WWW.JAMRIS.ORG pISSN 1897-8649 (PRINT)/eISSN 2080-2145 (ONLINE)
Indexed in SCOPUS
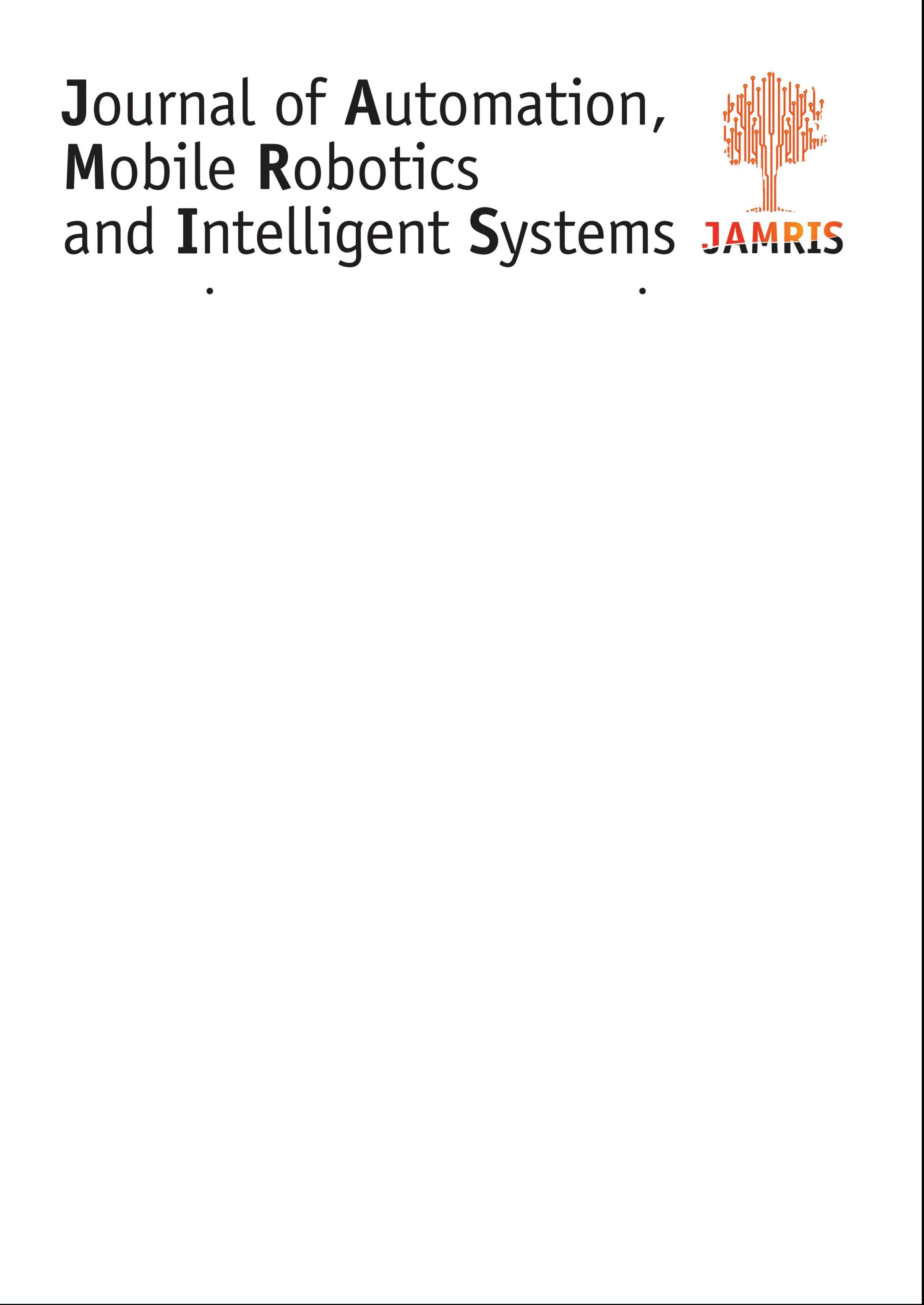
VOLUME 18, N° 1, 2024
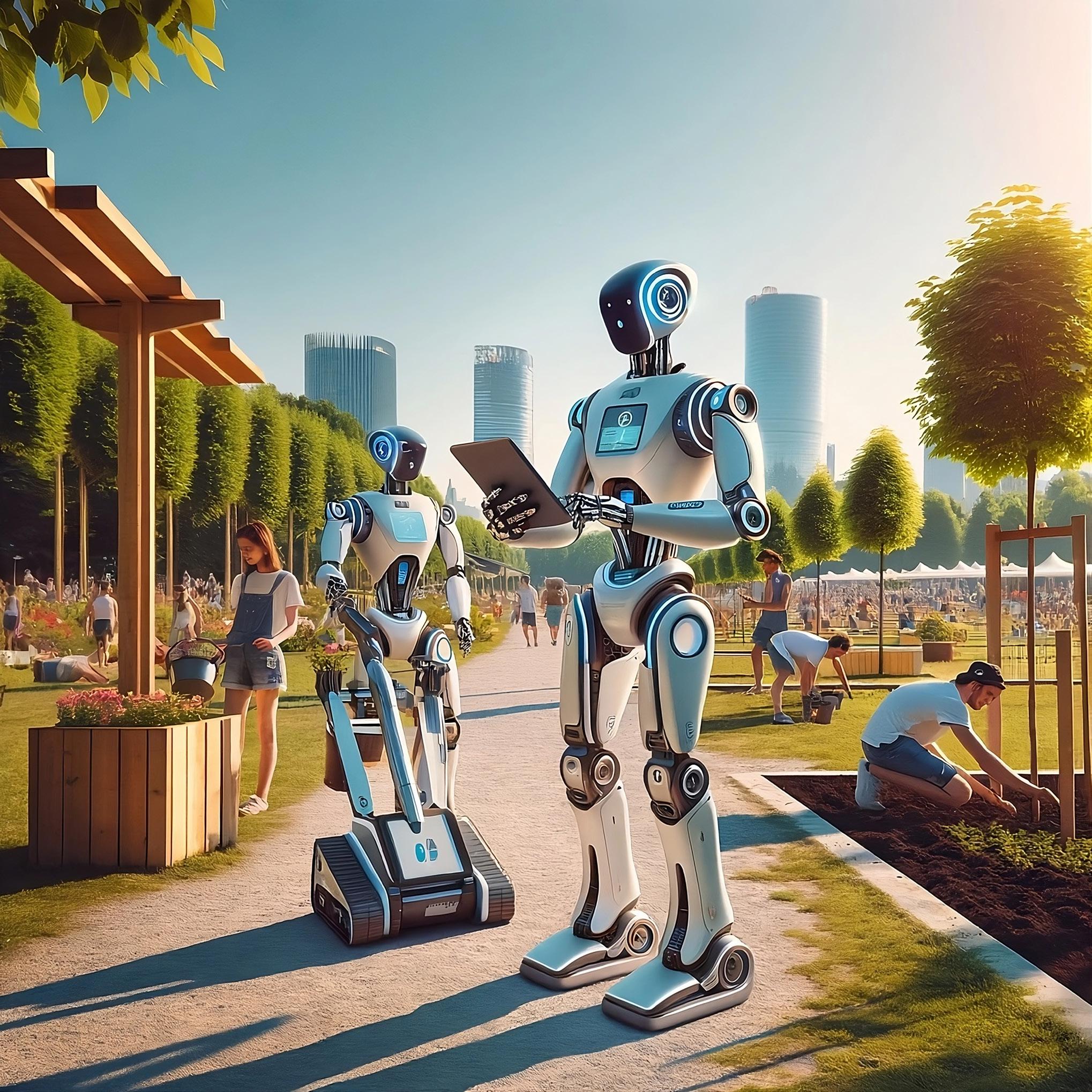

WWW.JAMRIS.ORG pISSN 1897-8649 (PRINT)/eISSN 2080-2145 (ONLINE)
Indexed in SCOPUS
VOLUME 18, N° 1, 2024
A peer-reviewed quarterly focusing on new achievements in the following fields:
• automation • systems and control • autonomous systems • multiagent systems • decision-making and decision support •
• robotics • mechatronics • data sciences • new computing paradigms •
Editor-in-Chief
Janusz Kacprzyk (Polish Academy of Sciences, Łukasiewicz-PIAP, Poland)
Advisory Board
Dimitar Filev (Research & Advenced Engineering, Ford Motor Company, USA)
Kaoru Hirota (Tokyo Institute of Technology, Japan)
Witold Pedrycz (ECERF, University of Alberta, Canada)
Co-Editors
Roman Szewczyk (Łukasiewicz-PIAP, Warsaw University of Technology, Poland)
Oscar Castillo (Tijuana Institute of Technology, Mexico)
Marek Zaremba (University of Quebec, Canada)
Executive Editor
Katarzyna Rzeplinska-Rykała, e-mail: office@jamris.org (Łukasiewicz-PIAP, Poland)
Associate Editor
Piotr Skrzypczyński (Poznań University of Technology, Poland)
Statistical Editor
Małgorzata Kaliczyńska (Łukasiewicz-PIAP, Poland)
Typesetting SCIENDO, www.sciendo.com
Webmaster TOMP, www.tomp.pl
Editorial Office
ŁUKASIEWICZ Research Network
– Industrial Research Institute for Automation and Measurements PIAP
Al. Jerozolimskie 202, 02-486 Warsaw, Poland (www.jamris.org) tel. +48-22-8740109, e-mail: office@jamris.org
The reference version of the journal is e-version. Printed in 100 copies.
Articles are reviewed, excluding advertisements and descriptions of products.
Details are available at: https://www.jamris.org/index.php/JAMRIS/ LicenseToPublish
Editorial Board:
Chairman – Janusz Kacprzyk (Polish Academy of Sciences, Łukasiewicz-PIAP, Poland)
Plamen Angelov (Lancaster University, UK)
Adam Borkowski (Polish Academy of Sciences, Poland)
Wolfgang Borutzky (Fachhochschule Bonn-Rhein-Sieg, Germany)
Bice Cavallo (University of Naples Federico II, Italy)
Chin Chen Chang (Feng Chia University, Taiwan)
Jorge Manuel Miranda Dias (University of Coimbra, Portugal)
Andries Engelbrecht ( University of Stellenbosch, Republic of South Africa)
Pablo Estévez (University of Chile)
Bogdan Gabrys (Bournemouth University, UK)
Fernando Gomide (University of Campinas, Brazil)
Aboul Ella Hassanien (Cairo University, Egypt)
Joachim Hertzberg (Osnabrück University, Germany)
Tadeusz Kaczorek (Białystok University of Technology, Poland)
Nikola Kasabov (Auckland University of Technology, New Zealand)
Marian P. Kaźmierkowski (Warsaw University of Technology, Poland)
Laszlo T. Kóczy (Szechenyi Istvan University, Gyor and Budapest University of Technology and Economics, Hungary)
Józef Korbicz (University of Zielona Góra, Poland)
Eckart Kramer (Fachhochschule Eberswalde, Germany)
Rudolf Kruse (Otto-von-Guericke-Universität, Germany)
Ching-Teng Lin (National Chiao-Tung University, Taiwan)
Piotr Kulczycki (AGH University of Science and Technology, Poland)
Andrew Kusiak (University of Iowa, USA)
Mark Last (Ben-Gurion University, Israel)
Anthony Maciejewski (Colorado State University, USA)
Krzysztof Malinowski (Warsaw University of Technology, Poland)
Andrzej Masłowski (Warsaw University of Technology, Poland)
Patricia Melin (Tijuana Institute of Technology, Mexico)
Fazel Naghdy (University of Wollongong, Australia)
Zbigniew Nahorski (Polish Academy of Sciences, Poland)
Nadia Nedjah (State University of Rio de Janeiro, Brazil)
Dmitry A. Novikov (Institute of Control Sciences, Russian Academy of Sciences, Russia)
Duc Truong Pham (Birmingham University, UK)
Lech Polkowski (University of Warmia and Mazury, Poland)
Alain Pruski (University of Metz, France)
Rita Ribeiro (UNINOVA, Instituto de Desenvolvimento de Novas Tecnologias, Portugal)
Imre Rudas (Óbuda University, Hungary)
Leszek Rutkowski (Czestochowa University of Technology, Poland)
Alessandro Saffiotti (Örebro University, Sweden)
Klaus Schilling (Julius-Maximilians-University Wuerzburg, Germany)
Vassil Sgurev (Bulgarian Academy of Sciences, Department of Intelligent Systems, Bulgaria)
Helena Szczerbicka (Leibniz Universität, Germany)
Ryszard Tadeusiewicz (AGH University of Science and Technology, Poland)
Stanisław Tarasiewicz (University of Laval, Canada)
Piotr Tatjewski (Warsaw University of Technology, Poland)
Rene Wamkeue (University of Quebec, Canada)
Janusz Zalewski (Florida Gulf Coast University, USA)
Teresa Zielińska (Warsaw University of Technology, Poland)
Publisher:
Copyright © 2023 by Łukasiewicz
Research Network - Industrial Research Institute for Automation and Measurements PIAP
1
VOLUME 18, N˚1, 2024
DOI: 10.14313/JAMRIS/1-2024
Aleksandra Urbanczyk, Krzysztof Kucaba, Mateusz
Wojtulewicz, Marek Kisiel‑Dorohinicki, Leszek
Rutkowski, Piotr Duda, Janusz Kacprzyk, Xin Yao, Siang Yew Chong, Aleksander Byrski
DOI: 10.14313/JAMRIS/1 2024/1 12
Maksym Grishyn, Kostiantyn Beglov
DOI: 10.14313/JAMRIS/1 2024/2
Low‐Cost Small‐Scale Autonomous Vehicle
Ismail Bogrekci, Pinar Demircioglu, Mustafa Yasir Goren
DOI: 10.14313/JAMRIS/1 2024/3
Application of Multilayer Neural Networks for Controlling a Line‐Following Robot in Robotic Competitions
Cesar Minaya, Ricardo Rosero, Marcelo Zambrano, Pablo Catota
DOI: 10.14313/JAMRIS/1 2024/4
Pearson Correlation and Ordered Weighted Average Operator in the World Stock Exchange Market
Martha Flores‑Sosa, Ernesto Leon‑Castro, Jose
M. Merigo
DOI: 110.14313/JAMRIS/1 2024/5
Using Reinforcement Learning to Select an Optimal Feature Set
Yassine Akhiat, Ahmed Zinedine, Mohamed Chahhou
DOI: 10.14313/JAMRIS/1 2024/6
67
Unlocking the Future of Secure Automatic Machines: Leveraging FaceReg with HRC & LBPH
Yamini Vijaywargiya, Mahak Mishra, Nitika Vats
Doohan
DOI: 10.14313/JAMRIS/1 2024/7
Submitted:8th February2023;accepted:17th July2023
AleksandraUrbanczyk,KrzysztofKucaba,MateuszWojtulewicz,MarekKisiel‑Dorohinicki,LeszekRutkowski, PiotrDuda,JanuszKacprzyk,XinYao,SiangYewChong,AleksanderByrski
DOI:10.14313/JAMRIS/1‐2024/1
Abstract:
Socio‐cognitivecomputingisaparadigmdevelopedfor thelastseveralyearsinourresearchgroup.Itconsists ofintroducingmechanismsinspiredbyinter‐individual learningandcognitionintometaheuristics.Differentver‐sionsoftheparadigmhavebeensuccessfullyapplied inhybridizingAntColonyOptimization(ACO),Particle SwarmOptimization(PSO),GeneticAlgorithms,Differ‐entialEvolution,andEvolutionaryMulti‐agentSystem (EMAS)metaheuristics.Inthispaper,wehavefollowed ourpreviousexperiencesinordertoproposeanovel mutationbasedonsocio‐cognitivemechanismandtestit basedonEvolutionStrategy(ES).Thenewlyconstructed versionswereappliedtopopularbenchmarksandcom‐paredwiththeirreferenceversions.
Keywords: metaheuristics,socio‐cognitivecomputing, globaloptimization
1.Introduction
Tacklingdif icultoptimizationproblemsrequires usingmetaheuristics[1],andveryoftenitisneeded tocreatenewones[2],i.e.bymodifyingorhybridizing theexistingalgorithms[3].
AlthoughSorensenhascriticizedthedevelopment ofnewmetaheuristics[4],wecontendthatusing metaphorsinourdailywork[5]notonlyfosterscre‐ativitybutalsomayresultinthediscoveryoftrulynew solutionsofconsideredissuesornovelmechanismsto solvethemautomatically.
Becauseclassicmetaheuriticsarefrequently inspiredbynature,theirfurthermodi ications frequentlycombinedifferentphenomenaobservedin therealworld.
Onedirectionofsuchmodi icationscomesfrom theveryin luentialSocial‐CognitiveTheoryintro‐ducedbyBandura[6].Accordingtothistheory, someofaperson’sknowledgecanbedirectlylinked toobservingothersduringtheirsocialinteractions, experiences,andexternalmediain luences.[7].Thus, despitelearningonlythroughherowntrialanderror, onecanreachhergoalssoonerthankstosuchobser‐vation[8].
Wehavealreadyintroduceddedicated mechanismsrootedinSocial‐CognitiveTheoryto selectedmetaheuristics(socio‐cognitiveACO[9]and
socio‐cognitivePSO[10]),obtaininggoodresults comparedtothereferencealgorithms.
Presently,wefocusonthegroupofevolutionary metaheuristics,andbymodifyingchosenalgorithms fromthisgroup,weaimtodevelopauniversalmech‐anismforvariationoperatorsthatwouldembodythe ideaofsocio‐cognitivelearningmechanisms.
Themaincontributionofthispaperisasocio‐cognitivelyinspiredmutationmechanism,thatmakes itpossibletoexchangetheinformationamongthe individualsinevolutionaryalgorithms.Theproof‐of‐conceptofthismechanismwasintroducedinthe researchpaperin2021[11]andwasredesigned andreimplementedbasedontheresultsachieved. Theef iciencyandef icacyofthenewversionof thealgorithmsaretestedusingwell‐knownhigh‐dimensional,multimodalbenchmarkfunctions.The proposedmethodisbasedoncopyingcertainparts ofthegenotypes(thuspassingtheknowledge)from thebetterones,andavoidingthepartsofsolutions oftheworstones.Inthispaper,weconsiderwell‐known (��+��) ES,butwebelievethatourmuta‐tionmechanismmaybeusedinabroaderrangeof algorithms.
Westartwiththereferencetostate‐of‐the‐art showingtheexistingmodi icationsofmetaheuristics, inparticularevolutionstrategies.Thenweshowthe novelmethodforintroducingsocio‐cognitivemecha‐nismsinto(��+��)evolutionstrategy.Weproviderele‐vantexperimentalresultsand,intheend,weconclude ourpapershowingthesummaryandthefuturework plans.
Thereareseveralmetaheuristicdiscoursesin whichthisworkcanbeanchored.Onthemostgeneral level(consideringthearchitectureoftheentirealgo‐rithm),itcanbetreatedasakindofhybridalgorithm [12]inthesamesensethatamemeticalgorithmisone [13]andmanyothersimilaralgorithms,developed intheresearchgroupoftheAuthors[14–16].The majorityofmemeticalgorithmsarebasedongenetic algorithm,andhaveintroducedsomelocalsearch orheuristiclearningmechanisms.Unlikethem,the describedalgorithmisbasedonanothermetaheuris‐ticoftheevolutionarycomputationgroup,namelythe evolutionstrategy[17,18].
Thesimilarityliesinthefactthatanovelmecha‐nism(i.e.,socio‐cognitivemutationoperator)isintro‐ducedinbetweenstandardstepsofthealgorithm.Our workshouldalsobeplacedinthecontextofvarious modi iedorhybridESs.Thepossiblemodi icationsof classicESsrangefromsimpletuningormanipulation ofcontrolparameterssuchasmutationstrengthor populationsize(step‐size)[19–21],throughcovari‐ancematrixadaptationevolutionstrategy(CMA‐ES) [22]toheterogeneoushybridsofES,whichareoften focusedonparticularapplication,e.g.vehiclerouting problem[23],optimizationofengineering,andcon‐structionproblems[24,25]andthenumberofwhich isapparentlynotveryhigh.
Takingintoaccountthelevelofthevariationoper‐atorsitself,ourpostulatedoperatorcanbecom‐paredtotheonepresentinthedifferentialevolution metaheuristic[26].ThecharacteristictraitofDEis themutationvariationoperator,whichoperateson parametervectorswithscaledpopulation‐deriveddif‐ferencevectors.Inthissense,itisnotjustarandomly performingoperator,asintraditionalEAsandESs,but itutilizestheinformationaboutcurrentpopulation, especiallyintheschemeshaving“best”inthenames, suchas ����/��������/1 and ����/������������−����−��������/1 thatusethebestsolutiontode inemutationdirec‐tions[27].Asimilaranalogyispresentbetweenclassic mutationandoursocio‐cognitivemutationoperator. Themechanicsofthenewoperatorcanberelatedto thewell‐knownTOPSIS(TechniqueforOrderofPref‐erencebySimilaritytoIdealSolution)method[28]. TOPSISisbasedontheideathatthechosenalterna‐tiveshouldbetheonewiththeshortestgeometric distancefromthepositiveidealsolutionandtheone withthegreatestgeometricdistancetothenegative idealsolution.
AsalreadymentionedintheIntroduction,we rootourworkinadiscourseofsocio‐cognitively inspiredalgorithms.The irstobjectiveofintroduc‐ingsociocognitivemechanismintoevolutionstrate‐giesservedasaproof‐of‐conceptthatturnedoutto bepromising[11],butpointedoutseveraldimen‐sionsformajorimprovements.The irstconclusion wasthatthesemechanismsthatoperatetowards bettersolutionsgivebetterresultsthanoperators basedonmovingawayfromtheworstindividuals.We decidedthatthecoreofourideawasasynergyof thesetwodirections,andthatthesecondpartmust betotallyredesignedinordertoworkasintended. Otherwise,itwouldbetoostraightforwardanalogy with����/��������/1andothersocio‐cognitivealgorithms describedin[29]and[30],sothenoveltywouldbe minimal.Thesecondlessonfromthepreviousattempt tomodifyESwasthatthealgorithmitselfshouldhave amoderatelevelofcomplexityinordertobeabasefor asuccessfulsocio‐cognitivemodi ication.Theexperi‐mentsperformedonthe(1+1)versionofES,aswell asthe (��,��) versionwerenotassuccessfulasthose basedonthe(��+��)versionofthealgorithm,which gavebetterresultsinallthebenchmarkstested,in contradictiontothe(��,��)versionthatwasbetteronly inoneofthem.Sowedecidedthatitwillbethebestto sticktothe(��+��)versionforourfurtherpurposes.
TheclassicalgorithmofEScanbedescribedas follows:
1) Initializeparentpopulation ���� ={��1,…,����}.Each oftheindividualscanbedescribedasfollows:��∋ ���� ={����,1,…,����,��,����,1,…,����,��},��,��∈ℕstandsfor anindividualcontainingagenotype ����,1,…,����,�� representingobjectiveparameters,andassociated ����,1,…,����,�� mutationstrategyparametersthatwill beadaptedinordertoguidethesearch.Thedimen‐sionalityoftheconsideredproblemis��. Later,we usethenotation ����,�� toreferto ����,��,whichis ��-th geneof ��-thgenotype.
2) Generate �� offspringindividualsformingtheoff‐springpopulation���� ={��1,…,����}inthefollowing procedure:
‐ Randomlyselect��parentsfrom���� (if��=��,then takeallofthem).
‐ Recombinethe��selectedparents(traditionallya pair)toformarecombinantindividual����,using anypossiblerecombinationmeans(traditionally averagingcrossoveroperatorwasused).
‐ Mutatethestrategyparameterset����,1,…,����,�� of therecombinant ���� (adaptinge.g.themutation diversitiesforthenextmutation).Traditionally, mutationisrealizedbyapplyingaperturbation basedon,forexampleuniformorGaussianran‐domdistributionoraddingorsubtractingacer‐tainvalueto(from)aselectedgene.
‐ Mutatetheobjectiveparameterset ����,1,…,����,�� oftherecombinant���� usingthemutatedstrategy parametersettocontrolthestatisticalproper‐tiesoftheobjectparametermutation.
3) Selectnewparentpopulation(usingdeterminis‐tictruncationselection)fromeithertheoffspring population ���� (thisisreferredtoascomma‐selection,usuallydenotedas“(��,��)‐selection”),or theoffspring���� andparent���� population(thisis referredtoasplus‐selection,usuallydenotedas “(��+��)‐selection”).
4) Goto2.untilterminationcriterionful illed. Wehavedecidedtointroducethesocio‐cognitive mechanismstothe (��+��) versionofES.Thisfol‐lowsfromtheapparentpotentialofsuchmechanisms developedearlierin[11].Wehavestudiedtheupdat‐ingpartoftheoperatorsappliedtherein,andintro‐ducedmodi icationsinordertoincreasetheiref icacy. Inparticular,wehaveaimedatincreasingthe exchangerateofinformationbetweentheindividuals incurrentpopulationwiththegoalofacceleratingthe learningrateofalgorithm.Inordertoachievethis,we splitasinglemutationstepintomultipleindependent sequentialmutations.The irstmutationisalwaysthe classicaloperatormeanttointroduceperturbationto thesolution’sgenome.Thefollowingoperatorormul‐tipleoperatorsaremeanttointroducefurthermodi i‐cationstothatsolutionthatareguidedbythecurrent stateofpopulation.
Inourexperimentswetestandevaluatethefol‐lowingsocialmutations:
1) FollowBest:
Outofthetop �� individuals ��1,…,���� incurrent populationrandomlyselectonethatwillbenow calledteacher ��.Withprobability ����,foreachof thecurrentlyoperatedonsolution’s �� genes ����, assignnewvalue���� ←���� +����(���� −����)where���� is thecorrespondinggeneof��and���� isfollowrate.
2) FollowBestDistinct:
Leteachindividual ���� beasequenceof �� genes ���� =(����,1,…,����,��).Outofthetop �� individ‐uals ��1,…,���� incurrentpopulationrandomly selectonethatwillbenowcalledteacher ��. Acrossthe��1,…,���� individualscalculatethestan‐darddeviationforeachofthegenepositions 1,…,�� resultingin ��1 ������,…,���� ������ where ���� ������ = ������(��1,��,…,����,��).Choose �� genepositionsper‐formingweightedrandomselectionacross1,…,�� using ��������������(��1 ������,…,���� ������) asvectorofproba‐bilities.Foreachof��chosengenepositionsofthe currentlyoperatedonsolution’s��genes���� assign newvalue ���� ←���� +����(���� −����) where ���� isthe correspondinggeneof��and���� isfollowrate.
3) RepelWorstGravity:
Outof �� worstindividualsinthecurrentpopu‐lationrandomlyselectoneindividual ������.While operatingonanindividual ������,withprobability ����,performthefollowingassignmentforevery gene ��: ����,�� ←����,�� +���� ⋅ ������(����) ��2 �� ,where ���� = (����,�� −����,��) iscalledadistanceingene ��, ������ isasignfunctionand���� isarepelrate.Thatway therepelmagnitudeisinverselyproportionalto thesquareddistanceforagivengene,andwitha directionawayfromthechosenworstindividual.
4) RepelWorstGravityMultistep:
Foreveryindividual ���� from �� worstindividu‐alsinthecurrentpopulationperformtheassign‐mentsdescribedabove.Thatwaytherepeleffect isstrongerandmoreversatile.
4.Experiments
Themainaimoftheexperimentsistoverifythe ef icacyofglobaloptimization(minimization)ofthe novelalgorithmsfortheselectedbenchmarkfunc‐tions(Ackley,DeJong,Rastrigin,andGriewank[31]) ofdimensions ��∈{100,500,1000}.Boththevalue obtainedinthelastiteration,andthetrajectoryofthe itnessfunctionsimprovementsareconsidered–in certainsituationsitisdesirabletohavearelatively fastconvergenceearlier,inothersituationsthefocus isplacedonthe inalresult.Theequationsusedforthe benchmarkfunctionsareasfollows:
‐ Ackley: ��(��)=−����−�� 1/��∑�� ��=1(��2 �� )
��1/��∑�� ��=1cos(������) +��+��;��=20;��=0.2;��= 2��;��∈[1∶��];−32.768≤��(��)≤32.768.��(��opt)= 0,��opt �� =0
‐ DeJong:��(��)= ∑�� ��=1 ��2 �� ,��∈[1,��];−5.12≤���� ≤ 5.12.��(��opt)=0,��opt �� =0
‐ Rastrigin:��(��)=10��+∑�� ��=1(��2 �� −10cos(2������)),��∈ [1,��];−5.12≤���� ≤5.12.��(��opt)=0,��opt �� =0.
‐ Griewank:��(��)= ∑�� ��=1 ��2 �� /4000−∏cos(����/√��)+ 1,��∈[1,��];−600≤���� ≤600,��(��opt)=0, ��opt �� =0
Thefollowingalgorithmshavebeenbenchmarked:
‐ Original(��+��)ES,
‐ FollowBestES–withtheFollowBestmutation,
‐ FollowBestDistinctES–withtheFollowBestDis‐tinctmutation,
‐ RepelWorstGravityMultistepES–withtheRepel WorstGravityMultistepmutation,
‐ ComboDistinctGravityES–withtheFollowBest DistinctandRepelWorstGravitymutations,
‐ ComboDistinctGravityMultistepES–withtheFol‐lowBestDistinctandRepelWorstGravityMultistep mutations.
Thestoppingcriteriawasreachingmaximum numberofiterationsofpopulationupdates(setas100 foralltheexperiments).Thenumberofindividuals inthepopulationwassetto ��= 200.Thefollowing settingshavebeenusedforthealgorithms:
‐ ��=20,��=140.
‐ ��good =0.1,��bad =0.1,��=0.01
‐ ��=1/��,where��isthenumberofdimensions,
‐ numberofthecurrentlybestorworstindividuals:5. Eachexperimenthasbeenrepeated12times,andthe meanvalueofthe itnessfunctionistakenasrefer‐ence.Thealgorithmshavebeenimplementedusing jMetalPy1 computingframework.Thesourcecodeis availableonrequest.Thecomputationshavebeen conductedonaPC‐classcomputer.
Westartwithobservationsofgeneralbehaviorand ontherepeatability(i.e.,consistencyofperformance inrepeatedruns)ofthealgorithmswhensolvingthe problemsforallthevariantsoftheproposedalgo‐rithms.Therefore,wehavepreparedhistogram‐like visualizationsofthecomputationruns.InFig. 1,the actualtrajectoriesofeachalgorithmscanbeseen. Moreover,eachverticalsliceshowsthecountofthe valuesobtainedateachiterationofthealgorithmfor allrepeatedexperiments.
Wecanclearlyseethatallthevariantsofthemod‐i ied (��+��) approachesarerepeatable.Moreover, theresultsobtainedforoneofbiggestproblemstack‐led,namelyAckleyin1000dimensionscanalsobe observedindetail.Beingconvincedoftherepeata‐bilityoftheexperimentswecanproceedwithsubse‐quentphasesofourstudies.
Nowwecanfocusonobservationsoftheaverages obtainedforallthebenchmarkproblemsaddressed withdifferentcon igurationsofthealgorithms.
Itisclearfromobservationsoftheresultsthat ourmethods(includingthebasealgorithm)arevery effectiveinthecaseofGriewankandAckley(see Figs.2and3)problems.Notallourproposedmethods areeffectiveforDeJongandRastriginproblem(see Figs.5and4). Forexample,therepelworstgravity approachdoesnotalwaysleadtoimprovementsin
theperformanceoverthebasealgorithm.Thisis notsurprisingfollowingthemainimplicationofthe well‐known NoFreeLunchTheorem byWolpertand MacReady[2],inwhichoneoftheimportantsteps wouldbetooptimizetheparametersofthesearchfor eachindividualproblem.
Ourmotivationforthisstudyistotesttheef i‐ciencyandef icacyofourproposedmechanismsin theirbaselinecon igurations.Assuch,wehavesought todeterminetheirgeneralcapabilitiestoimprovethe referenceESalgorithmoverthewholesetofselected benchmarkproblems.
Whenaparticularmechanismdidnotlead toimprovementbutleadtoloweraverageperformanceforaparticularbenchmarkproblem, resultsindicatethatthedifferenceisnotstatisticallysigni icant(e.g.,Table 2 forRepelWorst GravitycomparedwiththebaseorreferenceES algorithm)ontheGriewankProblemat ��=1000. Thissuggestsscopetooptimizetheparametercon‐igurationsofourproposedmechanismsthatwar‐rantfurther,futurestudies.Inadditiontoasystem‐aticparametersweeptoascertainoptimalparameter con igurationsforthemechanisms,otherapproaches wouldbetoapplysomededicatedalgorithmtuning methodsuchasiRace[32].Oneadditionalconclusion ofthisphaseisthatthebestofourmodi icationwas ComboDistGravityalongwithRepelBest.
Inadditiontoprovidingqualitativedescriptions ofthebehaviourofthealgorithmsissolvingthe benchmarkproblemsusinggraphs,wecorroborate
Table2. Dunntestp‐valuesofalgorithmpairsthat exceededthe0.01thresholdandareconsiderednot significantlydifferent
those indingswithquantitativeresults(e.g.,average withstandarddeviation)thatarepresentedinatabu‐larform.
TheseresultsareprovidedinTable1.Theobserva‐tionscon irmthe indingsperceivedwhenanalyzing thegraphs,andtheinformationobtainedfromthe spreadofresultswhentheindividualalgorithmsare repeatedviastandarddeviationfurtherconvincesus abouttherepeatabilityofthosealgorithmsandsignif‐icanceofthe indings.
Wehavesystematicallyperformedvarioussta‐tisticaltestingonthequantitativeresultswehave obtained.First,wehaveappliedtheShapiro‐Wilktest withsigni icancethresholdof 0.05 tocheckwhether theobservedsamplehadanormaldistribution. The nullhypothesisthatthesampleobtainedforeach proposedalgorithmisrejected.Assuch,wepro‐ceedwiththeKruskal‐Wallistestinordertocheck whethertheircumulativedistributionfunctionsdif‐fered,and inallypairwisecomparisonsviaDunn’s testinordertocheckwhichonesweresigni icantly different.ExceptfortheresultslistedinTable 2,all otheralgorithmsachievedstatisticallysigni icantval‐ueswithp‐valuesbelow0.01(assumingthisvalueas signi icancelevel��)usingDunn’stest.
Inthispaper,weproposedandstudiednovel methodsforhybridizingsocio‐cognitiveinspirations inES.Theproposedalgorithmsarebasedontheprin‐cipleofintroducingcertainmechanismsofattracting thecurrentlymodi iedgenotypestothebestonesand repellingthemfromtheworstonesinthepopulation.
Ourexperimentsyieldedinterestingresults.It turnsoutthattheproposedmechanismswereappar‐entlysuccessfulfortwooffourtackledBenchmark problems(AckleyandGriewank)inallthedimensions tested.Weveri iedthisclaimthroughbothqualitative analysisviaplotsofthesearchperformancesofthe algorithmsandquantitativeanalysisviatheuseof systematicstatisticalanalysisonthesamplesofsearch performancesfromrepeatedrunsofthealgorithms. However,thesocio‐cognitivemutationwassuccessful forthetwootherproblems,namelyDeJongandRas‐trigin,onlyinthecaseof100dimensions.Itshould benotedthatwedidnotperformindividualtun‐ingoftheparameterssoastoobtainimprovements. Ourcurrentmotivationistoestablishthegenerality oftheproposedmechanismsastheyareinbaseline con iguration.
Nevertheless,weshowedthatdifferentvariants ofourmethodssucceeded–thereforefollowingthe well‐known NoFreeLunch theorembyWolpertand MacReady,inourfutureresearchwewouldliketo tuneourmethodstomeetparticularneedsofallthe tackledproblem.Moreover,wewillstudyifourmod‐i icationofthebasealgorithm(inthiscase,ES)will workaswellwhenappliedinothermetaheuristics,as themodi icationitselfcanbeperceivedasgeneralone, notparticularlyconnectedwithESthatisstudiedin thispaper. Notes 1https://github.com/jMetal/jMetalPy
AUTHORS
AleksandraUrbanczyk –AGHUniversity, Al.Mickiewicza30,30‐059Krakow,e‐mail: aurbanczyk@agh.edu.pl.
KrzysztofKucaba –AGHUniversity,Al.Mickiewicza 30,30‐059Krakow,e‐mail:kkcba98@gmail.com.
MateuszWojtulewicz –AGHUniversity, Al.Mickiewicza30,30‐059Krakow,e‐mail: mateusz.wojtulewicz@gmail.com.
MarekKisiel-Dorohinicki –AGHUniversity, Al.Mickiewicza30,30‐059Krakow,e‐mail: doroh@agh.edu.pl.
LeszekRutkowski –InstituteofSystemsScience Research,Warsaw,Poland;AGHUniversity, Al.Mickiewicza30,30‐059Krakow,e‐mail: rutkowski@agh.edu.pl.
PiotrDuda –CzestochowaUniversityofTechnology, Poland,e‐mail:piotr.duda@pcz.pl.
JanuszKacprzyk –InstituteofSystemsScience Research,Warsaw,Poland;AGHUniversity, Al.Mickiewicza30,30‐059Krakow,e‐mail: janusz.kacprzyk@ibspan.waw.pl.
XinYao –SouthernUniversityofScienceandTechnol‐ogy,Shenzhen,China,e‐mail:xiny@sustech.edu.cn. SiangYewChong –SouthernUniversityof ScienceandTechnology,Shenzhen,China,e‐mail: chongsy@sustech.edu.cn.
AleksanderByrski∗ –AGHUniversity,Al. Mickiewicza30,30‐059Krakow,Poland,e‐mail: olekb@agh.edu.pl,www:https://orcid.org/0000‐0001‐6317‐7012.
∗Correspondingauthor
ACKNOWLEDGEMENTS
Theresearchpresentedinthispaperreceivedsup‐portfromthePolishNationalScienceCentreproject no.2019/35/O/ST6/00570(AU),thefundsassigned byPolishMinistryofEducationandScienceto AGHUniversity(MKD,JK),bytheprogram“Excel‐lenceinitiativeresearchuniversity”fortheAGHUni‐versityinKrakow,theARTIQproject:UMO‐2021/ 01/2/ST6/00004and84ARTIQ/0004/2021(LR)and NCNProjectno.2020/39/I/ST7/02285(AB).
References
[1] Z.MichalewiczandD.B.Fogel, HowtoSolveIt: ModernHeuristics.SpringerScience&Business Media,2013.
[2] D.H.WolpertandW.G.Macready,“Nofreelunch theoremsforoptimization,” IEEETransactions onEvolutionaryComputation,vol.1,no.1,Apr. 1997,pp.67–82,doi:10.1109/4235.585893.
[3] E.‐G.Talbi, Metaheuristics:FromDesigntoImplementation.JohnWiley&Sons,2009.
[4] K.Sörensen,“Metaheuristics—themetaphor exposed,” InternationalTransactionsin OperationalResearch,vol.22,no.1,2015, pp.3–18,doi:10.1111/itor.12001.
[5] G.LakoffandM.Johnson, MetaphorsWeLiveBy Chicago,IL:UniversityofChicagoPress,2003. Accessed:Feb.16,2024.[Online].Available:http s://press.uchicago.edu/ucp/books/book/chic ago/M/bo3637992.html
[6] A.Bandura,“Self‐ef icacy:Towardaunifying theoryofbehavioralchange,” Psychological Review,vol.84,no.2,1977,pp.191–215,doi: 10.1037/0033‐295X.84.2.191.
[7] A.Bandura, Socialfoundationsofthoughtand action:Asocialcognitivetheory.inSocialfoun‐dationsofthoughtandaction:Asocialcognitive theory.EnglewoodCliffs,NJ,US:Prentice‐Hall, Inc,1986,pp.xiii,617.
[8] A.Bandura,D.Ross,andS.A.Ross,“Transmis‐sionofaggressionthroughimitationofaggres‐sivemodels,” TheJournalofAbnormalandSocial Psychology,vol.63,no.3,1961,pp.575–582,doi: 10.1037/h0045925.
[9] A.Byrski, etal.,“Socio‐cognitivelyinspiredant colonyoptimization,” JournalofComputational Science,vol.21,Jul.2017,pp.397–406,doi: 10.1016/j.jocs.2016.10.010.
[10] I.Bugajski, etal.,“EnhancingParticleSwarm OptimizationwithSocio‐cognitiveInspirations,” ProcediaComputerScience,vol.80,Jan.2016,pp. 804–813,doi:10.1016/j.procs.2016.05.370.
[11] A.Urbanczyk,B.Nowak,P.Orzechowski,J.H. Moore,M.Kisiel‐Dorohinicki,andA.Byrski, “Socio‐cognitiveEvolutionStrategies,”in ComputationalScience–ICCS2021,M.Paszynski,D. Kranzlmüller,V.V.Krzhizhanovskaya,J.J.Don‐garra,andP.M.A.Sloot,Eds.,inLectureNotes inComputerScience.Cham:SpringerInter‐nationalPublishing,2021,pp.329–342.doi: 10.1007/978‐3‐030‐77964‐1_26.
[12] E.‐G.Talbi,“ATaxonomyofHybridMetaheuris‐tics,” JournalofHeuristics,vol.8,no.5,Sep.2002, pp.541–564,doi:10.1023/A:1016540724870.
[13] Y.‐S.Ong,M.‐H.Lim,N.Zhu,andK.‐W.Wong, “Classi icationofadaptivememeticalgorithms: acomparativestudy,” IEEETransactionsonSystems,Man,andCybernetics,PartB(Cybernetics),vol.36,no.1,Feb.2006,pp.141–152,doi: 10.1109/TSMCB.2005.856143.
[14] RobertSchaefer,AleksanderByrski,Joanna Kolodziej,andMaciejSmolka.Anagent‐based modelofhierarchicgeneticsearch. Comput. Math.Appl.,64(12):3763–3776,2012.
[15] KamilPietak,AdamWos,AleksanderByrski,and MarekKisiel‐Dorohinicki.Functionalintegrityof multi‐agentcomputationalsystemsupportedby component‐basedimplementation.InVladimír Marík,ThomasI.Strasser,andAloisZoitl, editors, HolonicandMulti-AgentSystemsfor Manufacturing,4thInternationalConference onIndustrialApplicationsofHolonicandMultiAgentSystems,HoloMAS2009,Linz,Austria,
August31-September2,2009.Proceedings, volume5696of LectureNotesinComputer Science,pages82–91.Springer,2009.
[16] RobertSchaefer,AleksanderByrski,andMaciej Smolka.Stochasticmodelofevolutionaryand immunologicalmulti‐agentsystems:Parallel executionoflocalactions. Fundam.Informaticae, 95(2‐3):325–348,2009.
[17] I.Rechenberg, CyberneticSolutionPathofan ExperimentalProblembyIngoRechenberg.Royal AircraftEstablishment,1965.
[18] H.‐P.Schwefel, NumerischeOptimierungvon Computer-ModellenmittelsderEvolutions strategie:MiteinervergleichendenEinführung indieHill-Climbing-undZufallsstrategie.Basel: Birkhäuser,1977.doi:10.1007/978‐3‐0348‐5927‐1.
[19] D.V.Arnold,“Weightedmultirecombinationevo‐lutionstrategies,” TheoreticalComputerScience, vol.361,no.1,Aug.2006,pp.18–37,doi: 10.1016/j.tcs.2006.04.003.
[20] D.Brockhoff,A.Auger,N.Hansen,D.V.Arnold, andT.Hohm,“MirroredSamplingandSequen‐tialSelectionforEvolutionStrategies,”in ParallelProblemSolvingfromNature,PPSNXI, R.Schaefer,C.Cotta,J.Kołodziej,andG.Rudolph, Eds.,inLectureNotesinComputerScience. Berlin,Heidelberg:Springer,2010,pp.11–21. doi:10.1007/978‐3‐642‐15844‐5_2.
[21] T.‐Y.HuangandY.‐Y.Chen,“Modi iedevolution strategieswithadiversity‐basedparent‐inclusionscheme,”in Proceedingsofthe2000. IEEEInternationalConferenceonControl Applications.ConferenceProceedings(Cat. No.00CH37162),Sep.2000,pp.379–384.doi: 10.1109/CCA.2000.897454.
[22] N.HansenandA.Ostermeier,“Completely DerandomizedSelf‐AdaptationinEvolution Strategies,” EvolutionaryComputation,vol.9, no.2,Jun.2001,pp.159–195,doi:10.1162/1063 65601750190398.
[23] P.P.Repoussis,C.D.Tarantilis,O.Bräysy,and G.Ioannou,“Ahybridevolutionstrategyfor theopenvehicleroutingproblem,” Computers& OperationsResearch,vol.37,no.3,Mar.2010, pp.443–455,doi:10.1016/j.cor.2008.11.003.
[24] D.Koulocheris,H.Vrazopoulos,andV.Dertima‐nis,“Hybridevolutionstrategyforthedesignof weldedbeams,”in Proc.ofInt.CongressonEvolutionaryMethodsforDesign,Optimizationand ControlwithApplicationstoIndustrialProblems EUROGEN2003,2003.
[25] L.DosSantosCoelhoandP.Alotto, “Electromagneticdeviceoptimizationbyhybrid evolutionstrategyapproaches,” COMPEL–Theinternationaljournalforcomputation andmathematicsinelectricalandelectronic engineering,vol.26,no.2,Apr.2007,pp. 269–279,doi:10.1108/03321640710727638.
[26] R.StornandK.Price,“DifferentialEvolution–ASimpleandEf icientHeuristicforglobalOpti‐mizationoverContinuousSpaces,” Journalof GlobalOptimization,vol.11,no.4,Dec.1997, pp.341–359,doi:10.1023/A:1008202821328.
[27] KennethPrice,RainerM.Storn,andJouniA. Lampinen. DifferentialEvolution.inNatural ComputingSeries.Berlin/Heidelberg:Springer‐Verlag,2005.doi:10.1007/3‐540‐31306‐0.
[28] C.‐L.Hwang,Y.‐J.Lai,andT.‐Y.Liu,“Anew approachformultipleobjectivedecisionmak‐ing,” Computers&OperationsResearch,vol.20, no.8,Oct.1993,pp.889–899,doi:10.1016/ 0305‐0548(93)90109‐V.
[29] M.Nabywaniec,etal.,“Socio‐cognitiveOpti‐mizationofTime‐delayControlProblemsusing Agent‐basedMetaheuristics,”in 2022IEEE11th InternationalConferenceonIntelligentSystems (IS),Oct.2022,pp.1–7.doi:10.1109/IS57118. 2022.10019693.
[30] P.Kipinski,etal.,“Socio‐cognitiveOptimization ofTime‐delayControlProblemsusingEvolution‐aryMetaheuristics.”arXiv,Oct.23,2022.doi: 10.48550/arXiv.2210.12872.
[31] J.DieterichandB.Hartke,“EmpiricalReviewof StandardBenchmarkFunctionsUsingEvolution‐aryGlobalOptimization,” AppliedMathematics, vol.03,Jul.2012,doi:10.4236/am.2012.330215.
[32] M.López‐Ibáñez,J.Dubois‐Lacoste,L.Pérez Cáceres,M.Birattari,andT.Stützle,“Theirace package:Iteratedracingforautomaticalgo‐rithmcon iguration,” OperationsResearchPerspectives,vol.3,Jan.2016,pp.43–58,doi: 10.1016/j.orp.2016.09.002.
Submitted:14th October2022;accepted:29th September2023 MaksymGrishyn,KostiantynBeglov
DOI:10.14313/JAMRIS/1‐2024/2
Abstract:
Thepaperdiscussesthethreatofdecommissioningtothe thermalpowerplant(TPP)heatexchangertubesbecause oferosionanddevelopsacomputer‐integratedcontrol system(CICS)fortheprocessofdistributionofsteam coalflowswithdifferentindicatorsofabrasivematerials content,whichisbasedonfuzzylogic.
TheproblemofrapiddecommissioningofTPPheat exchangers,particularlyabrasivedamagetofurnace screentubes,economizer,superheater,etc.Thismay indicateadiscrepancybetweentheexpectedfuelash contentandtheactualone,aswellasahighcontentof abrasiveimpuritiesinsteamcoal.
TheworkaimstodevelopaCICSofthewearresis‐tanceoftheheatexchangesurfaceofasteamboilerofa coal‐firedpowerplantbymeasuringandfuzzycontrolof thecontentofabrasiveimpuritiesinsteamcoal.
TheproblemsofdamagetotheequipmentoftheTPP boilerareinvestigated,andasystemforcontrollingthe wearresistanceofthesurfacebyautomaticfuzzycontrol ofthequalityofcoalisdeveloped.Theresultswere investigatedduringcoalpreparationandcombustionin thefurnaceofathermalpowerplanttoinvestigatethe effectivenessoftheproposedfuzzycontroller.Themodel resultsconfirmthefeasibilityofthefuzzycontrolmethod forthesystemwithdifferentcoalqualityparameters.
Keywords: Automaticcontrolsystem,Fuzzycontrol,Coal‐firedpowerplants,Variablequalityofcoal,Fuelenrich‐ment,Wearresistanceoftheheatexchanger
1.Introduction
Despitetheconstantincreaseintheuseofrenew‐ableenergysourcestocoverthedemandofmod‐ernenergysystems,accordingto[1,2],mostofthe world’selectricityisproducedbyclassicalthermal powerplants(TPP),inparticular,themainresource forelectricityproductioniscoal(about36.7%).Thus, theproblemsthatarisefromusingfuelarestillrel‐evanttoday.Inparticular,coalwithahighcontent ofabrasiveimpuritiesduringcombustioncreatesero‐sivewearoftheheatexchangesurfacetubescausedby themovementofsolidparticlesentrainedinthe lue gas,whichincreasestheriskofprematuredecommis‐sioningofthisparticularequipment.Further,inthis paper,itwillbereferredtoasabrasivewear.
Theproblemofqualityistheproblemof luctua‐tionsinthecompositionofcoalusedforcombustion, namely:highcontentofabrasivematerialintheash impurityofcoaloradiscrepancybetweenthespec‐i iedashcontent(declaredbythesupplier)andthe actualone.Thus,unscrupuloussupplierswhodeclare asmallashcontentcandeliverbadfueltoTPPs.
Currently,powerplantmanagementhasthe opportunitytosolvetheproblemoflow‐quality fuelinoneoftwoways:totrytoenrichlow‐quality fuel[3,4]ortomixitwithhigh‐qualityfuelinareserve warehouse.However,forthesuccessfulapplication ofthesesolutions,itisnecessarytounderstandhow usefulthefuelenrichmentwillbe,takingintoaccount thelossesduringenrichment,enrichmentcosts,and transportdelay,anditisalsonecessarytoknowthe exactcurrentcoalqualitytoeffectivelymanagethe fuelquality.
Sincemodernpowerplantsarenotequippedwith alaboratoryforadetailedinvestigationoftheabra‐sivecontentofashimpurities,thereisalsoaproblem withhowtocalculatedetailedcoalqualityindicators foraccurateassessmentofthewearresistanceofthe heatexchangesurfaceofthesteamboiler.Additional dif icultiesariseduetotheimpossibilityofpremature shutdownofthepowerplanttocheckthecondition oftheequipment.Itisalsoworthnotingthatitis dif iculttounambiguouslydividethequalityofcoal intocategoriestodistributethe lowsbetweenthe furnace,replenishmentofthereservestock,enrich‐mentequipment,andtheneedtoattractstocksfrom thereserveformixingandcombustioninthefurnace. Therefore,itisadvisabletocreateadvancedcontrol systemsforpowerplantsoperatingunderconditions ofchangingthequalityofsteamcoalbasedonfuzzy controlprinciples.
Thisworkdescribesthedevelopmentofapower plantautomationsystemtoenablethedetectionof inconsistenciesinthequalityofenergyfuelandto implementafuzzycontrollerforthedistribution offuel lowsdependingontheirquality.Section 2 presentsaliteraturereviewofcurrentresearchissues andsetsthemainobjectivesofthiswork.Thethird sectionisdevotedtothedevelopmentofafuzzy controlsystemforthewearresistanceoftheheat exchangesurfaceofasteamboilerofacoal‐ ired powerplantbycontrollingthequalityofsteamcoalat theexpenseoffuel lowdistribution,aswellasmod‐elingtheactionofthecontroldeviceatvariouscoal
qualityindicators,andthestudyoftheeffectiveness ofthefuzzycontrolsystem.
Theregulationwasbuiltonfuzzylogicbecause thedivisionofcoalqualityinto iveconditionalclasses wasproposed,butitisimpossibletodividetheclasses byabrasivenessindicators.Thefourthchapterispre‐sentedintheformofconclusionsandsuggesteddirec‐tionsforfurtherresearch.
Modernpowerplants,withtheirintricateoper‐ationaldynamics,oftengrapplewithuncertainties rangingfrom luctuatingfuelqualitytovariableenvi‐ronmentalconditions.Traditionalcontrolsystems, structuredaroundrigidmathematicalframeworks, sometimesfalterinthefaceofthesenonlinearitiesand ambiguities.Fuzzylogicstandsoutasasuperioralter‐native,adeptlymanagingsuchuncertaintiesthrough itsinherentdesignrootedinlinguisticvariablesand fuzzysettheory.Thisallowsformore lexible,intuitive decision‐makingthatmirrorshumanreasoningpat‐terns,makingitespeciallyvaluableintranslatingthe vastexperientialknowledgeofpowerplantoperators intoactionablecontrolalgorithms.Furthermore,its adaptivenatureensuresresilienceinchangingcon‐ditions,ensuringthatpowerplantsmaintainoptimal performanceevenamidstunforeseendisturbances.
Thecurrenttrendsofresearchinmodelingand managementscienceremainrelevanttomany ields ofendeavor[5].Despitespecialattentionbeingpaid tosuchareasassoftcomputing,uncertainty,biblio‐metrics,neuralnetworks,etc.,theenergy ieldisnot anexception.Nowadays,severalstudieshavebeen carriedoutonvarioustechnologiesforassessingthe harmfuleffectsoflow‐qualityfuelonthewearresis‐tanceoftheheatexchangesurface[6,7],predictingthe consequences,aswellasautomationofinstallations andtechnologicalcomplexestomaintainstableoper‐ationofthepowerplant.
Besides,[8]paysmuchattentiontothedescription andmodelingofplantsasawholeandtheirparts (heatexchangers,turbines,boilers,etc.),and[9]con‐siderssuchcontrolmethodsasPID‐law,fuzzylogic control,andothers.Thesematerialsexaminemeth‐odsofmaintainingthestabilityofpowersystemsby increasingthefuelsupply,butthetaskofsigni icantly reducingtheabrasivewearoftheheatexchangesur‐faceisnotsolved,whichcanleadtounpredictable consequencesintheformoftheprematureshutdown ofthepowerunitforunscheduledrepairs,whichwill beacriticalloadontheoverallpowersystem.
In[10],theissuesofashimpurityoffuel,its abrasiveeffectonTPPequipment,andassessmentof theef iciencyoffuelenrichmenttoreducetherisksof TPPcostsincaseofurgentrepairswereconsidered. TPPoperationispresentedintheformofamodel, whichwasexpressedinthesumoftotal inancialand othercostsassociatedwithmalfunctions:repairand replacementofequipment,additionalfuelpurchase, etc.Undertheconditionoffuelenrichment,thesavings inTPPcostsareexpressedduringthelifetimeofthe equipment,includingthecostsofenrichment[11,12].
Itwasconcludedthatitwouldbemore cost‐effectiveforTPPstopurchaseandsetup coalpreparationequipmentthantoshutdownthe powerunitforscheduledorunscheduledrepairs.
Itwouldbepossibletoabandonlow‐qualityfuel inadvanceandswitchtoreservefueltopreventthese risksfromapproaching,butthetaskissigni icantly complicatedbythefactthatitisimpossibletobe sureofthequalityoftheimportedsteamcoalorto measurethechangeinabrasivedamageoftheheat exchangersurfaceduringcombustiontoanalyzethe actualwearresistance[13,14].Eveniftherewassuch anopportunitytolearninreal‐timeaboutthedamage totheheatexchangesurfaceduringthecombustionof steamcoal,TPPsaretraditionallynotequippedwith alaboratorytostudythequalityofcoalcomposition. Additionaldif icultiesarealsoimposedbytheapprox‐imate(notexact)determinationofthepercentageof certaincomponentsinthecompositionofrawmateri‐als.Thus,tosuccessfullysolvethisproblem,itwasnec‐essarytocreateacomputer‐integratedcontrolsystem (CICS)fortheprocessofcoalfuel lowdistribution, regardlessofthecontentofabrasivematerialinthe ashimpurity,basedonfuzzylogic.
Muchattentionin[15,16]ispaidtocontrolbased onfuzzylogic,namely,acontrolmethodforregulat‐ingpowerandenthalpyintheboilerofa765MW coal‐ iredthermalpowerplantispresented,andfuzzy boilerpowercontrolbyasteamregulatingvalve.
Theapplicationoffuzzylogicincontrolsystems, especiallywithinthermalpowerplants,hasbeena topicofsigni icantinterestandstudyinrecentyears. Astheenergysectorfacesincreasedchallengesfrom varyingconditionsandtheneedforoptimizedper‐formance,fuzzycontrolsystemspresentanadaptable solution.
KondratenkoandKozlov’sexplorationintogener‐atingrulebasesforfuzzysystemsdelvesintotheuse ofModi iedAntColonyAlgorithms,demonstratingthe capabilityofsuchalgorithmstoenhancetheperfor‐manceandaccuracyofruleformulations[17].Fur‐thermore,a2022publicationbyKozlovetal.accen‐tuatestheimportanceoffuzzylogicinmanagingthe complexityofthepyrolysisprocess,especiallywhen dealingwithmunicipalsolidwasteofvaryingcompo‐sition[18].Thisunderscorestheadaptabilityoffuzzy systemsinhandlingheterogeneousinputs,asituation frequentlyencounteredinpowerplants.
Adifferentangletothestudyoffuzzylogicin powerfacilitieswaspresentedbySatyanarayanaetal. in2014,whoofferinsightsintoautomaticgeneration controlinpowerplants.Theirworkcomparatively evaluatestheperformanceofPID,PSS,andFuzzy‐PIDcontrollers,illuminatingtheuniquebene itsofthe Fuzzy‐PIDinachievingbetterstabilityandresponse times[19].
Theenvironmentallyconsciousfacetofpowergen‐erationishighlightedbyKozlovetal.,whoemphasize thedevelopmentandoptimizationof“GreenFuzzy Controllers”speci icallytailoredforreactorsinspe‐cializedpyrolysisplants[20].Theirapproachmarries theprinciplesofsustainablepowergenerationwith theadaptabilityoffuzzylogic.
[21]divesdeeperintotheparametricoptimiza‐tionoffuzzycontrolsystems.Byharnessinghybrid particleswarmalgorithmsequippedwithanelite strategy,theirresearchsetsanewbenchmarkin optimizingtheperformanceoffuzzycontrolsystems, openingnewdoorsforreal‐timeadaptivecontrolin powerplants.
Papers[22, 23]emphasizetheimportanceof fuzzycontrolsystemsinmanagingtemperatures, particularlyduringpyrolysisprocesses.Their researchunderscoreshowfuzzyPIDcontrolsystems canenhancethermalbehaviouranalysis,offering improvementsintemperatureregulationandoverall systemstability.
Themainpurposeofthepaperwastodevelop andstudyaCICSforthewearresistanceoftheheat exchangesurfaceofasteamboilerofacoal‐ ired powerplantbycontrollingthequalityofcoalbydis‐tributingthe lowofcoalsuppliedforcombustion.
Toachievethisgoal,thefollowingtaskswerefor‐mulated:
‐ todevelopamodelofthemeasuringchannelof abrasivematerialcontentinsteamcoalforaCICS;
‐ todevelopamathematicalmodelfordetecting inconsistencyoffuelqualityindicatorsduringits combustionintheTPPfurnace;
‐ todevelopacontroldevicebasedonfuzzylogicto controlthewearresistanceoftheheatexchangesur‐facebycontrollingthequalityofcoalbydistributing the lowofcoalsenttothefurnace;and
‐ tosimulatetheoperationoftheclosed‐loopcontrol systematdifferentindicatorsofcoalabrasiveness.
3.DevelopmentofaFuzzyCICSofWear ResistanceoftheHeatExchangeSurfaceof aSteamBoilerofaThermalPowerPlantby ControllingtheQualityofSteamCoal
BeforedevelopingtheCICS,itwasnecessaryto consider,andmodelthemeasuringchannelofsteam coalquality,andanalyzeandformmathematicalmod‐elsofthecontrolobject.
4.DevelopmentoftheModelofMeasuring ChannelofAbrasiveMaterialContentin SteamCoalfortheCICS
Usually,TPPsarenotequippedwithalaboratory totesteachbatchofcoal,butfromtimetotime,the qualityofpurchasedfuelmaydifferfromtheindicator inthedocuments,anditwasnecessarytodevelopa methodfordeterminingtheashcontentofthefuel.
Threemethodsofdeterminationwereformulated:
1) Basedonthepowerunitcapacityreductionata steadycoalconsumption,i.e.withanactivereduc‐tionofelectricitygeneration,itislikelythatthe carbonmassinthefuelismuchlowerthanspec‐i ied.
2) Basedonincreasedfuelconsumptionatconstant unitcapacity.Iftomaintaintheloadofthepower unit,itisnecessarytoincreasetheconsumptionof combustedfuel;italsoindicatesadecreaseinthe carboncontentofthecoalbatch.Inthismethod, fuelconsumptionisdeterminedusingautomatic conveyorscalesusedatTPPs.
3) Bydeterminingthemassofashintheashcollec‐torswhenusingelectrostaticprecipitatorsorsep‐aratorsinthepipesofTPPs,toanalyzewiththeir helpandknowledgeofthetechnicalcharacteristics oftheequipmentwhethermoreashisreceivedin theashcollectorthanispermissible.
Thesemethodswereconsideredinmoredetail.
The irstmethod:
LetEin(1)betheelectricitygenerationunderthe conditionofidealfuel.EnSiO2 istheelectricitygenera‐tion,includingthedeclarednSiO2 index,wherenSiO2 is thecontentofabrasivematerialincoal.
En������2 =��TPP ∗24∗Nturb ∗E(1−n������2), (1) where
Nturb –turbinepower;
��TPP –ef iciencyofthermalpowerplants;
Efact –actualelectricitygeneration.
IfEfact <EnSiO2,thentheactualabrasivenessofthe fuelexceedsthedeclaredone.
Thedisadvantageofthismethodisthatithas lowaccuracy.Atthesametime,evenanapproximate indicatoroftheactualabrasivenessofthematerialis unknown.
Inaddition,themainproblemwiththismethod isthatmostboilerunitshaveafuelsupplyregulator, whichdoesnotallowforthereductionofthepowerof turbines[9].
Accordingtothedisadvantagesofthemainprob‐lemofthe irstmethod, thesecondmethod isthat onecouldtrytodetermineiftheactualfuelcon‐sumptionincreasesfromthatwhichshouldbeatthe declaredabrasiveness.
Inthismethod,themaindrawbacksaresimilar tothe irstmethod.Thismethodofcalculatingabra‐sivenessisapproximate,anditwasverydif icultto understandtheactualashcontentandabrasivenessof thefuel.
Inthiscase,neithermethodwasveryeffective,but theyhadaplacetochecktheirdata.
Therefore, thethirdmethod wasadoptedasthe mainwaytocalculatetheashcontentofthematerial.
In[10],“ZaporizhzhiaTPP”inEnergodarcity (Ukraine)wasconsideredprototypeA.
Withoutlaboratoryanalysis,itisimpossibleto sayexactlywhatpartofthefueliscombustiblemin‐eralcontentandwhatpartisanabrasivematerial. Giventhatmostofthecombustiblemineralcontent simplyburns,andabrasivematerialaccumulateson the iltersandintheashdump,itwasassumedthat theactualashcontentduringcombustionwillbethe actualabrasiveness.Thatis,inthefuture,thesecon‐ceptswillbeidenti ied.
TheStateStatisticsServiceofUkraineregularly recordstheamountofgreenhousegasemissionsusing aformulaapprovedbytheMinistryofEnvironmental ProtectionandNaturalResourcesofUkraine.Thiswas usedtocalculateemissionsfromregularfuelcombus‐tion.Thus,from[26–28],thefollowingisformulated in(2):
EmCO2i=ACi∗LCVi∗EFi∗Ofi (2)
where:
EmCO2i –CO2 emissionsfromfuelcombustionof type(i),[tonsCO2]
ACi–activitydata:theamountoffuelcombustion oftype(i),[tonsorthousandm3 ].
LCVi–isthelowercalori icvalueoffueloftype(i) [TJ/torTJ/thousandm3].
EFi–istheCO2 emissionfactorforfueloftype(i) [tCO2/TJ].
OFi–istheoxidationfactorforfueloftype(i).
Themaincombustionproductsaccordingto[13, 26],whichneedtobepaidattentionto(listedasthe mainones)areCH4,N2O,andCO2
Thefollowingemissionvolumeswereobtainedfor TPPA:
CO2 –4,519,919.60m3; N2O–411.09m3; CH4 –373.10m3;
Total:4,520,703.79m3/h.
Inthecaseofsimultaneousoperationofatleast four ilters,itwasnecessaryto ind ilterswithacapac‐ityof1,309,880m3/h.
Thiswasdonetosavetimeonlaboratorytestsof unburnedfuelresidues.Further,themostpessimistic scenarioassumesthattheashcontentisanindicator oftheabrasivenessofsteamcoal.
Further,themethodofcalculatingtheactualash content(abrasiveness)ofthefuelwasconsidered.
Accordingto[24],“ZaporizhzhiaTPP”useselec‐trostaticprecipitatorsinitsproduction,whichisa moremodernandef icientwaytocollectash[25]. Usually,theef iciencyisabout97–98%,incontrastto outdatedwetashcollectors(Venturiscrubberswith remotedropletseparator)fromthe60sand70swith a iltrationef iciencyofabout50%.
Then,itbecamenecessarytoanalyzethediffer‐encebetweentheactualamountofashobtaineddur‐ingfuelcombustionandtheamountthatshouldhave beenobtainedaccordingtothedeclaredquality.
Thedif icultyofmeasuringtheconsumptionof mineralimpuritiespresentincoalfuelisthattheash residueformedaftercombustiondoesnotmoveina singlestreambutaccumulatesinsomecharacteristic places.Thisisfacilitatedbyashcollectors.
AccordingtotheashcollectingschemesofTPPs,in particularFigure2,itwassummarizedthatitispos‐sibletoestimatetheamountofashinashcollectors ofthreetypes:inthefurnace,intheeconomizerash collector,andthechimney ilterashcollector.
Filtersthatmeetthefollowingrequirementsare EGV2‐70‐12‐6‐6,EGV2‐70‐12‐6‐7,EGV2‐70‐12‐6‐8. Theconditionsof100%ashcapturewereconsidered tobuildthemodel.
Forfurtherconstructionofthetechnological model,theschematicdrawingofashandslagremoval fromtheprincipleof[8,14],Figure 3 isconsidered
Figure3. Schematicdrawingofashandslagremoval: 1–boilerfurnacechamber;2–ashcollector;3–bath withascraperconveyorforcontinuousashremoval;4–ashflushingapparatusoftheashcollector;5–slag crusher;6–flushingpump;7–ashchannel;8–sluice nozzle;9–receivinghopperofslurrywithmetal catcher;10–baghousepump;11–drainagepump;12–slurrypipelines;13–ashdump
toinvestigateotherplacesofashaccumulationduring fuelcombustion.
Theabovedrawingshowsthattheashsettlesin theashdisposalchannelduringcombustion.Fromthe ilterandeconomizer,theashfallsdirectlyintotheash collectorandthen,usingaconveyor,intothebooker andfurtherintotheashdisposalarea.Withthehelp ofconveyorscales,itispossibletodeterminethemass ofmaterialthathasnotburned,buttherewasstilla problemwithashthatremainsdirectlyinthefurnace.
Accordingtothetechnologicalprocedureofash removal[14],the lyashfromtheashcollectorsmixes withashandslagthat lowsoutofthefurnacethrough theashchanneland,togetherwiththeprocess luid, createsashandslagslurry,whichgoestotheash disposalareathroughtheslurrypipeline.Itwouldbe possibletomeasuretheslurry lowrateintheslurry pipelineand,whendeductingthetechnical luid,to understandtheash low,itsrelationtothefuel low, andthedifferencebetweentheactualandthedeclared ashcontent.However,morerelevantistheamount ofashthatisvolatileandsettlesinashcollectors.It cancauseabrasivedamagetothepipesintheheat exchanger.Itwasproposedtoinstalla lowmeter intheashcollectorpipesthatdeliverashfromthe ashcollectorstomixwiththeslurry.Thishelpedto determinethecorrelationbetweentheash lowthat potentiallydamagesthepipesandtheactualashcon‐tentofthefuelasawhole.
Itwasproposedtousetheultrasonicslurry low meterDENCELL®UDF‐2tokeeprecordsofslurry low.Typically,theobjectswherethese lowmeters areimplementedareindustrialandproductionfacili‐ties,miningenterprises,miningandprocessingplants, mines,open‐pitmines,rawmaterialextractionenter‐prises,etc.
Tokeeprecordsofash lowfromashcollec‐torstomixingwiththemainslurry,itispro‐posedtousea lowmeter,SiemensSolids lowmeter SITRANS®WF300Series.
Thus,takingintoaccountthetransportdelayand thedensityofthetechnicalliquidintheslurry,it becamepossibletocalculatetheactualashcontent ofthefuelandhowitaffectsthewearresistanceof theheatingsurfacesofboilerequipment.Therefore, withthehelpofasmallamountofadditionalequip‐ment,theproblemofdeterminingtheabrasivenessof fuelintheabsenceofalaboratorywithfreeaccess wassolved.Thenextstepwastobuildamathemat‐icalmodelto indoutthediscrepancybetweenthe speci iedandactualindicatorsofthe lowofabrasive materialduringthecombustionofcoal.
5.DevelopmentofaMathematicalModelfor theDetectionoftheInconsistencyofFuel QualityIndicatorsDuringitsCombustionin theTPPFurnace
In[10],aparametricschemewasconstructedto understandtheTPPlinks,andtheselinkswerecom‐binedintheformofasystemofequations.Now,it
wasnecessaryto indthelinkswithexpressionsinash lows.
Theconnectionsbetweentheseparametersare describedbyasystemofequations(3):
Msl =Mfa1 +Mf_aa2 +MAda3
Mloss =Mfb1 +Mf_ab2 +MAdb3
Vres =Mfc1 +Mresc2 +Menc3
Top =Mfd1 +Mf_ad2 +MAdd3 +Mresd4 +Mend5
N=Mfe1 +Mrese2 +Mene3
, (3)
wherean,bn,cn,dm,en –constantcoef icients;n = 1,3;m=1,5
Mf –isthefuelconsumption,kg/h;
Men –istheenrichedfuelconsumption,kg/h;
Mres –isthereservefuelconsumption,kg/h;
Mf_a –isthe lyash lowfromashcollectors,kg/h;
MAd –istheashcontentoffuel,%;
Msl –isthe lowoftotalashandslagslurry,kg/h;
Top –istheoperatingtimebeforereplacingthe heatexchangerpipes,h;
Mloss –isthecarbonlossesduetothediscrep‐ancybetweenthedeclaredandactualashcon‐tent,whichislacking,whichmakesitnecessaryto enrichorusereserves,kg/h;
Vres –isthefuelstockinthereservewarehouse,t;
N–istheplantcapacity,MW.
Tocalculatethethreemaintasks:thevolumeof thefuelreserve,the lowofabrasive lyash,andthe operatingtimeoftheequipmentatthecurrentabra‐sivewearofpipesduringfuelcombustion,thissystem waswritteninanotherform.
Thefollowingnotationsareusedinthe ig‐ure:Concentrator‐fuelenrichmentsystem,Grand Controller‐controlsystemconsistingoflocalregu‐lators,anddecision‐makingsystemforcoal low distribution.
Theparametricschemeandthesystemofequa‐tionswerepresentedinthefollowing(4):
Mf_a =Msl Ff_a(Mf,Ad)
Vres =V0 Fen(Mf,Ad,Men)
Top =T0 FT(Mf,Ad) (4)
Thefuelcombustionprocessintheinputfueland outputemissionstreamsisdescribed(5),withthe variableAdasafunctionoftime��:
dMAd d�� =(Mash+dMash)−(Msl+dMsl)
Mash Msl =0
dMAd d�� =dMash dMsl, (5)
whereMash –isthegeneralash lowconsumption.
Thus,theschemewasformulated,theregulator wasproposed,anditbecamepossibletocontrolthe lowofabrasivematerial.
6.DevelopmentofaControlDeviceBasedon FuzzyLogictoControltheWearResistance oftheHeatExchangeSurfacebyControl‐lingtheQualityofCoalbyDistributingCoal Flows
Thefollowingschemeofregulationofthemain lowsofTPPsisproposed.
Tosynthesizethecontroller,we irstconsid‐eredthesimulationmodeloftheCICSforregu‐latingthepowerunitpowersupplywithcoalfuel (Fig. 7),whichwasbuiltusing[8, 29].Thesimula‐tionwascarriedoutusingtheinteractivetoolMAT‐LAB®,Simulink®(LICENSING110721904–Math‐WorksTrial–22Oct2022).
Toregulatethepowerunitload,itissuf icientto useastandardPIDcontroller[30,31].
Inthiswork,attentionispaidtothedevelopment ofacontrolin luencetocomplywiththespeci ied abrasivenesscharacteristicsofcoalsuppliedforcom‐bustion.
In[6,7],theissuesofcalculatingtheratesofabra‐sivedamagetothepipepartoftheboilerunitduring fuelcombustionareconsidered.Thefollowingfor‐mula(6)isgiven:
T= (���� −��minw) (3,6⋅��sph ⋅GM), (6) whereTisthepossibleoperatingtimeoftheequip‐mentatthecurrentlevelofabrasiveness; ���� –pipelinewallthickness,mm; ��minw –standardmini‐mumpipelinewallthickness,mm;��sph –speci iclin‐earabrasivewearofthepipeline,mm/tofabrasivein thefuel lowofthecombustedmaterial;GM–mass lowrateofthematerial.
Thesheet(Fig.8)showsacomparisonoftheoper‐atingtimeatdifferentcoalabrasivenessvalues.The possibilityofdistributingthesuppliedcoal lowsin suchawayastoregulatetheabrasivenessofthemix‐turefeddirectlytothecombustionwasconsidered.
Takingintoaccounttheindicatorsoftheoperating time,theconditionalcoalclassesandthecontrolling in luenceonthecoalwereformulated,i.e.decisionson combustion,enrichment,refusalofcombustion,etc.
Giventhattheboundaryvaluesoftheclasseswere takenasconditional(fuzzy)sets,themostappropriate wasdecidedtousesystemsbasedonfuzzylogic.
In[32–35],thecontrolofprocessesbasedon fuzzylogicwasinvestigated,fromwherethreemain advantagesofusingafuzzycontroldeviceovertra‐ditionalregulatorsofautomaticcontroltheorywere emphasized:
‐ thepossibilityofcombiningadaptivetypecon‐trollersbasedonclassicalPIDcontrollers;
‐ developmentofcomplexcontrollersforcontrol objectsthataredif iculttodescribebyanalytical means;and
‐ afastertransitionbetweencontrolprocesses.
Usingthematerialfrom[36–38],wewillbuilda fuzzycontroldevice.
Asinputinformation lowsforthefuzzycontroller, wetaketheashcontentofthefuel(furtherAds in thecontroller’srules),thefullnessofthereserve store,andthedistributionofthefuel lowinthe correspondingfractionsinthefollowingdirectionsis takenasthecontrolin luence:toreplenishthereserve storedirectlyforcombustion(burning),toenrichment (concentrator),andcompleterejectionofthecurrent coalandtheuseofthereserve(reserve_out).
TheconditionproblemofFigure8.1.corresponds tothecoalclassesfromFigure8andwillin luencethe choiceoffuelaction,whileFigure 8.2.willin luence thereplenishmentanduseofTPPreserve.
Therulesof lowdistributionwerespeci iedas follows(Fig.9): Where:St– lowofre illofthewarehouse;Br–lowforfuelcombustion;Cn– lowtotheconcentra‐tor;Re– lowofthereservefuelusage;ands/n/lmean small/normal/large lowlevel.
Thus,theschemeofthecontrolsystem(Grand Controller)Figure10:
RulesarepresentedinFigure11
Theconstructedregulatorworksinsuchawaythat itdistributesinpercentagefractionsthedirectionsof steamcoal low.Itwasalsonecessarytocheckhow theregulatorworksatdifferentindicatorsofabrasive materialcontentinthefuel.
Modelingofthesystemshowedthat:
‐ Ifthecoalis“Perfect”,whenthereservestockis not illed,isalmostevenlydistributedbetweenthe furnaceandthereservestock,because,duetothe highcarboncontentandalmostzeroabrasivecon‐tent,thecombustionrequirementsarelowerthan forotherclassesofsteamcoal.
‐ Ifthecoalis“Good”,withanalmostfullandalmost emptystockpile,isdistributedbetweenthefurnace andthestockpileforreservereplenishmentinthe appropriateproportionsdependingontheneedfor areserve.
‐ Incaseofabrasivenessbetween“Normal”and “Unsatis ied”,thecoalisdividedbetweenthefur‐nace lowandtheenrichment lowandpartially mixedwiththereservecoal.
Atabrasivenessbetween“Unsatis ied”and“Bad” coalissentforbene iciationandmixedwiththe reserve.
AtaconstantAd =35%,thegraphshowsthat mostofthecoalfuel lowtothecombustionfurnace willcomefromthereserve,whilethedeliveredcoal willbedistributedbetweenthefurnaceandthebene‐iciation.
ThecasewhenAd isconstantlychangingiscon‐sidered.Itcontinuouslyincreasesfrom14%to35% during100‐timeunits.
TheresultsareshowninFigure13.2asfollows:
1) AttheinitialAd =14% (systemoperatingtime t0 =0 s),almosttheentirefuel lowissentto combustion.
2) Atthetimeofsystemoperationt1 =50s,theAd willchangeandwillbe24%.Therefore,thecon‐trollingin luencewillbethefollowingdistribution offuel lows–halfofthesteamcoalissentfor combustion,mixedwithreservefuel,andtherest issentforenrichment.
3) Attheendoftheexperiment(t2 =100)atAd = 35%,thelargestshareofthecombustedfuelwill bereserveenergycoal,whilethecoalfromthesup‐plierwillbepartiallyburnedandpartiallydirected toenrichment.
Withafuelashcontentof35%,withouta computer‐integratedcontrolsystem(CICS),TPP equipmentcanlastapproximately632days(less than2years)beforebreakingdown.However,with an ICS,iftheashcontentiskeptatthelevelofthe Normalclass,theequipmentcanlastfrom5to9 years.
Withasteadyincreaseinthecontentofabrasive materialinsteamcoal,theconsumption lowofthe reserveisincreased,andtheconsumption lowofthe suppliedashfuelisreduced.Thiswillhelpreducethe rateoferosionoftheheatexchangersurfacefromthe lowofabrasivematerialduringcombustion.Thus, itshouldbesummarizedthatwiththehelpofthe proposedregulator,thesettaskhasbeensolved.
Thispaperhasinvestigatedanddevelopedasys‐temforcontrollingthewearresistanceoftheheat exchangesurfaceofasteamboilerofacoal‐ ired powerplantbycontrollingthequalityofthecom‐bustedfuelbytheprocessofdistributingsteamcoal lowswithdifferentabrasivenesscontentusingfuzzy control.
Theproblemofmeasuringthecurrentquality ofcoalwasinvestigatedbycalculatingandcompar‐ingtheproposedequipmentandmodeledmeasuring channelofabrasivematerialcontentinsteamcoalfor aCICS.
Thenextstepwastodevelopamathematical modeltoidentifytheinconsistencyoffuelqualityindi‐catorsduringitscombustionintheTPPfurnace.The modelwasformulatedintheformofaparametric scheme,takingintoaccounttheregulator,asystemof equations,andtheprocessoffuelcombustioninthe lowsofinputfuelandoutputemissionswasrecorded intheformofadifferentialequation,wherethecoal abrasivenessindexwasvariable.
Subsequently,acontroldevicebasedonfuzzylogic wasdeveloped.Fortheintroductionofthefuzzycon‐troller,aconditionaldivisionofcoalqualityinto ive
classeswasproposed,and,accordingly, ivecontrol in luenceswereproposed.Therulesforthedistribu‐tionofcoal lows,whichwillguidetheregulatorofthe CICS,wereformulatedandwrittendown,andcom‐putersimulationwascarriedouttocontrolthewear resistanceoftheheatexchangesurfacebycontrolling thequalityofcoalbydistributingthe lowsofcoalsent forcombustion.
Thedevelopedcontrolsystemhasbeenvalidated bysimulatingtheplantcontroltodeterminetheopti‐malcontrolactionfordifferentcoalqualities.Inaddi‐tion,thisCICSsuccessfullyreducestheharmfuleffects ontheequipment.
Theobtainedresultsofcomputersimulationcon‐irmthehighef iciencyoftheuseoffuelenrichment andthefuzzyCICS,whichallowsfortheobservation ofthecombustionoftherequiredamountofcoal tomaintaintheproperlevelofgridcapacitybutto reducetheharmfuleffectsofwearresistanceofthe heatexchangerofthecoal‐ iredpowerplant.
Furtherresearchshouldconsiderthelogistical problem,inparticular,themanagementoftransport delayofsteamcoalsupplyundertheconditionof differentfuelquality,aswellasproposeamethodfor controllingthesystemasawholeincombinationwith afuzzycontrolsystemofTPP.
AUTHORS
MaksymGrishyn∗ –DepartmentofSoftware andComputer‐IntegrationTechnologies,National University“OdesaPolytechnic”,65000Odesa,Ukraine, e‐mail:grishyn.m.v@opu.ua.
KostiantynBeglov –DepartmentofSoftware andComputer‐IntegrationTechnologies,National University“OdesaPolytechnic”,65000Odesa,Ukraine, e‐mail:beglov.kv@op.edu.ua.
∗Correspondingauthor
References
[1] IEA,“Worldgrosselectricityproductionby source,”2019;https://www.iea.org/data‐and‐statistics/charts/world‐gross‐electricity‐production‐by‐source‐2019
[2] IEA,“EnergyStatisticsDataBrowser,”2022;http s://www.iea.org/data‐and‐statistics/data‐tools/energy‐statistics‐data‐browser.
[3] M.R.Kadagala,S.Nikkam,andS.K.Tripathy, “AReviewOnFlotationOfCoalUsingMixed ReagentSystems,” MineralsEngineering, vol.173,2021,107217,ISSN0892‐6875, doi:10.1016/j.mineng.2021.107217.
[4] M.Polat,H.Polat,andS.Chander,“PhysicalAnd ChemicalInteractionsInCoalFlotation,” InternationalJournalofMineralProcessing,vol.72, no.1–4,2003,pp.199‐213,doi:10.1016/S0301‐7516(03)00099‐1.
[5] J.Ferrer‐Comalat,S.Linares‐Mustarós, J.M.Merigo,andJ.Kacprzyk,“Modelling
andSimulationinManagementSciences,” ProceedingsoftheInternationalConferenceon ModellingandSimulationinManagement Sciences(MS-18):Proceedingsofthe InternationalConferenceonModellingand SimulationinManagementSciences(MS-18), 2020,doi:10.1007/978‐3‐030‐15413‐4.
[6] S.N.PelykhandM.V.Maksimov,“TheMethodOf FuelRearrangementControlConsideringFuel ElementCladdingDamageAndBurnup”, ProblemsofAtomicScienceandTechnology,vol.87, no.5,2013,pp.84–90, https://vant.kipt.khar kov.ua/TABFRAME.html
[7] M.V.Maksimov,S.N.Pelykh,andR.L.Gontar, “PrinciplesOfControllingFuel‐Element CladdingLifetimeInVariableVVER‐1000 LoadingRegimes”, AtomicEnergy,vol.112,no.4, 2012,pp.241–249,doi:10.1007/s10512‐012‐9552‐3.
[8] W.Wang,J.Liu,Z.Gan,Y.Niu,andD.Zeng,“Flex‐ibleControlOfCombinedHeatAndPowerUnits BasedOnHeat‐PowerEstimationAndCoordi‐nation”, InternationalJournalofElectricalPower &EnergySystems,vol.123,2020,106261,ISSN 0142‐0615,doi:10.1016/j.ijepes.2020.106261.
[9] W.Tan,J.Liu,F.Fang,Y.Chen,“Tuningof PIDControllersForBoiler‐TurbineUnits”, ISA Transactions,vol.43,no.4,2004,pp.571–583,ISSN0019‐0578,doi:10.1016/S0019‐0578(07)60169‐4.
[10] M.V.GrishynandK.V.Beglov,“EvaluatingThe EffectivenessOfFuelEnrichmentToReduce TheRiskOfPowerPlantCosts,” Včenìzapiski Tavrìjs’kogonacìonal’nogounìversitetuìmenìV. Ì.Vernads’kogo.SerìâTehnìčnìnauki, vol.32, no.3,2021,pp.82–89,doi:10.32838/2663‐5941/2021.3/14.
[11] Z.Dong,R.Wang,M.Fan,andX.Fu,“Switch‐ingAndOptimizingControlForCoalFlota‐tionProcessBasedOnAHybridModel,” PLoS ONE,vol.12,no.10,2017,e0186553,doi: 10.1371/journal.pone.0186553.
[12] Q.Tian,H.Wang,andY.Pan,“Associations ofGangueMineralsinCoalFlotationTailing andTheirTransportationBehaviorsin theFlotationProcess,” ACSOmega, vol.7, no.31,2022,pp.27542–27549,doi: 10.1021/acsomega.2c02988.
[13] A.I.BrunetkinandM.V.Maksimov,“The MethodForDeterminationOfACombustible GaseCompositionDuringItsCombustion,” NaukovyiVisnykNatsionalnohoHirnychoho Universytetu,vol.5,2015,pp.83–90, http://nvngu.in.ua/index.php/uk/arkhiv‐zhurnalu/za‐vipuskami/1132‐2015/zmist‐5‐2015/tekhnologiji‐energozabezpechennya/3 162‐metod‐viznachennya‐skladu‐goryuchikh‐gaziv‐pri‐jikh‐spalyuvanni.
[14] Y.V.Shcheglov,N.V.Fedorova,andD.A.Shaforost, “TheAbrasivePropertiesofCoalPower PlantsAshandSlagMaterials,” SolidState Phenomena,vol.299,2020,pp.845–851,doi: 10.4028/www.scienti ic.net/SSP.299.845.
[15] I.Kocaarslan,E.Çam,andH.Tiryaki,“A FuzzyLogicControllerApplicationFor ThermalPowerPlants”,EnergyConversion andManagement,vol.47,2006,pp.442‐458. doi:10.1016/j.enconman.2005.05.010.
[16] A.Z.Cipriano,“FuzzyPredictiveControl forPowerPlants”, AdvancedFuzzyLogic TechnologiesinIndustrialApplications,Advances inIndustrialControl,2006,pp.279–297,doi: 10.1007/978‐1‐84628‐469‐4_19.
[17] Y.P.KondratenkoandA.V.Kozlov,“Generation ofRuleBasesofFuzzySystemsBasedon Modi iedAntColonyAlgorithms,” Journalof AutomationandInformationSciences,vol.51, no.3,2019,pp.4–25,doi:10.1615/JAutomatIn‐fScien.v51.i3.20.
[18] O.Kozlov,Y.Kondratenko,H.Lysiuk,V.Kryvda, andO.Maksymova,“FuzzyAutomaticCon‐trolofthePyrolysisProcessfortheMunicipal SolidWasteofVariableComposition,” Journal ofAutomation,MobileRoboticsandIntelligent Systems,vol.16,no.1,2022,pp.83–94,doi: 10.14313/JAMRIS/1‐2022/9.
[19] S.Satyanarayana,R.K.Sharma,MuktaandA.K. Sappa,”AutomaticGenerationControlInPower PlantUsingPID,PSSAndFuzzy‐PIDController,” 2014InternationalConferenceonSmartElectric Grid(ISEG),Guntur,India,2014,pp.1–8,doi: 10.1109/ISEG.2014.7005618.
[20] O.Kozlov,G.Kondratenko,Z.Gomolka,andY. Kondratenko,“SynthesisandOptimizationof GreenFuzzyControllersfortheReactorsof theSpecializedPyrolysisPlants,” GreenITEngineering:Social,BusinessandIndustrialApplications,StudiesinSystems,DecisionandControl, V.Kharchenko,Y.Kondratenko,andJ.Kacprzyk, eds.,Springer,Cham,2019,pp.373–396,doi: 10.1007/978‐3‐030‐00253‐4_16.
[21] Y.P.KondratenkoandA.V.Kozlov,“Parametric OptimizationOfFuzzyControlSystemsBased OnHybridParticleSwarmAlgorithmsWithElite Strategy,” JournalofAutomationandInformation Sciences, vol.51,no.12,2019,pp.25–45.
[22] Q.Buetal.“TheEffectOfFuzzyPIDTemperature ControlOnThermalBehaviorAnalysis AndKineticsStudyOfBiomassMicrowave Pyrolysis”, JournalofAnalyticalandApplied Pyrolysis,vol.158,2021,105176,doi: 10.1016/j.jaap.2021.105176
[23] X.Liu,S.Wang,andL.Xing,”FuzzySelf‐Tuning PIDTemperatureControlForBiomassPyroly‐sisFluidizedBedCombustor,” 20102ndIEEE
InternationalConferenceonInformationManagementandEngineering,2010,pp.384–387, doi:10.1109/ICIME.2010.5477837.
[24] M.Ovcharenko,“DTEKinvestuie117mlnhrn naekolohichnumodernizatsiiuPrydniprovs’koii TES,” uprom.info;https://uprom.info/news/en ergy/dtek‐investuye‐117‐mln‐grn‐na‐ekologic hnu‐modernizatsiyu‐pridniprovskoyi‐tes/(in Ukranian).
[25] G.L.Fisher,D.P.Y.Chang,andM.Brummer, “FlyAshCollectedfromElectrostatic Precipitators:Microcrystal‐linesStructures andtheMysteryoftheSpheres,” Science, vol.192,no.4239,1976,pp.553–555,doi: 10.1126/science.192.4239.553.
[26] GuaranteesOfCitizens’EnvironmentalRights, Document1264‐XI,Article10,LawOfUkraine OnEnvironmentalProtection;https://zakon.ra da.gov.ua/laws/main/1264‐12?lang=en#Text
[27] V.G.Vasilenko,“MethodicalRecommendations forEvaluationofGreenhouseGasEmissionsby TypeofActivityofFacilities,AnnextotheOrder oftheMinistryofEnvironmentalProtectionand NaturalResourcesofUkraineonApprovalof MethodicalRecommendationsforEvaluationof GreenhouseGasEmissionsbyTypeofActivityof Facilities№404,”2021,https://mepr.gov.ua/fi les/docs/nakazy/2021/404%D0%BD%D0%B4 1.pdf(inUkranian).
[28] D.V.Chugunkov,G.A.Seyfelmliukova,V.P. Kuzema,andA.E.Bogdanova,“Researchon structureofash‐slagpulpanditsin luence onpipelines’attritionofathermalpower plants’hydraulicashremovalsystem,” Journalof Physics:ConferenceSeries,vol.1370,no.1,2019, doi:10.1088/1742‐6596/1370/1/012015.
[29] L.A.Kumar,A.Kalaiarasi,andY.U.Maheswari, PowerElectronicswithMATLAB,CambridgeUni‐versityPress,2017.
[30] L.Wang,S.Chai,D.Yoo,L.Gan,andK.Ng,“PID andPredictiveControlofElectricalDrivesand PowerConvertersusingMATLAB/Simulink,” IEEEPress,2015.
[31] L.Wang,“PIDControlSystemDesignandAuto‐maticTuningusingMATLAB/Simulink:Design andImplementationusingMATLAB/Simulink,” IEEEPress,2020.
[32] M.Jamshidi,V.Kreinovich,andJ.Kacprzyk, AdvanceTrendsInSoftComputing,Springer, 2013.doi:10.1007/978‐3‐319‐03674‐8.
[33] J.Kacprzyk,MultistageFuzzyControl:APrescriptiveApproach,JohnWiley&SonsInc.,1997.
[34] E.SzmidtandJ.Kacprzyk,”Distancesbetween intuitionisticfuzzysets,” FuzzySetsandSystems,vol.114,no.3,2000,pp.505–518,doi: 10.1016/S0165‐0114(98)00244‐9.
[35] E.SzmidtandJ.Kacprzyk.“AConsensus‐ReachingProcessUnderIntuitionisticFuzzy
PreferenceRelations”,InternationalJournalof IntelligentSystem,vol.18,2003,pp.837–852, doi:10.1002/int.10119.
[36] W.Pedrycz,K.Li,andM.Reformat,“Evolution‐aryReductionOfFuzzyRule‐BasedModels,” FiftyYearsofFuzzyLogicanditsApplications, Springer,2015,pp.459–481,doi:10.1007/978‐3‐319‐19683‐1_23.
[37] J.Jantzen, FoundationsofFuzzyControl:APracticalApproach,2nded.,JohnWiley&SonsInc, 2013,doi:10.1002/9781118535608.
[38] S.N.Sivanandam,S.Sumathi,andS.N.Deepa, IntroductiontoFuzzyLogicusingMATLAB, SpringerInternationalPublishing,2007,doi: 10.1007/978‐3‐540‐35781‐0.
Submitted:11th July2023;accepted:10th October2023
IsmailBogrekci,PinarDemircioglu,MustafaYasirGoren
DOI:10.14313/JAMRIS/1‐2024/3
Abstract:
Alow‐costsmall‐scaleautonomousvehiclereferstoa self‐drivingvehiclethatisdesignedtobeaffordable andsuitableforsmallerapplicationsorspecificpur‐poses.Inthisstudy,thefireflyalgorithmwasutilizedto addressobstacleavoidancechallengesinthepresenceof dynamicorstaticallypositioneduncertainobstacles.The autonomousvehiclesuccessfullyreachedtheintended destination,demonstratingasatisfactorylevelofaccu‐racy.Regardlessofthestartingpoint,thevehiclearrived atthepredeterminedpositionwithinanareameasuring 5metersindiameter.Theachievementofsuchresults canbeattributedtothecost‐effectiveselectionofsen‐sors,utilizationofasimplealgorithm,andtheimple‐mentationofamoderatelypoweredprocessorandcircuit components.
Keywords: Autonomousdrive,Unmannedgroundvehi‐cle,Sensors,Fireflyalgorithm
1.Introduction
Thesigni icanceoflow‐costsmall‐scale autonomousvehiclesinvariousdomainsand applicationsisnoteworthy.Severalkeyreasons contributetotheirvalue:
Accessibility:Low‐costsmall‐scaleautonomous vehiclesincreasetheaccessibilityofautonomoustech‐nologytoawiderrangeofusers.Thiseliminates inan‐cialbarriersandenablesindividuals,researchers, hobbyists,andsmallbusinessestoexploreandexper‐imentwithautonomoussystems.
EducationandResearch:Small‐scaleautonomous vehiclesprovideapracticalandhands‐onplatform foreducationalinstitutions,researchers,andstudents toengageinlearningandconductingexperimentsin ieldssuchasrobotics,arti icialintelligence,control systems,andcomputervision.Theyfacilitatethestudy ofautonomousvehiclealgorithms,behavior,andsen‐sorintegrationwithincontrolledenvironments.
TestingandPrototyping:Small‐scaleautonomous vehiclesarewell‐suitedfortestingandprototyping newalgorithms,software,andhardwarecomponents. Theyenabledeveloperstovalidatetheirideas,per‐formsimulations,andgatherreal‐worlddataona smallerandmoremanageablescalebeforetransition‐ingtolargerandmoreexpensiveplatforms.
InnovationandEntrepreneurship:Low‐cost small‐scaleautonomousvehiclesfosterinnovation andentrepreneurshipbyempoweringindividuals andstartupstodevelopnewapplicationsandservices basedonautonomoustechnology.Theyserveas afoundationforbuildingproofs‐of‐conceptand minimum‐viableproductsinindustriessuchas deliveryservices,agriculture,surveillance,and environmentalmonitoring.
SkillDevelopment:Engagingwithlow‐costsmall‐scaleautonomousvehiclespresentsanopportunity forindividualstodevelopskillsinareassuchas programming,robotics,sensorintegration,andsystem integration.Thisfacilitatesthegrowthofatalent poolcomprisingautonomoussystemdevelopersand professionalswhocontributetotheadvancementof the ield.
SafetyandTestingGrounds:Small‐scale autonomousvehiclescanserveastestinggrounds forevaluatingandre iningautonomoussystemsand safetyprotocolsbeforereal‐worlddeployment.They providecontrolledenvironmentsforidentifyingand addressingpotentialrisksandchallengeswithout compromisingsafety.
TechnologicalAdvancement:Thedevelopmentand adoptionoflow‐costsmall‐scaleautonomousvehi‐clesdrivetechnologicaladvancementsinsensortech‐nology,arti icialintelligence,machinelearning,and computervision.Thisfostersinnovationandpushes theboundariesofautonomoussystems,resultingin improvedef iciency,reliability,andperformance.
Insummary,low‐costsmall‐scaleautonomous vehiclesplayacrucialroleindemocratizing autonomoustechnology,promotingeducationand research,facilitatinginnovationandentrepreneur‐ship,andadvancingthe ieldofautonomoussystems asawhole.Theyserveassteppingstonesforindi‐vidualsandorganizationstoexplore,experiment,and contributetothegrowingecosystemofautonomous vehiclesandrelatedapplications.
Rapidadvancesinautonomousvehicle(AV)tech‐nologyareexpectedtobringaboutatransforma‐tionintransportationhabits.Despitetheirlimited presenceontheroad,publicpreferences,acceptance, andadoptionintentionsrelatedtoAVshavebeen thesubjectofinvestigationbyagrowingbodyof research[1].Autonomousvehicleliteraturereviews provideinsightsontech,control,sensors,human
factors,security,andprivacy,informingresearch’s currentstateandfuturedirections.
Theacceptabilityofdifferentautonomousvehi‐clebehaviorsincon lictsdependsonvariousfac‐torslikesocietalnorms,legalconstraints,andethical frameworks.Understandingthesein luentialfactors iscrucialforcreatingeffectiveguidelinesandpoli‐cies.Futureresearchcanexplorespeci icaspectslike ethics,safetyalgorithms,real‐timedecision‐making, andhuman‐machineinterfacesinmoredepth[2].
Thelong‐termeffectsofautonomousvehicleson thebuiltenvironmenthavegainedsigni icantatten‐tionduetothepotentialtransformativeimpactof thistechnology.Developingconceptualframeworksto studythelong‐termeffectsofautonomousvehicles onthebuiltenvironmentrequiresaninterdisciplinary approach.Incorporatingelementsfromurbanplan‐ning,transportationengineering,environmentalsci‐ence,socialsciences,andpublicpolicycanprovidea comprehensiveunderstandingofthecomplexinterac‐tionsandpotentialconsequences[3].
Designinganddevelopingthesoftwarestackof anautonomousvehicleusingtheRobotOperating System(ROS)inconjunctionwithhardwaremod‐ulesresponsibleforcontrollingthecarrequirescare‐fulintegrationbetweensoftwareandhardwarecom‐ponents.Throughoutthedevelopmentprocess,itis essentialtoconsidersafety,reliability,andsystem redundancy.Implementmechanismstohandlesen‐sorfailures,communicationerrors,andemergency situations.Adheretosafetyguidelinesandregulatory requirementstoensuretheautonomousvehicleoper‐atessafelyandcomplieswithapplicablelaws.Addi‐tionally,considerleveragingexistingROSpackages, libraries,andtoolsthatprovidefunctionalitiesforsen‐sorintegration,actuatorcontrol,andplanningand controlalgorithms.TheROSecosystemoffersnumer‐ousresourcesthatcanacceleratedevelopmentand provideasolidfoundationforautonomousvehicle softwarestacks[4].
AsAVtechnologyevolves,thereisapossibility thattraf iclanesandon‐streetparkingspotscould bedownsizedorrecon iguredtoaccommodatethe ef iciencyandsafetyfeaturesofAVs.Thisdownsizing couldresultintheavailabilityofadditionalspareroad spaceinfutureurbanstreets.Itisessentialforurban planners,policymakers,andcommunitiestoproac‐tivelyconsiderthepotentialrepurposingofspareroad spaceasAVtechnologyadvances.Throughcareful planningandcollaboration,citiescanleveragethis opportunitytocreatemorelivable,sustainable,and people‐centricurbanenvironments[5].
Researchonpathplanningforautonomousvehi‐clesbasedontheFrenetsystemhasgainedsigni icant attentioninrecentyears,providingamathematical frameworkfordescribingthemotionofaparticle alongacurveinthree‐dimensionalspace.Itisparticu‐larlyusefulforpathplanninginautonomousvehicles asitallowsforef icienttrajectorygenerationandcon‐trol.Theroadbehaviorofacarwassimulatedusing a ive‐foldpolynomialalgorithmmodel,whichallows
forthegenerationofpathtrajectoriesthatmimicdif‐ferentdrivingbehaviors.
Byanalyzingtherateofchangeoflateralandverti‐calvelocity,aswellaslateralandverticalacceleration undervariousbehaviors,itbecamepossibletoesti‐matethepredictiontimeforthecar[6].
Autonomousvehiclesrelyonacombinationof sensorstoperceivetheirsurroundingsandmake informeddecisions.Inthisreview,alistofsensorslike LiDAR(LightDetectionandRanging),Radar(Radio DetectionandRanging),andCameras(RGB,monocu‐lar,stereo,ormulti‐camerasetups)commonlyusedin autonomousvehiclesareexplainedindetail[7].
Of linemappingforautonomousvehicleswith low‐costsensorsgaveafeasibleapproach,especially whenhigh‐precisionmappingdatawasnotastrict requirement[8].Whilelow‐costsensorsmaynotoffer thesamelevelofaccuracyashigh‐endsensors,they canstillprovidevaluabledataforbasicmappingpur‐poses.
Vision‐basednavigationandguidancesystems offernumerousbene itsinagriculturalapplications, includingincreasedef iciency,reducedlaborrequire‐ments,improvedaccuracy,andoptimizedresource utilization.Ongoingadvancementsincomputervision, machinelearning,androboticscontinuetoenhance thecapabilitiesandreliabilityofthesesystemsinthe agriculturalsector[9].
Map‐basedlocalizationmethodsusing3D‐LiDAR (LightDetectionandRanging)sensorshaveproven tobeeffectiveinprovidingaccurateandrobustlocal‐izationforautonomousvehicles.Byleveragingthe richspatialinformationcapturedby3D‐LiDARsen‐sors,thesemethodsenablevehiclestodetermine theirpositionwithinapre‐builtmap.Curb‐map‐based localizationleveragestheuniquecharacteristicsof curbsidefeatures,whicharerelativelystableanddis‐tinguishableinurbanenvironments.Byfocusingon curbsandassociatedfeatures,thisapproachcanpro‐videpreciseandreliablelocalization,eveninchalleng‐ingscenarioswithlimitedGPSavailabilityorcom‐plexroadlayouts.Itisimportanttonotethatcurb‐map‐basedlocalizationmaybeusedincombination withothersensorinputs,suchasGPS,IMU,orcamera data,toenhancetheoveralllocalizationaccuracyand robustness[10].
Nonetheless,thefull‐scaledeploymentof autonomousvehiclescontinuestofacesigni icant obstaclesconcerningsafetyconcerns.Theseconcerns stemfromarangeofissueswithinthevehicles themselvesandexternalfactorsintheiroperational environments.Addressingthesesafetychallengesis imperative.Sensordataplaysacriticalroleinthis endeavorbyprovidingvaluableinsightsintothe currentoperationalstatusofautonomousvehicle systemsandthein luenceofexternalenvironmental factors.Suchdatahelpsinmonitoringandmitigating risks,contributingtotheoverallsafetyandreliability ofautonomousvehicletechnology[11,12].
IntheMaterialsandMethodssection,thestudy examineseachstageandresultseparately,encom‐passingequipment,algorithms,andmethods.
Firstly,thebillofmaterialsisprovidedinFig‐ure1.Whenselectingcomponents,considerationsare giventofactorssuchascost,functionality,compati‐bility,andeaseofaccesstoresources,aimingforsuc‐cessfulimplementationintheexperimentalresearch. Thematerialsaredescribedwithgeneralinformation, includingvisualsofthecomponents,technicalspeci i‐cations,andbriefcommentsbasedontheconducted experimentsandstudies.
Furthermore,inthemethodsection,thedesign stagesofthevehicleareexplained,includingcalcu‐lationsandthesoftwarealgorithmforautonomous driving.
TheL298NMotorDriverUnitisconsideredan optimalmotordrivermodulefordrivingDCandStep‐perMotors.Itiscomposedofa78M055Vregulator andL298motordriver.WiththeL298NModule,up to4DCmotorsor2DCmotorswithdirectionand speedcontrolcanbeoperated.Intheexperimental study,twoofthemwereusedasdirectionalandspeed controllers,resultinginthecontroloftwowheelsby1 L298Nmotordriver.
TheU‐BloxGY‐NEO6MV2GPSunitisaGPSmodule thatintegratesaU‐BloxNEO‐6MGPSreceiverwithan externalantenna.
TheArduinoMega2560isamicrocontrollerboard basedontheATmega2560microcontroller,responsi‐bleformanagingallthecomponentsoftheentirecir‐cuitandthefunctionsexpectedfromtheautonomous drive.TheselectionoftheArduinoMega2560was drivenbytheeaseofaccessingcodesources,thecost ofthemicrocontrollerunit,anditscompatibilitywith othercircuitmembers.
TheHC‐SR04isanultrasonicdistancesensor commonlyutilizedinvariousapplications,includ‐ingrobotics,automation,andproximitysensing.The ultrasonicmeasuringmoduleHC‐SR04technicallyis abletomeasurearangeof20mmto4000mmdueto itstechnicaldatasheetprovidedbythemanufacturer, witharangeaccuracythatmayreachupto3mm. Themodulesconsistofultrasonicsoundreceivers, transmitters,andcontrollingcircuits.Theessential workingprincipleinvolvesusingtheIOtriggertosend ahigh‐levelsignalforapproximately10us.Thesensor emitsan8,40kHzultrasonicpulseanddetectsthe returnsignalpulse.ThedurationofthehighoutputIO time,whenthesignalreturns,correspondstothetime takenfromultrasonictransmissiontoreception.
TheHMC5883Lisamagnetometersensor designedtomeasuremagnetic ieldstrengthand direction.Itiscommonlyemployedinapplications suchasnavigation,robotics,andmagnetometer calibration.
The6VDCbrushedmotorwithareducerand wheelrepresentsastandardcon igurationutilizedin thisexperimentalstudy.
Firstly,whendeterminingthebasicdimensionsof thevehicle,carefulconsiderationwasgiventoeluci‐datehowthedecisionsweremade.Oneofthemain factorstakenintoaccountwasthemanufacturability oftheparts,whichledtotherealizationthat3Dprint‐ingwasthemostsuitableoptionforimplementingthe experimentalcar.Consequently,thissetaconstraint onthedesigntokeepthedimensionsascompactas possible.Additionally,thefunctionalityofthesensors andelectromechanicalpartsposedfurtherconsidera‐tionsthatnecessitatedlongerdimensions.Ultimately, consideringthesevariousconditions,thedimensions oftheautonomouscarweredetermined.
InFigure 2,itcanbeobservedthatthewheels havebeenpositionedata5‐degreecamberangle.The reasonfortheselectionofapositive5‐degreecamber angleistoensurethatthetireremainsconnectedtoits reducerwithouttheneedforfasteningorgluing.Fur‐thermore,whentheshockabsorbersarecompressed, thecamberanglechangestoanegativevalueifitwas initiallysetatzerodegrees.However,ifthecamber angleissettoapositivevalue,evenwhenloadedat fullcapacity,thecamberanglewillremainpositiveor zero,therebymaintainingvehicledynamicsanddrive stability.
Pathplanningalgorithmsplayacriticalrolein autonomousvehicles’abilitytonavigatesafely,ef i‐ciently,andadaptivelyindynamicenvironments.
obstaclede initionisillustrated,whichisapplicableto objectswithaheightgreaterthan30mm,considering theirdynamiccapabilities.
Thesealgorithmsoptimizetrajectories,ensurecol‐lisionavoidance,handlecomplexmaneuvers,consider humaninteraction,andcontributetooverallef iciency andreliability.Thepathplanningalgorithmfunctions bydeterminingtheshortestlinebetweenthestarting pointanddestinationpoint,asillustratedinthe igure below.Sensors2,3,andfourservethesamepurpose asshowninFigure8,buttheplacementofthreesen‐sorsextendstherangeofobstacledetection.
BytheapplicationoftheFire lyAlgorithm(FA)to pathplanning,thesearchspaceofpossiblepathscan beexploredbytheautonomousvehicle.Thesepaths areevaluatedbasedontheir itnessvaluesanditer‐ativelyre inedto indoptimalornear‐optimalsolu‐tions,consideringthede inedobjectivefunction.
Itshouldbeemphasizedthattheperformanceand suitabilityoftheFire lyAlgorithmasanoptimization techniqueforpathplanningdependonthespeci ic problem,environment,andobjectives.Toassessand adapttheFire lyAlgorithmforoptimalresultsina givenapplicationscenario,comparisonswithother pathplanningalgorithmsandcarefulparametertun‐ingarenecessary.
Duringthecalculationofpositionsandbearing degrees,theshortestrouteisgeneratedtonavigate towardthedestination.Intheeventofanobstacle detectedbythesensorsatadistanceshorterthan 520mm,thevehiclewillsteerinanotherdirectionto bypasstheobstacle.Afterplacingsensorsat10degree anglebetweengroundandsensorsnormalaxis,ideal distancehadbeencalculatedas514.83mmtodetect 30mmobstacle.That514.83mmdistancehadbeen roundedto520mmbyself‐decisionduetomakethe calculationseasierandgainingadditionalsaferdis‐tance5.17mm.Atthispoint,theFire lyAlgorithm (FA)loopisinitiatedtonavigatearoundobstacles withintheshortestdistance.InFigure4,thevehicle’s
Theconditionforoneofthesensorsissetatasafe distanceof520mm(whichisanoptimizedvalue)to ensurethatobstaclesarenotapproached.Insitua‐tionswherethisconditionisnotmet,theAVbreaks itsheadingloopandinitiatestheFAalgorithmloop togenerate ire liesinthevicinityoftheobstacles,as demonstratedinFigure5.
Severalrandom ire liesareproducedandposi‐tionedneartheobstacles,andthebrighter ire lies areselectedfromthisgroup.Brighter ire liesreferto newstartingpointsthatprovidethemaximumsafe distancebetweentheobstacleandthe ire ly.TheAV maintainsthisgenerationandselectionprocessof thebrightest ire lyuntilitsuccessfullyavoidsobsta‐clesthatcanbedetectedbytheultrasonicsensor distances.
The lowchartoftheFire lyAlgorithmisexplained inFigure 6,andthepseudocodeoftheFire lyAlgo‐rithmisprovidedinFigure7
BeforetheapplicationoftheFire lyAlgorithm(FA) formulationtothesoftwareoftheautonomousvehicle (AV),theAV’sresponsetoencounteringanobstacle canbesummarizedinthefollowingsteps:
Initialization:TheAVandthetargetpositionare initialized.
CalculationofHeadingDegree:Theheading degreebetweentheAV’scurrentGPSpositionandthe targetpositioniscalculated.
ObstacleDetection:Duringtheheadingphase,if anobstacleisdetectedbytheAV,theheadingloopis interrupted,andtheFAloopisactivated.
Fire lyPopulationGeneration:Apopulationof ire‐liesisgeneratedbytheAVinthevicinityofthe detectedobstacle.
BrightestFire lySelection:TheAVemploysa it‐nessequationtoselectthebrightest ire lyfromthe generatedpopulation.
HeadingLoopActivation:TheAVactivatesthe headingloopagaintocalculateanewroutebetween thebrightest ire ly’spositionandthetargetposition.
Byfollowingthesesteps,theAVdynamically adjustsitspathandnavigatestowardthetargetwhile effectivelyavoidingobstaclesencounteredalongthe way.
Dfo = (xO xfi)2 +(yO yfi)2 (1)
TheEuclideandistance,referredtoasDfo, betweenthepositionofa ire lyandanearbyobstacle isacrucialparameterinthealgorithm.Inthisstudy, theDfovaluesareobtainedthroughsensorreadings, speci icallyfrom ivesensors(seeFig. 8).These sensorreadingsserveasthecalculatedDfovalues,as representedinEquation(1).
Dfg = (xg xfi)2 +(yg yfi)2 (2)
DfgistheEuclideandistancebetween ire lyand targetpointshowninEq.(2).
���� =��1 1 min���� ∈����‖��fo‖ +��2 ⋅‖Dfg‖ (3)
Thecalculationofthe ire ly’spathoptimization, denotedas i,isperformedusingtheformulapro‐videdinEquation(3).Inthisequation,K1represents aparameterthatsigni iesthesafetylevelofthepath, whileK2denotesaparameterde iningthemaximum andminimumpathlengthsforrouting.Aftertheposi‐tionsandparametersarecomputedandsetbythe user,themicrocontrollerunit(MCU)initiatestheexe‐cutionofthealgorithmdescribedinEquation(3),sub‐sequentlydeterminingtheoptimal ire ly.
NavigationwithObstacle:TheAVproceedsto movetowardthetargetpositionwhileencountering anobstacle.IftheAVencountersanotherobstacle duringthisprocess,steps3to6arerepeatedtoensure obstacleavoidance.
Finally,asillustratedinFigure8,theautonomous vehicle(AV)calculatesanewroutebetweentheinitial point(selected ire lybytheMCU)andthe inalpoint (targetsetbytheuser).
Thelibraryincludesallthenecessaryfunction keysforuser‐de inedoperations.Additionally,amath libraryhasbeenincorporatedtoperformcalculations relatedtopositionsdetectedbysatellites.Aftercon‐iguringthepowerandsignalpinsoftheGPSmodule, avoidGPSloophasbeenimplemented,asdepictedin Figure9.
Forthebearingdegreecalculationsofthemagne‐tometer,formulaswerewritteninthesoftwareedi‐torprogramofArduino.Magneticdeclinationisalso consideredwhilecalculatingthebearingdegreeofthe vehicle(Fig.10).
Thevehicleoperatesusingtwomainloops.The irstloopisresponsibleforcalculatingtheheading, bearingdegree,andpositionofthevehicle,allowingit tonavigatetowardadesireddestination.Asthevehi‐cleapproachesthedestination,theheadingdegree isrecalculatedbasedongeometricrulestoensure accuracy.
Thesecondloopisdedicatedtoobstacleavoid‐ance.Thevehiclereliesonsensorsasits“eyes”to detectobstacles.Ifthedistancebetweenthevehicle andanobstaclefallsbelowtheprede inedsafedis‐tanceof520mm,themicrocontrollerinterruptsthe mainloopandswitchestotheobstacleavoidanceloop. Inthismode,themicrocontrollerprovidesdirectives tosteerthevehicleandpreventcollisionsuntilasafe distanceismaintained.Onceallthesensorsdetectand con irmtheabsenceofobstaclesalongthevehicle’s path,themicrocontrollertransitionsbacktotheloop thatdirectsandguidesthevehicletowarditsdesig‐nateddestination.
Theexperimentwasdesignedtobeconductedin outdoorconditions,involvingobstacleswith ivedif‐ferentgeometricshapesconstructedfromcardboard. Thesespeci icshapeswerechosentoevaluatethe vehicle’sabilitytoavoidobstacles.The iguresbelow (Figure 11(a)–11(e))illustratethe ivedistinctgeo‐metricobjectsusedintheexperiment.
The ivedifferentshapeswereselectedbasedon theirlevelofdif icultyfordetectionbyultrasound sensors.Theseshapeswillbepositionedinvarious orientationsduringthetenmeasurementsofthevehi‐cle’sperformance.Thecrenel‐shapedobstacle,inpar‐ticular,waschosenduetoitscomplexstructure,pre‐sentingasigni icantchallengeforultrasoundsensors.
Thevehicle’sratedspeedhasbeenmeasuredas0.5 meterspersecond.Toevaluateitsspeedcapabilities, aspeedtestwasconductedonthestreet,coveringa distanceof2.5meterswithin5seconds.Byapplying theformulaspeed = distance ÷ time,aspeedof0.5 m/swascalculated.
Whileperformingthesmall‐scaleautonomous vehiclesoutdoortest,thetemperatureofthesunny daywas24degreesCelsius,thehumiditywas%52, andthewindspeedwas12km/h,1018hPa,anddur‐ingthetest,thevalueswerealmostexactlythesame duetotheshorttestdurations.Inadditiontothat, aircurrentsareimportantformeasurementaccuracy; however,aircurrentsmustbeatseriouslevels,such asinstormyweather,whichhasspeedsover60km/h. Duringtestday,thewindspeedwas12km/h,which isafairlevelofaircurrentthatwouldbeneglectedfor accuracy.
BasedontheinformationprovidedinFigure 12, theinitialpositionoftheautonomousvehicle(AV) wasdeterminedusingGPSreadingsas38.516534, 27.044097.ThetargetpositionfortheAVwasselected as38.516808,27.043947.Usingthebearingdegree calculationformula,themicrocontrollerunitoftheAV determinedthebearingdegreetobe 23.18degrees. Toobtaintheactualbearingdegree,360degreeswere addedtothenegativevalue,resultingina inalbear‐ingdegreeof336.81degrees.Additionally,thetravel distancewascalculatedtobe32.94meters.
WhentheAVwaspositionedtothetargetloca‐tions,sensorreadingswerepresentedabovein Figure 13.Withthesesensorreadings,thepercent‐ageoferrorwouldbecalculated.Firstly,theaverage valueoftheeightexperimentalreadingswillbecalcu‐latedas:
336.45+336.22+336.30+336.37
+336.60+336.30+336.29
+336.37
8 =336.36 (4)
%Error= |ExperimentalValue TheoreticalValue| TheoreticalValue ×100 (5)
ThenfollowingEq.(5)wasusedtocalculateper‐centageoferrorformagnetometersensor:
%Error= |336.36−336.81| 336.81 ×100=%0.13Error (6)
Thenavigationprocessexhibitedaremarkablylow leveloferror,whichishighlyfavorable.Additionally, thecontinuousmeasurementofsensorswhilethe autonomousvehicleisprogressingtowardthetar‐getdestinationsigni icantlyminimizesanypotential errors,especiallyasthedistancetraveledbecomes shorter.
Anotherroutingtestwasconductedatadifferent location,asdepictedinFigure14(a).Theinitialposi‐tionwasdeterminedas38.447223,27.226783,and thetargetpositionwassetas38.447524,27.227266. Thecalculatedbearingdegreeforthisparticularroute was51.49degrees.
Duringtheroutebetweenthetwopositions, ive differentobstacleswithvaryinggeometricshapes wererandomlyplaced,asdepictedinFigure 14(b) Theautonomousvehicle(AV)operatedatarated speedof0.5m/s.Atotaloftenmeasurementswere conducted,recordingthetimetakenandthepathtrav‐eledbytheAVduringeachmeasurement.
Thetimemeasurementswererecordedusinga stopwatchonamobilephone.Thisprocesswas repeatedtentimesforeachcase.Thepathstraveled bythevehicleweremanuallymarkedonasketch‐book.ThepointsweremeasuredusingGoogleMaps, asdepictedinFigure15(a).
Theidealpath,whichistheshortestlinebetween thetwopositions,wascalculatedtobe98.25meters. ThisidealpathisrepresentedbytheyellowlineinFig‐ure15(b).Ontheotherhand,theEuclideandistance betweenthetwopositionsis53.52meters.
Duringtheexperimentaldrive,asimpleroutewas chosentoconducttests.Theinitialpositionwasat coordinates38.447223,27.226783,andthetarget positionwasatcoordinates38.447524,27.227266. Thevehiclecalculatedthebearingdegreeas51.49 degrees.
Asthevehiclestartedtomove,itdeterminedthe shortestpath,representedbytheyellowhatchedline. However,duetothepresenceofsidewalks,thevehicle followedthepathindicatedbythebluecontinuous line.Thevehicledetectedthesidewalksasobstacles, soinsteadofdrivingoverthem,itchosetostayonthe road.Theexperimentaldrivewasrepeatedtentimes, asdepictedinFigure16.
Uponcompletingtheexperimentalmeasurements, the9thtrialdrewattentionduetoitstraveldistance of120meters,whichwas21.75meterslongerthan theidealpathlengthof98.25meters.Thisdisparity maybeattributedtoerrorsinobstacleavoidanceand GPS inalpositionestimation.Theremainingvalues appearedtobewithinanacceptablerange.Inorder tocalculatetheerror,itisnecessarytodeterminethe averagedistancetraveledbytheautonomousvehicle (AV).
113.4+108.1+106.7+105.5+110.8
ByusingEq.(6):
Theerrorvalueof12.3%needstobeevaluateddue toitseffectontraveltime.Itisevidentthatthis12.3% errorwillleadtoa12.3%increaseintraveltime.
Thevehicle’sarrivalwascompletedwithinacircle withadiameterof5meters,asdepictedbytheblue hatchedlinesinFigure14(a).Basedonobservations, anaccuracyof2.5metersinradiusisconsidered acceptable.
TheFire lyAlgorithm,knownforitseffective‐nessincomplexandcrowdedenvironments,has demonstrateditscapabilitytohandlebothlinearand nonlinearproblems.Itexhibitsahighconvergence speedwhilenotrequiringahigh‐performanceMCU (MicrocontrollerUnit)andhasshownfastresponses duringtheAV’smission.
TestsconductedwithoututilizingtheFire lyAlgo‐rithmresultedinconfusionduringseveraltrials.It appearedthattheAVencountereddif icultiesinsolv‐ingobstacleavoidanceproblems,leadingtoastateof confusion.
Ultimately,theadvantageofthisalgorithmlies initssimplestructure,whichdoesnotnecessitate acomplexandexpensivecontrollingunit.Thecost advantagesassociatedwithitfurtherenhanceits applicability.
Theprimaryobjectiveofthisstudywastodesign andmanufactureanautonomousvehicle(AV).Dur‐ingthedesignphase,carefulconsiderationwasgiven topotentialcomponentssuchascameras,ultrasonic sensors,andLiDARs,takingintoaccountfactorslike cost,compatibility,andalgorithms.Afterthorough researchandevaluation,itwasdeterminedthatultra‐sonicsensorswerecapableofdetectingobstacles effectivelyinoutdoorconditionswhilealsooffer‐ingacostadvantageandastraightforwardworking principle.
Thealgorithmwasintentionallydesignedtobe simpleinordertoavoidencounteringcomplexbugs duringsimulateddeliveryoperations.TheGPSposi‐tionerrorsremainedwithinamanageablerange,with adiameternotexceeding5meters,whichwasdeemed suf icientfortheintendedoperations.However,the magnetometerwasoccasionallyaffectedbyenviron‐mentalconditionsthatcouldnotbepreciselyidenti‐ied.Thisinterferencemayhavebeencausedbyfac‐torssuchascellphonesignalsorotherelectroniccom‐ponentswithinthevehicle.Despitetheseoccasional challenges,theAVsuccessfullyreachedthetargetafter deviatingmomentarily,whichcanbeconsideredneg‐ligiblesinceitwastheinitialtrialontheroadsduring theadaptationphase.
Theactualimplementationandfeasibilityofsuch low‐costsmall‐scaleautonomousvehicleswilldepend onvariousfactors,includingtechnologicaladvance‐ments,regulatoryframeworks,andmarketdemand.
Inconclusion,thefollowingsuggestionsandrec‐ommendationscanbemade:
‐ Forapplicationsotherthanmailandpackagedeliv‐ery,advancedpositioningsensorsmayberequired toensureoptimalperformancebasedonspeci ic operationalneeds.
‐ Creatinglow‐costautonomousrobotscancollect dataonairquality,temperature,humidity,and otherparameters,helpingresearchersandenviron‐mentalagenciesgathervaluableinformation.
‐ Thenextstageofthestudywouldinvolveincor‐poratingmobileapp‐controlledpositionsandreal‐timevehicletrackingononlinemaps.Thiswould necessitatewirelesscommunicationandpresenta newandchallengingtaskincon iguringtheentire system.
AUTHORS
IsmailBogrekci –Dept.ofMechanicalEngineering, FacultyofEngineering,AydinAdnanMenderes University,Efeler,Aydin,Turkey,e‐mail: ibogrekci@adu.edu.tr.
PinarDemircioglu∗ –InstituteofMaterials Science,TUMSchoolofEngineeringandDesign, TechnicalUniversityofMunich(TUM),Garching, Munich,85748,Germany/Dept.ofMechanicalEng, FacultyofEng,AydinAdnanMenderesUniversity, Aydin,Turkey,e‐mail:pinar.demircioglu@tum.de; pinar.demircioglu@adu.edu.tr.
MustafaYasirGoren –SchneiderElectricInc., MechanicalDesignEngineer,Izmir,Turkey,e‐mail: m.yasirgoren@hotmail.com.
∗Correspondingauthor
References
[1] Q.Zhang,T.Zhang,andL.Ma,“HumanAccep‐tanceOfAutonomousVehicles:ResearchSta‐tusAndProspects,” InternationalJournalof IndustrialErgonomics,vol.95,2023,1–15.doi: 10.1016/j.ergon.2023.103458.
[2] G.Nativel‐Fontaine,V.Lespinet‐Najib,R.Cazes, C.Dupetit,C.DeGasquet,M.Chevrie,F.Aïoun, andL.Ojeda,“ExplorationOfTheAcceptabil‐ityOfDifferentBehaviorsOfAnAutonomous VehicleInSo‐CalledCon lictSituations,” Accident Analysis&Prevention,vol.186,2023,pp.1–11. doi:10.1016/j.aap.2023.107041.
[3] A.R.Pimenta,M.Kamruzzaman,andG.Cur‐rie,“Long‐TermEffectsOfAutonomousVehi‐clesOnTheBuiltEnvironment:ASystematic ScopingReviewTowardsConceptualFrame‐works,” TransportReviews,2023,pp.1–35.doi: 10.1080/01441647.2023.2189325.
[4] A.O.Prasad,P.Mishra,U.Jain,A.Pandey,A. Sinha,A.S.Yadav,R.Kumar,A.Sharma,G. Kumar,K.H.Salem,A.Sharma,andA.K.Dixit, “DesignAndDevelopmentOfSoftwareStackOf
AnAutonomousVehicleUsingRobotOperat‐ingSystem,” RoboticsandAutonomousSystems, vol.161,2023,pp.1–12.doi:10.1016/j.robot. 2022.104340.
[5] Y.Joo,S.Kim,B.Kim,G.H.Cho,andJ.Kim, “AutonomousVehiclesAndStreetDesign: ExploringTheRoleOfMediansInEnhancing PedestrianStreetCrossingSafetyUsingA VirtualRealityExperiment,” AccidentAnalysis &Prevention,vol.188,2023,pp.1–12.doi: 10.1016/j.aap.2023.107092.
[6] Y.WangandZ.Lin,“ResearchOnPathPlan‐ningForAutonomousVehicleBasedOnFrenet System”, JournalofEngineeringResearch,2023, pp.1–6.doi:10.1016/j.jer.2023.100080.
[7] H.A.Ignatious,H.Sayed,andM.Khan,“An OverviewOfSensorsInAutonomousVehicles,” ProcediaComputerScience,vol.198,2022, pp.736–741,doi:10.1016/j.procs.2021.12.315.
[8] Z.Wang,X.Zhao,andZ.Xu,“Of lineMapping ForAutonomousVehiclesWithLow‐CostSen‐sors,” Computers&ElectricalEngineering,vol.82, 2020,pp.1–11.doi:10.1016/j.compeleceng.20 20.106552.
[9] Y.Bai,B.Zhang,N.Xu,J.Zhou,J.Shi,and Z.Diao,“Vision‐BasedNavigationAndGuid‐anceForAgriculturalAutonomousVehiclesAnd Robots:AReview,” ComputersandElectronics inAgriculture,vol.205,2023,pp.1–20.doi: 10.1016/j.compag.2022.107584.
[10] L.Wang,Y.Zhang,andJ.Wang,“Map‐Based LocalizationMethodforAutonomousVehicles Using3D‐LIDAR”, IFAC-PapersOnLine,vol.50, no.1,2017,pp.276–281.doi:10.1016/j.ifacol .2017.08.046.
[11] S.Kitajima,H.Chouchane,J.Antona‐Makoshi, N.Uchida,andJ.Tajima,“ANationwideImpact AssessmentofAutomatedDrivingSystemson Traf icSafetyUsingMultiagentTraf icSimula‐tions”, IEEEOpenJournalofIntelligentTransportationSystems,vol.3,2022,pp.302–312.doi: 10.1109/Ojits.2022.3165769.
[12] H.T.Zheng,C.Y.Chen,S.Li,F.Zheng,S.E.Li, Q.Xu,andJ.Q.Wang,“Learning‐BasedSafeCon‐trolforRobotandAutonomousVehicleUsing Ef icientSafetyCerti icate,” IEEEOpenJournalof IntelligentTransportationSystems,vol.4,2023, pp.419–430.doi:10.1109/Ojits.2023.3280573.
[13] M.Y.Goren,“DesigningandManufacturingSmall ScaleAutonomousVehicle,”UnpublishedM.Sc. Thesis,2023‐M.Sc.‐043,AydinAdnanMenderes University,Turkey,2023.
Abstract:
Submitted:6th September2023;accepted:27th October2023
CesarMinaya,RicardoRosero,MarceloZambrano,PabloCatota
DOI:10.14313/JAMRIS/1‐2024/4
Thepaperpresentsanapproachforcontrollingaline‐followingrobotusingartificialintelligencealgorithms. Thisstudyaimstoevaluateandvalidatethedesign andimplementationofacompetitiveline‐followingrobot basedonmultilayerneuralnetworksforcontrollingthe torqueonthewheelsandregulatingthemovements. Theconfigurationoftheline‐followingrobotconsistsof achassiswithasetofinfraredsensorsthatcandetect thelineonthetrackandprovideinputdatatotheneural network.Theperformanceoftheline‐followingrobot onarunningtrackwithdifferentconfigurationsisthen evaluated.Theresultsshowthattheline‐followingrobot respondedmoreefficientlywithanartificialneuralnet‐workcontrolalgorithmthanwithaPIDcontrolorfuzzy controlalgorithm.Atthesametime,thereactionandcor‐rectiontimeoftherobottoerrorsonthetrackisearlier byabout0.1seconds.Inconclusion,thecapabilitiesofa neuralnetworkallowtheline‐followingrobottoadaptto environmentalconditionsandovercomeobstaclesonthe trackmoreeffectively.
Keywords: Robotics,Line‐followingrobot,Artificialneu‐ralnetworks
1.Introduction
Autonomousline‐followingrobotsinthelast decadeshavebeenofincreasinginterestfortheir involvementinvarious ieldsrangingfromindustry tohealthcare,education,logistics,transportation,and roboticcompetitions[1,2].Nowadays,robotdevelop‐mentfocusesonachievinghighprecision,speed,and stabilitylevels.Intelligentmobilerobotscombinecon‐trolengineering,electronics,computerscience,soft‐ware,andmechanics.
Anautonomousline‐followingrobotrecognizes andfollowsapathtracedbyablacklineona latwhite surface.Thecontrolsystemdetectsthelineandregu‐latestherobottokeepitonitspathwhileconstantly correctingfordeviations[3].Theserobotsareoften implementedinacademicsettings,suchasteaching techniquesinrobotics,controlsystems,orarti icial intelligence[4].Thearchitecturesharedbymostline‐followingrobotsincludesachassis,linedetectionsys‐tem,locomotionsystem,andcontrolunit.Varioussen‐sorscandetecttheseblacklinesdescribingthetraced routeforthis—theserangefromlow‐costdetection modulestoexpansivevisionsystems[5].
Numerousstudies[6–8]havehighlightedtheuse‐fulnessofinfraredsensorsforlinedetectionsystems. Thesearelocatedontheundersideoftherobotbase andemitabeamofinfraredlight,whichallowsusto detecttheamountofinfraredlightre lectedfromthe groundsurface.Themainreasonforchoosingthissen‐sorisitsrangeforlinedetectionfromaminimumof 100cmtoamaximumof500cm.Inaddition,theycon‐sumelowpowerandcanbeplacedinsmallspaces[9]. However,severalstudies[10–12]haveshownthatthe useofcamerascanbeanalternativeforlinedetec‐tionbycapturingimagesanddescribingtheground environmentwhilesendingthemtoanimagepro‐cessingsystemtodetecttheline.However,inthelast decades,authorshaveexperimentedwithnewtech‐niquessuchascolorsegmentation,edgedetection, ormoreadvancedmethodswithconvolutionalneural networkstoidentifyandseparatethebackgroundline andallowmoreaccuratetracking[13].
Therobotcontrolsystemallowsmonitoringand takingactiononthecollecteddataordetermining whatactiontherobotshouldtaketostayonthe line,suchasadjustingdirectionorspeed.In[14],the authorsdescribetheimplementationofa ieldpro‐grammablegatearrayknownasFPGAinthecontrol systemoftheline‐followingrobottodevelopthesen‐sordataprocessingandcontrolalgorithmef iciently. Inaddition,theFPGAcanbeeasilyreprogrammed andadjustedtosuitdifferentscenariosorspeci ic requirementsoftheline‐followingrobot.Mostofthese FPGAimplementationsaretask‐orientedfortheentire roboticsystemorareusedforparticularapplica‐tions[15].
Severalauthorshaveinvestigatedalgorithms appliedtothecontrolofaline‐followingrobot. Kaderetal.havetriedtoexplaintheapplicationof aPIDcontrolalgorithmtocorrectthecurrenterror betweentherobotpositionandthetracedlineby calculatingacontrolsignalthatrecti iestherobot trajectoryinrealtime[16].Ontheotherhand,Nikolov etal.highlighttheneedtoapplyahistogram iltering oftheMarkovprocesseffectivelytothevelocityand lengthmeasurements,thusmitigatingthecurrent positionerror[17].Inadifferentstudy,Wuetal. highlighttheimplementationofanewfuzzysliding modecontrollerandbacktrackingalgorithmfor trajectorytracking.
Thisbacktrackingcontroltechniqueeliminates posedeviationsoftherobotbasedonitsmathemat‐icalmodel[18].Recently,anintelligenttechniquefor robotspeedcontrolusingacombinationoffuzzylogic andsupervisedmachinelearninghasbeenproposed usingnumericalsimulations[19].However,thereis littleprogressinthediscussionofintelligentcontrol tools.Therefore,aninvestigationfocusedonusing arti icialintelligencealgorithmsforthecontroland performanceofaline‐followingrobotisrelevant.
Thisstudyaimstoevaluateandvalidatethedesign andimplementationofaline‐followingrobotbasedon neuralnetworkstocontrolthetorqueonthewheels andregulatethemovements.Theline‐followingrobot isanautonomousguidedvehicle(AVG)thatfollowsa trajectorydeterminedbyablackorwhiteline.Using asetofanalogre lectancesensorsincorporatedinthe competitionrobot,itcandetectthelineonthetrack, andbasedonthevaluesacquiredbythecontroller, theneuralnetworkincorporatedintheprogramming willinterpretthesesignalsandsetthebestspeed parameterstofollowthetrajectoryinastraightlineor thecurves,inordertoguaranteethebestperformance oftherobotonthetrack.
Thearticleisorganizedintosixsections:Section1 Introduction,Section2Line‐followingrobotarchitec‐ture,Section 3 Implementationoftheneuralcontrol network,Section 4 Tests,Section 5 Results,andSec‐tion6Conclusions.
Inthissection,wedescribethearchitectureofthe line‐followingrobotthatconsistsofseveralessen‐tialcomponentswhichworktogethertoachieveits functionality.Figure 1 providesinformationonthe criticalelementsoftheline‐followingrobotstructure. Theseelementsaresystematicandcomplementeach other;inthis igure,itcanbeseenthatitconsistsof sevenblocksthatcanvaryaccordingtotheirapplica‐tion[20].
The irstelementfocusesontheenvironmentin whichitisimmersed.Then,thereisthesecondele‐mentthatincorporatesthephysicalcomponentsin chargeofcapturingthesignalsofthevariables.These signalsarethendirectedtothethirdelement,which consistsofacontrolboardinchargeofinterpreting themandissuingcorrespondingactions.
Itthenmovesontothefourthelement,repre‐sentingthepointofcontrolinteractioninsynchro‐nizationwiththemotorsinthe ifthelement.The sixthelementcoversthegeneralpowersupplyofthe robot.Finally,theseventhelementencompassesthe mechanicalstructurethatholdsalltheelectricaland mechanicalcomponentsoftherobot[21].
Thefollowingpartswereusedintheconstruction oftherobot:2wheels,2DCmotors,abasestructure,a controlboardconsistingofamicrocontroller,amotor controlcircuit,alinefollowermodule,aBluetooth connectionmodule,andapowersupplycircuit.The locomotionusedforitsconstructionisofdifferential type.Forthisreason,itisessentialtoconsiderparticu‐laritiessuchastherobot’schassis,thesensors’dimen‐sioningconcerningthechassis,andthedimensioning ofthemotor‐res[22].
Thechassisisthephysicalstructurethatsup‐portsalltheelementsoftherobot.Foritsdesign, theimplementationofaprintedcircuitboard(PCB) isconsidered,wheretheelectricalschematicthat showsallthecomponentsandtheirconnectionswith eachotherisintegrated.Theschematicincludesthe sensors,motorcontroller,microcontroller,communi‐cation,andpowersupply.Thestructure’sdesignis visualizedinFigure 2,wheretheprimaryconsider‐ationofthechassisdesignistheneedforastruc‐turethatensuresasolid,functionalbasethatcan accommodateallcomponents,ensuringsmoothand precisemovementsalongtheroute.Itisessentialto considertheweightofthechassistoavoidexcessive energyconsumptionand lexibilitytoimproverobot performanceondifferentsurfacesandmaintaintrac‐tion[23].
Microcontrollersareveryimportantinconstruct‐ingtheline‐followingrobotbecausetheyhelpmoni‐tor,control,andtakeactionwiththedataobtained. Thesedevicesintegrateasinglechip’scentralprocess‐ingunit,memory,andperipherals.Themostcommon controllersareArduino,RaspberryPi,andPIC.Thanks tomicrocontrollers,robotscanperformvarioustasks, fromcontrollingbasicmovementstoexecutingmore complexfunctionsinchangingenvironments[24].
Inthisstudy,theArduinoMega2560microcon‐trollerformsthebasisofthelinefollowerrobot,which receivesthesignalsfromeachconnectedcomponent andconsequentlyprovidesthedesiredoutput.Itisthe brainoftheentiresystemandiscodedasrequired. Themicrocontrollerinterpretsthedatareceivedby thesensorsandgeneratescontrolcommandsthat drivetherobot’smotors.Thesecommandsallow adjustingthespeedanddirectionofthemovementto keeptherobotfollowingthelineprecisely;Figure 3 showstheconnectionoftheelementsconnectedto themicrocontroller[25].Figure3alsoshowsthecom‐ponentsoftherobot:QTR‐8Aanalogre lectancesen‐sor(A),theQTR‐1RCRe lectanceSensor(B),Arduino Mega2560(C),Battery(D),andWheels(E).
Thelanedetectionsystemofaline‐followingrobot iscriticaltoitscorrectoperation.Thissystemisbased onopticalsensors,suchasphototransistorsorre lec‐tionsensors,whichcandetectthedifferencebetween re lectivitybetweenthelineandthebackground[26]. Inthisresearch,theQTR‐8Aanalogre lectancesensor wasimplementedforthetrackdetectionsystem.This electronicdevicehaseightinfraredsensors,i.e.apho‐totransistorLEDmounted9.5mmfromeachother, allowingamoreextendedrangewhendetectingthe tracedroute.Theselectedsensorbelongstothetype ofexteroceptivesensorthatdetectschangesinthe robot’sexternalenvironment.IthasanLEDthatemits radiationintheinfraredspectrum,whichhitsthe groundandcausesare lection,whichiscapturedby thephototransistor;theamountofre lectiondepends onthecoloroftheground[27].Todeterminetheline’s positionandgeneratecontrolcommands,astructure wasfabricated,asshowninFigure4.withtheanalog re lectancesensorQTR‐8A(A),whichallowstherobot tofollowthelinepreciselyandcontinuously.
Thissectionmentionsthedimensioningofthe enginesandtheirelectroniccontrolsystem.The motorsareresponsibleforthecorrectdisplacement oftherobotonthetrack,soitisessentialtocarryout correctdimensioning,takingintoaccountthemotor torquethattherobotneedsforeachwheel.
Thebehaviorofthetorqueaboutthewheelsis directlyproportional,i.e.ahightorqueisneededwhen theradiusofthewheelsislarge,thusreducingtherev‐olutionsofthewheeland,therefore,thespeedofthe robot;ontheotherhand,ifthemotortorqueissmall andtheradiusofthewheelsissmall,therevolutions ofthewheelwouldbefaster.Consequently,thespeed oftherobotwouldincrease,whichinourcase,isideal forlookingforasmalltorque[28].Equation(1)can beusedtocalculatetherequiredmotortorque;the accelerationtobeachievedisamatterofjudgment.
��=��⋅(��+��⋅sin(��))⋅�� (1)
Where:
T:Torqueofthemotor.
M:totalMassoftherobot.
a:Acceleration.
��:Angleoftheplane.
g:Gravity.
r:Radiusofthewheels
Thedatawehavearethemassoftherobotandits components,whichisequalto170grams;thismass wasobtainedbyweighingtherobotonascale.In additiontothis,wearelookingtomanageanaccel‐erationaround2m/s2,andtheradiusofthewheels tobeimplemented1(cm).Finally,theangleofthe trackiszero.FromEquation(1),itisobtainedthat therequiredtorqueisequivalentto0.0042Nm.To controlthemotorsthatallowthemovementofthe linefollowerrobotinamoreprecise,moreef icient wayinthedirectionandspeedofthemotors.Finally, acontrollerwasselectedTB6612FNGbecauseofits dimensionsanditsapplicationsinsimilarstudies[29] werechosenforthiscasestudy.
Thecontrolleroftheline‐followingrobotisafun‐damentalpartofitscorrectoperation;forthisreason, amultilayerneuralnetworkisusedtoimprovethe accuracyofdecision‐making.
Theneuralnetworkpredictsthepositionofthe robotontherouteandprovidesacontrolsignal thatallowstherobottocontrolthemovementofthe motors[30].Thestructureoftheimplementedneural networkisvisualizedinFigure 5.Thisnetworkwas constructedusingmultiplehiddenlayers.Theinput layerconsistsofoneneuron,ahiddenlayerofsix neurons,andanoutputlayeroftwoneurons.Each layeroftheneuralnetworkreceivesavalueofthe lossfunctioninthecurrentstate,andtheconnection weightofeachneuronisadjustedaccordingly.
3.1.ImplementationoftheNeuralNetworkController
Thissectiondescribesinputdatacollectiontothe neuralnetworkandtheoutputdata.Figure6.shows howtheinputdata�� areobtainedusingthesensors ineachpositionwheretherobotcanbeonthelineso thattheneuralnetworkcanrespondcorrectlytoany situationandmakedecisionstomaintainthetrajec‐torythatdescribestheroute.Thedataobtainedfrom theQTR‐8Aanalogre lectancesensorsarefedtothe inputlayerofthemultilayerneuralnetwork.
Theoutputdata��correspondstothePWMvalue requiredinthemotors,whichdependsontheposition oftherobotonthelineandtendstochangeconstantly.
Someinputdataandoutputdataareshownin(2).
Theextractionofweightsandbiasesarefunda‐mentalcomponentsintheneuralnetwork.These valuesallowthebehaviortobeadjustedandrepresent morecomplexnon‐linearfunctions.
3.2.ActivationFunction
Theactivationfunctionmakesthenon‐linearrela‐tionshipbetweentheinputandtheoutputmore effective.Differentactivationfunctionscanbeused forthedifferentneuralnetworklayers[31];themost commonlyusedoptionsareshowninFigure 7.Rec‐ti iedLinearUnit(ReLU)neuronsareusedforthe hiddenlayers;inmachinelearning,ReLUandLinear arethemostpopularactivationfunctions,expressed inFigure7.Inourstudy,theReLUactivationfunction passestheinformationfromtheinputlayertothe hiddenlayer,withthepeculiaritythatthenegative valuesarecanceled,lettingthepositivevaluespass withoutmodifyingorcancelingthem.Thelinearacti‐vationfunctionisusedattheneuralnetwork’soutput; thishasthecharacteristicoflettingthevaluesithasat itsinputpassthroughwithoutmodifyingthem.
3.3.ModelEvaluation
Thelearningoftheneuralnetworkwasaccom‐plishedoff‐linebyscanningdataintheworkenviron‐mentbyKeraslibraryinPython.Thefulldatasetwas usedtotraintheproposedneuralnetwork,andthe performanceofthenetworkwasdeterminedforthat samedataset.Theevaluationoftheimplementedpre‐dictionmodelwasperformedbychangingthetraining parametersandepochsinordertoachievesatisfac‐toryresults.Thehyperparametersusedinthismodel canbevisualizedinTable1.Thelossfunctionisamea‐surethatevaluateshowwellthemodelmakespredic‐tionsbasedonthepredictedoutputsandtheactual outputs.Ourstudyusedthemeanabsoluteerrorloss functionandobtainedalowerrorbutwithmanyinter‐actionsorepochs.TheSDG(StochasticDownward Gradient)optimizerwasusedtoreducetheerrorand thenumberofinteractionsorepochs.
Additionally,ameanabsoluteerrorregression metricwasused,whichdidnotminimizetheerrorbut servedtoevaluatethetrainingresults.Theoptimal networkstructureiscreatedbycomparingtheloss functionvaluesoverthegeneratedsamples.
Oncetheassemblyoftherobothasbeencom‐pletedanditisworkingcorrectly,thesoftwareand hardwarepartoftherobotistested.Oncetheassem‐blyoftherobothasbeencompletedandthesoftware andhardwareareworkingcorrectly,thenecessary testsarecarriedouttoevaluateitsoperation,for whichthetrackusedintheSUCREBOT2022robotics competition,whichcanbeseeninFigure8,istakenas areference.
Thisitemalsoexplainsthemodi icationsand improvementsmadetosolveerrorswhenthelinefol‐lowerrobotwasontrack.Thisistheresultofdifferent participationinroboticscompetitions.
The irsttestscarriedoutontherobotwerethe useofaprintedcircuitboardofdrillingtechnology, withathicknessof1mm,whichincreasedtheweight oftherobot.Atthisstage,the inalprototypewasalso modi iedusingsurfacemounttechnologywithathick‐nessof0.8mm.Thefollowingtestswerecarriedout abouttheadherenceoftheline‐followingrobotonthe track.Therefore,thewidthofthewheelswaschanged from2cmand3.7cm,respectively.Finally,another essentialpointtoobservethecorrectoperationof therobotonthetrackwastoconsiderthedistance thesensorsshouldhaveconcerningthechassis,for
whichtestswerecarriedoutwithdifferentdistances ofthesensorsrangingfrom6.8cmto10.5cm.Figure9 showsthe inalprototypeoftheline‐followingrobot.
5.ResultsandDiscussions
5.1.ModelTraining
Duringtheneuralnetworktraining,thebestper‐formanceachievedwasatepoch2500withanabso‐lutemeansquareerrorof2.2355.Thetrainingperfor‐manceplotisshowninFigure10
Theoptimizedweightandbiasmatrixesobtained duringthetrainingprocessareshownin(3).
0,
w
w
��
−0,0842,701] (3c)
���� =[2,7562,757] (3d)
where ������ istheweightvectorfortheweightsfrom theinputtothehiddenlayer,������ istheweightvector fortheweightsfromthehiddenlayertotheoutput layer,���� isthebiasvectorfromtheinputtothehidden layer,and���� isthevectorfrominputbiastopaoutput layer.
InthegraphofFigure11,itisevidentthatthereal outputisclosetotheestimatedone,whichisenough toconsiderthatthemodelworks.Aftertrainingand testingtheneuralnetwork,theobtainedweightsand biasparametersareimplementedfortorqueand motioncontrolintheArduinoIDEinC++.
Table4. Timeresultswithdifferentopticalsensor distancesfromthechassis
Fourtestswereperformedduringeachcon igura‐tionoftheprototyperobot,andtheaveragemeasured valuewastaken.FromthedatainTable 2,theline‐followingrobotshowedhighspeedandmaneuverabil‐ityonthelanebyusingsurfacemounttechnologyin thechassisduetoitslowweight.
Table 3 showsthattheline‐followingrobothas excellenttrackstabilitywhenthetirewidthincreases. Thismakesitasuitableoptionwhenthereareirregu‐laritiesinthelane.
Thedifferencebetweenthedistancesofthesen‐sorsfromthechassisishighlightedinTable 4.By equippingthesensorsatadistanceof6.8cm,the line‐followingrobotshowedaccurateabilitiesinscan‐ningthetrackwhileavoidingsigni icantdeviationsor irregularities.
Table 5 showstheresultsobtainedbetweenthe twocontrollersbyperformingfourtracktestswiththe bestfunctioningprototypeinthemodi ications.
Table5. TimingresultswithPIDcontrollerandneural network
Thisstudyindicatesthattheline‐followingrobot hadamoreeffectiveresponsewithanarti icialneural networkcontrolalgorithmbecauseofitsabilityto learncomplexpatternsandadaptindifferentenviron‐mentscomparedtothePIDcontrolalgorithm,whichis simpleandeffectiveinpredictablesystems,butdoes notworkwellinnon‐linearsituations.Ontheother hand,thefuzzycontrolalgorithmtendstobecom‐plicatedbycon igurationandtuningandneedstobe morerobustinpredictableenvironments.Thesecond important indingwasthat,withtheimplementation ofthiscontrolalgorithm,thereactiontimeandcorrec‐tionoftherobottoerrorsonthetrackisfaster.
Thepresent indingsalsosupportthestudiesof Farkhetal.[22],whoconcludethatneuralnetworks arewellsuitedformobilerobotsbecausetheycan operatewithimpreciseinformation,i.e.differentenvi‐ronments.Whenprocessingsignalsfromsensors, theneuralnetwork‐basedcontrolalgorithmresponds fastertotakeaction.
Theresultsofthepresentstudyalsosuggeststhe useofarti icialneuralnetworkstoimproveperfor‐mance,bothinaccuracyandabilitytoadapttovar‐ioussituations.Thelearningcapabilityoftheneural networkenabledtherobottofacereal‐timechallenges andeffectivelyfollowcomplicatedroutes.
ResultsofKaderandotherauthorsintheir research[16]proposeaPIDcontrolalgorithmthat alsoallowsasmoothandstableresponseoftherobot asitfollowstheline,butwithalatercorrectiontime comparedtotheresultsobtainedwithaneuralnet‐work,consideringthatthistimeisindispensablein roboticcompetitions.
Oncarryingouttestsontheline‐followingrobot betweenthePIDcontrollerandtheneuralnetwork,it wasestablishedthatthetimestakenbytherobotto travelalongthetrackarealmostthesameforthetwo controllers;thevariationbetweenthetwoisinmil‐liseconds.However,thePIDcontrollerpresentsspe‐ci icerrorswhentherobottravelsalongthetrackin astraightline;atthatmoment,itshowsveryconstant
zigzaggingmovements.Inthesameway,inthecurves, therobotmakesabruptmovements,unliketheneural networksthatmakethemsmoother,whichcausesthe movementsonthetracktotakelesstime.
Aspartofthemechanicaldesign,itwasfound thatthechassisofthelinefollowerrobotplaysa vitalrolesincethisiswherealltheelectronicand mechanicalelementsarelocated.Thispartwascar‐riedoutconsideringthesmallestpossiblesizebecause inthedifferentroboticscompetitions,therearelim‐itationsregardingthisparameter,anditissought thattherobotcanpassthehomologationwithout anyproblem.Consideringthesizeanddesignofthe linefollowerrobotinthisstudy,theidealweightis 170grams,whichguaranteestherobot’sgoodperfor‐manceandstabilityonthetrack.Inaddition,itallowed forgreateragilityandresponsivenesswhenchanging directiononobstacles.
Theinfraredsensorsthatmakeuptheline‐followingrobot,bothlateralandfrontal,arefunda‐mental,astheywillberesponsibleforkeepingthe robotontrack.Forthisreason,thedistanceofthe sensorsconcerningtherobotchassiswilldependon theradiusofthecurvesonthedifferenttracks;in ourstudy,theidealdistanceis6.8cm,representing amorepreciseandfasterperformanceindetecting theroute.Ifanincorrectlocationofthesesensors, nomatterhowwellthemechanical,electronic,and programmingpartsareworking,therobotwillnotbe abletoful illitsfunctionproperly.
Theneuralnetworkwasimplementedasamulti‐layerperceptronmodelwithlinearregression,which provedthatitcouldworksuccessfullyonaline‐followingrobot,takingintoaccountthatitisnotnec‐essarytoaddmorethanonehiddenlayerintheneural network,dependingonthecomplexityoftheproblem andtheamountoftrainingdataavailable.Thehidden layerusedtheReLUactivationfunctiontointroduce non‐linearitiesintothenetworkandallowedthecap‐tureofcomplexrelationshipsbetweenthesensorsand theoutput.
CesarMinaya∗ –DepartmentofElectronics,Instituto TecnológicoSuperiorRumiñahui,Sangolquí,171103, Ecuador,e‐mail:cesar.minaya@ister.edu.ec.
RicardoRosero –DepartmentofElectronics, InstitutoTecnológicoSuperiorRumiñahui,Sangolquí, 171103,Ecuador,e‐mail:ricardo.rosero@ister.edu.ec.
MarceloZambrano –Departmentof Electronics,InstitutoTecnológicoSuperior Rumiñahui,Sangolquí,171103,Ecuador,e‐mail: marcelo.zambrano@ister.edu.ec.
PabloCatota –DepartmentofElectronics,Instituto TecnológicoSuperiorRumiñahui,Sangolquí,171103, Ecuador,e‐mail:pablo.catota@ister.edu.ec.
∗Correspondingauthor
[1] O.Gumus,M.Topaloglu,andD.Ozcelik,“TheUse ofComputerControlledLineFollowerRobots inPublicTransport,” ProcediaComput.Sci., vol.102,no.August,2016,pp.202–208,doi: 10.1016/j.procs.2016.09.390.
[2] A.Latif,H.A.Widodo,R.Rahim,andK.Kunal, “ImplementationofLineFollowerRobot BasedMicrocontrolleratmega32a,” J.robot. Control,vol.1,no.3,2020,pp.70–74,doi: 10.18196/jrc.1316.
[3] M.Antony,M.Parameswaran,N.Mathew, V.S.Sajithkumar,J.Joseph,andC.M.Jacob, “DesignAndImplementationOfAutomatic GuidedVehicleForHospitalApplication,” Proc. 5thInt.Conf.Commun.Electron.Syst.ICCES 2020,no.Icces,2020,pp.1031–1036,doi: 10.1109/ICCES48766.2020.09137867.
[4] O.F.GómezandU.E.Gómez,“KinematicSimula‐tionOfALineFollowerRobotForTheCreation OfTheProgrammingVideogameRustyRoads InTheUnityFramework,” Inf.Tecnol.,vol.28, no.5,2017,pp.55–64,doi:10.4067/s0718‐07642017000500008.
[5] A.AharariandY.Ueda,“LowPassFilterApplied toColorSensorofLineFollowerRobot,” Procedia ComputerScience,vol.154,2018,pp.693–698, doi:10.1016/j.procs.2019.06.108.
[6] V.G.R.Caitite,D.M.G.DosSantos,I.C.Gregorio, W.B.DaSilva,andV.F.Mendes,“DiffusionOf RoboticsThroughLineFollowerRobots,” Proc.–15thLat.Am.robot.Symp.6thBrazilianrobot. Symp.9thWork.robot.Educ.LARS/SBR/WRE 2018,2018,pp.604–609,doi:10.1109/LARS/S BR/WRE.2018.00109.
[7] H.A.Calderón,C.Mejía,andL.Cobo,“Imple‐mentaciónDeUnRobotMóvilSeguidorDeLínea YDetectorDeObstáculosConComunicación Bluetooth,” ReviewOntare,vol.4,no.2,2017, pp.99–118,doi:10.21158/23823399.v4.n2.20 16.1639.
[8] S.Kokare,“UsingZigBee,” 2018FourthInt.Conf. Comput.Commun.ControlAutom.,2018,pp.1–5.
[9] J.Chaudhari,A.Desai,andS.Gavarskar,“Line followingRobotUsingArduinoForHospitals,” 20192ndInt.Conf.Intell.Commun.Comput.Tech. ICCT2019,2019,pp.330–332,doi:10.1109/IC CT46177.2019.8969022.
[10] W.K.BornandC.J.Lowrance,“Applicationof ConvolutionalNeuralNetworkImageClassi ica‐tionforaPath‐FollowingRobot,” 2018IEEEMIT Undergrad.Res.Technol.Conf.URTC2018,2018, pp.11–14,doi:10.1109/URTC45901.2018.924 4781.
[11] C.F.Hsu,C.T.Su,W.F.Kao,andB.K.Lee,“Vision‐BasedLine‐FollowingControlofaTwo‐Wheel Self‐BalancingRobot,” Proc.-Int.Conf.Mach. Learn.Cybern.,vol.1,2018,pp.319–324,doi: 10.1109/ICMLC.2018.8526952.
[12] J.Sarwade,S.Shetty,A.Bhavsar,M.Mergu,andA. Talekar,“LineFollowingRobotUsingImagePro‐cessing,” Proc.3rdInt.Conf.Comput.Methodol. Commun.ICCMC2019,no.Iccmc,2019,pp.1174–1179,doi:10.1109/ICCMC.2019.8819826.
[13] R.Javanmard,A.H.Zabbah,M.Karimi,andK. Jeddisaravi,“LineFollowingAutonomousDriv‐ingRobotUsingDeepLearning,” 6thIran.Conf. SignalProcess.Intell.Syst.ICSPIS2020,2020,doi: 10.1109/ICSPIS51611.2020.9349547.
[14] A.Moulay,F.Laou i,T.Benslimane,andO. Abdelkhalek,“FPGA‐BasedCar‐LikeRobotPath FollowerwithObstacleAvoidance,” Proc.2020 Int.Conf.Math.Inf.Technol.ICMIT2020,2020, pp.125–131,doi:10.1109/ICMIT47780.2020. 9047008.
[15] A.Ghorbel,N.BenAmor,andM.Jallouli,“Design OfAFlexibleRecon igurableMobileRobot LocalizationSystemUsingFPGATechnology,” SNAppliedScience,vol.2,no.7,2020,doi: 10.1007/s42452‐020‐2960‐4.
[16] M.A.Kader,M.Z.Islam,J.AlRa i,M.R.Islam, andF.S.Hossain,“LineFollowingAutonomous Of iceAssistantRobotwithPIDAlgorithm,” 2018Int.Conf.Innov.Sci.Eng.Technol.ICISET 2018,no.October,2018,pp.109–114,doi: 10.1109/ICISET.2018.8745606.
[17] D.Nikolov,G.Za irov,I.Stefanov,K.Nikov,andS. Stefanova,“AutonomousNavigationAndSpeed ControlForLineFollowingRobot,” 2018IEEE 27thInt.Sci.Conf.Electron.2018-Proc.,2018, pp.1–4,doi:10.1109/ET.2018.8549580.
[18] X.Wu,P.Jin,T.Zou,Z.Qi,H.Xiao,andP. Lou,“BacksteppingTrajectoryTracking BasedonFuzzySlidingModeControlfor DifferentialMobileRobots,” JournalofIntelligent Robotics,vol.96,no.1,2019,pp.109–121,doi: 10.1007/s10846‐019‐00980‐9.
[19] M.S.GharajehandH.B.Jond,“SpeedCon‐trolForLeader‐FollowerRobotFormationUsing FuzzySystemAndSupervisedMachineLearn‐ing,” Sensors,vol.21,no.10,2021,pp.1–14,doi: 10.3390/s21103433.
[20] S.Tayal,H.P.G.Rao,S.Bhardwaj,andH.Aggar‐wal,“LineFollowerRobot:DesignandHardware Application,” ICRITO2020-IEEE8thInt.Conf. Reliab.InfocomTechnol.Optim.(TrendsFutur. Dir.),2020,pp.10–13,doi:10.1109/ICRITO48 877.2020.9197968.
[21] I.P.Latini,W.E.Barioni,M.Teixeira,F.Neves‐Jr., andL.V.R.deArruda,“Comparisonbetween Line‐FollowersandFreeMovementRobotsin TasksExecutioninaSimulatedEnvironment,” in 2022LatinAmericanRoboticsSymposium (LARS),2022BrazilianSymposiumonRobotics (SBR),and2022WorkshoponRoboticsin Education(WRE),2022,pp.145–150.doi: 10.1109/LARS/SBR/WRE56824.2022.9995776.
[22] R.Farkh,K.AlJaloud,S.Alhuwaimel,M.T. Quasim,andM.Ksouri,“ADeepLearning ApproachForTheMobile‐RobotMotion ControlSystem,” IntelligentAutomation&Soft Computing,vol.29,no.2,2021,pp.423–435, doi:10.32604/iasc.2021.016219.
[23] A.RoyandM.M.Noel,“DesignOfAHigh‐SpeedLineFollowingRobotThatSmoothlyFol‐lowsTightCurves,” ComputerandElectrical Engineering,vol.56,2016,pp.732–747,doi: 0.1016/j.compeleceng.2015.06.014.
[24] B.G.Fernándezetal.,“Roboticsvs.Game‐Console‐BasedPlatformstoLearnComputer Architecture,” IEEEAccess,vol.8,2020, pp.95153–95169,doi:10.1109/ACCESS.2020. 2994196.
[25] M.H.Nushra,Q.A.Rahman,S.M.F.Mursalin,N.B. Asad,M.M.AsifSyeed,andM.M.Islam,“Smart CarParkingWithTheAssistanceOfLineFol‐lowingRobot,” 2019Int.Conf.Sustain.Technol. Ind.4.0,STI2019,vol.0,2019,pp.24–25,doi: 10.1109/STI47673.2019.9068046.
[26] J.W.Lok,W.M.W.Muda,andA.N.Woro,“Devel‐opmentOfWarehouseRobotWithAdvanced LineFollowingAndBackgroundColorSensors,” JournalofAdvancedManufacturingTechnology, vol.15,no.2,2021,pp.23–34.
[27] H.Murcia,J.D.Valenciano,andY.Tapiero,“Devel‐opmentofaLine‐FollowerRobotforRobotic CompetitionPurposes,” AppliedComputerSciencesinEngineering,2018,pp.464–474.
[28] L.ScrewandD.Loads,“MotorTorqueCalcula‐tion,” Wire,no.86,pp.1–5.
[29] B.Zeng,J.Zhang,L.Chen,andY.Wang,“Self‐balancingcarbasedonARDUINOUNOR3,” 2018IEEE3rdAdvancedInformationTechnology,ElectronicandAutomationControlConference(IAEAC),2018,pp.1939–1943.doi: 10.1109/IAEAC.2018.8577775.
[30] R.Chai,H.Niu,J.Carrasco,F.Arvin,H.Yin, andB.Lennox,“DesignandExperimental ValidationofDeepReinforcementLearning‐BasedFastTrajectoryPlanningandControlfor MobileRobotinUnknownEnvironment,” IEEE Trans.NeuralNetworksLearn.Syst.,2022,doi: 10.1109/TNNLS.2022.3209154.
[31] T.Guillod,P.Papamanolis,andJ.W.Kolar,“Arti i‐cialNeuralNetwork(ANN)BasedFastandAccu‐rateInductorModelingandDesign,” IEEEOpen J.PowerElectron.,vol.1,2020,pp.284–299,doi: 0.1109/OJPEL.2020.3012777.
Abstract:
Submitted:26th May2023;accepted:22nd October2023
MarthaFlores‑Sosa,ErnestoLeon‑Castro,JoseM.Merigo
DOI:10.14313/JAMRIS/1‐2024/5
Thestockmarketisofgreatimportanceforthefinan‐cialdevelopmentofacountryduetothelargevol‐umeoftransactionstherein.Analyzingthecorrelation betweenindicesintheworldhelpsusfigureoutwhich variablesaremostimpactful.Thispaperproposesthe useoforderedweightedaverage(OWA)operatorsin combinationwiththePearsoncoefficienttocreatea measureofcorrelationthatcananalyzeawiderangeof possiblescenariosthatgofromminimumtomaximum. Thenewframeworkscanaddadditionalinformationto theprocessofcorrelation.Theworkpresentsanapplica‐tionintenofthelargeststockexchangesintheworld. Theresultssuggestabroadpositivecorrelationthatis reinforcedintimesofinstability.
Keywords: Stockmarket,OWAoperator,Pearsoncoeffi‐cient,Financialdevelopment
1.Introduction
Theworld inancialmarketisessentialinthe developmentofeconomicprocessessinceitcon‐tributestothetransferof inancial lowsbetween agents.Thestockmarketestablishesacloseconnec‐tionwiththeproductivesectortotheextentthateach countryhasdevelopedits inancialsystem[1,2].Inthe lastdecades,therehasbeenaconsiderableincrease inthenumberoftransactionsandtheirvaluesin stockmarkets.Therefore,manyofitsaspectshave beeninvestigatedtosearchforknowledgeandclarify thephenomenainthemarkets.Inthissense,issues suchasvariablesthataffectit[3,4],modeling[5,6], forecasting[7,8],andintegrations[9,10]havebeen studied.
Marketintegrationhasallowedmanyofthestock marketstomoveinsynchronywhenfortuitousevents occur,andsomeindicestendtoaffectotherstoagreat extent.Baruniketal.[11]showthatintimesofinsta‐bility,thecorrelationofthestockmarketwithother indicators,suchasgoldandoil,becomesstronger. JungandChang[12]foundthatstockstendtocluster byPearsoncorrelationandpartialcorrelation.Intend‐ingtoknowtherelationshipofworldstockmarkets overtime,Wangetal.[13]proposeanetwork‐based Pearsoncoef icienttoanalyzesomestockexchanges.
ThisworkproposesaPearsoncoef icientwith OWAaggregationoperatorstoanalyzeworldstock markets.TheOWAoperators[14]areaparameterized familyofaggregationoperators,whosemaincharac‐teristicisthereorderingoftheattributesthatallowan analysisofmultiplescenariosthatgofromminimum tomaximum.Oneofthemostpopularextensionsisthe inducedoperatorIOWA[15].Itusesamorecomplex reorderusinginducedvariables.Forthetreatment ofuncertaindata,operatorswithadditionalvectors havebeenproposed.ThePOWAoperator[16]consid‐ersprobability,andtheorderedweightedaveraging‐weightedaverage(OWAWA)[17]operatorusesan extraweighting.Notethatalltheseideascanbeuni‐iedinasingleoperatorcalledIPOWAWA[18].Since itsinception,theOWAoperatoranditsextensions havebeenusedsuccessfullyinstatisticalprocedures suchasregressionsissue[19, 20],standarddevia‐tion[21],variance,andcovariance[22,23].
ThispaperusestheIPOWA,IOWAWA,and IPOWAWAoperatorsintheformofvariancesand covariancestocalculatethePearsoncorrelation coef icient.ThenewmethodologyiscalledPC‐IPOWA, PC‐IOWAWAandPC‐IPOWAWA.Themainobjective istoobtainacorrelationcoef icientthat,inaddition toconsideringscenariosthatgofromminimumto maximum,canconsiderprobabilitiesandweights whenenvironmentsofuncertaintyexist.Inorder to indimportantinformationin inancialmarkets, weanalyzethecorrelationofsomeofthemost representativestockexchangesintheworld.
Thepaperisdevelopedasfollows:Section 2 presentsasummaryofthemethodologiesused.Sec‐tion 3 showsthenewproposedPearsoncoef icient andOWAoperators.InSection 4,ageneralizationof thenewstructureispresented.Section5developsthe applicationoftheOWAcorrelationcoef icientsinthe stockmarket.Finally,theconclusionsoftheworkare describedinSection6.
Belowisabriefdescriptionoftheapproaches usedintheproposalofthiswork.TheOWAoperator, someofitsextensions,andthePearsoncoef icientare de ined.
Theorderedweightedaveraging(OWA)opera‐tor[14]providesamethodtoaggregateseveralargu‐mentsthatdiebetweenthemaximumandmini‐mum.Themaincharacteristicisthereorderingofthe attributevectorthatgoesfromminimumtomaximum (AOWA)orfrommaximumtominimum(DOWA).The OWAoperatorisde inedasfollows:
De inition1. AnOWAoperatorwithdimensions n isamodel������∶���� →�� suchthatithasassociated weightsvector�� thus���� =∈[0,1]and∑�� ��=1 ���� =1, then:
������(��1,��2,…,����)= �� ��=1 ��������, (1) where���� isthe��thlargest����.TheOWAoperatorisa meanoperatorasitsatis iestheconditions:
‐ Monotonicity:if ���� ≥̂���� then ��(��1,…,����)≥ ��(̂��1,…,̂����)for��.
‐ Commutativity:Theinitialindexingofdearguments doesn’tmatter.
‐ Idempotent:if���� =��forall j,so��(����,…,����)=��. IfthereorderingoftheOWAargumentsisnotcon‐sidered,thenwecanuseinducedvariablesforit.The inducedweightedaverageoperator(IOWA)[15]uses argumentpairscalledOWApairs,withtheobjective ofinducinganorderingandaggregationofthesecond components.Itcanbede inedasfollows:
De inition2. AnIOWAoperatorisamapping ��������∶���� →�� ofdimensionnwithanassociated weightsvector ��=[��1,��2,…,] wnT,suchthat 0≤���� ≤1 and ���� +⋯+���� =1,withan inducedIOWApair ⟨����,����⟩,where ���� isthevariable thatinducedorderand���� istheargumentofthevari‐able,theformulaisasfollows:
IOWA(⟨��1,��1⟩⟨��2,��2⟩,…,⟨����,����⟩)= �� ��=1 ��������, (2) where ���� isthevalue ���� intheIOWApairthathave the ��thmostextensive ����.TheIOWAoperatorsatis‐iestheconditions:Monotonicity,Commutativityand Idempotent.
Inpractice,probabilitycanbeofgreatimportance toknowthecharacteristicsofacurrentphenomenon. Merigó[16]proposestheprobabilisticOWA(POWA) operator,whichprovidesauni icationoftheprobabil‐itiesandtheOWAoperators.Itconsidersthedegreeof importanceofeachoneintheaggregationprocess.
Then: De inition3.APOWAoperatorisamapping POWA∶���� →�� associatedwithaweightvector �� whereitscomponentslieintheunitintervalandsum toone.Additionally,ithasanassociatedprobability vector �� with ∑�� ��=1 ���� =1 and ���� ∈[0,1],according tothefollowingequation:
��������(��1,��2,…,����)= �� ��=1 ��������, (3) where���� isthe jthlargestin��1,��2,…,����.Thereissuch arelationshipbetweenprobabilitiesandweightsas �� =������ +(1−��)���� with��∈[0,1].If��=0,the PAoperatorappears,andif��=1,theOWAoperator isobtained.
Insomecases,theimportantinformationin decision‐makingisgivenbyothertypesofweightings thatcancapturedifferentphenomena.TheOWAWA operatorwasproposedbyMerigó[17],anditusesthe OWAoperatorandweightedaverage(WA)inthesame formulation.Thede initionisasfollows:
De inition4. AnOWAWAoperatorofdimension n isamodel����������∶���� →��associatedwithaweight vector ��=[��1,��2,…,����]�� suchthat 0≤���� ≤ 1 and ∑�� ��=1 ���� =1.Additionally,ithasanassociated weightvector �� with ∑�� ��=1 ���� =1 and ���� ∈[0,1],so that:
����������(��1,��2,…,����)= �� ��=1 ��������, (4)
where ���� isthe jthlargest ����.Theweightvectoris composedas ���� =������ +(1−��)����.TheOWAWA operatorhassimilarpropertiestotheOWAoperator.
ThePOWAoperatorandOWAWAoperatorcanalso useadifferentreorderofarguments.TheIPOWAoper‐ator[24]andIOWAWAoperator[25]considerinduced variablesforthereorderprocess.Theformulasareas follows:
����������(⟨��1,��1⟩⟨��2,��2⟩,…,⟨����,����⟩)= �� ��=1 ��������, (5)
where ���� isthe ��thlargestvalueofthe ����.Thereisa weightvector��suchthat���� =∈[0,1];����+⋯+���� = 1,andaprobabilityvector �� with ∑�� ��=1 ���� =1;���� ∈ [0,1],thedegreeofimportanceis �� =������+(1−��)����.
������������(⟨��1,��1⟩⟨��2,��2⟩,…,⟨����,����⟩)= �� ��=1 ��������, (6) where���� isthevalue���� intheIOWAwiththe��thlargest ����.Theweightvectorconsiderstwovectors �� such that ���� =∈[0,1]; ���� +⋯+���� =1,and �� where ∑�� ��=1 ���� =1;���� ∈[0,1],thedegreeofimportanceis ���� =������ +(1−��)����
Itispossibletoputtogetheralltheideasseen aboveinoneformulation.TheIPOWAWAopera‐tor[18]uni iestheIOWA,theweightedaverage(WA)
andtheprobabilisticaggregation(PA)inoneformula‐tionthatcandealwithriskanduncertainty.Itcanbe de inedasfollows:
De inition5. AnIPOWAWAoperatorofdimension n isamapping ��������������∶���� →��,ifithastwo associatedweightingvectors W and V andprobability vector P,whereitscomponentslieintheunitinterval andsumtoone.
Additionally,aninducedIOWApair⟨����,����⟩iscon‐sidered,then:
��������������(⟨��1,��1⟩⟨��2,��2⟩,…,⟨����,����⟩) =��1 �� ��=1 �������� +��2 �� ��=1 �������� +��3 �� ��=1 ��������, (7)
where���� isthevalue���� withthe��thlargest����,and��1, ��2 and��3 ∈[0,1],with��1 +��2 +��3 =1.Thespecial casesappear:if��1 =1,wegettheIOWAoperator.If ��2 =1,theWAisformed.If��3 =1,thePAisobtained. If��1 =��,wecreatetheprobabilisticweightedaverage (PWA).
2.2.VariancesandCovariancesOWA
TheOWAoperatorhasamultidisciplinaryappli‐cationusingtheideaofweightingandreorderingin othermethodologies.TheOWAoperatorswithvari‐ances(Var‐OWA)[26]adaptthearithmeticvariance toavectorofparameterizedweights,accordingtothe followingequation:
De inition6. AvarianceOWAofdimension n is amodel ������∶���� →�� withanassociatedweights vector �� thus 0≤���� ≤1 and ∑�� ��=1 ���� =1,thena variancecomponent���� =(���� −��)2 isassociatedwith aweightvalue���� inthefollowingway:
������−������(��1,…,����)= �� ��=1 ��������, (8)
where���� isthelargestofthe(���� −��)2 ,�� istheOWA operatormean.Meanwhile,thecovarianceisformu‐latedusingasimilarprocedure.Merigó[27]proposed thecovariancewithOWAoperators(Cov‐OWA).So:
De inition7. AcovarianceOWAisamodel ������∶���� →�� ofdimension ��,wherethereisa weightsvector��=[��1,��2,…,����]�� thus0≤���� ≤1 and���� +⋯+���� =1,thenthevariancecomponent ���� =(���� −��)(���� −��)isassociatedwithaweight���� Theformulaisasfollows:
������−������(��,��)= �� ��=1 ��������, (9)
where���� isthe��thlargestofthe(���� −��)(���� −��),���� istheargumentvariableofthesetofelements ��, ���� istheargumentvariableoftheset��.�� and�� arethe OWAmeansofXandY,respectively.
Acommonframeworkformeasuringthelinear relationshipbetweentwovariablesisthePearson Correlation(PC)coef icient[28,29].Itcanbeanindex simpleandeasytoapplywithinterestingresultsin decision‐making.Then:
De inition8. ItisaPCcoef icientifgivenasetof variables(����,����),sothe��=1,…,��∶���� ∈���� ,���� ∈ ����,wehaveamodel ����∶���� →��.Theformulaisas follows:
����= ������(��,��) ������(��)������(��) , (10) where ������(��,��) isthecovariance (���� ��)(���� ��) Variance X is(���� ��)2 .Variance Y is(���� ��)2.The�� and��arethearithmeticmeans.
3.ProbabilisticWeightedOWAonPearson Correlation
Therelationshipoftwovariablescanincludesev‐eralaspectsthatarenotcapturedbythearithmetic Pearsoncoef icient.Probabilitymeasuresthecer‐taintywithwhichaneventcanoccur.Inthissense,a Pearsoncoef icientwithprobabilisticOWAoperators (PC‐POWA)offersacorrelationcoef icientthatcon‐nectstheprobabilityinthecalculationofthePC.The PC‐POWAcanbede inedasfollows:
Proposition1. APC‐POWAofdimension n isa model ��������∶���� →�� withtwosetsofvariables ���� ∈����,���� ∈���� thathasanassociatedweighting vector��with���� ∈[0,1]and���� +⋯+���� =1.Then:
����−��������(��1,…,����)
= ������−��������(��,��)
������−��������(��)×������−��������(��)
= ∑�� ��=1 ����(���� −��)(���� −��),
[∑�� ��=1 ����(���� −��)2][∑�� ��=1 ����(���� −��)2] , (11)
where ���� isthecalculationofvariancesandcovari‐ances jthlargest.Thecomponents���� =(���� −��)2 and ���� =(���� −��)(���� −��)invarianceandcovariancehave anassociatedweight����.ThePC‐POWAhasthesame proprietiesthatOWAoperators,thisis:
‐ Monotonic.If���� ≥̂���� then,wehave:
��(����−��������(��1,��2,…,����))
≥��(����−��������(̂��1,̂��2 …,̂����)).
‐ Symmetry.If ��=��1,��2,…,����;��′ =��′ 1��′ 2,…,��′ ��, then:
��(����−��������(��1,��2,…,����))
=��(����−��������(��′ 1,��′ 2,…,��′ ��)).
‐ Idempotent.If���� =��,forall��=1,…,��,then:
��(����−��������(��1,��2,…,����)=��.
Example1. Consideravariable(X=2,4,6)anda variable(Y=5,8,3),aweightvector(W=0.3,0.3,0.4) andaprobabilityvector(P=0.4,0.4,0.2)anda��=0.6.
��=0.34,0.34,0.32
POWAmeans:
��=(6×0.34)+(4×0.34)+(2×0.32)=4.04
��=(8×0.34)+(5×0.34)+(3×0.32)=5.38
VariancesandcovariancesPOWA:
������−��������(��)
=(6−4.04)2 +(4−4.04)2 +(2−4.04)2
=(4.16×0.34)+(3.84×0.34)
+(0.001×0.32)=2.72
������−��������(��)
=(8−5.04)2 +(5−5.04)2 +(3−5.04)2
=(6.86×0.34)+(5.66×0.34)
+(0.14×0.32)=4.30
������−��������(��,��)
=[(6−4.04)(8−5.38)]
+[(4−4.04)(5−5.38)]
+[(2−4.04)(3−5.38)]=(5.13×0.34)
+(4.85×0.34)+(0.01×0.32)=3.40
����−��������(��1,…,����)
= 3.40
√2.72×4.30 =0.99
Pearson’scoef icientcanalsobecalculatedbyadding additionalweightvectorswhereimportantinforma‐tionaboutthecorrelationscanbeadded.ThePC‐OWAWAcananalyzethecorrelationsinmorecomplex scenarios.Itcanbede inedasfollows:
Proposition2. APC‐OWAWAisamapping ����������∶���� →�� ofdimension n withtwosetsof variables ����;���� thathasanassociatedweighting vector��withcomponentsthatlieintheunitinterval andsumtoone.Theformulationisasfollows:
����−����������(��1,…,����)
where ������−���������� and ������−���������� arecal‐culatedasequations()()byOWAWAoperators.The PC‐OWAWAsharestheproprietiesonOWAoperators: monotonic,symmetricandidempotent.
Itisimportanttonotethattheassignmentof weightsisanessentialpointintheOWAaggregation operators.So,manywaysofmeasuringthedegreeof overestimationandunderestimationhavebeenpro‐posed.Yager[30]proposesthedegreeoforness.This is,if ��1 =1,wehaveapure“or”operator.The formulationisobtainedasfollows:
��(��)= �� ��=1 �� ∗ �� ��−�� ��−1 , (13)
where�� ∗ �� isthe���� withthe��thlargest���� value.
Additionally,Yager[30]alsosharestheentropyof dispersion,whichcapturesthevariabilityandtheuse oftheinputsbytheweightsasfollows:
��(��)=− �� ��=1 ����ln(����). (14)
Thebalance[31]measuresthedegreeofselec‐tionbetweenfavoringthehighervaluedelementsor lower‐valuedelements,then:
������(��)= �� ��−1 ��+1−2�� ��−1 ���� (15)
Thedivergence[32]distinguishesbetweentwo OWAweightsvectors,so:
������(��)= �� ��=1
(16)
Thevectorweightmeasurementcanbeusedto calculatethecharacteristicsofthePC‐OWAWAandall theproposalsseenhere.Insomecases,therelation‐shipbetweentwovariablesmaybeaffectedbyvari‐ouselementsthatchangevaluesfromonemomentto another.
Theapproachesdiscussedabovecanalsobe extendedtouseinducedvariables.ThePC‐IPOWAcan connecttheprobabilitiesandthein luenceofother variablesonthestudyintoacoef icientofcorrelation. Themainadvantageisthatwecananalyzesituations inamuchmorecomplexwayasrealitycanpresenton someoccasions.Itcanbede inedasfollows: Proposition3. APC‐IPOWAofdimension n isa model����������∶���� →��withtwosetsofvariables���� ∈ ����,���� ∈���� thathasanassociatedweightingvector �� with ���� ∈[0,1] and ∑�� ��=1 ���� =1,additionally,an inducedIPOWApair ⟨����,����⟩ andaprobabilityvector Pisconsidered.Theformulationcanbede inedas follows:
where ���� isthecalculationofvariancesandcovari‐anceswiththe jthlargest��1.The�� and�� areIPOWA means.
Inthissense,theIOWAWAoperatorcanalsobe usedtocalculatethePearsoncoef icient.ThePC‐IOWAWAisacorrelationcoef icientthatcombines somecharacteristics:1)inducedcriteriaforreorder‐ingargumentsand2)anadditionalweightedvector thatisconsideredwhittheweightedvectorOWA.Itis developedasthefollowingde inition:
Proposition4. APC‐IOWAWAisamodel ������������∶���� →�� withtwosetsofvariables����;���� withtwoweightingvectorsWand V suchthatboth have 0≤���� ≤1 and ∑�� ��=1 ���� =1,additionally,an inducedIPOWApair⟨����,����⟩.Sothat: ����−������������(⟨��1,��1⟩⟨��2,��2⟩,…,⟨����,����⟩)
= ������−������������(��,��) ������−������������(��)×������−������������(��)
= ∑�� ��=1 ����(���� −��)(���� −��), [∑�� ��=1 ����(���� −��)2][∑�� ��=1 ����(���� −��)2] , (18)
where ���� arethecalculationofvariancesandcovari‐anceswiththe jthlargest��1.The��and��areIOWAWA means.
Example2. Considerthedatapreviouslyseen:the variable(X=2,4,6)andthevariable(Y=5,8,3),a weightvector(W=0.3,0.3,0.4)aweightedvector(V =0.2,0.3,0.5),anda��=0.6.Additionally,aninduced vector(U=10,15,12).
��=0.26,0.3,0.44
IOWAWAmeans:
��=(4×0.26)+(6×0.3)+(2×0.44)=3.72
��=(8×0.26)+(3×0.3)+(5×0.44)=5.18
VariancesandcovariancesIOWAWA:
������−������������(��)
=(4−3.72)2 +(6−3.72)2 +(2−3.72)2
=(5.19×0.26)+(2.95×0.3)
+(0.07×0.44)=2.26
������−������������(��)
=(8−5.18)2 +(3−5.18)2 +(5−5.18)2
=(4.75×0.26)+(0.03×0.3)
+(7.95×0.44)=4.74
������−������������(��,��)
=[(4−3.72)(8−5.18)]
+[(6−3.72)(3−5.18)]
+[(2−3.72)(5−5.18)]
=(−4.97×0.26)
+(0.30×0.3)
+(0.78×0.44)=−0.85
����−������������(��1,…,����)
= −0.85
√2.26×4.74 =−0.26
Onecanobservethattheresultscanvaryinquan‐tityandsignwhenweuseinducedoperatorscompar‐ingexercises1and2.
ThePearsoncoef icientcanalsoconsidervery complexscenarioswhereuncertaintyandriskare present.ThePC‐IPOWAWAisacorrelationcoef icient thatusesinducedcomponents,weightedmeansand probabilitytomeasuretherelationshipoftwovari‐ables.Withinthesecharacteristics,itcancollecta seriesoffactorsthataffectthevariablesandprefer‐encesorprobabilitiesofeachdata.ThePC‐IPOWAWA canbede inedasfollows:
Proposition5. APC‐IPOWAWAofdimensionnis amapping ��������������∶���� →�� ifithastwosetsof variables����;���� andthreeweightingvectorsW,Pand V suchthathavecomponentsrangingfromzerotoone andthesumisone,soaninducedIPOWApair⟨����,����⟩ isused.Then:
����−��������������(⟨��1,��1⟩⟨��2,��2⟩,…,⟨����,����⟩)
= Cov IPOWAWA(��,��)
var IPOWAWA(��)×������−��������������(��)
= ∑�� ��=1 ����(���� −��)(���� −��), [∑�� ��=1 ����(���� −��)2][∑�� ��=1 ����(���� −��)2] , (19)
where��and��areIPOWAWAmeans.Thecomponent withtheweight���� istheonethathasthelargest��1 Theweightvectorcanbecalculatedas ��1 =��1��1 + ��2��1 +��3��1,where��1 +��2 +��3 =1 Example3. Considerthedatausedinprevious examples:thevariable(X=2,4,6)andthevariable (Y=5,8,3),aweightvector(W=0.3,0.3,0.4),proba‐bilityvector(P=0.4,0.4,0.2)aweightedvector(V= 0.2,0.3,0.5),andaC=0.3,0.4,0.2.Theinducedvector is(U=10,15,12).
��=0.33,0.35,0.32
IPOWAWAmeans:
��=(4×0.33)+(6×0.35)+(2×0.32)=4.06
��=(8×0.33)+(3×0.35)+(5×0.32)=5.29
VariancesandcovariancesIPOWAWA:
������−��������������(��)
=(4−4.06)2 +(6−4.06)2 +(2−4.06)2
=(3.76×0.33)+(4.24×0.35)
+(0.003×0.32)=2.72
������−��������������(��)
=(8−5.29)2 +(3−5.29)2
+(5−5.29)
2 =(5.24×0.33)
+(0.08×0.35)+(7.34×0.32)=4.10 ������−��������������(��,��)
=[(4−4.06)(8−5.29)]
+[(6−4.06)(3−5.29)]
+[(2−4.06)(5−5.29)]
=(−4.44×0.33)+(0.59×0.35)
+(−0.16×0.32)=−1.30
����−��������������(��1,…,����)
= −1.30 √2.72×4.10 =−0.39
Inthiscase,vectorCindicatesthatthecombinationof probabilityandweightsbringsusclosertoarithmetic means.
4.GeneralizedtheInducedPearson Coefficient
Atechniquethatcanbeusedforcomplexanalysis andgeneratingadditionalscenariosisthegeneralized orquasi‐arithmeticmean.Wecangeneralizethenew proposalspreviouslyseeninthequasi‐PC‐IPOWA,the quasi‐PC‐IOWAWA,andthequasi‐PC‐IPOWAWA.They arede inedasfollows:
Proposition6. Aquasi‐PC‐IPOWAofdimension n isamodel����������∶���� →��withasetofvariables���� ∈ ���� andasecondset ���� ∈���� whichhaveanasso‐ciatedweightingvector �� with ���� ∈[0,1] and ∑�� ��=1 ���� =1 andanassociatedprobabilityvector �� with ∑�� ��=1 ���� =1 and ���� ∈[0,1].Additionally,an inducedIPOWApair⟨����,����⟩isconsidered.Then:
����������−����−����������(⟨��1,��1⟩⟨��2,��2⟩,…,⟨����,����⟩)
= ����������−������−����������(��,��)
����������−������−����������(��)
×����������−������−����������(��) (20)
Particularcase Quasi-PC-IPOWA
���� = 1 ��,��������������
wherethequasi‐varianceandcovarianceIPOWAare calculatedasfollows:
��������−����������(⟨��1,��1⟩⟨��2,��2⟩,…,⟨����,����⟩)
=��−1 �� ��=1
������(����), (21)
��������−����������(⟨��1,��1⟩⟨��2,��2⟩,…,⟨����,����⟩)
=��−1 �� ��=1 ������(����), (22)
���� and���� arethevarianceandcovariancewiththe jth elementwiththelargestvalueof����;���� istheinduced orderofvariables; ��(����) and ��(����) arecontinuous strictlymonotonicfunctions.
Proposition7. Aquasi‐PC‐IOWAWAisamapping ������������∶���� →��withasetofvariables���� ∈���� and aset���� ∈���� suchasanassociatedweightingvector �� andaprobabilityvectorP,whichcomponentsare rangingfromzerotooneandthesumisone.Addition‐ally,aninducedIPOWApair⟨����,����⟩isconsidered.So:
Quasi PC IOWAWA(⟨��1,��1⟩⟨��2,��2⟩,…,⟨����,����⟩) = ����������−������−������������(��,��) ����������−������−������������(��) ×����������−������−������������(��) (23)
wherethevariancesandcovariancearecalculatedin aquasi‐formas()().
Proposition8. Aquasi‐PC‐IPOWAWAisamapping ��������������∶���� →�� withasetofvariables ����;���� suchasanassociatedweightingvector �� with ���� ∈ [0,1] and ∑�� ��=1 ���� =1,aprobabilityvector �� with ∑�� ��=1 ���� =1 andweightedvector �� with ∑�� ��=1 ���� =1 and ���� ∈[0,1].Additionally,aninducedIPOWApair ⟨����,����⟩isconsidered.Theformulationisasfollows:
Quasi PC IOWAWA(⟨��1,��1⟩⟨��2,��2⟩,…,⟨����,����⟩) = ����������−������−������������(��,��) ����������−������−������������(��)
×����������−������−������������(��) (24)
Additionally,thefamiliesofthegeneralizedPC‐IPOWA,PC‐IOWAWA,andPC‐IPOWAWAoperatorcan beseeninTables1–3.
Quasi‐arithmeticPearsoncoef icientinducedprobabilisticorderedweighted(Quasi‐PC‐IPOWA)
��(��)=���� GeneralizedPC‐IPOWA
��(��)=�� PC‐IPOWA
��(��)=��2
Pearsoncoef icientinducedprobabilisticorderedweightedquadraticaverage(PC‐IPOWQA)
��(��)→����,��������→0 Pearsoncoef icientinducedprobabilisticorderedweightedgeometricaverage(PC‐IPOWGA)
��(��)=��−1
Pearsoncoef icientinducedprobabilisticorderedweightedharmonicaverage(PC‐IPOWHA)
��(��)=��3 Pearsoncoef icientinducedprobabilisticorderedweightedcubicaverage(PC‐IPOWCA)
��(��)→����,��������→∞ Maximum
��(��)→����,��������→−∞ Minimum
Table2. FamiliesofgeneralizedPC‐IOWAWA
Particularcase Quasi-PC-IOWAWA
���� = 1 ��,��������������
��(��)=����
Quasi‐arithmeticPearsoncoef icientinducedorderedweightedaveraging‐weighted(Quasi‐PC‐IOWAWA)
GeneralizedPC‐IOWAWA
��(��)=�� PC‐IOWAWA
��(��)=��2
Pearsoncoef icientinducedorderedweightedaveraging‐weightedquadraticaverage(PC‐IOWAWQA)
��(��)→����,��������→0 Pearsoncoef icientinducedorderedweightedaveraging‐weightedgeometricaverage(PC‐IOWAWGA)
��(��)=��−1
��(��)=��3
Pearsoncoef icientinducedorderedweightedaveraging‐weightedharmonicaverage(PC‐IOWAWHA)
Pearsoncoef icientinducedorderedweightedaveraging‐weightedcubicaverage(PC‐IOWAWCA)
��(��)→����,��������→∞ Maximum
��(��)→����,��������→−∞ Minimum
Table3. FamiliesofgeneralizedPC‐IPOWAWA
Particularcase Quasi-PC-IPOWAWA
���� = 1 ��,��������������
��(��)=����
Quasi‐arithmeticPearsoncoef icientinducedprobabilisticorderedweightedaveraging‐weighted (Quasi‐PC‐IPOWAWA)
GeneralizedPC‐IPOWAWA
��(��)=�� PC‐IPOWAWA
��(��)=��2
��(��)→����,��������→0
Pearsoncoef icientinducedprobabilisticorderedweightedaveraging‐weightedquadraticaverage (PC‐IPOWAWQA)
Pearsoncoef icientinducedprobabilisticorderedweightedaveraging‐weightedgeometricaverage (PC‐IPOWAWGA)
��(��)=��−1 Pearsoncoef icientinducedprobabilisticorderedweightedaveraging‐weightedharmonicaverage (PC‐IPOWAWHA)
��(��)=��3 Pearsoncoef icientinducedprobabilisticorderedweightedaveraging‐weightedcubicaverage(PC‐IPOWAWCA)
��(��)→����,��������→∞ Maximum
��(��)→����,��������→−∞ Minimum
Duetothesigni icantgrowthofmarketsworld‐wide,itiscommonforturmoilinsome inancial marketstoaffectothers.Theimpactsofstockmar‐ketinterdependencebecomeclearerininstability [33–35].
SinceMarkowitz[36]considerstheinterdepen‐denceofmarketsasatriggerforrisk,manystudies haveemergedtomeasuretheexistingrelationship. Inthissense,severalstudieshavebeenproposedas theinterrelationofmarketsinemergingeconomies [37,38],therelationshipwithotherprices[39,40],and witheconomicgrowth[41,42].
Theyear2020wasaperiodofinstabilitywherethe COVIDpandemichadarelevantimpactonworldstock markets[43,44].Giventhisscenario,itisinterestingto knowthecorrelationobservedbetweensomeofthe mostin luentialstockexchanges.
Therefore,thisresearchconsidersanapplication ofthemethodologyofPearsoncorrelationwithOWA operatorsintenofthemostextensivestockindexes intheworld.TheperiodstudiedisfromJanuaryto
December2020.Theprocessforobtainingresultsis describedinthefollowingsteps:
Step1. Thedatastudiedarede inedintermsof indexandperiod.
Step2. OWAvectorsaredescribed.Vectorsof weights,probabilities,andinduced.
Step3. CalculationofOWAmeans.
Step4. Calculationofvariancesandcovariances withthedifferentOWAoperators.
Step5. Results.ThePearsoncorrelationwithOWA operatorsisde inedinthetenstockexchanges.
5.1.TheProcessinPearsonCorrelationwithOWAs
Inordertoanalyzethecorrelationbetweensome stockexchanges,thefollowinghasbeencarriedout:
Step1. Tenrepresentativeindexesofin luential stockexchangeshavebeenselected:NYSE,NASDAQ, HangSeng,Nikkei225(Nikkei),Euronext100 (Euronext),FTS100(FTS),BSESensex(BSE),S&P‐tsx,S&Pasx200(S&P‐asx).Thedataaremonthlyfor theyear2020.Table4showstheinformation.
Step2. OWAweightsvectors.Toestimatethe means,variancesandcovarianceswiththeproposed
Table13. PC‐IPOWAWAcorrelationsbyranges
0.9to1
NYSE‐Nikkei
NYSE‐Euronext
0.7-0.89
0.5-0-69 0.30-0.49
minor0.29andnegative
NYSE‐NASDAQ NYSE‐FTSE NASDAQ‐Hangseng NASDAQ‐FTSE
NYSE‐Shanghai NASDAQ‐Euronext NASDAQ‐S&P‐asx Shanghai‐FTSE
NYSE‐BSE NYSE‐Hangseng Shanghai‐Euronext Shanghai‐Hangseng
NYSE‐S&P‐tsx NNYSE‐S&P‐asx Hangseng‐Nikkei Shanghai‐S&P‐asx
NASDAQ‐Shanghai NASDAQ‐Nikkei Hangseng‐FTSE Nikkei‐FTS
Nikkei‐BSE NASDAQ‐BSE FTSE‐S&P‐tsx FTSE‐BSE
Euronext‐S&P‐tsx NASDAQ‐S&P‐tsx
Euronext‐S&Ptasx Shanghai‐Nikkei
BSE‐S&P‐tsx Shanghai‐BSE
S&Ptxs‐S&P‐asx Shanghai.S&P‐tsx
Hangseng‐Euronext
Hangseng‐BSE
Hangseng‐S&P‐tsx
Hangseng‐S&Ptasx
Nikkei‐Euronext
Nikkei‐S&P‐tsx
Nikkei‐S&P‐asx
Euronext‐FTSE
Euronext‐BSE
FTSE‐S&P‐asx
BSE‐S&P‐asx
OWAextensions,aseriesofadditionalvectorsare necessary.Theprobabilityvector(P)wasestablished withacriterionthatclosevaluesaremorelikelyto occur.TheOWAvector(W)isarandomselection.
Theweightedvector(WA)valuedmorethemonths whenCOVIDstarted.Forpracticalpurposes,the inducedvector(I)ineachcaseordersthedatabydate fromtheclosesttothefurthest.Table 5 showsthe information:
Step3.ThecalculationofthecorrelationwithOWA operatorsimpliesthatthemeansOWAareconsidered toreplacethearithmeticmeans.Table 6 showsthe meansOWAofeachoftheindices.
Step4. VariancesandcovariancesOWAcalculation. Previouslyseenmeansaresubstitutedforvariances andcovariances.Table7showsthevariancesforeach oftheindicatorsdependingontheOWAoperator used.
NotethattheIPOWAoperatoroverestimatesthe variances.TheIOWAWAoperatoristheonewiththe smallestvariances,whichindicatesthatthemonthsof thestartofthecoviddidnotin luencethevariation oftheindicesuntilmonthslater.Thecovariancesare describedinTable8.
Theideaabouttheestimationpreviouslyseen appliesthesameinthecovariancesandthechosen OWAoperator.
Step5. Usingthevariancesandcovariancesfor eachOWAoperatorinthePearsoncoef icientformula, theresultsareobtained.Inordertomakeacompari‐sonwitharithmeticcalculations,theresultsare irst presentedwithoutOWAoperators.Table9showsthe data.
TheindiceswiththemostcorrelationareNASDAQ‐Shanghai,Nikkei‐BSE,NYSE‐BSE,NYSE‐S&P‐tsx, BSE‐S&P‐tsx,Euronext‐S&P‐asxandS&P‐tsx‐S&P‐asx. Additionally,thereisanegativecorrelationbetween
NASDAQ‐FTSEandShanghai‐FTSE.Withtheseresults, wewentontoanalyzetheinformationwithOWA operators.Table10presentstheresultsofPC‐IPOWA.
Notethatwhenweuseprobabilitiesandsubesti‐matethemonthswithmorevariations,thecorrelation increasesslightlyforindiceswithacorrelationgreater than0.9.AninterestingissueisthattheNASDAQ‐FTSE correlationturnspositive.Intheuseoftheweighted vector,Table11showsthePC‐IOWAWAresults.
Whenacriterionthattakesthemonthsofthe onsetofCOVIDintoaccount,theresultisverysimilar tothearithmeticaverage.Onecanobserveonlya slightincreaseinthecorrelations.Thenweconnect thelasttwoproposalsandcalculatethePC‐IPOWAWA. Table12presentstheinformation.
NotethatwhenamorecomplexOWAoperatoris used,thenegativevaluesdisappear.However,thecor‐relationscontinuetoretainsimilarorslightlyhigher values.Inordertoknowtowhatextenteachofthe indicescorrelates,weplacethemindifferentranges. Table13showstheorder.
Onecanseethatthemostcommoncorrelationof theindicesisbetween0.7to0.89.Almost50%ofthe correlationsareinthisrange.OnlytheNASDAQ‐FTSE andShanghai‐FTSEcorrelatelessthan0.3.Withinthe correlationsgreaterthan0.9,theNYSEcorrelation withotherindicessuchasNikkei,Euronext,BSE,S&P‐tsxandhowthesearealsostronglyrelatedtoeach other.
Stockmarketsareessentialindevelopingcoun‐tries,giventhenumberofparticipants,themove‐ments,andthevariablesthatcausethemtobecomeof greatinterest.Withtheincreasingintegrationofmar‐kets,itisevidentthattheindicesofstockexchanges withsimilarcharacteristicstendtomovetogether.
Howimportantisthisrelationship,andwhatques‐tionsthatbecomeimportantfordecision‐makingin the inancialarea?
ThisworkproposesaPearsoncoef icientthat usesOWAaggregationoperatorsinitsformulation.In ordertoanalyzestockindicesandothercomplexdata, inducedaggregationoperators(IOWA),probabilistic (IPOWA),andweighted(IOWAWA)areused.Themain advantageistoobtainacorrelationcoef icientthatcan beoverestimatedorunderestimatedbythedecision‐makeraccordingtotheinformationavailable.Inthis sense,thePearsoncoef icientresultswithOWAoper‐atorscanbeanalyzedinawiderangeofscenarios.
Thenewmethodologyisappliedtotenindicesof majorstockexchangesintheworld.Themainresults showthattheseindicestendtohaveapositivecorrela‐tiontodifferentdegrees.Thecorrelationsincreasein timeswhenthevariancesarehigher.Inthe irstyearof COVID‐19,thecorrelationbetweenindicesincreased slightly.Evencorrelationsthatwereslightlynegative turnpositivewhenconsideringprobabilityandweight inthemonthsaftertheonsetofthepandemic.The highestcorrelationsarefoundbetweentheindices NYSE‐Nikkei‐Euronext,BSE,andS&P‐tsx.
MarthaFlores-Sosa –UniversidadAutónomade Occidente,BlvdLolaBeltrans/n,80020,Sinaloa, Mexico,e‐mail:martha. lores@uadeo.mx.
ErnestoLeon-Castro∗ –FacultyofEconomicsand AdministrativeSciences,UniversidadCatólicadela SantísimaConcepción,Concepción,Chile,Instituto TecnologicodeSonora,UnidadNavojoa,Ramon CoronasinnumeroColoniaITSON,Sonora,Mexico, C.P.85860,e‐mail:eleon@ucsc.cl.
JoseM.Merigo –SchoolofInformation,Systems &Modelling,FacultyofEngineeringandInforma‐tionTechnology,UniversityofTechnologySydney, 81Broadway,Ultimo,2007,NSW,Australia,e‐mail: jose.merigo@uts.edu.au.
∗Correspondingauthor
ACKNOWLEDGEMENTS
ResearchsupportedbyRedSistemasInteligentesand ExpertosModelosComputacionalesIberoamericanos (SIEMCI),projectnumber522RT0130inPrograma IberoamericanodeCienciaandTecnologiaparael Desarrollo(CYTED).
References
[1] R.Barro,“Thestockmarketandinvestment,” The ReviewofFinancialStudies,vol.3,no.1,1990, pp.115–131.
[2] L.Wang,“Stockmarketvaluation,foreign investment,andcross‐countryarbitrage,” Global FinanceJournal,vol.40,2019,pp.74–84.
[3] N.NguyenandC.Trouong,“Theinformation contentofstockmarketsaroundtheworld:A culturalexplanation,” JournalofInternational
FinancialMarkets,InstitutionsandMoney, vol.26,2013,pp.1–29.
[4] I.Tsai,“Thesourceofglobalstockmarketrisk:A viewpointofeconomicpolicyuncertainty,” EconomicModelling,vol.60,2017,pp.122–131.
[5] D.GilesandY.Li,“Modellingvolatilityspillover effectsbetweendevelopedstockmarketsand Asianemergingstockmarkets,” International JournalofFinance&Economics,vol.20,no.2, 2014,pp.155–177.
[6] W.Mensi,S.Hammoudeh,S.ShahzadandM. Shahbaz,“Modelingsystemicriskanddepen‐dencestructurebetweenoilandstockmarkets usingavariationalmodedecomposition‐based copulamethod,” JournalofBanking&Finance, vol.75,2017,pp.258–279.
[7] R.Efendi,N.ArbaiyandM.Deris,“Anew procedureinstockmarketforecastingbased onfuzzyrandomauto‐regressiontimeseries model,” InformationSciences,vol.441,2018, pp.113–132.
[8] A.Bukhari,M.S.S.Raja,S.Islam,M.Shoaiband P.Kuman,“Fractionalneuro‐sequentialARFIMA‐LSTMfor inancialmarketforecasting,” IEEE Access,vol.8,2020,pp.71326–71338.
[9] G.Caporale,K.YouandL.Chen,“Globaland regionalstockmarketintegrationinAsia:A panelconvergenceapproach,” International ReviewofFinancialAnalysis,vol.65,2019,p. 101381.
[10] C.BotocandS.Anton,“Newempiricalevidence onCEE’sstockmarketsintegration,” TheWorld Economy,vol.43,no.10,2020,pp.2785–2802.
[11] J.Barunik,E.KocendaandL.Vacha,“Gold,oil, andstocks:Dynamiccorrelations,” International ReviewofEconomics&Finance,vol.42,2016, pp.186–201.
[12] S.JungandW.Chang,“Clusteringstocksusing partialcorrelationcoef icients,” PhysicaA:StatisticalMechanicsanditsApplications,vol.462, 2016,pp.410–420.
[13] G.Wang,C.XieandH.Stanley,“Correlation structureandevolutionofworldstockmarkets: EvidencefromPearsonandpartialcorrelation‐basednetworks,” ComputationalEconomicsvolume,vol.51,2018,pp.607–635.
[14] R.Yager,“Onorderedweightedaveragingaggre‐gationoperatorsinmulti‐criteriadecisionmak‐ing,” IEEETrans.Syst.ManCybern.B,vol.18, 1988,pp.183–190.
[15] R.YagerandD.Filev,“Inducedorderedweighted averagingoperators,” IEEEtransactionsonsystems,manandcybernetics,vol.29,no.2,1999, pp.141–150.
[16] J.Merigó,“ProbabilitiesintheOWAoperator,” ExpertSystemswithApplications,vol.39,2012, pp.11456–11467.
[17] J.Merigó,“Auni iedmodelbetweentheweighted averageandtheinducedOWAoperator.,” ExpertSystemswithApplications,vol.39,no.9, pp.11560–11572,2011.
[18] J.Merigó,C.Lobato‐CarralandA.Carrillero‐Castillo,“DecisionmakingintheEuropeanUnion underriskanduncertainty,” EuropeanJournal InternationalManagement,vol.6,no.5,2012, pp.590–609.
[19] R.YagerandG.Beliakov,“OWAOperatorsin RegressionProblems,” IEEETransactionson FuzzySystems,vol.18,no.1,pp.106–113,2010.
[20] M.Flores‐Sosa,E.Avilés‐Ochoa,J.Merigó,and R.Yager,“VolatilityGARCHmodelswiththe orderedweightedaverage(OWA)operators,” InformationSciences,vol.565,2021,pp.46–61.
[21] E.León‐Castro,L.Espinoza‐Audelo,J.Merigó, E.Herrera‐ViedmaandF.Herrera,“Measuring volatilitybasedonorderedweightedaverage operators:Agriculturalproductspricescaseof use,” FuzzySetsandSystems,2020.
[22] J.Merigó,M.GuillenandJ.Sarabia,“TheOrdered WeightedAverageintheVarianceandthe Covariance,” InternationalJournalofIntelligent Systems,vol.30,no.9,2015,pp.985–1005.
[23] J.Merigó,“Auni iedmodelbetweentheweighted averageandtheinducedOWAoperator,” Expert SystemswithApplications,vol.38,no.9,2011, pp.11560–11572.
[24] R.Yager,K.EngemannandD.Filev,“Onthe conceptofimmediateprobabilities,” InternationalJournalofIntelligentSystems,vol.10,1995, pp.373–397.
[25] J.Merigó,“Auni iedmodelbetweentheweighted averageandtheinducedOWAoperator,” ExpertSystemswithapplicacions,vol.38,2011, pp.11560–11572.
[26] R.Yager,“Ontheinclusionofvarianceindecision makingunderuncertainty,” InternationalJournalUncertainFuzzyKnowl-BasedSystems,vol.4, pp.401–419,1996.
[27] J.Merigó,“Auni iedmodelbetweentheweighted averageandtheinducedOWAoperator,” Expert SystemswithApplications,vol.38,pp.11560–11572,2011.
[28] K.Pearson,“Mathematicalcontributionstothe theoryofevolution‐III.Regression,heredity,and panmixia,” PhilosoplicalTransactionsoftheRoyal SocietyA,vol.18,1896,pp.253–318.
[29] J.Benesty,J.Chen,Y.Huang,andI.Cohen,“Pear‐sonCorrelationCoef icient,” NoiseReductionin SpeechProcessing,vol.2,2009,pp.1–4.
[30] R.Yager,“Onarderedweightedaveragingaggre‐gationoperatorsinmulti‐criteriadecisionmak‐ing,” IEEETransactionsSystems.ManCybernetics B,vol.18,1988,pp.183–190.
[31] R.Yager,“ConstrainedOWAaggregation,” Fuzzy SetsandSystems,vol.81,1996,pp.89–101.
[32] R.Yager,“HeavyOWAoperators,” FuzzyOptimizationandDecisionMaking,vol.1,2002, pp.379–297.
[33] D.BlesserandJ.Yang,“Thestructureofinterde‐pendenceininternationalstockmarkets,” JournalofInternationalMoneyandFinance,vol.22, no.2,2003,pp.261–267.
[34] X.Zhang,X.Zheng,andD.Zeng,“Thedynamic interdependenceofinternational inancialmar‐kets:Anempiricalstudyontwenty‐sevenstock markets,” PhysicaA:StatisticalMechanicsandits Applications,vol.472,2017,pp.32–42.
[35] J.Chevallier,“Marketintegrationand inancial linkagesamongstockmarketsinPaci icBasin countries,” JournalofEmpiricalFinance,vol.46, 2018,pp.77–92.
[36] H.Markowitz,“Portafolioselection,” TheJournal ofFinance,vol.7,1852,pp.77–91.
[37] S.Paramati,A.Zakari,M.Jalle,S.KaleandP. Begari,“Thedynamicimpactofbilateraltrade linkagesonstockmarketcorrelationsofAus‐traliaandChina,” AppliedEconomicsLetters, vol.25,no.3,2018,pp.141–145.
[38] R.Dias,J.VidigaldaSilva,andA.Dionisio,“Finan‐cialmarketsoftheLACregion:Doesthecri‐sisin luencethe inancialintegration?,” InternationalReviewofFinancialAnalysis,vol.63,2019, pp.160–173.
[39] P.Ferreira,E.Pereira,M.F.daSilva,andH.Pereira, “Detrendedcorrelationcoef icientsbetweenoil andstockmarkets:Theeffectofthe2008crisis,” PhysicaA:StatisticalMechanicsanditsApplications,vol.517,2019,pp.86–96.
[40] S.Singhal,S.Choudhary,andP.Biswal,“Return andvolatilitylinkagesamongInternational crudeoilprice,goldprice,exchangerateand stockmarkets:EvidencefromMexico,” Resources Policy,vol.60,2019,pp.255–261.
[41] H.HouandS.Cheng,“Thedynamiceffectsof banking,lifeinsurance,andstockmarketson economicgrowth,” JapanandtheWorldEconomy, vol.41,2017,pp.87–97.
[42] T.FufaandJ.Kim,“Stockmarkets,banks,and economicgrowth:Evidencefrommorehomoge‐neouspanels,” ResearchinInternationalBusiness andFinance.,vol.44,2018,pp.504–517.
[43] S.Baker,N.Bloom,S.Davis,K.Kost,M.Sammon, andT.Viratyosin,“TheUnprecedentedStock MarketReactiontoCOVID‐19,” TheReviewof AssetPricingStudies,vol.10,no.4,2020,pp.742–758.
[44] B.Ashraf,“Stockmarkets’reactiontoCOVID‐19:Casesorfatalities?,” ResearchinInternational BusinessandFinance,vol.54,2020,p.101249.
[45]
J.RodgersandW.Nicewander,“Thirteenwaysto lookatthecorrelationcoef icient,” TheAmerican Statistician,vol.42,no.1,1988,pp.59–66.
[46]
[47]
G.Kabir,S.Tesfamariam,J.Loeppky,andR.Sadiq, “IntegratingBayesianLinearRegressionwith OrderedWeightedAveraging:UncertaintyAnal‐ysisforPredictingWaterMainFailures,” Journal ofRiskandUncertaintyinEngineeringSystems, PartA:CivilEngineering,vol.1,no.3,2015.
Submitted:23rd February2022;accepted:25th January2023
YassineAkhiat,AhmedZinedine,MohamedChahhou DOI:10.14313/JAMRIS/1‐2024/6
Abstract:
FeatureSelection(FS)isanessentialresearchtopicin theareaofmachinelearning.FS,whichistheprocess ofidentifyingtherelevantfeaturesandremovingthe irrelevantandredundantones,ismeanttodealwithhigh dimensionalityproblemstoselectthebestperforming featuresubset.Intheliterature,manyfeatureselection techniquesapproachthetaskasaresearchproblem, whereeachstateinthesearchspaceisapossiblefeature subset.Inthispaper,weintroduceanewfeatureselec‐tionmethodbasedonreinforcementlearning.First,deci‐siontreebranchesareusedtotraversethesearchspace. Second,atransitionsimilaritymeasureisproposedsoas toensureexploit‐exploretrade‐off.Finally,theinforma‐tivefeaturesarethemostinvolvedonesinconstructing thebestbranches.Theperformanceoftheproposed approachesisevaluatedonninestandardbenchmark datasets.TheresultsusingtheAUCscoreshowtheeffec‐tivenessoftheproposedsystem.
Keywords: Featureselection,Datamining,Decisiontree, Reinforcementlearning,Dimensionalityreduction
1.Introduction
Withtheadventofhigh‐dimensionaldata,typ‐icallymanyfeaturesareirrelevant,redundantand noisyforagivenlearningtaskastheyhaveharm‐fulconsequencesintermsofperformanceand/or computationalcost.Moreover,alargenumberoffea‐turesrequiresalargeamountofmemoryorstorage space.Applyingdataminingandmachinelearning algorithmsinhigh‐dimensionaldatausuallyleadsto thedowngradingoftheirperformanceduetoover‐ittingproblem[1,2].Giventheexistenceofalarge numberoffeatures,machinelearningmodelsbecome intricatelycomplicatedtointerpretastheircomplex‐ityincreasesleadingtotherestrictionofthegeneraliz‐ability.Therefore,reducingthedimensionalityofdata hasbecomeindispensableinrealworldscenariosto successfullybuildunderstandableandaccuratemod‐elsthatcanimprovedata‐miningperformanceand enhancemodelsinterpretability.Dataminingcantake advantageofdimensionalityreductiontoolswhich areintegralparameterscentraltodatapre‐processing toreducethehighnessofdatadimensionality[3].
Dimensionalityreductioncanbecategorizedintofea‐tureextractionandfeatureselection(see igure1) [4–6].Featureextractionaimsattransformingthe originalfeaturespacetoanewreducedone,where featureslosetheirmeaningduetothetransformation
[7–9,9,10].Incontrasttofeatureextraction,feature selectionistheprocessofidentifyingtherelevantfea‐turesandremovingtheirrelevantandredundantones withtheobjectiveofobtainingthebestperforming subsetoforiginalfeatureswithoutanytransformation [11–13].Thus,theconstructedlearningmodelsusing theselectedsubsetoffeaturesaremoreinterpretable andreadable.Thisgivespreferencetothereliable applicabilityoffeatureselectionasaneffectivealter‐nativeprioritizedoverfeatureextractioninmanyreal‐worlddatasets.Themajorreasonsforapplyingthe featureselectionarethefollowing:
‐ Makingmodelseasiertointerpret.
‐ Reducingresourcesrequirement(shortertraining time,smallstoragecapacityetc.).
‐ Avoidingthecurseofdimensionality.
‐ Avoidingtheover‐ ittingproblem,thus,abetter model.
‐ Improvingaccuracy:lessnoiseindatameans improvedmodelingaccuracy.
Ingeneral,featureselectionalgorithmsarecate‐gorizedinto:Supervised,Semi‐supervisedandUnsu‐pervisedfeatureselection[12,14–18].Inthispaper, weputmoreemphasisonsupervisedfeatureselec‐tion,whichisathreefoldapproach,Filter,Wrapper [19–23],andEmbedded[24–26](seeFig. 1).Filter Methodsrelyontherelationshipbetweenfeatures andclasslabel(suchasdistance,dependency,corre‐lationetc.)toassesstheimportanceoffeatures.This categoryisapre‐processingstep,whichisindepen‐dentfromtheinductionalgorithm.Filtersareknown bytheireaseofuseandlowcomputationalcost.On thecontrary,theWrapperapproachgeneratesmod‐elswithsubsetsoffeatures.Then,itusespredic‐tionperformanceasacriterionfunctionoraguiding‐compasstoorientthesearchforthebestfeaturesub‐set.Thisapproachtakesintoaccounttheinteractions betweenfeatures.Generally,Wrappersachievebetter performancethansomeFiltermethods.TheEmbed‐dedapproachperformsfeatureselectionbyimplica‐tionwhilesimultaneouslyconstructingmodels,which makesthemlesscostlyintermsofexecutiontimethan wrappersdo.
Inthispaper,weintroduceanewfeedbacksystem basedonreinforcementlearningtosolvethefeature selectionproblem.Thesystemkeepsexploringthe statespacewhileitismovingthroughtheavailable spaceoffeaturestoselectthebestsubset.Inthissys‐tem,wehaveusedthedecisiontreebranches.There‐fore,eachsubsetisrepresentedbyabranch.The mainideaoftheproposedfeatureselectionalgorithm istoselecttheapplicablesubsetoffeatures,which aremostlyinvolvedinconstructingef icientbranches. Initspreliminaryoutset,thesystemendeavorsto buildthe irstbranchwithoutanypre‐installedknowl‐edge(exploringtheenvironment).Asiterationstran‐spireinlinearlysuccessivealternation,thesystem accumulatesexperiencesthatfurnishthegroundfor constructingbetterbranches(diverse,relevant,etc.) usingthepropoundedTransitionSimilarityMeasure (TSM).Outofthebestbranches,weselectthemost utilizedfeaturesincreatingthem(SeetheFig.2).The contributoryaspirationsandthequintessentialmain‐springsofthisstudyarefourfold.
1) Areinforcementlearning‐basedmethodisdevel‐opedtobeusedinselectingthebestsubsetof features.
2) Theproposedsystemtraversesthestatespaceto selecttheinformativesubsetusingamodi iedver‐sionofdecisiontreebranches.Sincethetransi‐tionbetweenstates(featuresubsets)iscontrolled usingDecisiontreebranches,theproposedsys‐temisstraightforwardlyaccessible.Asaresult,the spotlightedsolution,througheffectiveimplemen‐tationofthesuggestedfeatureselectionmethod, ourproposedsystemisrenderedcomprehensively interpretable.
3) Transitionsimilaritymeasure(TSM)isintended tomaintaintheprogressivesustainabilitythe system’senvironmentalexplorationbycreating newbranchesandsimultaneouslyexploitingwhat haslearnedtoavoidredundancyandmaximize diversity.
4) Theproposedsystemcanbeadaptedtoanyprob‐lem(itisnotdependentonaspeci icdataset) becauseourfeatureselectionproblemisconsid‐eredasreinforcementlearning.
Theremainderofthispaperisorganizedasfol‐lows:sectiontwopresentstherelatedworks.Section threeisdevotedtotheproblemandourintroduced contributions.Inthefourthsection,theresultsofthe proposedsystemareintroduced.Astothelastsection, itisputforwardtoconcludethiswork.
Extensiveresearchanddeeplythoroughbreak‐throughshavebeendisclosedinthedomainoffeature selectionasanever‐evolving ieldofstudy[3,13,19, 27].Inthissection,somewrapperalgorithmssimilar tothefundamentaloneencompassedbythispaperare brie lyreviewed.The irstalgorithmisubiquitousin FSstateoftheart,whichisforwardselection[28,29]. (1)itstartswithanemptysubset;(2)addstothe subsetthefeaturethatincreasesitsperformance;(3) repeatsStep2untilallfeatureshavebeenexaminedor untilnobetterperformanceispossible;(4)returns thesubsetoffeaturesthatyieldsmaximumperfor‐manceonthevalidationset[30].Thismethodisfast andeffective,butittendstoover‐ itthevalidationset. In[20],theauthorsproposedanewalgorithmenti‐tledensemblefeatureselection,whichsigni icantly reducesthisproblem.
Traversingthesearchspace bycreatingDTbranches
DecisionTreebranches (Algorithm1)
Thebestbranchesare identifiedaccordingtothe (Bestfeaturesubbsets) Rewardfunction
TransitionSimilarityMeasure (TMS) BestConstructed Branches Optimalsubset
First,foreachfeature,theytraindifferentmodels usingdifferentclassi icationalgorithms.Then,they storetheminalibraryofmodels.Second,theyuse aselectionwithreplacementtechnique[30]to ind theoptimalsubsetofmodelsthat,whenaveraged together,achievesexcellentperformance.Another wrappermethodbasedongraphrepresentationis proposedin[14],wherethenodedegreeisusedas acriteriontoselectthebestfeaturessubsetamong thewholefeaturesspace.Thisalgorithmconsistsof twophases:(1)Choosingfeaturestobeusedingraph construction.(2)Constructingagraphinwhicheach nodecorrespondstoeachfeature,andeachedgehas aweightcorrespondingtothepairwisescoreamong featuresconnectedbythatedge.Finally,thebestfea‐turesarethenodeswiththehighestdegree.In[31],a pairwisefeatureselection(FS‐P)hasbeenintroduced, featuresareevaluatedinpairsusingdecisiontree classi ier.First,itranksfeaturesindividually.Second, itinvolvesthemachinelearningalgorithm(Decision tree)toevaluatepairsoffeatures.In[32,33],awell‐knownwrapperapproachispresented,RecursiveFea‐tureEliminationusingRandomForest(RFE).RFEper‐formsfeatureselectionrecursively.Atthe irstitera‐tion,themodel(Randomforest)istrainedonwhole setofattributes.Afterrankingfeaturesaccordingto themodel’simportance,theleastimportantfeatures areeliminated.Asiterationtakesplace,theconsider‐ingsetoffeaturesbecomesmallerandsmalleruntil thedesirednumberoffeaturesisreached.
Featurespacecontainesall features(irrelevant,noisy, redundant,andrelevant)
TMSisusedtoensure theexploit/exploretrade-off ofreinforcementlearning
Theoptimalsubsetincludes themostinvolvedfeaturesin constructingthebestbranches
Randomforestsareamongthemostpopular machinelearningalgorithms[34].Thankstoits performance,robustness,andinterpretability,RFhas provedthefrequencyofitsbene icialapplicability. Theycanselectinformativevariables[11].RFper‐formsfeatureselectionusingmeandecreaseimpu‐rityandmeansdecreaseaccuracycriteria[35].Mean decreaseimpurityisusedtomeasurethedecrease intheweightedimpurityintreesbyeachfeature. Therefore,thefeaturesarerankedaccordingtothis measure.Meandecreaseaccuracyisameasureof thefeatureimpactonmodelaccuracy.Thevalues ofeachfeaturearepermuted irst.Then,wemea‐surehowthispermutationdecreasesmodelaccu‐racy.Theinformativefeaturesdecreasethemodel accuracysigni icantly,whileunimportantfeatures donot.
Asopposedtothetraditionalfeatureselection(FS) formalizationandtheinspirationgeneratedfromthe reinforcementlearningapproach,thefeatureselec‐tionproblemcanbeeffortlesslyhandledwiththeprof‐itablereliabilityofourproposedsystem.Thefeature spaceusingourapproachcanbeseenasaMarkov decisionprocess(MDP)[36,37],whereeachsubset offeaturesisrepresentedbyastate(decisiontree branch).Oursystemexploresthestatespacewhileit exploitsthegatheredexperiencessofarusingthepro‐posedtransitionsimilaritymeasure(TSM).In[38],the authorsproposedamethodbasedonreinforcement learning(RL)forselectingthebestsubset.First,they useanAOR(averageofrewards)criteriontoidentify theeffectivenessofagivenfeatureindifferentcon‐ditions.AORistheaverageofthedifferencebetween
twoconsecutivestatesinseveraliterations.Second, theyintroduceanoptimalgraphsearchtoreducethe complexityoftheproblem.
Thewayoursystemtraversesfromonestate toanotherishandledusingdecisiontreebranches torepresenteachstate,asmentionedbefore.Inits totality,thistechniqueissimilartothewayRFcre‐atesbranch.TheRFmethodcreatesmultipletrees. Foreachtree,onlyarandomsubsetofinputvari‐ablesisusedateachsplittingnode.Therefore,the inaltreesofRFareindependentofeachother,and theydonotlearnfromthepreviouslycreatedtrees. Ontheotherhand,oursystemcanlearnfromprior attempts.Ateachiteration,itexploresnewbranches andexploitstheassimilatedknowledgetocreate highly‐performativeandqualitativeonesinthesubse‐quentiteration.
Thispaperforegroundstothebringsanewfea‐tureselectionsystembasedonreinforcementlearn‐ing;theproposedsystemprincipallycomprisesthree parts.First,decisiontreebranchesareusedtotra‐versethesearchspace(featuresspace)tocreatenew rules(branchesorfeaturesubsets)andselectthebest featuresubset.Second,atransitionsimilaritymeasure (TSM)isintroducedtoensurethatthesystemkeeps exploringthestatespacebycreatingnewbranches andexploitingwhatithaslearnedsofartocircum‐venttheproblematicimplicationsorthedrawbacksof redundancy.Finally,therelevantfeaturesarethemost involvedonesinconstructingthebranchesofhigh quality.Forfurtherillustrativeexplications,thesub‐sequentsectionwillaccessiblyresurfacethegeneral frameworkofreinforcementlearninganddelineate theknow‐howdimensionsinwhichoursystemcan synthesizethebene itsofthispowerfulapproach.
RListhemostactiveandfast‐developingareain machinelearningandisoneofthreebasicmachine learningapproaches,alongsidesupervisedlearning andunsupervisedlearning.RLconsistsofthefol‐lowingconcepts:Agent,environment,actions,and reward.TheagenttakesactionAandinteractswith anenvironmenttomaximizethetotalrewardreceived R.Atiterationt,theagentobservesstateStfromthe environment.Inreturn,theagentgetsarewardRt. Theagenttakesaction ����.Inresponse,theenviron‐mentprovidesthenextstate ����+1 andreward;the processcontinuesuntiltheagentwillbeabletotake therightactionsthatmaximizethetotalreward.The agentmustbalancebetweenexploitingwhathasbeen learnedsofarandcontinuouslyexploringtheenvi‐ronmenttogathermoreinformationthatmayhelpin maximizingthetotalreward.
‐ Agent:Anagenttakesactions.Inourcase,theagent istheproposedfeatureselectionsystem.
‐ Actions istheensembleofallpossiblemovesthe agentcanmake,foroursystem,theactionsarethe nodesthatmaybeusedtocreateabranch.
‐ Environment isthefeaturespacethroughwhich thesystemmoves.Itreceivesthesystem’scurrent stateandactionasinput;then,itreturnsthereward andthenextstateofthesystem.
‐ State isthecurrentsituationwheretheagent inds itself.Inourcontext,thisisthecurrentnodeofthe branch.
Asthereinforcementconceptsaretransparently tackledandhighlighted,thefollowingstepsmay unfoldindepthwiththeconstitutivemainstayorthe technicalinfrastructureofourproposedalgorithm.
Thefeatureselectionsystem(agent)scrutinizes theenvironment,andthenstartswithasinglenode arbitrarilywithoutanypre‐stockpiledknowledge (explorationphase),whichbranchesintopossibleout‐comes.Eachofthoseoutcomesleadstothenextnodes (action).Toindicatehoweffectivethechosenaction is,adifferencebetweentwoconsecutivestatesispro‐duced.Sincethedepthisnotyetreached,thesystem keepsaddingonenodeatatimeinordertocreate abranch.Asiterationstakeplace,thesystemassem‐blesexperiencesandbecomesabletotakeactions thatmaximizetheoverallrewardR.Asayieldedoff‐spring,branchesofhighqualityarecreated.Atran‐sitionsimilaritymeasureisproposedtoestablisha balancedequipoisebetweenexploitingwhathasbeen learnedsofartochoosethenextactionthatmaximizes rewards,andcontinuouslyexploringthefeaturespace toachievelong‐termbene its.Thewayweconstruct thebranchisthesameasthedecisiontree(c4.5),the differenceiswhenweaddanodetothebranch,we retainonlythebestbranchwiththehighestthresh‐old.Thefollowingstepsgivemorepreciseinformation aboutcreatingabranch.
3.2.StepstoCreateaDTBranch
Westartwitharandomfeatureastherootofthe branch.Aslongasthebranchdidnotreachthedesired depthorminsampleleafyet,thesystemkeepsadding tothebranchonenodeatatime.Theaddednodeisthe oneweobtainedusingthefeatureanditsthreshold thatproducesthehighestAUCscore(AreaUnderthe CurveROC).Theideabehindusingdepthandmin simpleleafparametersasstoppingcriteriaistoavoid asmuchaspossibletheover‐ ittingproblem.Themost commonstoppingmethodisminsampleleaf,whichis theminimumnumberofsamplesassignedtoeachleaf node.Ifthenumberislessthanagivenvalue,thenno furthersplitcanbedone,andthenodeisconsidereda inalleafnode.Besides,thedepthofthebranchisvery usefulincontrollingover‐ ittingbecausethedeeper thebranchis,themoreinformationcapturedbythe dataandmorethesplitsithas,whichleadstopredict wellonthetrainingdata.However,itfailstogeneralize ontheunseendata.
3.3.RewardFunction
ArewardfunctionR[38]isusedtocalculatethe scoreateachlevelofthebranchbycomputingthe differencebetweenthescoreofthecurrentbranch anditsscoreafteranewnodeisadded(DS).TheDS indicateshowusefulthenewlyaddedfeatureis.
Algorithm1:CreateaDTbranch
1: Createtherootnodeandchoosethesplitfeature.Choosethe irstfeaturerandomly.
2: Computethebestthresholdofthechosenfeature.
3: Splitthedataonthisfeatureintosubsetsinordertode inethenode.
4: ComputetheAUCscoreonleftandonrightofthenode,then,wekeepthebranchwiththebestAUCscore.
5: Addthechildrennodetorootnode.
6: Choosethenextbestfeature.
7: RepeatfromSTEP2toSTEP5untilthedesireddepthorminsampleleafofthebranchisreached.
Thisfunctionisde inedasfollows: (�������������� −��������������������)×log (‖��������������������������‖) (1)
Where�������������� and�������������������� isthescoreofthe currentbranchandthescoreafteraddinganewnode, �������������������������� isthelengthofsamplesusedtosplitan internalnode.
3.4.TransitionSimilarityMeasure
Definition(Transition)
Atransitionistheprocessinwhichsomething changesfromonestatetoanother.Inoursystem,the transitionisthelinkbetweentwosuccessivenodesof thesamebranch.
TransitionSimilarityMeasure
Weproposedatransitionsimilaritymeasure (TSM)toensurethatoursystemkeepsexploringthe statespace,learningnewrules,andpreventingthe redundantbranches.Foreachbranch,westockall transitionswiththecorrespondingsamplesusedto spliteachinternalnode.Sincethealgorithmisiter‐ative,differentbranchesmaysharethesametransi‐tions,whichisnotaproblem.Inthecasewhenthe majorityofthesamples(higherthanagiventhresh‐old)areequallyusedbythosetransitionsofdifferent branches,thosetwotransitionsaredeemedsimilar, whichisahugeproblem.Allowingsimilartransitions tobeindifferentbranchescanleadtoconstructing redundantanduselessbranches.
Therefore,thesystemkeepslearningthesame rulesandbranches.Thismeansthatthesystemwill beexpensiveintermsofexecutiontime,whilethe systemshouldbelessresourcesconsuming(runtime andstoragerequirement),andthebranchesshouldbe stronganddiverse.
Thesimilaritybetweentwotransitionsiscom‐putedbythefollowingformula:
������= |��1∩��2| ‖��������������������������‖ (2)
Where‖��1∩��2‖isthenumberofsharedsamples betweentwotransitions.
3.5.TheProposedFSmethod
Sincetheproposedalgorithmisiterative,thenum‐berofiterationNisgivenastheinput.Thereward functionissettozeroatthebeginning.Oursystem startswithanemptysetF,andateachiteration,the systemcreatesanewbranchandaddsittoF.Ifthe nextsubset(branch)isalreadyexperiencedbythe system(seenbythesystem),thesystemusesthis gatheredexperiencesintheupcomingiterations.Oth‐erwise,thesystemkeepsexploringnewrules,new patterns,andnewbranches.
3.6.StartingExample
Toexplaintheproposedalgorithmfurther,wesug‐gestthefollowingexample.Wesupposethatwehave adatasetof10features.The igurebellow(Fig. 4) containsthewholespaceoffeatures.Thepurposeis
toselectthebestsubsetoffeaturesusingtheproposed system.
1) Firstiteration 4(b):Thesystemtraversesthefea‐turesspaceandcreatesthe irstbranchwithout anypriorknowledge.Ateachlevelofthebranch, thesystemstorestheAUCscoreusingthereward functionR.Moreover,itstoreseachtransition(2← 3, 3←9, 9← 5, 5←6)anditscorresponding subsetofsamples.
2) Theseconditeration4(c):Aswecanseeinthesec‐onditeration,thetransition(3←9)appearedfor thesecondtime.HeretheTSM(transitionsimilar‐itymeasure)shouldbeinvolved.Iftwotransitions ofdifferentbranchesaresimilar(nodeswithgreen color),thesystemshouldnotallowthemtobein thenextbranches(thecurrentbranchincluded). Thesystemhastoexplorethestate’senvironment to indnewrulestopreventtheredundancyin creatingbranches.
3) TheNiteration4(d):AfterNiterations,thesystem iscapableofidentifyingthebestbranchesusing thegatheredexperiencesduringeachiteration. Thetoprankedbranchesconstructedusingthe systemaretheillustratedinthesub igure4(d).
FromtheaboveFigure 4,itisclearthatthe topsubsetoffeaturesis[3, 5, 10],becausethose featuresareinvolvedthemostincreatingthebest branches.
Thisexperimentalsectionatteststotheef iciency oftheproposedfeedbackfeatureselectionsystem (FBS)inselectingthebestfeatures.Twobenchmarks havebeenconducted,andthenthepro itableservice‐abilityofoursystemisappraisedbycomparingit withtwofeatureselectionalgorithms.The irstone isthepopularwrapperalgorithmnamedRecursive FeatureEliminationRFE(RFE‐RF).Thesecondoneis thepairwisefeatureselectionalgorithm(FS‐P),which isrecentlyproposedandproveditseffectivenessin identifyingthebestfeatures[31].
Inthispaper,ninebinaryclassi icationdatasets havebeenemployedindifferentexperimentaldesign aimingtoevaluatetheperformanceoftheproposed featureselectionmethod.
Thedatasetsarechosentobedifferentintermsof classdistribution(balancedorimbalanced),linearity, datasetshift,numberofinstancesandvariables.The datasets,whicharepubliclyavailable,arecollected anddownloadedfromUCIrepositoryandkaggleplat‐form[39].Anoverviewofthemaincharacteristicsof eachdatasetisillustrativelytabulatedinTable1
Twoexperimentalendeavorsareundertakento estimatetheworkableprospectsandtheconsequen‐tialrami icationsofourproposedsystem.Initially,we
(a) Dataset:creditcard
(d) Dataset:Eye
(g) Dataset:Caravan
(b) Dataset:sonar
(e) Dataset:musk
(h) Dataset:Numerai
willempiricallyembodytheapplicationsofthepro‐posedalgorithmsbasedonthedatasetsdisplayedin Table 1 intermsofAreaUndertheRocCurve(AUC) whereFBSiscomparedwiththepairwisemethod, namelyFS‐PandwithRFE.
Incorrelativeparallelismwiththepreviousstep, thesubsequentstagewilldemonstratetheeligible capabilityoftheFBSsysteminencirclingthepractical subsetasswiftlyaspossiblethroughtheexclusive employmentofthefewfeaturessupplementedbysec‐ondbenchmarking.
Alldatasetsaresegmentedintotwosubsets; onesubsetisemployedfortrainingandtestingthe branchesusingcross‐validationwith3‐foldswhilethe othersubsetisquarantinedandcastaside(holdout set)andtheperformanceofthe inalselectedfeature subsetisevaluatedonit.Forthesakeofafaircom‐parison,the inalselectedsubsetusingFBS,FS‐P,and RFEisevaluatedusingaRandomForestwithagrid searchstrategyforthehyper‐parameters.TheAUC scoreiscalculatedusingtheoutofbag(OOB)scoreof therandomforestclassi ier.Sincethebenchmarking datasetsusedinthispapertoevaluatetheproposed systemareunbalanced,theAUCmetricisconsidered thebestchoice.Moreover,theAUCmetricgenerally canbeviewedasabettermeasurethanaccuracy[40].
(c) Dataset:spambase
(f) Dataset:SPECT
(i) Dataset:Ionosphere
TheFeedbacksystemparametersincorporatea systematictrilogyofchangeableparameterswhich areinadynamicalterationinaccordancewitheach dataset.
‐ Sisthesimilarityvalue.
‐ Disconcernedwiththeindicationofthebranches’ depth.
‐ Nre lectsthenumberofiterations.
Toexemplifytheprobablechangeabilityofthese parameters.Datasetswithlargesize,theNandD valuesshouldbehighersincethebestbranches,in thiscase,shouldbedeeper.Thefollowingtablesup‐plementsapanoramicoverviewunderlyingthebest parametersusedforeachdataset.
Asclearlyarticulatedintheaforementionedsec‐tion,thechoiceofparametersisindispensable.The followinggraphdelineatesthein luenceofthedepth parameter(D)onthequalityoftheconstructed branchesusingthesonardataset.Thisgraphicplot displaysasummativesnapshotofthetrainandthetest AUCscoresafterthegraduallyexponentialvariationof Dparameterfrom1to15isful illed.
Therecordedoutcomesonthesonardatasetshow clearlythatthebranchespronetoover‐ itforlarge depthvaluesbecausethebranchesperfectlypredict
allofthetraindata(theblueline).However,theyfail togeneralizeonunseendata(theredline).Ascanbe visuallyobserved,thebestdepthforthesonardataset itselfequalsthree(D=3).
4.4.ConductedExperiments
TheproposedmethodiscomparedtotheRFEand FS‐PapproachsintermsofpredictionAUCscore.In thismanuscript,twoempiricallyconclusiveandthor‐oughgoingexperimentsareconducted.
1) FirstExperiments:Toevaluateourproposed approachFBS,wecomparetheobtainedperfor‐mance(intermsofAUCscore)byFBSwiththe wrappermethod(Recursivefeatureelimination withrandomforestRFE)andwiththepairwise algorithmFS‐P.
2) SecondExperiments:Thisexperimentiscon‐ductedtoshowtheabilityoftheproposedsystem FBSinachievingthemaximumperformanceusing justafewfeatures.Forafaircomparisonbetween FBS,FS‐P,andRFE,we ixthegeneratedsubsetsize forallalgorithmscomparedasfollows:subsetof size5(������5, ����−��5, ������5),asubsetofsize10
(������10,����−��10,������10)andsubsetof15(
4.5.ResultsandDiscussion
Afterselectingthefeaturesubset,thesameclas‐si ier(RF)isessentiallymandatorytocalculatethe AUCscore.TheRandomforestisutilizedtodetermine thetestperformanceforthetop‐rankedfeaturesof eachemployeddataset.Thecomparativejuxtaposi‐tionbetweenFBS,FS‐PandRFEisaccessiblyrepre‐sentedinFigure6(Firstexperiment).
Asstated,ourfeatureselectionalgorithmFBS exceedsandoutstripsFSPandRFEconsiderablyin almostalldatasets,suchasSPECT(Figure6(f)),credit card(Figure 6(a)),ionosphere(Figure 6(i)),musk (Figure 6(e)),caravan(Figure 6(g)),andsonar(Fig‐ure6(b)),exceptforspambasedataset(6(c)).
Forthenumeraidataset(Figure6(h)),ourmethod hasarestrictivelylimited,ifnotdowngradedperfor‐manceatthebeginningcomparedtoRFEandFS‐P.As ourmethoddoesnotselectjustthebest‐rankedfea‐tureasastartingpointtopreventselectingasubop‐timalsubsetbutalsoattempttomaximizetheoverall performanceoftheselectedsubsettakingintoaccount
theinteractionsbetweenfeatures.Aftertheselection ofthenumeraidataset’s ifthfeature(Figure 6(h)), theaforementionedbehavioralveracityisrendered observable,andFBSshowsitsdrasticallyimproved performanceoverFS‐PandRFE.
Table 2 showsthebestparametersusedinour feedbacksystem.Theinsightfulbottom‐lineconclu‐sionwecanexcerptfromthetableisthatthechoiceof thebestparameterstouseineachdatasetiscrucial, whichmeansthattheparametersshouldbecarefully chosentoconstructbrancheswithhighquality.
Thepurposeoftheproposedfeatureselection methodisnotonlytoimprovetheclassi ication performancebutalsotoyieldexcellentperformance usingaminimumnumberoffeatures(selectthe fewestpossiblenumberoffeatures).
Figure 7 showsthenumberofselectedfeatures withthehighestAUCscoreonninebenchmarksdata sets.Asitisillustratedthroughthisbenchmarking, FBSselectstheproperfeaturescomparedwithFS‐P andRFEalmostinalldatasets.Onepointtomention hereisthattheproposedfeedbacksystemcan ind thebestsubsetusingaminimumamountoffeatures, asshowninFigure 7.Thus,theminimumresources requirement,fastexecution,andbettergeneralization.
Inthispaper,wehaveproposedanewfeature selectionmethodbasedonthedecisiontreebranches concepttorepresentfeaturesubsets.Theproposed systemdealswiththeFSproblemasareinforcement learningproblem;thesystemtriesto indacompro‐misebetweenexploringthesearchspacebyexperi‐encingnewrules(creatingnewbranches)andexploit‐ingthegatheredexperiencessoastochoosetheright actions(relevantfeature).Theexploit/exploretrade‐offiscontrolledbytheproposedTSM.Theproposed systemcanconstructthebestbranches,hence,select‐ingthebestsubsetoffeatures.
Toassesstheeffectivenessoftheselectedfeatures usingourproposedmethod,wehaveconductedan extensivesetofexperimentsusingninebenchmark‐ingdatasets.Theresultscon irmthattheproposed feedbackfeatureselectionsystemisnotonlyeffective atselectingthebestperformingsubsetsoffeatures thatproducethebestperformancebutalsochoosethe fewestnumberoffeatures.
YassineAkhiat∗ –DepartmentofInformatics,fac‐ultyofsciencesdharelmahraz,USMBA,FezMorocco, e‐mail:yassine.akhiat@usmba.ac.ma.
AhmedZinedine –DepartmentofInformatics,fac‐ultyofsciencesdharelmahraz,USMBA,FezMorocco, e‐mail:ahmed.zinedine@usmba.ac.ma.
MohamedChahhou –DepartmentofInformatics, facultyofsciences,UAE,TetouanMorocco,e‐mail: mchahhou@hotmail.com.
∗Correspondingauthor
[1] R.Roelofs,S.Fridovich‐Keil,J.Miller,V.Shankar, M.Hardt,B.Recht,andL.Schmidt,“Ameta‐analysisofover ittinginmachinelearning,”in Proceedingsofthe33rdInternationalConference onNeuralInformationProcessingSystems,2019, pp.9179–9189.
[2] X.Ying,“Anoverviewofover ittinganditssolu‐tions,”in JournalofPhysics:ConferenceSeries,vol. 1168,no.2.IOPPublishing,2019,p.022022.
[3] M.Li,H.Wang,L.Yang,Y.Liang,Z.Shang,and H.Wan,“Fasthybriddimensionalityreduction methodforclassi icationbasedonfeatureselec‐tionandgroupedfeatureextraction,” ExpertSystemswithApplications,vol.150,p.113277,2020.
[4] H.Liu,H.Motoda,andL.Yu,“Aselectivesampling approachtoactivefeatureselection,” Arti icial Intelligence,vol.159,no.1‐2,pp.49–74,2004.
[5] Y.Akhiat,Y.Asnaoui,M.Chahhou,andA.Zine‐dine,“Anewgraphfeatureselectionapproach,” in 20206thIEEECongressonInformationScience andTechnology(CiSt).IEEE,2021,pp.156–161.
[6] D.M.Atallah,M.Badawy,andA.El‐Sayed,“Intel‐ligentfeatureselectionwithmodi iedk‐nearest neighborforkidneytransplantationprediction,” SNAppliedSciences,vol.1,no.10,pp.1–17,2019.
[7] I.Guyon,S.Gunn,M.Nikravesh,andL.A.Zadeh, Featureextraction:foundationsandapplications Springer,2008,vol.207.
[8] I.GuyonandA.Elisseeff,“Anintroductiontofea‐tureextraction,”in Featureextraction.Springer, 2006,pp.1–25.
[9] A.Yassine,“Featureselectionmethodsforhigh dimensionaldata,”2021.
[10] Y.Manzali,Y.Akhiat,M.Chahhou,M.Elmohajir, andA.Zinedine,“Reducingthenumberoftrees inaforestusingnoisyfeatures,” EvolvingSystems,pp.1–18,2022.
[11] Y.Akhiat,Y.Manzali,M.Chahhou,andA.Zine‐dine,“Anewnoisyrandomforestbasedmethod forfeatureselection,” CYBERNETICSANDINFORMATIONTECHNOLOGIES,vol.21,no.2,2021.
[12] S.Abe,“Featureselectionandextraction,”in Supportvectormachinesforpatternclassi ication Springer,2010,pp.331–341.
[13] J.Cai,J.Luo,S.Wang,andS.Yang,“Featureselec‐tioninmachinelearning:Anewperspective,” Neurocomputing,vol.300,pp.70–79,2018.
[14] Y.Akhiat,M.Chahhou,andA.Zinedine,“Fea‐tureselectionbasedongraphrepresentation,”in 2018IEEE5thInternationalCongressonInformationScienceandTechnology(CiSt).IEEE,2018, pp.232–237.
[15] J.C.Ang,A.Mirzal,H.Haron,andH.N.A.Hamed, “Supervised,unsupervised,andsemi‐supervised featureselection:areviewongeneselection,” IEEE/ACMtransactionsoncomputationalbiology
andbioinformatics,vol.13,no.5,pp.971–989, 2015.
[16] L.A.BelancheandF.F.González,“Reviewand evaluationoffeatureselectionalgorithmsinsyn‐theticproblems,” arXivpreprintarXiv:1101.2320, 2011.
[17] G.ChandrashekarandF.Sahin,“Asurveyonfea‐tureselectionmethods,” Computers&Electrical Engineering,vol.40,no.1,pp.16–28,2014.
[18] B.NithyaandV.Ilango,“Evaluationofmachine learningbasedoptimizedfeatureselection approachesandclassi icationmethodsfor cervicalcancerprediction,” SNAppliedSciences, vol.1,no.6,pp.1–16,2019.
[19] A.Bommert,X.Sun,B.Bischl,J.Rahnenführer, andM.Lang,“Benchmarkfor iltermethodsfor featureselectioninhigh‐dimensionalclassi ica‐tiondata,” ComputationalStatistics&DataAnalysis,vol.143,p.106839,2020.
[20] Y.Akhiat,M.Chahhou,andA.Zinedine,“Ensem‐blefeatureselectionalgorithm,” International JournalofIntelligentSystemsandApplications, vol.11,no.1,p.24,2019.
[21] L.ČehovinandZ.Bosnić,“Empiricalevaluation offeatureselectionmethodsinclassi ication,” Intelligentdataanalysis,vol.14,no.3,pp.265–281,2010.
[22] Y.Asnaoui,Y.Akhiat,andA.Zinedine,“Fea‐tureselectionbasedonattributesclustering,”in 2021FifthInternationalConferenceOnIntelligent ComputinginDataSciences(ICDS).IEEE,2021, pp.1–5.
[23] Y.Bouchlaghem,Y.Akhiat,andS.Amjad,“Feature selection:Areviewandcomparativestudy,”in E3SWebofConferences,vol.351.EDPSciences, 2022,p.01046.
[24] A.Destrero,S.Mosci,C.D.Mol,A.Verri, andF.Odone,“Featureselectionforhigh‐dimensionaldata,” ComputationalManagement Science,vol.6,pp.25–40,2009.
[25] V.FontiandE.Belitser,“Featureselectionusing lasso,” VUAmsterdamResearchPaperinBusiness Analytics,vol.30,pp.1–25,2017.
[26] I.GuyonandA.Elisseeff,“Anintroductiontovari‐ableandfeatureselection,” Journalofmachine learningresearch,vol.3,no.Mar,pp.1157–1182, 2003.
[27] R.Zebari,A.Abdulazeez,D.Zeebaree,D.Zebari, andJ.Saeed,“Acomprehensivereviewofdimen‐sionalityreductiontechniquesforfeatureselec‐tionandfeatureextraction,” JournalofApplied ScienceandTechnologyTrends,vol.1,no.2,pp. 56–70,2020.
[28] J.MiaoandL.Niu,“Asurveyonfeatureselection,” ProcediaComputerScience,vol.91,pp.919–926, 2016.
[29] L.C.Molina,L.Belanche,andÀ.Nebot,“Feature selectionalgorithms:Asurveyandexperimental evaluation,”in 2002IEEEInternationalConferenceonDataMining,2002.Proceedings. IEEE, 2002,pp.306–313.
[30] R.Caruana,A.Niculescu‐Mizil,G.Crew,and A.Ksikes,“Ensembleselectionfromlibrariesof models,”in Proceedingsofthetwenty- irstinternationalconferenceonMachinelearning,2004, p.18.
[31] A.Yassine,C.Mohamed,andA.Zinedine,“Fea‐tureselectionbasedonpairwiseevalution,”in 2017IntelligentSystemsandComputerVision (ISCV).IEEE,2017,pp.1–6.
[32] B.Gregorutti,B.Michel,andP.Saint‐Pierre, “Correlationandvariableimportanceinrandom forests,” StatisticsandComputing,vol.27,no.3, pp.659–678,2017.
[33] J.Kacprzyk,J.W.Owsinski,andD.A.Viattchenin, “Anewheuristicpossibilisticclusteringalgo‐rithmforfeatureselection,” JournalofAutomationMobileRoboticsandIntelligentSystems, vol.8,2014.
[34] L.Breiman,“Randomforests,” Machinelearning, vol.45,no.1,pp.5–32,2001.
[35] H.Han,X.Guo,andH.Yu,“Variableselec‐tionusingmeandecreaseaccuracyandmean decreaseginibasedonrandomforest,”in 2016 7thieeeinternationalconferenceonsoftware engineeringandservicescience(icsess).IEEE, 2016,pp.219–224.
[36] R.SuttonandA.Barto,“Reinforcementlearn‐ing:Anintroduction.2017.ucl,” ComputerScienceDepartment,ReinforcementLearningLectures,2018.
[37] Y.FenjiroandH.Benbrahim,“Deepreinforce‐mentlearningoverviewofthestateoftheart.” JournalofAutomation,MobileRoboticsandIntelligentSystems,pp.20–39,2018.
[38] S.M.H.Fard,A.Hamzeh,andS.Hashemi,“Using reinforcementlearningto indanoptimalsetof features,” Computers&MathematicswithApplications,vol.66,no.10,pp.1892–1904,2013.
[39] M.Lichman,“Ucimachinelearningrepository [http://archive.ics.uci.edu/ml].irvine,ca:Uni‐versityofcalifornia,schoolofinformationand computerscience,” URL:http://archive.ics.uci. edu/ml,2013.
[40] F.F.Provost,“T.,andkohavi,r.thecaseagainst accuracyestimationforcomparingclassi iers,” in ProceedingsoftheFifteenthInternationalConferenceonMachineLearning,1998.
Abstract:
Submitted:18th July2023;accepted:20th October2023
YaminiVijaywargiya,MahakMishra,NitikaVatsDoohan
DOI:10.14313/JAMRIS/1‐2024/7
WeproposeaComputerVisionandMachineLearning equippedmodelthatsecurestheATMfromfraudulent activitiesbyleveragingtheuseofHaarcascade(HRC) andLocalBinaryPatternHistogram(LBPH)classifierfor facedetectionandrecognitioncorrespondingly,whichin turndetectfraudbyutilizingfeatures,likePINandface recognition,helptoidentifyandauthenticatetheuserby checkingwiththetraineddatasetandtriggerreal‐time alertmailiftheuserturnsouttobeunauthorizedalso. Itdoesnotallowthemtologinintothemachine,which resolvestheATMsecurityissue.thissystemisevaluated onthedatasetofreal‐worldATMcamerafeeds,which showsanaccuracyof90%.Itcaneffectivelydetectmany frauds,includingidentitytheftandunauthorizedaccess whichmakesitevenmorereliable.
Keywords: ATM,Computervision,PIN,HRC,LBPHrecog‐nizer,Facedetection,Facerecognition,Frauddetection, SMTPmodule
1.Introduction
AnAutomatedTellerMachine(ATM)isanelec‐tronictelecommunicationdeviceinventedinearly 1970s,whichareoneoftheoldestandmostsecure machineryusedtodate,butfornearly30years,noth‐inghasbeendonetoimprovethissystem’ssecurity, andduetotheamelioration&globaldigitalization,it isevenmorevulnerabletotheftsandfrauds,which leadtoamassivelossofcapitaloftheusersandtheir banks.Thismachineenablescustomerstowithdraw cashfromtheirbankaccountswithouthavingdirect contactwiththebankstaffandhavebecomeapopu‐larmodeoftransactionfor inancialclients,including cashwithdrawals,deposits,andothertransactions. Banksarebecomingincreasinglyconcernedaboutthe securityofATMsduetotheincreaseincasesoffraud andmoneylossattheATMs.
Therapidameliorationoftechnologyandglobal digitalizationhaveledtonewandmoresecureATM models,asnewthreatsalsoemergedaybydaythat couldunderminetheirsecurity.Despitetheadvan‐tagesofautomation,ATMsystemsexpose inancial institutionstofraud.
ThecurrentATMmodelsuseacardandaPIN code,whichmaketheminclinedtosuchattacksas astolencard,staticPINs,cardfraud,andhackingof PINs.Fraudstersusenumeroustechniquestoextract sensitiveinformationfromATMusers,includingskim‐mingdevicesandfakekeypads.Theseincidentsnot onlyresultinsigni icant inanciallossesbutalsocause harmtothereputationofthebankingindustry.
OnewaytoincreasethesecurityofanAutomatic TellerMachineisbyprovidingPersonalIdenti ication Number(PIN),facedetection,andfacerecognition. FacedetectionalgorithmslikeHaarcascade(HRC) andforfacerecognitionLBPH(LocalBinaryPattern Histogram)canhelpidentifyindividualsattemptingto conductfraudulenttransactionsatATMs.
HRCarehighlyaccurate,fastspeed,andcandetect facesinreal‐timevideo/images,andontheother hand,LBPHusesmicro‐patterns,whichdescribethe looksandkeepexecutiontimeshort.
ByusingcamerasinstalledatATMstocapturethe facesofusers,theHaarcascadealgorithmcanquickly identifytheuserbymatchingthepinoftheregistered personwiththeirface,andiftheuserisunauthorized, itdoesnotallowthemtologintotheAutomaticTeller MachineandsendoutanalertemailtotheAuthorized useremailID.Thistechnologycanalertbankof icials ofsuspiciousactivity,allowingthemtotakeprompt actionandpreventfraud.
In2012,HosseinRezaBabaei,OfentseMolala‐pataandAbdulHayAkbarPandoretal.,[5]devel‐opedasystemusingBiometricsFacialRecognition methodtoincreasethesecurityoftheAutomatic tellerMachines.Inthisstudy,theybuiltthesystem usingRapidApplicationDevelopmentlifecycle,which makesitahighqualitysystem.
In2015,MohsinKarovaliyaa,SaifaliKarediab, SharadOzac,Dr.D.R.Kalbandedetal.,[8]Introduced anewconceptofrandomlygeneratingOTPthatfrees theuserfromrememberingthePINsduringtransac‐tionatAutomaticTellermachine(ATM),andfeatures likefacerecognitionareusedwithit,makingthesys‐temmoreconvenientandusable.Thisresearchstudy utilizesPCAbasedfacerecognitiontechnique.
In2018,T.S.VishnuPriya,G.VinithaSanchez, N.R.Raajanetal.,[7]cameupwithlocalbinarypattern algorithmforfacerecognition(FR)inordertoful il thedownsideofnotidentifyingtheidenticaltwinsin BiometricFRmethod.Inthisstudytheyexplainhow thelocalbinarypatternswereusedtoidentifytheface inidenticalsituationsbecausetheLBPmethodcan describeappropriatelythemicropatternspresentin theface.
AsS.Hazraetal.[11]proposed,anATMisanelec‐tronicdevicethatallowsbankingtransactionswithout staffinteraction.AuniqueIDcardwithaPINisneeded touseit.AproposedSmartATMserviceusesIoTand ComputerVision‐basedtechnologywith ingerprint, face,andOTPveri icationstoenhancesecurityand reducefraudrisk.
In2020,M.S.Minu,KshitijArun,AnmolTiwari, PriyanshRampuriaetal.[6]proposedanideaabout howhomesecuritycanbeimprovedbyleveraging MachineLearningalgorithmsforfacedetectionand recognitionusingHaarcascadeclassi ier.Inthis,they explainedcompletesystem lowonhowtheModules areworkingintheprojectandtellhowImageIden‐ti icationandRecognitionisbeingdone.TheKNN algorithmisusedtocomparethefeaturesfromthe imagedatabaseafterfeatureextractionfromthesam‐pleimage.
In2020,Dr.SSasipriya,Dr.P.MayilVelKumar,S. Shenbagadevietal.[9]proposethatthefacialrecog‐nitionsystemshouldreplaceATMcardswithanRFID tag.Thecapturedfaceimageofapersoniscompared withthedatabasestoredimageafterwhichtheoutput resultissenttocontrolunitthroughserialcommuni‐cation.Ifthepersonisunauthorized,analertmessage issenttotheauthorizeduser.ThisstudyutilizesHaar cascadeandLocalbinarypatternAlgorithm.
In2021,AnirudhaBShetty,Bhoomika,Deeksha, JeevanRebeiro,Ramyashree,etal.[13]comparedtwo facerecognitionalgorithms:HaarCascadeandLocal BinaryPatternfortheclassi icationoffacesinan image.TheyconcludedthataccuracyofHaarCascade Algorithmisgreater,butitsexecutiontimeisalso higherthanlocalbinarypattern.
In2022,J.Ferdinand,C.Wijaya,A.N.Ronal,I.S. Edbert,andD.Suhartonoetal.[4]proposedaface
Table1. Inputparameters
recognitionsystemusingFaceNetcombinedwiththe HaarCascadeClassi ier.Inthissystem,customers inserttheircard,anditwilldetectandstarttoidentify theirface.Ifitdoesnotmatch,thecardwillbeblocked. Thisproposedsystemachievesaccuracyof90.93%.
3.DatasetDescription
Inthisstudy,themodelregisterspeoplebytaking inputasName,Pin,andEmailID,andcapturesand storestheirfaceimagesfortrainingpurposesinaCSV File.
4.ProposedSystem CheckCamera
Thecheckcameramoduleisavitalcomponent ofanyfacialrecognitionsystemthatemployscam‐eratechnology.Itswholepurposeistoensurethat thecamerasarefunctioningproperlyandthatthe imagesitcapturesaresuitableforfacialrecognition,
whichneedsgoodqualityresolution.Thehigherthe cameraresolutions,thebetterthequalityofthe images,whichcanimprovetheaccuracyofthewhole system.Therefore,itisnecessarytoensurethatthe camerainstalledattheATMcancapturetheimage inthedesiredresolutionforoptimalperformanceof thesystem.Anothercrucialfactortocheckduringthis moduleisthecamera’spositioning.Ideally,thecamera shouldcapturetheentirefaceofthepersonstanding infrontoftheATMtoensurethatthefacialrecognition systemcanaccessallthenecessaryfacialfeaturesfor accurateidenti ication.
ThismoduleutilizestheHaarcascademachine learningalgorithmfromtheOpenCVmodule,as explainedin[3].Thiscomponentcapturesimagesof individualsstandinginfrontofanATMandprocesses themtodetectthepresenceandlocationoftheirfaces usingtheHaarcascadealgorithm.Duringthisprocess, theuserispromptedtoprovidetheirname,emailID, andPINtoregisterasanewcustomer.Thesedetails arestoredinaCSV ile(Fig. 2).Aftersubmittingthe details,thecameraisactivated,displayingtheuser’s faceinarectangularframe(Fig. 4(i)).Thecamera capturesover100imagesofthepersonandstoresthe resizedimagesfortrainingpurposes.Alltheseimages werestoredinafolderwiththename,ID,andlabelin theJPGformat(Fig.4(ii)).
Tocompletethetask,wefollowaseriesofsteps. Initially,weloadthecascade,whichwillactasa facedetector.Subsequently,weextractthefaces andtheircorrespondingIDsfromtheimages.then itproceedstotrainthefaceimagestogetherwith theirrespectiveIDsusingtheLBPH(LocalBinary PatternsHistogram)recognizerfunction(Figs. 4(i), 4(ii), 5).Thesefunctionswereimplementedthrough the‘cv2.face_LBPHFaceRecognizer.create()’method. Moreover,weemploythe‘Thread()’functionfrom thethreadingmoduletocreateaseparatethread speci icallyforthetrainingprocess.Finally,westore theobtainedembeddings,orfacialfeatures,fromthe traininginaYAML ileforfurtherstepofrecognizing (Fig.5).
Inthismodule,weanalyzeadatasetconsisting ofregisteredaswellasnon‐registeredfacestothe account.Todetectfacesaccurately,weutilizeahaar cascadeclassi ier.Subsequently,theLBPHrecognizer functionwasusedtoidentifyandauthenticatethe detectedfacesbyusingthetrainedembeddingsstored inaYAML ileearlier.therecognitionprocesscom‐prisesvariousscenarios.Asuccessfulmatchbetween aregisteredaccountandthedetectedfaceiscon‐sideredaTruePositiveoutcome,signifyingavalid veri ication.
Conversely,ifaregisteredaccountfailstomatch thedetectedface,itfallsintothecategoryofFalse Negative,indicatinganinconsistency.
Also,whenthemodelrecognizesthefaceofan unregisteredaccount,that’sthecaseofFalsePositive, representinganincorrectidenti ication.Lastly,when themodelfailstorecognizeafacethatisnotlinkedto anyregisteredaccount,itfallsunderthecategoryof TrueNegative,accuratelyindicatingtheabsenceofa linkedaccount.
Uponcompletingrecognition,thesystemallows theusertoproceedwiththetransactionifauthorized. However,ifitdetectsfraud,thesystemsendsanalert emailusingthesmtplibmodule,fetchingentriesfrom theCSV ile(Fig.2)oftheauthorizedaccountholder andstoppingthelogintotheATMmachine(Fig.10).
Withthisinformation,thebankandaccount holdercantakenecessarymeasurestopreventthe transaction,thuspreventinglossofcapitalandmaking thesystemmoresecure.
5.1.HaarCascadeClassifier(HRC)
TheHaarCascade,originallyproposedbyPaul ViolaandMichaelJonesetal.in2001[1],isawidely usedobjectdetectionalgorithmspeci icallydesigned foridentifyingfacesinimagesandvideos.
ItemploysHaarfeatures(Fig.6),whichconsistof whiteandblackpixelsrepresentingdifferentregions ofthefacebasedonbrightness(Fig.7).Todetectfaces, thealgorithmslidesawindowof ixedsizeacrossthe imageatvariousscales.
Ateachposition,itcomputes iverectangularfea‐turesbycomparingthesumofblackandwhiteregion pixels.Iftherearesigni icantvariationsinpixelinten‐sitiesorfeatures,thealgorithmidenti iestheregion asaface;otherwise,itisanon‐faceregion.Train‐ingtheHaarCascademodelinvolveslargenumberof positiveimagescontainingfacesandnegativeimages withoutfaces.Themodeliscomposedofmultiple stages,eachcomprisingasetofweakclassi iers. Theseclassi iersaretrainedusingAdaptiveBoosting, whichselectsthemosteffectivefeaturesfordistin‐guishingbetweenpositiveandnegativeobjects.Pre‐trainedHaarCascadeclassi iermodels,suchas“haar‐cascade_frontalface_default.xml”areavailableinXML formatontheOpenCVGitHubrepository.Byloading thesepre‐trainedclassi iers,real‐timefacedetection canbeperformedwithouttheneedforcustomtrain‐ingorparameteradjustment.
ToapplythisAlgorithm,weutilizedPythonand OpenCV[3]function“cv2.CascadeClassi ier(),”which loadscascadesasinput,andtodetectfaces“detect‐MultiScale()”functionwasused,whichparameters include.
Scalefactorparameterisutilizedtodecreasethe imagesize.Asmallerscalefactorcanresultinfaster detection,butsmallerfacesmaybemissed.However, amoresigni icantscalefactormayleadtoslower detectionbutcandetectsmallerfaces.So,ascalefactor of1.3isused.
Theminimumneighborsparameterspeci iesthe numberofneighborsaregionshouldhave.Increasing thisparameterwilldecreasefalsepositivesbutmay alsomisssomefaces.Therefore,avalueof5isused. minimumsizeparameter(30,30)speci iesthe minimumfacesizethatcanbedetected.Increasing thisparametercanboostthedetectionprocessspeed, butsmallerfacesmaybemissed.
The lagsparameterisusedtoenableordisable certainfeaturesofthedetector,suchasscalingthe imagewiththesameaspectratioasthedetector oroptimizingthedetectorforspeed,soweused “cv2.CASCADE_SCALE_IMAGE.”
5.2.LocalBinaryPatternHistogram(LBPH)
TheLocalBinaryPattern(LBP)isawell‐establishedvisualrepresentationwidelyemployed incomputervisionproposedin[10, 12]andis speci icallydesignedfortexturecategorization.It isavariationderivedfromtheTextureSpectrum modelproposedin1990andhasgainedsubstantial recognition.
Initiallyintroducedin1994,theLBPtechnique servesasarobustfeaturefortextureanalysis.It operatesbyapplyingtheLBPoperatortoexamine individualimagesascollectionsofmicro‐patterns. Thefrequencyofoccurrenceofthesemicro‐patterns throughouttheimageisthencapturedinahistogram ofLBPvalues.Toconstructthefeaturevector,theface imageisdividedintonon‐overlappingregions(R0, R1,…,Rm).
IntheoriginalLBPmethod,pixelsarelabeledby comparingtheircentralpixelvalue(threshold)with thevaluesoftheir 3×3 neighborhood(Fig. 8).This comparisonassignsdistinctnumericalvaluestocom‐monfeaturessuchasedges,lines,andpoints[2].Dur‐ingtherecognitionofatestface,thealgorithmcalcu‐latestheLBPofthetestface,dividesitintoregions, andcreatesahistogramforeachregion.Thesehis‐togramsarethenconcatenatedintoonehistogram (Fig.9)representingtheentireimage.Thenalgocom‐parestheEuclideandistancebetweenthehistogramof thetestfaceandthehistogramsofthetrainedfaces.If thedistancefallsbelowaprede inedtolerancevalue, itisconsideredamatch.Thisapproachenablesef i‐cientandrobustfacerecognitionbyusingthespatial informationcapturedbytheLBPoperatorandthe histogramrepresentation.
IntheOpenCVlibrary,thefunction “cv2.face_LBPHFaceRecognizer.create()”isemployed fortheLBPHalgorithm.Thisfunctionalsofacilitates readingtheYAML ilecontainingrelevantdata.The “predict()”methodisutilizedtopredictthelabeland con idencevalueofanewfaceinatestimage.
TheThreadingModuleinPythonisusedtocreate andmanagethreadsinaprogram.Itallowsmulti‐plethreadstorunconcurrentlywithinasinglepro‐cess,improvingtheperformanceandresponsiveness oftheprogram.Inthecontextofourproject,itis usedforimagetraining.Itcanalsobeusedtospeed
upthetrainingprocessbyallowingmultipleimages tobeprocessedsimultaneously.Thiscansigni icantly reducethetimerequiredfortraining.
Parametersinthemoduleusedinthesystem.
Target:IttakesanarrayofFacesandIDsfor training.
5.4.Smtplib
ThesmtplibmoduleinPythonprovidesawayto sendemailsusingSMTP(SimpleMailTransferPro‐tocol).ItallowsyoutoconnecttoanSMTPserver, authenticatewithausernameandpassword,and sendemailstooneormorerecipientsbyusingthis “server.sendmail(sender_email,receiver_email,mes‐sage)”functionofthemodule.
Withthis,youcansendtextorHTMLmessages, addattachments,andsetvariousemailheaders,such asthesubject,sender,andrecipient.Youcanalsouseit tohandleerrorsandexceptionsthatmayoccurduring theemail‐sendingprocess.
Wecanimplementthissysteminthe ieldbylever‐agingcloudserversofbanksthatstorethedataof theregisteredperson.Bydoingthis,theATMmachine doesnothavetostorethedataoftensofmillionsof customers,andinfact,itcanaccessthisinfoautomat‐icallybygeneratingtheAPIrequesttothoseservers, whichgivestheaccesstousedataoftheindividual foritsrecognitionsystemalsoverifywhetherthecus‐tomerislegitornotandthiswholeprocesswillbe completedwithin iveseconds.
Inthisstudy,theexecutionisperformedonareal‐timedatasetbyusingtheHaarcascadeforfacedetec‐tionandLBPHforfacerecognition.Asanoutcome,we foundoutthatthismethoddepictsadesirableresult forthevariousmeasuresandthusleadstothehigher ef iciencyofoursystem.
FortheAccuracycalculation, Case1– TruePositive(TP):Theaccountisreg‐istered,andtheModelmatchesthefaceoftheperson correctly.
Case2– FalseNegative(FN):Theaccountisreg‐istered,butthefacedoesnotmatch.
Case3– FalsePositive(FP):Theaccountisnot linkedyetthemodelstillmatchestheface.
Case4– TrueNegative(TN):Theaccountisnot linked,andthemodelalsodoesnotrecognizetheface oftheperson.
Accuracy=(TP+TN)/(TP+TN+FP+FN) (1)
Were,TP:TruePositive,TN:TrueNegative, FP:FalsePositive,FN:FalseNegative
ByusingEquation(1),theAccuracyobtainedfrom oursystemis90%.
ForPrecision,
Precision=TP/(TP+FP) (2)
ByusingEquation(2),thePrecisionobtainedis 0.933.
ForRecall,
Recall=TP/(TP+FN) (3)
ByusingEquation(3),theRecallwasobtainedas 0.89.
ForF1Score,
F1=(2∗Precision∗Recall)/(Precision+Recall) (4)
ByusingEquation(4),theF1scorewasobtained as0.91.
7.Conclusion
Inthisstudy,weproposeamachinelearning modelthatcanaccuratelydetectandprovidesecurity towardsanywrongfulintentionsofAutomaticteller machineFraudandthemoneywithinit.Itcanidentify andissuereal‐timealerts/warningmessagesifaper‐son’sfacedoesnotmatchtheauthorizedpost’sactual faceandstate,asthiscouldraisesuspicion.
Basedonthesemessages,necessaryactionscanbe takenimmediatelytopreventsigni icantproblemsin thefuture.
Thus,withthehelpofalgorithmslikeHaarCas‐cadeandLBPH(LocalBinaryPatternHistogram),a modelisdevelopedthatcanissuewarningsandalerts toauthoritiesbeforeanyunauthorizedtransactions occur.Thismodelresultsinanaccuracyof90percent withlowerfalsepositiverates,whichmakesitmore secure&trustworthy.
Facialrecognitioniswidelyrecognizedasoneof themostsecurebiometricsystems,especiallygood forhigh‐levelsecuritypurposeslikepreventingany wrongfulintentionforthemoneyofanyaccount holderandprovidingsecurityforATMs.
YaminiVijaywargiya∗ –Medi‐capsUniversity, Indore,MadhyaPradesh,India,e‐mail: yaminivijaywargiya2001@gmail.com.
MahakMishra –Medi‐capsUniversity,Indore,Mad‐hyaPradesh,India,e‐mail:missmahak.j@gmail.com.
NitikaVatsDoohan –Medi‐capsUniversity, Indore,MadhyaPradesh,India,e‐mail: nitika.doohan@gmail.com.
∗Correspondingauthor
References
[1] P.ViolaandM.Jones,“RapidObjectDetection UsingaBoostedCascadeofSimpleFeatures,” Proc.IEEEComp.Soc.Conf.USA,December2001, vol.1,p.1,doi:10.1109/CVPR.2001.990517.
[2] R.J.Rasras,etal.,“DevelopingDigitalSignalClus‐teringMethodUsingLocalBinaryPatternHis‐togram,” InternationalJournalofElectricaland ComputerEngineering(IJECE),vol.11,no.1, 2021,pp.872–878.doi:10.11591/ijece.v11i1.
[3] G.BradskiandA.Kaehler,“LearningOpenCV: ComputervisionwiththeOpenCVlibrary,” O’ReillyMed.Inc.USA,2008.
[4] J.Ferdinand,C.Wijaya,A.N.Ronal,I.S.Edbert, andD.Suhartono,“ATMSecuritySystemMod‐elingUsingFaceRecognitionwithFaceNetand HaarCascade,” 20226thInternationalConferenceonInformaticsandComputationalSciences (ICICoS),2022,pp.111–116,doi:10.1109/ICI‐CoS56336.2022.9930563.
[5] H.R.Babaei,O.Molalapata,andA.A.Pandor, “FaceRecognitionApplicationforAutomatic TellerMachines(ATM),” ICIKM,vol.45,2012, pp.211–216.doi:10.9756/BIJSESC.8273.
[6] M.S.Minu,etal,“FaceRecognitionSystemBased OnHaarCascadeClassi ier,” InternationalJournalofAdvancedScienceandTechnology,vol.29, no.5,2020,pp.3799–3805.
[7] T.V.Priya,G.VinithaSanchez,andN.R.Raajan, “FacialRecognitionSystemUsingLocalBinary Patterns(LBP),” InternationalJournalofPure andAppliedMathematics,vol.119,no.15,2018, pp.1895–1899.
[8] M.Karovaliya,S.Karedia,S.Oza,andD.R. Kalbande,“EnhancedSecurityforATMMachine withOTPandFacialRecognitionFeatures,” ProcediaComputerScience,vol.45,2015,pp.390‐396,ISSN:1877‐0509,doi:10.1016/j.procs.20 15.03.166.
[9] S.Sasipriya,D.P.Kumar,andS.Shenbagadevi, “FaceRecognitionBasedNewGenerationATM System,” EuropeanJournalofMolecular&Clinical Medicine,vol.7,no.4,2020,pp.2854–2865.
[10] T.Ahonen,A.Hadid,andM.Pietikainen,“Face DescriptionwithLocalBinaryPatterns:Appli‐cationtoFaceRecognition,” IEEETrans.Pattern AnalysisandMachineIntelligenceIEEEComp. Soc.,vol.28,2006,pp.2037–2041.
[11] S.Hazra,“SmartATMService,” 2019Devices forIntegratedCircuit(DevIC),Kalyani,India, 2019,pp.226–230,doi:10.1109/DEVIC.2019 .8783820.
[12] K.S.doPrado,“FaceRecognition:Understand‐ingLBPHAlgorithm,” Medium.Accessed:Feb.16, 2024.[Online].Available:https://towardsdatas cience.com/face‐recognition‐how‐lbph‐works‐90ec258c3d6b
[13] A.B.Shetty,Bhoomika,Deeksha,J.Rebeiro,and Ramyashree,“FacialRecognitionUsingHaarCas‐cadeAndLBPClassi iers,” GlobalTransitionsProceedings,vol.2,no.2,2021,pp.330–335,doi: 10.1016/j.gltp.2021.08.044.