a node is carried out on the basis of the rule (8), but for the selection of the next node, the entire list of nodes Lna,i (Lna,i ∈ n, where n is all orders), that i ant still has to visit is not used; only a list of the closest peaks, nlist, is used. The list nlist is a matrix of size n×n’, where n is the number of vertices in the graph, and n’ is the number of closest vertices.
Each i row of this matrix contains numbers of the nearest vertices ordered by the distance from a vertex. Since nlist is calculated and formed by the known distance matrix D at the beginning of the algorithm, the construction of the route at the stage of selecting the next vertex is significantly accelerated. If all vertices of the nlist set are exhausted, the selection of vertex b will be determined by taking into account the distance and level of edge pheromones according to Formula (10) [16-20].
bi bLn ab ab ai max,,,
So, considering all the modifications, the solution algorithm will appear as follows [7, 8].
7. We perform the construction of the initial routes simultaneously in the forward and reverse directions. Construction of the forward direction implies the sequence obtained after sorting. In fact, we take as such a sequence the sorted list of orders Lna,i (Lna,i ∈ n, where N is all orders). And the construction of the reverse direction implies formation from the last to the first order. When building in reverse order, an additional condition for selecting the next order is the time dti, for which it is necessary to produce products under the i order. After that we carry out the reversal. Thus, we obtain the value of Ltgl_best(ı) global and Ltı_best(ı) local (at the current iteration) optimum.
8. We form a population of ants, each of which on the first vertex corresponds to a certain order, which is formed using a random number generator. In fact, each ant at the beginning of its path must take a path on the graph corresponding to a certain order.
9. Execute the cycle on the life time of the colony ımax ⇐ 1..ı .
9.1. Performing the cycle on all ants i ⇐ 1..n
9.1.1. Construct a route for each new ant using formula (2), and calculate the length of Ltı(ı).
9.1.2. Apply a local 2-opt and/or 3-opt search to the route.
9.1.3. Carry out isolation of pheromones according to (5).
9.2. We evaluate each of the routes and compare them to the local and global optimal value to update the others.
9.3. Perform pheromone updates on all edges of graph (4).
10. Deriving the best local and global optimum.
11. Completion of the algorithm.
After completing the algorithm, we obtain the optimal plan for order fulfillment in the form of local and global optimum route.
As can be seen from the above algorithm, after the route has been constructed, an additional improvement is carried out over the obtained solution by one of the local optimization methods, namely by 2-opt and 3-opt methods [21, 22].
The modified “ant colony” algorithm in the form of a block diagram is shown in Fig. 1.
At the beginning of the “ant colony” algorithm, it is necessary to set whether one of the 2-opt or 3-opt methods will be automatically selected. One must use both methods and choose the best.
Local optimization by the 2-opt method (Fig. 2) consists of selecting two pairs of non-adjacent schedule elements and swapping them with each other, then evaluating and leaving the best option. If after swapping we get the best option, we leave it [22], which is shown in Fig. 2.
This improves the found route at each iteration, which will reduce the total number of iterations of the algorithm, as well as the total running time of the algorithm.
The modified ant colony algorithm was tested at various enterprises of the food industry, and its performance was compared to the following methods: The “bee colony” algorithm [21]; the “chaotic bat” algorithm [23-26];
the “bat algorithm based on the Levy flight search strategy” [24-26]; the “bat algorithm based on reduction factor” [25-26]; the “genetic algorithm” [27-28]; the “pack of wolves” algorithm [29-32]; the “ant colony algorithm using elite ants” [8]; the modified “ant colony algorithm.”
To test the modified algorithm, the corresponding software modules were created implementing the modified algorithm in the Java language. Most tests were performed on the Intel Core i5-4690K (3.5GHz) / RAM 16GB / NDD 1TB.
The application of local optimization by the 2-opt method should be carried out for each variant of the plan, which in the ant algorithm corresponds to the route of the ant.
The plan variant searches for two non-contiguous sequences, the exchange of which will provide the maximum effect according to the evaluation function or set of criteria. An example application of the 2-opt method is shown in Fig. 3. Option
The 3-opt method (Fig. 4) uses the approach to the 2-opt method, but selects 3 pairs of non-adjacent schedule elements to replace them with each other [22].
In order to obtain reliable information when applying the considered algorithms, data on order fulfillment plans for different previous periods of enterprises activity were selected. 25, 50, and 100 orders were randomly selected for different periods of time. At the beginning of the algorithms, the orders were placed in the same order as they came into the enterprise.
To compare the algorithms, each algorithm was first matched to the selected sample, and then the results of the algorithms were compared. The efficiency of the algorithms was evaluated on the basis of the following indicators:
time to find the optimal schedule;
– the effectiveness of the found plan in monetary terms as the difference between the estimates by the target function of the calculated plan and the actual plan;
– the efficiency of order fulfillment time reduction (calculated as the difference between the calculated plan and the actual plan in terms of execution time).
The efficiency of the found plan is calculated by Formula (11), and the efficiency percentage is calculated by Formula (12).
where ffact is the evaluation of the actual plan by the target function; fopt is the evaluation of the established plan by the target function.
Tables 1 shows a comparison of the use of algorithms for 100 orders.
From the data shown in Table 1, we can see that the search results for the algorithms are different. The best results were shown by the modified “ant colony” algorithm. Its search speed is almost 20% faster, and in comparison with its unmodified versions, is faster by 4.45%.
The proposed modified algorithm is based on the combination of a metaheuristic algorithm with a heuristic one. Most other modifications try to avoid this type of modification to prevent an increase in algorithm complexity [33]. The main advantage of the proposed modified algorithm lies in reducing the time to find a solution to the problem, which is very relevant in real production conditions when critical situations arise.
The main disadvantages of the proposed modification of the algorithm include that its application is possible using large amounts of data, but without the use of special means of storage, such as a database management system, it is impossible, because it is necessary to constantly save a multitude of additional calculations.
Further advancement of research and development is aimed at the inclusion and use of the proposed algorithm in the decision support system for the management of the food enterprise, as well as in the information technology presented in the paper by Hrybkov et al [7].
The developed modified ant colony algorithm allows for the reconfiguration of plans, while reducing the time to find the optimum by almost 20% compared to other versions of the algorithms. When unmodified versions are used, the time to find the optimum increases by 4.5%. Modification of the “ant colony” algorithm reduces the time by using local optimization approaches.
According to the results of calculations for 25 orders, it was found that all algorithms found the same plan, which is 2% more efficient than the actual plan, and its total execution time was 2 hours less than the actual plan. The search speed of our algorithm compared to the other algorithms was 2.56% faster.
According to the results of calculations for 50 orders, the search results for the algorithms were found to be different. The plan efficiencies for the “bee colony,” “chaotic bat,” “bat based on Levy flight search strategy,” and “bat based on reduction factor” algorithms were almost the same and minimal compared to other algorithms. The best result was shown by our modified “ant colony” algorithm. Its search speed compared to the first 4 methods was 9.25% faster, and compared to its unmodified versions, it was 1.8% faster.
The greatest effect of the use of the proposed modified algorithm is achieved in the decision support systems for planning the sequence of order fullfillment, which will allow for: quick formation of operational and calendar plan of order fulfillment with cost minimization and profit maximization; operational adjustment of the existing calendar plan of orders, allowing to react to the order in real time and ensure optimal use of technological equipment; efficiency in the use of raw materials and supplies, as well as minimizing storage costs; rapid response to negative and contingency situations by making appropriate changes to the current order fulfillment plan; clear distribution of all tasks for each order between production departments, which allows to take into account the sequence of fulfillment and necessary resources with time constraints; and optimal use of production capacities.
The use of the proposed modified algorithm is possible in various tasks of work sequences and related planning.
Igor Korobiichuk* – ŁUKASIEWICZ Research Network – Industrial Research Institute for Automation and Measurements PIAP, Jerozolimskie 202, 02-486 Warsaw, Poland, e-mail: igor.korobiichuk@piap.lukasiewicz.gov.pl.
Hrybkov Serhii – Ukrainian State University of Food Technologies, 68 Volodymyrska Street, 01033, Kyiv, Ukraine, e-mail: sergio_nuft@nuft.edu.ua
Olga Seidykh – Ukrainian State University of Food Technologies, 68 Volodymyrska Street, 01033, Kyiv, Ukraine, e-mail: olgased@ukr.net.
Volodymyr Ovcharuk – Ukrainian State University of Food Technologies, 68 Volodymyrska Street, 01033, Kyiv, Ukraine, e-mail: ovcharuk2004@ukr.net
Andrii Ovcharuk – Ukrainian State University of Food Technologies, 68 Volodymyrska Street, 01033, Kyiv, Ukraine, e-mail: ovch2011@gmail.com
* Corresponding author
[1] A. Oliinyk, S. Skrupsky, S. Subbotin, I. Korobiichuk, “Parallel Method of Production Rules Extraction Based on Computational Intelligence”, Automatic Control and Computer Sciences, 2017, Vol. 51, No. 4, 2017, pp. 215–223. 10.3103/S0146411617040058
[2] I. Korobiichuk, A. Ladaniuk, V. Ivashchuk, “Features of Control for Multi-assortment Technological Process”, n: Szewczyk R., Krejsa J., Nowicki M., Ostaszewska-Liżewska A. (eds) Mechatronics 2019: Recent Advances Towards Industry 4.0. MECHATRONICS 2019. Advances in Intelligent Systems and Computing, vol 1044. Springer, Cham, 2020, pp. 214-221. 10.1007/978-3-030-299934_27
[3] I. Korobiichuk, A. Ladanyuk, N. Zaiets, L. Vlasenko, “Modern development technologies and investigation of food production technological complex automated systems”. In: ACM International Conference Proceeding Series. 2nd International Conference on Mechatronics Systems and Control Engineering ICMSCE 2018, February 21-23, 2018, Amsterdam, Netherlands. 52-57, 10.1145/3185066.3185075
[4] V. Tregub, I. Korobiichuk, O. Klymenko, A. Byrchenko, K. Rzeplińska-Rykała, “Neural Network Control Systems for Objects of Periodic Action with Non-linear Time Programs”. In: Szewczyk R., Zieliński C., Kaliczyńska M. (eds) Automation 2019. AUTOMATION 2019. Advances in Intelligent Systems and Computing, vol 920. Springer, Cham, pp. 155-164 (2020), 10.1007/978-3-030-13273-6_16
[5] I. Korobiichuk, A. Lobok, B. Goncharenko, N. Savitska, M. Sych, L. Vihrova, “The Problem of the Optimal Strategy of Minimax Control by Objects with Distributed Parameters”. In: Szewczyk R., Zieliński C., Kaliczyńska M. (eds) Automation 2019. AUTOMATION 2019. Advances in Intelligent Systems and Computing, vol 920. Springer, Cham, 2020, pp. 77-85. 10.1007/978-3-03013273-68
[6] I. Korobiichuk, N. Lutskaya, A. Ladanyuk, S. Naku, M. Kachniarz, M. Nowicki, R. Szewczyk, “Synthesis of Optimal Robust Regulator for Food Processing Facilities”. In: Advances in Intelligent Systems and Computing, Vol. 550, ICA 2017: Automation 2017, pp. 58-66. 10.1007/978-3-31954042-9_5
[7] S. Hrybkov, O. Kharkianen, V. Ovcharuk, I. Ovcharuk, “Development of information technology for planning order fulfillment at a food enterprise”, Eastern-European Journal of Enterprise Technologies, vol. 1/3, no. 103, 2020, pp. 62–73. 10.15587/1729-4061.2020.195455
[8] S. Hrybkov, V. Lytvynov, H. Oliinyk, “Web-oriented decision support system for planning agreements execution”, Eastern-European Journal of Enterprise Technologies, vol. 3/2, no. 99, 2018, pp. 13–24. 10.15587/1729-4061.2018.132604
[9] O. Kharkianen, O. Myakshylo, S. Hrybkov, M. Kostikov, “Development of information technology for supporting the process of adjustment of the food enterprise assortment”, Eastern-European Journal of Enterprise Technologies, vol. 1/3, no. 91, 2018, pp. 77–87. 10.15587/17294061.2018.123383
[10] K. Senthilkumar, V. Selladurai, K. Raja, V. Thirunavukkarasu, “A Hybrid Algorithm Based on PSO and ACO Approach for Solving Combinatorial Fuzzy Unrelated Parallel Machine Scheduling Problem”, European Journal of Scientific Research, vol. 2, no. 64, 2011, pp. 293-313.
[11] F. Rodriguez, M. Lozano, C. García-Martínez, J. González-Barrera, “An artificial bee colony algorithm for the maximally diverse grouping problem”, Information Sciences, no. 230, 2013, pp. 183–196. 10.1016/j.ins.2012.12.020
[12] Yang-Kuei Lin, “Scheduling efficiency on correlated parallel machine scheduling problems”, Operational Research, vol. 18, 2018, pp. 603–624. 10.1007/s12351-017-0355-0
[13] T. Zheldak, “Application of the method of modeling the ant colony to the solution of combinatorial problems scheduling the execution of order by metallurgical plants”. Mathematical Machines and Systems, vol. 4, 2013, pp. 95–106.
[14] G. Georgiadis, A. Elekidis, M. Georgiadis, “Optimization-Based Scheduling for the Process Industries: From Theory to Real-Life Industrial Applications”, Processes, vol. 7, 2019, pp. 1–35. 10.3390/pr7070438.
[15] Pintea C-M., Advances in Bio-inspired Computing for Combinatorial Optimization Problem, Springer: Berlin, 2014, p. 188. 10.1007/978-3-64240179-4.
[16] S. Talatahari, “Optimum Performance-Based Seismic Design of Frames Using Metaheuristic Optimization Algorithms”, Metaheuristic Applications in Structures and Infrastructures, 2013, pp. 419–437. 10.1016/B978-0-12-3983640.00017-6
[17]. K. Jha. Manoj, “Metaheuristic Applications in Highway and Rail Infrastructure Planning and Design: Implications to Energy and Environmental Sustainability”, Metaheuristics in Water, Geotechnical and Transport Engineering, 2013, pp. 365384. 10.1016/B978-0-12-398296-4.00016-7
[18] V. Kureichik, A. Kazharov, “Using Fuzzy Logic Controller in Ant Colony Optimization”, Artificial Intelligence Perspectives and Applications, 2015, pp. 151–158. 10.1007/978-3-319-18476-0_16
[19] V. Kureichik, A. Kazharov, “The Development of the Ant Algorithm for Solving the Vehicle Routing Problems”, World Applied Sciences Journal, vol. 26, no. 1, 2013, pp. 114–121. 10.5829/idosi. wasj.2013.26.01.13468
[20] T. Stutzle, H. Hoos, “Max-min ant system”, Future Generation Computer Systems, vol. 8, no. 16, 2000, pp. 889–914.
[21] K. Rocki, R. Suda, “Accelerating 2-opt and 3-opt Local Search Using GPU in the Travelling Salesman Problem”, IEEE/ACM International Symposium on Cluster, Cloud and Grid Computing, 2012, pp. 705–706. 10.1109/CCGrid.2012.133
[22] F. Rodriguez, M. Lozano, C. García-Martínez, J. González-Barrera, “An artificial bee colony algorithm for the maximally diverse grouping problem”, Information Sciences, 230, 2013, pp. 183–196. 10.1016/j.ins.2012.12.020
[23] I. Fister, D. Fister, X.-S. Yang, “A hybrid bat algorithm”, Electrotechnical Review, vol. 1–2, no. 80, 2013, pp. 1–7.
[24] G. Wang, L. Guo, “A Novel hybrid bat algorithm with harmony search for global numerical optimization”. Hindawi Publishing Corporation, 2013, pp. 1–21. 10.1155/2013/696491
[25] X.-S. Yang, X. He, “Bat algorithm: literature review and applications”, International Journal
of Bio-Inspired Computation, vol. 5, no. 3, 2013, pp. 141–149. 10.1504/IJBIC.2013.055093
[26] X.-S. Yang, H. Gandomi, “Chaotic bat algorithm”, Journal of Computational Science, vol. 5, no. 2, 2014, pp. 224–232. 10.1016/j.jocs.2013.10.002
[27] Y. Zhang, S. Balochian, P. Agarwal, V. Bhatnagar, O. Housheya, “Artificial Intelligence and Its Applications”, Mathematical Problems in Engineering, vol. 2014, pp. 1–10. 10.1155/2014/840491
[28] Y. Virginia, A. Analía, “Deterministic crowding evolutionary algorithm to form learning teams in a collaborative learning context”, Expert System Appl., vol. 10, no. 39, 2012, pp. 8584-8592. 10.1016/j.eswa.2012.01.195
[29] Y. Jie, K. Nawwaf, P. Grogono, “Bi-objective multi population genetic algorithm for multimodal function optimization”, IEEE Trans. Evol. Comput., vol. 1, no. 14, 2010, pp. 80–102. 10.1109/ TEVC.2009.2017517
[30] W. Hu-Sheng, Z. Feng-Ming, “Wolf Pack Algorithm for Unconstrained Global Optimization”, Mathematical Problems in Engineering, vol. 2014, pp. 1–17. 10.1155/2014/465082
[31] S. Mirjalili, A. Lewis, “Grey Wolf Optimizer”, Advances in Engineering Software, vol. 69, 2014, pp. 46–61. DOI: 10.1016/j.advengsoft.2013.12.007
[32] A. Madadi, M. Motlagh, “Optimal Control of DC motor using Grey Wolf Optimizer Algorithm”, Technical Journal of Engineering and Applied Science, vol. 4, no. 4, 2014, pp. 373–379.
[33] М. Gendereau, J. I. Potvin, “Handbook of Metaheuristic”, 3rd ed., Springer International Publishing: Cham, 2019, p. 604. 10.1007/978-3-31991086-4
Submitted: 8th December 2021; accepted 6th September 20222
Jyoti Kukad, Swapnil Soner, Sagar PandyaDOI: 10.14313/JAMRIS/1-2022/7
Abstract
Nowadays, violence has a major impact in society. Violence metrics increasing very rapidly reveal a very alarming situation. Many violent events go unnoticed. Over the last few years, autonomous vehicles have been used to observe and recognize abnormalities in human behavior and to classify them as crimes or not. Detecting crime on live streams requires classifying an event as a crime or not a crime and generating alerts to designated authorities, who can in turn take the required actions and assess the security of the city. There is currently a need for this kind of effective techniques for live video stream processing in computer vision. There are many techniques that can be used, but Long Short-Term Memory (LSTM) networks and OpenCV provide the most accurate prediction for this task. OpenCV is used for the task of object detection in computer vision, which will take the input from either a drone or any autonomous vehicle. LSTM is used to classify any event or behavior as a crime or not. This live stream is also encrypted using the Elliptic curve algorithm for more security of data against any manipulation. Through its ability to sense its surroundings, an autonomous vehicle is able to operate itself and execute critical activities without the need for human interaction. Much crowd-based crimes like mob lynching and individual crimes like murder, burglary, and terrorism can be protected against with advanced deep learning-based Anamoly detection techniques. With this proposed system, object detection is possible with approximately 90% accuracy. After analyzing all the data, it is sent to the nearest concern department to provide the remedial approach or protect from any crime. This system helps to enhance surveillance and decrease the crime rate in society.
Key words: Autonomous vehicle, LSTM, Open CV, ECC, Crime and Streaming 1.
The advancement of technology is a boon to society, but a growing population equipped with the stateof-the-art technology at hand has led to an unprecedented rise of criminality. In the past, hotspots of
crime had cameras installed, and manual monitoring of those locations was completed by observing multiple screens. These types of systems work well for less crowded areas. With the introduction of data mining and deep learning techniques, autonomous violence detection techniques are widely used for video surveillance [1]. A lot of work has been carried out in recent years on recognition of human actions using vision and acoustic technologies. These technologies are used to monitor human behavior.
Violence in society is the biggest issue and the most important goal is to detect it. The rapid growth of violence in society is crucial for everyone. As per the above-mentioned report, many cases are not noticed, and the accused are not identified by the authorities. Society needs more digitization of the system wherein video surveillance will be required to detect violence. This system is easy to deploy and not very cost-effective to install. Currently available surveillance systems are sometimes ineffective and insufficient to predict the accurate result. For optimal understanding, a huge amount of video needs to be captured for surveillance. For the correct and accurate measurements, we need a system-trained system [3]. The automation process is a large application to understand when it comes to defining the meaning of crime with different weapons. The proposed kind of system requires the machine-leering method to train the data and predict accurate results. The system works on real-time incidents of violence in any place. Autonomous vehicles are the intelligent key for monitoring and detecting crime.
This system’s goal is to identify anomalies, which are noticed by a well autonomous vehicle attached with a camera. The system anomaly detection is related to the behavior of the public, especially carriable objects identified in a public crowd. Anomaly detection refers to crime detection, including different kinds of violence or detection of weapons such as a gun, sword, or knife. This type of detection is based on cameras which generate an alarm to the authorities. The entire system provides for a safe city and leads to a safer city over time [5]. Live surveillance with this automobile camera is also made secure using an ECC algorithm so that the live stream will not be able to be altered.
Bruno M. Peixoto et al. present a system where violence detection is based on different mechanics based on machine learning [1]. This proposed system breaks into different understood levels of violence (i.e., fights, explosions, blood, and gunshots) and combines all of them for a more detailed understanding of particular scene detection. Systems that explore the depth of different kinds of violence, e.g., gunshots, have more weight than audio features alone, implying the need for a multimodal approach trained on visual and audio features. The machine learning here was based on different DCNNs for detecting violence, focusing on the medieval 2013 VSD dataset. The system deliberated and trained different DCNNs (static and motion-based), each of which was responsible for detecting an object based on a single aspect.
OViF (Oriented Violent Flows) as a reliable feature for violence detection were investigated by Yuan Gao et al [2]. It is based on the concept of motion orientation for detecting changes in motion magnitude. OViF is a better choice for violence detection in non-crowded scenarios. However, for crowded scenarios, this approach fails to generate acceptable results. As such, the paper used a combination of both OViF and ViF (Violent Flows) as feature extraction techniques to detect crowded and uncrowded scenes for better violence detection. The results were generated by using Ada boost and Linear SVM as a multi-classifier way for attaining better classification performance. SVM was selected for its simplicity, effectiveness, and speed. AdaBoost was selected as it was the most efficient boosting algorithm. The two databases used for evaluating the effectiveness were the Hockey Fight Database and the Violent-Flows Database. The performance of OViF was better than ViF on the Hockey Fight Database, but on the ViF database, OViF did not generate satisfying results. The final results on violence detection were generated using ViF+OViF with AdaBoost and SVM. The most challenging task was monitoring crowded events, especially to detect the violence in real time in a timely manner. The proposed system of this model has provided a novel approach for detecting violence in real-time crowded scenes, which is based on flow vector magnitude.
In a paper by Tal Hassner et al, the system collected shot frame sequences and used the violent flow descriptor [3]. After dataset collection, SVM support vector machines based on linear classification classified the out frame as violent or nonviolent. Systems
used video surveillance to test the data and provide the accuracy with effectiveness. This kind of model required a high level of motion and shape analysis of the dataset. Despite its limitations and privacy concerns, video surveillance plays an important role in society in instilling a sense of safety, trust, and security. In a paper by Ding et al., violence detection used the 3D ConvNets without previous information, which is used for supervised learning for model training, and used the backpropagation approach for computing gradients [4]. There are several flaws in this system that need to be addressed in order to increase accuracy. On the other hand, it can learn video features automatically. In uncrowded circumstances, this method employs a CNN-based algorithm to recognize categories if a video contains aberrant human behaviors like falling, loitering, or aggression. It is efficient and precise since it operates directly on the picture pixel. It has the ability to learn video features on its own. On a hockey dataset, the system achieved a 91.00% accuracy rate.
G. Mu et al. presented two techniques for using CNNs for classification: one used them end-to-end, taking raw data as input and producing classification results at the last layer; the other used them in a layer-by-layer fashion [5]. For feature representations, CNNs were effective. Video clips with shots, screams, and heavy metal music received high ratings because of their explicit violent relevance, which is an advantage of adopting this model. This model has an Average Precision (AP) of 0.485 and 0.291 on the MediaEval 2015 dataset on the validation and testing sets for Visual- only + End-to-end CNN based on Audio-only. On numerous visual recognition tasks, such as object recognition, the usefulness of CNN models has been demonstrated by Q. Dai et al [13]. Many applications can benefit from these techniques for detecting violent scenes and predicting emotional effects in videos. Longer-term temporal dynamics are incorporated in this model by layering LSTM on top of the two stream CNN features. This approach is quite successful at detecting violence, but it requires more computing complexity. E. Ditsanthia et al analyzed the behavior of crowds, one of the most active study areas in computer vision [25]. The gathering of people in groups at one place is defined as a crowd. The crowd may be different at different locations. The identification of violence is one of the most difficult challenges in crowd behavior analysis. This algorithm was quite successful at detec-
1 Bruno M. Peixoto [1]
2 Yuan Gao et al. [2]
3 Tal Hassner et al. [3]
4 C. Ding et al. [4]
5 G. Mu et al. [5]
– Breaks into the different understood levels of violence
– DCNNs for detecting violence which focuses on the medieval 2013 VSD dataset
– Used a combination of both OViF and ViF (Violent Flows) as feature extraction techniques
– Ada boost and Linear SVM provided a multi-classifier way for attaining better classification performance
– The approach is based on flow vector magnitude to detect the real-time detection of violence in crowded scenes based on flow vector magnitude
– SVMs (support vector machines) based on linear classification classify the out frame as violent or nonviolent
– Backpropagation approach for computing gradients
– CNN-based algorithm to recognize and categorize
CNNs classification used for
ting violence, but it requires a massive training dataset of violent films. To calculate accuracy, this model was applied to several datasets, with 75.73% accuracy on the Real-Violent Dataset, 88.74% accuracy on the Movie Dataset, and 83.19% accuracy on the Hockey Fight Dataset. F. U. M. Ullah et al. presents a system where the violence is detected automatically; this prompt response is enormously useful, and it may greatly aid the relevant departments [26]. Violence is an aberrant behavior and activity that often involves the use of physical force to harm or kill, whether the victim be a human or an animal; these acts may be detected on a system baked on smart surveillance, which can be utilized to avoid more catastrophic occurrences. Pre-trained lightweights are used in this approach. Mobile Net CNN aids in the reduction of mass processing of ineffective frames. For feature extraction, this system employs 3D CNN. This device employs closed-circuit television, and if violence is detected, the nearest security agency or police station is notified. Unnecessary processing of worthless frames is eliminated by utilizing this method. It also performed better in terms of accuracy. This system necessitates good hardware, and devices with fewer resources may be unable to implement the suggested concept. This model was tested on a variety of datasets, including a violent crowd dataset with 98% accuracy, a movie dataset with 99% accuracy, and a hockey battle dataset with 96% accuracy.
A. Computational intelligence: Computational intelligence is the ability to learn from previous examples and data. It performs in the same way that human beings’ intelligence does. Artificial intelligence (AI) is the overarching discipline that covers anything related to making machines intelligent. Whether the machine is in the field of autonomous vehicles, robotics, home appliances, or daily-use system and software applications, if we apply artificial intelligence on it, it will become smarter and provide results that are easier for users [12]. AI-based systems perform high-level, intelligent functions like communicating in languages, learning, reasoning in the same way the human brain does, and so on. Artificial intelligence learning can be completed through neural networks. A neural network is a net of interconnected computing elements known as neurons. Figure 2 represents a single layer feedforward neural net: here, X is a input layer neuron, Y is the output layer, Yin is the net input calculated at output layer neuron, and w is the weight between neurons and f(Yin)
B. Machine learning: Machine learning can be defined as machines learning by themselves and adapting to changes in the environment in which they work. For example, we made our system learn by providing it with datasets for crime classification. However, if there are test data which are provided for validation, such as footage of a knife used to cut vegetables, and they are not labeled in the dataset, then our system must learn to classify this new data as non-crime. As such, it needs to cover its knowledge of previous examples which were provided for study, hence updating its own intelligence. For machine learning, there are many techniques which work the same as animal or human learning. Machines can be made to learn in two ways: either structure-based or parameter-based. Updating either structures or parameters in the desired way leads to the expected target output. But as updating structure for learning is not always possible, parameter learning is preferred. In our system, we have millions of parameters which get updated for expected performance [11]. One of the major flaws of machine learning is the quantity of data machines require. A small amount of data does not provide enough learning, which leads to poor performance of the ML model. Major applications of machine learning are in recognition tasks such as handwriting recognition, face recognition, etc., or predictions of some event or label, forecasting, etc. All of systems require intelligence. More epochs of learning with enough data continue to enhance the performance of ML models.
C. Deep learning: A major issue with machine learning models is to select the appropriate set of features which are relevant for the desired or expected output of the model. Selecting these features in the training dataset is up to the user. This requires expertise in the domain of implementation; also, it requires a high processing time for the dataset which includes high dimensions, e.g., signals or videos. Here, we use deep learning architectures which extract features from data that are more likely relevant for decision-making. Here, we have one input layer, one output layer, and multiple intermediate or hidden layers of computational elements, i.e., neurons. In Figure 3, X is the input layer, Y is the output layer, and all others are hidden layers; weights between different sets of layers can be different as activation functions [6]. Here, the processing is nonlinear, because the data here requires nonlinear features like in images, video streams, or sounds. These kinds of data contain many parameters which can easily be handled with deep learning rather than easier machine learning models like a support vector machine, which is a popular net for nonlinear classification. The performance of such models is greatly affected by high numbers of dimensions in data. More features improve performance until a limit, after which performance drops significantly. Deep learning architecture solves these issues better by taking each layer independently and training in a greedy way. First, one layer gets trained completely, and then the next layer starts training with input from the previous layer [16].
D. CNN: The concept of convolution networks is to combine local computations, i.e., on weight sharing units, where the convolution of the signal is performed and pooling is completed. The system is invariant due to the convolutions, as parameters such as weights are dependent on spatial separation rather than spatial position [7]. The pooling creates a more suitable collection of features through a nonlinear combination of the preceding level features and consideration of the input data’s local topology. By alternately employing convolution layers and pooling layers, the model collects and integrates local features and creates a better representation of the input vectors. The connectivity of the convolution networks, where each neuron of convolution or a pooling layer is fully connected to a small subset of the previous layer, trains the network with multiple layers. Through error gradient backpropagation, the supervised learning is easily accomplished. CNNs need relatively minimal preprocessing compared to other image classification algorithms. The usage of a variant of multilayer perceptions by CNN is intended to need as little preprocessing as possible. In comparison to other image classification algorithms, CNNs require very minimal pre-processing. This implies that the network learns the filters that were previously hand-engineered in traditional algorithms. Convolution layers apply the convolution operation to input before forwarding the output to the next layer. The convolution emulates the response of an individual neuron to visual stimuli [25]. This feature design independence from earlier knowledge and human effort is a significant advantage. The convolution operation solves this concern by limiting the number of free parameters in the network, permitting it to be deeper with fewer parameters [20]. In this way, backpropagation eliminates the issue of diminishing or exploding gradients in training typical multi-layer neural networks with several layers.
E. RNN: Recurrent neural networks are neural networks that take the output of previous iterations as input and learn it through all their hidden states. They use previous output as feedback, and also memorize the independent variables as input, thus providing accurate predictions. RNN works by capturing the input
data’s sequential information. RNNs process the sequence of inputs by using their internal state, i.e., memory. In Figure 4, X0 indicates the first input after applying activations at input, while the hidden and output layers provide output Y0. When we say that it takes the output of previous state as its input, that means that while taking input at state X1, it includes the output of the previous state Y0; similarly, the input for state X2 will combine Y1. This way, it keeps training with outputs generated previously and takes them as learning signals [24]. RNN works with sequential data, so it works well with temporal data. That is why for our system to classify input as crime or not crime, we used an LSTM, which is an RNN. It takes input video sequences and processes them frame by frame. RNNs follow gradient descent for learning in a backpropagation-of-error fashion. When the gradient becomes small and an RNN does not learn well by updating its weights, then it suffers from vanishing gradients.
F. LSTM: Long Short Term Memory is a kind of recurrent neural network (RNN). In RNN, it solves the problem of vanishing gradients. It solves this problem by removing the long-term irrelevant dependencies of the current prediction. It finds out which recent or past input is useful for the accurate decision and forgets all others. For this, LSTM architecture uses 3 gates at its hidden states, namely the input, output, and forget gates. These gates keep track of information relevant for its prediction.
The input gate checks which input should be used to change the memory of the cell. The forget gate (a neural network with sigmoid) finds details to be discarded. For this, it uses the sigmoid function. The output gate takes the input and the memory of the block and decides the output. If any detail needs to be kept, it outputs 1; otherwise, it outputs 0 [23]. In our application of generating sequences of events in video streams and making decisions, vanishing gradients may lead to inaccurate predictions, but LSTM works in its desired form. For this, LSTM includes cells at each hidden layer neuron. Each cell takes the input of the current state and the output of the previous state and cell; e.g., in Figure 5, the input of cell ct will be input xt and output ht-1 and previous cell state ct-1. Three logistic sigmoid gates and one tanh layer make up an LSTM. These gated layers control the information which flows from the cells.
G. ECC: Elliptic curve cryptography is a very important and modern algorithm based on the asymmetric public key cryptography system. This is based on the finite field and difficulty of the elliptic curve discrete logarithm problem. This algorithm uses the key as a small value and smaller signature for the security but is fast to generate the key and signature [9]. The ECC uses a curve that provides very strong security with speed for the whole process of cryptography. Then, mathematically, we can understand the curve and the point (p, q) and describe the equation:
The cryptography elliptic curve is defined as: q2=p3+_a_x+b
This encryption algorithm is used in this proposed system to protect the data from unauthorized users. Sometimes, hackers intentionally create a problem by manipulating the data, so we are using a more secure concept..
This is a system for identifying anomalies in any event which is seen by a camera equipped with an autonomous vehicle. Any anomaly related to the behavior of an individual in the public or in a crowd can be detected by this system. Crime which includes physical violence or use of weapons such as knives or guns can be monitored using this mobile camera, which can also generate an alarm to the relevant security system. This whole process can lead to a safer city. Live surveillance with this automobile camera is also made secure using the ECC algorithm, so this live stream will not be able to be altered.
To monitor any event as a crime or not a crime, we have tried multiple methods. We experimented with CONVO-3D, VGG16 + LSTM, VGG16 + CONVO-LSTM, VGG16 + ANN, InceptionV3 + ANN, and InceptionV3 +
GRU, but the highest accuracy we got was from GG16 + CONVO-LSTM.
In the proposed method, first, the upload or live stream of an image or video goes through the ECC algorithm for a security check [17]. After passing it, the input is then preprocessed using a video data generator, before presenting it prior to learning. Here, the video is converted to time series images. Then, these RGB images are processed to YUV representation. The Y grayscale information is in the image or component of the luminance, while the U and V components contain the chromatic or color data. With each step, images are subsampled for lower resolutions. We have used the Keras Image Data Generator to convert raw videos into images divided in batch sizes. This Image Data Generator, instead of returning frames, provides a set of frames according to temporal length, temporal size, and batch size. Data augmentation is applied to enhance the size and quality of datasets used for training, which leads to a better model.
The architecture starts with feature extraction using VGG16. Then, there are 2 ConvoLSTM layers, with each one followed by Batch Normalization and a max pooling layer. Then, there is one LSTM layer, and at last, there are two dense layers for performing the task of prediction. This architecture includes the stack of many layers, which includes convolution with Rely activations that are nonlinear, spatial pooling carried out of max pooling layers, and the output layer is a softmax layer [10].
VGG16, a visual geometry group with 16 layers, is a vision architecture in the CNN model which is used
for image classification. Its architecture includes a 224 x 224 RGB image as input, followed by a stack of convolutional layers, where it filters with a very small receptive field: 3 × 3 are present. Further, there are max pooling layers and a fully connected stack of convolution layers. ConvLSTM is also an RNN for spatio-temporal prediction that has convolutional structures in both the input-to-state and state-to-state transitions. ConvLSTM determines the future state of a certain cell in the grid by the inputs and past states of its local neighbors [24]. This is like LSTM, but internal matrix multiplications are exchanged with convolution operations. The model has a single input, and the trailer frames in sequence are generated by Custom Video Data Generator and 2 independent outputs, one for each category. Then it gets chopped into branches where each category has one branch. Here, the processing for each branch is the same. All the branches are the same, as they start with one ConvLSTM layer then a MaxPooling layer. Then, this is connected to a fully-connected Dense network. Finally, the last layer is a Dense with a single cell. The next image illustrates the simplified model with only two categories (crime or not). The model was trained in 10 epochs.
Here, we have used the OpenCV library that has a huge amount of content of computer vision and is helpful for real-time operations. Using this, we have converted the video streams into frames and also used them for detection of objects like guns and knives.
Datasets taken for training include: Gun detection dataset; hockey fight detection dataset; large-scale anomaly detection which includes burglary,
explosion, fighting, and shooting videos; a dataset for evaluating blood detection. For example, the below image includes physical violence so this is labeled as “crime” and an alert will be generated. The optimizer used here is ADAM. The loss function used here is Binary Cross entropy [19].
As stated above, we have tried multiple models to find the best performance out of them. Below is the comparison of different models’ performances over training and validation datasets.
The proposed model, VGG16 + ConvoLSTM + LSTM, provided a training accuracy of 99.4% and validation accuracy of 95.9%. The prediction performed by this model is the most accurate. The model loss at the time of training is 0.017 and at validation it was 0.143.
With the help of machine learning and stored data at different levels, systems can understand and detect different crimes. This detection of crime through the autonomous system process is crucial to maintaining the whole system with efficiency. This system, in particular, provides the accuracy to detect crime as well as associated objects. Our system may prove to create very useful devices for society.
Drone types of autonomous systems of smart cameras will help us to detect the crime. We use efficient algorithms to understand the learning of given data sets. With this detection, we have also developed the system to send an alarm to the authorities for any given location.
For better control and more efficiency in communicating with good design, autonomous vehicles need a well-equipped drone system and a high camera quality. In addition, our system’s learning methodology will help give an accurate result. When the system gives us the proper result, our performance will increase.
The Indian government still does not make their thoughts regarding new technologies for the detection of crime and creation of reports clear. All the authority related to crime is currently based on manual verification done by the police department. The government should accept, apply, and properly communicate with the citizens regarding this autonomous detection system and evidence collection.
Timely detection of crime can save lives. A still camera can work, but a camera with an autonomous device can enhance the security. There may be many anomalies in crowd behavior, but recognizing them and then classifying them as crime is a crucial task. While there are other systems designed to perform this task, we propose a novel model to detect criminal activities among people. To check performance, we brought up challenging datasets of crime, violence, and objectionable carried objects. We have implemented 5 models which are com-
binations of techniques and applied these models on a variety of datasets. We have concluded that the VGG16 + CONVOLSTM + LSTM Model built on a custom video data generator works well as per the Accuracy and Loss Comparison charts. To capture live streams, there are many challenges, including camera quality, background noise, lightning conditions, etc. Generating an alarm will help to keep the city safe against crime. This whole system is also secured with the highly secure algorithm of cryptography, but also collectively increases the complexity of the system which requires high end configuration, which can be a limitation to process live streams. In the future, we will work on audio systems that give better results to contribute to the surveillance of anomaly crime detection.
AUTHORS:
Jyoti Kukade – Medi-Caps University, Indore, Email: jyoti.kukade@medicaps.ac.in.
Swapnil Soner* – Medi-Caps University, Indore, Email: Swapnil.soner@gmail.com
Sagar Pandya – Medi-Caps University, Indore sagar. pandya@medicaps.ac.in.
[1] B.M. Peixoto, B. Lavi, Z. Dias, A. Rocha, “Harnessing high-level concepts, visual, and auditory features for violence detection in videos”, Journal of Visual Communication and Image Representation, 10.1016/j.jvcir.2021.103174
[2] Y. Gao, H. Liu, X. Sun, C. Wang, Y. Liu, “Violence detection using Violent Flows”, 10.1016/j.imavis.2016.01.006
[3] T. Hassner, Y. Itcher & Y. Itcher, Violent Flows: Real-Time Detection of Violent Crowd Behavior, IEEE, 2012, www.openu.ac.il/home/hassner/data/violentflows/ 978-1-4673-16125/12/$31.00
[4] C. Ding, S. Fan, M. Zhu, W. Feng, and B. Jia, “Violence detection in video by using 3D convolutional neural networks”, Lect. Notes Comput. Sci. (including Subser. Lect. Notes Artif. Intell. Lect. Notes Bioinformatics), vol. 8888, 2014, pp. 551–558. 10.3390/app11083523
[5] G. Mu, H. Cao, and Q. Jin, “Violent Scene Detection Using Convolutional Neural Networks and Deep Audio Features,” 2008, pp. 645–651. 10.1109/ ICCSP48568.2020.9182433
[6] G. Sakthivinayagam, R. Easawarakumar, A. Arunachalam, and M.Pandi, “Violence Detection System using Convolution Neural Network”, SSRG Int. J. Electron. Commun. Eng., vol. 6, 2019, pp. 6–9.
[7] B. Peixoto, B. Lavi, and P. Martin, “Toward subjective violence detection in videos”, ICASSP, IEEE Int. Conf. Acoust. Speech Signal Process. - Proc., 2019, pp. 8276–8280.
[8] A. Hanson, K. Pnvr, S. Krishnagopal, and L. Davis, “Bidirectional convolutional LSTM for the detection of violence in videos”, Lect. Notes Comput. Sci. (including Subser. Lect. Notes Artif. Intell. Lect.Notes Bioinformatics), vol. 11130 LNCS, 2019, pp. 280–295.
[9] S. Soner, R. Litoriya, and P. Pandey, “Exploring Blockchain and Smart Contract Technology for Reliable and Secure Land Registration and Record Management,” Wireless Personal Communications, Aug. 2021.
[10] C. Dhiman and D. K. Vishwakarma, “A review of state-of-the-art techniques for abnormal human activity recognition,” Eng. Appl. Artif. Intell., vol. 77, no. 2018 Aug, 2019, pp. 21–45.
[11] S. Soner, A. Jain, A. Tripathi, R. Litoriya, “A novel approach to calculate the severity and priority of bugs in software projects”, 2nd International conference on education technology and computer, vol. 2, 2010, pp. V2-50-V2-54. 10.1109/ ICETC.2010.5529438.
[12] O. Kliper-Gross, T. Hassner, and L. Wolf, “The action similarity labeling challenge”, TPAMI, vol. 99, 2012.
[13] Q. Dai et al., “Fudan-Huawei at MediaEval 2015: Detecting violent scenes and affective impact in movies with deep learning”, CEUR Workshop Proc., vol. 1436, 2015, pp. 5–7.
[14] Y. Pritch, S. Ratovitch, A. Hendel, and S. Peleg. “Clustered synopsis of surveillance video”, In Advanced Video and Signal Based Surveillance, 2009, pp. 195–200.
[15] R. Retoliya, S. Soner, “RSA Based Threshold Cryptography for Secure Routing and Key Exchange in Group Communication”, International Conference on Advances in Communication, Network, and Computing, vol. 142, pp. 624-627.
[16] E.Y. Fu, H.V. Leong, G. Ngai, S.C.F. Chan, „Automatic fight detection in surveillance videos”, Int. J. Pervasive Comput. Commun., vol. 13, no. 2, 2017, pp. 130–156.
[17] T. Senst, V. Eiselein, A. Kuhn, T. Sikora, “Crowd violence detection using global motion-compensated Lagrangian features and scale-sensitive video-level representation”, IEEE Trans. Inf. Forensics Secur., vol. 12, no. 12, 2017, pp. 2945–2956.
[18] A. Hanson, K. PNVR, S. Krishnagopal, L. Davis, “Bidirectional convolutional LSTM for the detec-
tion of violence in videos”, in: Proceedings of the European Conference on Computer Vision (ECCV) Workshops, 2018.
[19] X. Zhai, A. Oliver, A. Kolesnikov, L. Beyer, “S4L: Self-supervised semi-supervised learning”, in: Proceedings of the IEEE/CVF International Conference on Computer Vision (ICCV), 2019.
[20] C. Borrelli, P. Bestagini, F. Antonacci, A. Sarti, S. Tubaro, “Automatic reliability estimation for speech audio surveillance recordings”, in: The IEEE International Workshop on Information Forensics and Security, WIFS, 2019.
[21] K. Gkountakos, K. Ioannidis, T. Tsikrika, S. Vrochidis, I. Kompatsiaris, “Crowd Violence Detection from Video Footage”, 2021 International Conference on Content-Based Multimedia Indexing (CBMI), INSPEC Accession Number: 20729035, 10.1109/CBMI50038.2021.9461921
[22] K. Gkountakos, K. Ioannidis, T. Tsikrika, S. Vrochidis, and I. Kompatsiaris, „A crowd analysis framework for detecting violence scenes”, Proceedings of the 2020 International Conference on Multimedia Retrieval, 2020, pp. 276-280.
[23] S. Soner, A. Jain, D. Saxena, “Metrics calculation for deployment process”, 2010 2nd International Conference on Software Technology and Engineering, 2010. 10.1109/ICSTE.2010.5608760
[24] M. Sharma, R. Baghel, “Video Surveillance for Violence Detection Using Deep Learning”, In: Advances in Data Science and Management, Springer: Berlin/Heidelberg, Germany, 2020, pp. 411–420.
[25] E. Ditsanthia, L. Pipanmaekaporn, and S. Kamonsantiroj, “Video Representation Learning for CCTV-Based Violence Detection”, TIMES-iCON 2018 - 3rd Technol. Innov. Manag. Eng. Sci. Int. Conf., 2019, pp. 1–5.
[26] F. U. M. Ullah, A. Ullah, K. Muhammad, I. U. Haq, and S. W. Baik, “Violence detection using spatiotemporal features with 3D convolutional neural network”, Sensors (Switzerland), vol. 19, no. 11, 2019, pp. 1–15.
Submitted: 21th July 2021; accepted: 29th of March 2022
Burkhard Bamberger, Bastian KörberDOI: 10.14313/JAMRIS/1-2022/8
Abstract:
This research should help scholars and practitioners to manage the transition of monolithic legacy application systems to microservices and to better understand the migration process, its steps, and its characteristics. It should also provide guidance on how best to approach the migration process. We performed a systematic literature review and analyzed migration approaches presented by other research. We propose leveraging Robotic Process Automation technology to extract business logic and create and deploy bots, which are then used to mimic microservices. In essence, this represents a novel use case of integrating RPA technology into the migration approach in order to reduce uncertainty and risk of failure.
Keywords: Microservice, RPA, Monolithic Architecture, Reverse Code Engineering, Migration
1. Introduction
Organizations increasingly rely on information technology to create value. New digital business models, changes to business processes, and automation of tasks previously performed by office workers require investment in hardware and software. Management is then tasked with deciding how to best allocate resources in the field of information technology. Capital expenditure and implementation costs associated with introducing new or updating existing application systems are material. Therefore, management needs to assure that the organization’s information systems adequately support its business strategy.
Many application systems, however, were introduced years ago and have been continuously customized and upgraded. These “legacy systems” were designed following a monolithic architecture style that dates back to legacy mainframe computers [29]. Replacing or updating existing legacy systems to address changes in business strategy or higher system load requirements due to increased transactional volume is a key challenge, especially since monoliths often lack scalability.
Monolithic applications are self-contained, consist of a single code base, include every single functionality, and are easily implemented [34]. Modularity, however, is not considered as a design principle [35];
therefore, it functions within a monolith collectively sharing resources on the host system, which limits scalability [32]. Developing, maintaining and changing monolith applications also becomes increasingly difficult and slow, as they tend to grow in size and complexity [44]. Cloud services make automatic scaling easy and cost-efficient; however, large monolithic applications cannot take full advantage of these functionalities [28]. Amazon, Netflix, LinkedIn, Soundcloud and other leading technology companies were among the first to transition to microservices [16].
Microservices represent a fundamentally different architectural design principle. Prioritizing decentralization over centralization is the common pattern guiding the development of distributed applications on cloud platforms [36]. Suites of small, independent services, sharing as little as possible, each running in its own process, and communicating with lightweight mechanisms are key characteristics of this architectural design style [16] [17]. Each single service capsules small deployable chunks of application logic, built around business capabilities [24] which is separately developed and deployed by a small, dedicated team [24]. This should allow for agile development and operation [10] resulting in “high availability and redundancy, automatic scaling, easier infrastructure management and compliance with latest security standards…” [8].
Therefore, microservice-based applications are advantageous from a flexibility, scalability, complexity, agility, and maintainability perspective [36]. In microservices, business processes are split up into separate, manageable components, as task logic is codified within distinct, easily identifiable services. By comparison, task logic is hard to locate and change within monolithic systems. Since business processes are made up of multiple “logically-related tasks performed to achieve a defined business outcome” [14], changes in corporate strategy necessitate adaptions to the application logic. Therefore, in creating new application systems, microservices are favored over monolithic principles, as early adopters have demonstrated. In contrast, when legacy systems were designed, applications were almost exclusively built according to monolithic architectural principles. If monoliths require major modifications to address new business requirements or improve scalability, management needs to decide whether to (a) buy and implement new software, (b) build new application from scratch,
(c) patch-up the legacy system by modifying the existing code base to reflect the desired changes or (d) migrate the monolith toward microservices to improve flexibility, scalability, agility and maintainability. This paper researches option (d), the migration of monoliths to microservices.
Robotic Process Automation (RPA) is a technology designed to automate business processes or task sequences without changing existing back-end systems [46] by building and deploying digital agents that mimic activities of human users in a variety of different application systems [30]. This lightweight automation approach via the application´s user interface opens up previously untapped automation potentials due to its ease of use, speed of implementation, and cost-effectiveness (Czarnecki & Fettke, 2021).
Despite the compelling advantages of microservices, migrations are rare [35]. This may be rooted in a perceived risk and uncertainty surrounding the migration. Our literature review revealed that except for comprehensive migration approaches presented by Maisto et al. (2020) and Megargel et al. (2020), most research focuses on code reverse engineering, a multitude of methods aimed at extracting business logic from the monolith’s code base. We describe how migration processes are approached and which steps are critical. Rather than reengineering business logic from legacy code, we propose to use RPA to create and deploy bots, which mimic microservices. This not only provides an alternative solution to the critical extraction phase, but the bots also serve to improve and speed up the testing of microservices. This integrated approach should help reduce risk associated with migrating monoliths to microservices and, therefore, be of interest to both academics and practitioners.
Migrating from monolith to microservices is a complex undertaking. It requires a thorough understanding of the elements and characteristics of both the starting point (monolith) and end (microservices). A migration approach needs to address how to transform crucial elements, what steps to take and which steps warrant special attention, as they are mission critical.
Our key research questions are: (1) What migration approaches have other researchers or practitioners presented? (2) What are the benefits and challenges associated with each approach? (3) What alternative solutions or which modifications help reduce uncertainty and risk of failure?
Microservices is a relatively new design in software architecture, having gained popularity in the wake of cloud technology. Migrating monoliths to microservices has received limited attention from academia and migration to microservices is a rare phenomenon in practice [35]. There are few earlier studies; however, research efforts are at a preliminary stage. Therefore, qualitative, exploratory research seems most appropriate to establish an understand-
ing of the migration process, its steps and characteristics, clarification on proposed approaches, as well as new ideas complementing existing approaches. Furthermore, it can help to structure, clarify, and prioritize future research and assist practitioners with resource allocation.
This research applies a design science approach, aiming at introducing new and innovative artifacts as well as the process of creating artefacts [42]. There are three stages of the research process [21]: First, we established the relevance of our research by inquiring and documenting the state of the art process of migration. Then, we modified and enhanced an existing artefact (migration approach), evaluated earlier versions and refined them. Lastly, we assured research rigor by leveraging the existing knowledge base as well as personal experiences and shared knowledge with professionals in the software industry. As a result, we designed an innovative artefact to help solve the practical problem of migrating from monolith to microservices.
In this section, we summarize migration approaches by other researchers or practitioners. Our steps of identifying, selecting and documenting relevant sources followed the process proposed by Onwuegbuzie et al. (2012) and O’Brian and McGuckin (2016). We first performed an internet search on the Google and Google Scholar platform by using the search string “migrating monolith to microservices.” Based on these results, we modified and applied various alternative search strings and controlled for different spellings and synonyms. Additional test searches were then performed in the EBSCO, WISO, De Gruyter and Springer repositories. As a result of our preliminary searches, we identified a literature review by Silva Filho and Figueiredo Carneiro. Their search was conducted on May 4, 2018, covered a period of 10 years, and yielded 95 studies, of which only 12 contributions addressed monolith to microservice migration strategies. Of those, five articles focused on extraction techniques: Chen et al. (2017), Escobar et al. (2016), Baresi et al. (2017), Jamshidi et al. (2017), and Aiello et al. (2016).
We decided to build on these findings, limited our search to the period May 2018 through August 2020, again refined the search strategy, and performed our final search in the repositories listed above on September 1st 2020. Furthermore, we searched Scopus and Web of Science as well as ResearchGate to assure we did not miss relevant contributions. 48 sources were identified, of which 11 were categorized as highly relevant. Two sources provided a comprehensive migration approach: Maisto et al. (2020) and Megargel et al. (2020). Another nine sources addressed various extraction methods: Taibi and Systä (2020), Li et al. (2019), Abdullah et al. (2019), Ma et al. (2019), Nunes et al. (2019), Bucchiarone et al. (2020), Alwis et al. (2019a), Pigazzini et al. (2019), and Henry and Ridene (2020).
In total, the literature review performed by Silva Filho and Figueiredo Carneiro and our own search yielded 16 highly relevant sources as summarized in table 1 and 4:
Tab. 1.: General Migration Approaches (own illustration)
General Migration Approaches
Three phases
Six phases
Authors
Maisto et al. (2020) Megargel et al. (2020)
General migration approaches provide a comprehensive, sequential phase model, which describes each stage of the migration from monolith to microservice.
Tab. 2. Six migration phases proposed by Megargel et al. (2020)
Phase 1: Decoupling Monolith
1. Add Service Layer / Façade
2. Add Service Mediation Layer
Phase 2: Develop Local Microservices
3. Identify Microservices
4. Develop Interface Definitions
5. Develop Microservices
Phase 3: Implement Local Microservices
6. Migrate Data
7. Testing / Parallel Run
8. Swing Channels to Microservices
First, the monolith is decoupled by introducing a layer between the frontend user interface layer and the backend business logic layer. The service mediation layer is added to provide run-time control over the channel-to-service mapping. With this capability, it is possible to swing the entire channel to consume microservices. Next, local microservices are programmed using standard development and testing tools. In addition, design time governance tools to manage the microservices design lifecycle are recommended. As a result, microservices reflect the same business logic and data scheme as the original function within the monolith. Implementation of local microservices starts with data migration, such that the
Maisto et al. (2020) proposes a three-step model, starting with the decomposition phase, in which the application´s source code is analyzed and candidates for microservices are identified. Next, in the microservice production and ranking phase, designers receive a set of guidelines and a priority index. Communication stubs provide designers with development proposals for microservices, re-engineering the monolith´s functionalities. After all microservice candidates are defined, the new architecture is established, existing code is modernized, and new microservices are generated. Finally, the new microservices architecture is evaluated and services are deployed to the cloud [34].
Megargel et al. (2020) take a broader perspective, presenting a six-phase model, consisting of 14 steps as summarized below:
Phase 4: Deploy Microservices to Cloud
9. Implement API Gateway
10. Deploy Microservices
Phase 5: Implement Microservices on Cloud
11. Migrate Data to Cloud
12. Parallel Run in Cloud
13. Swing Channels to API Gateway
Phase 6: Decommission Monolith
14. Unplug Monolith
channel invokes monolith and microservices, allowing both running in parallel. Reconciling data generated by both systems is used for testing each microservice before swinging the channel to exclusively invoke the microservice. This loop is repeated for every single microservice or a batch of services until all are implemented locally. The “swing” to microservices can be effected without changing a single line of code, because both use exactly the same interface [35]. The remaining phases relate to cloud deployment and implementation as well as the eventual decommissioning of the monolith.
Both migration models are summarized below and condensed into five migration phases:
Maisto et al. (2020)
Decomposition
Identify microservice candidates via decomposition
Microservice production and ranking
Define architecture
Create documentation describing all functionalities
Ranking / prioritizing
Re-engineering
Cloud deployment
Evaluation of microservice architecture
Megargel et al. (2020)
Decouple monolith
Develop local microservices
Migration Phase
Decouple
Extract
Develop interface definitions
Develop microservices
Implement local microservices
Migrate data
Testing / parallel run
Swing channels to microservice
Deploy microservices to cloud
Implement microservices on cloud
Decommission monolith
Maisto et al. (2020) focus on the extraction and development phase. They recommend extracting process information from code by identifying classes and methods that make up the project, assuming that for each class a corresponding microservice can exist. In contrast, Megargel et al. (2020) place emphasis on the testing and deployment phase, even though they state that identifying microservice candidates in the extraction phase “… is both the most tedious step and the most critical step in the entire migration process.” [35]. Irrespective of
Develop
Test / deploy
Decommission
the different emphasis both contributions place on various steps of the process, we derived five stages, which describe the steps to follow in a migration project: decouple, extract, develop, test/deploy and decommission.
The remaining highly relevant sources discuss extraction methods. By analyzing existing code, granular information on business logic can be extracted in order to generate microservices via code reverse engineering, providing the same functionality as the monolith:
Extraction Method Author
Data Flow Driven
• via business process mining
• via dataflow-driven semi-automatic decomposition approach
• via detailed dataflow diagram
• a black-box approach that uses the application access logs and unsupervised machine-learning algorithm
Graph Dependencies
• via GSMART
• via Java-call-graph
• via visualising dependencies between components or layers.
Sematic Similarities
• via semantic similarity of functionalities
• via a text-based meta-modelling framework
Pattern Driven
• pattern-driven Architecture Migration (V-PAM)
• structure the architecture by software functions and their interactions
Tool Supported
• Arcan
• Service Cutter
• Blue Age Analyzer
Taibi and Systä (2020)
Li et al. (2019)
Chen et al. (2017)
Abdullah et al. (2019)
Ma et al. (2019)
Nunes et al. (2019)
Escobar et al. (2016)
Baresi et al. (2017)
Bucchiarone et al. (2020)
Jamshidi et al. (2017)
Alwis et al. (2019a)
Pigazzini et al. (2019)
Aiello et al. (2016)
Henry and Ridene (2020)
Data flow-driven extraction methods build on transaction data generated by the monolith. Data analytics combined with pattern recognition help to identify service candidates. Several contributions are based on this principal idea: a data flow-driven semi-automatic decomposition method using finegrained Data Flow Diagrams (DFD) to cluster service candidates [31] [12]; a black-box approach that uses application access logs and unsupervised machine-learning algorithms to map URL partitions with similar performance and resource requirements [1]; and a method using business process mining to identify service candidates [43].
Graph dependencies methods provide a visual representation of the dependencies between elements of the code to identify service candidates. Java-callgraphs are generated by collecting data using a static code analyzer, and then assessing communication rates in order to identify classes that have a high coupling. The architect generates a dendrogram using hierarchical clustering algorithms. The generated information is then visualized to assist the architect in informed experimentation until a fair balance is achieved between the microservices service covered and the communication rate [37]. GSMART (Graph-based and Scenario-driven Microservice Analysis, Retrieval and Testing) generates a different type of visual representation, while Service Dependency Graphs (SDG) visualize relationships and accelerate the development of new microservices [33]. Alternatively, dependencies between components or layers of applications (business and data layer) can be visualized [15].
Semantic similarity-based extraction methods use algorithms trained to detect linguistic patterns in order to identify relationships between sections and lines of code, using a text-based metamodeling framework [11] or a reference vocabulary, to identify potential candidates as groups of cohesive operations and associated resources. This should help in decomposing the monolith and also generate insights about granularity and cohesiveness of obtained microservices [7].
Pattern-driven methods use empirical data generated by observations from prior migration projects. Cloud architecture migration patterns, migration process frameworks, and variability models are complemented by secondary source analysis and derived to compose a migration plan (Jamshidi et al. 2017). Alternatively, the architectual structure of the monolith can be decomposed using queuing theory and business object relationship analysis [3].
Finally, various software tools are available to support architects in decomposing mostly Java-based applications. These tools use a variety of methods discussed above: “Arcan” analyses the monolith´s static structure, generates dependency graphs, and uses algorithms to detect and extract specific topics from the code without human supervision. These topics could help identify service candidates. Algorithms, such as Latent Dirichlet Allocation (LDA), Seeded Latent Dirichlet Allocation (SLDA), and a semi-supervised variant of the original LDA algorithm, extract topics [40].
“Service Cutter” extracts coupling information and engineering artefacts, such as domain models and use cases, to find and score densely connected clusters. The resulting candidate service cuts promise to reduce coupling between, and promote high cohesion within, services [2]. “Blue Age Analyzer” uses queuing theory and business object relationships to identify candidates. It automatically identifies all entry points into the system and organizes the dependencies into concentric rings. Microservice candidates appear as local trees starting from the outside [19].
In summary, except for the comprehensive threephase approach presented by Maisto and the sixphase approach put forward by Megargel, all other contributions focus on extraction methods rather than a holistic view of the migration process.
Next, we may address the second research question on the benefits and challenges associated with each of the approaches summarized in the previous chapter: General migration approaches as put forward by Maisto et al. (2020) and Megargel et al. (2020) provide a comprehensive model on how to approach migration projects. Their phased model provides insight on how to perform the steps associated with each phase. However, the extraction phase is the most challenging and critical, but the authors only offer limited guidance on how to identify and implement microservices. The value of both approaches predominantly lies in the comprehensive framework and the orientation it provides for migration projects. However, the critical extraction phase would need to be complemented by extraction methods summarized above, or by leveraging RPA technology, such as a novel RPA use case we present in section 5.
Most research on migration projects focuses on extraction methods. Data-driven extraction methods are business-focused and provide quick information on processes and variants as they are performed in the organization. However, this black box approach does not detect hidden business logic and the quality of the analysis greatly depends on the input data. In addition, the architectural structure of the code is disregarded and there is no reuse of code. Graph dependencies depict the architectural structure and provide transparency on input-output relationships; however, this requires a lot of manual input. Semantic-based extraction is highly automated; however, results also need substantial manual rework, especially if coding and naming conventions of the legacy system are inconsistent. Pattern-driven approaches are solely based on professional experience in comparable migration projects and, therefore, are highly subjective in nature. There is no transparency on how architectural, business, and process perspectives guide the migration effort. Finally, dedicated extraction tools often feature a combination of different methods and work best with Java, though dedicated tools only provide limited assistance to architects.
In essence, the idea of extracting business logic from the legacy code base is appealing, since recreating and documenting business processes, even when equipped with dedicated extraction tools, is a tedious task developers are ill equipped to perform. Since business logic needs to be documented on the most granular (click) level, only process owners and dedicated staff would be able to provide this input. Therefore, code reverse engineering potentially speeds up the project and reduces the need for developers to interview and solicit input from domain and process experts. In addition, the potential reuse of existing code is helpful and works best if the monolith is comparably small and of limited complexity. Taking a look “under the hood” of the monolith may provide a good starting point for these tasks and reduce the need of having to revert to business personnel to mine for business logic and recreate detailed process documentation.
Even though there is a wide range of extraction methods, all but the data driven approach require access to the source code of a legacy system. This is not always possible, as many legacy systems were made from off-the-shelves software packages, bought many years ago. Vendors no longer support these applications. Some legacy ERP or CRM systems are run as terminal solutions. Therefore, the extraction methods discussed above typically are limited to proprietary software, where developers have access to code.
In addition, legacy systems are usually outdated, and their features and functionality do not reflect current and anticipated business requirements. Therefore, re-engineering, i.e., recreating outdated business logic and software functionality by using a new architectural style, does not address the full potential of a major migration effort.
Ultimately, code reverse engineering is building a new structure on an existing, presumably outdated foundation. There is the potential to save time and resources if existing elements of code are reused; however, on the flip side are limitations in terms of outdated structures hampering progress and innovation in designing the new microservice-based application.
The above evaluation of migration approaches has revealed substantial challenges; therefore, we may now address our last research question: What alternative solution or modifications may help reduce uncertainty and risk of failure in migration projects? We propose a novel approach, in which RPA bots mimic microservices. This requires certain modifications to standard RPA design principles (section 5.1). We propose a new systematic framework on how to integrate RPA into the standard migration process (section 5.2). The following is our evaluation as to whether the integrated migration approach helps reduce uncertainty and risk of failure (section 5.3).
For a seamless integration of RPA bots into the migration process and the ultimate replacement of the bot by a corresponding microservice, we propose modifications to the RPA process:
Tab. 5. Standard RPA approach and required modifications for bots to mimic microservices (own illustration)
Identification
Process identification based on business and technical criteria
Description
Detailed process documentation
Development
Iterative implementation, testing, technical and commercial acceptance
Deployment
Roll out bot to vendorspecific RPA platform, test against productive systems
Decommission
Split processes and define interfaces
Apply microservice principles to bot architecture
Control via RESTful API, parallel testing
Swing bot to microservice, decommission bot
The first step in RPA projects is process identification, which is supported by technical tools such as Process Mining, Task Mining, and Process Discovery (Reinkemeyer 2020, p. 185). If RPA technology facilitates the migration to microservices, process identification does not require any modification.
Next, processes selected for automation are analyzed and documented via vendor tools such as AM Muse [5] or UI Task Capture [45]. The resulting process descriptions and additional information on application programming interfaces form the basis for creating the bots. Discussions between application managers and IT may result in amendments to the development roadmap. In the case of synergies or redundancies, tasks or parts of a process can be automated by using existing interfaces or simple extensions to the interface. However, since monoliths usually do not provide technical interfaces, bots can simulate the interface and thus allow for quick automation. Furthermore, the process documentation as well as transaction data generated by the bot is useful for developing microservices.
Bot development is mission critical. Since the bot will mimic a microservice, and eventually be replaced by one, adoption of microservice architectural principles to bot creation is essential. Important characteristics of this architectural design style are: capsuled, deployable sequence of application logic, sharing as little as possible, independence from other services,
and lightweight communication mechanisms [16] [17] [24]. Key microservice design principles compare with RPA as follows:
• Microservice architecture is based on a sharenothing philosophy [18]. This is a challenge for RPA since bots access an application such as a human user via the presentation layer. Therefore, the bot always requires a predetermined state of an application as a start and end. For example, each bot first must log on to an application before it can execute tasks within the application. Due to the share-nothing principle, the log-on routine cannot be shared between different bots.
• Microservices should contain only limited application logic to allow for independent deployment. It should be small enough that a team of developers can build and maintain it. A single person should be able to understand the full context of the microservice. Ideally, it should have less than a few hundred lines of code [35].
• The principle of independence calls for a system of services which consists of independent microservices (slices) that are mostly independent to each other [22]. This should be the case if services are individually deployable, run as selfcontained units, and encompass an operating system along with the necessary runtimes, frameworks, libraries, and code [10].
• Microservices need to tolerate the unavailability of the services they access [10] and minimize fallout from unexpected constellations [16]. These design principles are addressed in the bot development phase as follows:
• Processes are split into small, distinct tasks. Some tasks are performed multiple times within one process. These standard tasks are assigned to microbots, small reusable bots designed to perform a single dedicated task only. Microbots have a defined starting and ending state, as well as defined input and output parameters. Since bots invoke microbots, the underlying business logic is implemented only once.
• For bots to be independently deployable and resilient against unavailability of services, they need to have a predetermined starting and ending point with clearly defined properties. In a Windows environment, this is the login window, because this is where the operating system will return after the computer reboots in the case of unforeseen events.
• Bots should execute a transaction one case at a time and only start the next case if the last run is successful. Batch processing is incompatible with microservice architectural principles. For example, a purchase order confirmation issued by a bot must be completed by one business process instance before the bot is restarted and the next purchase order is manipulated [24].
• Bots perform tasks in a sequential fashion. If an application is not responding, this creates a roadblock for process execution. Rather than
aborting the sequence, the bot should pause and restart automatically after a pre-set amount of time has lapsed. This results in higher rate of successfully completed processes.
After successful development, bots are deployed. Microservices communicate through lightweight mechanisms, often a RESTful API [17]. The same mechanism should control the bot. Many RPA vendors offer this feature in their product. It is important to define input and output parameters according to RESTful API standards, since both bot and microservices use this interface. According to the open-close principle [23], the bots and subsequent microservices should be open to extension, but closed to modification. This implies that adding new functionality to software should not affect existing code. Stability on the interface level safeguards the eventual migration from bot to microservice.
In classical RPA, the decommissioning of bots is rarely discussed, as they are used for as long as they function properly and serve a purpose. The value of a bot predominantly lies in its encapsulated process knowhow, which may become obsolete if underlying business models or processes change. Therefore, decision-making on the decommissioning of bots is mostly discussed in the context of creating superior automation solutions, which then render the bots superfluous. In this context, bots are decommissioned once the microservice fully functions and parallel tests are completed.
We established that bots can mimic microservices if certain design principles are observed. Next, we will demonstrate how to integrate RPA technology into the migration process. For simplicity reasons, the flow chart does not depict process loops necessary to address the great number of different processes in a migration project:
First, the monolith is decoupled by introducing a façade layer between the user interface and the business logic layer [35]. A service mediation layer provides run-time control. The refactoring towards microservices should be done in small parts [28]. Next, monoliths are decomposed by identifying microservice candidates. If the code base is accessible, developers can use standard extraction methods as outlined in Section 3. Alternatively, RPA technology can extract business logic via Process Mining, Task Mining, and Process Discovery, described and documented via RPA vendor tools, and complemented by additional information on application programming interfaces. As a result, developers create a ranking of microservice candidates based on technical (e.g.., outdated code ratio) and commercial criteria (e.g., cost benefit ratio).
Starting with top-ranked microservice candidates, a decision as to whether or not a bot should be created is made. When a service does not perform updates on the database or call other services [19], or when it performs complex calculations, it is recommended to develop microservices without creating bots first. Some examples are rewards services from an online shop which exclusively uses customer information or authentication services [19]. For all other service candidates, a business case determines whether to build the bot.
If yes, the existing process documentation and interface definitions are the basis for the bot. Since the bot shall mimic a microservice, and eventually replace it, adoption of microservice architectural principles as outlined above is essential. Running the bot generates transaction data, which helps identify process variants that were potentially overlooked in the description phase. RPA developers can develop and test bots in parallel to software architects focusing on reengineering microservices. Therefore, having two teams with different skill sets working towards the same goal should expedite the migration process.
If no, the microservice architecture mirrors information derived by extraction methods as discussed earlier. In addition to business logic extracted via decomposing existing code, the process documentation represents valuable information to software architects and is helpful for reengineering and developing microservices.
Good test coverage is required due to the risk of new bugs ending up in the existing features [34]; therefore, the required data of the monolith must be migrated into the new data structure of the microservice, unit tested, and deployed for each service.
If there is no bot to test, developers need to write unit tests. After successful tests, the microservice runs parallel to the monolith until no errors occur. If there is a bot, it is orchestrated via microservice-compatible mechanisms. Both the bot and microservices run in parallel and perform the same request with identical input data. If the output is identical, the test was successful.
The monolith is decommissioned if test results confirm the full set of features of the monolith is covered by microservices [35].
Leveraging RPA in a migration context is helpful because it provides an alternative solution in case there is no access to the code base and most extraction methods do not work. In addition, in many cases, it is easier and quicker for software architects to draw on business resources to extract business logic, via process identification and description, to obtain detailed guidance for developing microservices. Especially in large and complex monoliths it is tedious and difficult for software architects to extract business logic from code [28].
Software architects often are in short supply; therefore, leveraging business resources for providing input to the developer saves time by easing resource bottlenecks. It is quick and comparatively simple to document processes and create bots via RPA software even for non-IT staff lacking coding skills. Furthermore, not being bound to a potentially outdated structure of the legacy application provides developers with a higher degree of freedom.
In the testing phase, bots are also beneficial, since automatic testing by running bots and microservices in parallel is a safe and fast way to validate that the software does what it needs to do [28].
After completion of the testing, bots are decommissioned. This alleviates potential maintenance problems associated with RPA. Since bots are bound to the presentation layer of legacy applications, any change to the presentation layer (for instance, due to updating to a new release) requires bot modification. This is more likely to happen the longer a bot is in use. In the integrated migration approach, we propose to use bots only temporarily until microservices take over. Therefore, bot maintenance should not turn out to represent technical debt.
On the other hand, RPA technology is non-invasive and mandates no changes to existing application systems [6]. Therefore, bots build on existing, often outdated applications, which may turn out to be a limiting factor, potentially reducing flexibility.
In summary, based on the above evaluation, applying RPA technology in migration projects is advantageous and conducive to mitigating migration risks, especially in the extraction and testing phase.
Almost 90% of business leaders in the U.S. and U.K. expect IT and digital technologies to make an increasing strategic contribution to operations in the coming decade [20]. This technology-induced change involves improvements to existing processes, exploration of digital innovation, and potentially a transformation of the business model [9]. Managing change successfully provides organizations with opportunities to create value. IT contributes to value creation through automation effects (productivity improvements, labor savings and cost reductions), informational effects (collected, stored, processed, and disseminated information improves decision quality) and transformational effects (facilitate and support process
innovation and transformation) [27]. Therefore, IT strategies need to ensure efficient management of IT infrastructure and application systems. In particular, keeping IT systems aligned with business models and processes underscores the need for IT to become more agile and business-centric [20].
It Is not surprising that leading tech companies like Amazon or Netflix have transitioned from creating and running monolithic applications to applying microservice design principles to their software architecture because of flexibility, scalability, complexity, agility, and maintainability considerations. Still, many organizations operate and maintain legacy monolith systems despite the compelling advantages of microservices, and migrations to microservices are still rare [35]. Helping organizations to keep IT systems up to date and aligned with changing business models by facilitating a smooth migration to microservices offers substantial potential to create value. We, therefore, researched state-of-the-art migration approaches and discovered weaknesses, especially in the extraction phase.
In search of alternative solutions, we realized that instead of extracting business logic from code, RPA draws on business resources to understand business processes and requirements. This represents a novel use case for RPA technology in a migration context. We proposed to integrate RPA into the migration process and determined design principles for bots to mimic microservices. Once microservices are parallel tested and operational, bots are decommissioned, and microservices replace the monolith. Based on logical reasoning, we showed that our integrated approach is conducive to reducing migration risk. This should provide IT management and software engineers with guidance on how to manage migration projects.
The key limitation of this paper is a lack of empirical evidence, as the feasibility of the integrated migration approach is yet to be tested in practice. With this paper, we promote the idea to leverage RPA technology in the migration to microservices. However, empirical findings from real-life applications of this approach are as yet outstanding.
Burkhard Bamberger* – ISM International School of Management GmbH, 44227 Dortmund, Germany, email: Burkhard.Bamberger@ism.de.
Bastian Körber – ISM International School of Management GmbH, 44227 Dortmund, Germany, email:bastian.koerber@outlook.de.
[2] M. Aiello, E.B. Johnsen, S. Dustdar, I. Georgievski, (Eds.), Service-Oriented and Cloud Computing. Cham: Springer International Publishing (Lecture Notes in Computer Science), 2016.
[3] A. A. C. de Alwis, A. Barros, C. Fidge, A. Polyvyanyy, “Availability and Scalability Optimized Microservice Discovery from Enterprise Systems”, in H. Panetto, C. Debruyne, M. Hepp, D. Lewis, C. A. Ardagna, R. Meersman (Eds.), On the Move to Meaningful Internet Systems: OTM 2019 Conferences, vol. 11877, Cham: Springer International Publishing (Lecture Notes in Computer Science), 2019, pp. 496–514.
[4] A. A. C. de Alwis, A. Barros, C. Fidge, A. Polyvyanyy, “Business Object Centric Microservices Patterns”, in H. Panetto, C. Debruyne, M. Hepp, D. Lewis, C. A. Ardagna, R. Meersman (Eds.), On the Move to Meaningful Internet Systems: OTM 2019 Conferences, vol. 11877. Cham: Springer International Publishing (Lecture Notes in Computer Science), 2019, pp. 476–495.
[5] Another Monday Intelligent Process Automation GmbH, AM Muse, Version 1.35: Another Monday Intelligent Process Automation GmbH, 2020. https://www.anothermonday.com/products/ am-muse
[6] G. Auth, C. Czarnecki, F. Bensberg, “Impact of Robotic Process Automation on Enterprise Architectures”, in Lecture Notes in Informatics (LNI), 2019. DOI: 10.18420/INF2019_WS05
[7] L. Baresi, M. Garriga, A. de Renzis, “Microservices Identification Through Interface Analysis”, in F. de Paoli, S. Schulte, E. B. Johnsen (Eds.): Service-Oriented and Cloud Computing, vol. 10465. Cham: Springer International Publishing (Lecture Notes in Computer Science), 2017, pp. 19–33.
[8] L. Bass, I. M. Weber, L. Zhu, DevOps, A software architect’s perspective, New York: Addison-Wesley Professional (The SEI series in software engineering), 2015.
[9] S. Berghaus, A. Back, “Stages in Digital Business Transformation: Results of an Empirical Maturity Study”, MCIS 2016 Proceedings, 22, 2016. https://aisel.aisnet.org/mcis2016/22, checked on 2/19/2020
[1] M. Abdullah, W. Iqbal, A. Erradi, “Unsupervised learning approach for web application auto-decomposition into microservices”, Journal of Systems and Software, 151, 2019, pp. 243–257. DOI: 10.1016/j.jss.2019.02.031
[10] F. Boyer, X. Etchevers, N. de Palma, X. Tao, “Architecture-Based Automated Updates of Distributed Microservices”, in C. Pahl, M. Vukovic, J. Yin, Q. Yu (Eds.): Service-Oriented Computing, vol. 11236, Cham: Springer International Publishing (Lecture Notes in Computer Science), 2018, pp. 21–36.
[11] A. Bucchiarone, K. Soysal, C. Guidi, “A Model-Driven Approach Towards Automatic Migration
to Microservices”, in Jean-Michel Bruel, Manuel Mazzara, Bertrand Meyer (Eds.): Software Engineering Aspects of Continuous Development and New Paradigms of Software Production and Deployment, vol. 12055, Cham: Springer International Publishing (Lecture Notes in Computer Science), 2020, pp. 15–36.
[12] R. Chen, S. Li, Z. Li, “From Monolith to Microservices: A Dataflow-Driven Approach”, in 2017 24th Asia-Pacific Software Engineering Conference (APSEC), 2017 24th Asia-Pacific Software Engineering Conference (APSEC), Nanjing, 04/12/2017 - 08/12/2017: IEEE, 2017, pp. 466–475.
[13] C. Czarnecki, P. Fettke, “Robotic Process Automation: De Gruyter”, 2021. https://doi. org/10.1515/9783110676693
[14] T. H. Davenport, The new industrial engineering: information technology and business process redesign” in Sloan management review, 1954 (1990).
[15] D. Escobar, D. Cardenas, R. Amarillo, E. Castro, K. Garces, C. Parra, R. Casallas, “Towards the understanding and evolution of monolithic applications as microservices” in 2016 XLII Latin American Computing Conference (CLEI), 2016 XLII Latin American Computing Conference (CLEI), Valparaíso, Chile, 10/10/2016 – 14/10/2016: IEEE, 2016, pp. 1–11.
[16] M. Fowler, J. Lewis, „Microservices: Nur ein weiteres Konzept in der Softwarearchitektur oder mehr“, in Journal of Systems and Software, 2015, pp. 14–20.
[17] M. Garriga, “Towards a Taxonomy of Microservices Architectures”, in Software Engineering and Formal Methods, 2018, pp. 203–218.
[18] J. Ghofrani, D. Lübke, “Challenges of Microservices Architecture: A Survey on the State of the Practice”, 2018.
[19] A. Henry, Y. Ridene, “Migrating to Microservices”, in A. Bucchiarone, N. Dragoni, S. Dustdar (Eds.), Microservices. Science and engineering, Cham: Springer International Publishing, 2020, pp. 45–72.
[20] T. Hess, C. Matt, A. Benlian, F. Wiesboeck, “Options for formulating a digital transformation strategy”, in MIS Quarterly Executive (15), 2016, pp. 123–139.
[21] A. R. Hevner, “A Three Cycle View of Design Science Research”, Scandinavian Journal of Information Systems, Volume 19, Issue 2, 2007, pp. 87-92.
[22] M. Hilbrich, “In Microservices We Trust – Do Microservices Solve Resilience Challenges?”,
Tagungsband des FB-SYS Herbsttreffens 2019. Bonn: Gesellschaft für Informatik e.V., 2019. DOI: 10.18420
[23] A. Hochrein, “Anatomy of a Microservice”, in Akos Hochrein (Ed.): Designing microservices with Django, An overview of tools and practices, 1st edition, [S.l.]: Apress, 2019, pp. 49–68.
[24] A. Hochrein, “From Monolith to Microservice”, in Akos Hochrein (Ed.): Designing microservices with Django, An overview of tools and practices, 1st edition, [S.l.]: Apress, 2019, pp. 111–137.
[25] J. Holmström, M. Ketokivi Mikko, A.-P. Hameri, “Bridging Practice and Theory: A Design Science Approach”, in Decision Sciences Volume 40, Issue 1, 2009, pp. 65-87.
[26] P. Jamshidi, C. Pahl, N. C. Mendonça, “Pattern-based multi-cloud architecture migration”, in Software Practice and Experience 47 (9), 2017, pp. 1159–1184. DOI: 10.1002/spe.2442
[27] J. Mooney, V. Gurbaxani, K. L. Kraemer, “A Process Oriented Framework for Assessing the Business Value of Information Technology”, Forthcoming in the Proceedings of the Sixteenth Annual International Conference on Information Systems, 2001.
[28] M. Kalske, N. Mäkitalo, T. Mikkonen, “Challenges When Moving from Monolith to Microservice Architecture”, in I. Garrigós, M. Wimmer (Eds.): Current Trends in Web Engineering, Cham: Springer International Publishing, 2018, pp. 32–47.
[29] R. Khadka, A. Saeidi, S. Jansen, J. Hage, G. P. Haas, “Migrating a large scale legacy application to SOA: Challenges and lessons learned”, in 2013 20th Working Conference on Reverse Engineering (WCRE), 2013 20th Working Conference on Reverse Engineering (WCRE), Koblenz, Germany, 14/10/2013 - 17/10/2013: IEEE, 2013, pp. 425–432.
[30] M. C. Lacity, L. P. Willcocks, “What Knowledge Workers Stand to Gain from Automation”, Harvard Business Review, 2015. https://hbr. org/2015/06/what-knowledge-workersstand-to-gain-from-automation, last checked 23.08.2021
[31] S. Li, H. Zhang, Z. Jia, Z. Li, C. Zhang, J. Li et al., “A dataflow-driven approach to identifying microservices from monolithic applications”, Journal of Systems and Software 157, 2019, p. 110380. DOI: 10.1016/j.jss.2019.07.008
[32] W. Lloyd, S. Ramesh, S. Chinthalapati, L. Ly, S. Pallickara, “Serverless Computing: An Investigation of Factors Influencing Microservice Performan-
ce”, in 2018 IEEE International Conference on Cloud Engineering (IC2E), 2018 IEEE International Conference on Cloud Engineering (IC2E), Orlando, FL, 17/04/2018 - 20/04/2018: IEEE, 2018, pp. 159–169.
[33] S.-P. Ma, C.-Y. Fan, Y. Chuang, I.-H. Liu, C.-W. Lan, “Graph-based and scenario-driven microservice analysis, retrieval, and testing”, Future Generation Computer Systems, 100, 2019, pp. 724–735. DOI: 10.1016/j.future.2019.05.048
[34] S. A. Maisto, B. Di Martino, S. Nacchia, “From Monolith to Cloud Architecture Using Semi-automated Microservices Modernization”, in L. Barolli, P. Hellinckx, J. Natwichai (Eds.): Advances on P2P, Parallel, Grid, Cloud and Internet Computing, vol. 96, Cham: Springer International Publishing (Lecture Notes in Networks and Systems), 2020, pp. 638–647.
[35] A. Megargel, V. Shankararaman, D. K. Walker, “Migrating from Monoliths to Cloud-Based Microservices: A Banking Industry Example”, in M. Ramachandran, Z. Mahmood (Eds.), Software Engineering in the Era of Cloud Computing, Cham: Springer International Publishing (Computer Communications and Networks), 2020, pp. 85–108.
[36] S. Newman, “Building microservices. Designing fine-grained systems”, Sebastopol, CA: O’Reilly Media, 2015.
[37] L. Nunes, N. Santos, A. R. Silva, “From a Monolith to a Microservices Architecture: An Approach Based on Transactional Contexts” in T. Bures, L. Duchien, P. Inverardi (Eds.): Software Architecture, vol. 11681, Cham: Springer International Publishing (Lecture Notes in Computer Science), 2019, pp. 37–52.
[38] A. M. O’Brien, C. Mc Guckin, The Systematic Literature Review Method: Trials and Tribulations of Electronic Database Searching at Doctoral Level, 2016.
[39] A. J. Onwuegbuzie, N. L. Leech, K. M. T. Collins, “Qualitative Analysis Techniques for the Review of the Literature”, The Qualitative Report, 17, 2012, pp. 1–28.
[40] I. Pigazzini, F. A. Fontana, A. Maggioni, “Tool Support for the Migration to Microservice Architecture: An Industrial Case Study”, in T. Bures, L. Duchien, P. Inverardi (Eds.): Software Architecture, vol. 11681, Cham: Springer International Publishing (Lecture Notes in Computer Science), 2019, pp. 247–263.
[41] H. C. da Silva Filho, G. de Figueiredo Carneiro, “Strategies Reported in the Literature to Migrate to Microservices Based Architecture”, in Shah-
ram Latifi (Ed.): 16th International Conference on Information Technology-New Generations (ITNG 2019), vol. 800, Cham: Springer International Publishing (Advances in Intelligent Systems and Computing), 2019, pp. 575–580.
[42] H. A. Simon, “The Sciences of the Artificial, Third Edition”, 2019.
[43] D. Taibi, K. Systä, “A Decomposition and Metric-Based Evaluation Framework for Microservices”, in D. Ferguson, V. Méndez Muñoz, C. Pahl, M. Helfert (Eds.): Cloud Computing and Services Science, vol. 1218, Cham: Springer International Publishing (Communications in Computer and Information Science), 2020, pp. 133–149.
[44] J. Thones, “Microservices”, In IEEE Softw., 32(1), 2015, p. 116. DOI: 10.1109/MS.2015.11
[45] UiPath (2020): UI Task Capture: UiPath. Available online at https://www.uipath.com/product/ task-capture, checked on 5/25/2020.
[46] W. M. P. van der Aalst, M. Bichler, A. Heinzl, “Robotic Process Automation, Bus Inf Syst Eng, 60(4), 2018, S. 269–272. DOI: 10.1007/s12599018-0542-4
Submitted: 15th December 2022; accepted 20th December 2022
Oleksiy Kozlov, Yuriy Kondratenko, Hanna Lysiuk, Viktoriia Kryvda, Oksana MaksymovaDOI: 10.14313/JAMRIS/1-2022/9
Abstract:
This paper is devoted to the issues of the fuzzy automatic control of the pyrolysis process of municipal solid waste (MSW) of variable composition and moisture content. The fuzzy control method that is developed and studied makes it possible to carry out the proper automatic control of a pyrolysis plant with the determination of the optimal ratio of air/MSW for various types of waste and with different moisture content values to ensure high efficiency of the MSW disposal process. The effectiveness study of the proposed fuzzy control method is performed in this paper on a specific example, in particular, when automating the pyrolysis plant for MSW disposal with a reactor volume of 250 liters. The obtained simulation results confirm the high efficiency of the developed method, as well as the feasibility of its use for designing automatic control systems of various pyrolysis plants that operate under conditions of changes in the composition and moisture content of input waste.
Keywords: Automatic control system, Fuzzy control method, Pyrolysis plant, Municipal solid waste disposal, Variable composition, and moisture content
Environmental pollution is one of the most urgent problems of our time, caused by the rapid development of industry and the growth of urbanization in many countries of the world [1, 2]. To date, to solve this problem, there have been proposed quite a lot of different ways to dispose of municipal solid waste, which is generated by the population, in industrial enterprises, trade institutions, and municipal services. The analysis of world experience has shown that the most affordable and cost-effective method of MSW disposal is through various thermal treatments [3]. The roadmap of the European Union for the disposal of municipal waste envisages no more than 10% of municipal waste by volume being buried in landfills by 2035. The rest of the volume would be recycled or incinerated. Spiegel [4] notes that currently only 16% of plastic waste is recycled. The two-stage pyrolysis process with subsequent use of the obtained combustible substances in energy equipment is considered to be an alternative to the incineration of MSW. However,
for the successful application of this pyrolysis technology that obtains all its advantages, it is necessary to effectively control the process of waste thermal destruction in automatic mode.
Since modern equipment samples for the implementation of the two-stage pyrolysis technology are quite complicated control plants, then traditional control theory means are insufficient in most cases for adequate implementation of their reliable control [5, 6]. Additional difficulties are imposed when changing the composition of the input waste, as well as its moisture content during the operation of the pyrolysis plant. As some modern studies show, to automate complex thermal power and chemical facilities for increasing the efficiency of their operation and reliability, in many cases, it is necessary to develop new control methods [7-10]. Moreover, advanced research confirms that intelligent control principles can be applied quite effectively to automate complex plants of this type [11-13]. Namely, for the control of non-linear and non-stationary plants, the characteristics of which can be determined only approximately, and for which the parameters can change randomly, the most appropriate is the use of systems based on fuzzy logic [14-16]. The given fuzzy systems make it possible to effectively use expert information and experimental data, implement complex and flexible control and decision-making strategies with high logical transparency and interpretability, and they can also be effectively trained like neural networks based on training samples or objective functions [17-20]. Therefore, it is advisable to carry out the creation of advanced control systems for pyrolysis plants that operate under changing conditions in the composition and moisture content of the input MSW, based on the principles of fuzzy control.
This paper is devoted to the development of the method of fuzzy control for the pyrolysis process of municipal solid waste of variable composition and moisture content. The rest of the paper is organized as follows. The brief literature review of the studied area and the main purpose of this work are presented in section 2. Section 3, in turn, describes in detail the proposed method of fuzzy control of the pyrolysis process. Section 4 is an effectiveness study of the fuzzy control system for a specific pyrolysis plant designed based on the proposed method. Finally, section 5 concludes the work and suggests directions for future research.
To date, many studies have been conducted that are dedicated to various technologies for waste disposal using pyrolysis, as well as automation of installation and technological complexes for their implementation [11, 21, 22]. In turn, papers [23, 24] present various algorithmic and circuit solutions, as well as developed software and hardware tools that allow automating both separate circuits and entire pyrolysis plants for MSW disposal.
Furthermore, sufficient attention has been paid to the development and research of mathematical and simulation models of various pyrolysis plants and their separate components (reactors, circulation systems, heat exchangers, etc.) [25-28], including the use of fuzzy logic, soft computing and other intelligent techniques [29, 30]. Also, currently, a sufficient number of examples of the successful application of intelligent and other advanced algorithms and devices for the implementation of effective control of certain technological variables of waste utilizing complexes have been presented [31, 32]. In particular, fuzzy self-tuning PID [33], robust [34], model reference adaptive [35], and other types of controllers [36] have been proposed.
These control means make it possible to solve mainly individual automation tasks (stabilization of temperature mode, reactor load level, MSW feed rate), but to significantly increase the efficiency of the MSW disposal process, it is necessary to carry out a comprehensive control of the pyrolysis plant with the provision of optimal values for several indicators. Namely, it is necessary to ensure the optimal ratio of air/MSW and the optimal temperature mode to supply the required flow rate of the output pyrolysis gas to the consumer with the maximum calorific value [37]. This problem becomes much more complicated when the composition and moisture content of the input raw materials changes [38]. The use of any type
of extremal controller does not allow determining the optimal air/MSW ratio quite accurately due to large errors in determining the measured parameter when approaching the extremum point [39-41]. Additional difficulties are also imposed due to the possibility of only an approximate determination of the percentage ratio of certain components in the composition of raw materials. Thus, to successfully solve this problem, it is necessary to create a unified method for controlling the pyrolysis process of MSW of variable composition and moisture content based on fuzzy logic.
The main aim of this work is the development and research of the method of fuzzy control for the pyrolysis process of municipal solid waste of variable composition and moisture content.
Before presenting the main aspects of the proposed fuzzy control method, it is advisable to consider a brief description of the operation features, and main control tasks of the generalized pyrolysis plant used for MSW thermal disposal.
Figure 1 shows a 3D model (a) and a schematic diagram (b) of the generalized pyrolysis plant, which is used for the thermal disposal of municipal solid waste.
The pyrolysis plant shown in Fig. 1 operates as follows [37]. The MSW with the flow rate G1 is supplied to the reactor inlet through collector I. In turn, the required amount of air with the flow rate Q1 is fed through the collector IV, to carry out the pyrolysis process in a certain mode. The main pyrolysis process takes place in reactor III, as a result of which the py-
rolysis gas is produced with the flow rate Q2, which exits through collector II at the outlet of the reactor. Furthermore, the generated pyrolysis gas is divided into 2 streams: with flow rate Q4, which is supplied to the consumer; with flow rate Q3, which enters through the recirculation line to the inlet V to the reactor for the MSW drying and preheating. In addition, the gasholder VI is installed after the pyrolysis plant, which makes it possible to ensure the presence of a buffer capacity for smoothing the pyrolysis gas flow in the event of sudden changes in its consumption.
Thus, in the stationary operation mode, the presented pyrolysis plant gives the opportunity to completely gasify the organic component of the MSW with a defined composition, while calculating the minimum required amount of air and regulating its supply. For the most efficient processing of waste and the production of pyrolysis gas with the highest calorific value, it is necessary to ensure the supply of such an amount of air that the ratio of air/MSW is optimal. Moreover, the optimal value of this ratio significantly depends on the type of waste being processed. In turn, the considered pyrolysis plant (Fig. 1) can process 4 main types of MSW: 1) hydrocarbon (СНm); 2) hydrocarbon that contains oxygen (СНnОf); 3) hydrocarbon that contains oxygen and nitrogen (СНwОqNk) and 4) hydrocarbon that contains active elements that form “sour gases” (СНaFx, СНbCly, СНdSz) [37]. However, since in practice the exact sorting of waste is rather difficult, any one type of MSW from the above may contain small impurities of MSW of other types. For example, incoming waste may consist of more than 90% of the first type and up to 10% in total of the second, third and fourth types. There may also be mixtures between the main composition of some two types of MSW and small impurities of two other types. For example, such an MSW mixture may consist of more than 45% of the first type, more than 45% of the third type, and up to 10% in total of the second and fourth types. Also, the efficiency of the disposal process is significantly affected by the MSW moisture content for some of their respective types and their mixtures.
To ensure high efficiency of waste disposal, it is necessary to carry out proper automatic control of the pyrolysis process with the determination of the optimal ratio of air/MSW for various ratios of the 4 types of considered MSW that have different moisture content values.
Thus, the following main tasks of automatic control of this pyrolysis plant can be distinguished:
1) control of MSW G1 and air Q1 supply to ensure the production of the required amount of pyrolysis gas Q4 for the consumer;
2) control of the air/MSW ratio α for the MSW of variable composition and moisture content to maximize the calorific value Qir of the obtained pyrolysis gas;
3) stabilization of the required temperature mode of the pyrolysis process;
4) stabilization of the required level value LG in the gasholder.
To implement the above tasks of multi-connected control for the pyrolysis plant, it is necessary to apply
the highly efficient fuzzy control method developed by the authors, which is presented in the next subsection.
In the stationary operation mode of the pyrolysis plant, the amount of the produced pyrolysis gas Q4 is defined by the sum of the flow rate values of MSW and air that are fed to the reactor [38]:
Q4 = G1 + Q1 (1)
Thus, to ensure the desired value of the pyrolysis gas flow Q4 required by the consumer, it is necessary to control the MSW supply G1 and air flow Q1 in such a way that equation (1) is fulfilled.
At the same time, for the optimal disposal of waste of various compositions, a certain ratio between the MSW supply G1 and air flow Q1 must also be observed. To do this, it is advisable to introduce a specific coefficient Kα that will determine the consumption of MSW and air, depending on the required amount of pyrolysis gas Q4 at the outlet. Using the coefficient Kα, the equation (1) takes the form
Q4 = G1 + Q1 = Q4kα + Q4(1 – kα), (2)
where Kα theoretically can take values from 0 to 1.
Thus, according to the proposed method, based on equation (2), the required values of the flow rate of MSW and air are calculated using equations (3) and (4), respectively:
G1 = Q4kα; (3)
Q1 = Q4(1 – kα). (4)
Moreover, the specific coefficient Kα correlates with the value of the air/MSW ratio α as follows
k 1 1 . (5)
Since for the effective implementation of the pyrolysis process of the organic waste, the ratio α must be in the range from 0.3 to 0 [38], depending on the type of MSW, then the coefficient Kα can vary from 0.769 to 1 following the equation (5).
Thus, to solve the first two tasks of automatic control of the pyrolysis plant, the control signals for the flow of MSW uG1 and air uQ1 should be calculated using equations (6) and (7), as follows:
uG1 = uQ4kα; (6)
uQ1 = uQ4(1 – kα), (7)
where uQ4 is the signal corresponding to the set value of the pyrolysis gas flow Q4 required by the consumer.
In turn, the signal uQ4 should be set by the operator of the control system at the upper level. The optimal values of the coefficient Kα should be calculated for MSW of certain compositions and depend on the moisture content, based on the mathematical model of the pyrolysis process developed in the paper [38].
The implementation of such control actions based on equations (6) and (7) will allow effective control of the pyrolysis plant in a stationary mode. In turn, to ensure the efficient automation of the pyrolysis process in transient modes, it is necessary to solve tasks 3 and 4 in addition to the 1st and 2nd control tasks.
To stabilize the temperature mode of the pyrolysis process (task 3), it is necessary to carry out additional control of the air flow in the transient modes.
In turn, to stabilize the required level value LG in the gasholder (task 4), it is necessary to carry out additional control of the MSW supply to the reactor in the transient modes.
Thus, equations (6) and (7) will take the form:
uu ku uk f L G1 Q4 LC Q4 (); (8)
uu ku uk f T Q1 Q4 TC Q4 () () (), 11 (9)
where uLC and uTC are the control signals of the level controller (LC) and the temperature controller (TC) that should stabilize the required level value LG in the gasholder and the reactor temperature in the transient modes; εL is the level control error, which is determined by the deviation of the real level value LR in the gasholder from the set one LGS, εL = LGS – LR; εT is the temperature control error, which is determined by the deviation of the real temperature value TR in the reactor from the set one TS, εT = TS – TR.
The set value of the gasholder level LGS should be set by the operator of the control system at the upper. The temperature value TS must be set in such a way that it corresponds to the optimal value of the coefficient Kα, depending on the MSW composition and moisture content.
The specific coefficient Kα should be determined in such a way as to provide the maximum possible value of the calorific value Qir of the obtained pyrolysis gas and, at the same time, the highest temperature T in the reactor to intensify the pyrolysis process.
In the stationary mode of the pyrolysis plant operation, the calorific value Qir of the obtained pyrolysis gas and the temperature T of the pyrolysis process, depending on the MSW supply G1 and air flow Q1, can be calculated based on expressions (10) and (11), respectively [38]:
MSW. kC, kG, and kQ are the proportionality coefficients, which depend on the MSW’s specific composition and its moisture content, kf
kf
where QW1, QW2, QW3, QW4 are the percentage of the 1st, 2nd, 3rd, and 4th types of MSW in the mixture; hW is the MSW moisture content wherein,
QQQ Q WWW W 12 34 100%. (15)
In turn, the dependencies (12)-(14) are determined using the mathematical model presented in [38]. For example, for the case in which the mixture consists of 100% waste of the 2nd type with 15% moisture content (QW1 = 0%; QW2 = 100%; QW3 = 0%; QW4 = 0%; hW = 15%), the coefficients take the following values: kC = 0.27; kG = 300; kQ = 570.
As can be seen from equations (10) and (11), the calorific value Qir of the resulting pyrolytic gas increases when decreasing the air flow Q1 in the pyrolysis process, and the maximum value Qir� can be achieved at Q1 = 0. However, with a decrease in air flow, the temperature T in the reactor also decreases, and with its zero value (Q1 = 0), there will not be enough energy to carry out the pyrolysis process. Therefore, it is advisable to choose the value of the coefficient Kα in such a way that a compromise is observed between the calorific value Qir and the temperature T of the pyrolysis process. It is advisable to introduce the objective function J (16), the optimal value of which will correspond to the optimal value of the coefficient Kα for a certain composition of the MSW and its moisture content.
JQ K TK T i r () () max, max ± ± 03 (16)
where Tmax is the maximum temperature value corresponding to the maximum values of the flow rate of MSW and air for a certain composition and moisture content.
To find the coefficient Kα corresponding to the optimal value of the objective function (16) for a certain MSW composition and moisture content, it is advisable to use the mathematical model of the pyrolysis process given in the paper [38]. So, for example, for the composition described above (QW2 = 100%; hW = 15%), the calculated dependence of the objective function J (16) on the coefficient Kα has the form shown in Fig. 2.
where Qir� is the calorific value of the MSW; V0 is the volume of air required for the pyrolysis of 1 mol of
As can be seen from the graph in Fig. 2, the optimal value of the coefficient Kαopt for this MSW composition and moisture content is equal to 0.81. In turn, the temperature set value TS that corresponds to the given optimal value of the coefficient Kα is equal to 703 °C.
Fig.
The mathematical model of the pyrolysis process [38], on which the optimal value of the objective function is calculated, includes the equations of chemical kinetics and requires significant computational resources and time costs to determine the required values of the coefficient Kα. This causes certain difficulties when using it in the real-time mode in the automation system of the pyrolysis plant in the control process. Therefore, it is advisable to perform a preliminary calculation of the optimal values of the coefficient Kα and temperature TS for the main possible compositions of MSW and to use the given values in the control process. Since the composition of the waste can only be determined approximately, it is advisable to use a fuzzy subsystem to approximate the preliminary calculated data, which will determine the
values of the coefficient Kα and temperature TS at various percentages of waste and moisture content.
Thus, taking into account all of the above, for the implementation of the proposed fuzzy control method, the automatic control system of the pyrolysis plant will have the structure presented in Fig. 3.
In turn, Fig. 3 adopts the following designations: UCL is the upper-level control, SD is the setting device, FSS is the fuzzy subsystem, TS is the temperature sensor, LS is the level sensor, uLS and uTS are signals that correspond to the set values of level LGS and temperature TS, and uLR and uTR are signals that correspond to the real values of level LR and temperature TR, measured by the sensors LS and TS.
To determine the optimal values of the coefficient Kα and set temperature TS in this system, a fuzzy subsystem of the Takagi-Sugeno type is used, which implements the following dependencies:
Kf QQQ Qh (, ,, ,); WWW WW 12 34 (17)
Tf QQQ Qh S WWW WW = (, ,, ,) 12 34 (18)
Further, in subsection 3.3, the development of the fuzzy subsystem is presented, which determines the optimal values of the coefficient Kα and temperature TS of the pyrolysis process, depending on the composition of MSW and moisture content.
The fuzzy subsystem of the Takagi-Sugeno type presented here has 5 input variables (QW1; QW2; QW3; QW4; hW) and 2 output variables (Kα; TS). To fuzzify the first four input variables (QW1; QW2; QW3; QW4), 3 linguistic terms are used: S – small; A – average; L –large. For the fuzzification of the fifth input variable hW, 4 terms are used: L – low; A– average; AA– above average; H – high.
In turn, for all linguistic terms, a triangular membership function is chosen. The appearance of the given linguistic terms is shown in Fig. 4.
1, 2, 3, 4)
Taking into account all possible combinations of the presented linguistic terms, the maximum number of rules of the rule base (RB) can be equal to 324. However, after analyzing all the actual operating conditions of the pyrolysis plant (taking into account condition (15) and the fact that not all types of MSW can have any percentage of moisture) it was determined that the number of rules is 31.
In general form, the r-th rule of this RB is represented by the expression:
where AW1, AW2, AW3, AW4, and A1 are the certain linguistic terms of the FSS input variables, and Kαr and TSr are the certain values of the output variables Kα and TS for the r-th rule (r = 1, 2, …, 31).
In turn, the developed rule base of the FSS is presented in Table 1.
The values of the output variables Kα and TS for each RB rule (Table 1) are determined in the optimization process using the objective function (16) and the mathematical model of the pyrolysis process given in the paper [38].
Moreover, for this fuzzy subsystem, the “min” operation is used as an aggregation operation, and the discrete gravity center method is used as a defuzzification method.
The effectiveness study of the proposed fuzzy control method is performed in this work on a specific example, in particular, when automating the pyrolysis plant for the MSW of variable composition with a reactor volume of 250 liters.
The efficiency of the proposed fuzzy control method is studied when automating the pyrolysis plant with the reactor volume of 250 liters in a transient mode.
The filling of the gasholder occurs when there is a difference ΔQG between the consumption of consumed pyrolysis gas and the supplied MSW and air. In turn,
G114 . QGQQ ∆=+− (20)
To simulate transients of the gasholder filling for the pyrolysis plant with a given volume, it is advisable to use the following transfer function [37]
3
The Fig. 5 (a, b) shows the transients graphs when controlling the temperature of the pyrolysis process and the level in the gasholder for the following composition of MSW and moisture content: QW1 = 44%; QW2 = 47%; QW3 = 4%; QW4 = 5%; hW = 20%.
R LG G
()5 () . ()(501) s Lse Ws Qsss == ∆+ (21)
To simulate transients of temperature T change and calorific value Qir of the pyrolysis process for the plant with a given volume, it is advisable to use the following transfer functions [37]:
3
R TR () () ; ()(501) s Tse Ws Tss == + (22)
3
Fig. 5. Transients graphs of controlling: (a) temperature; (b) level in the gasholder (QW1 = 44%; QW2 = 47%; QW3 = 4%; QW4 = 5%; hW = 20%)
r s i r i
R QR () () , ()(501)
Qs e Ws Qss == + (23)
where TR and R rQi are the real values of temperature and calorific value in the transient mode, and T and Qir are the values of temperature and calorific value in the stationary mode calculated by the equations (11) and (10) respectively.
In this case, PD controllers are used as the level (LC) and temperature (TC) controllers for the pyrolysis plant control system, which have transfer functions:
Also, for this composition, the graphs of transients for changing the calorific value Qir of the obtained pyrolysis gas and the objective function (16) are presented in Fig. 6 (a, b). Herewith, using the fuzzy system, the required temperature value TS was set at 868°C, which is optimal for the given waste composition and moisture content.
The specified value of the gasholder filling level LGS was set in relative units as 0.5 of the maximum level value.
us Wskks s ε ==+ (24)
LC LC PLDL () () ; ()
L
Moreover, in Fig. 6 the calorific value Qir of the obtained pyrolysis gas is given in relative units from the maximum value, corresponding to the given composition of the MSW (Qir = 0.986). Also, for the given waste composition and moisture content, the optimal value of the objective function J is 1.224.
TC TC PTDT () () , ()
us Wskks s ==+ ε (25)
T
where kPL, kDL, kPT and kDT are the controllers gains that are found in the process of parametric optimization, kPL = 48.8; kDL = 43.4; kPT = 0.015; kDT = 0.054.
Further, for the developed control system, based on the proposed method and using the presented transfer functions, the computer simulation of the pyrolysis plant operation for the various compositions, including moisture content, of the MSW is carried out.
Further, the similar graphs of transients were obtained for a completely different composition of MSW and moisture content: QW1 = 6%; QW2 = 45%; QW3 = 7%; QW4 = 42%; hW = 2.5%. In turn, Fig. 7 (a, b) shows the transients graphs for controlling the temperature T of the pyrolysis process and the level LR in the gasholder, and the Fig. 8 (a, b) presents the graphs of transients for changing the calorific value Qir of the obtained pyrolysis gas and the objective function (16).
For the given MSW composition and moisture content, the optimal values of the temperature TS and ob-
Fig. 6. Transients graphs for changing: (a) calorific value;
(b) objective function (16) (QW1 = 44%; QW2 = 47%; QW3 = 4%; QW4 = 5%; hW = 20%)
Fig.
Transients graphs for changing: (a) calorific value; (b) objective function (16) (QW1 = 6%; QW2 = 45%; QW3 = 7%; QW4 = 42%; hW = 2.5%)
Fig.
Fig.
graphs of controlling: (a) temperature; (b) level in the gasholder (QW1 = 6%; QW2 = 45%; QW3 = 7%; QW4 = 42%; hW = 2.5%)
graphs of controlling: (a) temperature; (b) level in the gasholder (Changing of MSW composition and moisture content during the operation of the plant)
jective function J are 720 °C and 1.238, respectively. Moreover, the calorific value Qir of the obtained pyrolysis gas is equal to 0.99 (Qir = 0.99).
As can be seen from Fig. 5-8, the developed system makes it possible to effectively control the pyrolysis plant under conditions of variable waste composition and moisture content. At the same time, all 4 tasks of automatic control, set in Subsection 3.1, are successfully solved.
The production of the required amount of the pyrolysis gas with the optimal value of the calorific value Qir is ensured due to the effective control of the coefficient Kα, which determines the supply of MSW and the air flow rate. Also, stabilization of the required level value in the gasholder and the optimal temperature value, which corresponds to a certain composition and moisture content of the input waste, is carried out. When controlling these interrelated variables (T and LG), sufficiently high-quality indicators are provided in particular, zero overshoot and static error with short enough regulating time (tr = 81 s for the 1st case; tr = 92 s for the 2nd case) for temperature control of the pyrolysis process. Also, 44% maximum overshoot and zero static error with short enough regulating time (tr = 69 s for the 1st case; tr = 73 s for the 2nd case) is required to control the gasholder level.
In the two cases considered the composition of MSW and moisture content were established before the start of the pyrolysis plant and did not change during its operation. For a more detailed study of the developed system and the proposed fuzzy control method, we further consider the case in which the composition and moisture content of the input waste changes during the operation of the plant. Fig. 9 (a, b) shows the transients graphs for controlling the temperature of the pyrolysis process and the level in the gasholder.
In turn, these graphs are obtained when studying the case of changing the input MSW composition during the plant operation.
Also, the graphs of transients of changing the calorific value Qir of the obtained pyrolysis gas and the objective function (16) for this case are presented in Fig. 10 (a, b).
Initially, before the plant was launched, the composition and moisture content of the MSW were as follows: QW1 = 45%; QW2 = 7%; QW3 = 46%; QW4 = 2%; hW = 35%. For the given composition and moisture content, the optimal values of the temperature T1opt and objective function J1opt are 956 °C and 1.242, respectively.
Then, during the operation of the pyrolysis plant at the moment of time t = 300 s, the composition and moisture content of the waste changed in the following way: QW1 = 5%; QW2 = 90%; QW3 = 3%; QW4 = 2%; hW = 0.5%. Herewith, the optimal values of the temperature T2opt and objective function J2opt are 738 °C and 1.228, respectively, for the given MSW composition and moisture content.
The obtained results of computer simulation in the form of graphs of transients (Fig. 9, 10) show that the system also allows quite effectively control of the pyrolysis process with changes in the composition and
moisture content of the waste during the operation of the plant. Herewith, at the moment of time t = 300 s, when changing the composition and moisture content, the level value deviated by no more than 40% for up to 48 s. At the same time, for the temperature control channel, at the moment of changing the MSW composition and moisture content (t = 300 s), the transient was aperiodic with the regulating time of 48.
Thus, the results obtained confirm the high efficiency of the proposed fuzzy control method and the feasibility of its application for the development of automatic control systems for various pyrolysis plants that operate under conditions of changes in the composition and moisture content of input waste.
The development and research for a method of fuzzy control for the pyrolysis process of municipal solid waste of variable composition and moisture content are presented in this paper. The obtained method makes it possible to carry out the proper automatic control of the pyrolysis plant with the determination of the optimal ratio of air/MSW for various types of waste and with different moisture content values to ensure the high efficiency of the MSW disposal process. Moreover, using this method, the tasks of stabilizing such interrelated controlled coordinates as the temperature of the pyrolysis process and the gasholder level in transients are successfully solved.
To evaluate the effectiveness of the proposed method, the development of the fuzzy control system for the pyrolysis plant for the MSW of variable composition with the reactor volume of 250 liters is carried out in this study. In particular, for detailed research, the computer simulation of the pyrolysis plant operation is performed for 4 various compositions and moisture content of the MSW: 1) QW1 = 44%; QW2 = 47%; QW3 = 4%; QW4 = 5%; hW = 20%; 2) QW1 = 6%; QW2 = 45%; QW3 = 7%; QW4 = 42%; hW = 2.5%; 3) QW1 = 45%; QW2 = 7%; QW3 = 46%; QW4 = 2%; hW = 35%; 4) QW1 = 5%; QW2 = 90%; QW3 = 3%; QW4 = 2%; hW = 0.5%. In addition, the case in which the composition and moisture content of the input waste changed during the operation of the plant is also studied.
The obtained computer simulation results confirm the high efficiency of the developed system and the fuzzy control method, which allows solving all 4 tasks of automatic control (set in Subsection 3.1) of the pyrolysis plant under conditions of variable MSW composition and moisture content. Namely, the production of the required amount of the pyrolysis gas with the optimal value of the calorific value Qir is ensured due to the effective fuzzy control of the coefficient Kα, which determines the flow rate of the MSW and air. The stabilization of the optimal temperature value, which corresponds to a certain composition and moisture content of the input waste and the required level value in the gasholder, is performed with sufficiently high-quality indicators.
In further research, we plan to consider the issues of parametric and structural optimization of the proposed fuzzy subsystem for determining the coefficient Kα, as well as develop fuzzy level and temperature controllers to improve the quality indicators of the pyrolysis plant control.
Oleksiy Kozlov* – Department of Intelligent Information Systems, Petro Mohyla Black Sea National University, Mykolaiv, Ukraine, 54003, e-mail: kozlov_ov@ukr.net.
Yuriy Kondratenko – Department of Intelligent Information Systems, Petro Mohyla Black Sea National University, Mykolaiv, Ukraine, 54003, e-mail: y_kondrat2002@yahoo.com.
Hanna Lysiuk – Department of software and computer-integration technologies, Odesa Polytechnic National University Odesa, Ukraine, 65044, e-mail: lysyukann@gmail.com.
Viktoriia Kryvda – Department of Power Supply and Energy Management, Odesа Polytechnic National University, Odesа, Ukraine, 65044, e-mail: kryvda@op.edu.ua.
Oksana Maksymova – Scientific Center of the Naval Institute, Odesa Maritime National University Odesa, Ukraine, 65029, e-mail: m.oxana.b@gmail.com.
*Corresponding author
[1] A. Tozlu, E. Ozahi, A. Abusoglu, “Waste to energy technologies for municipal solid waste management in Gaziantep”, Renewable and Sustainable Energy Reviews, Vol. 54, 2016, 809-815. DOI:10.1016/j.rser.2015.10.097
[2] R. Kothari, V. Tyagi, F. Pathak, “Waste to energy: A way from renewable energy sources to sustainable development”, Renewable and Sustainable Energy Reviews, Vol. 14, № 9, 2010, 31643170. DOI:10.1016/j.rser.2010.05.005
[3] C. Guizani, et al., “Biomass Chars: The Effects of Pyrolysis Conditions on Their Morphology, Structure, Chemical Properties and Reactivity”, Energies, 10(6), 2017, 796. DOI:10.3390/ en10060796
[4] “Nur 16 Prozent des Plastikmülls werden wiederverwendet”, Newspaper website Spiegel, 2019. https://www.spiegel.de/wissenschaft/ natur/plastikmuell-nur-16-prozent-werden-indeutschland-wiederverwendet-a-1271125.html
[5] Y. Li, R. Gupta, S. You, “Machine learning assisted prediction of biochar yield and composition via pyrolysis of biomass”, Bioresource Technology, Vol. 359, 2022, 127511. DOI:10.1016/j.biortech.2022.127511
[6] S. Wu, et al., “Simulation and optimization of heating rate and thermal uniformity of microwave reactor for biomass pyrolysis”, Chemical Engineering Science, Vol. 250, 2022, 117386. DOI:10.1016/j.ces.2021.117386
[7] S.N. Pelykh, M.V. Maksimov, M.V. Nikolsky, “A method for minimization of cladding failure parameter accumulation probability in VVER fuel elements”, Problems of Atomic Science and Technology, 92(4), 2014, 108-116. https://www.researchgate. net/publication/289947827_A_method_for_minimization_of_cladding_failure_parameter_accumulation_probability_in_VVER_fuel_elements
[8] S.N. Pelykh, M.V. Maksimov, “The method of fuel rearrangement control considering fuel element cladding damage and burnup”, Problems of Atomic Science and Technology, 87(5), 2013, 84-90. https://vant.kipt.kharkov.ua/ARTICLE/ VANT_2013_5/article_2013_5_84a.pdf
[9] M.V. Maksimov, S.N. Pelykh, R.L. Gontar, “Principles of controlling fuel-element cladding lifetime in variable VVER-1000 loading regimes”, Atomic Energy, 112(4), 2012, 241-249. DOI:10.1007/ s10512-012-9552-3
[10] I. Atamanyuk, J. Kacprzyk, Y. Kondratenko, M. Solesvik, “Control of Stochastic Systems Based on
[11]
the Predictive Models of Random Sequences”, In: Y.P. Kondratenko, A.A. Chikrii, V.F. Gubarev, J. Kacprzyk (Eds) Advanced Control Techniques in Complex Engineering Systems: Theory and Applications. Dedicated to Professor Vsevolod M. Kuntsevich. Studies in Systems, Decision and Control, Vol. 203. Cham: Springer Nature Switzerland AG, 2019, 105-128. DOI: 10.1007/9783-030-21927-7_6
O. Kozlov, G. Kondratenko, Z. Gomolka, Y. Kondratenko, “Synthesis and Optimization of Green Fuzzy Controllers for the Reactors of the Specialized Pyrolysis Plants”, Kharchenko V., Kondratenko Y., Kacprzyk J. (Eds) Green IT Engineering: Social, Business and Industrial Applications, Studies in Systems, Decision and Control, Vol 171, 2019, Springer, Cham, 373-396. DOI:10.1007/978-3030-00253-4_16
[18] W. Pedrycz, K. Li, M. Reformat, “Evolutionary reduction of fuzzy rule-based models”, Fifty Years of Fuzzy Logic and its Applications, STUDFUZ 326, Cham: Springer, 2015, 459-481. DOI:10.1007/978-3-319-19683-1_23
[19] N. Ben, S. Bouallègue, J. Haggège, “Fuzzy gains-scheduling of an integral sliding mode controller for a quadrotor unmanned aerial vehicle”, Int. J. Adv. Comput. Sci. Appl., Vol. 9, no. 3, 2018, 132141. DOI: 10.14569/IJACSA.2018.090320
[12]
Y.P. Kondratenko, O.V. Kozlov, O.V. Korobko, “Two Modifications of the Automatic Rule Base Synthesis for Fuzzy Control and Decision Making Systems”, J. Medina et al. (Eds), Information Processing and Management of Uncertainty in Knowledge-Based Systems: Theory and Foundations, 17th International Conference, IPMU 2018, Cadiz, Spain, Proceedings, Part II, CCIS 854, Springer International Publishing AG, 570-582, 2018. DOI:10.1007/978-3-319-91476-3_47
[13] Y.P. Kondratenko, A.V. Kozlov, “Generation of Rule Bases of Fuzzy Systems Based on Modified Ant Colony Algorithms”, Journal of Automation and Information Sciences, Vol. 51, Issue 3, 2019, New York: Begel House Inc., 4-25. DOI: 10.1615/ JAutomatInfScien.v51.i3.20
[14] “Advance trends in soft computing”, M. Jamshidi, V. Kreinovich, J. Kacprzyk, Eds. Cham: Springer-Verlag, 2013. DOI:10.1007/978-3-319-03674-8
[15] Y.P. Kondratenko, O.V. Korobko, O.V. Kozlov, “Synthesis and Optimization of Fuzzy Controller for Thermoacoustic Plant”, Lotfi A. Zadeh et al. (Eds.) Recent Developments and New Direction in Soft-Computing Foundations and Applications, Studies in Fuzziness and Soft Computing, Vol. 342, 2016, Berlin, Heidelberg: Springer-Verlag, 453467. DOI:10.1007/978-3-319-32229-2_31
[16] J. Zhao, et al., “The fuzzy PID control optimized by genetic algorithm for trajectory tracking of robot arm”, 2016 12th World Congress on Intelligent Control and Automation (WCICA), Guilin, China, 2016, 556-559. DOI: 10.1109/WCICA.2016.7578443
[17] J. Kacprzyk, “Multistage Fuzzy Control: A Prescriptive Approach”, John Wiley & Sons, Inc., New York, NY, USA, 1997.
[20] J. Kacprzyk, Y. Kondratenko, J. M. Merigo, J. H. Hormazabal, G. Sirbiladze, A. M. Gil-Lafuente, “A Status Quo Biased Multistage Decision Model for Regional Agricultural Socioeconomic Planning Under Fuzzy Information”, In: Y.P. Kondratenko, A.A. Chikrii, V.F. Gubarev, J. Kacprzyk (Eds) Advanced Control Techniques in Complex Engineering Systems: Theory and Applications. Dedicated to Professor Vsevolod M. Kuntsevich. Studies in Systems, Decision and Control, Vol. 203. Cham: Springer Nature Switzerland AG, 2019, 201-226. DOI: 10.1007/978-3-030-21927-7_10
[21] D. Ghosh, S. K. Bandyopadhyay, G. S. Taki, “Green Energy Harvesting from Waste Plastic Materials by Solar Driven Microwave Pyrolysis,” 2020 4th International Conference on Electronics, Materials Engineering & Nano-Technology (IEMENTech), 2020, 1-4. DOI: 10.1109/IEMENTech51367.2020.9270122
[22] A.J. Bowles, G.D. Fowler, “Assessing the impacts of feedstock and process control on pyrolysis outputs for tyre recycling”, Resources, Conservation and Recycling, Vol. 182, 2022, 106277. DOI:10.1016/j.resconrec.2022.106277
[23] B. Zhang, D. -L. Xu, X. -D. Hu, Y. Liu, “Automatic control system of biomass pyrolysis gas carbon compound furnace based on PLC”, 2020 3rd World Conference on Mechanical Engineering and Intelligent Manufacturing (WCMEIM), 2020, 435-442. DOI: 10.1109/WCMEIM52463.2020.00098
[24] Y . P. Kondratenko, O. V. Kozlov, O. S. Gerasin, A. M. Topalov, O. V. Korobko, “Automation of control processes in specialized pyrolysis complexes based on Web SCADA Systems”, Intelligent Data Acquisition and Advanced Computing Systems: Technology and Applications (IDAACS): Proceedings of the 9th IEEE International Conference. Bucharest, Romania, volume 1, 2017, 107-112. DOI: 10.1109/IDAACS.2017.8095059
[25] Z. Fu, J. Wang and C. Yang, “Research on heat transfer function modeling of plastic waste pyrolysis gasification reaction kettle,” 2017 Chinese Automation Congress (CAC), 2017, pp. 26982701, DOI: 10.1109/CAC.2017.8243233
[26] Y.P. Kondratenko, O.V. Kozlov, “Mathematic Modeling of Reactor’s Temperature Mode of Multiloop Pyrolysis Plant”, Modeling and Simulation in Engineering, Economics and Management, Lecture Notes in Business Information Processing, Vol. 115, 2012, 178-187. DOI:10.1007/9783-642-30433-0_18
[27] J. Hofmann, H. Holz, L. Gröll, “Relative Gain Array and Singular Value Analysis to Improve the Control in a Biomass Pyrolysis Process”, 2019 IEEE 15th International Conference on Control and Automation (ICCA), 2019, 596-603, DOI: 10.1109/ICCA.2019.8900025
[28] D. V. Tuntsev, et al., “The mathematical model of fast pyrolysis of wood waste”, 2015 International Conference on Mechanical Engineering, Automation and Control Systems (MEACS), 2015, 1-4, DOI: 10.1109/MEACS.2015.7414929
[29] Y.P. Kondratenko, O.V. Kozlov, L.P. Klymenko, G.V. Kondratenko, “Synthesis and Research of Neuro-Fuzzy Model of Ecopyrogenesis Multi-circuit Circulatory System”, Advance Trends in Soft Computing, Studies in Fuzziness and Soft Computing, Berlin, Heidelberg: Springer-Verlag, Vol. 312, 2014, 1-14. DOI:10.1007/978-3-31903674-8_1
[30] Y.P. Kondratenko, O.V. Kozlov, “Mathematical Model of Ecopyrogenesis Reactor with Fuzzy Parametrical Identification”, Recent Developments and New Direction in Soft-Computing Foundations and Applications, Studies in Fuzziness and Soft Computing, Vol. 342, Lotfi A. Zadeh et al. (Eds.). Berlin, Heidelberg: Springer-Verlag, 2016, 439-451. DOI:10.1007/978-3-319-32229-2_30
[31] F. S. Tudor, F. M. Boangiu, C. Petrescu, “First order controller for a petrochemical pyrolysis reactor”, 2nd International Conference on Systems and Computer Science, 2013, 20-25, DOI: 10.1109/ IcConSCS.2013.6632017
[32] Q. Bu et al. “The effect of fuzzy PID temperature control on thermal behavior analysis and kinetics study of biomass microwave pyrolysis”, Journal of Analytical and Applied Pyrolysis, Vol. 158, 2021, 105176. https://doi.org/10.1016/ j.jaap.2021.105176
[33] X. Liu, S. Wang, L. Xing, “Fuzzy self-tuning PID temperature control for biomass pyrolysis flu-
idized bed combustor”, 2010 2nd IEEE International Conference on Information Management and Engineering, 2010, 384-387. DOI: 10.1109/ ICIME.2010.5477837
[34] M. Mircioiu, E. -M. Cimpoeşu, C. Dimon, “Robust control and optimization for a petrochemical pyrolysis reactor”, 18th Mediterranean Conference on Control and Automation, MED’10, 2010, 1097-1102, DOI: 10.1109/MED.2010.5547645
[35] P. Cristina, P. Alexandru, “Improving FCC plant performance with model reference adaptive control based on neural network”, 2016 8th International Conference on Electronics, Computers and Artificial Intelligence (ECAI), 2016, 1-4. DOI: 10.1109/ECAI.2016.7861074
[36] D. Popescu, C. Petrescu, C. Dimon, M. Boangiu, “Control and optimization for a petrochemical reactor”, 2nd International Conference on Systems and Computer Science, 2013, 14-19. DOI: 10.1109/IcConSCS.2013.6632016
[37] M.V. Maksymov, et al., “Automatic Control for the Slow Pyrolysis of Organic Materials with Variable Composition”, in Advanced Control Systems: Theory and Applications. Series in Automation, Control and Robotics River Publishers, Y.P. Kondratenko et al. (Eds.), Chapter 14, 2021, 397430. ISBN:978-87-7022-341-6
[38] O. Brunetkin, et al., “Development of the unified model for identification of composition of products from incineration, gasification, and slow pyrolysis”, EasternEuropean Journal of Enterprise Technologies, 4/6 (100), 2019, 25–31. DOI: 10.15587/1729-4061.2019.176422
[39] V.P. Sabanin, et al., “Load control and the provision of the efficiency of steam boilers equipped with an extremal governor”, Therm. Eng. 61, 2014, 905-910. DOI:10.1134/S004060151411007X
[40] Y.M. Kovrigo, T.G. Bagan, A.S. Bunke, “Securing robust control in systems for closed-loop control of inertial thermal power facilities”, Therm. Eng. 61, 2014, 183–188. DOI:10.1134/ S0040601514030057
[41] S.A. Morales, D.R. Barragan, V. Kafarov, “3D CFD Simulation of Combustion in Furnaces Using Mixture Gases with Variable Composition”, Chemical Engineering Transactions, Vol. 70, 2018, 121126. DOI: 10.3303/CET1870021