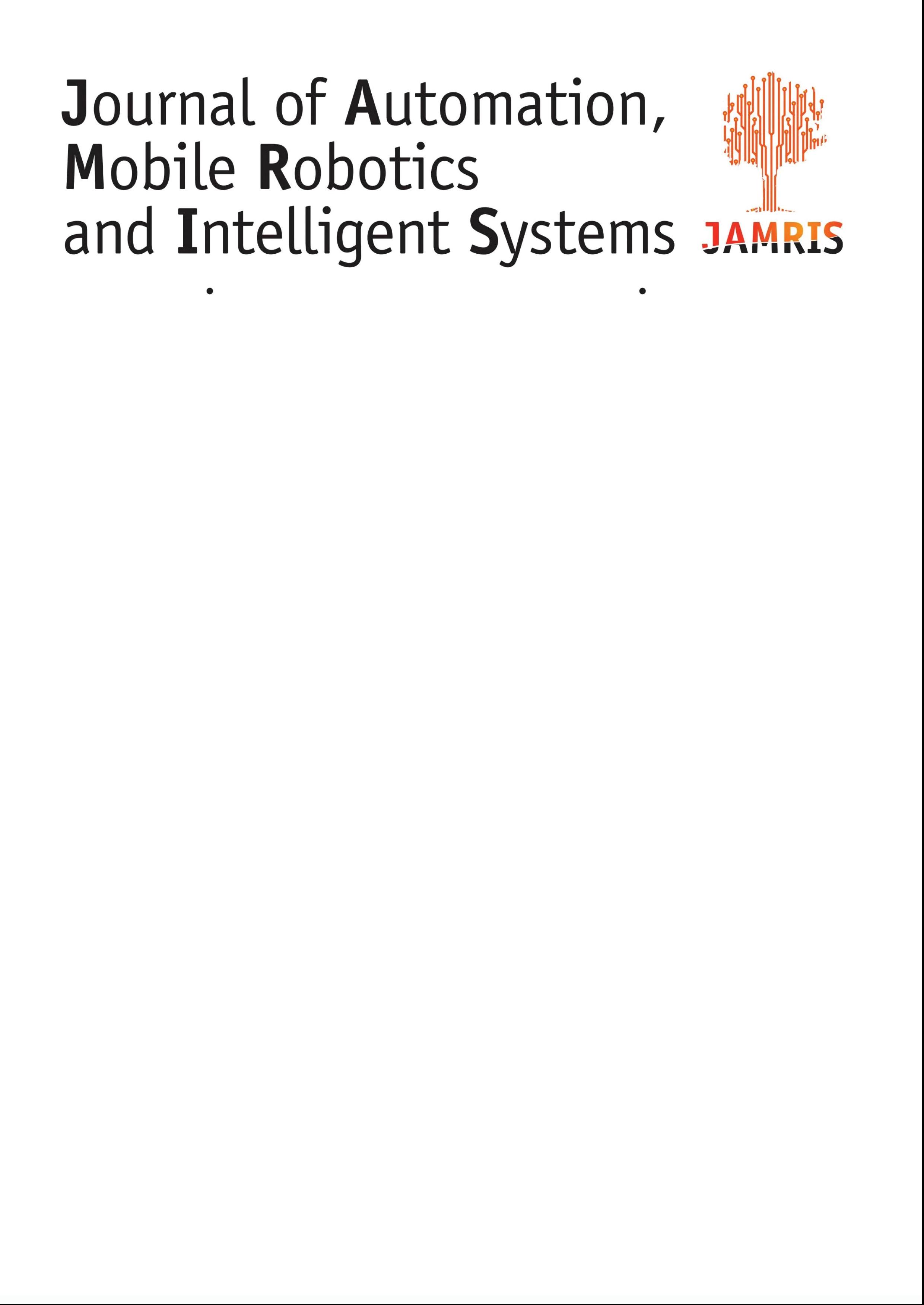
WWW.JAMRIS.ORG pISSN 1897-8649 (PRINT)/eISSN 2080-2145 (ONLINE) VOLUME 18, N° 3, 2024
Indexed in SCOPUS
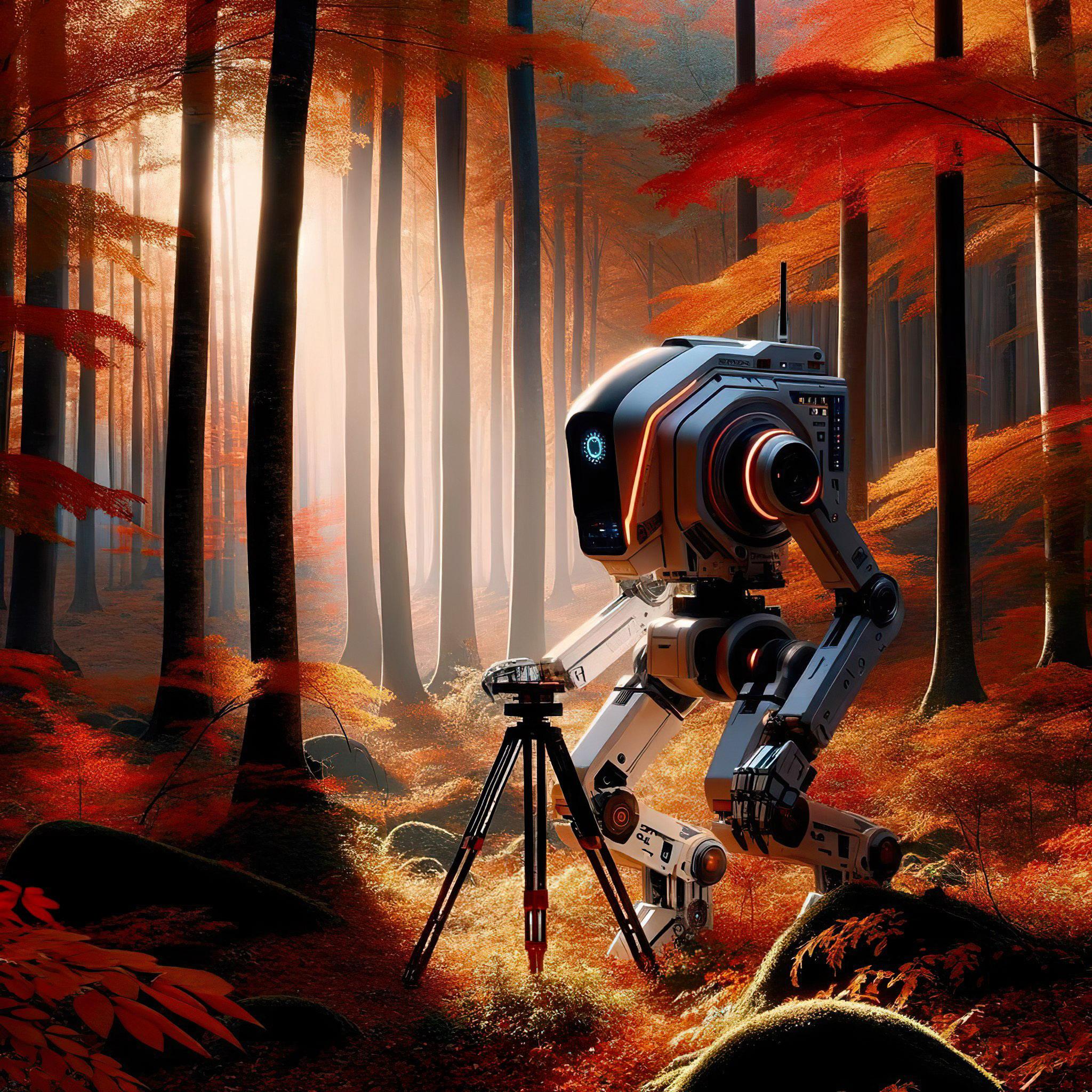
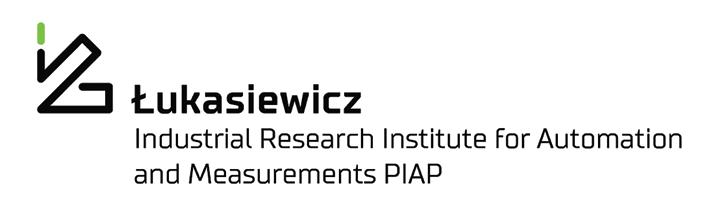
WWW.JAMRIS.ORG pISSN 1897-8649 (PRINT)/eISSN 2080-2145 (ONLINE) VOLUME 18, N° 3, 2024
Indexed in SCOPUS
A peer-reviewed quarterly focusing on new achievements in the following fields: • automation • systems and control • autonomous systems • multiagent systems • decision-making and decision support • • robotics • mechatronics • data sciences • new computing paradigms •
Editor-in-Chief
Janusz Kacprzyk (Polish Academy of Sciences, Łukasiewicz-PIAP, Poland)
Advisory Board
Dimitar Filev (Research & Advenced Engineering, Ford Motor Company, USA)
Kaoru Hirota (Tokyo Institute of Technology, Japan)
Witold Pedrycz (ECERF, University of Alberta, Canada)
Co-Editors
Roman Szewczyk (Łukasiewicz-PIAP, Warsaw University of Technology, Poland)
Oscar Castillo (Tijuana Institute of Technology, Mexico)
Marek Zaremba (University of Quebec, Canada)
Executive Editor
Katarzyna Rzeplinska-Rykała, e-mail: office@jamris.org (Łukasiewicz-PIAP, Poland)
Associate Editor
Piotr Skrzypczyński (Poznań University of Technology, Poland)
Statistical Editor
Małgorzata Kaliczyńska (Łukasiewicz-PIAP, Poland)
Editorial Board:
Chairman – Janusz Kacprzyk (Polish Academy of Sciences, Łukasiewicz-PIAP, Poland)
Plamen Angelov (Lancaster University, UK)
Adam Borkowski (Polish Academy of Sciences, Poland)
Wolfgang Borutzky (Fachhochschule Bonn-Rhein-Sieg, Germany)
Bice Cavallo (University of Naples Federico II, Italy)
Chin Chen Chang (Feng Chia University, Taiwan)
Jorge Manuel Miranda Dias (University of Coimbra, Portugal)
Andries Engelbrecht ( University of Stellenbosch, Republic of South Africa)
Pablo Estévez (University of Chile)
Bogdan Gabrys (Bournemouth University, UK)
Fernando Gomide (University of Campinas, Brazil)
Aboul Ella Hassanien (Cairo University, Egypt)
Joachim Hertzberg (Osnabrück University, Germany)
Tadeusz Kaczorek (Białystok University of Technology, Poland)
Nikola Kasabov (Auckland University of Technology, New Zealand)
Marian P. Kaźmierkowski (Warsaw University of Technology, Poland)
Laszlo T. Kóczy (Szechenyi Istvan University, Gyor and Budapest University of Technology and Economics, Hungary)
Józef Korbicz (University of Zielona Góra, Poland)
Eckart Kramer (Fachhochschule Eberswalde, Germany)
Rudolf Kruse (Otto-von-Guericke-Universität, Germany)
Ching-Teng Lin (National Chiao-Tung University, Taiwan)
Piotr Kulczycki (AGH University of Science and Technology, Poland)
Andrew Kusiak (University of Iowa, USA)
Mark Last (Ben-Gurion University, Israel)
Anthony Maciejewski (Colorado State University, USA)
Typesetting
SCIENDO, www.sciendo.com
Webmaster TOMP, www.tomp.pl
Editorial Office
ŁUKASIEWICZ Research Network
– Industrial Research Institute for Automation and Measurements PIAP
Al. Jerozolimskie 202, 02-486 Warsaw, Poland (www.jamris.org) tel. +48-22-8740109, e-mail: office@jamris.org
The reference version of the journal is e-version. Printed in 100 copies.
Articles are reviewed, excluding advertisements and descriptions of products.
Papers published currently are available for non-commercial use under the Creative Commons Attribution-NonCommercial-NoDerivs 4.0 (CC BY-NC-ND 4.0) license. Details are available at: https://www.jamris.org/index.php/JAMRIS/ LicenseToPublish
Open Access.
Krzysztof Malinowski (Warsaw University of Technology, Poland)
Andrzej Masłowski (Warsaw University of Technology, Poland)
Patricia Melin (Tijuana Institute of Technology, Mexico)
Fazel Naghdy (University of Wollongong, Australia)
Zbigniew Nahorski (Polish Academy of Sciences, Poland)
Nadia Nedjah (State University of Rio de Janeiro, Brazil)
Dmitry A. Novikov (Institute of Control Sciences, Russian Academy of Sciences, Russia)
Duc Truong Pham (Birmingham University, UK)
Lech Polkowski (University of Warmia and Mazury, Poland)
Alain Pruski (University of Metz, France)
Rita Ribeiro (UNINOVA, Instituto de Desenvolvimento de Novas Tecnologias, Portugal)
Imre Rudas (Óbuda University, Hungary)
Leszek Rutkowski (Czestochowa University of Technology, Poland)
Alessandro Saffiotti (Örebro University, Sweden)
Klaus Schilling (Julius-Maximilians-University Wuerzburg, Germany)
Vassil Sgurev (Bulgarian Academy of Sciences, Department of Intelligent Systems, Bulgaria)
Helena Szczerbicka (Leibniz Universität, Germany)
Ryszard Tadeusiewicz (AGH University of Science and Technology, Poland)
Stanisław Tarasiewicz (University of Laval, Canada)
Piotr Tatjewski (Warsaw University of Technology, Poland)
Rene Wamkeue (University of Quebec, Canada)
Janusz Zalewski (Florida Gulf Coast University, USA)
Teresa Zielińska (Warsaw University of Technology, Poland)
Publisher:
1
VOLUME 18, N˚3, 2024
DOI: 10.14313/JAMRIS/3-2024
Tackling Non‐IID Data and Data Poisoning in Federated Learning Using Adversarial Synthetic Data
Anastasiya Danilenka
DOI: 10.14313/JAMRIS/3‐2024/17
14
Gradient Scale Monitoring for Federated Learning Systems
Karolina Bogacka, Anastasiya Danilenka, Katarzyna Wasielewska‐Michniewska
DOI: 10.14313/JAMRIS/3‐2024/18
Efficiency of Artificial Intelligence Methods for Hearing Loss Type Classification: An Evaluation
Michał Kassjański, Marcin Kulawiak, Tomasz Przewoźny, Dmitry Tretiakow, Jagoda Kuryłowicz, Andrzej Molisz, Krzysztof Koźmiński, Aleksandra Kwaśniewska, Paulina Mierzwińska‑Dolny, Miłosz Grono
DOI: 10.14313/JAMRIS/3‐2024/19
39
Analysis of Dataset Limitations in Semantic Knowledge‐Driven Multi‐Variant Machine
Translation
Marcin Sowański, Jakub Hościłowicz, Artur Janicki
DOI: 10.14313/JAMRIS/3‐2024/20
49
Identification and Modeling of the Dynamical Object with the Use of HIL Technique
Łukasz Sajewski, Przemysław Karwowski
DOI: 10.14313/JAMRIS/3‐2024/21
Advanced Perturb and Observe Algorithm for Maximum Power Point Tracking in Photovoltaic Systems with Adaptive Step Size
Amal Zouhri
DOI: 10.14313/JAMRIS/3‐2024/22
EEG based Emotion analysis Using Reinforced Spatio‐Temporal Attentive Graph Neural and Contextnet techniques
C. Akalya Devi, D. Karthika Renuka
DOI: 10.14313/JAMRIS/3‐2024/23
69
Enhancing Stock Price Prediction in the Indonesian Market: A Concave LSTM Approach with RunReLU
Mohammad Diqi, I Wayan Ordiyasa
DOI: 10.14313/JAMRIS/3‐2024/24
78
Atlantic Blue Marlin, Boops, Chironex Fleckeri, and General Practitioner – Sick Person Optimization Algorithms
Lenin Kanagasabai
DOI: 10.14313/JAMRIS/3‐2024/25
89
Network Optimization Using Real Time Polling Service with and Without Relay Station in WiMax Networks
Mubeen Ahmed Khan, Awanit Kumar, Kailash Chandra Bandhu
DOI: 10.14313/JAMRIS/3‐2024/26
This part of the Journal of Automation, Mobile Robotics and Intelligent Systems is devoted to current studies in Computer Science and Information Technology presented by young, talented contributors working in the field – it is the fourth edition of this series. Among the included papers, one can find contributions dealing with diagnosing machine learning problems, natural language processing procedures, AI classification and clustering methods, optimization tasks, and learning procedures. This part of JAMRIS was inspired by broad and interesting discussions during the Eight Doctoral Symposium on Recent Advances in Information Technology (DS-RAIT 2023), held in Warsaw, Poland, on September 17-20, 2023, as a satellite event of the Federated Conference on Computer Science and Information Systems (FedCSIS 2023). The Symposium facilitated the exchange of ideas between early-stage researchers, particularly PhD students, in Computer Science. Furthermore, the Symposium gave all participants an opportunity to obtain feedback on their ideas and explorations from the experienced members of the IT research community who had been invited to chair all DS-RAIT thematic sessions. Therefore, submitting research proposals with limited preliminary results was strongly encouraged. Here, we highlight the contributions entitled “Mitigating the effects of non-IID data in federated learning with a self-adversarial balancing method,” written by Anastasiya Danilenka (Warsaw University of Technology). This paper received the Best Paper Award at DS-RAIT 2023.
This issue contains the following DS-RAIT papers in their special, extended versions.
The first paper, entitled “Tackling Non-IID Data and Data Poisoning in Federated Learning Using Adversarial Synthetic Data,” authored by Anastasiya Danilenka, explores crucial aspects of federated learning (FL). FL involves collaborative model training across diverse devices while safeguarding data privacy. However, managing heterogeneous data across these devices poses a significant challenge, exacerbated by the potential presence of malicious clients aiming to disrupt the training process through data poisoning. The article addresses the issue of discerning between poisoned and non-Independently and Identically Distributed (non-IID) data by proposing a technique that leverages data-free synthetic data generation, employing a reverse adversarial attack concept. This approach enhances the training process by assessing client coherence and favouring trustworthy participants. The experimental findings garnered from image classification tasks on MNIST, EMNIST, and CIFAR-10 datasets are meticulously documented and analysed, shedding light on the efficacy of the proposed method. As already mentioned, the DS-RAIT Program Committee voted this work the Best Paper of the event because of its excellent presentation of applicational aspects and promising results.
The paper entitled “Gradient Scale Monitoring for Federated Learning Systems” was written by Karolina Bogacka, Anastasiya Danilenka, and Katarzyna Wasielewska-Michniewska. In this paper, the authors delve into the burgeoning realm of Federated Learning amidst edge and IoT devices’ expanding computational and communicational capabilities. While FL holds promise, particularly in cross-device settings, existing research often needs to be more focused on critical operationalisation and monitoring challenges. Through a case study comparing four FL system topologies, the paper uncovers periodic accuracy drops and attributes them to exploding gradients. Proposing a novel method reliant on the local computation of the gradient scale coefficient (GSC) for continuous monitoring, the study expands to explore GSC and average gradients per layer as potential diagnostic metrics for FL. By simulating various gradient scenarios, including exploding, vanishing, and stable gradients, the paper evaluates resulting visualizations for clarity and computational demands, culminating in introducing a gradient monitoring suite for FL training processes.
In their study titled “Efficiency of Artificial Intelligence Methods for Hearing Loss Type Classification: An Evaluation,” Michał Kassjański, Marcin Kulawiak, Tomasz Przewoźny, Dmitry Tretiakow, Jagoda Kuryłowicz, Andrzej Molisz, Krzysztof Koźmiński, Aleksandra Kwaśniewska, Paulina Mierzwińska-Dolny, and Miłosz Grono address critical issues surrounding the evaluation of hearing loss. Traditionally, hearing loss assessment relies on pure tone audiometry testing, considered the gold standard for evaluating auditory function. Once hearing loss is identified, distinguishing between sensorineural, conductive, and mixed types becomes paramount. The study compares various AI classification models using 4007 pure-tone audiometry samples meticulously labelled by professional audiologists. Models tested
range from Logistic Regression to sophisticated architectures like Recurrent Neural Networks (RNN), Long ShortTerm Memory (LSTM), and Gated Recurrent Unit (GRU). Furthermore, the study explores the impact of dataset augmentation using Conditional Generative Adversarial Networks and different standardisation techniques on the performance of machine learning algorithms. Remarkably, the RNN model emerges with the highest classification performance, achieving an out-of-training accuracy of 94.4% as determined by 10-fold Cross-Validation.
Finally, Marcin Sowanski, Jakub Hoscilowicz, and Artur Janicki contributed a paper titled “Analysis of Dataset Limitations in Semantic Knowledge-Driven Multi-Variant Machine Translation.” This research explores the intricacies of dataset constraints within semantic knowledge-driven machine translation, tailored explicitly for intelligent virtual assistants (IVA). Departing from conventional translation methodologies, the study adopts a multi-variant approach to machine translation. Instead of relying on single-best translations, their method employs a constrained beam search technique to generate multiple viable translations for each input sentence. The methodology’s expansion is noteworthy beyond the constraints of specific verb ontologies, operating within a broader semantic knowledge framework. This enables a more nuanced interpretation of linguistic nuances and contextual intricacies, thereby enhancing translation accuracy and relevance within the IVA domain.
We want to thank all those who participated in and contributed to the Symposium program and all the authors who submitted their papers. We also wish to thank all our colleagues and the members of the Program Committee for their hard work during the review process, their cordiality, and the outstanding local organisation of the Conference.
Editors: Piotr A. Kowalski
Systems Research Institute, Polish Academy of Sciences and Faculty of Physics and Applied Computer Science, AGH University of Science and Technology
Szymon Łukasik
Systems Research Institute, Polish Academy of Sciences and Faculty of Physics and Applied Computer Science, AGH University of Science and Technology
Submitted:27th December2023;accepted:11th March2024
AnastasiyaDanilenka
DOI:10.14313/JAMRIS/3‐2024/17
Abstract:
Federatedlearning(FL)involvesjointmodeltrainingby variousdeviceswhilepreservingtheprivacyoftheir data.However,itpresentsachallengeofdealingwith heterogeneousdatalocatedonparticipatingdevices. Thisissuecanfurtherbecomplicatedbytheappear‐anceofmaliciousclients,aimingtosabotagethetrain‐ingprocessbypoisoninglocaldata.Inthiscontext, aproblemofdifferentiatingbetweenpoisonedand non‐identically‐independently‐distributed(non‐IID)data appears.Toaddressit,atechniqueutilizingdata‐free syntheticdatagenerationisproposed,usingareverse conceptofadversarialattack.Adversarialinputsallow forimprovingthetrainingprocessbymeasuringclients’ coherenceandfavoringtrustworthyparticipants.Exper‐imentalresults,obtainedfromtheimageclassification tasksforMNIST,EMNIST,andCIFAR‐10datasetsare reportedandanalyzed.
Keywords: federatedlearning,non‐IIDdata,labelskew, datapoisoning,labelflipping
1.Introduction
Federatedlearning(FL)[1]focusesondeveloping aglobalmodelbycoordinatinglearningonmultiple deviceswhilemaintainingtheprivacyoflocaldata. ThetypicalprocessofFLconsistsoftrainingrounds andinvolvesseveralsteps:(1)theglobalmodelis initializedontheserver;(2)thesubsetofclientsof aspeci iedsizeisrandomlychosenfromallavail‐ableclients;(3)theglobalmodelissharedamong theselectedsubsetofclients;(4)clientsperform localtrainingwiththereceivedglobalmodelfora limitednumberofepochsusingtheirprivatedata; (5)clientsreturntheirmodelupdatestotheserver; and(6)modelupdatesareaggregatedontheserver intoanewversionoftheglobalmodel[1].
EachofthedefaultFLstepsisopentochangesand re inements.Thus,thesubsetofclientsforatraining roundmaybecreatednotrandomly,butbyfollowing astrategy,clientsmaynotsendweightupdatesto theserver,buttheresultsofSGD[1],orcommuni‐catefullmodelweights.Moreover,aggregationofthe modelupdatesontheservermaynotsimplyaver‐ageallreceivedmodelupdatesasproposedinthe FedAvgalgorithm,butprioritizeoneclient’supdates overanother’s.Forinstance,byusingthesizeoftheir localdatasetsasweights[1],participantswithbig
localdatasetsarefavoredastheycontributemoreto thenewglobalmodelduringaggregation.
DuetotheprivacyrestrictionsofFL,clients’local datasetsremainontheirlocaldevices,makingit impossibletoperformcentralizeddataanalyticsand inferpropertiesofbothglobalandlocaldatasets. Moreover,inreal‐lifecases,datamaynotbeiden‐ticallyindependentlydistributed(non‐IID)among clients,whichwasprovedtocauseproblemsforFL, asthequalityoftheglobalmodelanditsconvergence canbenegativelyimpactedbythepresenceofsuch data[2,3].Non‐IIDdatacanbecategorizedinto ive types[4],i.e.,(1)featuredistributionskew(different clientshavevariationsinfeaturestylesforthesame label);(2)labeldistributionskew(clientshavevary‐inglabeldistributionsbutsimilarfeaturesforspe‐ci iclabels);(3)samelabel,differentfeatures(differ‐entclientspresentdifferentfeaturedistributionsfor thesamelabel);(4)samefeatures,differentlabels (clientsassigndifferentlabelstothesamefeatures); and(5)quantityskew(differencesintheamountof dataacrossclients).
Ingeneral,thesedata‐relatedskewsaresupposed tobetheresultofthenaturalcharacteristicsofthe data,highlightingthecomplexityanddiversityofreal‐lifefederateddatasets.However,anothersetofdata issuescancomefrommaliciousactors,whichhave accesstoclientdevicesandclientdata,resultingina securityissueknownasadatapoisoningattack[5]. Inthiscase,theadversarymayperformdatapoi‐soningattacksandaimtocompromisethetraining process,reducetheglobalmodelperformance,and causeincorrectmodelpredictionsduringtheinfer‐encestage[6].Despitepoisoneddatabeingdifferent fromthenon‐IIDdataproblem,FLbydefaultequally protectstheprivacyofnon‐IIDandmaliciousclients, naturallymakingthetaskofdistinguishingbetween themmorechallenging.
Toaddressthechallengesposedbylabelskewnon‐IIDdata,the AdversarialFederatedLearning (AdFL) algorithmwasintroduced[7].Thismethodoriginated fromtheconceptofadversarialattacksandismainly applicabletoneuralnetworksdealingwithimage data.TheAdFLalgorithmallowsforgainingvaluable insightsaboutclients’localdatasetswithoutrequest‐inganyadditionalinformationfromlocaldevicesby utilizingsyntheticdatageneratedontheserver.
Thealgorithmimprovestheperformanceofglobal modelsinthepresenceoflabelskewdataandresults inmorestabletrainingandmorebalancedper‐class accuracyoftheglobalmodel.
Thisworkisanextensionoftheresearchpre‐sentedin[7]andexploresthepossibilityofusingself‐adversarialsamplesfordistinguishingmaliciousnon‐IIDclientsfromthosethatarebenign,focusingon untargetedandtargetedlabel‐ lippingattacks.
Followingthis,inSection 2,relatedresearchon datapoisoningattacksinthepresenceofnon‐IIDdata withinFLisoutlined.Section3presentskeyconcepts ofadversarialattacks.InSection4,theAdFLalgorithm isdescribed.Section 5 de inesthedatapoisoning attacksadoptedinthispaper.Section 6 coversthe experimentalresultsandtheiranalysis,collectedfrom MNIST[8],EMNIST[9],andCIFAR‐10[10]datasets. Thisworkconcludeswithasummaryof indingsand futureresearchsuggestions.
ThedatapoisoningattacksinFLasastandalone issuearebeingaddressedinmultipleways.For instance,maliciousclientscanbedetectedand iltered outfromthetraining.Here,methodswereproposed totracktheconsistencyoftheclient’supdatesto verifyitsintent[11],applydimensionality‐reduction andclusteringtechniques(suchaskernelprincipal componentsanalysisandk‐means)[12],orusing Euclideandistance‐measure[13, 14]todistinguish betweenmaliciousandbenignclientsand ilterout suspiciousclients.Anotherapproachproposedisto maintainasmallcleantrainingdatasetandasepa‐ratemodelontheserver,usingthemtoassessthe trustworthinessofclients’updatesbycomparingthe directionoflocalmodelsupdateswiththeserver‐side modelupdateobtainedfromthecleandatasetand furtherusingtrustworthinessscoreasaggregation weightsfornormalizedclients’modelupdates[15]. Anotherlineofresearchfocusedonmodifyingthe aggregationstrategytowardsoutlierresistance,for example,isbytakingnotthemean,butthemedian foreachdimensionfromthemodelupdates[16]or trimmingtheupdatesbeforeaveraging[17]toavoid extremevalues.However,thesemethodsmainlyrely ontheassumptionthatbenignclientswillhavesimilar modelupdates,whichcannotbeguaranteedunder thenon‐IIDdata.
Toaddressthejointproblemofpossibledatapoi‐soningattacksandnon‐IIDdata,methodsforboth maliciousclientsdetectionandnon‐IIDdatamiti‐gationwereproposed.Forinstance,thealgorithm utilizingacosinesimilaritymeasurewaspresented toassessclients’contributionsimilarity,assuming thatbenignclientswillhavemorediversegradi‐entupdatesthancoordinatedmaliciousclients[18]. Anotherapproachsuggestedusingasmallproxy datasetasatooltoperformon‐serveroptimization to indthebestmodelupdatesfusionandmitigate possiblemaliciousclientseffectbynaturallyassigning themsmallaggregationweights[19].
Adifferentsolutionproposedanalyzingthecrit‐icalparametersofthelocalmodelstoreliablyiden‐tifymaliciousclientsanduseitforweightedupdates aggregation[20].Anattack‐tolerantFLmethodwas alsoproposed,presentinglocalmetaupdatesand globalknowledgedistillationtomitigatepossible maliciousclientseffectontheglobalmodel[21].
Althoughtheresearchhasbeguntosimultane‐ouslyaddresstheproblemofbothnon‐IIDdataand potentialdatapoisoningattacksinFL,theproposed solutionscanstillrelyonproxydatasetsavailableon theserversideorcomplicatethelocaltrainingprocess withadditionalcomputations.Suchassumptionsmay notbefeasibleinsomeFLscenarios.Moreover,the complexityofthenon‐IIDdataproblemandthevari‐etyofdatapoisoningattackscenariosmakeitharder to indsolutionsthatcansatisfybothperformance andavarietyofsecuritygoals,leavingthischallenging areaopenforfurtherresearch.
AdversarialdataareadoptedbymanyFLalgo‐rithms.Thecommonideaisutilizingadversarialtech‐niquesasdatageneratorsinorderto(a)defend themodelagainstadversarialattacks[22, 23],or (b)augmentthequantityoflocallyaccessibledata withsyntheticsamples[24–26].Thisworkextends theapplicabilityofthepreviouslyproposedalterna‐tivemethod,incorporatingadversarialdataintoFL.
3.1.AdversarialAttack
Theessenceofadversarialattacksliesintheability tomodifyasamplefromthetrainingdataofaneural networkinamannerthatisimperceptibletohumans, yetcausesthetrainednetworktoincorrectlyclassify whatwasonceacorrectlyclassi iedsample[27].This phenomenonwasillustratedtobecausedbytheabil‐ityoftheadversarytoalterthetargetdatasampleina waythatmakesitcrosstheclassi ier’sdecisionbound‐ary,and,therefore,resultinmisclassi ication[28].
Theclassi icationofadversarialattacksfallsinto twomaincategories: untargeted attacks,whichsim‐plyfocusoncausinganyincorrectclassi ication,and targeted attacks,wherethegoalistotriggermisclas‐si icationintoaspeci icclass.Attackmethodologies arefurtherdividedinto white-box attacks,whenthe involvedadversaryhasaccesstothemodel’sarchi‐tectureandparameters,and black-box attacks,which relysolelyontheattacker’saccesstooutputdata. Asetofgradient‐basedalgorithmswaspreviously presentedthatreliesonthemodel’sgradientsanda lossfunctiontocreatethenecessarychangestothe sourcedatainordertoperformanattack.Forinstance, gradient‐basedalgorithmsare:one‐stepFastGradi‐entSignMethod(FGSM)[29],itsiterativeversionI‐FGSM[30],anditsversionenhancedwithmomentum MI‐FGSM[31].
Inthisstudy,themomentumiterativefastgradient signmethod(MI‐FGSM)isusedtoperformtargeted adversarialattacks[31](seeEquations1and2).
Here,���� representstheaccumulatedgradients,��∗ �� istheperturbedadversarialimageiniteration��,�� is atargetclass, �� isalossfunction, �� isadecayfactor introducedforbetterattacksuccessrateand �� isa stepsize.Ateachiteration,��∗ �� isclippedinthevicinity ��,topreservetheresultingadversarialimagewithin ��∞ distancefromthesourceimage.
3.2.TransferabilityofAdversarialInputs
Adversarialinputspossesstheabilitytotrans‐feracrossmodels,meaningthatadversarialinputs designedforonemodelcanalsocausemispredictions fromothermodels,withthetransferabilityofadver‐sarialsamplesbeinghigherbetweenmodelstrained ondataandtasksthataresimilar.Thisphenomenonis attributedtothefactthatmodelsaddressingthesame tasktendtodevelopsimilardecisionboundaries.In thecontextofFL,whereclientsworkonthesametask withsharedmodelarchitectureandfeaturespace,this transferabilityisparticularlyuseful.Itwasshown, thatadversarialsamplesgeneratedbyanyclientcan provideinsightsaboutlocaldatadistribution[7].The propertyoftransferabilityofadversarialsamplesand itsrelevancetodecisionboundariesoftrainedclassi‐iersinFLformedthebasisoftheAdFLalgorithm.
IntheAdFLalgorithm,adversarialimagesareuti‐lizedasanadditionalsourceofinformationtoimprove andguidethetrainingprocess.Thegenerationof theseimagesisdoneontheserver,usingthemodels thathavebeenupdatedandarandomnoisesample imageasastartingpointforthegenerationprocess. Thisway,theadversarialimagesaregeneratedin adata‐freeway,meaning,thatnoaccesstoactual clients’dataisneeded.Thespeci icstepsperformed bytheserverintheAdFLareoutlinedinAlgorithm1
Note,thatintheAdFLalgorithm,theweightsofthe modelarecommunicatedbetweenclientsandserver.
Intotal,sixstepssummarizetheAdFLalgorithm: 1) Duringthe irstfederatedtraininground,allclients receivetheinitializedglobalmodel,performlocal training,andreturntheresultingmodelsbackto theserver.
2) Updatedmodelsreturnedbyclientsareusedto generateadversarialsamples(Section4.1).
3) Theestimationofthedistributionofclassesacross clientsisperformedusingthegeneratedadversar‐ialsamples,asdiscussedinSection4.2
4) EachclientgetsaCScalculatedwiththehelp ofupdatedmodelsandthegeneratedadversarial samples(seeSection4.4fordetails).
Algorithm1 AdFLalgorithm(Server); ���� –client; ������ –subsetofclientspickedfortrainingonepoch ��; globaldistribution –distributionofclassesduring FLtraining; ���������������� –estimatedclassespresencein clients’localdatasets
Ensure: globalmodel ��0, globaldistribution,clients ready for ��in��������ℎ�� do if ��==0 then ������ ← allclients else ������, globaldistribution ← pickclientsfor training(����������������, globaldistribution) endif for ����in������ do ������ �� ←runtraining(����) endfor advdata ←createadvdata([��0 ��,...,�� ������ �� ]) if ��==0 then ���������������� ←estimatedistribution(advdata) endif C��[0−������] ←calculateCS(advdata,��[0,..,������] �� ) ���� ←FedAvg([��[0,..,������] �� ],����[0−������]) endfor
5) Theaggregationsteputilizesclients’coherence scoresasweightstoformthenextversionofthe globalmodel.Thisnewglobalmodelisthendis‐tributedtoanewsubsetofclients,initiatingthe nexttraininground.
6) Thereafter,theclient‐pickingstrategy,guidedby theglobalclassesdistribution(seeSection 4.3), regulateswhichsubsetofclientswillengagein thenextroundoftraining,andtheprocessrepeats fromstepone,omittingtheestimationofthedis‐tributionofclassesacrossclients.
Itshouldbeemphasized,thatallstepsintroduced bytheAdFLthatexpandtheclassicalFLpipelineare performedontheserver.Theadversarialimagescre‐ation,clientpickingstrategy,andcoherencescores calculationarecoveredinthenextsubsections.
AdversarialdataintheAdFLalgorithmiscreated basedonthemodelsreturnedbyclientsanddoesnot requireactualclients’data.Therefore,theadversarial inputgenerationstartsfromarandomnoiseimage andisperformedwiththeMI‐FGSMalgorithm(see Equations 1 and 2).Thisattackisparameterizedby ��, ��,thenumberofsteps,andtheclippingbound‐ary.AstheadversarialinputsproducedbytheAdFL algorithmarenotusedtoperformactualadversar‐ialattacks,theconstraintsontheamountofchange appliedcanberelaxed.Forexample,thenumberof steps,��,andtheclippingboundarycanbeviewedas constraintsonthealgorithmsothatthe inaladver‐sarialimageisnotfarfromitssource,therefore,they wereadjustedaccordingtotheobjective.
Itwasexperimentallyvalidatedthatgoingbeyond 30adversarialstepsdoesnotimprovetransferability, thus,thenumberofstepswassetto30.Thestepsize �� wassetto1,whiletheclippingboundaryand �� wereleftunchanged,followingtheoriginalMI‐FGSM research.
Algorithm 2 outlinestheprocessforcreating adversarialinputs,whilethedefaultfederatedsteps arenotincluded.
Algorithm2 Adversarialdatageneration
Ensure: targets ←[0,...,��−1]
Ensure: ��[0,..,������] �� ▷Updatedmodelsatepoch�� for ��in�������������� do for ���� �� in��[0,..,������] �� do advimg ←randomnoise[��ℎ,��,��] for ��������in numsteps do ▷MI‐FGSMstep advimg�� �� ←step(���� ��, advimg�� ��,��) endfor endfor endfor
Afterthelocaltraining,eachupdatedmodel returnedtotheserverisusedtogenerate �� images, i.e.,oneimageisgeneratedperclassthatispresentin theclassi icationtask.
4.2.LocalDistributionEstimation
Asspeci iedintheAlgorithm 1,the irsttraining roundintheAdFLalgorithminvolvesallclientsinper‐formingthelocaltraining.Thesemodelsarethenused tocreateadversarialsamples(Section4.1).Duringthe research,itwasdeterminedthatwhenoneclient’s updatedmodelmakespredictionsonadversarialsam‐plescreatedbyanotherclient’supdatedmodelatthe endofthe irstepoch,thesepredictionsareindicative ofthespeci icclassespresentinthelocaldatasetofthe clientthatperformedthepredictions[7].Therefore,at theendofthe irstround,itispossibletoestimatethe labeldistributionamongallclients,thatparticipated inthetraininground.
Detectinglabels’presenceinlocaldatabyinspect‐ingtheadversarialdatapredictionspresentsthe opportunityforfurtherimprovementsinthefeder‐atedtrainingprocess,basedontheinsightsgathered. However,itisworthemphasizing,thatthediscovered labeldistributionisstillanestimation.
4.3.Client‐pickingStrategy
Oncetheclassesintheclients’localdatasetsare estimated,aclient‐pickingstrategycanbeusedto reducetheeffectsoflabelskewinthelocaldatasets.In theAdFLalgorithm,thein luenceoflabelskewonthe trainingprocessisbeingaddressedwithabalanced client‐picking.
Thebalancedclient‐pickingisperformedbyuti‐lizingtheinformationretrievedduringthelocaldis‐tributionestimationstepdescribedintheprevious subsectionandaimsathavingtheclientswithdiverse localdatalabeldistributionspickedforeachtraining round.
Thisstrategyensuresequalrepresentationofcom‐monandrareclassesineachtraininground,there‐fore,continuouslyexposingthemodeltoallpossible classesintheclassi icationtask,leadingtoamore balancedperformanceacrossallclasses.
Totrackwhichclasseswerepresentontheclients thatparticipatedinthetrainingprocess,agloballabel frequencyvectorofsize��ismaintainedontheserver, accumulatingthenumberofclientsthatparticipated intrainingepochsuptonowandhadacertainclass�� intheirlocaldataset.Asanewsubsetofclientsisbeing formedforatraininground,thevectorisupdatedwith thelabeldistributioninformationofeachclientadded tothesubsetforthisfederatedtraininground.
TomaintainthebalancedFLtrainingandcon‐sistentinvolvementofallclassesinthetraining,the clientsforeachnewfederatedroundarepickedin suchawayastobringthevaluesintheglobalfre‐quencyvectorclosertoauniformdistribution.Todo so,aKullback–Leiblerdivergenceisused(Equation3).
Therefore,priortoaddingacertainclienttoasub‐setofclientsforthetraininground,theKL‐divergence iscalculatedwithrespecttotheuniformdistribution andthegloballabelfrequencyvectorassumingthat thisclientisaddedtothetraining,i.e.,itsclassesare admittedtotheglobalclassesfrequency.Thistech‐niqueensuresthatclientswhopossessrarelabelsin theirdataareconsistentlyincludedinthetraining.
4.4.ClientsCoherenceMeasurement
Transferabilityofadversarialsamplesisnotguar‐anteedbydefaultforallfederatedclients,asitrelieson theinternalpropertiesofthemodelandthedataitwas trainedon.AspresentedinSection4.2,examiningthe predictionsofthemodelsthatonlycompletedtheir irstfederatedtrainingroundcanhelpidentifytheir localdistribution,sincethisiswhatcanbeseenin thepredictionsthemodelsmakebasedonadversarial samples.Consequently,thesepredictionscanidentify whichclassesarenotinthelocaldistribution,locating nodeswithraredata.However,thispropertycanbe usednotonlyforlabeldistributionestimationbutalso forassessinghowclosetoeachothertheupdated clients’modelsare.ThisassessmentintheAdFLalgo‐rithmiscalledacoherencescore(CS)andisemployed to indclientswithahighabilitytocorrectlypredict adversarialsamplesaswellasproducethosethatare correctlypredictedbyothermodels.
Thus,theCSconsistsoftwoparts,i.e.,themodel’s abilityto(1)producesamplestransferabletoother modelsand(2)predictadversarialsamplesfrom othermodels.Thecalculationofthesemetricsisper‐formedeachtrainingroundaftertheupdatedclient modelsreturntotheserverafterperforminglocal training.Eachupdatedmodelgenerates �� adversar‐ialsamplesandmakespredictionsforalladversarial samplesgeneratedbyotherupdatedmodelsreturned byclientsparticipatinginthecurrenttraininground.
Afterthepredictionsaredone,thescorecalcula‐tionproceedswithcalculatingthemodel’sabilityto predictadversarialimagesproducedbyothermodels accordingtoEquation4.Foreachmultiplication,there isabinaryindicatordeterminingifthepredictionfor theadversarialsampleforclass �� frommodel �� was accurateandtheclassprobabilitygivenbythemodel. Theresultsobtainedforthemodelpredictingitsown adversarialinputsarenotincluded.
predictedothers
Thesameformulaisusedtoassessthemodel’s abilitytoproducetransferablesamplesthatarerecog‐nizedbyothermodels,wherethecorrectpredictions areanalyzedacrossthemodelsthatmadepredictions ofthesamplesproducedbythecurrentlyevaluated model.
The inalCSisasummationofthetwoassessment resultsandiscalculatedas:
coh.score=predictedothers+waspredicted (5)
Theresultingnormalizedcoherencescoresareused tofavormodelswithgoodtransferabilityandare employedasweightsduringtheupdatedmodelsaver‐agingprocess,therefore,haveadirectin luenceonthe globalmodelaggregation.
TheAdFLalgorithmcanutilizecoherencescores toidentifyclientswhichcannotreliablyclassifyadver‐sarialinputsorcreatesuchinputs.Thispropertyof thealgorithmcanbeusefulwhendealingwithdata poisoningattacks,thatinterferewiththeclient’slocal dataduringthetrainingprocess.Moreover,inconfor‐mitywiththeliteratureoverview,itprovidesaweight‐ingschemeforpotentiallyassigningmoreimportance tobenignclientsovermaliciousones.Therefore,this propertyofCSsinspiredthisresearch,extendingthe applicationoftheAdFLalgorithmbeyondnon‐IID label‐skewscenarios.
5.DataPoisoningAttacksinFL
Datapoisoningattackscanbeclassi iedintotwo categoriesbasedonthetargetofadversarialmanipu‐lation:cleandataattacksanddirty‐labelattacks[32]. The irsttypeofattacksdoesnotchangethelabelsof thedata,onthecontrary,itinjectschangestothesub‐setoftrainingdata[33]anddoesnotrequireaccess todatalabeling,whilethesecondattacktype,changes thelabelsofthesamplesinsidethedataset,accord‐ingtotheadversary’sgoalandleavingdatafeatures unchanged[34].
Asthenon‐IIDscenariosconsideredinthiswork arerepresentedbythelabelskew,thenaturaltypeof attacktoconsiderasits“companion”isadirtylabel label‐ lippingattack.Meaning,thatinadditiontothe limitedclassesbeingpresentinsidethelocaldata,it canfurtherbeasubjectoflocaldatasufferingfrom labelsbeing lipped.
Intheseterms,datapoisoningattackscanbe performedbyfederatedclients.Here,theattackcan bedescribedfromtheperspectiveofthenumberof clientsparticipatingintheattack—whetherthere isonlyalimitednumberofadversariesorifthere aremany—aswellasfromthewaythesourcedata labelsarebeingaffected,whethertheadversariesdo nothaveaspeci icstrategyandthelabelsare lipped randomly[35],ortheyhaveaspeci icobjectiveand liplabelsaccordingtosomerule[36].
Inthecurrentresearch,twolabel‐ lippingstrate‐giesarebeingstudied:untargeted(random)label lip‐pingandtargetedlabel lipping,meaningthatlabels foroneclassareconsistentlysubstitutedbylabels fromanotherclass.Inbothscenarios,adversariesdo nothaveawaytoseebenignclientsdatadistribu‐tions,however,inthetargetlabel‐ lippingscenarios maliciousclientshaveajointpairofsourceandtarget labelsfortheattack.Thispairisknowntoalladver‐saries.Thedetaileddescriptionofattackscenarios employedinthisworkisgiveninSection6.4
Therandomlabel‐ lippingattackprimarilyfocuses ontheoverallperformancedegradationoftheglobal model,whiletargetedattackshaveatargetclass whoseperformancetheyaimtodamage.Inorderto assesswhetherthetargetedattacksweresuccessful, theAttackSuccessRate(ASR)measureisemployed (Equation 6)withrespecttothelabelwhoseperfor‐manceistargeted.
������= numberofsuccessfulattacks totalnumberofattacks (6)
Itisalsoworthsayingthatinthepresenceofhighly skeweddatapartitionwithequalclassprobabilities insidelocaldata,randomlabel lippingresultsina softerattackscenario.Forexample,with2classes beingpresentonalocalnode,therearearound50%of correctlyassignedlabelsinsideeveryclass,asrandom assignmentisnotprohibitedfrompickingtheactual class.
6.1.Datasets
Forexperiments,threeimagedatasetswereused –MNIST[8],EMNIST[9],andCIFAR‐10[10].The datasetsrepresenttasksofvaryingdif icultyforthe algorithmsandarecommonlyutilizedasbenchmark datasetsinFLresearch.MNISToffers10‐class,28x28 grayscaleimages,andisoftenusedasabasicimage classi icationtask.EMNISTexpandsthetaskwith hand‐writtenletters,increasingthenumberofclasses to62,addingcomplexitytolabel‐skeweddata,and makingtargetedlabelattackshardertospotand counter.CIFAR‐10furtherescalatesthechallenge, introducing10classes,and32x32pixelsRGBimages withmorecomplexfeatures.
6.2.ExperimentalSetup
TheprojectwasimplementedinPython(version 3.7.9),usingPyTorchmachine‐learningframework (version1.10.0[37]).Datasetsandcustommodel architectureswereprovidedbythetorchvisionpack‐age(version0.11.1[38]).Allexperimentswererun onGPUhardware,speci ically,NVIDIAGTX1070and NVIDIAA100.
6.3.DataPartitioning
Non‐IIDlabelskewwasemulatedonlocaldevices accordingtothefollowingprocedure:(1)inthe parametersoftheexperiment,thenumberofunique classes��andthetotalnumberofdatasamples��are de inedandappliedforallclients,(2)randomseed issetforrandomoperationsreproducibility,(3)the probabilityofeachclassappearingonthelocalmodel isde inedbytakingasamplefromanormaldistribu‐tion,(4)foreachclientasetofclassesintheirrespec‐tivelocaldatasetisdeterminedbydrawingasample ofsize��fromtheclassprobabilitydistribution,(5)a uniquesubsetoftotal��datasamplesoftheselected classesisassignedtotheclient,witheachlabelhaving ��/��samplesinthelocaldataset.
Asthenumberofuniquelabel‐skewdistributions thatcanbegeneratedwiththisapproachisimmense andtheresultsobtainedacrossdifferentdistributions cannotbesimplyaggregatedduetothesedifferences instatisticalpropertiesofthedatasets,theexperi‐mentswereperformedona ixeddatadistribution. Thishelpedpreventdata‐dependentfeaturesfrom interferingwithperformancemetricsandmadeit morereliabletoattributedifferencesinmodelper‐formancetospeci icalgorithmsanddatapoisoning attacksused.
Theprobabilityoflabeloccurrenceusedforclas‐si icationtaskswith10classes(MNISTandCIFAR‐10) isillustratedinFigure1.
Duetothenormaldistributionbeingusedtocreate thelabelprobabilitydistributionforthewholeexper‐iment,someclassesnaturallyappearmoreoftenin thelocaldatasetthanothers.Itadditionallyintroduces theglobalclassimbalancetotheFLpipeline.Inacase whenthenumberoffederatedclientsisloworthe numberofuniqueclassesinthelocaldatasetsislow, someclassesmaynotappearatall.
6.4.AssumptionsandDataPoisoningAttackModel Intheconsideredattackscenariositisassumed thattheserverisfairandnotcompromised–onlya setofmaliciousclientsarethreateningtheFLpipeline. Moreover,theattackersarepresentintheFLpipeline fromthebeginningandstaytilltheendoftraining–no attackerleavesorjoinsthetrainingintheprocess.The designoftheFLexperimentisadaptedfromtheFools‐Goldalgorithmnon‐IIDscenario[18]andfeatures15 federatedclients:10honestclientsand5malicious clients.However,changesweremadetothedatapar‐titionscenariocomparedtothereferenceexperiment accordingtothedatapartitionstrategypresentedin theprevioussection.Thesechangesmodifythedata distributionstrategyandintroducethelabelskewto theclients’localdatasetsasadoptedintheexperi‐mentsdesignedforthealgorithmsfocusedonmitigat‐ingtheeffectsofnon‐IIDdata.
AccordingtothescenariospresentedinSection5, threeattacksweredesignedforexperiments:(1) untargetedrandom lippingattack,(2)targetedattack onacommonlabel,(3)targetedattackonanuncom‐monlabel.
Duringtheuntargetedattack,everymalicious clientrandomlyassignslabelstolocaldatasamples basedontheavailablelocallabelsset.Thetargeted attacksincludemaliciousclientsjointlypickingtheir target.First,maliciousclientsrevealthesetoflabels presentintheirlocaldata.Then,maliciousclientsesti‐matelabeldistributionbasedontheobservedlocal distributions.Theattackruleisde inedasapairof labels��������������,��������������,where�������������� isalabelthatwill be lippedwith��������������,therefore,�������������� performance willbetargeted.Aftersettingtheattackpair,every maliciousclientinspectsitsdataandlooksfor�������������� Iffound,the lippingisperformedaccordingtothe attackrule.Moreover,thetargetedattacksutilizenon‐IIDdataproperties,de inedintheprevioussection,by targetingeithercommonoruncommonlabelsbased ontheempiricallabeldistributioncollectedbythe maliciousclients.Commonlabelsarede inedaslabels, whoseprobabilityofoccurrenceexceedsthe66% quantileoftheprobabilityvector,whileuncommon arede inedasthoseunderthe66%quantile.
Thisway,targetedattackschemesnaturallylimit theactivenumberofactiveattackers,astheyare basedontheempiricallabelprobabilitydistribution estimatedbyattackersbeforetrainingstarts.Com‐monlabelsarenotguaranteedtobepresentinevery attacker’slocaldataduetothe66%quantilethresh‐old,makingsomepotentiallymaliciousclientsbenign duringtraining,however,stillcontributingtothe overalldistributionestimation.
Inthepresentedscenarioswith5maliciousfeder‐atedclients,anuntargetedattackresultsinall5clients beingmaliciousduringthetraining,atargetedattack oncommonlabelsresultsinaround3clientsperform‐ingthejointattackonacertainlabel,whiletheattack onanuncommonlabelisdonebyonemaliciousclient, therefore,re lectingtheadaptationoftheattacking partytotheobservednon‐IIDdatadistribution.
Table1. Hyperparametersusedintheexperiments
Parameter
Totallabels 10 62 10
Totalclients 15 15 15
6.5.ModelsandHyperparameters
Convolutionalneuralnetworks(CNNs)werecho‐senfortheimageclassi icationtasksrepresentedin thedatasets.ThebasicLeNet5[39]architecturewas adoptedforbothMNISTandEMNISTtasks.ForCIFAR‐10amoresophisticatedarchitecturewaschosen, namely,mobilenetv2[40].Thepre‐trainedversionof themodelwasprovidedbythetorchvisionpackage withweightscomingfromtheImagenet[41]dataset.
Eachexperimentusedthecross‐entropylossfunc‐tionandtheStochasticGradientDescentoptimizer. Thefulllistofparametersforexperimentswith respecttodatasetsisgiveninTable1
Foreachalgorithm,dataset,andattacktype,the trainingwasperformed5timeswithdifferentmodel initializationscontrolledbyasetofseeds,andmean resultswereusedforfurtheranalysis.
6.6.ExperimentalResultsandAnalysis
ToevaluatetheAdFLalgorithm’sabilitytoiden‐tifymaliciousclientsandmitigatetheireffectinthe presenceofnon‐IIDdata,twowell‐knownalgorithms werechosenasbaselinesforevaluation.The irstone isMulti‐Krum[13]whichusestheEuclideandistance metricto ind �� closestmodelstouseforglobal modelaggregation,rejectingtherestoftheupdates collectedontheserverduringtheFLtraininground. Thisapproachfavorsmodelupdatesthataresimilar toeachotherandtreatsunusualupdatesasmalicious. Thesecondapproachtakenintocomparisonisthe FoolsGold(implementationfortheexperimentswas basedonthesourcecodeofthealgorithmprovidedby theauthors[18]).Thisapproachemploysadifferent strategyandusescosinesimilaritymeasuretoiden‐tifyclientswithsimilargradientupdatesandassigns themsmallerimportanceduringglobalmodelupdate. Thisalgorithmservesasanexampleofaweighting approachthatwasinitiallyevaluatedonnon‐IIDdata. Moreover,itisanexampleofadefensethatisnot suitedforuntargetedattacks[42].Therefore,thetwo selectedbaselinealgorithmspresenttwodifferent approachestodefenseagainstlabel‐ lippingattacks.
AstheAdFLalgorithmwasnotdesignedas adefenseagainstdatapoisoningandthecurrent researchaimsatextendingtheusageofgeneratedself‐adversarialsamplestotheFLsecuritydomain,these baselinemethodsservemoreasrepresentativesofthe algorithmsdesignedtodefendFLtrainingratherthan competitorsintermsofdefenseef iciency.
Itisimportanttonote,thattheMulti‐Krumalgo‐rithmexpectstheservertoknowbeforehandthenum‐berofpotentialmaliciousclientstakingpartineachFL traininground,asthisparametercontrolsthenumber ofupdatestobeeliminatedfromtheaggregationpro‐cess.Thisconditionwasful illedintheexperiments, andtheMulti‐Krumalgorithmwasempoweredwith theknowledgeoftheactualnumberofattackerswho performedthelabel lippingintheirlocaldata.As thisparameterissetforthewholelearningprocess anddoesnotadaptdependingonthesubsetofclients pickedforacertainFLiteration,itwasdecidedtoelim‐inatetheclient‐pickingstepfromtheexperiments, makingall15clientsalwaysparticipateineachtrain‐inground.Insuchcases,theMulti‐Krumalgorithm alwayshasachancetoeliminateallmaliciousclients andforweightingalgorithms(FoolsGoldandAdFL), theaggregationweightsforeachclientcanbetracked throughoutthewholetrainingprocessuninterrupt‐edly.
Duringallexperiments,theaggregationweights fortheclientaretrackedforeachepochreportedby re‐weightingalgorithms(AdFLandFoolsGold),while fortheMulti‐Krumalgorithm,theaggregationweights areassignedequallyfortheclientupdateschosen foraggregation,e.g.ifthereare �� chosenclientsfor aggregation,eachoftheclientsreceivesaggregation weightof 1 ��
Eachexperimentwasanalyzedwithrespecttothe meanaccuracyreachedbythealgorithminagiven scenarioandthemeanaggregationweightsthateach algorithmgavetothemalicious/benignclients.Mean valuesarecalculatedacrossall5repetitionsper‐formedforeachuniquealgorithm,dataset,andattack type.
The irstdatasetanalyzedwasMNIST.Theresults fortheuntargetedattackformodelaccuracyand meanbenign/maliciousclientaggregationweightsare showninFigure2.
AdFL:Benign FoolsGold:Benign Multi-Krum:Benign
AdFL:Malicious FoolsGold:Malicious
Figure3. MNISTtargetedattackonthecommonlabel
Figure4. MNISTtargetedattackonuncommonlabel
Hereitisvisible,thattheAdFLalgorithmscores irstinaccuracy,whiletheFoolsGoldalgorithm reachesfarloweraccuracy(68%and42.5%forthe AdFLandFoolsGoldalgorithmsrespectively).Among thepresentedalgorithms,Multi‐Krumgivesthehigh‐estweighttobenignclients,however,atthebegin‐ningoftraining,forthe irst10epochstheweightof maliciousclientswashigher.TheFoolsGoldalgorithm continuouslyfavorsmaliciousclients,whiletheAdFL algorithmmanagestoassignhigherweightstobenign clients.
Thecomparisonofthreealgorithmsforthetar‐getedattackonthecommonlabelontheMNIST datasetispresentedinFigure3
Itcanbeseen,thatbothAdFLandFoolsGoldalgo‐rithmsmanagetoreachaccuracyaround85%,while Multi‐Krumscoressigni icantlylower,despiteprop‐erlyfavoringbenignclientsduringmodelaggregation. Here,FoolsGoldshowsanotablechangeintheweight‐ingdynamic,withmaliciousclients irstscoringhigh‐estandthen,afterepoch53,switchingwithbenign clients.
Thecomparisonofthreealgorithmsforthetar‐getedattackontheuncommonlabelontheMNIST datasetispresentedinFigure4
TheplotillustratestheAdFLalgorithmreaching higheraccuracy,anditcanbeseen,thattheweight oftheonlymaliciousclientwasalsodifferentfrom thebenign,althoughthepreferencetowardsbenign clientsissmallerthanthoseoftheMulti‐Krumalgo‐rithm.Whatismore,inthepresentedscenario,the mechanismoftheFoolsGoldalgorithmfavoringthe uniqueupdatescanbeseeninaction,assigningthe highestweightstothemaliciousclient.
Figure5. EMNISTuntargetedattack
Figure6. EMNISTtargetedattackonthecommonlabel
Thecomparisonofthreealgorithmsfortheuntar‐getedattackontheEMNISTdatasetisillustratedin Figure5
BothMulti‐KrumandAdFLalgorithmssuccess‐fullyidentifymaliciousclients,whilefortheFoolsGold algorithm,ittakestimetostartcorrectlyre‐weighting clientsandthepositivebenignclientweighting dynamicvanishesasthetrainingprogressesafter epoch100.Still,Multi‐Krumscoreshigherinboth accuracyandbenignclients’weightasitmanagesto successfully ilteroutallmaliciousclients,whilethe AdFLalgorithmonlylowerstheirweights.
Thetargetedattackonthecommonlabelonthe EMNISTdatasetispresentedinFigure6.
Itisseen,thattheMulti‐Krumalgorithmmanages to ilteroutsomeofthemaliciousclients,butscores lowerinaccuracy,whiletheFoolsGoldalgorithmisnot abletoreliablyidentifythemaliciousclients.However, togetherwiththeAdFLalgorithmitreachesanaccu‐racyof60%,whiletheMulti‐Krumalgorithm–only 57.5%.TheAdFLalgorithmshowsaslightpreference forbenignclients,withbothmaliciousandbenign clientweightschanginginthenarrowrange.There‐fore,themeanandstandarddeviationvalueswere computedforthedifference(notabsolute)between aggregatedweightsassignedtobenignandmalicious clientsandarepresentedinTable2
Thetargetedattackontheuncommonlabelonthe EMNISTdatasetispresentedinFigure7.
Table2. Meanandstandarddeviationofthedifference betweenaggregationweightsfortargetedattackonthe commonlabelfortheEMNISTdataset Algorithm
Figure7. EMNISTtargetedattackontheuncommon label
Table3. Meanandstandarddeviationofthedifference betweenaggregationweightsfortargetedattackonthe uncommonlabelfortheEMNISTdatase Algorithm
Thisscenariowasthemostcomplexforallthree algorithmstodealwith.Astheclassi icationtask,in thiscase,consistsof62uniquelabels,andoneof therarestlabelswaspoisonedbyonlyoneadver‐sary,detectingthemaliciousclientwasnottrivial. Therefore,itcanbeseenthattheMulti‐Krumalgo‐rithmfailedto ilteroutthemaliciousclient,while theFoolsGoldalgorithm,asinthescenariowiththe targetedattackonthecommonlabel,failstoperform re‐weightingatall.AsfortheAdFLalgorithm,the luctuationsofweightscanbeobserved,however,the weightsarechangingwithinasmallrange(Table 3 statesthemeanandstandarddeviationforthediffer‐encebetweentheaggregationweightsofbenignand maliciousclients),moreover,thebenignclientsare beingcontinuouslyfavoredonlyafterepoch70.
Thecomparisonofthreealgorithmsfortheuntar‐getedattackontheCIFAR‐10datasetispresentedin Figure8
Intheobservedscenario,theMulti‐Krumalgo‐rithmmanagestocorrectlyidentifythemalicious clientsandscores irstinaccuracy,whileboththe FoolsGoldandtheAdFLalgorithmsshowsimilar loweraccuracyof32%comparedto66%fortheMulti‐Krumalgorithm.However,despiteloweraccuracy, theAdFLalgorithmstillproperlyre‐weightsclients, favoringbenignclientsfromthebeginningofthetrain‐ing,whentheFoolsGoldalgorithmprefersmalicious clients.
AdFL FoolsGold Multi-Krum
AdFL:Benign FoolsGold:Benign Multi-Krum:Benign
AdFL:Malicious FoolsGold:Malicious Multi-Krum:Malicious
Figure8. CIFAR‐10untargetedattack
AdFL FoolsGold Multi-Krum
AdFL:Benign FoolsGold:Benign Multi-Krum:Benign
AdFL:Malicious FoolsGold:Malicious Multi-Krum:Malicious
Figure9. CIFAR‐10targetedattackonthecommonlabel
AdFL FoolsGold Multi-Krum
AdFL:Benign FoolsGold:Benign Multi-Krum:Benign
AdFL:Malicious FoolsGold:Malicious Multi-Krum:Malicious
Figure10. CIFAR‐10targetedattackontheuncommon label
Thecomparisonofthreealgorithmsforthetar‐getedattackonthecommonlabelontheCIFAR‐10 datasetispresentedinFigure9.
Inthepresentedcase,itcanbeobserved,thatall threealgorithmsmanagetocorrectlyfavorbenign clientsandreachtheaccuracyof62%,59.5%,and 58%respectivelyfortheMulti‐Krum,FoolsGold,and AdFLalgorithms.
Thecomparisonofthreealgorithmsforthetar‐getedattackontheuncommonlabelontheCIFAR‐10 datasetispresentedinFigure10
Here,theMulti‐Krumalgorithmmanagesto ilter outthemaliciousclientinsomeexperiments,while theAdFLalgorithmonlydecreasestheweightofthe maliciousclientuntilepoch90andtheFoolsGoldalgo‐rithmcontinuouslyfavorsthemaliciousclient.
Table4. MeanASR(%)atthefinaltrainingepochfora targetedattackonthecommonlabel
Dataset
Table5. MeanASR(%)atthefinaltrainingepochfora targetedattackonuncommonlabel
Dataset Algorithm
Fortargetedattacks,ASRsforeachofthealgo‐rithmswerealsotrackedwithrespecttothehold‐outtestdatasetaccordingtoEquation 6.Thus,the meanASRoncommonanduncommonlabelsatthe endofthetrainingfortheMNIST,EMNIST,andCIFAR‐10datasetsareshowninTable4andTable5,respec‐tively.
ThereisanotabledifferenceintheASRbetween theMulti‐KrumalgorithmandtheAdFLandFoolsGold algorithmswhenitcomestotheCIFAR‐10dataset. Fortargetedattacksonbothcommonanduncom‐monlabels,theMulti‐Krumalgorithmreachessignif‐icantlylowerASR(under10%),whiletheothertwo algorithmsreachASRbetween25and93%.Forthe EMNISTdataset,allthreealgorithmsshowsimilar ASRregardlessoftheattacktarget.However,forthe MNISTdataset,thetargetedattackontheuncommon labelshowsanexceptionallyhighASRfortheFools‐Gold.
ThedynamicoftheASRchangefortheattackon thecommonlabelispresentedinFigure11
Here,thedifferencesintherangeofASRvalues isfurtherspeci iedwiththedynamicthroughoutall epochs,highlightingthatfortheMNISTdataset,the endofthetrainingalignedwiththelowestASR,while forbothEMNISTandCIFAR‐10datasets,theendofthe trainingyieldedahigherASR,withtheexceptionof Multi‐KrumalgorithmontheCIFAR‐10dataset.
ThechangesinASRsduringthescenarioswiththe targetedattackontheuncommonlabelarepresented inFigure12
ItisclearlyvisiblehowtheFoolsGoldalgorithm’s tendencytofavoruniqueupdatesimpactstheASR onallthreedatasets.Another indinghereillustrates thatalthoughtheEMNISTdatasethasarelativelylow absoluteASR,theASRgrowsdynamicallyastraining progresses,showcasinghowallthreealgorithmsfail todefendthemodelfromtargetedattacksregardless ofthetargetlabel.
ASRonthecommonlabelperalgorithmper dataset
Tosumup,theexperimentsshowthatthelabel skewcombinedwithdifferentlabel‐ lippingattacks presentsachallengingtaskforallthreealgorithms whencomparedtooneanother.However,itcanbe seen,thattheaggregationweightsgivenbytheAdFL algorithmtomaliciousandbenignclientsdifferin allexperimentsconducted,withbenignclientsbeing favoredbythealgorithm.Still,therangeofthediffer‐encebetweentheseweightsvariesdependingonthe datasetandattacktype.Moreover,experimentsonthe MNISTandCIFAR‐10datasetsshowthattheweights ofmaliciousandbenignclients,reportedbytheAdFL algorithm,tendtobecomeevenasthetrainingpro‐gresses,asmoremodelaggregationhappens,andthe accuracyoftheglobalmodelincreases.
Therefore,thesyntheticsamplesgeneratedby bothmaliciousandbenignclientsbecomesimilarand receivesimilarcoherencescores.Anotherobservation highlightsthattheASRsforalgorithmsdifferdepend‐ingonthedatasetandattacktype,withtheMulti‐KrumhavingthemoststablemeanASRacrossall datasetsandattacktargets,whileboththeAdFLand FoolsGoldalgorithmsshowedhighASRsfortheCIFAR‐10dataset,whileEMNISTdatasetwasthemostchal‐lengingdatasettoprotectfromthetargetedattack regardlessofthetargetlabelbeingcommonoruncom‐monamongfederatedclients.Still,theAdFLalgorithm showedanASRcomparablewiththeselecteddefense algorithms,despitenotbeingdesignedwithprotec‐tionfromdatapoisoningattacksinmind.
Figure12. ASRontheuncommonlabelperalgorithm perdataset
Table6. Wilcoxonsigned‐ranktestp‐valueand significance
Thesigni icanceoftheaggregationweightsdiffer‐enceprovidedbytheAdFLalgorithmwasadditionally assessedwiththehelpoftheWilcoxonsigned‐rank test[43]basedonthemeanaggregationweightsfor malicious/benignclientsinsideeachtrial,i.e,foreach repetitioninsidetheexperiment,themeanaggre‐gationweightswerecalculatedformaliciousand benignclientsacrossallepochsandusedasapair fortheWilcoxonsigned‐ranktest.Aseparatetestwas performedforalltrialsperformed,foreachdataset (regardlessoftheattacktype),andforeachattacktype (regardlessofthedataset).Theresultsarepresented inTable6
TheWilcoxonsigned‐ranktestrevealedasigni i‐cantdifferenceacrossallconsideredcombinationsof datasetsandattacktypes.However,itisseen,that thedifferencebetweenmaliciousandbenignclients aggregationweightsfortheEMNISTdatasetandfor targetedattacksonuncommonlabelsislesssignif‐icantthanintherestofthecases,whichhighlights thatfortheAdFLalgorithm,itishardertooperatein presenceofattacksaimedatuncommonlabelsand withintheclassi icationtaskswithabiggersetof uniquelabels.
Inthiswork,theapplicabilityofsyntheticadver‐sarialsampleswasexploredinthecontextofnon‐IIDdataanddatapoisoningattacks.Threetypesof attackswereperformedonthreebenchmarkimage classi icationdatasetsandtheresultswerecompared withrespecttoglobalmodelaccuracy,theabilityof thealgorithmstodistinguishmaliciousclientsfrom benign,andtheASRofthetargetedattacks.
Theresultsrevealedthatutilizingadversarialdata ontheserversideduringFLtrainingcansuccess‐fullyre‐weightmaliciousclientsandgivethemless importanceduringmodelaggregationforalluntar‐getedandtargetedattacks.However,themagnitudeof theweightdifferenceisnotsuf icienttofullymitigate thedamageperformedbythemaliciousclientsincom‐parisonwiththesecuritymethodsspeci icallycrafted tobattledatapoisoningattacksofcertaintypes.Still, astheAdFLalgorithmshowedtheabilitytofavor benignclientsovermaliciousonesduringtheexperi‐mentsconducted,futureresearchcanfurtherimprove theresultsbyensuringamorepowerfulweighting schemetopromoteagreaterin luenceoftheAdFL coherencemeasuresteponthemodelaggregation andverifytheAdFLalgorithmperformanceinmore populatedFLscenariosthatincludeclientpickingand introducemorediversedatadistributions.
AUTHOR
AnastasiyaDanilenka∗ –FacultyofMathematics andInformationScience,WarsawUniversityof Technology, Koszykowa75,00‐662Warsaw,Poland,e‐mail: anastasiya.danilenka.dokt@pw.edu.pl, www: orcid.org/0000‐0002‐3080‐0303.
∗Correspondingauthor
ThisworkwassupportedbytheCentreforPriority ResearchAreaArti icialIntelligenceandRoboticsof WarsawUniversityofTechnologywithintheExcel‐lenceInitiative:ResearchUniversity(IDUB)pro‐grammeandbytheLaboratoryofBioinformaticsand ComputationalGenomicsandtheHigh‐Performance ComputingCenteroftheFacultyofMathematicsand InformationScienceatWarsawUniversityofTechnol‐ogy.
[1] H.B.McMahan,E.Moore,D.Ramage,S.Hamp‐son,andB.A.yArcas.“Communication‐ef icient learningofdeepnetworksfromdecentralized data”,2017.
[2] X.Li,K.Huang,W.Yang,S.Wang,andZ.Zhang. “Ontheconvergenceoffedavgonnon‐iiddata”, 2020.
[3] T.‐M.H.Hsu,H.Qi,andM.Brown.“Measuring theeffectsofnon‐identicaldatadistributionfor federatedvisualclassi ication”,2019.
[4] X.Ma,J.Zhu,Z.Lin,S.Chen,andY.Qin, “Astate‐of‐the‐artsurveyonsolvingnon‐iid datainfederatedlearning”, FutureGeneration ComputerSystems,vol.135,2022,244–258, https://doi.org/10.1016/j.future.2022.05.003.
[5] R.Gosselin,L.Vieu,F.Loukil,andA.Benoit,“Pri‐vacyandsecurityinfederatedlearning:Asur‐vey”, AppliedSciences,vol.12,no.19,2022.
[6] P.ErbilandM.E.Gursoy,“Detectionand mitigationoftargeteddatapoisoningattacksin federatedlearning”.In: 2022IEEEIntlConfon Dependable,AutonomicandSecureComputing, IntlConfonPervasiveIntelligenceandComputing, IntlConfonCloudandBigDataComputing,Intl ConfonCyberScienceandTechnologyCongress (DASC/PiCom/CBDCom/CyberSciTech),2022, 1–8,10.1109/DASC/PiCom/CBDCom/Cy55231. 2022.9927914.
[7] A.Danilenka,“Mitigatingtheeffectsofnon‐iid datainfederatedlearningwithaself‐adversarial balancingmethod”, 202318thConferenceon ComputerScienceandIntelligenceSystems(FedCSIS),2023,925–930.
[8] Y.LeCunandC.Cortes,“MNISThandwrittendigit database”,2010.
[9] G.Cohen,S.Afshar,J.Tapson,andA.vanSchaik. “Emnist:anextensionofmnisttohandwritten letters”,2017.
[10] A.Krizhevsky.“Learningmultiplelayersoffea‐turesfromtinyimages”,2009.
[11] Z.Zhang,X.Cao,J.Jia,andN.Z.Gong,“Fldetector: Defendingfederatedlearningagainstmodelpoi‐soningattacksviadetectingmaliciousclients”. In: Proceedingsofthe28thACMSIGKDDConferenceonKnowledgeDiscoveryandDataMining,NewYork,NY,USA,2022,2545–2555, 10.1145/3534678.3539231.
[12] D.Li,W.E.Wong,W.Wang,Y.Yao,andM.Chau, “Detectionandmitigationoflabel‐ lipping attacksinfederatedlearningsystemswithkpca andk‐means”.In: 20218thInternational ConferenceonDependableSystemsand TheirApplications(DSA),2021,551–559, 10.1109/DSA52907.2021.00081.
[13] P.Blanchard,E.M.ElMhamdi,R.Guerraoui, andJ.Stainer,“Machinelearningwithadver‐saries:Byzantinetolerantgradientdescent”.In:
I.Guyon,U.V.Luxburg,S.Bengio,H.Wallach, R.Fergus,S.Vishwanathan,andR.Garnett,eds., AdvancesinNeuralInformationProcessingSystems,vol.30,2017.
[14] D.Cao,S.Chang,Z.Lin,G.Liu,andD.Sun, “Understandingdistributedpoisoningattack infederatedlearning”.In: 2019IEEE25th InternationalConferenceonParalleland DistributedSystems(ICPADS),2019,233–239, 10.1109/ICPADS47876.2019.00042.
[15] X.Cao,M.Fang,J.Liu,andN.Z.Gong.“Fltrust: Byzantine‐robustfederatedlearningviatrust bootstrapping”,2022.
[16] D.Yin,Y.Chen,K.Ramchandran,andP.Bartlett. “Byzantine‐robustdistributedlearning: Towardsoptimalstatisticalrates”,2021.
[17] C.Xie,O.Koyejo,andI.Gupta.“Generalized byzantine‐tolerantsgd”,2018.
[18] C.Fung,C.J.M.Yoon,andI.Beschastnikh, “Thelimitationsoffederatedlearninginsybil settings”.In: 23rdInternationalSymposium onResearchinAttacks,IntrusionsandDefenses (RAID2020),SanSebastian,2020,301–316.
[19] Y.Xie,W.Zhang,R.Pi,F.Wu,Q.Chen,X.Xie, andS.Kim.“Robustfederatedlearningagainst bothdataheterogeneityandpoisoningattackvia aggregationoptimization”,2022.
[20] S.Han,S.Park,F.Wu,S.Kim,B.Zhu,X.Xie, andM.Cha,“Towardsattack‐tolerantfederated learningviacriticalparameteranalysis”.In: ProceedingsoftheIEEE/CVFInternationalConferenceonComputerVision(ICCV),2023,4999–5008.
[21] S.Park,S.Han,F.Wu,S.Kim,B.Zhu,X.Xie,and M.Cha,“Feddefender:Client‐sideattack‐tolerant federatedlearning”.In: Proceedingsofthe29th ACMSIGKDDConferenceonKnowledgeDiscoveryandDataMining,NewYork,NY,USA,2023, 1850–1861,10.1145/3580305.3599346.
[22] C.Chen,Y.Liu,X.Ma,andL.Lyu.“Calfat:Cali‐bratedfederatedadversarialtrainingwithlabel skewness”,2023.
[23] G.Zizzo,A.Rawat,M.Sinn,andB.Buesser.“Fat: Federatedadversarialtraining”,2020.
[24] Z.Li,J.Shao,Y.Mao,J.H.Wang,andJ.Zhang. “Federatedlearningwithgan‐baseddatasynthe‐sisfornon‐iidclients”,2022.
[25] Y.Lu,P.Qian,G.Huang,andH.Wang.“Person‐alizedfederatedlearningonlong‐taileddatavia adversarialfeatureaugmentation”,2023.
[26] X.Li,Z.Song,andJ.Yang.“Federatedadversarial learning:Aframeworkwithconvergenceanaly‐sis”,2022.
[27] C.Szegedy,W.Zaremba,I.Sutskever,J.Bruna, D.Erhan,I.Goodfellow,andR.Fergus.“Intrigu‐ingpropertiesofneuralnetworks”,2014.
[28] O.Suciu,R.Marginean,Y.Kaya,H.D.III,and T.Dumitras,“WhendoesmachinelearningFAIL? generalizedtransferabilityforevasionandpoi‐soningattacks”.In: 27thUSENIXSecuritySymposium(USENIXSecurity18),Baltimore,MD,2018, 1299–1316.
[29] I.J.Goodfellow,J.Shlens,andC.Szegedy. “Explainingandharnessingadversarialexam‐ples”,2015.
[30] A.Kurakin,I.Goodfellow,andS.Bengio.“Adver‐sarialexamplesinthephysicalworld”,2017.
[31] Y.Dong,F.Liao,T.Pang,H.Su,J.Zhu,X.Hu,and J.Li.“Boostingadversarialattackswithmomen‐tum”,2018.
[32] G.Xia,J.Chen,C.Yu,andJ.Ma,“Poisoning attacksinfederatedlearning:Asurvey”, IEEEAccess,vol.11,2023,10708–10722, 10.1109/ACCESS.2023.3238823.
[33] A.Shafahi,W.R.Huang,M.Najibi,O.Suciu, C.Studer,T.Dumitras,andT.Goldstein,“Poison frogs!targetedclean‐labelpoisoningattackson neuralnetworks”.In: NeuralInformationProcessingSystems,2018.
[34] V.Shejwalkar,A.Houmansadr,P.Kairouz,and D.Ramage,“Backtothedrawingboard:Acriti‐calevaluationofpoisoningattacksonfederated learning”, ArXiv,vol.abs/2108.10241,2021.
[35] H.Xiao,H.Xiao,andC.Eckert,“Adversariallabel lipsattackonsupportvectormachines”.In: Proceedingsofthe20thEuropeanConferenceon Arti icialIntelligence,NLD,2012,870–875.
[36] V.Tolpegin,S.Truex,M.E.Gursoy,andL.Liu. “Datapoisoningattacksagainstfederatedlearn‐ingsystems”,2020.
[37] A.Paszke,S.Gross,F.Massa,A.Lerer,J.Bradbury, G.Chanan,T.Killeen,Z.Lin,N.Gimelshein, L.Antiga,A.Desmaison,A.Kopf,E.Yang, Z.DeVito,M.Raison,A.Tejani,S.Chilamkurthy, B.Steiner,L.Fang,J.Bai,andS.Chintala. “Pytorch:Animperativestyle,high‐performance deeplearninglibrary”.In: AdvancesinNeural InformationProcessingSystems32,8024–8035. CurranAssociates,Inc.,2019.
[38] S.MarcelandY.Rodriguez,“Torchvisionthe machine‐visionpackageoftorch”.In: Proceedingsofthe18thACMInternationalConference onMultimedia,NewYork,NY,USA,2010,1485–1488,10.1145/1873951.1874254.
[39] Y.Lecun,L.Bottou,Y.Bengio,andP.Haffner, “Gradient‐basedlearningappliedtodocument recognition”, ProceedingsoftheIEEE,vol.86,no. 11,1998,2278–2324,10.1109/5.726791.
[40] M.Sandler,A.Howard,M.Zhu,A.Zhmoginov,and L.‐C.Chen.“Mobilenetv2:Invertedresidualsand linearbottlenecks”,2019.
[41] J.Deng,W.Dong,R.Socher,L.‐J.Li,K.Li,andL.Fei‐Fei,“Imagenet:Alarge‐scalehierarchicalimage database”.In: 2009IEEEconferenceoncomputer visionandpatternrecognition,2009,248–255.
[42] L.Lyu,H.Yu,X.Ma,C.Chen,L.Sun, J.Zhao,Q.Yang,andP.S.Yu,“Privacyand robustnessinfederatedlearning:Attacks anddefenses”, IEEETransactionsonNeural NetworksandLearningSystems,2022,1–21, 10.1109/TNNLS.2022.3216981.
[43] F.Wilcoxon. Individualcomparisonsbyranking methods,196–202.Springer,1992.
Submitted:27th December2023;accepted:11th March2024
KarolinaBogacka,AnastasiyaDanilenka,KatarzynaWasielewska‑Michniewska DOI:10.14313/JAMRIS/3‐2024/18
Abstract:
Asthecomputationalandcommunicationalcapabilities ofedgeandIoTdevicesgrow,sodotheopportunitiesfor novelmachinelearning(ML)solutions.Thisleadstoan increaseinpopularityofFederatedLearning(FL),espe‐ciallyincross‐devicesettings.However,whilethereisa multitudeofongoingresearchworksanalyzingvarious aspectsoftheFLprocess,mostofthemdonotfocuson issuesconcerningoperationalizationandmonitoring.For instance,thereisanoticeablelackofresearchinthetopic ofeffectiveproblemdiagnosisinFLsystems.Thiswork beginswithacasestudy,inwhichwehaveintendedto comparetheperformanceoffourselectedapproachesto thetopologyofFLsystems.Forthispurpose,wehave constructedandexecutedsimulationsoftheirtraining processinacontrolledenvironment.Wehaveanalyzed theobtainedresultsandencounteredconcerningperi‐odicdropsintheaccuracyforsomeofthescenarios.We haveperformedasuccessfulreexaminationoftheexper‐iments,whichledustodiagnosetheproblemascaused byexplodinggradients.Inviewofthosefindings,wehave formulatedapotentialnewmethodforthecontinuous monitoringoftheFLtrainingprocess.Themethodwould hingeonregularlocalcomputationofahandpickedmet‐ric:thegradientscalecoefficient(GSC).Wethenextend ourpriorresearchtoincludeapreliminaryanalysisofthe effectivenessofGSCandaveragegradientsperlayeras potentiallysuitableforFLdiagnosticsmetrics.Inorderto performamorethoroughexaminationoftheirusefulness indifferentFLscenarios,wesimulatetheoccurrence oftheexplodinggradientproblem,vanishinggradient problemandstablegradientservingasabaseline.We thenevaluatetheresultingvisualizationsbasedontheir clarityandcomputationalrequirements.Weintroduce agradientmonitoringsuitefortheFLtrainingprocess basedonourresults.
Keywords: federatedlearning,explodinggradientprob‐lem,vanishinggradientproblem,monitoring
FederatedLearning(FL,[17,25])asaDistributed MachineLearning(DML)paradigmprioritizesmain‐tainingtheprivacyofthedevices(calledclients).It aimstodosobyleveragingthecomputingandcom‐municationalcapabilitiesoftheclients.AstandardFL trainingprocessbeginswiththeserverinitializinga machinelearning(ML)modelandsubsequentlycom‐municatingitsweightstotheclients.
Theclientsthenusethemtoconductlocaltraining andreturntheirresultstotheserver,wheretheyare aggregatedintoanewglobalmodel.Thewholepro‐cessrepeatsmultipletimesuntilstoppingcriteriaare met.
Asofnow,mostoftheMLmodelsusedforFLtrain‐ingare irstdesignedinacentralizedsetting,withthe developerhavingunrestrictedaccesstoarepresenta‐tivesampleoftheglobaldataset.Becauseofthat,they areabletoemployavarietyofpreexistingtechniques andtoolstomakesurethattheinitialmodelarchi‐tecturehasbeenoptimallyselected.Manyofthedata preprocessingstepsandhyperparametersdeveloped inthatinitialphaseformabaseforlaterFLtraining. Unfortunately,thiswork lowcanonlybeutilizedfor usecaseswheretherepresentativeglobaldatasetcan beconstructed,excludingsettingsthatdemandaddi‐tionalprivacyorjusthavelargelydistributed,heavily localizedandclient‐speci icdata.Inthatcase,theFL modeldevelopmentphasehastobeconductedina distributedenvironmentovermultipleruns,causing ittobepotentiallymuchslower.Distributedenvi‐ronmentsalsoinvolvetheunexpectedoccurrencesof otherpotentialhazardsintheformofsuddenclient dropoutanddifferingclientdatadistributions,caus‐ingthediagnosisofproblemssuchasvanishingor explodinggradientstobesigni icantlymoredif icult. Thisnecessitatesthedevelopmentofeffectivetoolsof FLsystemdiagnosis,forexamplethroughcontinuous monitoringofselectedmetrics.Asthisisaproblem thataffectsthedevelopmentandmaintenanceofFL systems,itcanbeunderstoodasbelongingtothe domainofFederatedLearningOperations(FLOps)[4], whichaimstoimprovetheFLlifecycleasawhole.
Wehaveconfrontedtheaforementionedissues duringourworkontheAssist‐IoTproject 1.Wehave conductedtrialstodeterminethemostsuitableFL topologytoimplementintheAssist‐IoTprojectpilots centeredaround:(1)constructionworkers’health andsafetyassurance,(2)vehicleexteriorcondition inspection[6].Morespeci ically,itwasimportantto providealightweightandscalablesystemforfall detectionofconstructionworkersinpilot1andauto‐maticvehicledetectioninpilot2.Inordertoascertain thebestFLtopologyforthepilots,wehaveconducted apreliminaryanalysisoftheissue[1]andselected4 especially“promising”approachesintheformofthe centralized,centralizedwithdynamicclusters,hierar‐chical,andhybridarchitectures.
Ourinitialsimulationshaveinsteadrevealedsome ofthoseapproaches(hierarchicalandhybrid)tobe especiallysensitivetotheexplodinggradientproblem, whichintheircasespresentsitselfasperiodicdrops inaccuracy.Theexplodinggradientproblemhereis de inedasasituationinwhichthegradientbackprop‐agationinneuralnetworktrainingincreasesexponen‐tially.Thiscausesthetrainingprocesstostall,with theresultingmodeldeterioratinginsomecases[31]. Wehaveappliedmodi icationstotheexperiment designinordertomitigatethisproblem.Wehavethen describedthewholeprocessasacasestudy.
Thisarticleisanextensionoftheresearchpre‐sentedintheconferencepaper[3].Weexpandthe theoreticalpartofthiswork,whichnowincludes moreinformationaboutthecurrentstateofFLOps withspecialimportancegiventothediagnostictools. Descriptionsofboththeexplodingandvanishinggra‐dientproblemsarebroadenedaswell,includingboth theircommoncausesandmitigationtechniques.A proposedgradientmonitoringmetricsuiteisdesigned bycombiningamodi iedversionofthepreviously proposedGradientScaleCoef icient(GSC)withthe newlyaddedaveragegradientperlayer.Theef icacy ofthesuiteistestedinthreesimulatedscenarios(van‐ishinggradient,explodinggradient,andbaseline)for twoselectedtopologies(centralizedandhierarchical). Resultsareanalyzed,bothinvestigatingtheclarityof thevisualizationsproducedbythesuiteaswellasits necessarycommunicationcost.
2.1.FederatedLearningOperations
FederatedLearningOperations(abbreviatedas FLOps)isacross‐disciplinesoftwaredevelopment methodology.Itsaimstoimprovetheef iciencyand qualityofthedevelopment,deployment,andmain‐tenanceprocessesofFLsystems[4].Assuch,FLOps extendstheprinciplesdevisedforthepurposesof MLOpsandDevOpsmethodologies,suchascontinu‐ousintegration,deploymentautomationandmodel monitoring[18]toFLenvironments.
Itisworthmentioningthatthede initionofFLOps formulatedin[4]refersonlytocross‐siloenviron‐ments.However,therearenoclearreasonsmen‐tionedwhyitcouldnotbeextendedtocross‐device settings.Onthecontrary,therearemanyexam‐plesofcross‐devicebusinessusecasessuchasthe Gboard[37].Althoughtheparticularactivitiescom‐posingtheFLOpslifecycleincross‐devicescenario maychange,involvinglessnegotiationsbetweenbusi‐nessentitiesanddatainterfaceformulationsthanin thecross‐siloenvironments,thescenariostillposes asigni icantchallengeintermsofautomationand operationalization.Thisworkwillfocusondiagnosing problemscausedbythegradientinstabilityincross‐deviceFLsystems.AseffectivesolutionsforFLdiag‐nosticsin luencetheef iciencyandqualityofFLdevel‐opment,itcanthereforebeconsideredascontributing totheresearchonFLOps.
Figure1. AsimplifieddiagramoftheFLOpsprocess flowsfrom[4]
TheinteractionbetweenvariousFLOps lowsis visualizedinFigure1.FDstandsforFederatedDesign, whichencompassestheprocessesofdataanalysisand modeldesign.FLmarksthe lowofFLtraining,and OPSindicatesthemaintenanceandmonitoringofFL solutionsdeployedinproduction.Eventhoughagiven FLdevelopmentprocessalwaysbeginswiththeFD phase,otherphasescan lexibly lowintoeachother basedontheresultsachievedatagivenstage.For example,amodelthatdoesnotperformwellmayindi‐catethenecessityofareturntoFD,andinsuf icient performancemetricsachievedduringOPSmaycause FLtorestart.
Ourresearchcanbeplacedattheintersectionof FDandFL,enablinganearliertransitionfromthe lattertotheformer.Itcanbethereforeunderstood asameansofoptimizingthewholework lowina holisticmanner.Movingbeyondtheideaofoptimiz‐ingasingulartrainingprocess,effectiveFLdiagnostic toolscanshortenthelengthofthewholefederated developmentprocess.
2.2.TheStateofFLDiagnosticTools
Asofnow,theresearchonFLsystemdiagno‐sisoftencentersaroundmonitoringtheclientsina secureandprivatemannerinordertoeffectivelydis‐tinguishthosethataremarkedbytheirexception‐allybadperformance[21][24][26].Asmuchasthe solutionspresentedintheaforementionedworksare interesting,theymaynotbesuf icienttoidentifyprob‐lemswithabadchoiceofhyperparametersormodel architecture.FedDebug[11]offersthemostcompre‐hensiveapproachofallofthosemonitoringframe‐works,enablingthedevelopertousemetricsgathered throughoutthetrainingtoreplaypreviousroundsor setbreakpoints.Thisisaneffectivesolutiontothe problemofrecognizingfaultyclients.
However,someworksinvolvingotheraspectsof diagnosingFLsystemscanalsobefound.[20]pro‐videsaworthwhilecontributiontotheproblemofFL modeldebuggingbydelineatinghowtheintegration ofinterpretablemethodsintoFLsystemsmayresult inapotentialsolution,makingitaverypromising researchdirection.[7],ontheotherhand,concen‐tratesonthesoftwareerrorsfrequentlyencountered bytheusersofselectedFLframeworks.Finally,Fed‐DNN‐Debugger[8]aspirestomitigatesomeofthe problemsaffectingFLmodels(biaseddata,noisydata, orinsuf icienttraining)byin luencingtheirlocalcom‐putation.Structurebugs,suchasinsuf icienttraining aswellasbiasedornoisydata,arebeyondthescopeof thissolution.Fed‐DNN‐Debuggercontainstwomod‐ules,withthe irstoneprovidingnon‐intrusivemeta‐datacapture(NIMC)andgeneratingdatathatisthen usedforautomatedneuralnetworkmodeldebugging (ANNMD).
2.3.TheExplodingGradientProblem
Theproblemofexplodinggradientiscausedby asituation,inwhichtheinstabilityofgradientval‐uesbackpropagatedthroughaneuralnetworkcauses themtogrowexponentially,aneffectthathasan especiallysigni icantin luenceontheinnermostlay‐ers[31].Thisproblemtendstogetoccurmoreoften themoredepthagivenMLarchitecturehas,forming anobstacleintheconstructionoflargernetworks. Additionally,explodinggradientproblemmayinsome casesbecausedbythewrongweightvalues,which tendtobene itfromnormalizedinitialization[12]. Whentalkingaboutactivationfunctions,theproblem maybeavoidedbyusingamodi iedLeakyReLUfunc‐tioninsteadoftheclassicReLUfunction.Thereason forthisbehaviourliesintheadditionofaleakyparam‐eter,whichcausesthegradienttobenon‐zeroeven whentheunitisnotactiveduetosaturation[27]. Anotherapproachtostabilizingneuralnetworkgradi‐ents(fortheexplodingaswellasthevanishinggradi‐entproblem)involvesgradientclipping.Theoriginal algorithmbehindgradientclippingsimplycausesthe gradienttoberescaledwhenevertheyexceedaset threshold,whichisbothveryeffectiveandcomputa‐tionallyef icient[29].
Thereareotherexistingtechniques,suchasweight scalingorbatchnormalization,whichminimizethe emergenceofthisproblem.Unfortunately,theyare notsuf icientinallcases[31].Somearchitectures,for instancefullyconnectedReLUnetworks,areresistant totheexplodinggradientproblembydesign[14]. Nonetheless,asthesearchitecturesarenotsuitable forallMLproblems,thismethodisnotauniversal solution.
Thereverseoftheexplodinggradientproblem,the vanishinggradientproblemisconsideredoneofthe mostimportantissuesin luencingthetrainingtime onmultilayerneuralnetworksusingthebackpropa‐gationalgorithm.Itappearswhenthethemajorityof theconstituentsofthegradientofthelossfunction approacheszero.Inparticular,thisproblemmostly involvesgradientlayersthataretheclosesttothe input,whichcausestheparametersoftheselayers tonotchangeassigni icantlyastheyshouldandthe learningprocesstostall.
Theincreasingdepthoftheneuralnetworkandthe useofactivationfunctionssuchassigmoidmakesthe occurrenceofthevanishinggradientproblemmore likely[32].Alongwiththesigmoidactivationfunction, thehyperbolictangentismoresusceptibletotheprob‐lemthanrecti iedactivationfunctions(ReLU),which largelysolvesthevanishinggradientproblem[13]. Finally,similarlytotheexplodinggradientproblem, theemergenceofthevanishinggradientproblemhas beenlinkedtoweightinitialization,withimprove‐mentsgainedfromaddingtheappropriatenormaliza‐tion[12].
2.5.AdvancesinResearchonTopologyofFederated Learning
Thedefault,centralizednetworktopologyusedfor aFLsystem,whichinvolvesasinglepowerfulcloud servercommunicatingwithafederationofclients locatedonedgeandIoTdevicesmaynotbethe mostsuitablesolutionforallusecases[35].Some requireef icientcommunication,whichmaybemore effectivelyprovidedbythesolutionsthathaveeither reducedtheimportanceoftheserverorremovedit alltogether[2].Othersfocusonleveragingnetwork topologytominimizeproblemscausebydatahetero‐geneity.Therealsothosethatattempttocombinethe twoapproachesdescribedabovebycarefullygrouping theclients[5].
[35]includesacatalogueofmanycommonly encounteredtrendsinresearchinvolvingFLtopol‐ogy,classifyingFLtopologytypesto 7 categories, includingcentralized[25],tree[28],hybrid[19],gos‐sip[16],grid[33],mesh[35],clique[2],andring[9]. Here,FederatedAveragingdescribedin[25]isan exampleofthecentralizedtopology.TornadoAggre‐gate,ontheotherhand,isunderstoodasbelonging tothehybridcategoryduetoitconstructingSTAR‐ringsandRING‐starsbycombiningstarandring topologies[19].STAR‐ringsindicatestheexistence ofaserver,whichperformsregularclientweight aggregationalongwithring‐basedgroups.RING‐stars constructsalargeglobalring,withsmallcentralized groupsconductinglocalcomputationandpassingit periodicallytoothergroupsinthechain.Outofthose two,STAR‐ringsreceivesmuchbetterperformance resultswhilemaintainingthesamescalability.
Somesystemscombinedifferenttopological approachesinordertocreateamoreresponsive system,thatcan,forinstance,adaptivelyrespond toproblemswithheterogenousdata.IFCA[10] integratesacentralizedtopologywithdynamic clusteringbyperiodicallygroupingtheclientsand simultaneouslytrainingapersonalizedmodelforeach oftheobtainedgroups.Unfortunately,asthismethod necessitatesawarmstarttothetrainingandprior knowledgeaboutthenumberofclustersnecessary, itleavessigni icantspaceforimprovement[15].This improvementcomesintheshapeofSR‐FCA,whichcan automaticallydeterminetherightamountofclusters, makingitmorerobustandresource‐ef icient.
Allinall,thereisawidevarietyofFLtopological approachesdevelopedtoprioritizedifferentaspects ofthesystem,suchasscalability,robustness,orpri‐vacy.Thesedeviationsinprioritiesmakethecompari‐sonofthoseapproachespeciallydif icult,astheyoften involvemodi icationsnotonlytothearchitecture,but totheclusteringoraggregationalgorithmsaswell. Moreover,itshouldbenotedthatmanyoftheworks involvingthetopicofFLtopologyfocusonalim‐itedrangeofexperimentsaimingtoachievethebest performance.Asaresult,furtherissuessuchasthe expressioncharacteristicsoftheexplodinggradient probleminselectedtopologiesandhowitdiffersfrom MLinmostcasesremainunexplored.
Ourinitialgoalforthecasestudywastoascertain thebesttopologyfortheAssist‐IoTpilotsaccording toourcriteriaofmaintainingthebestpossibleper‐formancewhileexposedtonegativefactorssuchas clientdropoutornon‐IIDclientdatadistribution.We havealsotakenintoaccounttheeaseofinfrastruc‐turesetupforagiventopologyandthescalability ofthewholesystem.Here,scalabilityinFLsystems isunderstoodasthecapabilitytomaintainstringent performancerequirementsinenvironmentsthatare massivelydistributed[39],thatis,containaverylarge numberofclients.
Toachievethisgoalwehaveselectedfourpromis‐ingsolutions,eachrepresentingadifferentapproach totheproblemand,therefore,allowingustoexam‐ineabroadrangeoftrends.Thetopologiesofthose solutionsarevisualizedinFigures 2, 3, 4,and 5 Theiraccompanyingdescriptionscanbefoundinsec‐tions3.1,3.2,3.3,and3.4,respectively.
AvisualizationoftheFLcentralizedtopology
AvisualizationoftheFLcentralizedtopology withdynamicclusters
AvisualizationoftheFLhierarchicaltopology
AvisualizationoftheFLhybridtopology
3.1.Centralized
Thecentralizedtopologyhasbeenintroduced alongwiththeconceptofFLasaparadigmin[25]. Thistopologyconsistsofaserver(whichsendsthe initialmodelparameterstotheclientsandperiodi‐callyaggregatestheirresultstoconstructanewglobal model)andmultipleclients(whichhandlelocalcom‐putation).Assuch,itisoftendistinguishedbyitsasym‐metricdata lowandinformationconcentrationon theserver,whichmayresultinpotentialrisksand unfairness[22].Asthecentralizedtopologyisoften consideredadefaultinFLsystems,wehaveincluded itasabaselineforcomparisonwithnewer,potentially morescalablesolutions.
Onthesurface,thecentralizedtopologywith dynamicclustersstronglyresemblesthecentralized topologyasdescribedinsection3.1.Ininvolvesperi‐odiccommunicationofasingularserverwithagroup ofclients,wheretheclientshandlemodeltrainingand theservermanagesweightaggregation.However,this topologyadditionallyincludesadynamiccomponent intheformofmultiplepersonalizedmodels(each ofthemodelsisdevelopedonlyforafractionofthe clients).
Moreover,theassignmentoftheclientstoapar‐ticularmodelvariesaswell.Itisrecomputedreg‐ularlybytheservertoensurethatitisstillopti‐mal.Theassignmentisbasedonweightsimilar‐ity,whichisestimatedusingtheEuclideandistance. Theweightsofeachmodelarethencomputedonly basedontheclientsassignedtoagivenclusterat thegivenmoment[15].Thisversionofthecentral‐izedtopologywithdynamicclustersincludesanaddi‐tionalimprovement.Asitisimplementedaccording totheSR‐FCA(SuccessiveRe ineFederatedClustering Algorithm),itusestheTrimmed‐mean‐basedGradient Descent[38]algorithminsteadofFederatedAverag‐ingforweightaggregation.AsTrimmed‐mean‐based GradientDescentexcludesoutliersfromaggregation, itsemploymentcausesthesystemtobemoreresis‐tanttoabnormalclientbehavioursuchasByzantine failure[38].SR‐FCAcanrespondtoenvironmental changesandadjusttovaryingclientdatadistributions withoutanypriorinformationaboutthenecessary numberofclustersnoradditionallocalcomputation. Italsoprovides lexiblepersonalization,automatically producingmultiplemodelsfordifferinggroupsof clients.However,itisimportanttomentionthatitmay notleadtoanincreaseinscalability.
3.3.Hierarchical
Hierarchicaltopology(describedastreetopology in[36])innovatesthepreviouslydescribedcentral‐izedtopologybyaddingathirdcategoryofdevice: theedgenode.Theedgenodesactasanintermediary serverbetweenthemainserverandclients,aggregat‐inglocalmodelweightsfromallclientsassignedto themaftereachiterationandsubsequentlypassing theaggregatedmodelsontotheservereachglobal round.Theserverthenaggregatestheedgeresults andformsanewmodel,thatislatercommunicatedto theclientsonlyfortheprocesstobeginagain[23].In ordertomaintainconvergence,theoveralldatadistri‐butionoftheclientsallottedtoeachedgenodeshould resembletheglobaldistributionasmuchaspossible. Thisassumptionismaintainedinoursimulationusing themethodfrom[28],whichadvisestodividegroups ofclientswithsimilardistributionsbetweenmultiple edgenodes.Wehavebeenmotivatedtoselectthehier‐archicaltopologyforourtrialsbyitscombinationof simplicityandscalability,asitmanagestosigni icantly reducethecommunicationalloadputontheserver whileavoidingcomputationally‐intensiveclustering algorithms.
Forour inalexampleofahybridtopologywe havedecidedtoexamineTornadoes(describedalso asSTAR‐ringsin[19]).Tornadoesintegratesacentral‐izedarchitecturewithlocalcomputationperformed insideparticularlyformedring‐basedgroups.After eachglobalroundtheclientsreceiveanewmodel fromtheserver.Theytrainitforaniterationandpass theresultstothenextclientintheirring.Inturn, theyreceiveanewmodelfromthepreviousclientin theirring,whichtheytrainandpassontothenext client.Thisprocessrepeatsofasetnumberoflocal iterations.Afterwards,theserveraggregatesalllocal modelsfromallclientsbelongingtoallrings,forming thenewmodelwhichislatercommunicatedtothe clients,lettingtheprocessrestart.Thishybridtopol‐ogyprovidesadditionalscalabilitytothesystemby decreasingcommunicationbetweentheserverand clientswithoutincreasingthenecessaryinfrastruc‐turetodoso.
Moreover,thedecisiontousedecentralizedlocal groupsinsteadofedgeserversmakesthesystemasa wholemorerobusttofailure.However,asring‐based groupswithhighvariancebetweenclientdistribu‐tionsarevulnerabletocatastrophicforgetting[19], theclientshavetobedividedintogroupsusingdedi‐catedalgorithms.Theinformationrequiredtodivide theclientsdiffersbetweenapproaches,withsome beingsigni icantlylessprivatethanothers.Theafore‐mentionedgroupingalgorithmsmayinsomecasesbe verycompute‐intensiveaswell.Allinall,inspiteof potentialdrawbacksvisibleinthedesignofTorna‐does,wehavedecidedtoinvestigatepotentialscala‐bilityincreaseitmightprovide.
WehaveelectedtousetheGermanTraf icSign RecognitionBenchmarkDataset[34]foroursimu‐lations,asitisbothlightweightandlessfrequently usedthanthedatasetsmentionedin[25],[15],[28], and[19]andwouldthereforeprovideacomple‐mentarysourceofinformationtotheresearchpre‐sentedinthoseworks.Thedatasethasbeendesigned foramulti‐class,single‐imageclassi icationchallenge organisedasapartoftheInternationalJointConfer‐enceonNeuralNetworksin 2011.Itconsistsof 43 distinctclassesandcontainsaglobaltestsetwith 12630 examplesandtrainingsetwith 39209 exam‐ples.Forthepurposeofourexperiments,thetraining sethasbeenshuf ledanddividedequallybetween 100clients,with80%ofeachclient’slocaldatabeing usedfortrainingand20%fortesting.Theglobaltest setwasusedtocomputemodelaccuracyeachround, whereasthelocaltestsetswereemployedtocalculate aggregatedaccuracy.Asawaytoreducethecompu‐tationalcostofthetrainingprocess,thedatasetasa wholehasbeenresizedto32by32pixels.
Theneuralnetworkarchitecturepreparedforthe simulationscontained 2 convolutionallayersand 1 denselayer.At irst,itutilizedtheAdamoptimizer withoutanygradientclipping,whichaftermodi ica‐tionschangedtogradientclippingforweightsexceed‐ingthevalueof 1.Inbothcases,thehyperparame‐tersusedincludedaninitiallearningrateof 0.001, ��1 of 0.9, ��2 of 0.999, �� of 10−7,batchsizeof 16, 25 globalrounds, 20 localiterations,andcategorical cross‐entropyusedasalossfunction.
Theclientswerenotgroupedatallfortheexper‐imentsinvestigatingthecentralizedtopology.The experimentshavebeenconductedfor25fullrounds, eachincludingeveryclienttrainingfor 20 iterations onlocaldatabeforesendingtheweightstotheserver. Theclientsthenreceivedthenewweightsinorderto computeonthemmetricssuchasaccuracyandloss, whichwerethenaggregatedontheservertoobtain aggregatedaccuracyandaggregatedloss,respectively. Theserveralsocalculatedglobaltestsetaccuracyand globaltestsetloss.
Theparametersusedforclusteringinsimulations conductedonthecentralizedtopologywithdynamic clustersincludedsizeparameter�� of3,�� of0.1have beenused,andthreshold��of5.Here,localtrainingon theclientshassimilarlylasted20localiterations,with clientreclusteringbeingperformedaftereach4global rounds.
Forthepurposesofhierarchicaltopologysimu‐lation 5 edgenodeshavebeenusedwith 20 clients assignedtoeach.Astheinclusionofedgenodesmin‐imizestheadditionalcommunicationalloadonthe server,amoreintensecommunicationprotocolhas beenusedforlocalcomputation.Toaccuratelyrecre‐atetheapproachpresentedin[28],aftereachofthe20 localiterationseveryclientcommunicateditsresult‐ingweightstoitsassignededgenodes,wherethey wereaggregatedandsentbacktotheclients.This processhasbeenrepeatedfor25globalrounds.
Inordertoaccuratelyreproducetheselected hybridtopologywhileminimizingthecomputational intensityofthesimulations,alloftheclientswere groupedinto 33 ringsofvaryinglengthusingthe algorithmdescribedin[19].Eachglobalroundhas contained 20 localiterations,withalloftheclients acceptingtheweightssentbythepreviousclientinthe ring,trainingthemforoneiteration,andpassingthem ontothenextclientinthering.Afterwards,theglobal modelisformedbyaggregatingallofthelocalmodels ontheserver.
Figure6showstheinitialtestresults.Twoofthe selectedtopologies,centralized(yellow)andcentral‐izedwithdynamicclusters(blue),convergedtoasat‐isfyingsolutionwithminordisturbances,whichcan‐notbesaidaboutaboutthehybridtopology(green) andhierarchicaltopology(purple).Aseachofthe experimentswasrepeatedthreetimestoformamore robust,smoothercurve,theperiodicdropsinaggre‐gatedaccuracyvisibleforthehierarchicaltopology cannotbeexplainedbytheexistenceofanoutlier. Instead,eachofthedropsoccursforadifferent run.Additionally,theresemblanceinresultsobtained forthecentralizedtopologytothoseforthecen‐tralizedwithdynamicclustersmaystemfromthe IIDclientdatadistributioninthesimulatedenviron‐ment.Intheseconditionsthecentralizedsolutionwith dynamicclusterstendstoformasingleclientgroup, behavingsimilarlytoaplaincentralizedtopology.
Figure6. Theaccuracytrainingcurvesforinitial experiments
Figure7depictsourfurtherinquiryintotheissue. Itvisualizesmeanaggregatedlossforeachclusterof clientsmeasuredaftereachlocaliterationforahier‐archicaltopologysimulation,whereaclustermeans alloftheclientsassignedtoagivenedgenode.In ordertoclearlydistinguishbetweentheclustersthey wereassigneddifferentcolors.Asuddenincreasein meanaggregatedlosscanbeobservedafteriteration 201 foroneoftheclusters,withsomeoftheother clustersexperiencingsimilarinstabilities.Theymay havebeencausedbythecombinationofanAdam optimizer,whichwasdesignedwiththeassumption ofacentralizedMLenvironment,withfrequentlocal communicationbetweentheclientandtheedgenode, whichcausesgradientinstabilities.Interestingly,the nextglobalaggregationiniteration 221 seemingly partiallymitigatestheissue.
Inordertoverifythatthedropsinaccuracyforthe hierarchicaltopologywerecausedbygradientinsta‐bilities,wehavedesignedamakeshiftmetric.Themet‐ricsubtractsweightsafterandbeforelocaltrainingin eachiterationforeveryclient,andthensumsupallof thosedifferences.Figure8visualizestheresults,with theassignmentofacolortoagivenclusterexactlythe sameasinFigure7.Thesuddenriseinthevaluesof themetricforeachoftheclusterscorrelatedwiththe increasingaggregatedloss,supportingthehypothesis aboutitbeingagradientexplosionproblem.
Figure7. Meanclusteraggregatedlossforhierarchical FLininitialexperiments
Figure8. ClientweightdifferencesforhierarchicalFLin initialexperiments
Havingformulatedaninitialexplanation,thetrain‐ingprocesshasbeenmodi iedtoincludegradientclip‐pingasasamplemethodforstabilizingthetraining. Subsequently,thetrialhasbeenrepeatedtoensure thatourexplanationhasbeensuf icient.Figures 10 and 9 bothshow indingsagreeingwiththisstate‐mentintheformofsmallerandmorestablevalues inthecaseofFigure10andnosudden,extremeloss increasesinthecaseofFigure9.
Figure11recreatesthe irsttrialwiththemodi ied trainingprocess,achievingamuchsmoothercurvefor allofthetopologies.Thehierarchicaltopology(pur‐ple)experiencesthemostvisibleimprovement,which indicatesittobepotentiallythemostvulnerabletothe problemofexplodinggradient.
Figure9. Meanclusteraggregatedlossforhierarchical FLinimprovedexperiments
Figure10. ClientweightdifferencesforhierarchicalFLin improvedexperiments
Figure11. Theaccuracytrainingcurvesforimproved experiments
6.PreliminaryMetricChoice
6.1.GradientScaleCoefficient
Inordertomaximizetheusefulnessoftheaddi‐tionalmetricscollectedthroughoutthetrainingin thediagnosticprocess,intheinitialworkwehave proposedcontinuousmonitoringofthegradientscale ofthelocalmodelsthroughtheregularcomputation ofthegradientscalecoef icientontheclients.The gradientscalecoef icientisde inedasfollows.
Itmeasurestherelativesensitivityoflayer�� with regardstorandomchangesinlayer ��,capturingthe sizeofthegradient lowingbackgroundrelativetothe sizeoftheactivationvaluesgrowingforward.
Adetailedexplanationofthismetricandhow touseittocanbefoundin[31].Itspracticality stemsfromitsrobustnesstonetworkscaling,which introducesthepossibilityofresultstandardization (althoughthevalidityofthispropertyneedstobe testedfurther,astheoriginalworkfocusedonlyon limitedneuralnetworkarchitectures).Additionally, theabilitytosummarizethedegreeinwhichthegra‐dientiscurrentlyvanishingorexplodingcouldcon‐tributetoaneffectivevisualizationforapotential futureusercomparingmultipleFLrunsonasingle plot.
Inourwork,weuseaversionofGSCmodi ied accordingtotheequationshownbelow:
7.1.ScenarioDescription
Tosimulatethethreescenariosofexplodinggra‐dients,vanishinggradients,andthatofthebaseline, appropriatemodi icationsareappliedtothemodel architectureandtrainingprocedureaccordingtothe theorypresentedinsections2.3and2.4
Thebaselinescenarioisanalogoustothecorrected modeldescribedinsection 4.Itconsistsoftwocon‐volutionallayersandonedenselayer.Theactivation functionusedforthetwoconvolutionallayersisLeaky ReLU.Theweightsoftheconvolutionallayersare initializedbytheGlorotUniforminitializer.Inthis example,theAdamoptimizerismodi iedtoinclude gradientclipping,withthethresholdforeachweight beingthatthenormofitsgradientdoesnotexceed1
Theexplodinggradientscenarioismodi iedto includeReLUasactivationfunctioninsteadofLeaky ReLU.Theweightsoftheconvolutionallayersare initializedusingtheuniformdistributionwithvalues rangingfrom 8 to 10.Additionally,thegradientclip‐pingmechanismisremoved.Asidefromtheaforemen‐tionedchanges,thearchitectureofthemodeldoesnot differfromthebaseline.
Asthearchitectureusedinourproblem(convolu‐tionalneuralnetwork)differedfromthearchitectures testedin[31](multilayerperceptron),theoriginal formuladidnotaccountforthedifferenceinlayer shapes.Weperformnecessarymodi icationsonthe GSCwhilekeepingitasclosetotheoriginalsolution aspossiblethroughchangingthetypeofthenorms usedfromsecondordertoFrobeniusandomittingthe bias.Inspiredbythediagramspresentedin[31],in ordertoextractasmuchinformationaspossiblewhile minimizingthecomputation,wedecidetocompute theGSConlyfortheinteractionbetweenthe irstand lastlayer.
Toaddasourceofinformationaboutspeci iclay‐erswhilemaximizingthefrugalityofourresulting metricsuite,wesetouttoincludetheaveragegradi‐entoftheweightsperlayer.Wedonotincludebias inourcomputations.Whilesimple,wesuspectthis informationtobebene icialincaseswhentheextent towhichaspeci iclayerisaffectedbythevanish‐ingorexplodinggradientproblemmayplayarole. Forinstance,whendeterminingtowhichdegreethe modelsuffersfromavanishinggradientproblem,it maybehelpfultoanalyzewhetherthegradientvalues remaincloseto0onlyforasinglelayer,ormultiplelay‐ersclosetotheinput.Inthisapproach,weareinspired by[12].Whereasthisworkusesstandarddeviation intervalsofweightgradientsperlayerintimetocheck whethertheirproposednormalizationaffectsthegra‐dientthroughouttraining,wecanfocusonthegeneral scaleofthegradientexpressedthroughtheaverage value.Utilizingthisapproachinsteadof,forinstance, gradienthistogramsperlayer,willallowustoclearly depictingthetimecomponentwhileminimizingthe communicationloadofthemetric.
Theweightsfortheconvolutionallayersinthe vanishinggradientscenarioareinitializedusingthe uniformdistributionwithvaluesrangingfrom4to7 Tanh,orhyperbolictangent,isusedastheactivation functionfortheconvolutionallayers.Thegradient clippingisremovedinthisscenarioaswell.Asidefrom that,therearenofurtherchangesappliedtothemodel inthisscenariocomparedtothebaseline.
7.2.MetricMeasurementandOtherModifications
ThealgorithmsforcomputingtheGSCandaverage gradientperlayerusedinthisworkaredescribed moreindepthinsection6.Here,wewillfocusonthe detailsofintegratingthealgorithmsintoFLtopolo‐gies.Inbothcentralizedandhierarchicaltopology, theGSCandaveragegradientperlayeriscomputed aftereachlocaliterationusingthewholetraining setoftheclientinordertofullycapturechanges inthegradientbroughtonbylocalcomputation.As thehierarchicalarchitectureinvolvestheexistenceof multiplelocaliterationspereachglobalround,we gathermetricsaftereachoftheseiterations.Then weanalyzethediagramsconstructedfromallofthe measurements,aswellasonlythosecomputedforthe lastiterationbeforeaglobalaggregationround.This actionisperformedinordertodeterminetheextent oftheinformationlossthatcouldresultfromthis muchmorecommunicationallyandcomputationally effectivescheme.
Thereisanopenpossibilityofintegratingthe computationofGSCandaveragegradientperlayer withthelocaltrainingbyusingthegradientsthatare alreadycomputedasapartofthetraining.Wedecide toavoiditduetotheassumptionspresentedin[31], whichreferredtothewholeavailabledatasetinstead ofjustabatch.However,thisopportunitymaystill beexploredinscenarioswithsmalllocaltrainingsets availableforeachclient.
Toensurethatweminimizetheimpactofrandom factorsontheresultsobtainedthroughexperiments, eachofthetrialshasbeenconductedthreetimes. The inalresultofaselectedtrialisameanofallof therunswithadditionalinformationincludedabout thedifferencesbetweenthem.Similarly,toincrease thequalityoftheresults,eachexperimenthasbeen conductedfor50globalroundsinsteadof25.Inorder tominimizetheamountofcomputationnecessary,we havedecidedtofocusontwoofthefourFLtopologies describedinthiswork‐thecentralizedtopology,as it’sthemostcommonlyusedandcanthereforeserve asaneffectivebaseline,andthehierarchicaltopology, asitisthemostvulnerabletotheexplodinggradient problemfromalloftheinitiallyinvestigatedexamples. Apartofthemodi icationsdescribedinthissection, theextendedexperimentsareconductedaccordingto section4
The irstpartofouranalysisfocusesonexamining thebehaviourofourscenariosandensuringthatit agreeswithourassumptionsembeddedintheexper‐imentdesign.Toaccomplishthis,welookatthetrain‐ingaccuracycurves.
Figures 12, 13,and 14 depictthetrainingpro‐cessconductedforthebaseline,explodinggradient, andvanishinggradientscenario,respectively.Inall threeexamples,theFLtopologyemployedwascen‐tralized.Inthe irst,baselinescenario,thedepicted curveissmoothandreachesanaggregatedaccuracy exceeding95%.InthesecondscenariodepictedinFig‐ure13,the inalaccuracyissigni icantlylower,witha jaggedcurveandmuchmorepronounceddifferences betweenrunsmarkedinthe igurebythelightblue color.
Thisindicatesinstabilityinherentintheexploding gradientscenario.Finally,thetrainingprocessvisual‐izedinFigure14isfullydysfunctional,withextreme variationsinaggregatedaccuracy,whichisneverthe‐lessunabletoexceedthethresholdof6%.Thismarksa scenariowithavanishinggradientproblemsosevere thatthemodelisfunctionallyunabletolearn.
Figure12. TheaccuracytrainingcurveforcentralizedFL inthebaselinescenario
Figure13. TheaccuracytrainingcurveforcentralizedFL intheexplodinggradientscenario
Figure14. TheaccuracytrainingcurveforcentralizedFL inthevanishinggradientscenario
Figures 15, 16,and 17 representsimilartraining processesforthehierarchicalFLtopology.Here,the traininginstabilitiesareevenmorepronouncedfor theexplodingandvanishinggradientscenarios(Fig‐ures 16 and 17),resultinginmuchmoreextreme changesinaccuracybetweentrainingroundsand runs.
Movingontotheanalysisofourmetricsuite,in Figure18wecanobservetheGSCvaluesforallthree scenariosreenactedinacentralizedFLarchitecture. Here,thevanishinggradientproblemismarkedby aGSCof 0 unchangingforthedurationoftheentire run.Thismarksisaseasilydistinguishablewithout anyadditionalknowledgeaboutthecurrenttraining accuracyofthegradientstabilityinotherscenarios.
Figure15. TheaccuracytrainingcurveforhierarchicalFL inthebaselinescenario
Figure16. TheaccuracytrainingcurveforhierarchicalFL intheexplodinggradientscenario
Figure17. TheaccuracytrainingcurveforhierarchicalFL inthevanishinggradientscenario
Figure18. Gradientscalecoefficient(GSC)valuesfor centralizedFLindifferentscenarios
Additionally,theGSCvaluesallowallthescenarios tobevisuallyidenti iablefromeachother,withthe scaleofthevaluesfortheexplodinggradientbeing visiblylargerthanforthebaseline.Thismaybeben‐e icialfortheiterativeprocessofconductingmultiple FLtrainingruns,asitwouldprovideadeveloperwith informationaboutthecurrentgradientscaleinthe contextofotherruns.Forexample,foradeveloper seekingto ixanexplodinggradientproblemandtest‐ingapotentialsolutionitwouldbehelpfultoknowthe scaleoftheGSCwhencomparedtopreviousruns.
Unfortunately,itisnotobviouswhethertheGSCof asinglerunwouldbesuf icienttodiagnoseitassuffer‐ingfromavanishingorexplodinggradientproblem. Thereissomediscussionaboutitbeingpotentially truein[31].However,astheaforementionedwork focusesonthemultilayerperceptronarchitecture,fur‐therresearchinvolvingothermodelarchitecturesis stillnecessary.
Figure19. Gradientscalecoefficient(GSC)valuesfor hierarchicalFLindifferentscenarios
Figure20. Gradientscalecoefficient(GSC)values measuredforthelastlocaliterationforhierarchicalFLin differentscenarios
Figure19showcasesGSCvaluesfordifferenttest scenariosreenactedinahierarchicalFLsystem.The GSCintheseexperimentsissimilartoanalogous resultsforthecentralizedtopologybothinscaleand clearseparabilitybetweenscenarios.Additionally,the GSCdepictedinFigure 19 seemstomaintaintraits speci ictothehierarchicaltopology,suchasperiodic changesingradientcausedbyglobalweightaveraging andspecialvulnerabilitytogradientinstabilitiesvisi‐bleforthebaselinescenario.
Figure20depictsasimpli iedversionoftheprevi‐ousdiagramcontainingonlytheGSCvaluesmeasured afterthelastlocaliterationbeforetheglobalcompu‐tationround.ItcanbenotedthatFigure20effectively preservesmostinformationfromFigure19,including thescaleandstabilityofGSCforagivenscenario, omittingonlythevisibleindicatorsofperiodicity.
Figure21. Averagegradientvalueperlayerfor centralizedFLinthebaselinescenario
Figure22. Averagegradientvalueperlayerfor centralizedFLintheexplodinggradientscenario
Figure23. Averagegradientvalueperlayerfor centralizedFLinthevanishinggradientscenario
Figures21,22,and23presenttheaveragegradient valueforagivenlayerforexperimentsconductedona centralizedFLsystem.Thelayersarenumberedfrom theinputtotheoutput,marking1asthelayerclosest totheinputand 3 asthelayerclosesttotheoutput. Thisisasimpleyeteffectivevisualization,asitallows theviewertoeasilycomparegradientvaluesbetween layers.
InFigure 21 (baselinescenario),thismeansthat averagegradientvaluesperlayerarebothrelatively lowandclosetoeachother.Althoughtheaverage gradientvalueforlayer1isoftenlargerthanforvalue 3,layer 1 frequentlyshiftsandintersectswithlayer 3.WecancontrastitwithFigure 21 (explodinggra‐dientscenario),wheretheaveragegradientoflayer 1 isnoticeablygreaterthanlayer 2 and 3 witha largedifferenceinscale.Figure22(vanishinggradient scenario)marksatrainingprocesswherelayers 1 and2areextremelycloseto0throughoutthewhole training,withthevaluesoflayer 3 varyinglargely betweeniterationsandruns.Thesecleardifferences inplotsindicate,thataveragegradientvaluesperlayer asametricmaybeenoughtoeffectivelyrecognizean explodingorvanishinggradientprobleminFLsys‐tems.
Figures24,25,and26moveontodepictingaver‐agegradientvaluesperlayerforthehierarchical topology.Here,theresultsaresimilartothescenarios simulatedforthecentralizedsystem,ifnotablyless readableduetoalargeramountoflocaliterations (andthereforealsogradientmeasurements).Interest‐ingly,periodicdropsintheaveragegradientofthe irst layerdepictedinFigure25seemtocon irmourprior suspicionaboutglobalweightaggregationservingas aformofregularization.
Figures 27, 28,and 29 areverysimilartoFig‐ures 24, 25,and 26,withtheonlydifferencebeing theamountofinformationdepicted.Figures 27, 28, and29containonlytheaveragegradientvaluesmea‐suredperlayerafterthelastlocaliterationineach round.Interestingly,theselimitationsseemtoin lu‐encethevisualizationspositively.Theplotsarenow easiertoread,withFigure 27 depictingaveragegra‐dientsthataremoreclearlysimilarinscale.Theonly importantinformationlostistheperiodicityinFig‐ure 28,whichisnotnecessarytodetermineittobe anexampleoftheexplodinggradientastheaverage valuesoflayer1remainvisiblylargerfromlayer3
The inalgradientscalemonitoringframework thereforeincludestheGSCvisualization(asshown, forinstance,inFigure 20)toenableeasyandread‐ablegradientscalecomparisonformultiplerunsof thesystem,aswellastheaveragegradientperlayer, whichprovidessimplegradientproblemdiagnosisfor particularruns.TheGSCshouldbedisplayedusing thelogarythmicscale.Tominimizeadditionalcompu‐tationalloadcausedbythemonitoring,themetrics shouldbecomputedonlyforthelastlocaliteration incentralizedtopologieswithlocalgroups(suchas hierarchicalorhybrid).
Eventhoughthereisplentyofresearchfocusedon manydifferentaspectsoftheFLparadigm,weiden‐tifyapotentialgapinthetopicofeffectivediagnosis andmonitoringofFLsystems.Forinstance,ourini‐tialexperimentsshowcasehowdifferentlyaproblem commonlyencounteredinMLmaypresentinmore sophisticatedFLtopologies.
Figure24. Averagegradientvalueperlayerfor hierarchicalFLinthebaselinescenario
Figure25. Averagegradientvalueperlayerfor hierarchicalFLintheexplodinggradientscenario
Figure26. Averagegradientvalueperlayerfor hierarchicalFLinthevanishinggradientscenario
Weproposeandtestapotentialmonitoringframe‐workdesignedfortheearlydetectionofsuchissues. Wecon irmitsabilitytoenableeasydifferentiation betweenscenariosofvanishing,exploding,andstable gradientincentralizedandhierarchicalFLsystems withtheassumptionofIIDdata.Alongwithananal‐ysisfocusedonthevisualclarityofourresults,we investigatethepossibilityofamorecommunication‐allyandcomputationallyef icientapproachbyinclud‐ingonlythemeasurementsconductedafterthelast localiteration.
Figure27. Averagegradientvalueperlayermeasured forthelastlocaliterationforhierarchicalFLinthe baselinescenario
Figure28. Averagegradientvalueperlayermeasured forthelastlocaliterationforhierarchicalFLinthe explodinggradientscenario
Figure29. Averagegradientvalueperlayermeasured forthelastlocaliterationforhierarchicalFLinthe vanishinggradientscenario
Basedonthat,weintroduceajointtoolincluding themeasurementofGSCandaveragegradientper layertoenablelightweightandcomprehensivegradi‐entmonitoring.WeincludeGSCtoenableeasyvisu‐alizationofrelativegradientstabilityinthecontextof previousruns,aswellasaveragegradientperlayerto allowade initediagnosisofthecurrentrunasaffected bytheproblemofvanishingorexplodinggradients.In thecaseofhierarchicalFL,bothshouldbecomputed onlyforthelastlocaliterationeachround.
Ourworkaf irmstheneedtofurtherexaminethe ef icacyofexistingtoolsdesignedformonitoringin diagnosticsinFLsystemsduetotheircomplex,dis‐tributednatureanduniqueproblemssuchasclient dropoutordivergingclientdistributions.Aninterest‐ingresearchareawewouldliketoshedlightoncan alsobefoundintestingtoolslikethemetricsuite describedinthisworkinenvironmentssimulating varying,heterogenoussetsofobstacles,includingthe aforementionedclientdropout,differencesinlocal datadistribution,andbadhyper‐parameterselection. Futureworkcanalsoconsidertheinclusionofother potentiallysuitablemetrics,suchastheNonlinearity Coef icient[30],whichisanevolutionoftheGradient ScaleCoef icient.
Notes
1https://assist‐iot.eu
AUTHORS
KarolinaBogacka∗ –WarsawUniversityofTechnol‐ogy,PlacPolitechniki1,00‐661Warszawa,Poland, e‐mail:karolina.bogacka.dokt@pw.edu.pl. AnastasiyaDanilenka –WarsawUniversityofTech‐nology,PlacPolitechniki1,00‐661Warszawa,Poland, e‐mail:anastasiya.danilenka.dokt@pw.edu.pl. KatarzynaWasielewska-Michniewska –Systems ResearchInstitute,PolishAcademyofSciences, Newelska6,01‐447Warszawa,Poland,e‐mail: katarzyna.wasielewska@ibspan.waw.pl.
∗Correspondingauthor
TheworkofKalinaBogackaandAnastasiyaDanilenka wasfundedinpartbytheCentreforPriorityResearch AreaArti icialIntelligenceandRoboticsofWarsaw UniversityofTechnologywithintheExcellenceInitia‐tive:ResearchUniversity(IDUB)programme.
References
[1] IntroducingFederatedLearningintoInternetof Thingsecosystems–preliminaryconsiderations, 072022.
[2] A.Bellet,A.Kermarrec,andE.Lavoie,“D‐cliques:Compensatingnoniidnessindecentral‐izedfederatedlearningwithtopology”, CoRR,vol. abs/2104.07365,2021.
[3] K.Bogacka,A.Danilenka,andK.Wasielewska‐Michniewska,“Diagnosingmachinelearning problemsinfederatedlearningsystems:A casestudy”.In:M.Ganzha,L.Maciaszek, M.Paprzycki,andD.Ślęzak,eds., Proceedings ofthe18thConferenceonComputerScienceand IntelligenceSystems,vol.35,2023,871–876, 10.15439/2023F722.
[4] Q.ChengandG.Long,“Federatedlearning operations( lops):Challenges,lifecycle andapproaches”.In: 2022International
ConferenceonTechnologiesandApplications ofArti icialIntelligence(TAAI),2022,12–17, 10.1109/TAAI57707.2022.00012.
[5] L.Chou,Z.Liu,Z.Wang,andA.Shrivastava,“Ef i‐cientandlesscentralizedfederatedlearning”, CoRR,vol.abs/2106.06627,2021.
[6] A.‐I.Consortium.“D7.2PilotScenarioImplemen‐tation–FirstVersion”,2022.
[7] X.Du,X.Chen,J.Cao,M.Wen,S.‐C.Cheung, andH.Jin,“Understandingthebugcharacteris‐ticsand ixstrategiesoffederatedlearningsys‐tems”.In: Proceedingsofthe31stACMJoint EuropeanSoftwareEngineeringConferenceand SymposiumontheFoundationsofSoftwareEngineering,NewYork,NY,USA,2023,1358–1370, 10.1145/3611643.3616347.
[8] S.Duan,C.Liu,P.Han,X.Jin,X.Zhang,X.Xiang, H.Pan,etal.,“Fed‐dnn‐debugger:Automatically debuggingdeepneuralnetworkmodelsinfeder‐atedlearning”, SecurityandCommunicationNetworks,vol.2023,2023.
[9] H.Eichner,T.Koren,H.B.McMahan,N.Srebro, andK.Talwar,“Semi‐cyclicstochasticgradient descent”, CoRR,vol.abs/1904.10120,2019.
[10] A.Ghosh,J.Chung,D.Yin,andK.Ramchandran. “Anef icientframeworkforclusteredfederated learning”,2021.
[11] W.Gill,A.Anwar,andM.A.Gulzar.“Fedde‐bug:Systematicdebuggingforfederatedlearn‐ingapplications”,2023.
[12] X.GlorotandY.Bengio,“Understandingthedif‐icultyoftrainingdeepfeedforwardneuralnet‐works”.In:Y.W.TehandM.Titterington,eds., ProceedingsoftheThirteenthInternationalConferenceonArti icialIntelligenceandStatistics, vol.9,ChiaLagunaResort,Sardinia,Italy,2010, 249–256.
[13] F.Godin,J.Degrave,J.Dambre,andW.DeNeve, “Dualrecti iedlinearunits(drelus):Areplace‐mentfortanhactivationfunctionsinquasi‐recurrentneuralnetworks”, PatternRecognition Letters,vol.116,2018,8–14.
[14] B.Hanin,“Whichneuralnetarchitecturesgive risetoexplodingandvanishinggradients?”.In: S.Bengio,H.Wallach,H.Larochelle,K.Grauman, N.Cesa‐Bianchi,andR.Garnett,eds., Advancesin NeuralInformationProcessingSystems,vol.31, 2018.
[15] Harshvardhan,A.Ghosh,andA.Mazumdar. “Animprovedalgorithmforclusteredfederated learning”,2022.
[16] I.Hegedűs,G.Danner,andM.Jelasity,“Gossip learningasadecentralizedalternativetofeder‐atedlearning”.In:J.PereiraandL.Ricci,eds., DistributedApplicationsandInteroperableSystems, Cham,2019,74–90.
[17] L.U.Khan,W.Saad,Z.Han,E.Hossain,and C.S.Hong,“Federatedlearningforinternetof
things:Recentadvances,taxonomy,andopen challenges”, CoRR,vol.abs/2009.13012,2020.
[18] D.Kreuzberger,N.Kühl,andS.Hirschl,“Machine learningoperations(mlops):Overview,de ini‐tion,andarchitecture”, IEEEAccess,vol.11,2023, 31866–31879,10.1109/ACCESS.2023.3262138.
[19] J.Lee,J.Oh,S.Lim,S.Yun,andJ.Lee,“Tornadoag‐gregate:Accurateandscalablefederatedlearn‐ingviathering‐basedarchitecture”, CoRR,vol. abs/2012.03214,2020.
[20] A.Li,R.Liu,M.Hu,L.A.Tuan,andH.Yu,“Towards interpretablefederatedlearning”, arXivpreprint arXiv:2302.13473,2023.
[21] A.Li,L.Zhang,J.Wang,F.Han,andX.‐Y.Li, “Privacy‐preservingef icientfederated‐learning modeldebugging”, IEEETransactionsonParallel andDistributedSystems,vol.33,no.10,2022, 2291–2303,10.1109/TPDS.2021.3137321.
[22] Q.Li,Z.Wen,Z.Wu,S.Hu,N.Wang,Y.Li,X.Liu, andB.He,“Asurveyonfederatedlearningsys‐tems:Vision,hypeandrealityfordataprivacy andprotection”, IEEETransactionsonKnowledge andDataEngineering,vol.35,no.4,2023,3347–3366,10.1109/TKDE.2021.3124599.
[23] L.Liu,J.Zhang,S.Song,andK.B.Letaief,“Edge‐assistedhierarchicalfederatedlearningwith non‐iiddata”, CoRR,vol.abs/1905.06641,2019.
[24] Y.Liu,W.Wu,L.Flokas,J.Wang,andE.Wu, “Enablingsql‐basedtrainingdatadebuggingfor federatedlearning”, CoRR,vol.abs/2108.11884, 2021.
[25] H.B.McMahan,E.Moore,D.Ramage,and B.A.yArcas,“Federatedlearningofdeep networksusingmodelaveraging”, CoRR,vol. abs/1602.05629,2016.
[26] L.Meng,Y.Wei,R.Pan,S.Zhou,J.Zhang,and W.Chen,“Vadaf:Visualizationforabnormal clientdetectionandanalysisinfederatedlearn‐ing”, ACMTrans.Interact.Intell.Syst.,vol.11,no. 3–4,2021,10.1145/3426866.
[27] M.A.MercioniandS.Holban,“Themostused activationfunctions:Classicversuscurrent”.In: 2020InternationalConferenceonDevelopment andApplicationSystems(DAS),2020,141–145.
[28] N.Mhaisen,A.A.Abdellatif,A.Mohamed, A.Erbad,andM.Guizani,“Optimaluser‐edge assignmentinhierarchicalfederatedlearning basedonstatisticalpropertiesandnetwork topologyconstraints”, IEEETransactionson
NetworkScienceandEngineering,vol.9,no.1, 2022,55–66,10.1109/TNSE.2021.3053588.
[29] R.Pascanu,T.Mikolov,andY.Bengio,“Onthe dif icultyoftrainingrecurrentneuralnetworks”. In: Internationalconferenceonmachinelearning, 2013,1310–1318.
[30] G.Philipp,“Thenonlinearitycoef icient‐aprac‐ticalguidetoneuralarchitecturedesign”, arXiv preprintarXiv:2105.12210,2021.
[31] G.Philipp,D.Song,andJ.G.Carbonell.“The explodinggradientproblemdemysti ied‐de i‐nition,prevalence,impact,origin,tradeoffs,and solutions”,2018.
[32] M.Roodschild,J.GotaySardiñas,andA.Will,“A newapproachforthevanishinggradientprob‐lemonsigmoidactivation”, ProgressinArti icial Intelligence,vol.9,no.4,2020,351–360.
[33] Y.Shi,Y.E.Sagduyu,andT.Erpek.“Feder‐atedlearningfordistributedspectrumsensingin nextgcommunicationnetworks”,2022.
[34] J.Stallkamp,M.Schlipsing,J.Salmen,andC.Igel, “Manvs.computer:Benchmarkingmachine learningalgorithmsfortraf icsignrecognition”, NeuralNetworks,vol.32,2012,323–332, https://doi.org/10.1016/j.neunet.2012.02.016, SelectedPapersfromIJCNN2011.
[35] J.Wu,S.Drew,F.Dong,Z.Zhu,andJ.Zhou. “Topology‐awarefederatedlearninginedge computing:Acomprehensivesurvey”,2023.
[36] J.Wu,S.Drew,F.Dong,Z.Zhu,andJ.Zhou, “Topology‐awarefederatedlearninginedge computing:Acomprehensivesurvey”, arXiv preprintarXiv:2302.02573,2023.
[37] T.Yang,G.Andrew,H.Eichner,H.Sun,W.Li, N.Kong,D.Ramage,andF.Beaufays.“Applied federatedlearning:Improvinggooglekeyboard querysuggestions”,2018.
[38] D.Yin,Y.Chen,R.Kannan,andP.Bartlett, “Byzantine‐robustdistributedlearning: Towardsoptimalstatisticalrates”.In:J.Dy andA.Krause,eds., Proceedingsofthe35th InternationalConferenceonMachineLearning, vol.80,2018,5650–5659.
[39] M.Zhang,E.Wei,andR.Berry,“Faithful edgefederatedlearning:Scalabilityand privacy”, IEEEJournalonSelectedAreasin Communications,vol.39,no.12,2021,3790–3804,10.1109/JSAC.2021.3118423.
CLASSIFICATION:ANEVALUATION
Submitted:9th December2023;accepted:26th March2024
MichałKassjański,MarcinKulawiak,TomaszPrzewoźny,DmitryTretiakow,JagodaKuryłowicz,AndrzejMolisz, KrzysztofKoźmiński,AleksandraKwaśniewska,PaulinaMierzwińska‑Dolny,MiłoszGrono
DOI:10.14313/JAMRIS/3‐2024/19
Abstract:
Theevaluationofhearinglossisprimarilyconductedby puretoneaudiometrytesting,whichisoftenregarded asthegoldstandardforassessingauditoryfunction. Thismethodenablesthedetectionofhearingimpair‐ment,whichmaybefurtheridentifiedasconductive, sensorineural,ormixed.Thisstudypresentsacompre‐hensivecomparisonofavarietyofAIclassificationmod‐els,performedon4007puretoneaudiometrysamples thathavebeenlabeledbyprofessionalaudiologistsin ordertodevelopanautomaticclassifierofhearingloss type.Thetestedmodelsincluderandomforest,support vectormachines,logisticregression,stochasticgradient descent,decisiontrees,convolutionalneuralnetwork (CNN),feedforwardneuralnetwork(FNN),recurrentneu‐ralnetwork(RNN),gatedrecurrentunit(GRU)andlong short‐termmemory(LSTM).Thepresentedworkalso investigatestheinfluenceoftrainingdatasetaugmenta‐tionwiththeuseofaconditionalgenerativeadversarial networkontheperformanceofmachinelearningalgo‐rithms,andexaminestheimpactofvariousstandard‐izationproceduresontheeffectivenessofdeeplearning architectures.Overall,thehighestclassificationperfor‐mancewasachievedbyLSTM,withanout‐of‐training accuracyof97.56%.
Keywords: classification,hearinglosstypes,pure‐tone audiometry,RNN,LSTM,evaluation
Hearingisregardedasavitalsensoryorgan,asit furnishesuswithcrucialinsightsintooursurround‐ings.Itenhancesourperceptionoftheenvironmentby complementingourvisualandtactilesenses,thereby facilitatinganextensivecomprehensionofourenvi‐ronments.Furthermore,possessingadequateaudi‐toryperceptionallowsustoengageineffectivecom‐munication,maintainoursafety,andreceivegrati ica‐tionfromadiverserangeofaudioactivities,suchaslis‐teningtomusicorwatchingtheatricalperformances. Inconsequence,hearinglosshaswide‐rangingand signi icantconsequences,whichencompass,interalia, theinabilitytoengageincommunicationwithothers, aswellasadelayintheacquisitionoflanguageskills inyoungsters.
Thiscanresultinsocialisolation,whichin turnmayleadtofeelingsoflonelinessandfrustra‐tion,especiallyinelderlyindividualsexperiencing impairedhearing.Accordingtodatapresentedbythe WorldHealthOrganization(WHO),thecurrentglobal prevalenceofhearinglossaffectsmorethan1.5billion people,ofwhich430millionsufferfrommoderateto severehearinglossintheirsuperiorear.Asstated bytheWHO,itisprojectedthatby2050,almost2.5 billionindividualswouldexperiencevaryinglevelsof hearingimpairment,andatleast700millionofthem willneedrehabilitationtreatments[1].Atthesame time,however,WHOalsoclaimsthatalmosthalfofall casesofhearinglosscanbeavoidedbyimplementing publichealthinterventions.Additionalreductionsin hearingimpairmentcanbeachievedbyconducting screeningsandimplementingearlyinterventionsdur‐ingchildhood,suchasutilizingassistivedevicesor consideringsurgicalalternatives.
Theevaluationofhearinglossisprimarilycon‐ductedbypuretoneaudiometrytesting,whichhas beenconsideredasthemostdependableapproachfor assessingauditoryfunction.Theprocedureinvolves presentingpuretonesatspeci icfrequencies,either throughheadphones(airconduction)orbyusinga vibratorplacedonthemastoidsectionofthetemporal bone(boneconduction).Theobjectiveisto indthe lowestlevelatwhichtheindividualcanperceivethe sound,knownasthethreshold,foreachfrequency [2].Theresultsofahearingtestarepresentedonan audiogram,whichallowsfortheidenti icationofthe particulartypeanddegreeofhearingimpairment.
Inmedicalpractice,theclassi icationofhearing lossisdeterminedbythecon iguration,severity,type (locationoflesion),andsymmetryfoundintheout‐comesofpure‐toneaudiometryexaminations.
Thetypeofhearinglossmaybecategorizedas conductiveloss,whichiscausedbyproblemsinthe outerormiddleear,orsensorineuralloss,whichis aresultofdif icultiesintheinnerearandauditory nerve.Alternatively,itcouldbeacombinationofboth, knownasmixedhearingloss.Thisclassi icationmust beperformedbyprofessionalaudiologistsaftereach puretoneaudiometrytest.Particularlyproblematic onaglobalscaleisthescarcityofspecializedaudiol‐ogists;innearly93%oflow‐incomenations,thereis fewerthanoneaudiologistpermillioncitizens[1].
Giventhe inancialandsocialobstaclesinreducing thelargediscrepancybetweenthedemandandsupply ofhearingspecialists,itisimportanttoinvestigate thecapabilityofarti icialintelligence(AI)methodsin resolvingthisissue.Anautomateddecisionsupport systemcouldpotentiallyofferarangeofbene its,from minimizinghumanerrorstoentirelyexpeditingthe evaluationofpure‐toneaudiometryteststogeneral practitioners.Thedevelopmentofsuchasystemcould leadtoareductionintheworkloadrequiredbyspe‐cialistsandadecreaseinthewaitingtimeforpatients’ diagnoses.Moreover,practicalapplicationofsucha systemwouldnecessitatetheestablishmentofclinical guidelinesandbestpractices,ensuringthathealth‐careprovidersadheretoauniformtreatmentprocess, improvingpatientdiagnosisanddecreasingtreatment variability.
Intheabovecontext,thepaperpresentsacompar‐isonofmachinelearninganddeeplearningmethods appliedtotheclassi icationof4007tonalaudiometry testresultsthatwerepreviouslyanalyzedandlabeled byexpertaudiologists.Theobjectiveofthisstudywas toexaminetheef icacyofdifferentarti icialintelli‐gence(AI)techniqueswhenutilizedwithrawtone audiometrydata.Thelatterisparticularlysigni icant becausepre‐classi iedpuretoneaudiometrydatais relativelydif iculttoobtaininlargequantities,which iswhynopriorworkshadtheopportunitytoperform anin‐depthclassi icationusingstate‐of‐the‐artmeth‐ods.
Furthermore,thepresentedworkwillserveasa basisforselectinganoptimalmodelforclassifying differenttypesofhearinglossinclinicalsettings.
Thisarticleisanextensionoftheresearchpre‐sentedinthe18thConferenceonComputerSci‐enceandIntelligenceSystemsFedCSIS2023during theDoctoralSymposium—RecentAdvancesinInfor‐mationTechnology(DS‐RAIT)[3].Thestudywas expandedtoincludeseveralnewAImodelsandpro‐videamorethoroughassessmentoftheapplieddeep learningalgorithms,includinganexaminationofthe impactofvariousdatapreprocessingmethods.More‐over,theextendedpaperalsodiscussestheeffects ofexpandingthetrainingdatasetwiththeuseofa generativeadversarialnetwork(GAN).
Researchonautomaticaudiometrydataclassi ica‐tionhasbeenongoingforanextendedperiodoftime. Inpastyears,severalendeavorshavebeenmadeto developanautomaticclassi icationsystemthatissuf‐icientlyaccuratetojustifyitspracticalimplementa‐tion.Thepaperscanbecategorizedintotwoprimary themes:onerelatedtothedeterminationofinitial con igurationsofhearingaids,andtheotherfocused ontheclassi icationofhearinglosstypes.Inthelit‐eraturetherearenumerouspublicationsthatdiscuss theformersubject[4–6];however,thesubjectofauto‐maticclassi icationofdifferentformsofhearinglossis substantiallylessexplored.
The irstattemptatanautomatedclassi ierof hearinglosstypeswasdonebyElbaşıandObaliin 2012[7]whocarriedacomparativeanalysisofvari‐ousmethodsforidentifyingthetypeofhearingloss, includingtheimplementationofmultilayerpercep‐tron(MLP)modeclassi iers,DecisionTreeC4.5,and NaiveBayes.Theinvestigationwasconductedona datasetof200samples,whichwereclassi iedinfour distinctgroups:normalhearing,sensorineuralhear‐ingloss,conductivehearingloss,andmixedhearing loss.Theinputdatawasformattedasasequence ofnumericalvaluesthatrepresenteddecibels,which correspondedtoconstantfrequencylevels.TheDeci‐sionTree(C4.5)approachproducedanaccuracyof 95.5%,theNaiveBayesmethodachievedanaccuracy of86.5%,andtheMLPalgorithmobtainedanaccuracy of93.5%.
Adifferentmethod,whichfocusedonraster imagesinsteadoftabulardata,waspresentedseveral yearslaterbyCrowsonetal.(2020)[8],whoclassi‐iedaudiogramimagesusingtheResNetmodelinto threedistincthearinglosscategories(conductive,sen‐sorineural,ormixed)inadditiontonormalhearing.A datasetconsistingof1007audiogramswasutilizedfor bothtrainingandtestingobjectives.Insteadofstarting theclassi iertrainingprocessfromthebeginning,the scientistsimplementedtransferlearningfortraining theclassi ierbyutilizingwell‐establishedrasterclas‐si icationmodels.Theclassi icationaccuracyofthis approachreached97.5%.
Overall,theintegrationofmachinelearningwith enhancedcomputationalresourcesincutting‐edge hardwarearchitecturesholdsthepromiseofproduc‐ingquickeroveralltestoutcomesandmorecompre‐hensiveassessmentsinthe ieldofaudiology[9]. Regardingthecategorizationofhearinglosstypes, thecurrentlysuggestedmethodsexhibitclassi ication accuracyrangingfrom86%to97%.Althoughthis accuracyisremarkablyhigh,itstillallowsforasig‐ni icantmarginoferror.Furthermore,althoughthe audiogramclassi ierdevelopedbyCrowsonetal.[8] demonstratedthehighestaccuracythusfar,itisnot suitableforanalyzingtheoriginaltabulardatagen‐eratedbytonalaudiometry,asitisdesignedonly forimageclassi ication.Priortoclassi ication,the datasetsmustbetransformedintoaspeci icformat ofaudiogramimages.Althoughaudiogramsgener‐allyhaveasimilarstructure,thoseproducedbydif‐ferenttoolscansigni icantlydifferinformandcon‐tent.Someaudiometrysoftwaregeneratesindividual audiogramsforeachear,whereasotherscombinethe datafrombothintojustoneaudiogram.Thisposes aconsiderabledif icultywhenattemptingtoanalyze allcasesinacomprehensivemanner.Hence,animage classi ierisnotsuitableasthecentralcomponentofa lexiblesystemforcategorizingpuretoneaudiometry results.
Inaddition,theaforementionedstudieswhich attemptedtocreatehearinglossclassi ierswerecon‐ductedusingverysmalldatasets.Thesamplesizes inthestudiesconductedbyElbaşıandObali[7]and Crowsonetal.[8]rangedfrom200to1007test results,respectively.Withlargerdatasets,AImodels caneffectivelycaptureagreaternumberofunique casesofhearingloss,resultinginmoreunbiasedout‐comes.
Theobjectiveofthisstudywastoevaluatethe effectivenessofseveralarti icialintelligence(AI)tech‐niquesinclassi icationofpuretoneaudiometrydata. Theperformanceofdifferentalgorithmswasevalu‐atedbymeansoftheaccuracywithwhicheachsample wasclassi iedassensorineuralhearingloss(S),mixed hearingloss(M),orconductivehearingloss(C)by eachmethod.
3.1.Data
Thestudyemployedadatasetconsistingof4007 samples,whichincludedtheresultsofpuretone audiometrytestsconductedbydoctorsattheDepart‐mentofOtolaryngologyoftheUniversityClinicalCen‐treinGdanskbetween2017and2021.Figure1illus‐tratesthedistributionofthedataacrossdifferent classes.Thereare674examplesofconductivehear‐ingloss,1594instancesofmixedhearingloss,and 1739samplesofsensorineuralhearingloss.Theclass imbalancearisesfromthepatienttreatmentproto‐colsimplementedbymedicalinstitutions.Conductive hearinglosstypicallyresultsfrompathologyaffect‐ingtheearcanal,obstructingthepassageofair.The diagnosisofthisconditionisusuallymadewithan otoscopeduringtheinitialexaminationofthepatient, thuseliminatingtherequirementforapure‐tone audiometrytest.
Eachpatientcontributedamaximumoftwoexam‐inationresults,withoneresultassignedtotheleftear andtheothertotherightear,thereforeeliminating anydataredundancyforthesamepatientandassur‐ingasuf icientdiversityofdata.
Thehearingofthepatientswasassessedusing puretoneaudiometryinaccordancewiththeguide‐linessetforthbytheAmericanSpeech‐Language‐HearingAssociation(ASHA)[10].Everyexperiment wasperformedwithinsoundproofenclosures(ISO 8253,ISO8253).TheTDH39Pheadphoneswereused forairconductiontesting,whiletheRadioearB‐71 bone‐conductionvibratorwasemployedforbonecon‐ductiontesting.
Alongsideanaudiogram,whichisastandardvisual representationofpure‐toneaudiometrytest indings, audiologysoftwareproducesXML ilesthatcontain comprehensivedataonthetonalpointsintheaudio‐gram.ThisstudyemploysXML ilescontainingraw audiometrydata,concentratingon ivefundamental frequencies(250Hz,500Hz,1000Hz,2000Hzand 4000Hz)acquiredusingbothboneaswellasair conduction.
3.2.DatasetExpansion
Becausethesizeofthetrainingdatasetisrather smallformachinelearningstandards,duringthepre‐sentedresearchthisdatabasewasexpandedthrough theapplicationofaconditionalgenerativeadversarial network[11].Agenerativeadversarialnetwork(GAN) isadeeplearningnetworkthathastheabilityto producedatathatcloselyresemblesthepropertiesof thetrainingdataitwasprovidedwith.Aconditional generativeadversarialnetwork(CGAN)isavariant oftheGANarchitecturethatincorporateslabelsas additionalinformationduringthetrainingphase.A CGANcomprisesapairofinterconnectednetworks thatundergojointtraining:
1) Generator—thisnetworktakesalabelandaran‐domarrayasinputandproducesdatathathas thesamestructureasthetrainingdatasamples associatedwiththegivenlabel.
2) Discriminator—thisnetworkaimstocategorize observationsas“real”or“generated”byusing labeledbatchesofdatathatincludeobservations fromboththetrainingdataandthegenerateddata. InordertotrainaconditionalGAN,itisnecessary toconcurrentlytrainbothnetworkswiththeobjective ofoptimizingtheperformanceofboth.Thisinvolves trainingthegeneratortoproducedatathatdeceives thediscriminator,whilesimultaneouslytrainingthe discriminatortoaccuratelydifferentiatebetweenreal andcreateddata.
ThisresearchusedCTAB‐GAN[12]toaugment thedatasetbyafactoroftwo.TheCTAB‐GANisan expandedversionoftheinitialresearchonCGANfor tabulardata[13],enablingthehandlingofimbalanced data.
Inthe irststage,featurescalingwasutilizedas adatapreparationtechniqueforstandardizingthe valuesoffeaturesinadatasettouniformscale.As mentionedintheliterature[14,15],datastandardiza‐tionisadvantageousintermsofenhancingef iciency throughoutthetrainingphase.Thisstudyusedthe widelyusedZ‐Score(1)standardizationapproach:
(1) wherexistherawscore, �� isthemeanand �� isthe standarddeviation.
Inaddition,twomorestandardizationformulas, MinMax(2)andMaxAbsScaler(3),weretestedon deeplearningnetworks
wherexistherawscore,ministheminimumvalue ofthefeatureandmaxisthemaximumvalueofthe feature.
3.4.MachineLearningModels
Theresearchwasinitiatedbyevaluatingtheper‐formanceofvariousmachinelearningclassi ication methods,includingrandomforest(RF),Gaussian NaiveBayes,supportvectormachines(SVMs),logis‐ticregression,stochasticgradientdescent(SGD),K‐nearestneighbors(KNN)anddecisiontree(DT).The tabulardataformatwasusedastheinputforallthe describedalgorithms.
Allalgorithmshavebeentestedwithdifferentpre‐processingmethods,bothontheinitialaswellas expandeddataset.
3.5.MachineLearningModels
Thesubsequentstageoftheinvestigationentailed evaluatingthefollowingANNarchitectures:convolu‐tionalneuralnetwork(CNN),recurrentneuralnet‐work(RNN)andfeedforwardneuralnetwork(FNN). Furthermore,twoofthemostwidelyusedRNNcon‐cepts,namelylongshort‐termmemory(LSTM)and gatedrecurrentunit(GRU),wereevaluated.Both LSTMandGRUattempttoovercometheproblemof vanishinggradientsbyintroducingdata lowcontrol mechanisms[16].
Previously,thesemethodshadbeenemployedto classifyrelevantmedicaldata[17,18].
3.6.EvaluationProcess
Theperformanceofalltestedmodelswasassessed withtheuseofK‐foldcross‐validation.Thispro‐cessentailedpartitioningthedatasetintoKsub‐sets,referredtoasfolds,whereK‐1subsetswere allocatedfortrainingpurposesandonesubsetwas reservedforvalidation.Followingthis,thesubsets havebeensequentiallyrotatedinsubsequenttests, whichenabledamorepreciseevaluationofthebest, worst,andaverageperformanceoftheclassi ication. InthepresentedworkthevalueofKwasestablishedat 10inaccordancewiththeliteraturestandardandthe scaleofthedataset.Thus,theproportionoftraining totestingdatasetsistenpercenttoninetypercent. Duringtheevaluationofmodels,thedefault10‐fold setwasdecreasedto90%,withtheremaining10% formingadedicatedtestdataset.Thishasbeendone toensurethattheperformanceofmodelstrainedwith andwithoutdatageneratedwiththeuseofCGANcan beeffectivelycompared.
Thegeneralwork lowofthepresentedstudyis showninFigure2.
Figure2. Theworkflowofthepresentedresearchinto applicationofmachinelearningmethodsforthe classificationofhearinglosstypesbasedonpure‐tone audiometrydata
Inadditiontotraditionalmeasuressuchasaccu‐racy,thepresentedresearchalsoemployedprecision‐recallmetricsderivedfromaconfusionmatrix[19] aswellasreceiveroperatingcharacteristics(ROC) curveswhichencompassthepertinentarea‐under‐the‐curve(AUC)data.
Thesecurveseffectivelydemonstratethediscrim‐inationperformanceoftheevaluatedmodelsbycom‐paringtruepositivesandfalsepositives.Further‐more,inadditiontoevaluatingtheef icacyofbinary classi icationmodels,thereceiveroperatingcharac‐teristic(ROC)curveandtheareaundertheROC curve(ROCAUC)scorearevaluableinstrumentsfor assessingmultipleclassi icationchallenges.Thecho‐senapproachisOvR,anacronymfor“oneversusthe rest,”whichassessesmulticlassmodelsbycomparing eachclasstotheotherssimultaneously.Inthiscase, oneclassisdesignatedasthe“positive”class,while theremainingclassesaredesignatedasthe“negative” class.Thistransformstheoutputofmulticlassclassi‐icationintobinaryclassi ication,enablingtheappli‐cationofestablishedbinaryclassi icationmetricsto evaluatethissituation[20].
Table1. ComparativeanalysisofperformanceoutcomesofmachinelearningmodelswithoutGAN
Table2. ComparativeanalysisofperformanceoutcomesofmachinelearningmodelswithGAN
Theinitialstepofthepresentedstudyinvolved evaluationoftheclassi icationperformanceoffered byacollectionofmachinelearningalgorithms.The outcomeshavebeenevaluatedinrelationtoaccuracy, precision,recall,andF1score.Macroaveragingin 10‐foldcrossvalidationwasusedtooffsettheclass imbalanceinthetrainingdataset.Thetestresultsare presentedinTable1
Thesupportvectormachineclassi ierhas achievedthehighestlevelofsuccessamongmachine learningalgorithms,withanaccuracyrateof85.15%. Thealgorithmachievedthehighestratingsin precision,recall,F1,andAUC.InclosepursuitofSVM, thelogisticregressionandrandomforestmodelsboth exceeded82%intermsofaccuracy.
Stochasticgradientdescentachievedanaccu‐racyof74.74%,whileK‐nearestneighborsobtained 77.02%,whichputsbothofthemwellbelowthetop threealgorithms,butstillsigni icantlyhigherthan GaussianNaiveBayeswhichonlyreached62.34% accuracy.
Tree‐basedclassi iershavedemonstratedsuperior accuracystabilityin10‐foldvalidation.Thedecision treeclassi ierexhibitsastandarddeviationofroughly 4%,whiletherandomforestclassi ierhasastan‐darddeviationofaround4.65%.Incontrast,allother modelshaveastandarddeviationover6%.Theissue ofimbalanceddata,whichiscertainlyvisibleinthis study,isoneofthefactorsthatmightadverselyaffect theeffectivenessofmachinelearningalgorithms,as exempli iedbythesubparresultsofGaussianNaive Bayes.
TheresultsinTable 2 depicttheoutcomes obtainedbyaugmentingthetrainingsetusingCTAB‐GAN.TheapplicationofCGANyieldedpositiveout‐comesforonly4outofthe7algorithmsthatwere examined.Doublingthesizeoftrainingdatadidnot in luencetheaccuracyofNaiveBayesanddecision tree,whichproducedresultsdifferingbylessthan1 percentagepoint.TheKNNmodelexhibitedaslight reductioninoverallclassi icationperformance,losing lessthan2percentagepointsinaccuracyandrecall. Ontheotherhand,thegenerationofadditionaltrain‐ingdataresultedinincreasingtheclassi icationaccu‐racylevelinSVMsandlogisticregressionbyapproxi‐mately5%.Thelargestincrease,amountingtoan8% increase,isshownintheSGDresultsascomparedto thosewithoutCGAN.
Thisbeingsaid,theincreaseinaccuracy,aswell asimprovementsinothermeasuressuchaspreci‐sion,recall,andF1scoreshownbyallthreealgo‐rithmscouldbeconsideredtobewithintheirrespec‐tivemarginsoferror.Inordertosidesteptheissueof increasedmarginsoferrorintheexpandeddatasets, theclassi icationaccuracyofselectedmethodswas testedagainonthededicatedtestdataset,whichhad beenextractedfromtheoriginaldatabeforetraining. Resultsofthesetestsarepresentedintheformof confusionmatricesdisplayedinFigures 3, 4, 5 and Table 3.Thematrixontheleftdepictstheoutcomes obtainedwithouttheuseofCGAN,whilethematrix ontherightillustratestheresultsfollowingtheimple‐mentationofCGAN.TheS,M,andCindicesrepresent sensorineuralhearingloss,mixedhearingloss,and conductivehearingloss,respectively.
ConfusionmatricesofthelogisticregressionmodeltrainedwithoutCGAN(left)andwithCGAN(right)
ConfusionmatricesofthestochasticgradientdescentmodeltrainedwithoutCGAN(left)andwithCGAN(right)
Figure5. ConfusionmatricesofthesupportvectormachinesmodeltrainedwithoutCGAN(left)andwithCGAN(right)
Comparingthe indingsobtainedfrom10‐fold crossvalidationtothoseobtainedfromadedicated test,thereisasimilarimprovement(Table3).Logistic regression,supportvectormachines,andstochastic gradientdescentexhibitconsiderableenhancements inaccuracy,similartotheoutcomesshownin10‐fold(Table 2).TheresultsforGaussianNaiveBayes andrandomforestshowminimalvariation,witha differenceoflessthanonepercentagepointThemost signi icantdeclinewasobservedintheperformance ofKNNanddecisiontrees,withadifferenceof1.24%, whichisstillcomparabletotheresultsobtainedfrom the10‐foldanalysis.
Theimprovementsbroughtbyarti iciallyexpand‐ingthetrainingdatasetarebestvisibleintheconfu‐sionmatricespresentedinFigures3,4,and5.
Inthecaseofthelogisticregressionmodelresults depictedinFigure3,itisnoteworthythat,subsequent totheadoptionofGAN,thenumberofconduc‐tivehearinglosscases(C)incorrectlylabeledas sensorineuralandmixedhasdemonstratedadrop of30%and50%,respectively.Theimprovements toclassi icationoftheremainingtypesaremuch smallerbutpersistent,withonlytheclassi ication ofmixedhearinglossasconductiveshowingno improvements.TheperformanceofStochasticGradi‐entDescentmodelhasshownthelargestimprove‐mentsaftertrainingwithGAN‐deriveddata(Figure4). Thenumberofmixedhearinglosscasesincorrectly classi iedassensorineuraldecreasedby73%(from 33to9),whilethenumberofconductivehearingloss caseslabeledassensorineuralwasreducedby25% (12to9).
Table3. Comparisonoftheaccuracyofthetested machinelearningmodelstrainedwithandwithoutthe useofCGAN,analyzedonthededicatedtestdataset
Atthesametime,thenumberofsensorineural hearinglosscasesimproperlyrecognizedasconduc‐tivedecreasedby29%(from14to10)andthenumber ofmixedhearinglossdatasetsincorrectlylabeledas conductivedecreasedby73%(from11to3).How‐ever,thesegainsareoffsetsomewhatbyareductionin theaccuracyofmixedhearinglossclassi ication.After trainingondatageneratedbyGAN,SGDhasshown anincreasedtendencytolabelmixedhearingloss aseithersensorineural(22casesversus11,a100% increase)orconductive(5casesversus1,a400% increase).Thisbeingsaid,thetotalnumberofprop‐erlyrecognizeddatasetsstillshowsaconsiderable8% increase(343from319).
Outofthethreeanalyzedmachinelearningmod‐els,supportvectormachines(SVMs)istheonlyone whichshowsconsistentimprovementstoallcases ofclassi icationinaccuracyaftertrainingwithGAN‐deriveddata.Thenumberofsensorineuralhearing losscasesimproperlylabeledasmixedandconduc‐tiveisreducedby38%(16to10)and50%(2to1), respectively.Thenumberofmixedhearinglosscases improperlylabeledassensorineuralandconductiveis reducedby14%(7to6)and50%(2to1),respectively. Finally,thenumberofconductivehearinglosscases incorrectlyrecognizedassensorineuralandmixedis reducedby50%(4to2)and13%(8to7),respectively. Theseimprovementsincreasethetotalnumberofcor‐rectlyclassi ieddatasetsfrom362to375.
Giventhatinthecurrentstateoftheart,deep learningmodelssurpasstheclassi icationaccuracy ofallmachinelearningmethods,thepresentedstudy alsoevaluatedtheperformanceofseveraldeeplearn‐ingarchitectures.Theseincludefeedforwardneu‐ralnetworks(FNN),convolutionalneuralnetworks (CNN),andrecurrentneuralnetworks(RNN),which encompassgatedrecurrentunits(GRU)andlong short‐termmemory(LSTM).Theevaluationwasper‐formedusinga10‐foldcross‐validationmethod‐ology,andinvolvedassessmentoftheimpactof implementingdifferentdatastandardizationmeth‐ods.Theresultsoftheseexperimentsaredisplayedin Tables4–6.
Table4. Classificationperformanceofdeeplearning modelsusingZ‐Scorenormalization
Table5. Classificationperformanceofdeeplearning modelsusingMinMaxScalernormalization
Table6. Classificationperformanceofdeeplearning modelsusingMaxAbsScalernormalization
AsitcanbeseeninTables 4–6,normaliza‐tionstrategyplaysafundamentalpartinobtaining goodclassi icationperformanceusingdeeplearn‐ingmodels.Undoubtedly,theZ‐Scorenormalization methoddeliveredoutstandingperformanceacrossall architectures(Table4).Theseclassi icationaccuracy resultsareonaverage35%betterthaninthecaseof MinMaxScaler(Table5)andabout120%betterthan thoseproducedbyMaxAbsScaler(Table 6),whichis clearlynotsuitableforaudiometrydata.
Concerningtheresultsobtainedbyallnetworks withtheZ‐Scorenormalizationmethod,LSTMexhib‐itedthehighestperformanceintermsofaccuracy, recall,precisionandF1score.Speci ically,itachieved anaccuracyof95.63%andanF1scoreof95.63%.It waspredictablethattheinputdatasets,beingsequen‐tialdata,wouldbewell‐suitedfortheRNNfamily ofmodels,whichisknownforitsstrengthinhan‐dlingthistypeofdata[18].Theresultsappearto validatetheconclusionsofapreviousstudy[21] whichassessedseveralneuralnetworkcon igura‐tionstocreateabinaryclassi ierfordistinguishing betweenpathologicalhearinglossandnormalhear‐ingusingsimilardata.Saidinvestigationalsocon‐cludedthattheLSTMarchitectureyieldedthemost favorableresults.Thesecond‐bestresultshavebeen
Figure6. ROCcurveswiththeAUCparametersfor testeddeeplearningmodelsduring10‐Foldvalidation
achievedbythesimpleRNNmodel,withadifference ofapproximately0.6%.Whilethedifferenceiswithin themarginoferror,thisresultissomewhatexpected, consideringthatLSTMmodelstypicallyoffersuperior performanceoversimpleRNNmodels.Thethirdplace oftheCNNmodel,whichisprominentlyusedforpro‐cessingrasterdata,couldbeexplainedbythefactthat eachdatasetinthecurrentstudyisrepresentedbya two‐dimensionaltablewhichsomewhatresemblesa verysmallraster.
Theclassi icationperformanceofthepresented deeplearningmodels(Table 4)isvisualizedinFig‐ure 6 intheformofROCcurveswithcorrespond‐ingAUCparameters.Theseillustratethediscrimina‐torycapabilityoftheevaluateddeeplearningmodels quanti iedbytheratiooftruepositivestofalseposi‐tives.
AllCNN,RNN,LSTM,andGRUmodelshavethe sameAUCparameterscoreof0.94.WithanAUCvalue of0.91,theFNNmodelisconspicuouslyinferiortothe others.
Ingeneral,thescalingtechniquehasasubstantial impactontheperformanceofclassi icationmodels. Furthermore,thisimpactmayvarydependingonthe speci ictypesofmodelsemployed,suchasmonolithic andensemblemodels[22].
Basedontheseresults,allsubsequenttestswere performedwiththeuseofZ‐Scorenormalization,asit isthesolemethodthatyieldsoutcomescomparableto thestate‐of‐the‐art.
The inalstepofthepresentedresearchanalyzed theperformanceofdeeplearningmethodstrained onthedatasetaugmentedwiththeuseofCGAN.The resultsaredisplayedinTable7.
Table7. Performanceofdeeplearningmodelstrained ondataaugmentedwithCGAN
Table8. Comparisonoftheperformanceofdeep learningmodelstrainedwithandwithouttheuseof CGAN,analyzedonthededicatedtestdataset
AsitcanbeseeninTable 7,trainingonthe expandeddatasethassigni icantlyincreasedtheper‐formanceofcertaindeeplearningmodelswhile impactingtheperformanceofothers,whichmirrors thesituationwithmachinelearningalgorithms.In particular,theclassi icationaccuracyofrecurrentnet‐workshasincreasedbynearly1%inthecaseofRNN, around1.5%forGRUandnearly3%forLSTM.Onthe otherhand,theclassi icationeffectivenessofFNNand CNNhasreducedbynearly3%.Thisbeingsaid,con‐sideringthepotentialimpactoftestingthenetworks onCGAN‐augmenteddata(whichhasbeenshownpre‐viouslyformachinelearningmethods),asubsequent analysiswasconductedusingthededicatedtestset. TheresultsofthistestarepresentedinTable8
Similarlytothecaseofmachinelearningmodels, testingonthededicateddatasetyieldssimilarover‐allresults,howeverwithsomewhatdifferentperfor‐mancevalues.TheperformancesofLSTMandRNN modelshaveshownanincrease,whereasthoseof FNNandCNNexperiencedadecline.Anexception tothiscorrelationistheGRUmodel,asits indings remainconsistentregardlessoftheapproachused. TheLSTMmodelachievedthehighestaccuracy,reach‐ing97.56%.Thisresultislowerbyonepercentage pointcomparedtothe igurereportedinTable 7 for the10‐foldwithGANapproach.
Ingeneral,arti icialneuralnetworksexhibitsupe‐riorperformancetodeeplearningmodelswhencom‐paringthetwo.However,theutilizationofCGANfor trainingmachinelearningmethodsenablessomeof themtocomeclosertotheaccuracydeliveredby thelessperformantdeeplearningmethods.Still,the optimaloutcomesareachievedbyRNN‐basedmodels withZ‐ScorenormalizationandGANaugmentation,in particularsimpleRNNandLSTMmodels.
Theachievedresultssigni icantlyexceedthose ofpriorinvestigations(conductedbyElbaşıand Obali[7]),whichutilizedaDecisionTreetoclassify rawaudiometrydatawithanaccuracyof95.5%.Inter‐estingly,whenevaluatedonthepresenteddata,the sameDecisionTreealgorithmachievedanaccuracyof approximately83%onthededicatedtestdataset.Yet, thevalidityofthecited indingsmaybequestioned duetothelimitedsamplesizeofjust200,whichis signi icantlysmallerthanthedatasetemployedinthe presentstudy.Moreover,theresultscannotbedirectly comparedbecausethecitedstudywasconductedon fourclasses(asopposestothreeclassesinthepre‐sentedwork),whichincludedindividualswithnormal hearing,andthereisnodataregardingclassdistribu‐tionnorthemethodusedforcross‐validation.
Atthesametime,thegreatestclassi icationaccu‐racyof97.56%attainedbyLSTMonthededicated testdatasetiscomparabletothepresentstateofthe artinclassifyingpuretoneaudiometrytestresults (97.5%)reportedbyCrowsonetal.[8]forraster datasets.Similartothatwork,trainingdataaugmen‐tationhasprovidedsigni icantlybetterclassi ication results(althoughthepresentedworkaugmentedtab‐ulardata,whereasCrowsonetal.augmentedraster data).Again,theseresultscannotbedirectlycom‐paredduetothelowernumberofclasses(three insteadoffour)usedinthepresentedstudy.Moreover, Crowsonetal.[8]classi iedrasteraudiogramsinstead ofactualtestresults,andimagesproducedbydifferent typesofaudiometrysoftwarevarysigni icantly.These variationscanrangefromminordifferencesinthe coloroftheplotandthesizeofthemeasurement pointindicatorstomoresigni icantchangesthatmay adverselyaffecttheperformanceofautomatedclassi‐iers(e.g.,presentingoutcomesfrombothearsona solitaryplot).Inorderforimage‐trainedclassi ication modelstobeeffectivewithalltypesofaudiometry data,itisnecessarytocreateacomprehensiveaudio‐gramdatabase.Thiswouldincludecollectingandclas‐sifyingthousandsofaudiogramscreatedbydifferent audiometryapplications.Bycontrast,aclassi ierthat utilizesunprocessedaudiometrydataoffersgreater versatilityandbroaderpotentialforuseintheclinical setting.
Onthewhole,despiteattainingarelativelyhigh classi icationaccuracyof97.56%,thepresented LSTM‐basedclassi iermaynotbeadequateforclinical useduebeingtrainedondataaugmentedwithCGAN. Whilethisdatahassigni icantlyimprovedtheperfor‐manceofcertainclassi iers,ithasalsodecreasedthe performanceofothermethods,suggestingthatnotall ofthegenerateddatasetsmayproperlyre lectreal‐worldaudiometrydata.Therefore,thecreationofa reliableandpreciseclassi ierforrawaudiometrydata necessitatestheestablishmentofatrainingdataset thatissuf icientlylargeandrepresentative,whilealso beingcloselycontrolledbymedicalexperts.
Theobjectiveofthepresentedstudywastoassess theef icacyofdifferentarti icialintelligencealgo‐rithmsinclassifyingdiscretetonalaudiometrydata seriesintothreespeci ictypesofhearingloss:con‐ductive,sensorineural,andmixed.Forthispurpose, thestudyinvolvedtestingmachineanddeeplearn‐ingmodelscomprisedofGaussianNaiveBayes,sup‐portvectormachines,randomforest,K‐nearestneigh‐bors,logisticregression,stochasticgradientdescent, decisiontrees,feedforwardneuralnetwork,convolu‐tionalneuralnetworkandrecurrentneuralnetwork (includinglongshort‐termmemoryandgatedrecur‐rentunit).Themodelsindicatedabovehavebeen trainedandassessedusing4007setsoftonalaudiom‐etrydata,whichhadbeenanalyzedandlabeledby audiologistswhoareexpertsinthe ield.
Furthermore,theinvestigationalsoexploredthe impactoftrainingdatasetaugmentationusingacon‐ditionalgenerativeadversarialnetworkandexamined howdifferentstandardizationproceduresaffectthe effectivenessofdeeplearningarchitectures.
Thebestoverallresultswereobtainedwiththe longshort‐termmemorymodel,whichattainedthe maximumclassi icationaccuracyof97.56%withZ‐ScorenormalizationandCGANdataaugmentation.On thewhole,alldeeplearningmodelsachievedsubstan‐tiallybetterclassi icationresultsthanmachinelearn‐ingalgorithmswhentrainedonthestandarddataset, buttrainingontheGAN‐augmenteddatasetallowed supportvectormachinestoachieveresultssimilarto thatoflessperformantdeeplearningmodels.
Thus,ontheonehandthestudy’s indingscon‐irmedtheoverallrankingofclassi icationperfor‐mancethatearlierresearchhadestablished.Onthe otherhand,the indingsalsosuggestthattheclassi i‐cationaccuracylevelspreviouslydocumentedinliter‐ature,whichwereattainedusingconsiderablysmaller datasets,mighthavebeenoverlysanguine.
Finally,theresultsofthepresentedresearchindi‐catethatusingaGANaugmentationoftrainingdata mayproduceverypositiveresults,however(asexem‐pli iedbytheperformanceofthestochasticgradient descentmodel)unsupervisedgenerationofinputdata maynotalwaysleadtooptimaloutcomes.Inthiscon‐text,futureworkcouldconcentrateonenhancingthe accuracyoftheRNN‐basedclassi ierandincreasing thesizeoftrainingdatasetaswellasdesigningaGAN modelwhichismoreef icientlytunedforproducing properlylabeledtonalaudiometrytestdata.
Ingeneral,thedemonstratedoutcomesindicate thattheproposedAI‐drivenpuretoneaudiometry dataclassi iermayhavepracticalimplicationsinclin‐icalsettings,functioningaseitheraclassi icationsys‐temforgeneralpractitionersorasupportsystemfor professionalaudiologists.Inbothscenarios,theimple‐mentationoftheclassi ierhasthepotentialtomini‐mizehumanerror,enhancediagnosticaccuracy,and reducethewaitingtimeforpatientstoreceivetheir diagnosis.
AUTHORS
MichałKassjański∗ –DepartmentofGeoinformat‐ics,FacultyofElectronics,Telecommunicationsand Informatics,GdanskUniversityofTechnology,80‐233, Gdansk,Poland,e‐mail:michal.kassjanski@pg.edu.pl.
MarcinKulawiak –DepartmentofGeoinformatics, FacultyofElectronics,Telecommunications andInformatics,GdanskUniversityof Technology,80‐233,Gdansk,Poland,e‐mail: marcin.kulawiak@eti.pg.edu.pl.
TomaszPrzewoźny –DepartmentofOtolaryngology, MedicalUniversityofGdansk,Smoluchowskiego Str.17,80‐214Gdansk,Poland,e‐mail: tomasz.przewozny@gumed.edu.pl.
DmitryTretiakow –DepartmentofOtolaryngol‐ogy,theNicolausCopernicusHospitalinGdansk, CopernicusHealthcareEntity,PowstancowWarsza‐wskichstr.1/2,80‐152,Gdansk,Poland,e‐mail: d.tret@gumed.edu.pl.
JagodaKuryłowicz –DepartmentofOtolaryngology, MedicalUniversityofGdansk,80‐214,Gdansk,Poland, e‐mail:jagoda.kurylowicz@gmail.com.
AndrzejMolisz –DepartmentofOtolaryngology, MedicalUniversityofGdansk,80‐214,Gdansk,Poland, e‐mail:andrzej.molisz@gumed.edu.pl.
KrzysztofKoźmiński –Student’sScienti icCircleof Otolaryngology,MedicalUniversityofGdańsk,80‐214 Gdansk,Poland,e‐mail:krzyk@gumed.edu.pl. AleksandraKwaśniewska –Department ofOtolaryngology,LaryngologicalOncology andMaxillofacialSurgery,UniversityHospital No.2,85‐168,Bydgoszcz,Poland,e‐mail: kwasniewska.aleks@gmail.com.
PaulinaMierzwińska-Dolny –Student’sScienti ic CircleofOtolaryngology,MedicalUniversity ofGdańsk,80‐214Gdansk,Poland,e‐mail: paulinamierzwinska@gumed.edu.pl.
MiłoszGrono –Student’sScienti icCircleofOtolaryn‐gology,MedicalUniversityofGdańsk,80‐214Gdansk, Poland,e‐mail:milosz.grono@gumed.edu.pl.
∗Correspondingauthor
References
[1] WorldHealthOrganization, Worldreporton hearing.Geneva:WorldHealthOrganization, 2021.
[2] R.W.BalohandJ.C.Jen,“HearingandEqui‐librium,”Jan.2012,doi:10.1016/b978‐1‐4377‐1604‐7.00436‐x.
[3] M.Kassjańskietal.,“Detectingtypeof hearinglosswithdifferentAIclassi ication methods:aperformancereview,” Computer ScienceandInformationSystems(FedCSIS), 2019FederatedConference,Sep.2023,doi: 10.15439/2023f3083.
[4] C.Belitz,H.Ali,andJ.Hansen,“AMachineLearn‐ingBasedClusteringProtocolforDetermining HearingAidInitialCon igurationsfromPure‐ToneAudiograms,” PubMedCentral,Sep.2019, doi:10.21437/interspeech.2019‐3091.
[5] F.Charih,M.Bromwich,A.E.Mark,R.Lefrançois, andJ.R.Green,“Data‐DrivenAudiogramClassi i‐cationforMobileAudiometry,” Scienti icReports, vol.10,no.1,Mar.2020,doi:10.1038/s41598‐020‐60898‐3.
[6] A.Elkhoulyetal.,“Data‐drivenaudiogramclas‐si ierusingdatanormalizationandmulti‐stage featureselection,” Scienti icReports,vol.13, no.1,Feb.2023,doi:10.1038/s41598‐022‐25 411‐y.
[7] E.ElbaşıandM.Obali,“Classi icationofHearing LossesDeterminedthroughtheUseofAudiom‐etryUsingDataMining,” Conference:9thInternationalConferenceonElectronics,Computerand Computation
[8] M.G.Crowsonetal.,“AutoAudio:DeepLearning forAutomaticAudiogramInterpretation,” JournalofMedicalSystems,vol.44,no.9,Aug.2020, doi:10.1007/s10916‐020‐01627‐1.
[9] H.ShojaeemendandH.Ayatollahi,“Automated Audiometry:AReviewoftheImplementation andEvaluationMethods,” HealthcareInformatics Research,vol.24,no.4,pp.263–275,Oct.2018, doi:10.4258/hir.2018.24.4.263.
[10] GuidelinesforManualPure‐ToneThreshold Audiometry,” AmericanSpeech-LanguageHearingAssociation.https://www.asha.org/pol icy/GL2005‐00014/(accessedDec.5,2023).
[11] M.MirzaandS.Osindero,“ConditionalGener‐ativeAdversarialNets,” arXiv.org,2014. https: //arxiv.org/abs/1411.1784
[12] Z.Zhao,A.Kunar,Van,R.Birke,andL.Y.Chen, “CTAB‐GAN:EffectiveTableDataSynthesizing,” arXiv(CornellUniversity),Feb.2021.
[13] L.Xuetal.,“ModelingTabularDatausingCondi‐tionalGAN.”Available:https://proceedings.ne urips.cc/paper_files/paper/2019/file/254ed 7d2de3b23ab10936522dd547b78‐Paper.pdf (accessedDec5,2023).
[14] A.M.AnnaswamyandMassoudAmin, IEEE VisionforSmartGridControls:2030andBeyond Piscataway,UsaIeee,2013.
[15] M.Shanker,M.Y.Hu,andM.S.Hung,“Effectof datastandardizationonneuralnetworktrain‐ing,” Omega,vol.24,no.4,pp.385–397,Aug. 1996,doi:10.1016/0305‐0483(96)00010‐2.
[16] S.HochreiterandJ.Schmidhuber,“Long Short‐TermMemory,” NeuralComputation, vol.9,no.8,pp.1735–1780,Nov.1997,doi: 10.1162/neco.1997.9.8.1735.
[17] I.Banerjeeetal.,“Comparativeeffectivenessof convolutionalneuralnetwork(CNN)andrecur‐rentneuralnetwork(RNN)architecturesfor radiologytextreportclassi ication,” Arti icial IntelligenceinMedicine,vol.97,pp.79–88,Jun. 2019,doi:10.1016/j.artmed.2018.11.004.
[18] “RecurrentNeuralNetworksinMedicalData AnalysisandClassi ications,” AppliedComputing inMedicineandHealth,pp.147–165,Jan.2016, doi:10.1016/B978‐0‐12‐803468‐2.00007‐2.
[19] C.Ferri,J.Hernández‐Orallo,andR.Modroiu, “Anexperimentalcomparisonofperformance measuresforclassi ication,” PatternRecognition Letters,vol.30,no.1,pp.27–38,Jan.2009,doi: 10.1016/j.patrec.2008.08.010.
[20] D.J.HandandR.J.Till,“ASimpleGenerali‐sationoftheAreaUndertheROCCurvefor MultipleClassClassi icationProblems,” Machine Learning,vol.45,no.2,pp.171–186,2001,doi: 10.1023/a:1010920819831.
[21] M.Kassjański,M.Kulawiak,andTomaszPrze‐woźny,“DevelopmentofanAI‐basedaudiogram classi icationmethodforpatientreferral,” ComputerScienceandInformationSystems(FedCSIS), 2019FederatedConferenceon,Sep.2022,doi: 10.15439/2022f66.
[22] L.B.V.deAmorim,G.D.C.Cavalcanti,andR.M. O.Cruz,“Thechoiceofscalingtechniquemat‐tersforclassi icationperformance,” AppliedSoft Computing,vol.133,p.109924,Jan.2023,doi: 10.1016/j.asoc.2022.109924.
ANALYSISOFDATASETLIMITATIONSINSEMANTICKNOWLEDGE‐DRIVEN
Submitted:27th December2023;accepted:10th March2024
MarcinSowański,JakubHościłowicz,ArturJanicki
DOI:10.14313/JAMRIS/3‐2024/20
Abstract:
Inthisstudy,weexploretheimplicationsofdataset limitationsinsemanticknowledge‐drivenmachinetrans‐lation(MT)forintelligentvirtualassistants(IVA).Our approachdivergesfromtraditionalsingle‐besttransla‐tiontechniques,utilizingamulti‐variantMTmethodthat generatesmultiplevalidtranslationsperinputsentence throughaconstrainedbeamsearch.Thismethodextends beyondthetypicalconstraintsofspecificverbontologies, embeddingwithinabroadersemanticknowledgeframe‐work.
Weevaluatetheperformanceofmulti‐variantMT modelsintranslatingtrainingsetsforNaturalLanguage Understanding(NLU)models.Thesemodelsareapplied tosemanticallydiversedatasets,includingadetailed evaluationusingthestandardMultiATIS++dataset.The resultsfromthisevaluationindicatethatwhilemulti‐variantMTmethodispromising,itsimpactonimproving intentclassification(IC)accuracyislimitedwhenapplied toconventionaldatasetssuchasMultiATIS++.However, ourfindingsunderscorethattheeffectivenessofmulti‐varianttranslationiscloselyassociatedwiththediversity andsuitabilityofthedatasetsutilized.
Finally,weprovideanin‐depthanalysisfocusedon generatingvariant‐awareNLUdatasets.Thisanalysis aimstoofferguidanceonenhancingNLUmodelsthrough semanticallyrichandvariant‐sensitivedatasets,maxi‐mizingtheadvantagesofmulti‐variantMT.
Keywords: machinetranslation,intelligentvirtualassis‐tants,naturallanguageunderstanding
1.Introduction
Multilingualnaturallanguageunderstanding (NLU)modelsareamajorfocusinnaturallanguage processing(NLP)astheyenablevirtualassistantsto managemultiplelanguages.However,thescarcity ofmultilingualtrainingdataoftenleadstounder‐representationofsomelanguages.Whilethemanual translationoftrainingsentencescanaddressthis problem,itisatime‐consumingandcostlyprocess pronetoerrorsandambiguitiesthatcancompromise modelquality.Moreover,manualtranslationstruggles toadapttolanguagechangesortheintroductionof newlanguagestothevirtualassistant.
Inthiscontext,usingmachinetranslation(MT) systemsasasourceoftranslationsseemstobean attractivealternativeforacquiringmultilinguallearn‐ingdata.CreatingmultilingualNLUmodelsbytrans‐latingalearningsentenceintomultiplelanguages usingMTmodelsseemspossibleandpromising.
MTsystems,usedtogeneratesentencesfortrain‐ingNLUmodels,shouldproducemultiplecorrect translationvariants.Thisiscrucialaslanguagesoften havenumerousgrammaticalformsandwaysofcon‐veyinginformation.Forinstance,Englishhasvari‐ousverbforms,suchasregular,irregular,andmodal verbs,withpotentiallydifferenttranslationsinother languages.IfanMTsystemgeneratesonlyonetrans‐lationvariant,theNLUmodelmightnotlearntorecog‐nizeothers,compromisingthemodel’squality.Hence, MTsystemsshouldcreatemultipleaccuratetransla‐tionvariantstocoverallpossiblepatterns,enhancing theperformanceofNLUmodels.
Figure 1 illustratestheschemaoftheMTsys‐temdiscussedinthisarticle.Sourceutterancesare translatedtothetargetlanguagewithMTsystem thatusesverbontology.Theresultingtranslations exhibitextensiveverbcoverage,andimprovementsin theNLUmodelcanbeobservedwhentheevaluation datasetencompassesmultiplevariants.
Intheearlystagesofmachinelearning,thecom‐monviewinthe ieldwasthatenhancingMTwithlin‐guisticresources,suchasdictionaries,wasnoteffec‐tive.
Figure1. SchemaofNLUtrainingcomparing single‐variantMTwithmultivariantMTutilizingverb ontologyforenhancedperformance
Thisviewemergeddespitenumerousinitialexplo‐rationsintotheintegrationoftheseresources.How‐ever,inthisarticle,wechallengethisnotion,propos‐ingthattheeffectivenessofaugmentingMTwithlin‐guistictechniquesishighlydependentonthedataset andspeci ictasksutilized.Wehavedesignedaseries ofexperimentstodemonstratethatincorporatinga verb‐ontologycanindeedenhanceMTperformance indownstreamtasks.Intasksthatareparticularly sensitivetoverbvariation,weaimtoshowthatthe augmentationofMTwithlinguisticresourcesremains aviableandpotentstrategy.
2.RelatedWork
Thisarticlereferstoearlymachinelearningefforts tointroducelinguisticresourcestoimprovethequal‐ityofNLUsystems.Moneglia[18]createdtheontology ofactionverbstoimprovetheperformanceofNLUand MTsystems.
Thisworkalsorelatestothemethodsofgenerating multiplecorrecttranslations.Fomichevaetal.[9]used MTmodeluncertaintytogeneratemultiplediverse translations.Inourwork,weusedconstrainedbeam searchproposedbyAndersonetal.[2]togenerate multiplecorrectvariantsoftranslations.
AnotherarearelatedtothisworkisusingMTto translatethetrainingresourcesofNLU.Gaspersetal. [10]use,MTtotranslatethetrainingsetofIVAand reportedimprovementinperformancecomparedto grammar‐basedresourcesandin‐housedatacollec‐tionmethods.Abujabaletal.[1]usedtheMTmodelin conjunctionwithanNLUmodeltrainedforthesource languagetoannotateunlabeledutterancesreporting that56%oftheresultingautomaticallylabeledutter‐anceshadaperfectmatchwithground‐truthlabels, and90%reductioninmanuallylabeleddata.
Inourexplorationoftheimpactofdatasetlimita‐tionsinsemanticknowledge‐drivenMTonNLUsys‐tems,weemployedamethodologythatalignswith theapproachesdetailedinSowanskietal.[25].This approachistwofold,involvingthedevelopmentofa verbontologyanditssubsequentapplicationinMT.
Figure2presentsthemethodto indverbequiva‐lentsinthetargetlanguagetoincreasethevariance oftrainingresources.Theverbontology,acentral elementofthismethod,wasderivedbyanalyzinga diversearrayofeightNLUcorpora.Inthisprocess,a primarysetofverbswasextracted,chosenfortheir prevalenceandsigni icancewithinthesecorpora.This setofverbswasthenlinkedtoVerbNet,utilizingLevin classestocategorizeverbsbasedontheirsyntactic andsemanticcharacteristics.ThislinkagetoVerbNet servedasafoundationalstepincreatingarobust verbontology.Theontologywasfurtherenrichedby incorporatingadditionalverbsthatweresemantically relatedtotheinitiallyextractedones,utilizingWord‐Netsynsetsforthispurpose.
ThismethodofexpansionthroughWordNet ensuredacomprehensiveandnuanced representationofverbsemanticsintheontology.
FortheapplicationofthisverbontologyinMT, themethodologyinvolvedusingthemultiverb_iva_mt library.Thislibraryisdesignedtoleveragetheverb ontologyforgeneratingmultipletranslationvariants foreachinputsentence,akeyfeatureofthemulti‐variantMTapproachweadopted.
Inassessingtheeffectivenessofthismulti‐variant MTmethodology,comparisonsweremadewithother translationmethodsforNLUresources.Thesemeth‐odsincludedsingle‐besttranslation,whichtypically producesthemostprobabletranslationforaninput sentence,back‐translation,aprocessoftranslating asentencetoadifferentlanguageandbacktothe original,samplingfromthemodeloutputprobability distribution,andtranslationsgeneratedusinglarge languagemodels(LLMs)likeGPT‐3.
Thismethodology,whichalignswiththeapproach usedinSowanskietal.[25],wasinstrumentalinour study.Itallowedustoinvestigatehowtheapplication ofaverbontologyinmulti‐variantMTcanin luence theperformanceofNLUsystems,especiallyinthe contextofIVA.Thisapproachwasnotonlycrucialin highlightingthepotentialofmulti‐variantMTbutalso providedacomparativeanalysiswithexistingtrans‐lationtechniques,therebyenrichingthediscussionon optimizingNLUsystems.
Inourstudy,weconductedtwosetsofexperi‐mentstoevaluatetheimpactofmulti‐variantMTon NLU.The irstexperimentutilizedtheMultiATIS++ dataset,speci icallyitsEnglish‐TurkishandEnglish‐Japanesesubsets,toexaminewhetheradatasetnot focusedonlinguisticvariantswouldshowimprove‐mentswithmulti‐variantMT.
Forthesecondexperiment,weshiftedourfocus totheLeyzerdataset,anEnglish‐Polishdatasetthatis designedtobeawareoflinguisticvariants.Thisexper‐imentaimedtoexploreifavariant‐orienteddataset willshowpositivein luenceofthemulti‐variantMT.
Inbothexperiments,wecomparedbaselineNLU modelstrainedonuntranslateddatawithmodelsthat usedtwotranslationapproaches:thestandardsingle‐besttranslationandourproposedmulti‐verbtrans‐lation.Thesingle‐bestmethodusesabeamsearch algorithmtoproduceonelikelytranslation,whileour multi‐verbapproachgeneratesmultipletranslations guidedbyverbontology,aimingtocapturelinguistic richnessinexpressingthesameintent.
Theseexperimentscollectivelyaimtoshedlight onhowincorporatinglinguisticknowledgeintoMT cansigni icantlyenhanceNLUsystems,particularlyin datasetsthataredesignedtoaccommodatelinguistic diversityinexpressingintents.
4.1.Data
InourexperimentsweusedtwoNLUdatasets: MultiATIS++andLeyzer.
INTENT1(email_query)
findallemails readmethelastemail checkmyemails
INTENT2(news_query)
findnewsaboutbrexit readmenewheadlines showmenewsabout(...)
CLASS13.1
CLASS13.2
CLASS13.3
CLASS13.4
CLASS13.5
CLASS13.6
CLASS13
{give,pass,rent} {submit} {extend,grant} {provide,present}
{find,get,call,take,save,...} {change,exchange,replace}
GETEN SYNSET
GET LEMMAS find.v.03
GETTGT LEMMAS
Lemma('find.v.03.find'), Lemma('find.v.03.regain')
Lemma('find.v.03.encontrar'), Lemma('find.v.03.recuperar')
Figure2. OverviewofthemethodtofindnewverbsvariantsforIVAproposedin[25].NLUverbsarematchedtoVerbNet, whichconsistsofaWordNetsynsetfromwhichalemmainthetargetlanguagecanbeextracted
TheMultiATIS++dataset[29]isanexpandedver‐sionoftheoriginalAirTravelInformationSystem (ATIS)dataset,adaptedformultilingualNLUand designedtosupportresearchinmultilingualMTand NLU.
ThisdatasetwasformedbytranslatingtheEnglish ATISdatasetintomultiplelanguageswhilekeeping theoriginalsentencestructuresandsemanticannota‐tions.Itincludesover40,000sentencesacrossvarious domainssuchas lightinformation,faredetails,and groundservices.Thecarefulprocessoftranslatingand adaptingitintoseverallanguages,likeSpanish,Ger‐man,andFrench,makesMultiATIS++avaluabletool fortrainingandevaluatingMTsystemsindifferent languagesettings.
Weusedthesecondversion(0.2.0)oftheLeyzer1 datasettoconducttheexperiments.Leyzerisamul‐tilingualdatasetcreatedtoevaluatevirtualassis‐tants.Itcomprises192intentsand86slotsacross threelanguages(English,Polish,andSpanish)and 21IVAdomains.WeselectedLeyzertoconductour experimentsbecauseeachintentcomprisesseveral verbpatternsandlevelsofnaturalness.Forexam‐ple, ChangeTemperature intent,whichrepresentsthe goalofchangingthetemperatureofahomethermo‐statsystem,distinguishesthreelevelsofnaturalness, wherethemostnaturalway(level0)ofutteringthis goalbytheuserwouldbetosay“changetemperature onmythermostat”,lessnatural(level1)wouldbe“set thetemperatureonmythermostat”,and inallyleast natural(level2)yetstillcorrectwouldbe“modifythe temperatureonmythermostat”.Thesetwopiecesof informationthatarealsoavailableinthetestsetofthe Leyzercorpusallowustomeasuretheimpactofthe multi‐verbtranslationbetter.
ThetrainingsubsetofPolishcorporathatweused inthesecondexperimentincludes15748trainutter‐ances,4695developmentutterances,and5839test utterances.TheEnglishsubsetofcorporathatweused totranslateandreportresultsofsingle‐bestandmulti‐verbincludes17289trainingandvalidationutter‐ances.Weextracted3997utterancesfromthetrans‐latedtrainingsetforvalidation,ensuringatleastone sentenceisavailableforeveryintent,level,andverb pattern.
WeusedverbontologyforIVAs[25]togener‐atemultiplevariantsoftranslations.Inourexperi‐mentsweusedEnglish‐to‐Polish[22]andEnglish‐to‐Turkish[23]models.Wetestedmulti‐variantMTon theNLUtrainingsettranslationtask,whereEnglish corporaweretranslatedtoPolish,andtheNLUmodel wastrainedfromthem.Inourexperiments,weshow thatverbontologycanimproveICresultsonlyintasks (datasets)whereverbdiversityistakenintoaccount.
4.3.NaturalLanguageUnderstanding
InthecaseofexperimentsontheLeyzerdataset, weusedmultilingualXLM‐RoBERTa[7]modelsfor intentclassi ication(IC)andslot‐ illing(SF).Wechose thisarchitectureforNLUasitcanbeeasilycompared tomodelspresentedinMASSIVEandachievesbetter resultsinamultilingualsettingwhencomparedto multilingualBERT(mBERT).FortheMultiATIS++we appliedasimilarapproachbuttopreservecompara‐bilitywithbaselines[6,19]weusedmBERTasNLU coremodel.
XLM‐RoBERTawastrainedon2.5TBof iltered CommonCrawldatacontaining100languages.During ine‐tuning,weusedAdam[14]foroptimizationwith aninitiallearningrateof2��−5
ThequalityoftheICmodelwasevaluatedusing theaccuracymetricthatrepresentsthenumberof utterancescorrectlyclassi iedtothegivenintent.SF modelwasevaluatedusingamicro‐averagedF1‐score.
4.4.ComparativeAnalysisofMulti‐VariantTranslation Methods:Back‐translation,Sampling,andGPT‐3 InthedomainofMT,generatingmultiplevariants ofatranslationhasbeenafocalpointforenhanc‐ingtherobustnessandexpressivenessoftranslated text.Twoprevailingtechniquesforgeneratingthese variantsareback‐translation[21]andsampling[28], whichhavebeenwidelyadoptedduetotheirproven effectivenessingeneratingdiverseyetcoherenttrans‐lations.Back‐translationinvolvestranslatingasen‐tencetoatargetlanguageandthenbacktothesource language,whileSamplingusesprobabilisticmodelsto choosedifferentpossibletranslations.Thesemethods serveasstrongbaselinesforevaluatinginnovative approachestoMT.
Inthissection,wecompareourMTlibrary,which leveragesacustomverbontologyforgeneratingtrans‐lationvariants,againstthesewell‐establishedtech‐niques.
Weaimtodemonstratetheadvantagesofincorpo‐ratingsemanticunderstandingthroughverbontology ingeneratingmultipletranslationvariants.
Anothercontemporaryapproachtogenerating multipletranslationvariantsinvolvesusinglarge‐scalelanguagemodelslikeGPT‐3,speci icallyits textdavinci-003 version.Byemployingasophisticated promptingmechanism,GPT‐3cangeneratemany coherentandcontextuallyrelevanttranslationvari‐ants.Brownetal.[4]havedemonstratedthatGPT‐3performsatornearstate‐of‐the‐artlevelsacross awiderangeofNLPtasks,makingitacompelling baselineforcomparison.Inthisstudy,weutilizeGPT‐3asanadvancedcontrolgroup,contrastingitsperfor‐mancewithBackTranslation,Sampling,andourverb ontology‐basedmethodtoprovideacomprehensive evaluationlandscape.
4.5.ImpactofMulti‐verbonBaselineDataset(Multi‐ATIS++)
InTable1,weexaminedtheperformanceoflow‐resourcelanguages,speci icallyJapaneseandTurkish, usingtheMultiATIS++datasetfortesting.Thisdataset, aprominentbenchmarkinNLU,waschosenforits limitedfocusonutterancediversity,acommontraitin manyNLUdatasets.Ourgoalwastodemonstratethat datasetsnotdesignedtoencompassawiderangeof utterancevariantsmaynotsigni icantlybene itfrom multi‐variantMTapproaches.Our indingsshowthat, insuchcontexts,themulti‐variantMTmethodout‐performsFC‐MTLF[6],thecurrentstate‐of‐the‐art,in bothintentaccuracyandslot ��1 score.However,the applicationofmulti‐verbMTdoesnotyieldimproved resultsoversingle‐bestMTinthisscenario.
WhencomparedtobothFC‐MTLFandGL‐CLeF[19],whicharebasedonconceptslike contrastivelearningormultitasklearning,our approachdoesnotrequireachangeofproduction inNLUarchitecture.ThefactthatitisbasedonMT oftrainingdatamakesiteasilyapplicableinvarious productionenvironments(includingOn‐Device).
4.6.ImpactofMulti‐verbTranslationonaVerb‐aware Dataset(Leyzer)
Toassesstheef icacyoftheproposedmultivari‐anttranslationtechnique,asetofexperimentswas designedtocompareitagainstestablishedparaphrase generationalgorithms.Forcontextualevaluation,two referencemodelsarealsointroduced.Thesereference modelsaretrainedandtestedsolelyonanuntrans‐latedsubsetofthedatasetinquestion.
TheexperimentalsetupemploystheEnglishtrain‐ingcorpusfromtheLeyzerdataset,comprising17,290 utterances.Eachmethodtranslatestheseutterances intoPolish,generatingmultipletranslationvariants intheprocess.Subsequently,thetranslatedoutput ispartitionedintoanewtrainingandvalidationset, followingan80:20ratio.TheInferentialConsistency (IC)andSemanticFidelity(SF)models,ifapplicable, arethentrainedonthesesets.Evaluationisconducted usinganindependentPolishtestsetthathasnot undergonetranslation.
Intheprecedingsection,themethodologiesof Back‐translation,Sampling,andChatGPTprompting havebeenelaborated.Forsingle‐besttranslation,the methodtermed“Single‐bestIVA”isemployed;thisuti‐lizestheM2M100modeladaptedfortheIVAdomain andidenti iesthemostaccuratetranslationusing abeam‐searchalgorithm.Conversely,themulti‐verb translationapproachgeneratesanarrayoftranslation alternatives.Thisisachievedthroughaconstrained beamsearch,steeredbytheproposedverbontol‐ogy,toyieldmultiplesemanticallynuancedoutput variants.
Table 2,presentstheimpactofmultiplevariant generationonICandSFmodelresults.Reference modelsinEnglishandPolishyieldresultsabove95% forbothICandSF,af irmingthathigh‐qualitytrans‐latedtrainingdatacanleadtostrongperformance metrics.Asforthemethodsaimedatgeneratingmul‐tipletranslationvariants,Back‐translationandSam‐plingachievelowerperformance,withintentaccu‐raciesof77.07%and79.00%,respectively.Although popular,thesemethodsdemonstrateanoticeableper‐formancegapcomparedtothereferencemodels.GPT‐3prompting,ontheotherhand,performssigni icantly betterwithanintentaccuracyof84.58%,thoughit stillfallsshortofthereferencemodels.Ourproposed method,multi‐verbtranslation,outperformsallother methodswithanintentaccuracyof87.53%,closely approachingthehigh‐performancebenchmarkssetby thereferencemodels.Theseresultsunderscorethe effectivenessofgeneratingtranslationvariantsbased onverbontology,especiallywhencomparedtoBack‐translation,Sampling,andGPT‐3prompting.
Themulti‐verbimprovementtothetranslation generationpositivelyimpactsICmodelresultsin Leyzer(verb‐diverse).Theaccuracyofmulti‐verb translationis3.8%,relativelybetterthansingle‐best translation.However,itis7.95%relativelylowerthan thebaselinemodel.AspresentedinTable 3,each Englishsentencegeneratesanaverageof2.63Polish translations.This,inouropinion,isthemainfactorof whymulti‐verbtranslationgeneratesabettertraining datasetfortheICmodel.Leyzertestsetevaluates multiplevariantsinwhichgivenintentcanbeuttered, includingdifferentlevelsofnaturalnessandverbpat‐terns;therefore,morevarianttrainingsetimproves results.FurtherimprovementstoICcouldbemadeif morevariantswerecreatedinverbontology.
Table1. ComparisonofNLUIntentAccuracyandSlot ��1‐scorebetweenbaselines,single‐besttranslation,andmulti‐verb translationonMultiATIS++dataset(JapaneseandTurkish)
Method
Figure3. VerbfrequencyandverbpositionontherankinglistforselectedVAdatasetspresentedinlogarithmicscale
Table2. ComparisonofNLUIntentAccuracyandSlot ��1‐scorebetweenbaseline,single‐besttranslation,and multi‐verbtranslationontheLeyzerdataset (English‐Polish)
5.InsightsintoIVALanguageandCorpusCon‐structionfromAnalyzingLevinClasses
IVAcommandscanbesimpli iedasacomposition ofaverbanditsparameters.Westartourinvestigation byanalyzingverbsfromtheeightmostpopularNLU corpora,asthisallowsustogaincrucialinformation abouttheeventoractionbeingdescribed[17].
InTable 4,thetoptenmostfrequentverbsinall NLUcorporaarepresented.Thehighest‐rankedverbs representmostfrequentlyusedfeaturesofvirtual assistants:calendar,alarm,andmusicdomains,which explainwhygivenverbsaremostpopular.
Table3. Averagenumberoftargetverbsgeneratedin verbontologythatcorrelateswiththenumberof variationsthatwillbegeneratedforasingleinput Englishsentence
Multi‐verbtranslationdoesnotimprovethe resultsoftheSFmodel.Ourmethoddoesnot generatedifferentvariantsofslotvalues;therefore, duringtraining,theSFmodelcannotgeneralizeto newtestcases.Thedifferencein ��1‐scorebetween single‐bestandmulti‐variantisnotstatistically signi icant.
Whileanalyzingverbfrequency,wenoticedthat eachNLUcorpuspresentsthesametrendwherethe mostfrequentverbscanbefoundinaround20%of utterances.Figure 3 illustratesthatthetrendinIVA corporacloselyresemblestheZipfdistribution,albeit withsomedeviations.Asimilartrendcanbefoundin otherlinguisticresources,forexample,VerbNet[13].
VerbsextractedfromNLUcorporaoftenspanmul‐tipledomains.Forinstance,theverb set couldbeused tosetanalarmoradjustscreenbrightness.Toaddress thiscomplexity,weutilizedLevin’sverbclassi ica‐tion[15]tocategorizeverbsofsimilarsemanticprop‐erties.Levinclassi ied3,024verbsinto48broadand 192 ine‐grainedclassesbasedonpatternsofsyntactic alternationsthatcorrelatewithsemanticproperties. Theseclassesareemployedinthisarticletoidentify IVAverbframes.AlthoughLevin’sclasseswereini‐tiallydesignedtounderstandsyntacticandsemantic alternationsinverbs,theycanbeadaptedtocompre‐hendIVAcapabilities.Thekeyistointerpretthese verbsinthecontextofvirtualactionsandoutputs. WhileIVAscannotperformallhumantasks,theycan simulateawidearrayofactionsinavirtualsetting.
Table4. Top10EnglishverbsfromoccurrencerankingandoccurrencefrequencyineachofselectedNLUcorpora Dataset SetShowRemindPlayGive TellAddFindMakeCancel
Leyzer[24]0.7%11.6%0.3%1.1%6.5%1.2%1.9%6.4%4.6%0.1% MASSIVE[8]1.8%1.5%1.3%4.6%1.1%2.7%1.5%1.12%0.9%0.3% MTOD[20]15.4%3.1%10.8%0.0%0.4%0.5%0.7%0.1%0.2%5.5% MTOP[16]6.2%2.1%4.7%3.5%1.2%1.9%1.4%1.0%1.2%0.8% PRESTO[11]0.4%3.1%0.2%0.7%0.3%0.9%4.0%1.0%1.2%1.2%
SLURP[3]1.8%1.5%1.3%4.6%1.1%2.7%1.5%1.1%0.9%0.3%
TOP[12]0.1%0.7%0.1%0.1%0.7%1.0%0.1%0.4%0.1%0.1%
NLU++[5]0.1%0.2%0.0%0.0%0.1%0.3%0.0%0.0%0.3%0.2%
Whileautomatedverbclassi icationmethodshave beenexplored[26],theseapproachesprimarilyfocus ongenerallanguageandrelyonsyntacticfeatures.
Theyhaveshownpromisingresultsinclassifying verbsintoLevinclasses,buttheirapplicabilitytothe specializedlanguageofIVAsremainsuncertain.Anno‐tatedcorporaandtheorieslikespeechacttheory[27] providevaluableinsightsintohuman‐machineinter‐actions.However,theyoftendonotfocusonthespe‐ci icverbsemployedinIVAs,norarethereresources readilyavailablefortheautomaticorsemi‐automatic classi icationofsuchverbs.Thiscreatesaveri ica‐tionchallenge,asexistingmethodscannotbede ini‐tivelycross‐referencedforaccuracyinthisspecialized domain.Therefore,wedevelopedourownclassi ica‐tionmethodtobetteraddresstheuniquelinguistic featuresofIVAinteractions.
Below,wepresentverbsfoundinNLUcorporathat havebeensuccessfullymatchedtoVerbNetclasses. Usingthoseclasses,otherinstances(verbs)ofthe sameframecanbefound.Thetenmostfrequent classesfoundinNLUcorporaare:
5.1.VerbsofChangeofPossesion(Class13)
Representing10.73%ofIVAinteractionsfromana‐lyzedcorpora,predominantlyfacilitatetransactions ofgoods,services,orinformationbetweentheuser andtheassistant.ThisclassiscentraltoIVAfunc‐tionality,asitmirrorseverydayexchangeswhere userscommandtheassistanttoretrieve,provide,or exchangeitems.Forinstance,ausermightuse“give” torequestspeci icdata(“givemetheweatherfore‐cast”),or“order”fore‐commercepurposes(“ordermy usualpizza”).TheseverbsembodythecoreofIVA‐user interactions:theassistantactingasanintermediaryin obtainingordeliveringwhattheuserneeds.
IncorporatingdiversevariantsinClass13isessen‐tialtodevelopanIVAcapableofhandlingvarious transactionaltasks.Thisapproachnotonlyallows theIVAtounderstandandrespondtonuanceduser requestsbutalsoenhancesitsversatilityanduser engagement.Toexpandthedatasetwithmorevari‐antsinClass13,thefollowingstrategiescanbe applied:
1) ContextualAdaptations:Lookatexistingverbsin theclassandbrainstormcontext‐speci icvaria‐tions.Forexample,“give”(13.1)couldextendto “handover”inscenariosofphysicalitemexchange, or“transfer”indigitalcontexts.
2) SemanticExpansion:Introduceverbswithsimilar meaningsbutdifferentnuances.Forinstance, alongside“buy”(13.5.1),include“purchase” (13.5.2)tocoverformaltransactions,or“acquire” forabroadersenseofobtainingsomething.
3) SynonymsandCollocations:Utilizesynonymsthat itdifferentinteractionstyles.“Order”(13.5.1)can beexpandedto“request”formoreformalorpolite interactions,and“book”(13.5.1)to“reserve”for appointmentsorservices.
4) Cross‐ClassIntegration:Someverbsbelongtomul‐tipleclasses,like“pass”(11.1,13.1).Exploresuch verbstoprovidecross‐contextualunderstanding. Forinstance,“exchange”(13.6)couldbepaired with’trade’toencompassbarter‐likeinteractions.
5) UserIntentVariability:Addverbsthatchange meaningbasedoncontext.“Get”(13.5.1)might mean“acquire”inashoppingcontextbut“under‐stand”inaninformationalone.
6) Action‐Speci icVerbs:Includeverbsspeci ic toIVAcapabilities,like“retrieve”(13.5.2)for dataretrievaltasks,or“grantaccess”(13.3)for permission‐relatedactions.
7) ExtensionExamples:From“rent”(13.1):Expand to“lease”forlong‐termagreements,or“hire”for services.From’save’(13.5.1):Include“store”for datapreservation,or“archive”forlong‐termstor‐age.From“provide”(13.4.1):Extendto“supply” forcontinuousprovision,or“furnish”forequip‐pingwithnecessaryitems.From“select”(13.5.2): Add“choose”forpersonalpreferencescenarios,or “pickout”formorecasualselections.
5.2.VerbsofCommunication(Class37) Class37,encompassing9.34%ofIVAverbs,ispiv‐otalinfacilitatinginformationandactionrequests. TheseverbsrepresenttheIVA’sevolutionfromabasic tooltoasophisticatedcommunicationfacilitator.To constructaversatileIVAdataset,anuancedunder‐standingoftheseverbsandtheirvariancesiscru‐cial.Thisunderstandingnotonlyensuresaccurate responsestouserqueriesbutalsobroadenstheIVA’s communicationabilities,enhancinguserinteraction.
VerbsinClass37areintegralforrequestinginfor‐mation(“ask”,“inquire”)orspeci icactions(“tellme thenews”,“explainthistopic”).Theyalsoinclude verbsforindirectcommunication(“email”,“phone”), re lectingtheIVA’sroleinfacilitatingdigitalinterac‐tions.ThisclasshighlightstheIVA’scapabilitytohan‐dlevariouscommunicationforms,fromdirectcom‐mandstomorecomplex,context‐dependentrequests.
ToenrichClass37forDiverseCommunication Needs:
1) ContextualVariability:Incorporateverbsusedin differentcommunicationstylesandcontexts.For example,alongside“tell”(37.1),include“inform” forformalscenariosor“relay”forindirectcommu‐nication.
2) SynonymsandColloquialisms:Usesynonymsto catertodiverseuserexpressions.“Chat”(37.6)can beexpandedwith“converse”foraformaltoneor “talk”(37.5)forcasualinteractions.
3) TechnologicalAdaptations:Giventhedigital natureofIVAs,includeverbslike“text”or “message”alongside“email”(37.4),re lecting moderncommunicationmethods.
5.3.VerbsofCreationandTransformation(Class26) Class26,constituting6.92%ofIVAverbs,playsa uniqueroleinIVAs,signifyingthecreationortransfor‐mationofvirtualoutputs.AlthoughIVAsdon’tengage inphysicalcreationoralteration,theyareinstru‐mentalingeneratingormodifyingdigitalcontentin responsetousercommands.
ThisclassincludesverbswheretheIVAactsasan agentto“create”or“transform”virtualentities.For example,“arrange”(26.1)in“arrangemymeetings” involvestheIVAorganizingdatatocreateastruc‐turedschedule.“Convert”(26.6),asin“convertUSD toEUR”,demonstratestheIVA’sabilitytotransform information,offeringanewformofoutput.Thisclass encapsulatestheIVA’scapabilitytoproduceoralter digitalinformationinameaningfulwayfortheuser.
StrategiesforEnrichingClass26inIVADatasets:
1) Context‐Speci icVariations:Extendverbstocover variousdigitalcreationortransformationscenar‐ios.For“make”(26.1),include“generate”forcre‐atingreportsor“fabricate”forcreating ictional responses.
2) Action‐OrientedVerbs:Addverbsthatrepresent speci icdigitalactions.“Compile”(26.1)couldbe expandedto“assemble”forgatheringinformation, or“synthesize”formergingdata.
3) SemanticEnrichment:Includeverbswithnuanced meanings.“Transform”(26.6)canbeaccompanied by“morph”forsubtlechanges,or“revise”foredit‐ingcontent.
DiverseverbsinthisclassempowertheIVAto handleavarietyofcreationandtransformationtasks, enhancingitsutilityanduserinteraction.Thisdiver‐sity:
ImprovesFunctionality:Awiderrangeofverbs allowstheIVAtounderstandandexecutemorecom‐plexcreationandtransformationtasks.EnhancesUser Interaction:Byaccuratelyinterpretingandrespond‐ingtovariedcommands,theIVAoffersamoredynamic andengagingexperience.CaterstoUserNeeds:Aver‐satileIVA,skilledinvariouscreationandtransfor‐mationtasks,meetsdiverseuserrequirements,from organizingdatatoconvertinginformation.
5.4.AspectualVerbs(Class55)
Thisiswhere5.19%oftheIVAverbsbelong.These verbsdescribetheinitiation,termination,orcontin‐uationofanactivity.Usersoftenemploytheseverbs tocontrolthestart,continuation,orcessationoftasks performedbytheVA.Therelationshipbetweenthe user’sutteranceandtheexpectedactionisdirect:the aspectualverbprovidesclearcuesaboutthedesired phaseofthetask,whetheritisaninitiation,continua‐tion,ortermination.
Toextendthisclasseffectively,considerthefollow‐ingstrategies:
1) InitiationVerbs:Focusonverbsthatsignalthe startofanactivity.Examplesinclude:
‐ “Initiate”:forformallybeginningaprocess.
‐ “Launch”:forstartingapplicationsordigitalpro‐cesses.
‐ “Activate”:forturningonfeaturesorfunctions.
2) ContinuationVerbs:Theseverbsindicatethe ongoingnatureofanactivity.Examplesinclude:
‐ “Proceed”:forcarryingonwithaprocess.
‐ “Sustain”:formaintainingongoingtasksoroper‐ations.
‐ “Persist”:toindicatecontinuousaction,espe‐ciallyunderchallengingcircumstances.
3) TerminationVerbs:Thesearecrucialforsignal‐ingtheendofanactivity.Examplesinclude:
‐ “Terminate”:forformallyconcludingaprocess.
‐ “Conclude”:forendingtaskswithasenseofcom‐pletion.
‐ “Cease”:forastrongindicationofstopping immediately.
5.5.VerbsofChangeofState(Class45)
Where4.50%oftheIVAverbsbelong.Allofthe verbsinthisclassrelatetothechangeofstate,with severalsub‐classesthatde inethisstateinmore detail.Whenusersemploytheseverbsintheirutter‐ances,theytypicallyexpecttheIVAtoeitherprovide informationrelatedtothechangeorexecuteanaction thatresultsinthedesiredchange.Therelationship betweentheuser’sutteranceandtheexpectedaction isdirect:theverbprovidesclearcuesaboutthenature anddirectionofthedesiredchange.
Toeffectivelyextendthisclass,focusonverbsthat signifyspeci ictypesofstatechanges.Forinstance, includeverbslike“transform”forcomprehensive changes,“adjust”forminormodi ications,and“revise” forcorrectionsorupdates.Additionally,consider context‐speci icverbslike“upgrade”fortechnology‐relatedchangesor“refresh”forupdatinginforma‐tion.ThistargetedapproachensuresthattheIVAcan accuratelyinterpretandrespondtoawiderangeof state‐changingcommands,enhancingitsresponsive‐nessandutility.
5.6.VerbsofPutting(Class9)
Where4.15%oftheIVAverbsbelong.These verbsrefertoputtinganentityatsomelocation.For instance,usersmightusePutVerbstosetreminders orarrangetasks.E.g.,“Setareminderfortomorrow.” withVerbsofPuttinginSpatialCon iguration,“suspend”isrelevantincontextslikepausingtasksor suspendingprocesses.FunnelVerbscouldbeusedin contextslikeaddingitemstolistsorpushingtaskstoa queue.Finally,CoilVerbsareconnectedwithprogram‐mingcapabilities,i.e.,“loop”mightbeusedtoindicate repetitivetasks.
5.7.VerbsofPredicativeComplements(Class29)
Thisiswhere4.15%oftheIVAverbsbelong.Verbs belongingtothatclassarefoundationaltohuman communication,especiallywhenseekinginforma‐tion,validation,orexpressingopinions.Whenusers employtheseverbsintheirinteractionswithIVAs, theytypicallyexpecttheassistanttoproviderelevant information,con irmtheirbeliefs,orassistincate‐gorizingornamingitems.AppointandCharacterize Verbsareusedwhenseekingspeci icinformationor categorization.Forinstance,thiscanbeseenin“How wouldyouratethissong?”or“Describethisimage.” DubVerbscanbeusedincontextslikenamingalarms orplaylists,e.g.,“Callthisplaylist“WorkoutTunes”. DeclareVerbsmightbeusedtoexpressopinionsor seekvalidation,e.g.,“Ibelieveitisgoingtoraintoday. Whatdoyouthink?”.ConjectureVerbscanbeused whenusersareunsureaboutsomethingandseekthe assistant’sinput.Forexample,“Iguessitislate.What’s thetime?”.
5.8.VerbsofSendingandCarrying(Class11)
Where3.81%oftheIVAverbsbelong.Users employtheseverbstocommandtheIVAtotrans‐fer,move,orretrieveinformationorperformspeci ic tasksrelatedtosendingandcarrying.Recognizing theseverbsandtheirnuancesiscrucialforIVAsto ensuretheyrespondappropriatelytousercommands, especiallyincontextslikemessaging,reminders,and navigation.SendVerbsarefrequentlyusedinthecon‐textofmessagedispatching.Forinstance,usersmight say,“SendthismessagetoJohn”or“Mailthisdocu‐menttomyboss.”TheexpectedactionisfortheIVAto facilitatethedispatchingofthemessageordocument totheintendedrecipient.BringandTakeverbscanbe employedincommandslike“Bringupmylastemail” or“Takemetothehomescreen.”
TheuserexpectstheIVAtoretrievespeci icinfor‐mationornavigatetoaparticularinterface.Carry Verbsmightbeusedmetaphorically.Forinstance, “Carrythisreminderovertotomorrow”wouldmean theuserwantstheIVAtorescheduleareminder.
5.9.VerbsofRemoving(Class10)
Where3.11%oftheIVAverbsbelong.Therela‐tionshipbetweenusersemployingtheseverbsand theexpectedactionisthatuserscommandtheIVAto remove,eliminate,orre inesomething.RemoveVerbs arecommonlyusedintaskslike ilemanagementor editing.Forinstance,“Deletethethirdparagraph”or “Removethiscontactfrommylist.”BanishandClear Verbsmightbeusedincontextslikeclearingnoti ica‐tions,“Clearallmynoti ications”,ormanagingtasks, and“RecalltheemailIjustsent.”
5.10.VerbsofAssumingPosition(Class51)
Thisiswhere2.77%oftheIVAverbsbelong.The relationshipbetweenusersemployingtheseverbs andtheexpectedactionisthatusersarecommanding theIVAtonavigate,guide,ormovethroughdigital spacesortasks.VerbsofInherentlyDirectedMotion canbeusedinnavigationaltasksorbrowsing.For example,“Gotothenextemail”or“Exitthecurrent application.”LeaveVerbsinadigitalcontextmightbe usedas“Leavethisgroupchat”or“Leavethecurrent session.”MannerofMotionVerbscanbemetaphori‐callyusedindigitaltasks.Forinstance,“Slidetothe nextphoto”or“Jumptothemainmenu.”ChaseVerbs canbeusedin“Followthelatestnewsonthistopic”or “Followthisartistonmymusicapp.”
Inconclusion,ourstudyrevealsthatwhilemulti‐variantMTshowspromise,itsef icacyissigni icantly contingentonthediversityoftheinputdataset.The experimentsconductedusingtheMultiATIS++and Leyzerdatasetsdemonstratethatincontextswhere linguisticdiversityisnotaprimaryfocus,asinthe caseofMultiATIS++(withintentaccuracyimprove‐mentsfrom84.65%to84.83%inEnglish‐Japanese translations),theadvantagesofmulti‐variantMTare negligibleorevennegative(asincaseofEnglish‐Tukrish).However,inmorevariant‐richenviron‐mentslikeLeyzer,there’sanotableimprovementin intentaccuracy(from83.73%to87.53%inEnglish‐Polishtranslations),underliningtheimportanceof datasetselectioninleveragingmulti‐variantMT.Fur‐thermore,thepracticalanalysisofverbclassesoffers valuableinsightsforNLUdatasetcreation,extend‐ingitsutilitybeyondspeci iclinguisticsettingsto abroaderrangeofapplicationsinvirtualassistant development.Thisstudyunderscorestheneedfor carefuldatasetcuration,particularlyincapturinglin‐guisticdiversity,tofullyexploitthebene itsofmulti‐variantMTinNLUsystems.
Notes 1Datasetavailableathttps://github.com/cartesinus/leyzer
AUTHORS
MarcinSowański∗ –TCLResearchEuropeul. Grzybowska5A,00‐132Warsaw,Poland,e‐mail: marcin.sowanski@tcl.com.
JakubHościłowicz –SamsungR&DInstitutePoland‐placEuropejski1,00‐844Warsaw,Poland,e‐mail: j.hoscilowicz@samsung.com.
ArturJanicki –WarsawUniversityofTechnologyul. Nowowiejska15/19,00‐665Warsaw,Poland,e‐mail: artur.janicki@pw.edu.pl.
∗Correspondingauthor
References
[1] A.Abujabal,C.D.Bovi,S.‐R.Ryu,T.Gojayev, F.Triefenbach,andY.Versley,“Continuous modelimprovementforlanguageunderstanding withmachinetranslation”.In: NorthAmerican ChapteroftheAssociationforComputationalLinguistics,2021.
[2] P.Anderson,B.Fernando,M.Johnson,and S.Gould,“Guidedopenvocabularyimagecap‐tioningwithconstrainedbeamsearch”.In: Proceedingsofthe2017ConferenceonEmpirical MethodsinNaturalLanguageProcessing,2017, 936–945.
[3] E.Bastianelli,A.Vanzo,P.Swietojanski,and V.Rieser,“SLURP:ASpokenLanguageUnder‐standingResourcePackage”.In: Proceedingsof the2020ConferenceonEmpiricalMethodsinNaturalLanguageProcessing(EMNLP),2020.
[4] T.Brown,B.Mann,N.Ryder,M.Subbiah,J.D. Kaplan,P.Dhariwal,A.Neelakantan,P.Shyam, G.Sastry,A.Askell,etal.,“Languagemodels arefew‐shotlearners”, Advancesinneuralinformationprocessingsystems,vol.33,2020,1877–1901.
[5] I.Casanueva,I.Vulić,G.Spithourakis,and P.Budzianowski,“Nlu++:Amulti‐label,slot‐rich,generalisabledatasetfornaturallanguage understandingintask‐orienteddialogue”.In: FindingsoftheAssociationforComputational Linguistics:NAACL2022,2022,1998–2013.
[6] X.Cheng,W.Xu,Z.Yao,Z.Zhu,Y.Li,H.Li,and Y.Zou,“Fc‐mtlf:a ine‐andcoarse‐grainedmulti‐tasklearningframeworkforcross‐lingualspo‐kenlanguageunderstanding”.In: Proceedingsof Interspeech,2023.
[7] A.Conneau,K.Khandelwal,N.Goyal,V.Chaud‐hary,G.Wenzek,F.Guzmán,É.Grave,M.Ott, L.Zettlemoyer,andV.Stoyanov,“Unsupervised cross‐lingualrepresentationlearningatscale”. In: Proceedingsofthe58thAnnualMeetingofthe AssociationforComputationalLinguistics,2020, 8440–8451.
[8] J.FitzGerald,C.Hench,C.Peris,S.Mackie, K.Rottmann,A.Sanchez,A.Nash,L.Urbach, V.Kakarala,R.Singh,S.Ranganath,L.Crist, M.Britan,W.Leeuwis,G.Tur,andP.Natara‐jan,“MASSIVE:A1M‐examplemultilingualnat‐urallanguageunderstandingdatasetwith51 typologically‐diverselanguages”.In:A.Rogers, J.Boyd‐Graber,andN.Okazaki,eds., Proceedingsofthe61stAnnualMeetingoftheAssociationforComputationalLinguistics(Volume1: LongPapers),Toronto,Canada,2023,4277–4302,10.18653/v1/2023.acl‐long.235.
[9] M.Fomicheva,L.Specia,andF.Guzmán,“Multi‐hypothesismachinetranslationevaluation”.In: Proceedingsofthe58thAnnualMeetingofthe AssociationforComputationalLinguistics,2020, 1218–1232.
[10] J.Gaspers,P.Karanasou,andR.Chatterjee, “Selectingmachine‐translateddataforquick bootstrappingofanaturallanguageunderstand‐ingsystem”.In: ProceedingsofNAACL-HLT,2018, 137–144.
[11] R.Goel,W.Ammar,A.Gupta,S.Vashishtha, M.Sano,F.Surani,M.Chang,H.Choe,D.Greene, C.He,R.Nitisaroj,A.Trukhina,S.Paul,P.Shah, R.Shah,andZ.Yu,“PRESTO:Amultilingual datasetforparsingrealistictask‐oriented dialogs”.In:H.Bouamor,J.Pino,andK.Bali, eds., Proceedingsofthe2023Conferenceon EmpiricalMethodsinNaturalLanguage Processing,Singapore,2023,10820–10833, 10.18653/v1/2023.emnlp‐main.667.
[12] S.Gupta,R.Shah,M.Mohit,A.Kumar,and M.Lewis,“Semanticparsingfortaskoriented dialogusinghierarchicalrepresentations”.In: Proceedingsofthe2018ConferenceonEmpirical MethodsinNaturalLanguageProcessing,2018, 2787–2792.
[13] A.Huminski,F.Liausvia,andA.Goel,“Semantic rolesinverbnetandframenet:Statisticalanaly‐sisandevaluation”.In: ComputationalLinguisticsandIntelligentTextProcessing:20thInternationalConference,CICLing2019,LaRochelle, France,April7–13,2019,RevisedSelectedPapers, PartII,2023,135–147.
[14] D.P.KingmaandJ.Ba,“Adam:Amethodfor stochasticoptimization”.In: Proc.ofthe6thInternationalConferenceonLearningRepresentations (ICRL2015),SanDiego,CA,2015.
[15] B.Levin, Englishverbclassesandalternations:A preliminaryinvestigation,UniversityofChicago press,1993.
[16] H.Li,A.Arora,S.Chen,A.Gupta,S.Gupta, andY.Mehdad,“Mtop:Acomprehensivemul‐tilingualtask‐orientedsemanticparsingbench‐mark”.In: Proceedingsofthe16thConference oftheEuropeanChapteroftheAssociationfor ComputationalLinguistics:MainVolume,2021, 2950–2962.
[17] O.MajewskaandA.Korhonen,“Verbclassi ica‐tionacrosslanguages”, AnnualReviewofLinguistics,vol.9,2023.
[18] M.Moneglia,“Naturallanguageontologyof action:Agapwithhugeconsequencesfornatural languageunderstandingandmachinetransla‐tion”.In: LanguageandTechnologyConference, 2011,379–395.
[19] L.Qin,Q.Chen,T.Xie,Q.Li,J.‐G.Lou,W.Che, andM.‐Y.Kan,“Gl‐clef:Aglobal‐localcontrastive learningframeworkforcross‐lingualspokenlan‐guageunderstanding”.In: Proceedingsofthe60th AnnualMeetingoftheAssociationforComputationalLinguistics(Volume1:LongPapers),2022, 2677–2686.
[20] S.Schuster,S.Gupta,R.Shah,andM.Lewis, “Cross‐lingualtransferlearningformultilingual taskorienteddialog”.In: ProceedingsofNAACLHLT,2019,3795–3805.
[21] R.Sennrich,B.Haddow,andA.Birch,“Improving neuralmachinetranslationmodelswithmono‐lingualdata”.In: 54thAnnualMeetingofthe AssociationforComputationalLinguistics,2016, 86–96.
[22] M.Sowański.“iva_mt_wslot‐m2m100_418m‐en‐pl”,2023.HuggingFaceModelHub.
[23] M.Sowański.“iva_mt_wslot‐m2m100_418m‐en‐pl”,2023.HuggingFaceModelHub.
[24] M.SowańskiandA.Janicki,“Leyzer:Adataset formultilingualvirtualassistants”.In:P.Sojka, I.Kopeček,K.Pala,andA.Horák,eds., Proc.ConferenceonText,Speech,andDialogue(TSD2020), Brno,Czechia,2020,477–486.
[25] M.SowańskiandA.Janicki,“Optimizingmachine translationforvirtualassistants:Multi‐variant generationwithverbnetandconditionalbeam search”.In: 202318thConferenceonComputer ScienceandIntelligenceSystems(FedCSIS),2023, 1149–1154,10.15439/2023F8601.
[26] L.Sun,A.Korhonen,andY.Krymolowski,“Verb classdiscoveryfromrichsyntacticdata”, Lecture NotesinComputerScience,vol.4919,2008,16.
[27] D.R.Traum, Speechactsfordialogueagents, Springer,1999,169–201.
[28] A.Vaswani,N.Shazeer,N.Parmar,J.Uszkoreit, L.Jones,A.N.Gomez,Ł.Kaiser,andI.Polosukhin, “Attentionisallyouneed”, Advancesinneural informationprocessingsystems,vol.30,2017.
[29] W.Xu,B.Haider,andS.Mansour,“End‐to‐end slotalignmentandrecognitionforcross‐lingual NLU”.In: Proceedingsofthe2020Conferenceon EmpiricalMethodsinNaturalLanguageProcessing(EMNLP),2020,5052–5063.
Submitted:26th May2023;accepted:2nd February2024
ŁukaszSajewski,PrzemysławKarwowski
DOI:10.14313/JAMRIS/3‐2024/21
Abstract:
Thisarticlepresentsacomparisonofaclassicalapproach toidentificationofunstableobjectandanapproach basedonartificialneuralnetworks.Modelverification iscarriedoutbasedontheQuanserQube‐Servoobject withtheuseofmyRIOreal‐timecontrollerasthetarget. Itisshownthatmodelidentificationusingneuralnet‐worksgivesamoreaccuraterepresentationoftheobject. Inaddition,thehardware‐in‐the‐loop(HIL)techniqueis discussedandused,forimplementationofthecontrol algorithm.
Keywords: HIL,neuralnetworks,invertedpendulum
Identi icationinvolvesdeterminingthetemporal behaviorofasystemorprocessusingmeasuredsig‐nals,andthetemporalbehaviorisdeterminedwithin theclassesofmathematicalmodels.Themaingoal istoobtainthesmallestpossibleerrorbetween theactualprocessorsystemanditsmathemati‐calmodel[1].Modelingbyusingneuralnetworks, althoughmorecomplex,isoftenmoreaccurateand allowsustobettermapthedynamicsofthetested object.Oneofthebasicissuesinmodelingofareal objectisitsvalidation.ThisiswheretheHILtechnique comestotherescue.Hardware‐in‐the‐loop(HIL)sim‐ulationisatechniquefortestingembeddedsystemsat thesystemlevelinacomprehensiveandcost‐effective manner.HILismostoftenusedfordevelopmentand testingofembeddedsystems.Aprerequisiteforthe useofthistechniqueisthatthetestingcanbeaccu‐ratelyreproducibleintheoperatingenvironments. HILsimulationrequiresareal‐timesimulationthat modelstheindividualcomponentsoftheembedded systemundertest(SUT)andallrelevantinteractions withinagivenoperatingenvironment.Thesimulation monitorstheSUT’soutputsignalsandforcessyn‐theticallygeneratedinputsignalsintotheSUTatthe appropriatetime.TheSUT’soutputsignalsaretypi‐callyparameterssetontheactuatorandinformation displayedbytheoperator.InputsignalstotheSUTcan includedatareadfromsensorsandparametersset bytheoperator.Outputsfromtheembeddedsystem serveasinputstothesimulation,andthesimulation generatesoutputshatbecomeinputstotheembedded system[2].
Therotatingpendulumsystemisaclassicsystem. Itismostcommonlyusedforteachingmodelingand control.ThedesignationsusedtomodeltheQUBE‐ServorotarypendulumareshowninFigure1[3].
Therotaryarmattachedtothemotoraxisis denotedbythevariable ��,whilethependulum attachedtotheendofthepivotarmisdenotedbythe angle��
Notethefollowingrelationship:
‐ angle �� istheanglewithrespecttothevertical position.Mathematically,thisisdeterminedbythe formula:
��=����������������2��−��, (1) where ���������� istheangleofthependulumasmea‐suredbytheencoder;
‐ themovementofbothanglesispositiveifthemove‐mentiscounterclockwise(CCW)andapplyingapos‐itivevoltagetothemotorcausescounterclockwise rotation.
Therotatingaxisofthearmisconnectedtothe QUBE‐Servosystem.Thearmhaslength����,momentof inertia����.Theservoarmshouldrotatecounterclock‐wisewhenthecontrolvoltageispositive.
Thelinkofthependulumisconnectedtotheend oftherotatingarm.Itstotallengthis����,anditscenter ofmassisatthepoint��= ���� 2 .Themomentofinertia withrespecttothecenterofmassis����
Theangle��oftherotarypendulumtakesthevalue ofzerowhenitispointedverticallydownward.Coun‐terclockwisemotionresultsinincreasingvaluesofthe rotationangle.
Theequationsofmotion(EOM)forthependu‐lumsystemweredevelopedusingtheEuler‐Lagrange method.Thismethodismostoftenusedwhenmod‐elingcomplexsystems‐forexample,robotmanipula‐torswithmultiplejoints.Thisgivesthetotalkinetic andpotentialenergyofthesystemunderstudy.Then thederivativesarecalculatedto indtheequationsof motion.TheresultingnonlinearEOMsare[3]:
Figure2. QuanserQube‐Servoparameters[3]
InFigure2,wehaveQuanserQube‐Servoparame‐tersgivenbythemanufacturer.
where
3 isthemomentofinertiaoftherotary armwithrespecttothepivot(i.e.rotaryarmaxisof rotation)and
3 isthemomentofinertia ofthependulumlinkwithrespecttothependulum’s axisofrotation(i.e.,thependulum’saxisofrotation). Theviscousdampingactingonthepivotarmandthe pendulumlinkis ���� and ����,respectively.Thetorque generatedbytheservomotoratthebaseofthepivot armis
WhenthenonlinearEOMarelinearizedabout theoperatingpoint,theresultantlinearEOMforthe rotarypendulumarede inedas[3]:
UsingtheseparametersandEOMswecanwritea linearizedmodelofanobjectbythefollowingequa‐tions[6]:
Solvingfortheaccelerationtermsyields:
Sincewearedealingwithnonlinearunstabledynami‐calsystem,thepracticalidenti icationprocessismuch morecomplicated.
where ���� isequivalentviscousdampingcoef icient rotary((N*m*s)/rad),���� isequivalentviscousdamp‐ingcoef icientpendulum((N*m*s)/rad).
Byaddactuatordynamics
Connectiondiagram[3] ultimatelyweget
Figure4. MATLAB/SIMULINKrealtimetargetsettings
‐ MATLABCoderversion5.3.
‐ QuanserQUARC2021SP1
‐ NIMyRio‐1900:
‐ XilinxZ‐7010processorwith2cores,
‐ Processorspeedis667MHz.
Thepoolsarethefollowing
whichcon irmsthattheconsideredsystemisunsta‐ble.
NImyRIOreal‐timecontrollerhasbeenusedto managetheQuanserQube‐Servoobject.Thiscon‐trollerisaportablerecon igurableI/O(RIO)device thatcanbeusedtodesigncontrol,robotics,and mechatronicssystems[4].Encodersignalsanda motorcontrolsignalwereconnectedtothemyRIO. MATLAB/SIMULINKsoftwarehasbeenusedasthe developmentenvironmentalongwiththeQuanser QUARCadd‐on,givingthepossibilitytocontrolaplant withtheuseofreal‐timetarget(QUARCLinuxRT ARMv7Target).QUARCTM isthemostef icientway tocreatereal‐timeapplicationsonhardware.QUARC generatesreal‐timecodedirectlyfromdriverspro‐grammedwithSimulink.Itrunstheprogramonthe targetdeviceinrealtime[3].Thisapproachallows ustocompilethecodeusingaPCandthenrunit onareal‐timecontroller(myRIO),whichisconnected directlytotheplant.WhenRTTargetisstarted,thePC isusedonlyforthepresentationofprocessvariables. TheconnectiondiagramisshowninFigure3
Inthediscussedtask,thefollowinghardwareand softwarehavebeenused:
‐ KomputerPC
‐ Processor:Intel(R)Corei5‐126003,3GHz,
‐ RAM:32GB,
‐ System:MicrosoftWindows11Pro
‐ MATLABVersion:9.11.0.2022996(R2021b),
‐ SIMULINKversion10.4,
‐ SIMULINKCoderversion9.6,
Fullstatefeedbackmethodhasbeenusedtostabi‐lizethesystem,andtheLQRtechniquehasbeenused todeterminethematrix K stabilizingthesystem.
Newpoleslocationisthefollowing
Newpoleslocationhasbeentakenbasedontestson realplant.Figure5presentstheMATLAB/SIMULINK controlschema,whereQUARCHILblockshavebeen used.Havingthestabilizedsystemathand,wecan comparestepresponsesoftherealplantandthe modelcreatedbytheuseofEOMsandfactorydata. ThestepresponseofthesystemscanbeseeninFig‐ures6and7
Ascanbeseen,theresponseofthesimulatedsys‐tem(black)andtherealobject(blue)arenotequal, thisisduetoasmalldeviationtoonesideofpendulum androtor(amongotherfactors).
SincetheEOMsmodelisnotprecise,wewillpro‐ceedwiththeconstructionoftheneuralmodel.Todo this,itisnecessarytorecordtheresponseofthereal systemtoaspeciallypreparedinputsignal.Thenext stepistotrainthenetwork.Anonlinearautoregres‐siveneuralnetworkwithexternalinput(NARX)was selectedtotrainthenetwork.Thistypeofnetworkis usefultopredicttimeseriesdata.Thenetworkhad 2hiddenlayers,eachwith10neurons.Thenetwork had10backwardsamplesoftheforcingsignaland 10backwardsamplesofthefeedbacksignalasinput arguments.Thesignal,whichisusedtotrainthenet‐work,isshowninFigure8
4.ModelingResults
Figure 9 showstheschematicprogramthathas beenimplementedintothemyRIOcontroller.The forcingsignalfromthesignalgeneratorissenttoboth theQuanseQube‐Servoobjectandthemathematical andneuralmodel.Theresponsesarethenshownin theScopewindow.
Duringthemeasurements,itwasnecessarytover‐ifythelooptimeofthemyRIOcontroller.Initiallythis parameterhasbeensetto0.01[s].Figure10showsthe actuallooptimeduringthetest.
Figures 11 and 12 comparerotaryandpendu‐lumresponsesbetweenmeasurement,mathematical model,andNeural‐Networksmodel.InFigures13and 14wecanseetheerrorbetweentherealobjectsignals andthosegeneratedfrommodels.
Theperformanceassessmentbytheuseofinte‐gralabsoluteerror(IAE)criteriahavebeengivenin Table1
Table1. Integralabsoluteerrorcriterion
InthecaseoftheQuanserQubeServodevice,the pendulumencodercableactedlikeaspringpushing thearmaway,thusinterferingwiththemovements.In thestepresponse(Figs.6and7),itcanbeseenthatthe tiltineachdirectionisnotperfectlysymmetrical:this wasrelatedtothementionedcable.Fromtheerror signalsplot,itcanbeseenthataproperlytrainednet‐workgivesmuchmoreaccurateresultsthanamathe‐maticalmodel.Sincetheplantisnonlinear,itiscrucial tochoosetheappropriatetestingsignalforNeural Networktraining.
UsingtheHILtechnique,itisimportanttoremem‐berthelimitationsofthetargethardware(inthiscase, myRIO).Whenimplementingcontrolalgorithmsto targethardware,itisnecessarytocheckrealHILloop timesinceitcanin luencethequalityofthecontrol.
Havingagoodqualitymathematicalmodelopens upalotofpossibilitieswhendesigningalgorithmsto controlanobject.Forthemathematicallydetermined model,itisnecessarytoselecttheappropriatehard‐waregain,whichresultsfromtheuseofelectronic componentsintheobject—forexample,anencoderor amotor.
AUTHORS
ŁukaszSajewski∗ –FacultyofElectricalEngineering, BialystokUniversityofTechnology,Bialystok,15‐351, Poland,e‐mail:l.sajewski@pb.edu.pl.
PrzemysławKarwowski –FacultyofElectricalEngi‐neering,BialystokUniversityofTechnology,Bialystok, 15‐351,Poland,e‐mail:pkarw1@wp.pl.
∗Correspondingauthor
ACKNOWLEDGEMENTS
Thestudieshavebeencarriedoutintheframeworkof workNo.WZ/WE‐IA/5/2023and inancedfromthe fundsforsciencebythePolishMinistryofScienceand HigherEducation.
References
[1] R.Isermann,M.Münchhof, Identi ication ofDynamicSystems:AnIntroductionwith Applications,Springer‐VerlagBerlinHeidelberg 2011.10.1007/978‐3‐540‐78879‐9.
[2] J.Ledin,“SimulationEngineering:BuildBetter EmbeddedSystemsFaster”, CRCPress 2001.doi: 10.1201/9781482280722.
[3] J.Apkarian,M.Lévis,QuanserStudentWorkbook QUBE‐ServoExperimentforMATLAB/Simulink Users,Markham,Ontario,2014.
[4] NImyRIO‐1900UserGuideandSpeci ications, NationalInstruments,2018.
[5] L.Zadeh,“Probabilitymeasuresoffuzzy events”, JournalMath.AnalysisandAppl.,vol. 23,no.2,1968,421–427.doi:10.1016/0022‐247X(68)90078‐4.
[6] W.Rudin,“Principlesofmathematicalanalysis”, McGraw‐Hill:NewYork,1967,10–54.
[7] T.Gabor,S.Illium,M.Zorn,C.Lenta,A.Mattausch, L.Belzner,C.Linnhoff‐Popien,“Self‐Replicationin NeuralNetworks”, ArtifLife,vol.28,no.2,2022, 205–223.doi:10.1162/artl_a_00359.
ADVANCEDPERTURBANDOBSERVEALGORITHMFORMAXIMUMPOWERPOINT
ADVANCEDPERTURBANDOBSERVEALGORITHMFORMAXIMUMPOWERPOINT
TRACKINGINPHOTOVOLTAICSYSTEMSWITHADAPTIVESTEPSIZE
TRACKINGINPHOTOVOLTAICSYSTEMSWITHADAPTIVESTEPSIZE TRACKINGINPHOTOVOLTAICSYSTEMSWITHADAPTIVESTEPSIZE
Submitted:2nd April2023;accepted:1st August2023
DOI:10.14313/JAMRIS/3‐2024/22
Abstract:
Maximumpowerpointtracking(MPPT)algorithmsare commonlyusedinphotovoltaic(PV)systemstoopti‐mizethepoweroutputfromthesolarpanels.Among thevariousMPPTalgorithms,theperturbandobserve (P&O)algorithmisapopularchoiceduetoitssimplicity andeffectiveness.However,thebasicP&Oalgorithm hassomelimitations,suchasoscillationsandsteady‐stateerrorunderrapidlychangingirradianceconditions. Theenhancedalgorithmincludesamodifiedperturba‐tionstepandadynamicstepsizeadjustmentscheme. Thisreducestheoscillationsandimprovesthetracking accuracy.Inthedynamicstepsizeadjustmentscheme, thestepsizeisadjustedbasedontherateofchangeof thePVoutputpower.Thisimprovesthetrackingperfor‐manceunderrapidlychangingirradianceconditions.In ordertoprovetheperformanceofthedesignedcontrol algorithm,wewilltestitundersimpleclimaticconditions offixedtemperature(30∘C)andvariableirradiationin theformofsteps(500W/m2 and2000w/m2)andseethe systemresponse.TheperformanceoftheenhancedP&O algorithmhasbeenevaluatedusingMATLABsimulations.
Keywords: improvedtrackingaccuracy,dynamicstep sizeadjustment,reducedoscillations,maximumpower pointtracking,perturb&observealgorithm,photovoltaic systems
Theperturb&observe(P&O)implementationis widelyemployedfortrackingtherealizationofthe MPPTalgorithmforphotovoltaicsystems.Thereare manyresearchpapersandarticlespublishedonthe P&Oalgorithm,inboththeacademicandindus‐trialdomains.SomeworksrelatedtotheP&Oalgo‐rithmare:“EnhancedAdaptivePerturbandObserve TechniqueforEf icientMaximumPowerPointTrack‐ingUnderPartialShadingConditions”byMahmod MohammadANetal.(2020)[1],“Simulationand AnalysisofPerturbationandObservation‐BasedSelf‐AdaptableStepSizeMaximumPowerPointTracking StrategywithLowPowerLossforPhotovoltaics”by Zhuetal.(2019)[2],“Classi icationandComparisonof MaximumPowerPointTrackingTechniquesforPho‐tovoltaicSystem”byA.Reisietal.(2013)[3],
“AnEnhancedP&OMPPTAlgorithmforPVSys‐temswithFastDynamicandSteady‐StateResponse underRealIrradianceandTemperatureConditions” byAmbeHarrisonetal.(2022)[4],“AModi iedPer‐turbandObserveMethodwithanImprovedStepSize forMaximumPowerPointTrackingofPhotovoltaic Arrays”byMohammadMohammadinodoushanetal. (2021)[5].Theseresearchpaperspresentvarious modi icationsandimprovementstotheP&Oalgo‐rithmtoincreaseitsef iciencyandaccuracyintrack‐ingthemaximumpowerpointofaphotovoltaicsys‐tem.
Similarly,astudybyM.Ghaffarietal.(2018)[6] evaluatedtheperformanceofthefuzzylogic‐based P&Oalgorithmandfoundthatitwasabletotrack theMPPmoreaccuratelyandwithfeweroscillations comparedtothetraditionalP&Oalgorithm.Another studybyKatcheetal.(2023)[7]comparedtheper‐formanceofdifferentMPPTalgorithms,includingthe traditionalP&Oalgorithmanditsvariants,andcon‐cludedthattheadaptivestep‐sizeP&Oalgorithmwas themostef icientintermsoftrackingaccuracyand convergencespeed.Overall,theenhancedP&Oalgo‐rithmshaveshownpromisingresultsinimprovingthe trackingaccuracyandreducingoscillationsaround theMPP.However,theirimplementationmayrequire morecomplexhardwareandsoftwarecomparedto thesimulationofP&Oprogram.Therefore,thechoice ofMPPTprogramdependsonthespeci icapplication requirementsandconstraints.Thisarticlefocuseson thePerturbationsandObservations(P&O)algorithm fortrackingthemaximumpowerpointin luencedby thenonlinearcharacteristicsofthephotovoltaicpanel dependsonthevariableenvironmentalconditions, suchassolarradiationandambienttemperature[8].
TheenhancedPerturbandObserve(P&O)algo‐rithmforMaximumPowerPointTracking(MPPT) offerspracticaladvantagesintermsofimproved energyharvesting,enhancedsystemperformance, simplicityofimplementation,adaptabilitytovary‐ingclimaticconditions,andreducedmaintenance requirements.TheuseofMATLABsimulationsfor evaluationfurtheraddstothealgorithm’sfeasibility andpracticalityinreal‐worldapplications.However, it’simportanttonotethatreal‐worldimplementa‐tionmaystillrequireconsiderationsofhardwarecon‐straints,noise,andotherpracticalchallengesthatsim‐ulationsmaynotfullycapture.
Therestofthepaperisorganizedasfollows:after theintroduction,theproposedmethodforPVcell modelispresentedinSection 2,Section 3 provides anMPPTcommandapproach,simulationresultsare giveninSection4,and inallySection5offersconclu‐sionandperspective.
Aphotovoltaic(PV)cellisanelectronicdevicethat convertssunlightintousableelectricity.Itmadeupof severallayersofsemiconductormaterials,eachwitha differentelectricalproperty.
Theequivalentcircuitmodelofasolarcellisa toolthatmakesitpossibletorepresenttheelectrical behaviorofthephotovoltaiccell.Thismodelisbased ontheassociationofelectricalcomponents,which representtheelectricalcharacteristicsofthecell.The equivalentcircuitofasolarcellconsistsofacurrent sourceIph,aninternalseriesresistanceRs,anexternal loadresistanceRload,andadiodeinparallelwiththe currentsource,calleddiodephotovoltaic.
Theequivalentcircuitmodelisusedtodetermine theelectricalcharacteristicsofthesolarcell,suchas theopencircuitvoltage(Voc),theshortcircuitcurrent (Isc),themaximumpowerpoint(Pmax),andthecell conversionef iciency.Theseparametersareimpor‐tantforthedesignandoptimizationofsolarphoto‐voltaicsystemsFigure1.
TheexpressionofthePVsolarcellforcurrent voltage(I‐V)equation:
Where:
‐ Iisthecurrentgeneratedbythesolarcellinamperes (A)
‐ Iphisthephotocurrent,whichrepresentsthecur‐rentproducedbytheabsorptionofsunlight,in amperes(A)
‐ I0isthereversesaturationcurrentofthesolarcell intheabsenceoflight,inamperes(A)
‐ qisthechargeofanelectron,inCoulombs(C)
‐ Visthevoltageacrossthesolarcellinvolts(V)
‐ kisBoltzmann’sconstant,equalto1.38 × 10(‐23) J/K
‐ TisthetemperatureinKelvin(K)
Thephotocurrentofaphotovoltaic(PV)cellisthe electricalcurrentgeneratedbytheabsorptionoflight bythesemiconductormaterialinthecell
Where:
‐ Iph,refistheshort‐circuitcurrentofthesolarcell underreferenceconditions.
‐ ��scistheshort‐circuittemperaturecoef icientofthe solarcell.
Ontheotherhand,thereversesaturationcurrent ofthecellispresentedby
Where:
‐ I0,refistheoptimalshort‐circuitcurrentofthesolar cellunderreferenceconditions.
‐ ���� isthebandgapenergyinthesolarcell.
3.1.Perturbation&ObservationTechnique
ThePerturb&Observeimplementationisoneof themostcommonlyemployedalgorithmsfortrack‐ingofthemaximumpowerpoint(MPPT)command approach.TheperformanceoftheP&Oalgorithmcan beanalyzedintermsofaccuracy,ef iciency,andstabil‐ity.Thekeyfactorsthataffecttheperformanceofthe P&OalgorithminaPVsystemcanbesummarizedin thefollowingpoints:
‐ Steadystateaccuracy:TheP&Oalgorithmworksby perturbingtheoperatingpointofthePVarrayand observingtheresultingoutputpowertodetermine theMPP.TheaccuracyoftheP&Oprogramvariesin relationtotheproximityofdisturbancestotheMPP. Ifthedisturbancesaretoosmallortoolarge,the
Figure2. FlowchartofenhancedP&Oalgorithm
algorithmmayconvergetoanincorrectoperating point,resultinginreducedoutputpower.Therefore, thesizeandfrequencyofdisturbancesshouldbe optimizedtoachievehighsteady‐stateaccuracy.
‐ Dynamicresponse:TheP&Oalgorithmmust respondquicklytochangesinirradianceor temperatureofthePVpanelstotracktheMPP.If thealgorithmrespondstooslowly,itmaycausea reductioninoutputpower.Thedynamicresponse ofthealgorithmcanbeimprovedbyadjustingthe sizeandfrequencyofdisturbancesorbyusinga modi iedP&Oalgorithm,suchastheIncremental Conductancealgorithm.
‐ Oscillations:TheP&Oalgorithmisknowntoexhibit oscillationsaroundtheMPP,whichcancauseinsta‐bilityandreducedoutputpower.Theamplitudeand frequencyofoscillationscanbereducedbyopti‐mizingthesizeandfrequencyofdisturbancesor byusingamodi iedP&Oalgorithmthatincludesa dampingfactor.
‐ Environmentalfactors:ThequalityoftheP&O implementationisaffectedbysituations,suchas solarirradiance,temperature,andshading.Inlow lightconditions,theP&Oprogramcanbeableto accuratelydeterminetheMPP,resultinginreduced outputpower.Likewise,shadingcancausetheP&O programtoincreasetoaglobalmaximumrather thantheglobalMPP,thusreducingtheoutput power.
Table1. PVmoduleelectricalspecifications Parameters Value
MaximumpowerPmax(W) 21.02
Cellspermodule(Ncell)54
Maximumpointvoltage
Vpm(V)19.16
Ipm(A)1.05
Vco(V)23.81
Isc(A)1.08
MaximumpowerPmax(W) 21.02
Cellspermodule(Ncell)54
Maximumpointvoltage
Vpm(V)19.16
Ipm(A)1.05
Vco(V)23.81
Isc(A)1.08
Thephotovoltaicsystemstudiedcanbemodeled inMatlabasfollows:
Thecharacteristicsofthephotovoltaicmoduleare presentedinTable1.Thereafterthedifferentsimula‐tionparametersarepresentedinMATLAB/Simulink.
ThesimulationunderMATLAB/Simulinkisdone undertheparametersmentionedinTable1.Inorder toprovetheperformanceofthedesignedcontrolalgo‐rithm,wewilltestitundersimpleclimaticconditions of ixedtemperature(30∘C)andvariableirradiationin theformofsteps(500W/m2 and2000w/m2)andsee thesystemresponse.Thesimulationtimeis ixedat3s. Followingthevariationoftheirradiationfrom 800W/m2 to2000W/m2 (Fig. 4)whilemaintaining
Figure3. FlowchartofproposedsimulationofenhancedofP&Oalgorithm
Figure4. CurveofproposedsimulationofenhancedofP&Oalgorithm
Figure5. IllustrationEnhancedP&Oalgorithmwith ��Dvariation
the ixedtemperature(30∘C),weseethatthealgo‐rithmoffersagoodfollowupofthemaximumvoltage ofthepanelwithrespecttoitsreferencegivenbythe manufacturer,whichisequaltoVpm=19.25.
Thespeedandstabilityofoutputpowercanbe seenveryclearlyinFigure 5.Aresponsetimeof tr=50msismorethanenoughforthepowertoreach itsreferencevalue.
Theoutputofelectriccurrentperfectlyfollows theshapeoftheoutputpower.Themaximumoutput currentfor2000W/m2 illuminationreachestheman‐ufacturer’svalueasshowninFigure5
Theripplesoftheelectricalquantities,namelythe power,thevoltage,andtheoutputcurrent,remain largelyacceptablegiventhenatureofthephotovoltaic generatorandtheconditionsofuse.
Finally,andaccordingtothesedifferentresults, wecanseethattheeffectoftheP&Oprogramfora photovoltaicisimpressiveeitherintermsofspeedor intermsofmonitoringinstructionsinthesteadystate.
Inconclusion,MaximumPowerPointTracking (MPPT)algorithmsplayacrucialroleinphotovoltaic (PV)systemstooptimizethepoweroutputfromsolar panels.AmongthevariousMPPTalgorithms,theper‐turbandobserve(P&O)algorithmstandsoutasa popularchoiceduetoitssimplicityandeffectiveness. However,thebasicP&Oalgorithmhassomelimi‐tations,suchasoscillationsandsteady‐stateerrors, particularlyunderrapidlychangingirradiancecondi‐tions.
Toaddresstheselimitationsandimproveperfor‐mance,anenhancedP&Oalgorithmhasbeendevel‐oped.Thisenhancedalgorithmincorporatesamodi‐iedperturbationstepandadynamicstepsizeadjust‐mentscheme.Asaresult,thealgorithmachievesa morestableandaccuratetrackingofthemaximum powerpoint,leadingtoimprovedenergyharvesting andenhancedsystemperformance.
Thedynamicstepsizeadjustment,basedonthe rateofchangeofthePVoutputpower,enablesthe algorithmtoadapttovaryingirradiationlevelsef i‐ciently.Thisadaptabilitymakesitsuitableforreal‐worldconditionswheresolarirradiancecanchange rapidly.
Tovalidatetheperformanceofthedesignedcon‐trolalgorithm,testswereconductedundersim‐pleclimaticconditionswitha ixedtemperatureof 30∘Candvariableirradiationintheformofsteps (500W/m2 and2000W/m2).MATLABsimulations wereemployedforevaluation,providingacost‐effectiveandef icientmeansofanalyzingthealgo‐rithm’sbehaviorunderdifferentscenarios.
Thepracticaladvantagesoftheenhanced P&Oalgorithmincludeimprovedenergy harvesting,enhancedsystemperformance,simple implementation,adaptabilitytovaryingclimatic conditions,andreducedmaintenancerequirements.
Overall,theenhancedP&Oalgorithmdemon‐stratesitspotentialtooptimizethepoweroutput ofphotovoltaicsystems,makingitavaluablechoice forpracticalapplicationsintherenewableenergy domain.However,furthervalidationthroughphysical testingandconsiderationsofreal‐worldconstraints arenecessarytoensureitssuccessfulimplementation inoperationalsolarenergysystems.
AUTHOR
AmalZouhri∗ –SidiMohammedBenAbdellah University,FacultyofSciencesDharElMahraz, LISACLaboratory,Fez,Morocco,e‐mail: amal.zouhri@usmba.ac.ma.
∗Correspondingauthor
References
[1] MahmodMohammadAN,MohdRadziMA,AzisN, Sha ieS,AtiqiMohdZainuriMA,“AnEnhanced AdaptivePerturbandObserveTechniqueforEf i‐cientMaximumPowerPointTrackingUnderPar‐tialShadingConditions,” AppliedSciences.2020; 10(11):3912.DOI:10.3390/app10113912.
[2] ZhuY,KimMK,WenH,“SimulationandAnalysis ofPerturbationandObservation‐BasedSelf‐AdaptableStepSizeMaximumPowerPoint TrackingStrategywithLowPowerLossfor Photovoltaics,” Energies.2019;12(1):92.DOI: 10.3390/en120100920.
[3] R.Reisi,M.H.Moradi,andS.Jamasb, “Classi icationandcomparisonofmaximum powerpointtrackingtechniquesforphotovoltaic system:Areview,” RenewableandSustainable EnergyReviews,vol.19,pp.433–447,2013.DOI: 10.1016/j.rser.2012.11.052.
[4] AmbeHarrison,EustaceMbakaNfah,Jeande DieuNguimfackNdongmo,NjimbohHenryAlom‐bah,“AnEnhancedP&OMPPTAlgorithmfor PVSystemswithFastDynamicandSteady‐State ResponseunderRealIrradianceandTemperature Conditions”, InternationalJournalofPhotoenergy, vol.2022,ArticleID6009632,21pages,2022. DOI:10.1155/2022/6009632.
[5] MohammadMohammadinodoushan,Rabeh Abbassi,HoussemJerbi,FaraedoonWalyAhmed, HalkawtAbdalqadirkhahmed,AlirezaRezvani, “AnewMPPTdesignusingvariablestepsize perturbandobservemethodforPVsystem underpartiallyshadedconditionsbymodi ied shuf ledfrogleapingalgorithm–SMCcontroller,” SustainableEnergyTechnologiesandAssessments, Volume45,2021,101056,ISSN2213‐1388,DOI: 10.1016/j.seta.2021.101056.
[6] Rezoug,M.R.,Chenni,R.,Taibi,D.,“Fuzzy Logic‐BasedPerturbandObserveAlgorithm withVariableStepofaReferenceVoltage forSolarPermanentMagnetSynchronous MotorDriveSystemFedbyDirect‐Connected
PhotovoltaicArray,” Energies 2018,11,462.DOI: 10.3390/en11020462.
[7] Katche,M.L.,Makokha,A.B.,Zachary,S.O., Adaramola,M.S.,“AComprehensiveReview ofMaximumPowerPointTracking(MPPT) TechniquesUsedinSolarPVSystems,” Energies 2023,16,2206.DOI:10.3390/en16052206.
[8] A.Harrison,E.M.Nfah,J.d.D.N.Ndongmo,andN. H.Alombah,“AnEnhancedP&OMPPTAlgorithm forPVSystemswithFastDynamicandSteady‐StateResponseunderRealIrradianceandTem‐peratureConditions,” InternationalJournalofPhotoenergy,Volume2022,ArticleID6009632,21 pages,DOI:10.1155/2022/6009632.
Abstract:
EEGBASEDEMOTIONANALYSISUSINGREINFORCEDSPATIO‐TEMPORALATTENTIVE GRAPHNEURALANDCONTEXTNETTECHNIQUES
EEGBASEDEMOTIONANALYSISUSINGREINFORCEDSPATIO‐TEMPORALATTENTIVE
EEGBASEDEMOTIONANALYSISUSINGREINFORCEDSPATIO‐TEMPORALATTENTIVE GRAPHNEURALANDCONTEXTNETTECHNIQUES GRAPHNEURALANDCONTEXTNETTECHNIQUES
Submitted:21st October2022;accepted:6th February2023
C.AkalyaDevi,D.KarthikaRenuka
DOI:10.14313/JAMRIS/3‐2024/23
EEG‐basedemotionclassificationisconsideredtosep‐arateandobservethementalstateoremotions.Emo‐tionclassificationusingEEGisusedformedical,security andotherpurposes.Severaldeeplearningandmachine learningstrategiesareemployedtoclassifytheEEG emotionsignals.Theydonotprovidesufficientaccu‐racyandhavehighercomplexityandhigherrorrate. Inthismanuscript,anovelReinforcedSpatio‐Temporal AttentiveGraphNeuralNetworks(RSTAGNN)andCon‐textNetforemotionclassificationwithEEGsignalsis proposed(RSTAGNN‐ContextNet‐GWOA‐EEG‐EA).Here, theinputEEGsignalsaretakenfromtwobenchmark datasets,namelyDEAPandK‐EmoCondatasets.Then, theinputEEGsignalsarepre‐processed,andthefea‐turesareextractedutilizingContextNetwithGlobalPrin‐cipalComponentAnalysis(GPCA).Afterthat,theEEG signalemotionsareclassifiedusingReinforcedSpatio‐TemporalAttentiveGraphNeuralNetworksmethod. RSTAGNNweightparametersareoptimizedunderthe GlowwormSwarmOptimizationAlgorithm(GWOA).The proposedmodelclassifiestheEEGsignalemotionswith highaccuracy.Theefficacyoftheproposedmethodusing theDEAPdatasetattainshigheraccuracyby24.05%, 12.64%relatedtoexistingsystems,likeMulti‐domain featurefusionforemotionclassification(DWT‐SVM‐EEG‐EA‐DEAP),EEGemotionfindingutilizingfusionmode ofgraphCNNwithLSTM(GCNN‐LSTM‐EEG‐EA‐DEAP) respectively.Theefficiencyoftheproposedmethodusing theK‐EmoCondatasetattainshigheraccuracy32.64%, 15.65%relatedtoexistingsystems,likeTowardRobust WearableEmotionRealizationalongContrastiveRepre‐sentationLearning(CAT‐EEG‐EA‐K‐EmoCon)andHuman EmotionRecognitionusingPhysiologicalSignals(CAT‐EEG‐EA‐K‐EmoCon)respectively.
Keywords: emotionrecognition,electroencephalogram (EEG),reinforcedspatio‐temporalattentivegraphneu‐ralnetworks(RSTAGNN),glowwormswarmoptimization algorithm(GWOA)
Emotionshaveasigni icantroleinhuman decision‐making,interaction,andcognitive processes[1].Astechnologyandknowledgeof emotionsadvance,therearemoreprospectsfor autonomousemotionidenti icationsystems[2].
Therehavebeensuccessfulscienti icadvances inemotionidenti icationutilizingtext,audio,facial expressions,orgesturesasstimuli[3].However, oneofthenewandintriguingroutesthisresearch istakingistheuseofEEG‐basedtechnologyfor automaticemotionidenti ication,whichisbecom‐inglessinvasiveandmoreeconomical,leadingto widespreadusageinhealthcareapplications[4].The emotionsofapersoncanbeidenti iedusingphysio‐logicalsignalsornon‐physiologicalsignalslikevideo andaudio.Betweenthese,thephysiologicsignals suchasEEG(Electroencephalogram),ECG(Electro‐cardiogram),SC(SkinConductance),andElectromyo‐gram(EMG)accuratelyde inetheemotionofhumans relatedtotheothercounterparts,butitdoesnotpro‐videenoughresultsofclassi icationofemotions[5]. ThisreasonliesinthefactthatEEGsignalsaremea‐sureddirectlyatthesurfaceofthebrain,represent‐ingtheactualhumancondition.EEG‐basedemotion analysisisusefulforpatientssufferingfromstroke, seizurediagnosis,autism,attentionde icit,andmental retardation[6].Severaldeeplearningandmachine learningmethodsareusedtocategorizetheEEGemo‐tionsignalsfromtheinputdataset,butthosemethods donotprovidesuf icientaccuracy,andthecomplexity anderrorratewerehigh[10–14].Thegoalofthis paperistoovercometheseissues.
Themaincontributionsofthismanuscriptare summarizedbelow:
‐ AnovelRSTAGNNandContextNetforemotionclas‐si icationwithEEGsignalsisproposed(RSTAGNN‐ContextNet‐GWOA‐EEG‐EA).
‐ TheinputEEGsignalsaretakenfromtwobench‐markdatasetssuchasDEAP[14]andK‐EmoCon dataset[15].
‐ TheinputEEGsignalsarepre‐processed,andfea‐tureextractionisdoneusingContextNetwithGlobal PrincipalComponentAnalysis(GPCA)[7].
‐ Afterthat,theEEGsignalemotionsareclassi‐iedusingtheReinforcedSpatio‐TemporalAttentive GraphNeuralNetworks(RSTAGNN)[8]method.
‐ RSTAGNNweightparametersareoptimizedusing GWOA[9].Finally,themodelclassi iestheEEGsig‐nalemotionswithhighaccuracy.
‐ TheproposedtechniqueisexecutedintheMATLAB. Themetrics,likeaccuracy,precision,recall,andf‐score,areevaluated.
‐ Then,theef iciencyofRSTAGNN‐ContextNet‐GWOA‐EEG‐EAmethodusingDEAPdataset isevaluatedwithexistingDWT‐SVM‐EEG‐EA‐DEAP[10],GCNN‐LSTM‐EEG‐EA‐DEAP[11]andthe performanceofK‐EmoCondatasetiscomparedwith existingsystems,likeCAT‐EEG‐EA‐K‐EmoCon[12] andCAT‐EEG‐EA‐K‐EmoCon[13]respectively. Theremainingmanuscriptisspeci iedasfol‐lows:section2divulgesrelatedworks,theproposed methodologyisillustratedinSection 3,theresults anddiscussionareexempli iedinSection 4,andthe conclusionofthemanuscriptisgiveninSection5
AmongvariousresearchworksonEEGbasedEmo‐tionanalysisusingDEAPandK‐EmoCondataset,afew recentinvestigationsareassessedhere, Khateebetal.[10]presentedmultipledomain featurefusionforemotioncharacterizationutiliz‐ingtheDEAPdataset(DWT‐SVM‐EEG‐EA‐DEAP).The imagerieswerepre‐processedtotransferdataas wellasreducedatadimensionality.Afterthat,multi‐domainfeatureswereextractedtoidentifystablefea‐turestoclassifytheEEGemotionsignals.Then,these signalswereclassi iedusingsupportvectormachine classi ier.But,thecomplexitywashigh.
Yinetal.[11]presentedmultipledomainfeature fusionforemotioncategorizationunderDEAPdataset (GCNN‐LSTM‐EEG‐EA‐DEAP).Initially,theinputdata wascalibratedusing3sbaselinedatathatweresplit into6ssegmentsusingatimewindow;afterthat,the differentialentropywasextractedfromeverysegment forconstructingthefeaturecube.Then,thesefeature cubeswerefusedwithgraphconvolutionalneuralnet‐worksincludinglong‐orshort‐termmemoriesneural networksforclassifyingEEGsignalemotionaldata.
Dissanayakeetal.[12]presentedToward RobustWearableEmotionIdenti icationincluding ContrastiveRepresentationLearning(SigRep‐EEG‐EA‐K‐EmoCon).TheinputEEGemotionsignals weretakenfromtheK‐EmoCondataset.Then,these signalswerepre‐processedtolowerthesignal resampling.Afterthat,thestatisticalfeatureswere extracted.Thoseextractedfeatureswereusedinthe self‐supervisedtechniquetoclassifytheEEGemotion signalwithhighaccuracy.Butthecomplexitywas greater.
Yangetal.[13]presentedMobileEmotionIden‐ti icationutilizingMultiPhysiologicalSignalswith Convolution‐augmentedTransformer(CAT‐EEG‐EA‐K‐EmoCon).TheinputEEGemotionsignalswere takenfromtheK‐EmoCondataset.Particularly,ituses arousalandvalencedimensions,learningconnections, andrelianceacrossseveralmodalphysiologicaldata toidentifytheusers’emotions..Thismethodprovides betteraccuracybuttheerrorratewashigh.
3.ProposedMethodology
Inthissection,anovelRSTAGNNandContextNet foremotionclassi icationusingEEGsignalsis explained.Figure 1 depictstheblockdiagramofthe proposedsystem.
3.1.DataAcquisition
TheinputdatasetsaretakenfromtheDEAPand K‐EmoCondatasets.TheDEAPdatasetismadeupof physiologicalrecordingsfrom32peoplewhoviewed 40one‐minute‐longmusicvideos.K‐EmoConisamul‐tiplemodaldatasetthatinvolvesadetailedexplana‐tionofongoingemotionsexperiencedthroughnatu‐ralisticconversations.Thedatasethasmultiplemodal measurementstakenwithcommercialdevicesduring 16sessionsofpartnerdiscussionsofabout10minutes durationonasocialtopic,includingvideorecordings, EEG,andperipheralphysiologicalcues.Thesetwo datasetsarethenpre‐processed,andfeaturesare extractedwithContextNetwithGPCA.
3.2.Pre‐processingandFeatureExtractionUsingCon‐textNetwithGlobalPrincipleComponentAnalysis (GPCA)
Inthis,pre‐processingisdonefortwodatasets, suchastheDEAPdatasetandtheK‐EmoCon.Thedata setsarecapturedwithseveraldevicesalongdissimilar samplingrates.Tomergethesignalfrequency, irst splitthecontinualsignalsintofour‐secondwindow sizesthroughaone‐secondoverlap.Thedatatransfor‐mationanddatareductionprocessisused.
Here,thepre‐processingisdoneforreducingthe individualdifferencesofthedatasetforvaryingage, gender,andpersonality.Thepre‐processingisdone usingtheconvolutionlayerofthemulti‐tasklearning ContextNetbydatatransformationanddatareduc‐tion.
Here,thedatatransformationsareusedtoreduce theEEGdatavaluesfrombothdatasetsduringthe trainingprocess;otherwise,thismayaffecttheperfor‐manceoftheclassi ication.Then,thedatatransforma‐tionoftheinputdatasetusingtheconvolutionlayerof themultitasklearningContextNetanditsequationis giveninEquation(1)–(2) ������������������(��������������������������)(ℎ)[���� �� ;����] =ℎ��(���� �� ;���� �� ) (1) where
whereℎ�� isrepresentedasthecontextawarefunction withdatatransformationparametersare ���� �� , ℎ��, ℎ��, thespeci iedtaskwithcontextrepresentationofdata isrepresentedasthe���� �� ,���� �� ,andtheparticulartask withcontextfortransformingthedataisgivenwith task��,ℎisthenumberofinputEEGemotionalsignals fromthedatasetwith����ℎcontext.Then,thedatareduc‐tionprocesstakesplaceaftertransformingthedata usingequation(2)andthedatareductionequationis givenin(3).
�� =ℎ��(����;����) (3)
BrainWavedataalsocontainssomeduplicateentries andareremoved.The inal2‐dimensionalvectoristhe pre‐processedinputforConvolutionlayer.Then,the inalpre‐processedequationisgiveninEquation(4).
Itemploys100initialconvolution iltersanda three‐row,one‐columnconvolutionalkernel.Between eachconvolutionallayer,dropoutisused.MaxPool‐ingLayeristhefollowinglayer.Over3x3blocks, thispoolingisatypical2‐dimensionalmaxpooling. ToobtainCNNaccuracy,themaximumpooledout‐putis lattenedandappliedwithsoftplusactivation. UsingGPCAwiththeContextNetmethod,statistical featuressuchasmeanandvarianceareextracted,and timedomainfeatures,suchasHjorthparametersand entropyfeatures,areextractedasEEGsignals.Here, Hjorthparametersareactivity ����,mobility ����,com‐plexity����,where��isrepresentedasthefeaturesand itsformulasaregiveninequations(6)–(8)
Equation(4)isknownasthe inalpre‐processedequa‐tion,andthedatadimensionsarereducedtoimprove theclassi icationprocess.Then,thepre‐processing signalsaregiventoGPCAandtheReLulayerfor extractingstatisticalfeatures,domainfeatures,and thefrequencyfeaturesfrominputEEGsignaldatasets. GPCAcreatesalow‐dimensionaldatarepresentation thatcapturesasmuchofthedata’sdiversityaspossi‐ble.GPCAisappliedwiththenumberoffeaturesset as32.So,thetwodatasetshapesafterpreprocessing are32participantsx40trailsx32channelsx32 data.Here,thedataisnormalizedforeliminatingthe dimension,andthenormalizedglobaldataisgivento thefeatureextractionprocessusingGPCA,andisgiven inEquation(5),
(5) where
isrepresentedasthenormalized datafromthepre‐processedusedtoextractthefea‐tures,and ���� isrepresentedastheglobaldataofthe GPCA.
Where ���� implicatesinputEEGsignal, ����������������(��′ ��) implicatesvarianceofinitialderivativeofinputsignal, ����������������(����) isrepresentedassignalvariance,and ��′ �� isrepresentedasmobilityofinitialderivativeof inputEEGsignal(��
)
Afterthat,theentropyfeatureisextractedbysplit‐tingEEGsignalsinto10equalpartswithnooverlap‐ping,anditsequationisgivenin(9)
(9)
where��1 isrepresentedasthe1/10��ℎ ofthetotalEEG signals(��),ℎreferscountoffeatures.
FrequencydomainfeaturesoftheEEGsignalfea‐turesareextractedusingthenon‐stationaryandnon‐linearanditssubbandsarerepresentedasthealpha subbands(8–15Hz),beta(16–32Hz),andgammasub bands(>32Hz),thenthepowerratesareestimated usingthesesub‐bandsisgiveninEquation(10),
where���� isrepresentedasfrequencydomain,power ratesaredesignedtoalpha,beta,andgammasub‐bands.Theseextractingfeaturesaregiventothe RSTAGNNtocategorizeEEGsignalemotionsbasedon arousal,valance,anddominance.
3.3.EEGSignalEmotionsClassificationUsingReinforced Spatio‐TemporalAttentiveGraphNeuralNetworks (RSTAGNN)
RSTAGNNisusedtoclassifyEEGsignalemotions, suchasarousal,valance,anddominance.Itconsistsof threeparts:diffusionconvolutionondirectedgraph, spatial‐temporalencoder,andmulti‐stepprediction decoder.Inthis,thefeature‐extractedEEGsignalsare giventotheinputofdiffusionconvolutionondirected graph.Itisan��‐orderdirectedgraphconvolutionnet‐work,anditsequationisgiveninEquation(11)
whereℎ�� isrepresentedastheconvolution ilterwith featureextractedEEGsignals, ∗ referstodiffusion convolution,��referscountofdiffusionsteps,����,1 and ����,2 ∈ℑ�� representstrainableparametersofthetwo graphdirections,���� =����������������(��)isrepresentedas theout‐degreediagonalmatrix, ���� =����������������(����) isrepresentedasin‐degreediagonalmatrixandits complexityisgiveninEquation(12).
��(��)=��(��|��|)≪��(��2) (12)
where �� isrepresentedastheweightparameterfor representingcomplexity.
ThespatialattentionweightsoftheEEGsig‐nalsarerepresentedusingtheSpatio‐temporalTraf‐icEncoder,andtheirequationisprovidedinEqua‐tion(13) ���� �� = exp(���� ��)
�� ��=1 exp(���� ��) (13)
where�� referstothetimestepwith����ℎ and����ℎ EEG signals, �� referstonumberofsamples, �� refersto theweightparameterforrepresentingaccurateness oftheEEGsignalemotionclassi ication.ThentheEEG emotionsignalsareclassi iedusingtheMulti‐stepPre‐dictionDecoder,anditsequationisgivenin(17)with theattentionweights ��′ ��,�� foreveryhiddenstate, namelysoftfunctionsarenormalizedto[0,1],andits equationisgivenin(14)
′ ��,��=�������� max(��′ ��,��)= exp(��′ ��,��) ∑�� ��=1 exp(��′ ��,��) (14)
where �� isrepresentedastheweightparameterfor representingtheerrorrateoftheEEGsignalemotion classi ication.
Toenhancetheclassi icationaccuracyof RSTAGNN,GWOAisusedforoptimizingtheproposed model.Heretheweightparametersare��,��,��,where ��isrepresentedasthecomplexity,��isrepresentedas theaccuracy,��isrepresentedastheerrorrate,these parametersareoptimizedusingGWOAbyminimizing ��,��andmaximizing��
Figure2. FlowchartforGWOAtooptimizeRSTAGNN
3.4.StepwiseProcessofGWOAforOptimizing RSTAGNN
GWOAoptimizestheparametersofRSTAGNN. Theseparametersareoptimizedforassuringaccu‐rateclassi icationoftheEEGemotionsignals.GWOis de inedasswarmcognizance.Figure 2 portraysthe lowchartfortheGWOAforoptimizingRSTAGNN.The stepwiseprocessingofGWOAisdelineatedbelow,
Step1: Initialization
Initially,allglowwormshaveapproximatelyequal levelsofluciferindependingonthelesserandupper boundsofglowwormsproductionpowerandcontrol parameters.Theinitialpopulationofglowwormis representedas��.
Step2: RandomGeneration
Afterwardtheinitializationprocedure,theinput parametersarecreatedrandomly.Themaximal it‐nessvaluesaredesignatedwithrespecttotheexact classi icationoftheEEGemotionsignals.
Step3: FitnessFunction
Itisexaminedtoattaintheobjectivefunction, whichisanexactclassi icationoftheEEGemotion signalswithoptimumvalue.RSTAGNNweightparam‐etersareselectedas��,��,��,where��isrepresentedas thecomplexity,�� isrepresentedastheaccuracy,�� is representedastheerrorrate,andtheseparameters areoptimizedusingGWOAbyminimizing ��, �� and maximizing ��.The itnessfunctionisarticulatedin Equation(15),
Step4: Updateluciferinvaluetoincreaseaccu‐racy��
InGWOA,everyglowwormupdatesitslocation throughapre‐determinedamountoftrials.Theglow‐worm’spositionupdateisexhibitedinEquation(16),
(16)
here �� refersrandomcountofnormaldistribution at[0,3],������������referstangentsigmoidoperations,�� referstothecurrentiterationcount,��max referstothe maximalnumberofiterations,and ���� isrepresented astheoptimizingparameterforincreasingaccuracy.
Step6: Updateluciferinvolumeforreducingcom‐plexity��.
Here,luciferinvolumeisusedtoreducethecom‐plexityofthesystemwhileclassifyingtheEEGemo‐tionsignals.Explorationofglowwormforidealsolu‐tionsisdeterminedusingEquation(17),
(17)
Let �� implyarandomlychosenlocation, �� forglow‐worm, �� forreducingcomputationalcomplexityto classifyEEGemotionalsignal,and������ �� representsthe newsource.
Step7: Performmutationoperationtominimize errorrate��
Themutationprocessactsunderprobabilityval‐uesonthebasisof itnessvaluespresentedbyglow‐worm.Forthispurpose,a itnessbaseselectionstrat‐egyisemployed.ThisisarticulatedinEquation(18),
4.ResultsandDiscussion
Inthissection,anovelRSTAGNNandContextNet foremotionclassi icationwithEEGsignalsisdis‐cussed.TheexperimentsareconductedusingMAT‐LABontheGPUworkstationwithanIntelXeonCPU@ 3.20GHzand32.0GBRAM.Theperformancemetrics, likeprecision,Accuracy,f‐score,recallareexaminedto authenticatetheeffectivenessoftheproposedsystem. TheperformanceoftheproposedsystemusingDEAP datasetisanalyzedtotheexistingsystems,likeDWT‐SVM‐EEG‐EA‐DEAP[10],GCNN‐LSTM‐EEG‐EA‐DEAP [11]respectively,andtheperformanceofK‐EmoCon datasetiscomparedwithexistingsystemlikeCAT‐EEG‐EA‐K‐EmoCon[12]andCAT‐EEG‐EA‐K‐EmoCon [13]respectively.
4.1.DatasetDescription
ExperimentsareconductedusingDEAPandK‐EmoCondatasets.Ofthetotaldataset,80%wasused fortrainingand20%fortesting.
4.2.PerformanceMetrics
Theevaluationparameters,suchastheaccuracy, precision,recall,f‐scorefordetectingemotionfrom inputEEGsignals,areanalyzed,andtheperformance equationisgivenin(19).
where,thetrainingdataofRSTAGNNforclassify‐ingEEGemotionsignalswithhighaccuracydenotes ��(��),��impliescurrentiterationcount,��max denotes ideallocation,��refersmaximalcountofiterations,�� refersroundforminimizingerrorrate.
Step8: Termination.
Theoptimumweight‐parameters��,��,��arechosen atRSTAGNNunderGWOAiterativerepeatstep3until ful illingthehaltingcriterion ��=��+1.Attheend, RSTAGNNclassi iesEEGemotionaccuratelybydimin‐ishingtheerrorandcomplexityutilizingGWOA.
Inthismanuscript,anovelRSTAGNNandContext Netforemotionclassi icationusingEEGsignals iseffectivelyexecuted.TheRSTAGNN‐ContextNet‐GWOA‐EEG‐EAmethodisexecutedinMATLAB environment.Theoutputoftheproposedmethod usingDEAPdatasetattainshigherprecisionby 32.99%,46.64%estimatedtotheexistingsystems, likeDWT‐SVM‐EEG‐EA‐DEAPandGCNN‐LSTM‐EEG‐EA‐DEAPandtheperformanceoftheproposedsystem usingK‐EmoCondatasetattainshigherprecision 15.75%,31.86%relatedtoexistingsystems,like SigRep‐EEG‐EA‐K‐EmoConandCAT‐EEG‐EA‐K‐EmoConrespectively.
here (����) indicatesTruePositive, (����) refersTrue Negative, (����) representsFalsePositive, (����) indi‐catesFalseNegative.
4.3.ComparisonofPerformanceAnalysiswithvarious methodsusedforEEGEmotionAnalysis
Thebelowsectionportrayscomparisontablesof theproposedmethodcomparedwiththeexisting method.
Table 1 showstheperformanceanalysisofthe EEGemotionusingtheDEAPdatabase.Theaccu‐racyanalysisoftheproposedmethodshows34.94%, 28.94%higherValenceaccuracy,23.95%,28.94%, higherArousalaccuracy,and28.94%,27.84%,higher Dominanceaccuracy.Theprecisionanalysisofthe proposedmethodshows34.94%,28.94%,higher Valenceprecision,23.95%,28.94%,higherArousal precision,and28.94%,27.84%,higherDominance precision.Therecallanalysisoftheproposedmethod shows34.94%,28.94%higherValencerecall,23.95%, 28.94%,higherArousalrecall,and28.94%,27.84%, higherDominancerecall.
Table1. PerformancemetricsofEEGemotionAnalysisusingtheDEAPdataset
Performancemetrics
Table2. PerformancemetricsofEEGemotionAnalysisusingK‐EmoCondataset Performancemetrics
TheF‐scoreanalysisoftheproposedmethod shows34.94%,28.94%,higherValenceF‐score, 23.95%,28.94%,higherArousalF‐score,28.94%, 27.84%,higherDominanceF‐scorerelatedtothe existingsystemlikeDWT‐SVM‐EEG‐EA‐DEAPand GCNN‐LSTM‐EEG‐EA‐DEAPrespectively.
Table 2 showstheperformanceanalysisofthe performancemetricsofEEGemotionnalysisutilizing K‐EmoCondataset.Theaccuracyanalysisofthepro‐posedmethodattains32.75%,35.75%higherValence accuracyand25.75%,26.86%higherArousalaccu‐racy.Theprecisionanalysisoftheproposedmethod shows32.86%,26.86%higherValenceprecision, 31.86%,26.86%higherArousalprecision.Therecall analysisshows32.86%,44.75%higherValencerecall, 25.75%,25.87%higherArousalrecall.TheF‐score analysisshows25.86%,31.75%higherValenceF‐score,25.86%,33.86%,higherArousalF‐scorerelated totheexistingsystemlikeSigRep‐EEG‐EA‐K‐EmoCon andCAT‐EEG‐EA‐K‐EmoConrespectively.
Emotionsarecrucialfordecision‐making,plan‐ning,reasoning,andotheraspectsofhumanmental‐ity.Fore‐healthcaresystems,itisincreasinglyimpor‐tanttorecognizetheseemotions.Theuseofbiosen‐sorsliketheElectroencephalogram(EEG)toidentify patients’mentalstateswhomayrequireparticular careprovidescrucialfeedbackforambientassisted
living(AAL).Thisstudyexploredthepurposeofdeep learningclassi icationforEEG‐basedemotionanaly‐sisandevaluateditsperformanceonDEAPandK‐EmoCondatasets.Therateofemotionrecognition con irmsthatthereissuf icientinformationinthe EEGdatatodistinguishbetweenvariousemotional states.Notably,thesuggested indingssupportthe feasibilityofusingfewerelectrodestotrainclas‐si iersforreal‐timeHCIapplications.Theaccuracy betweenotherkindsoffeaturesissomewhatdif‐ferent,thentheoutcomesshowthatstatisticalfea‐turesareappropriateforemotionrecognition.Per‐formanceislikelytoimprovewhentrainingincorpo‐ratesmoredataorbetter‐quality,higher‐resolution videosareveri ied.Comparedtoasinglemodelusing thesameinputvideosize,abiggerone,theRein‐forcedSpatio‐TemporalAttentiveGraphNeuralNet‐worksperformedbetteroverallandsavedasignif‐icantamountoftimeregardingtrainingandinfer‐ence.ItenableEEGsignalemotionsclassi ication usingvideorecordings,EEG,andperipheralphysio‐logicalcues,alsoscienti icallyinterestingalongclin‐icallyimpactful.Simulationoutcomesshowthatthe RSTAGNN‐ContextNet‐GWOA‐EEG‐EAprovidehigher accuracyof38.58%,and43.87%,higherF‐scoreof 23.64%,31.91%,higherprecisionof32.67%,and 45.39%,higherrecallof34.09%and45.51%for DEAPdatasetcomparedwithexistingmethods,like
DWT‐SVM‐EEG‐EA‐DEAPandGCNN‐LSTM‐EEG‐EA‐DEAPrespectively.FortheK‐EmoCondataset,thepro‐posedRSTAGNN‐ContextNet‐GWOA‐EEG‐EAmethod provideshigheraccuracyof58.31%and56.34% higherF‐Measureof45.56%and23.31%higherpre‐cisionof25.69%,54.39%,higherrecallof45.17% and21.33%comparedwithexistingmethodslike CAT‐EEG‐EA‐K‐EmoConandCAT‐EEG‐EA‐K‐EmoCon respectively.
5.Conclusion
Inthismanuscript,RSTAGNNandContextNetfor emotionclassi icationusingEEGsignalsiseffectively executed.TheRSTAGNN‐ContextNet‐GWOA‐EEG‐EA methodisactivatedinMATLABenvironment.The ef icacyoftheproposedmethodusingDEAPdataset attainshigherprecision32.99%,46.64%compared withtheexistingsystems,likeDWT‐SVM‐EEG‐EA‐DEAPandGCNN‐LSTM‐EEG‐EA‐DEAP[11]respec‐tively.Theperformanceoftheproposedmethodusing K‐EmoCondatasetattainshigherprecision24.17% and12.39%comparedwiththeexistingsystems,like CAT‐EEG‐EA‐K‐EmoConandCAT‐EEG‐EA‐K‐EmoCon respectively.
AUTHORS
C.AkalyaDevi∗ –DepartmentofInformationTech‐nology,PSGCollegeofTechnology,Coimbatore,India, e‐mail:akalya.jk@gmail.com.
D.KarthikaRenuka –DepartmentofInformation Technology,PSGCollegeofTechnology,Coimbatore, India,e‐mail:dkr.it@psgtech.ac.in.
∗Correspondingauthor
References
[1] S.Kim,H.Yang,N.Nguyen,S.PrabhakarandS. Lee,“WeDea:ANewEEG‐BasedFrameworkfor EmotionRecognition,” IEEEJournalofBiomedicalandHealthInformatics,vol.26,no.1,2022, pp.264‐275.Doi:10.1109/jbhi.2021.3091187.
[2] N.Salankar,P.MishraandL.Garg,“Emotion RecognitionFromEEGSignalsUsingEmpir‐icalModeDecompositionAndSecond‐Order DifferencePlot,” BiomedicalSignalProcessing andControl,vol.65,2021,p.102389.Doi: 10.1016/j.bspc.2020.102389.
[3] A.Subasi,T.Tuncer,S.Dogan,D.TankoandU. Sakoglu,“EEG‐BasedEmotionRecognitionUsing TunableQWaveletTransformAndRotationFor‐estEnsembleClassi ier,” BiomedicalSignalProcessingandControl,vol.68,2021,p.102648.Doi: 10.1016/j.bspc.2021.102648.
[4] P.V.andA.Bhattacharyya,“HumanEmotion RecognitionBasedOnTime–FrequencyAnal‐ysisOfMultivariateEEGSignal”, KnowledgeBasedSystems,vol.238,2022,p.107867.Doi: 10.1016/j.knosys.2021.107867.
[5] J.WangandM.Wang,“ReviewOfTheEmotional FeatureExtractionAndClassi icationUsingEEG Signals,” CognitiveRobotics,vol.1,2021,pp.29‐40.Doi:10.1016/j.cogr.2021.04.001.
[6] X.Zhou,X.TangandR.Zhang,“ImpactOfGreen FinanceOnEconomicDevelopmentAndEnvi‐ronmentalQuality:AStudyBasedOnProvin‐cialPanelDataFromChina,” EnvironmentalScienceandPollutionResearch,vol.27,no.16, 2020,pp.19915‐19932.Doi:10.1007/s11356‐020‐08383‐2.
[7] N.Garcia,B.RenoustandY.Nakashima, “ContextNet:representationandexplorationfor paintingclassi icationandretrievalincontext,” InternationalJournalofMultimediaInformation Retrieval,vol.9,no.1,2019,pp.17‐30.Doi: 10.1007/s13735‐019‐00189‐4.
[8] F.Zhou,Q.Yang,K.Zhang,G.Trajcevski,T. ZhongandA.Khokhar,“ReinforcedSpatiotem‐poralAttentiveGraphNeuralNetworksforTraf‐icForecasting,” IEEEInternetofThingsJournal,vol.7,no.7,2020,pp.6414‐6428.Doi: 10.1109/jiot.2020.2974494.
[9] A.ChowdhuryandD.De,“Energy‐ef icient coverageoptimizationinwirelesssensor networksbasedonVoronoi‐GlowwormSwarm Optimization‐K‐meansalgorithm,” AdHoc Networks,vol.122,2021,p.102660.Doi: 10.1016/j.adhoc.2021.102660.
[10] M.Khateeb,S.AnwarandM.Alnowami, “Multi‐DomainFeatureFusionforEmotion Classi icationUsingDEAPDataset,” IEEE Access.,vol.9,2021,pp.12134‐12142.Doi: 10.1109/access.2021.3051281.
[11] Y.Yin,X.Zheng,B.Hu,Y.ZhangandX.Cui, “EEGemotionrecognitionusingfusionmodel ofgraphconvolutionalneuralnetworksand LSTM,” AppliedSoftComputing,vol.100,2021,p. 106954.Doi:10.1016/j.asoc.2020.106954.
[12] V.Dissanayake,S.Seneviratne,R.Rana,E.Wen, T.KaluarachchiandS.Nanayakkara,“SigRep: TowardRobustWearableEmotionRecognition WithContrastiveRepresentationLearning”, IEEE Access,vol.10,2022,pp.18105‐18120.Doi: 10.1109/access.2022.3149509.
[13] K.Yang,B.Tag,Y.Gu,C.Wang,T.Dingler, G.Wadley,J.Goncalves.“Mobileemotion recognitionviamultiplephysiologicalsignals usingconvolution‐augmentedtransformer”. InProceedingsofthe2022International ConferenceonMultimediaRetrieval.pp.562‐570 2022.Doi:10.1145/3512527.3531385
[14] S.Koelstra,C.Muhl,M.Soleymani,J.S.Lee,A. Yazdani,T.Ebrahimi,T.Pun,A.Nijholt,andI. Patras,“DEAP:ADatabaseforEmotionAnalysis UsingPhysiologicalSignals,” IEEETransactions onAffectiveComputing,vol.3,no.1,2012,pp.18‐31.Doi:10.1109/t‐affc.2011.15.
[15] C.Y.Park,N.Cha,S.Kang,A.Kim,A.H.Khan‐doker,L.Hadjileontiadis,A.Oh,Y.Jeong,andU. Lee,“K‐EmoCon,amultimodalsensordatasetfor continuousemotionrecognitioninnaturalistic conversations,” Scienti icData,vol.7,no.1,2020. Doi:10.1038/s41597‐020‐00630‐y.
ENHANCINGSTOCKPRICEPREDICTIONINTHEINDONESIANMARKET:ACONCAVE
Submitted:4th October2023;accepted:26th January2024
MohammadDiqi,IWayanOrdiyasa DOI:10.14313/JAMRIS/3‐2024/24
Abstract:
Thisstudyaddressesthepressingneedforimprovedstock pricepredictionmodelsinthefinancialmarkets,focusing ontheIndonesianstockmarket.Itintroducesaninno‐vativeapproachthatutilizesthecustomactivationfunc‐tionRunReLUwithinaconcavelongshort‐termmemory (LSTM)framework.Theprimaryobjectiveistoenhance predictionaccuracy,ultimatelyassistinginvestorsand marketparticipantsinmakingmoreinformeddecisions. Theresearchmethodologyusedhistoricalstockprice datafromtenprominentcompanieslistedontheIndone‐siaStockExchange,coveringtheperiodfromJuly6,2015, toOctober14,2021.EvaluationmetricssuchasRMSE, MAE,MAPE,andR2wereemployedtoassessmodelper‐formance.TheresultsconsistentlyfavoredtheRunReLU‐basedmodelovertheReLU‐basedmodel,showcasing lowerRMSEandMAEvalues,higherR2values,and notablyreducedMAPEvalues.Thesefindingsunderscore thepracticalapplicabilityofcustomactivationfunctions forfinancialtimeseriesdata,providingvaluabletoolsfor enhancingpredictionprecisioninthedynamiclandscape oftheIndonesianstockmarket.
Keywords: stockpriceprediction,concaveLSTM,Run‐ReLU,Indonesianstockexchange,financialforecasting
Thepricesofstocksareintricateandever‐changingvariablesimpactedbymanyexternalele‐ments,includingpoliticaloccurrences,economicindi‐cators,naturalcalamities,andinternalfactors.These factorsmakestockpricemovementschallengingto predictaccurately[1].Thestockmarketisanonlin‐earandhighlyunpredictableenvironment,affected bynumerouselements[2].Thefuturepriceofstocks dependsonmanyfactors,makingitelusivetopredict basedsolelyonavailableinformation[3].However, researchershaveexploredmethodsandtechniques, suchasarti icialintelligencemodels,deeplearning, andmathematicalanalysis,toimprovestockprice predictionaccuracy[4, 5].Accurateforecastingof stockpricesiscrucialforinvestorstomakeinformed decisionsandincreasetheirreturns[6].Whileno methodcanguaranteeperfectpredictions,technolog‐icaladvancementsanddataanalysishaveprovided toolstoenhancepredictionmodelsanddevelopeffec‐tivetradingstrategies[7].
Theuniquecharacteristicsofstockpricemove‐mentsinIndonesia,whichdifferentiatethemfrom othercountries,canbeattributedtoseveralfactors. Firstly,theIndonesianstockmarketishighlydynamic andnonlinear,makingitchallengingtopredictfuture stockpricesaccurately[7].Additionally,thestock marketisin luencedbyvariousexternalfactors,such aspoliticalevents,naturaldisasters,and inancial crises,whichcancausesharpandunpredictable luc‐tuationsinstockprices[8].Moreover,theuseof advancedtechniquesindeeplearning,suchasLSTM andGRU,instockpricepredictionhasgainedpop‐ularityinIndonesia,indicatingashifttowardsmore sophisticatedmodelingapproaches[9].Thesefactors contributetotheuniquecharacteristicsofstockprice movementsinIndonesia,highlightingtheneedfor specializedpredictionmodelsandstrategiestailored totheIndonesianmarket.
Forseveralreasons,investors,traders,compa‐nies,capitalmarketregulators,and inancialana‐lystsneedaccuratestockpricepredictioninformation. Firstly,accuratepredictionscanhelpinvestorsmake informeddecisionsaboutbuyingorsellingstocks, potentiallyresultinginsigni icantpro its[10].Sec‐ondly,traderscanusestockpricepredictionstoiden‐tifytrendsandpatternsinthemarket,allowingthem tomaketimelyandpro itabletrades[11].Compa‐niescanbene itfromaccuratestockpricepredictions byadjustingtheirstrategiesandmakinginformed inancialdecisions[1].Capitalmarketregulatorsrely onaccuratepredictionstomonitorandregulatethe marketeffectively,ensuringfairandtransparenttrad‐ingpractices[12].Finally, inancialanalystsusestock pricepredictionstoprovidevaluableinsightsandrec‐ommendationstoinvestorsandcompanies,helping themnavigatethecomplexandvolatilestockmar‐ket[4].
ConventionalmethodslikeAutoregressiveInte‐gratedMovingAverage(ARIMA),machinelearning, anddeeplearningtechniquesarewidelyemployed inpredictingstockprices.ARIMAmodelsarepara‐metricstatisticalmodelscommonlyusedintime seriesanalysis,includingstockpriceprediction[10]. Machinelearningmethods,suchask‐nearestneighbor algorithm(KNN),arti icialneuralnetworks(ANNs), supportvectormachines(SVMs),andrandomforest (RF),havealsobeenappliedtolearntherelationship betweentechnicalanalysisfeaturesandpricemove‐ment[13].
Thepopularityofdeeplearningtechniques, includingconvolutionalneuralnetworks(CNN), longshort‐termmemory(LSTM)networks,and gatedrecurrentunits(GRU),hasincreasedin the ieldofstockpriceforecastingbecauseoftheir capabilitytoaddressnonlinearandmulti‐dimensional challenges[11].Thesemodelshaveshownpromising resultsinforecastingstockpricesbyconsidering variousfactorsandfeatures,includinghistoricalstock data,technicalindicators,andexternalfactorslike COVID‐19cases[12].
Theuncertaintyofthedirectionofmovementand theaccuracyoffuturestockpricesremainissuesin stockpricepredictionsduetoseveralfactors[14]. Firstly,stockmarketsarein luencedbyvarious complexfactorssuchaspolitics,economicgrowth, andinterestrates,makingitchallengingtopredict theirmovements[10]accurately.Additionally,the stockmarketishighlyvolatileandsubjecttosud‐denchanges,makingitchallengingtoforecastalto‐gether[11].Moreover,usingdifferentpredictionmod‐elsandtechniquesintroducesvariationsintheaccu‐racyofforecasts,leadingtouncertaintyinthedirec‐tionofstockpricemovement[1].Lastly,theavail‐abilityofvastamountsofdata,includingsocialmedia sentiments,introduceschallengesineffectivelyana‐lyzingandincorporatingthesedatasourcesintopre‐dictionmodels[3].Therefore,despiteadvancements inpredictionmodels,thestockmarket’sinherentcom‐plexityanddynamicnaturecontributetotheongoing uncertaintyinpredictingstockpricemovements[14].
Theactivationfunctionindeeplearningcanleave weaknessesinstockpricepredictionduetostockmar‐kets’complexandvolatilenature[15].Stockpricesare in luencedbyvariousunpredictableexternalfactors suchas inancialnews,sociopoliticalissues,andnatu‐ralcalamities[16].Activationfunctionsarecrucialin deeplearningmodelsutilizedinstockpriceprediction byintroducingnon‐linearitytocaptureintricatedata patternseffectively[17].Nevertheless,selectingan activationfunctioncanin luencethemodel’scapacity tomakeprecisestockpricepredictions.Variousacti‐vationfunctionspossessdistinctcharacteristicsand maynotbeappropriateforcapturingtheintricate non‐linearconnectionswithinstockmarketdata[18]. Therefore,selectingaproperactivationfunctionis crucialforimprovingtheaccuracyofstockpricepre‐dictionmodels.
Addressingthechallengeofhandlingintricate temporalpatternswithinstockpricedataremains anissueinstockpricepredictionforvariousrea‐sons.Firstly,conventionalapproachesthatrelysolely ontime‐seriesdataforindividualstocksareinsuf i‐cient,astheylackacomprehensiveviewofthesit‐uation[19].Secondly,theintricacyofmultipleele‐mentsaffectingstockpricescallsfortheutilization ofmoreexpansivedatasets,whichshouldencom‐passinformationregardingstockrelationships[10]. Thirdly,obtainingpreciseandup‐to‐dateinforma‐tionaboutstockrelationshipsischallengingsince industryclassi icationdatafromthird‐partysources
isfrequentlyapproximatedandmaybedelayed[11]. Lastly,predictingstockpricesinvolvesintegrating temporalinformationandrelationshipsamongstocks, whichrequiresadvancedmodelssuchasdeeplearn‐ingmethods[1].Therefore,theproblemofresponding tocomplicatedtemporalsignalsinstockpricedata persistsduetothelimitationsoftraditionalmethods andtheneedformorecomprehensiveandaccurate dataandadvancedpredictionmodels[3].
ThecharacteristicsoftheIndonesianstockmarket differfromotherglobalstockmarkets,creatingagap inthedevelopmentofstockpricepredictionmodels. TheIndonesianstockmarketisin luencedbypolitics, economicgrowth,andinterestrates[20].Thesefac‐torsandthemarket’svolatilitymakeaccuratefore‐castingchallenging[19].Additionally,thecomplex‐ityofthestockmarketandtheinterdependenceof stockswithinthemarketrequiremorecomprehensive dataandmodels[21].Conventionalapproachesthat exclusivelydependontime‐seriesdataforanindivid‐ualstockareinadequate[16].Therefore,thereisa needformodelsthatintegratetimeseriesinforma‐tion,relationshipinformation,andsentimentanalysis fromsocialmedia[4].Byincorporatingthesefactors, stockpricepredictionmodelscanprovidemoreaccu‐rateforecastsfortheIndonesianstockmarket.
Thisresearchishighlysigni icantasitintroduces aninnovativeapproachtostockpricepredictionin theIndonesianstockmarket,aimingtoenhancepre‐dictionaccuracyandassistinvestorsandmarketpar‐ticipantsinmakingmoreinformeddecisions.The noveltyofthisresearchliesinutilizingthecustom activationfunction,RunReLU,withinaconcaveLSTM modelthatcombinesvariousLSTMtypesforstock pricepredictionintheIndonesianstockmarket.The researchseekstocreateandassessanovelstockprice forecastingmodelutilizingtheconcaveLSTMarchi‐tecture,incorporatingthecustomizedRunReLUacti‐vationfunction,toimprovepredictionaccuracywithin theIndonesianstockmarket.
Thissectiondelvesintothefoundationaltheories underpinningourresearch,focusingonlongshort‐termmemory(LSTM)networksandtheinnovative concaveLSTMarchitecture,includingtheintroduction oftheRunReLUactivationfunction.
2.1.LongShort‐TermMemory(LSTM)
LSTM,classi iedasarecurrentneuralnetwork (RNN),wasdevelopedtoaddressthevanishinggra‐dientchallengepresentinconventionalRNNs.Its intricatearchitectureenablesittograspandretain extendedpatternsofrelianceinsequentialdata.The LSTMcellconsistsofthreegates:inputgate����,forget gate ����,andoutputgate ����,alongwithacellstate ���� [22],asformulatedinEquations(1)–(6).
2.1.1.InputGate
2.1.2.ForgetGate
2.1.3.OutputGate
2.1.4.CandidateCellState
2.1.5.NewCellState
2.1.6.HiddenState
Where ���� istheinputattimestep ��, ℎ��−1 isthe hiddenstatefromtheprevioustimestep, �� and �� areweightmatricesandbiasterms, �� isthesigmoid activationfunction, ������ℎ isthehyperbolictangent activationfunction.
2.2.ConcaveLSTM
BuildinguponthestandardLSTMframework,the ConcaveLSTMintegratesbothstackedandbidirec‐tionalLSTMlayerstoenhancemodelperformancefor complextime‐seriespredictions.Thishybridmodel isparticularlyadeptatcapturingnuancedpatterns in inancialmarkets,offeringarobustfoundationfor stockpriceforecasting.
ApivotalenhancementinourconcaveLSTMmodel istheincorporationoftheRunReLUactivationfunc‐tion[23].Designedtooptimizethemodel’slearning process,RunReLUintroducesadynamic,data‐driven approachtoactivation,allowingforadaptivethresh‐oldingbasedonthedistributionofinputs.This lexi‐bilityenhancesthemodel’sabilitytomodelnonlinear relationshipsinthedata,acommoncharacteristicof inancialtimeseries.
2.3.RunReLU
Activationfunctionsplayapivotalroleinneural networks,introducingnon‐linearityandenablingthe modeltolearncomplexpatternsindata.Traditional activationfunctions,suchastheRecti iedLinearUnit (ReLU),havebeenwidelyadoptedduetotheirsim‐plicityandeffectivenessinvarioustasks.However, thestaticnatureofthesefunctionscanlimittheir adaptability,especiallyinthevolatileandnon‐linear domainof inancialmarkets.
RunReLUisdesignedtoovercometheselimita‐tionsbyincorporatingadynamicelementintothe activationprocess.Itmodulatestheactivationthresh‐oldbasedonaGaussiandistribution,withparameters �� (mean)and �� (standarddeviation)tailoredtothe speci iccharacteristicsoftheinputdata,asshownin
Equation(7).Thisrandomizationallowsforamore lexibleresponsetotheinputfeatures,enhancingthe model’sabilitytocapturetheintricatedependencies within inancialtimeseries.
TheprimaryadvantageofRunReLUliesinits unparalleledadaptability,whichdynamicallyadjusts theactivationthresholdforeachinput,allowingitto handleadeptlythevolatilityandnon‐linearitytypical of inancialdata.Thisadaptabilitynotonlyenhances featurerepresentationbydynamicallyemphasizing ordeemphasizingfeaturesbasedontheirrelevance tothetaskathand,leadingtoaricherandmore nuancedunderstandingofthedata,butalsosigni i‐cantlyimprovesmodelgeneralization.Byintroducing variabilityintheactivationprocess,RunReLUmiti‐gatesover itting,enhancingthemodel’sabilitytoper‐formwellonunseendata.Furthermore,itscapacityto adjustactivationthresholdscontributestoincreased robustness,makingthemodelmoreresilienttothe noiseandanomaliesthatfrequentlyoccurin inancial datasets.Thissuiteofbene itsunderscoresRunReLU’s criticalroleinre iningpredictiveaccuracyandrelia‐bilityin inancialmodeling.
3.ResearchMethod
3.1.ResearchDesign
Theresearchdesigninvolvesdevelopingandeval‐uatingahybridmodel,concaveLSTM,thatcombines stackedandbidirectionalLSTM[12]withcustomRun‐ReLUactivationforstockpricepredictionondatafrom theIndonesianstockexchange.ThestackedLSTM modelutilizestheRunReLUactivationfunction,while thebidirectionalLSTMmodelusestheReLUactivation function.Figure1illustratesthearchitectureofcon‐caveLSTM.
3.2.Dataset
Thedatasetusedinthisstudyisobtainedfrom YahooFinanceandincludesthestockpriceinforma‐tionofthetenhighest‐rankedstocksontheIndonesia StockExchange.Thisdatasetcoverstheperiodfrom July6,2015,toOctober14,2021.Thestocksymbols andcompanynamesanalyzedinthisresearchare detailedinTable1.
3.3.ResearchProcedureandDataAnalysis
Theresearchmethodologyemployedinthisstudy revolvesaroundanalyzingandpredictingstockprices usingadatasetspanningfromJuly6,2015,toOctober 14,2021.Thedatasetencompassesdailystockprice dataandconsistsof iveprimaryfeatures:Open,High, Low,Close,andVolume[7,16].Recordswithavol‐umegreaterthanzerowereretainedtoensuredata quality,resultingin1269records.Thestudyfocuses exclusivelyontheClosefeatureforpriceprediction.
Figure1. ConcaveLSTMarchitecture
Table1. TenIndonesianstocks
Symbol Company
ACES AceHardwareIndonesiaTbk.
ADRO AdaroEnergyTbk.
AKRA AKRCorporindoTbk.
JPFA JAPFAComfeedIndonesiaTbk.
MIKA MitraKeluargaKaryasehatTbk.
PTBA TambangBatubaraBukitAsam (Persero)Tbk.
TKIM PabrikKertasTjiwiKimiaTbk.
TLKM TelkomIndonesia(Persero)Tbk.
TPIA ChandraAsriPetrochemicalTbk.
WIKA WijayaKarya(Persero)Tbk.
NormalizationwasperformedusingtheMinMax Scalertopreparethedataformodeling,ensuringthat allvaluesfallwithinaspeci iedrange[24].Thelast50 datapointsweresetasideforreferencetoactualdata. Ofthe1219datapoints,975weredesignated fortrainingthepredictivemodel,leavingtheremain‐ing244forvalidation.Thetrainingandvalidation stagesencompassed100epochs,allowingforgradual enhancementsinthemodel’sperformance. Followingthat,forecastsweregeneratedforthe stockpricesovertheforthcoming50days,utilizing thetestingdataset.Toevaluatethemodel’seffective‐ness,arangeofmetricswasused,encompassingroot meansquarederror(RMSE),meanabsoluteerror (MAE),meanabsolutepercentageerror(MAPE),and R‐squared(R2)[25,26].
Table2. PerformancemetricsofReLU‐basedmodel
Furthermore,thisresearchintroducedacompar‐ativeanalysisbetweenmodelsbasedontwoactiva‐tionfunctions:RunReLUandReLU.Thepredictive resultsofbothmodelsandtheactualdataweregraph‐icallyrepresented,providingavisualillustrationofthe model’sperformanceinpredictingstockprices.
4.ResultsandDiscussion
4.1.ModelPerformance
InTable 2,wepresenttheperformancemetrics ofourmodelutilizingtheReLUactivationfunctionto predictstockpricesfortenprominentcompaniesin themarket.Theseperformancemetrics,comprising RMSE,MAE,MAPE,andR2,providevaluableinsights intotheprecisionandef iciencyofourmodel’sfore‐castsforeachcompany’sstock.Theresultsdisplayed inthetableunderscorethemodel’sabilitytoprovide precisepredictions,withlowRMSEandMAEvalues andhighR2values,demonstratingitspotentialasa valuabletoolforinvestorsandmarketanalystsinthe assessmentofstockperformance.
InTable 3,wepresenttheperformancemetrics ofourmodelutilizingtheinnovativeRunReLUacti‐vationfunctiontopredictstockpricesfortenleading companiesinthemarket.Theseperformanceindica‐tors,encompassingRMSE,MAE,MAPE,andR2,sup‐plyvitalinformationregardingtheaccuracyandef i‐ciencyofourmodel’sforecastsforindividualcom‐panystocks.Theresultsdisplayedinthistableunder‐scoretheremarkableaccuracyachievedbyourmodel withtheRunReLUactivationfunction,showcasinglow RMSEandMAEvalues,highR2values,andminimal MAPEvalues.These indingsaf irmthepotentialof ournovelapproachtosigni icantlybene itinvestors andmarketanalystsinmakinginformeddecisionsand assessingstockperformancewithgreateraccuracy andreliability.
InFigures2through11,weprovideacomprehen‐sivevisualrepresentationofthepredictedandactual stockpricesforeachoftheselectedtencompanies overa50‐dayhorizon.Thebluelinedepictsthestock prices,offeringareferencepointformarketperfor‐mance.
Table3. PerformancemetricsofRunReLU‐basedmodel
Company
ACES 0,00760 0,00588 0,00918 0,97343 ADRO 0,00727 0,00379 0,00777 0,99479 AKRA 0,00318 0,00228 0,00627 0,99441 JPFA 0,00289 0,00220 0,00405 0,99434 MIKA 0,00227 0,00189 0,00363 0,99651 PTBA 0,00434 0,00320 0,00831 0,99482 TKIM 0,00835 0,00776 0,02007 0,92064 TLKM 0,00562 0,00444 0,01190 0,99335 TPIA 0,01163 0,01049 0,01565 0,98070 WIKA 0,00703 0,00567 0,05609 0,98925
PerformanceofAKRA
Concurrently,theredlinecorrespondstothestock pricepredictionsgeneratedbyourReLU‐basedmodel, whilethegreenlinerepresentspredictionsderived fromourRunReLU‐basedmodel.Closerproximity betweenthepredictedandactualdatapointssigni ies higheraccuracyinourmodels.
PerformanceofTKIM
Theaboveresearchoutcomesareinstrumentalin addressingourstudy’sprimaryresearchquestions andobjectives.Theperformancemetricsofourmod‐els,bothbasedonReLUandRunReLUactivations, shedlightontheiref icacyinpredictingstockpricesin theIndonesianstockmarket.These indingshavecru‐cialimplicationsforinvestorsandmarketparticipants seekingtomakemoreinformeddecisions.
Firstly,theresultshighlightthepotentialofour innovativeapproach,leveragingtheRunReLUactiva‐tionfunctionwithinaconcaveLSTMmodel,tosignif‐icantlyenhancepredictionaccuracy.ThelowerRMSE andMAEvaluesandhigherR2valuesindicatehigher precisionandreliabilityinthepredictions.Thisaligns withourresearchobjectivetoimprovepredictionpre‐cisionintheIndonesianstockmarket,whichiscrucial foreffectiveinvestmentstrategies.
Furthermore,thesubstantialreductioninMAPE values,particularlyevidentintheRunReLU‐based model,suggeststhatourapproachreducesprediction errors,enhancingthemodels’practicalutility.These indingsdirectlyaddresstheneedformoreaccurate predictionmodels,asidenti iedinourresearchmoti‐vation.
Insummary,theresearchoutcomesdemonstrate thatourinnovativemodels,especiallytheRunReLU‐basedmodel,offerpromisingavenuesforstockprice predictionintheIndonesianstockmarket.This researchcontributessigni icantlytothe ield,provid‐inginvestorsandmarketanalystswithenhancedtools formakingwell‐informeddecisionsandimproving theoverallaccuracyofstockpricepredictionsinthis dynamic inanciallandscape.
4.2.SummarizationofKeyFindings
Thisresearchtacklesthechallengeofenhanc‐ingstockpricepredictionaccuracyintheIndonesian stockmarketbyintroducingapioneeringapproach thatleveragescustomactivationfunctions,including RunReLU,withinconcaveLSTMmodels.Theresearch addressesthecriticalneedformoreprecisepredic‐tionmodelsinthecomplexandvolatile inancialmar‐ketcontext.Thesigni icant indingsrevealthatthe RunReLU‐basedmodeloutperformstheReLU‐based counterpart,showcasinglowerRMSEandMAEvalues, higherR2values,andsigni icantlyreducedMAPEval‐ues,demonstratingsubstantialimprovementsinpre‐dictionprecision.Theseoutcomesmarkasigni icant contributiontothe ieldandofferinvestorsandmar‐ketanalystsvaluabletoolsformakingmoreinformed decisionsinthedynamiclandscapeoftheIndonesian stockmarket.
4.3.InterpretationsoftheResults
Theresultsrevealedaconsistentandnotablepat‐ternwhereintheRunReLU‐basedmodelconsistently surpassesitsReLU‐basedcounterpartacrossmultiple evaluationmetrics,includingRMSE,MAE,MAPE,and R2,signifyingenhancedpredictionaccuracy.These outcomesalignwiththeresearch’sexpectations,as thenovelutilizationoftheRunReLUactivationfunc‐tionwasanticipatedtoimproveprecisioninstock priceprediction.The indingsareconsistentwith priorresearchemphasizingthesigni icanceofcus‐tomactivationfunctionsandhybridmodelsinre ining deeplearningmodels’performancein inancialpre‐dictiontasks.
Anunexpectedobservationistherelativelylow R2valueforTKIMintheRunReLU‐basedmodel,indi‐catingpotentialexternalfactorsin luencingitsstock behaviorthatnecessitatefurtherinvestigation.The investigationintoTKIM’sanomalyrevealsthatits lowerR2value,indicativeofamismatchbetween themodel’spredictionsandactualstockperformance, maystemfromacon luenceofexternalfactorsspeci ic toTKIManditsindustry.ThesensitivityofTKIM,akey playerinthepaperandpulpsector,tomarketdynam‐icslikerawmaterialcostsandinternationaltradepoli‐ciespotentiallyexacerbatesthisdivergence.Addition‐ally,sector‐speci icvolatility,drivenbyenvironmen‐talregulations,sustainabilitytrends,andthepivot towardsdigitalmediums,couldintroduceunpre‐dictabilitynotaccountedforbythemodel.More‐over,unforeseenevents—operational,regulatory,or corporate—mighthaveprecipitatedstockprice luc‐tuationsbeyondthemodel’spredictivecapacitybased onhistoricaldata.Thisanomalyunderscoresthe necessityofintegratingexternalfactoranalysisand sector‐speci icconsiderationstoenhancemodelaccu‐racyandreliability.
4.4.ImplicationsoftheResearch
Theresultsobtainedinthisresearchholdsignif‐icantrelevanceandimplicationsforbothstockprice predictionand inancialmarkets.Firstly,theconsis‐tentsuperiorityoftheRunReLU‐basedmodelinterms oflowerRMSE,MAE,MAPE,andhigherR2values emphasizesitspracticalapplicabilityinenhancing predictionaccuracy.
These indingsalignwiththeexistingliterature thatunderscorestheimportanceofcustomactiva‐tionfunctionsandinnovativemodelarchitectures forimprovingdeeplearningmodels’performancein inancialpredictiontasks.Furthermore,theresearch contributesnewinsightsbyintroducingtheRunReLU activationfunctionasavaluabletoolforstockprice prediction,offeringapracticalalternativetostandard activationfunctions.TheunexpectedlylowerR2value forTKIMhighlightstheneedforfurtherresearchinto company‐speci icexternalfactorsaffectingstockper‐formance.Overall,thisresearchenhancesourunder‐standingofthepotentialofcustomactivationfunc‐tionsandinnovativemodelapproachestore inestock pricepredictionaccuracy,providingvaluableinsights forinvestorsandmarketanalysts.
4.5.MarketSpecificityandGeneralizability
TheconcaveLSTMmodel,enhancedwiththeRun‐ReLUactivationfunction,hasshownnotablesuccess withintheIndonesianstockmarket,showcasingits capabilitytonavigatethemarket’suniquevolatility, economicpolicies,andinvestorbehaviors.Thesespe‐ci icattributesoftheIndonesianmarketplayedapiv‐otalroleinthemodel’sinitialdevelopmentandsubse‐quentre inement,ensuringitwaswell‐suitedtoman‐agethepronounced luctuationsandunpredictability typicalofemergingmarkets.Theadaptabilityofthe RunReLUfunction,inparticular,waskeyinaddressing thesemarketcharacteristics,allowingforanuanced approachtothenonlineardynamicsencountered.
DespiteitsoptimizationfortheIndonesiancon‐text,thefoundationalprinciplesoftheconcaveLSTM modelholdpotentialapplicabilityacrossabroadspec‐trumof inancialenvironments.Themodel’sarchi‐tecture,whichemphasizestheprocessingoflong‐andshort‐termmemorythroughLSTMlayers,coupled withthedynamicnatureoftheRunReLUactivation function,isdesignedtouniversallycapturecomplex temporalrelationshipsinherentinstockdata.How‐ever,toeffectivelyextenditsapplicationtoothermar‐kets,considerationsaroundmarketvolatility,regula‐toryandeconomicfactors,andthequalityandavail‐abilityofdatamustbethoroughlyaddressed.This suggestsatheoreticalandpractical lexibilityinthe model’sapplication,indicatingthat,withappropriate adjustments,theconcaveLSTMmodelcouldserveasa powerfultoolfor inancialanalysisandpredictionon aglobalscale,offeringinsightsintotheintricaciesof variousstockmarketsaroundtheworld.
Thestudy’sconclusionsunderscorethe substantialbene itsofemployingthecustom activationfunctionRunReLUwithinconcaveLSTM modelstoenhancestockpricepredictionaccuracy intheIndonesianstockmarket.These indingsare robust,withconsistentpatternsoflowerRMSE,MAE, MAPE,andhigherR2valuescomparedtostandard ReLUactivationfunctionmodels.Theresearch introducesthevaluableinsightthatcustomactivation functions,tailoredtospeci icpredictiontaskslike stockprices,canbepracticalalternativestostandard activations.
Whileofferingsigni icantinsightsintostockprice predictionusingtheconcaveLSTMmodelwithinthe Indonesianmarket,thisstudypresentslimitations tiedtothedataset’sscopeandthemarket’sdistinctive characteristics.Theanalysisisrootedindatafromten leadingIndonesiancompanies,re lectingthemarket’s volatility,trends,andsectoralidiosyncrasies.Such depthprovidesafertiletestingground,yetthemar‐ket’semergingstatus,uniqueregulatorylandscape, andtheeconomicbackdropcouldhinderthedirect transpositionofthese indingstodissimilar,particu‐larlydeveloped,markets.Thetailoredcalibrationof themodel’sparametersandtheRunReLUfunctionto theIndonesiancontextunderscoresapotentialchal‐lengeingeneralizingtheseresultsacrossmarkets withdivergentcharacteristicsintermsofvolatility, liquidity,andinvestmentpatterns,highlightingareas forfutureresearchtoexpandthemodel’sglobalrele‐vanceandapplicability.
Despitetheselimitations,theresultsremainvalid, supportedbyarigorousresearchdesign,statisti‐calanalysis,andtheconsistentperformanceofthe RunReLU‐basedmodel,offeringvaluableinsightsfor stockmarketparticipantsand inancialanalysts.
4.7.RecommendationsforFutureResearch
Asweanticipateadvancementsin inancialmod‐elingandthepredictionofstockprices,theenhance‐mentoftoolsliketheconcaveLSTMmodelbecomes paramount.Ourrecommendationsaimtonavigate theintricaciesof inancialmarkets,augmentthe interpretabilityofcomplexmodels,andsolidifytheir reliabilityacrossvariedmarketscenarios.Enhanc‐ingmodelinterpretabilityiscrucial,astheintricate natureofdeeplearningoftenobscuresthemodel’s decision‐makingprocess.Byimplementingfeature importanceanalysistechniquessuchasSHAPorLIME, wecanelucidatethein luenceofspeci icinputson predictions,offeringtangibleinsightsintothedriv‐ingfactorsbehindstockmovements.Additionally, leveragingvisualizationtoolstoillustratethemodel’s internalmechanicsdemysti iesitsoperations,aiding bothdevelopersandstakeholdersinunderstanding itsfunctionality.
Furthermore,integratingexternalfactorslikeeco‐nomicindicatorsandgeopoliticaleventscansigni i‐cantlyre inethemodel’spredictiveprecision.Devel‐opingacomprehensiveframeworktoincorporatea diversearrayofdatasources—includingnewsfeeds andsocialmediasentiment—intothetrainingdataset willallowforanuancedunderstandingofstockprice in luencers.Adoptingevent‐drivenmodelingtech‐niques,supportedbyNLPanalysisof inancialreports andnewsarticles,cancapturethemarket’sreaction tounforeseenevents.Moreover,exploringensemble methodsandsentimentanalysispromisestoenhance accuracybyamalgamatingpredictionsfromvarious modelsandintegratingmarketsentiment.Conduct‐ingcomparativestudiesacrossdifferentmarketsand devisingadaptationstrategiesforthemodelwill ensureitsapplicabilityandrobustness,makingita versatiletoolforglobal inancialanalysis.
Theresearch’sobjectivewastodevelopandassess aninnovativestockpricepredictionmodelbasedona hybridLSTMarchitecturewiththecustomRunReLU activationfunctiontoenhancepredictionaccuracyin theIndonesianstockmarket.Thesupportingevidence forthisobjectiveliesintheresearchoutcomes,which consistentlydemonstratethattheRunReLU‐based modeloutperformstheReLU‐basedmodelacrossvar‐iousevaluationmetrics,includingRMSE,MAE,MAPE, andR2.These indingsvalidatetheeffectivenessof theinnovativeapproachinimprovingtheaccuracyof stockpricepredictionswithintheIndonesianmar‐ketcontext.Consequently,theresearch’scontribution isintroducingandvalidatingtheRunReLUactivation functionasavaluabletoolforstockpriceprediction, offeringapracticalalternativetoconventionalactiva‐tionfunctionsandenhancingtheprecisionofpredic‐tionsforinvestorsandmarketanalysts.
AUTHORS
MohammadDiqi∗ –Dept.ofInformatics,Universi‐tasRespatiYogyakarta,Yogyakarta,55281,Indonesia, e‐mail:diqi@respati.ac.id. IWayanOrdiyasa –Dept.ofInformatics,Universi‐tasRespatiYogyakarta,Yogyakarta,55281,Indonesia, e‐mail:wayanordi@respati.ac.id.
∗Correspondingauthor
References
[1] B.Li,X.Gui,andQ.Zhou,“ConstructionofDevel‐opmentMomentumIndexofFinancialTechnol‐ogybyPrincipalComponentAnalysisintheEra ofDigitalEconomy,” Comput.Intell.Neurosci.,vol. 2022,2022,doi:10.1155/2022/2244960.
[2] Y.Zhao,“ANovelStockIndexIntelligentPredic‐tionAlgorithmBasedonAttention‐GuidedDeep NeuralNetwork,” Wirel.Commun.Mob.Comput., vol.2021,2021,doi:10.1155/2021/6210627.
[3] A.H.Dhaferetal.,“EmpiricalAnalysisfor StockPricePredictionUsingNARXModel withExogenousTechnicalIndicators,” Comput.Intell.Neurosci.,vol.2022,2022, doi:10.1155/2022/9208640.
[4] S.K.Kumaretal.,“StockPricePrediction UsingOptimalNetworkBasedTwitter SentimentAnalysis,” Intell.Autom.SoftComput., vol.33,no.2,pp.1217–1227,2022,doi: 10.32604/iasc.2022.024311.
[5] Z.Bao,Q.Wei,T.Zhou,X.Jiang,andT.Watanabe, “Predictingstockhighpriceusingforecasterror withrecurrentneuralnetwork,” Appl.Math.NonlinearSci.,vol.6,no.1,pp.283–292,2021,doi: 10.2478/amns.2021.2.00009.
[6] G.A.Altarawneh,A.B.Hassanat,A.S.Tarawneh, A.Abadleh,M.Alrashidi,andM.Alghamdi, “StockPriceForecastingforJordanInsurance CompaniesAmidtheCOVID‐19Pandemic UtilizingOff‐the‐ShelfTechnicalAnalysis Methods,” Economies,vol.10,no.2,2022,doi: 10.3390/economies10020043.
[7] S.Hansun,A.Suryadibrata,andD.R.Sandi,“Deep LearningApproachinPredictingPropertyand RealEstateIndices,” Int.J.Adv.SoftComput. itsAppl.,vol.14,no.1,pp.60–71,2022,doi: 10.15849/IJASCA.220328.05.
[8] D.S.N.UlumandA.S.Girsang,“Hyperparameter OptimizationofLong‐ShortTermMemoryusing SymbioticOrganismSearchforStockPrediction,” Int.J.Innov.Res.Sci.Stud.,vol.5,no.2,pp.121–133,2022,doi:10.53894/ijirss.v5i2.415.
[9] D.Satria,“PredictingBankingStockPricesUsing Rnn,Lstm,andGruApproach,” Appl.Comput.Sci.,vol.19,no.1,pp.82–94,2023,doi: 10.35784/acs‐2023‐06.
[10] W.Lu,J.Li,J.Wang,andS.Wu,“aNovelModel forStockClosingPricePredictionUsingCnn‐Attention‐Gru‐Attention,” Econ.Comput.Econ. Cybern.Stud.Res.,vol.56,no.3,pp.251–264, 2022,doi:10.24818/18423264/56.3.22.16.
[11] M.RatchagitandH.Xu,“ATwo‐Delay CombinationModelforStockPricePrediction,” Mathematics,vol.10,no.19,2022,doi: 10.3390/math10193447.
[12] M.MohtashamKhani,S.Vahidnia,andA.Abbasi, “ADeepLearning‐BasedMethodforForecast‐ingGoldPricewithRespecttoPandemics,” SN Comput.Sci.,vol.2,no.4,pp.1–12,2021,doi: 10.1007/s42979‐021‐00724‐3.
[13] A.Ntakaris,J.Kanniainen,M.Gabbouj,andA. Iosi idis, Mid-pricepredictionbasedonmachine learningmethodswithtechnicalandquantitativeindicators,vol.15,no.6June.2020.doi: 10.1371/journal.pone.0234107.
[14] S.Mishra,T.Ahmed,V.Mishra,S.Bourouis, andM.A.Ullah,“AnOnlineKernelAdaptive
Filtering‐BasedApproachforMid‐PricePre‐diction,” Sci.Program.,vol.2022,2022,doi: 10.1155/2022/3798734.
[15] M.A.Ledhem,“Deeplearningwithsmalland bigdataofsymmetricvolatilityinformationfor predictingdailyaccuracyimprovementofJKII prices,” J.Cap.Mark.Stud.,vol.6,no.2,pp.130–147,2022,doi:10.1108/jcms‐12‐2021‐0041.
[16] N.DeepikaandM.Nirapamabhat,“Anoptimized machinelearningmodelforstocktrendanticipa‐tion,” Ing.desSyst.d’Information,vol.25,no.6, pp.783–792,2020,doi:10.18280/isi.250608.
[17] M.K.Daradkeh,“AHybridDataAnalyticsFrame‐workwithSentimentConvergenceandMulti‐FeatureFusionforStockTrendPrediction,” Electronics,vol.11,no.2,2022,doi:10.3390/elec‐tronics11020250.
[18] X.Teng,T.Wang,X.Zhang,L.Lan,andZ. Luo,“EnhancingStockPriceTrendPrediction viaaTime‐SensitiveDataAugmentation Method,” Complexity,vol.2020,2020,doi: 10.1155/2020/6737951.
[19] C.Zhao,P.Hu,X.Liu,X.Lan,andH.Zhang, “StockMarketAnalysisUsingTimeSeries RelationalModelsforStockPricePrediction,” Mathematics,vol.11,no.5,2023,doi: 10.3390/math11051130.
[20] K.E.Rajakumari,M.S.Kalyan,andM.V.Bhaskar, “ForwardForecastofStockPriceUsingLSTM MachineLearningAlgorithm,” Int.J.Comput.TheoryEng.,vol.12,no.3,pp.74–79,2020,doi: 10.7763/IJCTE.2020.V12.1267.
[21] L.LiandB.M.Muwafak,“Adoptionofdeeplearn‐ingMarkovmodelcombinedwithcopulafunc‐tioninportfolioriskmeasurement,” Appl.Math. NonlinearSci.,vol.7,no.1,pp.901–916,2022, doi:10.2478/amns.2021.2.00112.
[22] M.C.Lee,J.W.Chang,S.C.Yeh,T.L.Chia,J.S. Liao,andX.M.Chen,“Applyingattention‐based BiLSTMandtechnicalindicatorsinthedesign andperformanceanalysisofstocktradingstrate‐gies,” NeuralComput.Appl.,vol.34,no.16,pp. 13267–13279,2022,doi:10.1007/s00521‐021‐06828‐4.
[23] M.Diqi,“TwitterGAN:robustspamdetectionin twitterusingnovelgenerativeadversarialnet‐works,” Int.J.Inf.Technol.,vol.15,no.6,pp.3103–3111,2023,doi:10.1007/s41870‐023‐01352‐1.
[24] E.K.Ampomah,G.Nyame,Z.Qin,P.C.Addo,E. O.Gyam i,andM.Gyan,“Stockmarketpredic‐tionwithgaussiannaïvebayesmachinelearning algorithm,” Inform.,vol.45,no.2,pp.243–256, 2021,doi:10.31449/inf.v45i2.3407.
[25] A.Y.Fathi,I.A.El‐Khodary,andM.Saafan, “AHybridModelIntegratingSingularSpectrum AnalysisandBackpropagationNeuralNetwork forStockPriceForecasting,” Rev.d’Intelligence Artif.,vol.35,no.6,pp.483–488,2021,doi: 10.18280/ria.350606.
[26] J.Zhang,“ForecastingofMusicalEquipment DemandBasedonaDeepNeuralNetwork,” Mob.Inf.Syst.,vol.2022,2022,doi: 10.1155/2022/6580742.
Submitted:14th February2024;accepted:25th March2024
LeninKanagasabai
DOI:10.14313/JAMRIS/3‐2024/25
Abstract:
InthispaperAtlanticbluemarlin(ABM)optimization algorithm,Boopsoptimization(BO)algorithm,Chironex fleckerisearchoptimization(CSO)algorithm,general practitioner‐sickperson(PS)optimizationalgorithmare appliedforsolvingfactualpowerlossreductionproblem. NaturalactionsofAtlanticbluemarlinareemulatedto designtheAtlanticbluemarlin(ABM)optimizationalgo‐rithmandpopulaceintheexaminationspaceiscapri‐ciouslystimulated.Boopsoptimization(BO)algorithm isdesignedbyimitatingthestalkingphysiognomiesof Boops.CSOisbasedonthedriveandsearchbehavior ofChironexfleckeri.Ageneralpractitionerwilltreata sickpersonwithvariousprocedureswhichhavebeenimi‐tatedtomodeltheProjectedPSalgorithm.Inoculation, medicineandoperationaretheproceduresconsideredin thePSalgorithm.Atlanticbluemarlin(ABM)optimiza‐tionalgorithm,Boopsoptimization(BO)algorithm,Chi‐ronexfleckerisearchoptimization(CSO)algorithm,gen‐eralpractitioner–sickperson(PS)optimizationalgorithm validatedinIEEE57,300systemsand220KVnetwork. Factualpowerlosslessening,powerdivergencerestrain‐ing,andpowerconstancyindexamplificationhavebeen attained.
Keywords: atlanticbluemarlin,Boops,Chironexfleckeri, generalpractitioner,sickperson
1.Introduction
Factualpowerlossreductionisaleadingfeature intheelectricalpowertransmissionsystem. Manymethodologiesareappliedtosolvethe problem[5–11].Inthispaperfouralgorithms havebeende inedandmodeledtosolvethefactual lossreductionprobleminanelectricalpowerloss reductioninelectricalpowertransmissionsystem.
KeyObjectives
Factualpowerlosslessening,powerdivergence restrainingandpowerconstancyindexampli ication arekeyobjectivesinthispaper.
Design
TheAtlanticbluemarlin(ABM)optimizationalgo‐rithm,Boopsoptimization(BO)algorithm,Chironex leckerisearchoptimization(CSO)algorithm,general practitioner–sickperson(PS)optimizationalgorithm arealldesignedtobeappliedforsolvingtheproblem.
AtlanticBlueMarlinOptimizationAlgorithm
‐ NaturalactionsofAtlanticbluemarlin(Fig. 1)are emulatedtodesigntheAtlanticbluemarlin(ABM) optimizationalgorithm.
‐ EntrantsolutionsintheproposedABMalgorithm areAtlanticbluemarlin,andthepopulaceinthe examinationspaceisquixoticallystimulated.
‐ Hegemonyinvolvesrepetitionoftheunpretentious appropriatesolutiontosucceedinggenerations.
Boopsoptimizationalgorithm
‐ Boopsoptimization(BO)algorithmisdesignedby imitatingstalkingphysiognomies.
‐ Asaclustertheystalkthequarrybyformingthekey andsubordinateclusters.OneBoops(Fig.2)willset uppursuitbehindthequarryandtheaccompanying Boopswillformawallsuchthatthequarrycan’t moveaway.
‐ OncethevictimreachesoneoftheBoopsinthewall formationtheninevitablyitwillbeafreshpursuer.
Chironexfleckerisearchoptimizationalgorithm
‐ TheChironex leckerisearchoptimization(CSO) algorithmisbasedonthedriveandsearchbehavior ofChironex leckeri(Fig.3).
‐ Chironex leckeriwillexploittheirlimbstoparalyze theirpreybyinjectingvenom.Countlesstimesinthe oceanChironex leckeri’saremassedoverallandit isknownasthespreadofChironex leckeri(ina speci iclocation).
‐ Whenthecircumstancesareoptimisticforthemin theoceanChironex leckeriwillformaswarminto oceancurrents.
Generalpractitioner–sickpersonoptimizationalgo‐rithm
‐ Generalpractitionertreatsthesickperson(Fig. 4) withvariousprocedures;thishasbeenimitatedto modeltheprojectedPSalgorithm.
‐ Ingeneral,peoplewillbeinoculated,Withrespect todisorderanddisease–medicaltreatmentwillbe givenbymedicines.Ifneededanoperationonthe sickpersonwillbedonewhichcompletelydepends ontheconditions.
‐ Inoculation,medicine,andoperationaretheproce‐duresthathavebeenconsideredasthephasesofthe projectedPSalgorithm.
Validationofthealgorithms
TheAtlanticbluemarlin(ABM)optimizationalgo‐rithm,Boopsoptimization(BO)algorithm,Chironex leckerisearchoptimization(CSO)algorithmandgen‐eralpractitioner–sickperson(PS)optimizationalgo‐rithmarevalidatedinIEEE57,300systemsand220 KVnetwork.
2.ProblemFormulation
Powerlossminimizationisde inedby
m,
→controlanddependentparameters
F objectivefunction gk conductancebranch
ViandVjarevoltagesatbusesi,j Nbr numberoftransmissionlines
��ij phaseangles
VLk →Loadvoltageinkth loadbus
Vdesired Lk →Voltagedesiredatthekth loadbus
QGK →reactivepowergenerated atkth loadbusgenerators
QLim KG →reactivepowerlimits
NLB,Ng→ numberloadandgeneratingunits
NB→ numberofbuses
PG →realpowerofthegenerator
QG → reactivepowerofthegenerator
PD →realloadofthegenerator
QD → reactiveloadofthegenerator
Gij →mutualconductanceofbusiandbusj
Bij →susceptanceofofbusiandbusj
Equalityandinequalityconstraintsarede inedas,
Pg activepowerofslackbus
Qg reactivepowerofgenerators
max, min→ maximumandminimumvalue
VLi →busvoltagemagnitude
Ti →transformerstapratio
Objectivefunctioninmultiobjectivemodeisde ined as,
Capriciouslocationintheprocedureisde inedas,
nc →numberofswitchablereactivepowersources ng → numberofgenerators ����→����������������������������������������
3.AtlanticBlueMarlinOptimizationAlgorithm
Atlanticbluemarlinsarerapaciouspredatorocean ishthatschoolandcanstalksardinesingroups, attackformtheaboveherdthesardinesdynamically andprey ishcannotescapeandtheprey ishcannot escapeformtheschoolofAtlanticbluemarlin[1]. ThesehabitsofAtlanticbluemarlinareemulatedto designtheAtlanticbluemarlin(ABM)optimization algorithmforthepowerlosslesseningproblem.
EntrantsolutionsintheproposedABMalgorithm areAtlanticbluemarlin,andthepopulaceintheexam‐inationspaceiscapriciouslystimulated.Inthepene‐tratingspaceexistinglocationoftheithadherentis de inedas
ABML → locationofcapriciouslocation Atlanticbluemarlin Fitnessrateiscomputedas,
TheSardinegroupisamalgamatedintheAtlantic bluemarlinapproachandintheexaminationarea it’swhirling.Atthattimethesardineslocationand appropriatenessareiscomputedas,
specifythelocationofSardines
Intermittentlygrandersolutionscanbemisplaced whilestreamliningthelocationofexaminationrepre‐sentativesandfreshlocationsmaybemoremeager thantheprecedinglocationssogranderselectionis linked.Hegemonyinvolvesrepetitionoftheunpreten‐tiousappropriatesolutiontosucceedinggenerations. ThelocationofthegranderAtlanticbluemarlinand thebruisedsardineswhichownthesuperlativeappro‐priatenessrateisindicatedas,
where
specifyHegemonyAtlanticbluemarlin
indicatethebruisedSardines. IntheproposedAtlanticbluemarlinapproachthe freshlocationofAtlanticbluemarlindesignatedas,
where��israndomand����ispreycompactness
Atlanticbluemarlin ����.����Atlanticbluemarlin +����.����Sardines
ThroughoutthestalkingthefreshlocationoftheSar‐dinesisspeci iedas,
(19)
where ���������� specifyBoutcontrolofAtlanticblue marlin
���� ���������� indicateprecedingAtlanticbluemarlin ����=��×(2×��������×��)
ThroughBoutcontrolquantityofSardineswill streamlinethelocation(��),parameterno.(��), ��=Q_Sar����
�� ������specifythequantityofSardines
ProbabilitiesofAtlanticbluemarlintostalkfreshSar‐dinesisde inedas,
<��(��������) (20)
1) Start
2) EngendertheAtlanticbluemarlinpopulation
3) ArbitrarilyCreatepopulationofSardines
4) Factorvaluesareselected
5) Computethe itnessrateofAtlanticbluemarlin
6) Computethe itnessrateofSardines
7) ������HegemonyAtlanticbluemarlin
8) ��������ℎ��bruisedSardines
9) ��ℎ��������������criterionnotattained
10) ������������ℎAtlanticbluemarlin����������������ℎ������������ ��������
11) ���� =2.0×��(0,1)×����−����
12) RationalisedtheAtlanticbluemarlinlocation
13) ����
× ��(0,1)×
14) Endfor
15) CalculatetheBoutcontrolofAtlanticbluemarlin
16) BC=m×(2×iter×��)
17) whileBC<0.5;������������������������
18) ��=Q_Sar×BC
19) ��=par×BC
20) SelectthesetofSardinesbasedon��and��
21) RationalizedthelocationofSardines
22) ����
23) Endif
24) Calculate itnessrateofallSardines
25) OncesuperiorSardinesfoundthenexchangewith bruisedSardines
26) ���� ������ =���� �� ������(��������)<��(��������)
27) Atthatmomentengenderthepopulaceand removethehuntedSardines
28) StreamlinethepremiumAtlanticbluemarlin
29) Rationalisethe inestSardines
30) Endif
31) Endwhile
32) ReturnbestAtlanticbluemarlin
33) End
Boopsoptimization(BO)algorithmisdesigned byimitatingtheactionsofBoops.Boopspossessthe obligingstalkingphysiognomies[2].Asaclusterthey stalkthequarrybyformingthekeyandsubordi‐natebunches.OneBoopswillstartpursuitbehindthe quarryandthewaitingBoopswillformawallsuch thatquarrycan’tmoveaway.Oncethevictimreaches oneoftheBoopswhichisinthewallformationthen inevitablyitwillbeafreshpursuer.Boopswhichis apursuerwillbeconvertedtowallmakerandBoops whichiswallformationmaybeturnedtobeapur‐suerdependinguponlocationandcircumstances.The examinationareaiscreatedonthefoundationofa stalkingzone.Contingentonthethree‐dimensional disseminationoftheentity’spopulaceandsubsti‐tuteclustersBoopsoptimization(BO)algorithmis designed.
Boopspopulationiscapriciouslycreated,
Limitations–maximumandminimumareexpressed as,
Thentheresolutionparametersareexpressedas
TheentirepopulaceofBoopswillbealienatedinto substitutepopulationandclustersaredesignedfor amalgamatedstalking.Boopsoptimizationapproach has
Theinfowillbe ixedonthefoundation ofthepopulaceofBoops,thentheinfoideas ����������1,����������2,…,������������ andshapederror ���� inthe��������ℎ��isde inedas,
��(��������ℎ��)=
��∈��������ℎ�� ������������ −����
��=1,2,…,ℎ;��������ℎ�� =1,2,…,�� (23)
For ��������ℎ�� thequantityofalignederrorrateis describedas,
����(��)= �� ��=1 ��(��������ℎ��) (24)
ThroughoutstalkingtherewillbeonePursuerBoops anditslocationwillbealtered,whichwillbecontin‐genttoplaceanddriveofthevictim.Selectingthe PursuerBoopsamongthebunchwillbegrounded onthevictimlocation;atanyinstantoncethevictim touchtheBoopswhichisinwallformation,atthat timethatspeci icBoopswillbethefreshpursuer.At thatjuncturethefreshpositionofthepursuerBoops isde inedas,
��������ℎ(����+1 �� )=������������������������������(���� ��) +��⊕��(��) 0<��≤2 (25)
InthisprojectedBoopsoptimization(BO)algorithm�� isemployedfortheregulatingofthephasesizethen theratewillbeaugmented. ��=2.0+ 0.001⋅�� ��������/10 (26)
Levy(L)[5]issmearedas,
(��)=��⊕��(��)∼�� �� |��|1/��
∼N 0,��
(27)
Renderingtothelocationofthevictim,thefreshplace of���� isde inedas,
(30)
SpaceamongstwallandpursuerBoopsismathemati‐callydescribedas,
(31)
AtanyinstantthepursuerBoopswillturnoutto beawallBoopsandviceversa.Itiscontingenton theappropriatenessrateoftherole.Ifauniquezone hasbeenentirelyexploited,atthatmomentinstantly alterationofthezonewillhappen,anditwillbe de inedas,
Groundedonthelevydissemination,thefreshlocation ofthepursuerBoopsisde inedas
(��) (28)
FreshlocationofpursuerBoopsrenderingtocompre‐hensive inestisde inedas,
(29)
InthestratagemofthestalkingthewallBoops���� is,
1) Start
2) InitializetheBoopspopulation
3) ����������1,����������2,…,������������ 4) Computethe itnessvalue
5) ������������������ℎ������������
6) ��������������ℎ��Boopspopulationintobunches
7) {��������ℎ1,��������ℎ2,..��������ℎ��}
8) ������������������������ℎ�������������������� ����������
9) ��ℎ������(��<��������)
10) Foreach��������ℎ��
11) ApplystalkingagendaforpursuerBoops
12) ApplywallplanforanothersetofBoops 13) Calculatethe itnessrateforBoops
14) �������� ����������������������>���� ��ℎ���� 15) Exchangetherolebystreamlining���� 16) EndIf 17) �������� ����������������������>���������� ��ℎ����
18) Streamline����������
19) EndIf
20) �������� ������������������������������������������������,��ℎ���� 21) ��←��+1 22) EndIf 23) ������>��
24) Applyanagendaforchangingthezone 25) ��←0
26) EndIf
27) EndFor 28) ��←��+1
29) EndWhile
30) Returnthe����������
31) End
TheChironex leckerisearchoptimization(CSO) algorithmisdesignedtosolvetheproblem.CSOis basedonthedriveandsearchbehaviorofChironex leckeri.ObviouslyChironex leckeriwillexploittheir limbstoparalyzetheirpreybyinjectingthevenom[3]. Chironex leckeriwillexploittheirlimbstoparalyze theirpreybyinjectingvenom.Countlesstimesinthe oceanChironex leckeri’saremassedoverallanditis knownasthespreadofChironex leckeri(inaspeci ic location).Whenthecircumstancesareoptimuminthe oceanChironex leckeriwillformaswarm,andusing currents.ButChironex leckeriwon’tbemarooned atanylocation.Withattentionpaidtothenutrition locationandamountofnutritionChironex leckeri movementwillbespurredandalsooncethenutri‐tionavailabilityisextraordinaryinthatlocationevery Chironex leckeriwillmoveintheswarm.Because ofoceancurrentsofferabanquetatthattimethe Chironex leckeriwillconverge.
Unsurprisingly,theoceancurrentsdirectmore quantityofnutritionwillbethereandChironex leck‐erifascinated(sg)towardsthat.Scienti icallyitcanbe demarcatedas,
InallmagnitudesofChironex leckerimaintaina distanceof ±���� (postulate)inastandardthree‐dimensionalruckusmode(��−����������������������������������).
Chironexfleckeri
Chironexfleckeri
Rf (0,1)���� (0,1)�� (37)
R(0,1
Chironexfleckeri
EveryChironex leckerifreshpositioniscomputedby,
(41)
PrimarilymostoftheChironex leckerimoveactively butinconcludingstagesitmovestopassivemethod. OncetheChironex leckeripassesinactivemanner thenthepositionisdemarcatedas,
������→differencebetweenpresent andaveragepositionofallChironex leckeri
ThecrusadeoftheChironex leckerimaybedueto oceancurrentsanditwill indpassageinsidethe swarm.Inthispaperapoint–in‐timedealingsystem hasbeenpremeditatedtoruletheswitchingbetween themovements.LogicallyChironex leckeripassagein thedirectionoftheaccessibilityofnutritionisextraor‐dinary.Perceptiblythemagnetismtowardscompact‐nessofthenutritionplaceishighandthecrusadeof allChironex leckeriwill lowtowardsthatposition. Placeandanalogousobjectivefunctionwilldescribe theamountofthenutrition.
Then ⃗ ����isdeterminedby,
Chironexfleckeri
∗ ����presentbestpositionofChironexfleckeri, ��������indicatesthenumberofChironexfleckeri ������averagelocationofChironexfleckeri (35)
MathematicaldesignforthepassivecrusadeofChi‐ronex leckeriispremeditated.Itisgroundedonthe crusadeoftheChironex leckeriinthedirectionof thenutritionobtainability.OnceaChironex leckeri passesfromapositioninthedirectionofanother positioninacertainhabitationatthatmomentthere willberepositionoftheChironex leckeriandthis aspectimitatesthelocalexploration.Inthissegment exploitationhasbeenaccomplished.Thedrive(p) de inedas,
ThedriveoftheChironex leckeriinactive,passiveand throughoutoceanstreamsisorganizedbyanideain timehandlingorganization(THO).
Logisticchaotic[5]equationforthepopulaceinitial‐izationisdemarcatedas,
����+1 =������ (1−����),0≤��0 ≤1;��0 ∈(0,1); ��0 ∉{0.00,0.25,0.75,0.5,1.0};��=4.00 (49)
LimitsettingsaredemarcatedfortheChironex leck‐eri,eversinceassoonastheypassageyonderthelimit thenithastobindbacktothemargin.
21) ���� (��+1)=���� (��)+��×��(0,1)×(������−������);��− ����
22) OtherwiseChironex leckeriinpassivemovement
23) IdentifytheChironex leckeridirection
24) ⃗ ������= ���� (��)−���� (��) ������(����)≥�� ���� ���� (��)−���� (��) ������(����)<�� ����
25) FindtheFreshpositionofChironex leckeri
26) ���� (��+1)=���� (��)+ ⃗ ������
27) Endif
28) Endif
29) Limitconditionsaretested
1) Start
2) Initializationofparameters
3) Explorationspace,extremenumberofiterations andpopulationsizearepre ixed
4) PopulationofChironex leckeriinitializedby applyinglogisticchaoticmap
5) ���� ��=1,2,3,…,��������
6) Nutritionvolumeiscomputed– ����;i.e.��(����)
7) PositionoftheChironex leckerirecognisedwith nutritionobtainability(��∗)
8) ��������∶��=1
9) Repeat
10) ��������=1;������������
11) Calculationoftimehandlingorganization(THO).
12) {����������
��������=1;��������������������������
��������������timehandlingorganization(THO)
����������≥0.50;��ℎ����Chironexfleckeri followoceanstream
����ℎ������������Chironexfleckeri moveintotheswarm
������(0,1)>(1−������);��ℎ���� Chironexfleckeriisinactivemovemnet
����ℎ������������Chironexfleckeriisin passivemovemnet
13) ����������≥0.50;��ℎ����Chironexfleckeri followoceanstream
14) DetermineOceanstream
15) ⃗ ����=��∗ −��×��(0,1)×��
16) FreshpositionofChironex leckeriisdetermined
17) ���� (��+1)=���� (��)+��(0,1)×��∗ −��×��(0,1)× ��;��−������
18) OtherwiseChironex leckeripassageintoswarm
19) ������(0,1)>(1−������);��ℎ����Chironex leckeriis inactivemovement
20) Freshpositionisdetermined
30) Infreshpositionamountofnutritionchecked
31) PositionoftheChironex leckeri(����)rationalized
32) PositionoftheChironex leckeriwhichownplenti‐fulnutrition(��∗)
33) Endfori
34) ��=��+1
35) ��>��������������
36) ��������������ℎ������������������������
37) End
Intherealtimeworldageneralpractitionerwill treatassickpersonwithvariousprocedures.Thispro‐cesshasbeenimitatedtomodeltheprojectedgeneral practitioner–sickperson(PS)optimizationalgorithm algorithm.Ingeneralpeoplewillbeinoculatedand thenwithrespecttodisorderanddisease,–medical treatmentwillbegivenbymedicines[4].Atutmost anoperationonthesickpersonwillbedonewhich completelydependsontheconditions.Inoculation, medicine,andoperationaretheproceduresthathave beenconsideredasthephasesoftheprojectedPS algorithm.
Populationiscreatedonthebasisofnumbersof thesickpersontreatedbythegeneralpractitionerand mathematicallyde inedasfollows,
Where“V”isthesickperson’spopulation,“Oand“N” arethenumberofvariablesandsickperson.Inocula‐tion,medicine,andoperationaretheprocessesthat havebeenconsideredasthephasesoftheprojected PSalgorithm.
(54)
=��(����������������(������������������������)) (55)
�������� =��������������(��������������) (56)
�������� =��(����������������(����������������������)) (57)
������������ and���������� arethepositionoftheSickperson Inthe irstphasepeoplearegetInoculationandit mathematicallyformulatedasfollows,
8) Modernizethevalueof
Withrespecttodisorderanddisease–treatmentwill begivenbymedicinesanditformulatedasfollows,
9) ��������=1;��
10) Modernizethevalueof������������������������������������������
11) Modernize��
12) Modernize
(60)
(61)
WhentheconditionoftheSickpersonisverystern, thentheGeneralpractitionerwillmovetowardsthe performanceoftheoperationandithasbeenmathe‐maticallyde inedasfollows,
13) Modernize����
1) Begin
2) Determinethevaluesfortheparameters
3) Preliminarypopulationofsickpersonengendered
4) ������������������������=1;��������������������������������
5) Computethe itnessvalue
6) Modernizethevalueof��������������
7) Modernizethevalueof
15) ��������������ℎ�������������������������������������������� 16) End
Atlanticbluemarlin(ABM)optimizationalgo‐rithm,Boopsoptimization(BO)algorithm,Chironex leckerisearchoptimization(CSO)algorithm,general practitioner–sickperson(PS)optimizationalgorith‐marevalidatedinIEEE57bussystem[13].Table 1 showsthefactualpowerloss(FLO(MW)),Voltage deviation(VDT(PU))andVoltagestability(VSL(PU)). Figures5–7showtheassessmentofFLO,VDTandVSL.
Table1. AssessmentofParameters(IEEE57Bus)
Figure5. AssessmentofFLO(MW)(IEEE57bus)
Figure6. AssessmentofVDT(PU)(IEEE57bus)
Atlanticbluemarlin(ABM)optimizationalgo‐rithm,Boopsoptimization(BO)algorithm,Chironex leckerisearchoptimization(CSO)algorithm,general practitioner‐sickperson(PS)optimizationalgorithm arevalidatedinIEEE300bussystem.Table2shows thefactualpowerlossandvoltagedevianceassess‐mentforIEEE300bussystem.Figures8and9show theevaluationofassessment.
Figure7. AssessmentofVSL(PU)(IEEE57bus)
Table2. OutcomeAssessment(IEEE300BUS)
Figure8. AssessmentofFLO(MW)(IEEE300bus)
Atlanticbluemarlin(ABM)optimizationalgo‐rithm,Boopsoptimization(BO)algorithm,Chironex leckerisearchoptimization(CSO)algorithm,general practitioner–sickperson(PS)optimizationalgorithm arevalidatedinEgyptianGridsystem(WDSTN)220 KV[15].Table3andFigures10,11showthevaluation. Table 4 andFigure 12 showthetimetakenbyAt‐lanticbluemarlin(ABM)optimizationalgorithm, Boopsoptimization(BO)algorithm,Chironex leck‐erisearchOptimization(CSO)algorithmandgeneral practitioner–sickperson(PS)optimizationalgo‐rithm.
Table4. TimeTakenByABM,BO,CSO,PS
Figure9. AssessmentofVDT(PU)(IEEE300bus)
Table3. ValuationofParameters(WDSTN)220KV
Method FLO(MW) VDT(PU) PEPSO[14] 32.31 0.58
33.87 0.63
30.78 0.67
28.09 0.53
27.12 0.51
28.09 0.53
27.12 0.51
Figure10. AssessmentofFLO(MW)(220KV)
Figure11. AssessmentofVDT(PU)(220KV)
Figure12. TimetakenbyABM,BO,CSO,PS
Atlanticbluemarlin(ABM)optimizationalgo‐rithm,Boopsoptimization(BO)algorithm,Chironex leckerisearchoptimization(CSO)algorithm,general practitioner–sickperson(PS)optimizationalgorithm solvedtheproblemcompetently.Truepowerlossless‐ening,powerdivergencecurtailing,andpowercon‐stancyindexaugmentationhasbeenattained.Natu‐ralactionsofAtlanticbluemarlinareemulatedto designtheAtlanticbluemarlinoptimizationalgo‐rithm.Intermittentlygrandersolutionscanbemis‐placedwhilestreamliningthelocationofexamination representativesandfreshlocationsmaybemoremea‐gerthantheprecedinglocations,sogranderselection islinked.Boopspossesstheobligingstalkingphysiog‐nomies.Asaclustertheystalkthequarrybyforming thekeyandsubordinatebunches.CSOisbasedonthe driveandsearchbehaviourofChironex leckeri.The crusadeoftheChironex leckerimaybeduetoocean currentsanditwillpassinsidetheswarm.General practitionerwilltreatthesickpersonwithvarious procedures;thisprocessithasbeenimitatedtomodel theprojectedPSalgorithm.Theoperationonthesick personwillbedone,completelydependingonthecon‐ditions.Inoculation,medicineandoperationarethe proceduresthathavebeenconsideredasthephases oftheprojectedPSalgorithm.
Atlanticbluemarlin(ABM)optimizationalgo‐rithm,Boopsoptimization(BO)algorithm,Chironex leckerisearchoptimization(CSO)algorithm,general practitioner–sickperson(PS)optimizationalgorithm arevalidatedinIEEE57,300systemsand220KV network.
Factualpowerlosslessening,powerdivergence restraining,andpowerconstancyindexampli ication hasbeenattained.
FutureScopeofWork
Infuture,projectedalgorithmscanbeappliedto solvetotherengineeringproblems.Incancerdiagno‐sisthepresentedalgorithmscanbeappliedtodetect cancerinanearlystage.
AUTHOR LeninKanagasabai∗ –PrasadV.PotluriSiddhartha InstituteofTechnology,ChalasaniNagar,Kanuru, Vijayawada,AndhraPradesh,520007,India,e‐mail: gklenin@gmail.com.
∗Correspondingauthor
References
[1] C.P.Goodyearetal.,“Verticalhabitatuseof AtlanticbluemarlinMakairanigricans:inter‐actionwithpelagiclonglinegear,” Mar.Ecol. Prog.Ser., vol.365,pp.233–245,2008.Doi: 10.3354/meps07505
[2] A.T.Dahel,M.Rachedi,M.Tahri,N.Benchikh, A.Diaf,andA.B.Djebar,“Fisheriesstatusofthe bogueBoopsboops(Linnaeus,1758)inAlgerian EastCoast(WesternMediterraneanSea),” Egypt. J.Aquat.Biol.Fish.,vol.23,no.4,pp.577–589, 2019.Doi:10.3354/meps07505
[3] M.Pionteketal.,“ThepathologyofChironex leckerivenomandknownbiologicalmecha‐nisms,” ToxiconX, vol.6,no.100026,p.100026, 2020.Doi:10.1016/j.toxcx.2020.100026
[4] R.A.Damarell,D.D.Morgan,andJ.J.Tie‐man,“Generalpractitionerstrategiesforman‐agingpatientswithmultimorbidity:asystem‐aticreviewandthematicsynthesisofqualitative research,” BMCFam.Pract., vol.21,no.1,2020. Doi:10.1186/s12875‐020‐01197‐8
[5] K.Lenin,”QuasiOpposition‐BasedQuantum PierisRapaeandParametricCurveSearch OptimizationforRealPowerLossReductionand StabilityEnhancement,” IEEETransactions onIndustryApplications,vol.59,no. 3,pp.3077‐3085,May‐June2023Doi: 10.1109/TIA.2023.3249147.
[6] K.Nagarajan,“Multi‐objectiveoptimalreactive powerdispatchusingLevyInteriorSearchAlgo‐rithm,” Int.J.Electr.Eng.Inform.,vol.12,no.3,pp. 547–570,2020DOI:10.15676/ijeei.2020.12.3.8
[7] R.NgShinMei,M.H.Sulaiman,Z.Mustaffa, andH.Daniyal,“Optimalreactivepower dispatchsolutionbylossminimizationusing moth‐ lameoptimizationtechnique,” Appl. SoftComput.,vol.59,pp.210–222,2017.Doi: 10.1016/j.asoc.2017.05.057
[8] K.Nuaekaew,P.Artrit,N.Pholdee,and S.Bureerat,“Optimalreactivepower dispatchproblemusingatwo‐archive multi‐objectivegreywolfoptimizer,” Expert Syst.Appl.,vol.87,pp.79–89,2017.Doi: 10.1016/j.eswa.2017.06.009
[9] A.H.KhazaliandM.Kalantar,“Optimal reactivepowerdispatchbasedonharmony searchalgorithm,”Int.J. Electr.PowerEnergy Syst.,vol.33,no.3,pp.684–692,2011Doi: 10.1016/j.ijepes.2010.11.018
[10] C.Gonggui,L.Lilan,G.Yanyan,H.Shanwai,“Multi‐objectiveenhancedPSOalgorithmforoptimizing powerlossesandvoltagedeviationinpowersys‐tems,“COMPEL,Vol.35No.1,pp.350‐372,2016. Doi:10.1108/compel‐02‐2015‐0030
[11] AnilKumar,ArunaJeyanthy,Devaraj,“Hybrid CAC‐DEinoptimalreactivepowerdispatch (ORPD)forrenewableenergycostreduction,” SustainableComputing:Informaticsand Systems, Volume35,2022,100688,Doi: 10.1016/j.suscom.2022.100688.
[12] Abd‐ElWahab,Kamel,Hassan,Mosaad, AbdulFattah,“OptimalReactivePowerDispatch UsingaChaoticTurbulentFlowofWater‐Based OptimizationAlgorithm,” Mathematics. 2022; 10(3):346.Doi:10.3390/math10030346
[13] TheIEEE57‐BusTestSystem[online],available athttp://www.ee.washington.edu/research/p stca/pf57/pg_tca57bus.htm.
[14] M.T.Mouwa i,A.A.A.El‐Ela,R.A.El‐Sehiemy, andW.K.Al‐Zahar,“Techno‐economicbased staticanddynamictransmissionnetworkexpan‐sionplanningusingimprovedbinarybatalgo‐rithm,”Alex.Eng.J.,vol.61,no.2,pp.1383–1401, 2022.Doi:10.1016/j.aej.2021.06.021.
[15] A.A.El‐Ela,M.Mouwa i,andW.Al‐Zahar,“Opti‐maltransmissionsystemexpansionisplanning viabinarybatalgorithm,”in201921stInt.Mid. EastPowerSys.Conf,(MEPCON),2019.Doi: 10.1109/MEPCON47431.2019.9008022.
NETWORKOPTIMIZATIONUSINGREALTIMEPOLLINGSERVICEWITHAND
Submitted:20th April2023;accepted:22nd May2023
MubeenAhmedKhan,AwanitKumar,KailashChandraBandhu
DOI:10.14313/JAMRIS/3‐2024/26
Abstract:
IEEE802.16canbeseenasacompellingreplacement forconventionalbroadbandtechnologiesbecauseits primarygoalistoprovideBroadbandWirelessAccess (BWA).Thevariableanduncertainnatureofwireless networksmakesitmuchmorechallengingtoensureQoS inthisnetwork.WiMAXTechnologyisusedtosupport variousqualityofserviceswhichincludesUGS,rtps,nrtps, ertps,andBestEffort.ThisstudyemploysanIEEE802.16 networksimulator,whichoffersadaptableandreliable featuresforassessingaparticularQoSparametersfor rtps.Achievingbetterinternetperformanceinrealtime servicesiscurrentlyachallenge,anditisinneedofa presentscenario.Thisworkemphasizedbetterinternet service,withgoodqualityofserviceusingrtpswithRelay StationandWithoutRelayStation.InthisworktheCBR packetsize,CBRdatarate,anddataratewithrtpsservice arefine‐tunedforachievingbetterperformancewith goodqualityofservice.Whencomparinguplinkconnec‐tionsinrtpswithandwithoutrelaystation,itisfound thatthethroughputintheuplinkis200%greaterwhen usingarelaystation.Thethroughputandgoodputare evaluatedinuploadinganddownloadingwithsingleand multiplesubscriberstationsandweobservedthatthe multiplesubscriberstationsindownloadinggivebetter performance,ascomparedtosinglesubscriberstations. Thethroughputandgoodputinsinglesubscriberstations isbetterthanmultiplesubscriberstationsinuploading. Theacademicresearchersandcommercialdevelopers canusethisanalysistovalidatedifferentWiMAXNet‐workimplementationmechanismsandparameters.
Keywords: WiMAX,realtimepollingservice,QoS,relay station,withoutrelaystation
Inordertooffereffectivetransmissionservices, aWorldwideInteroperabilityforMicrowaveAccess (WiMAX)[1]networkmakesuseofthesamemedium asothernetworks.Examplesofwirelessnetworksthat cansharewireless ilesincludepoint‐to‐multipoint (PMP)andmeshtopologies.Inapoint‐to‐multipoint modegroundstation,manyusers’stationsarecon‐nectedviaadownlinkconnection.Subscriberstations (SSs)receivethesametransmission,oraportionof it,overaparticularfrequencychannelandwithinthe basestation’s(BS)areaofreception.
Theonlytransmitterthatoperatesinthiswayis theBS.Asaresult,itbroadcastswithoutrequiringany stationcoordination.Informationtransmissiontakes placeoverthedownlink.SSssplitthetransmissionto theBSbasedondemand.Differentservicesarepro‐videdtothesubscriberstationfromthebasestation basedondifferentqualityofservicesandtherequests arrivingatbasestations.Servicessuchasbroadcast, unicast,ormulticastarehandledbybasestationsin theformofmessagesandsometimesaredirectedto speci icsubscriberstationstoo.Foreverysector,the mediaaccesscontrollayeranditsassociatedalgo‐rithmsgovernseachsubscriberstation.Thislayer isalsoresponsibleforhandlingotherfunctionalities suchasdelay,bandwidth,andotherrelatedapplica‐tions.Variousothertypesofservices,suchasunso‐licitedbandwidthgrants,pollingandbandwidthshar‐ing,anduplinksharingarealsohandledbytheselay‐ers.Allthisishandledinconnection‐orientedservices inwhichresponseisalsoimportant.TheMAClayer usesaconnection‐orientedtransmissionalgorithm.In theframeworkofaconnection,alldatacommunica‐tionsarede ined.Service lowsarecreatedatsub‐scriberstations,eachhavingadifferentservice low; adifferentbandwidthisalsoassociatedwitheach connection.Differentqualityofserviceusesdifferent packetdataoneachtypeofconnection.MACprotocol isbasedontheideaofdifferenttypesofservice lows andtypeofconnections.Wheneverbandwidthisallo‐cated,eachtypeofservice lowhasadifferentmethod fordatatransferforbothuplinkanddownlinkcon‐nectionsofQoS.Eachtimeaconnectionisestablished, anSSseeksuplinkbandwidth.Accordingtoeachcon‐nection,anSSseeksuplinkbandwidth.Wheneverany requestarrivesatabasestation,thenonthebasisof requestabandwidthisallocatedtoeachsubscriber station.Alltheactiveconnectionsarekeptupuntil theyarenotsatis iedfromthebasestation.Generally, threetypesofconnectionsareusedinWiMAXnet‐works,whichincludestaticcon igurationsincluding dynamicadditionofnodes,modi icationofconnec‐tions,anddeletionofconnectionsinthenetwork.The basestationandsubscriberstationcommonlytrigger connectionendsbetweeneachother.
Real‐timeservicechannels,likeMPEGvideo,that periodicallyproducevariable‐sizedatapacketsare supportedbytherealtimepollingservice(RTPS).
Serviceslikerealtime,unicast,andrecurring, whichsatis iesthe lowonthebasisofservices,are alsograntedonthebasisofrequestandasperthe desiredsize.Theattemptisalwaystoprovidethebest datatransportservicewithef iciencyalongwiththe variablegrantsizes,butthismayrequiremorerequest overheadthanUGS.Foreffectivecommunicationsin thenetwork,thebasestationperiodicallyoffersuni‐castrequestfordatatransfer.Andforproperopera‐tionsbetweenthegroundstationanduserstation,no contentionrequestisofferedfromtheuserstationfor thelinkonthoseservices.Incasetherequestisnot ful illed,aunicastrequestisprovidedbytheBSfor therequestopportunitiesasrequiredbythisservice. Toacquiretransmissioninuplinkopportunitiesas aresult,theuserstationonlyusesunicastrequest opportunities.Thepolicyforrequestandtransmis‐sionpolicyshouldbeinaccordancewithnetworkpol‐icybecauseithasnobearingonhowthisscheduling serviceactuallyworks.Themainissueswhichneedto beeffectivelyhandledbyanynetworkincludehighest sustainablerateoftraf ic,prede inedrateoftraf ic, maximumlatency,andtransmissionpolicyrequests.
IEEE802.16isthelatesttechnologyusingthelat‐esthardwareandstructures,whichisapplicablefor upcomingtechnologiestoo.Thistechnologyissup‐portedbyvarioustoolssuchasQualnet,Simulink, andNetworksimulators.InordertoevaluateIEEE 802.16standardsusingtheNS‐2simulator,thispaper providesde‐factostandardsfortheWEIRTproject.In ordertocarryoutsomespecialissuesthatarecrucial forconductingtrustworthyresearchbasedonthese toolsinrealisticscenarios,thisarticleprovidessome generalissuesbasedonresearchbasedonthistool. Thisstudydemonstratesthathardwarefrequently onlypartlycomplieswithstandards.ItemploysNS2 simulationstodisplayreal‐worldsituations.Aproject calledWEIRD,whichsupportsNS‐2IEEE802.16,can alsobene itfromsuchstudy.Thisessaydiscussesthe concernsnecessarytoconducttrustworthyNS2tool‐basedresearch[2].
Thedepictedworkinthispaperisbasedon lexible bandwidthallocationandQualityofServices(QoS) schemesofIEEE802.16MAClayerforclientswith differentrequirements.InrealscenariosQoSisdepen‐dentonusersinwhichtheycancreateormodify, updatedaspertheneeds.Thispapergivesanuplink scheduler,whichisusedbyRTPSinWiMAXnetworks. Aleakybucketisproposedinthiswork,inwhichRTPS connectionsforschedulingusesthetechniquefortraf‐icmanagementandforuplinkconnectionmanage‐menttoo.SimulationofthisworkisdoneontheMAT‐LABtoolinwhichthroughputandfairnessareidenti‐ied.Inthisstudy,anuplinkscheduleforWiMAXbase stations’connectionstoreal‐timepollingservicesis proposed.For lexiblebandwidthdistribution,IEEE 802.16MACpresentsthemajorityofthese.
Inordertohandleuplinktraf ic,itissuggestedin thispaperthatthegroundstationkeepaleakybucket foreachRTPSconnection.Thesuggestedscenario wascreatedusingMATLABandaddressedissueswith throughputandfairness[3].
Fourclassi icationsoftraf icinQoSareprovided byIEEEinWiMAXnetworksbythestandards.Each classhastheirownbandwidthrequirements,which needtobemanagedbyqualityofservice(QoS)stan‐dards.Thisworkisdonebyusingthreetypesofcon‐nections,includingUGS(unsolicitedgrantservice), NRTPS(nonrealtimepollingservice)andRTPSfor calculatingperformance.Onthebasisofclassofqual‐ityofservices,differentlevelsofpriorityareassigned. Afterthatananalysismodeisproposedwhichgives admissioncontrolforeachtypeofqualityofservices. Thearticlesuggestsandkeepsaleakybucketfor eachRTPSconnectiontomanageuplinktraf icand scheduleRTPStraf ic.InMATLABsimulations,the suggestedschedulerisexamined,anditsthroughput andfairnesscharacteristicsareshown[4].
Increasingmobileapplicationhasgrownupwith broadbandwirelessaccess(BWA)whichenhances mobilityandneedfordataservicesatalltimesin mobileapplications.Bestservicesformobiledatause newstandardsofIEEE802.16ewhichareavailablefor qualityexperiencesforusers.SinceWiMAXnetworks enableanumberofcharacteristicsofwirelessLANs, soamediumaccesscontrollayeronthebasisofthese characteristicsensuresvideo,data,andvoiceservices bytheMAClayer.Allocatingresourcestocustomers inawaythatsatis iesallqualityrequirementslike delay,jitter,andthroughputisacrucialservice.Most ofthetechniquesde inedbyIEEEareleftfreesothat userscanimplementthemontheirown.Oneimpor‐tantaspectisscheduling,whichisneededtoimple‐mentdifferentiations.Thisworkisgiventodesign‐ingthosefactorsneededforscheduling.Thisdocu‐mentprovidesanoverviewofcurrentchannel‐based schedulingmethods.Inordertoimproveoutputwhile usinglessenergy,thisarticlepresentsanalgorithm withafeasiblelevelofcomplexityandscalability.The workexaminesthecentralconcernsanddeterminants ofscheduledesign.Thisarticleprovidesathorough overviewofcontemporaryschedulingmethodologies. Recentstudiesareusedasthefoundationforan extremesurveythatclassi iesthesuggestedmecha‐nismaccordingtochannelconditions.Thispaperout‐linesthebestuseofresourcestoguaranteeservice qualityandgreaterthroughputwhileconsumingless powerandmaintainingmanageablealgorithmcom‐plexityandsystemscalability[5].
ThisworkisdiscussedabouttheQoSdeployment overcellularWiMAXnetwork.Onthebasisofdeliv‐eries,twoqualityofserviceUGSandERTPSaredis‐cussedinthispaperintermsofdelivery.Thispaper looksatinstancesoftraf icrisingbeyondanominal rateor luctuatingmorethanbeyondnominalrate;we lookatthepossibilityofrevertingthefreebandwidth outofreserve[6].
Thisworkprovidesanoveldownlinkschedul‐ingschemethattakesintoaccountthethroughput requirementsfordelays,fairnessoptimizationswith regardtoNRTPS,andbestefforttomeettheideal QoSrequirementwithoutusingexcessiveamounts ofresources.Thegoalofthisworkistoaccomplish thebestQoSrequirementwithoutconsumingexces‐siveamountsofresourcesbyproposingadownlink schedulingschemethattakesintoaccountthedelay requirementsofRTPSconnectionsrelativetothevar‐iousNRTPSandBEconnections[7].
TheWiMAXOFDMdownlinksubframeusesatwo‐dimensionalchanneltimestructure,whichresultsin additionalcontroloverheadsanddecreasednetwork ef iciency.Theef iciencyofthenetworkisincreased byconductingnumerousteststodeterminethedesign issuesofMAClayerschedulerortheburstallocationin thephysicallayer.APUSCmodelissupportedinthis work,whichidenti iescrosslayerframeworkusing aschedulerandaburstallocator.Thedatatraf ic issueisresolvedbyresourceallocationbyburstallo‐cator;also,theschedulercaneffectivelyutilizethe frameareaandcutdownonIEoverheads.Maintain‐inglong‐termfairness,reducingcurrenttraf icdelays, andimprovingframeutilizationallimprovenetwork speed[8].
QualityofExperience(QoE)isusedasabasemet‐ricinthiswork,whichsuggestswaystoenhancethe capacityofuploadingtraf icinsatellitecommunica‐tionandWiMAXnetworksintheschedulingalgo‐rithm.TheFC‐MDI(FrameClassi ication‐MediaDeliv‐eryIndex)isusedintheschedulingalgorithmfor real‐timeconnection.Thealgorithmisassessedintwo differentiterations.Theresultshowstheperformance oftheWiMAXnetwork,whichincreasesthedelayand qualityofexperienceinreal‐timeconnections[9].
QualityofserviceinWiMAXnetworksisanimpor‐tantconsiderationforvariousapplicationssupported bywirelesscommunications.Alltheservicesused forwirelessbroadbandnetworkscanpresentachal‐lenge,sothatservicesofvideo,audiovoice,anddata couldbeenhancedandimproved.Animportantchal‐lengeofwirelessservicesisitsunpredictableand variablerequirements,whichmakesitcomplexto applyinnature.Duringthetransmissionofvideoand voiceservices,allocationofavailableQoScriterialike delay,throughput,andjitterareusedtomaximize thegoodputandminimizepowerconsumptionwith suitablealgorithmssoastogivescalableandfeasi‐bleservices.WiMAXnetworksproposequalityofser‐viceguaranteesbyusingvariousmechanismsatMAC layer,includingschedulingandadmission.Thisalso includespacketschedulinginresolvingcontentionfor bandwidthamongusersandtodotransmissioninan orderedmanner.Foreffectivetransmissionvarious classi icationsintermsofschedulingalgorithmsare proposed,includinghomogenousalgorithms,hybrid algorithms,aswellasopportunisticschedulingalgo‐rithms.Thispapergivesperformancemetricsfor developingtheschedulerforWiMAXnetworks.
Thispaperalsogivestheimprovementsassoci‐atedwithuplinkscheduling.Numerousscheduling algorithmclassi ications,includinghomogenousalgo‐rithms,hybridalgorithms,andopportunisticschedul‐ingalgorithms,aresuggestedfortransmissioninan ef icientway.Thispaperprovidesef iciencymetrics forcreatingtheWiMAXnetworkschedule.Thisarti‐clealsodiscussestheadvantagesofuplinkschedul‐ing[10].
RelayinginWiMAXnetworks,anemergingtopic inrecentyearsthatalsocoversmobilemulti‐hop relaying,iscoveredinthiswork.At irst,itwasonly consideredtheoretically,butnowthatitispracti‐callyfeasible,signi icantresearchisbeingdoneinthis ield.Thisarticlediscussestheschedulingchallenge facedbymulti‐hoprelaynetworksusedinOFDM.For user‐speci icservicesthatrequiretheallocationof bandwidthataspeci icmomentonaspeci icchan‐nel,schedulinginsuchsystemsisasigni icantissue. Accordingtofairnessrequirements,theauthorofthis papersuggestedthe“Eliminaterepeat”algorithmto addressrelayissuesinWiMAXnetwork’scurrentsys‐tems.Bysuggestinga“ServicePrioritizedOpportunis‐ticSchedulingAlgorithm,”theissueisresolved.byallo‐catingbandwidthbasedonthedifferentiatingband‐widthneededbytheuser,whichdecreasesthedelay andproblemsofstarvationinthenetworks[11].
Thejobhereisprimarilyconcernedwithinstal‐lationcoststhatareonatightbudgetandnetwork performanceissues.Usersrequiremorecoveragein ordertoensureeffectiveradioenhancementsanddata rates.RelaystationsareusedinWiMAXnetworksfor networkoptimizationinmobilemulti‐hopnetworks. TheIEEE802.16forumprovidesvariousservice lows (suchasUGS,RTPS,ERTPS,NRTPS,andBE)forvar‐ioususes.Thereplacingofthebasestationfromthe relaystationsatthebestpossiblelocationsbecame costeffectiveandenhancedthecoverage.Thiswork depictsaspectsofthenetworkqualityandcoverage enhancementsforruralandhillyareaswherecon ig‐uringmanybasestationsisstillanissue[12].
Theeffortprovidesbettersupportfordata,video, andsoundservices.Thisstudyaimstosatisfynetwork designforqualityofservice[13].
Amultipathchannelmodelisproposedinthis work,whichincludesbandwidthoverthestartrajec‐tory.Fourcellscenariosareconsideredinthiswork inWiMAXnetworks.Inthisproposedwork,everycell hasonesubscriberstationandonebasestation.VoIP codecperformanceisevaluatedintermsofthrough‐putandMOSinthiswork.Thewholeworkisanalyzed inOPNET‐14.5.Abetteroutcomeisobservedusing themultipathchannelmodel(disabled)thanwhen usingtheITUpedestrianmodelproposedinthiswork. ThisanalysisshowsthatMOSvalueforthemultipath channelmodelwithdisabletypeissuperiorforITU PedestrianType[14].
Thetopologyandbandwidthofanetworkaffect itsperformance,andthemajorityofresearcherswork toreachhighperformanceinthemostef icientman‐nerpossible.Thisarticlegivesabetterscheduling algorithmforchannelreuseandnetworkperformance basedonthedesignandtransmissionrequestsinsub‐scriberstations’uplinkconnectionrequests.
Thispapergivesimprovementsforthroughputby reducingtransmissiondelaysinmeshnetworktopol‐ogy[15,16].
VoiceservicestandardsofIEEE802.16e‐2005are speci icallydesignedforextendedrealtimepolling services.Forbetterandoptimizedresultsintermsof adaptivemodulation,andtomaximizetransmissions, thisadaptiveandcodingmethodgivesvariablerates inaccordingtousers’timevaryingchannelconditions. Thisstudygivestheideaofcelldivisionintwozones withdistinctaverageSNRs,eachwithsingletrans‐missionmodes.Thispaperproposeda3‐dimensional MarkovprocessofM/G/1tomaximizepairofadmis‐sibleVOIPusersforsteadystateprobabilityandprob‐abilitydistributions[17].
Inthisstudy,QoSisdeployedinWiMAXnet‐worksforwirelesscellularnetworks.Theperfor‐manceachievedwithdifferentQoScon igurationsfor VoIPtraf icdelivery,suchasUGSorertPS,arecom‐paredinthispaper.Theconclusionofthispaper demonstratesthatthetransmissionofBEtraf icis startedifdelay‐sensitivetraf ic luctuatesbeyondits usualratefromitsERTPSreservedbandwidth[18].
Thisworkcomparedtheperformanceevalua‐tionofdifferenttechnologieslikeWi‐Fi,WiMAX,and UMTS.Testingisdonebasedonmodulationand channelbandwidthtechniques.Performanceofnet‐workcongestionisidenti iedusingnetworksim‐ulationtoolstoevaluatetheresults.Theobtained results,basedondifferentdatarates,verticalhan‐dover,anddifferenttechnologies,offerdifferentser‐vicesforbandwidthallocations[19].
Thefollowingarticleintroducesanoveluplink algorithmcalledInstantaneouslyReplacingAlgorithm (IRA),whichmakesuseoftheNS‐2simulationmodel. Theresultsofthisworkrepresentthatthequality ofserviceisincreasedduetothedelayreduction andnetworkresourcesfairlyusedbysubscribersta‐tionstomaintainthethroughputusingSNRbased approaches[20].
Wirelessnetworks’limitedresourcesandtime‐varyingchannelcircumstancespresentdif icultiesfor real‐timevideostreaming.Wirelesschannelcircum‐stancesthatchangeovertimecausevideopackets tobelostordelayedundercurrentcircumstances. Streamingisencodedanddelivereddependingon howlongitwillbeplayedback.Losingbaselayerpack‐ets,particularlyinerror‐pronenetworkslikewire‐lessnetworks,canhaveasigni icantimpactonthe transmittedvideoqualityandoccasionallycausean interruption.Thispaperisbasedonbehaviorofreal timepublishedsubscriber‐basedmiddleware.
Theperformanceoftheproposedmethodisshown inIEEE802.11gWLANnetworks.Thispapergivesa demonstrationofgoodvideoqualitybystreamand stablevideofreefromobviouserrorsorinterrup‐tions[21].
ThisworkproposesacasestudyofWiMAXnet‐workinterconnectionsthataresupportedonMPLS core.Also,theadvantagesandbene itsintermsoftraf‐ic,virtualprivatenetworksandDiffservtechnologies arestudied.Wholeanalysisisdoneusinganetwork OpnetsimulatorwithMPLS,MPLS‐TP,andGMPLS technologiesbasedontheircomparisonswithvalida‐tiononthesameinfrastructure[22].
InthisworkvariousparametersofWiMAXnet‐worksarementioned,whichincludelatencyvaria‐tionsbasedonapplicationruntime,libraryperfor‐mancesandpacketdelivery.Anetworklatencyinjec‐torisdesignedandproposedinthiswork,whichis suitableforthemajorityofQLogicandMellanoxIn ini‐Bandcards.Theresultsshowthattheperformance ishighlyaffectedwithnetworkupdating,changesin networkvariance,andmeannetworklatency[23].
Thecloud‐based,micro‐services‐basedarchitec‐tureoftoday’scontemporarybusinessapplications requiresa lexible,high‐performancenetworkinfras‐tructure.Operators’dependencyoncloudservice platformsisincreasing:forinstance,theOpenShift ContainerPlatformonZtoguaranteehighlyavailable andhigh‐performanceapplications.Forthesetypesof technologies,Openv‐Switch(OVS)technologiesare used.Animportantchallengeinnetworkingistohave asystemwithbestqualityservices;manyenhance‐mentsarestillpossibleinupcomingtechnologiestoo. In‐depthanalysesoftheOVSpipeline’seffectsand afewspeci icOVSproceduresareprovidedinthis paper.TheperformanceofvariousOVScon iguration systemsintheindustryisusedtoidentifyvarious situations.ThisstudydemonstratedhowwelltheOVS pipelineperformed,howitoperated,andwhatimpact ithad[24].
TheexistingsolutionbesidetheWiMAXare4G and5GLTE(LongTermEvolution)whichused theconceptofusercapacityincrementandsignal strengthenhancementtoincreasethecoveragearea byinstallingtheusercapacitysiteandsinglestrength incrementsite.
Thenetworkcon igurationusedinthisworkto evaluatetheeffectivenessoftheWiMAXrelaystation isdepictedinFigure1.Thenetworksetupconsistsof onebasestation,tworelaystations,andsubscriber stations.Thedatatransmissionismadedirectlyfrom basestationstosubscriberstationusingdirectTCP connection,andtheotherismadethroughrelaysta‐tionsforuploadingandviceversafordownloading. AscenarioisconstructedusingNS2andtheperfor‐manceisexaminedwithuplinkanddownlinkdata transferfrombasestationtosubscriberstationand viceversaalongwithvariousparameters[25].
AscenarioofRTPSserviceincludingbasestationandrelaystations
AbasestationusesTCPconnectionstosenddata tosubscriberstationsfordownlinktransmissionsand uplinkdatatransferscontainingtheacknowledgment. Inthisstudy,basestationtosubscriberstationdown‐linkTCPconnectionswithandwithoutrelayarecre‐ated,asshowninFigure1
Inthisworktheperformanceisanalyzedfor uploadinganddownloadingofdatafromsubscriber stationtobasestationandbasestationtosubscriber stationrespectivelyusingdirectTCPconnectionand viarelaystationTCPconnection.
Inthisscenariothesingleandmultiplesubscriber stationsareconsideredinuploadinganddownloading bothforthroughputandgoodputmeasurement.
PerformanceisanalyzedusinglightWiMAXsimu‐latorinwhichtwocasesareconsidered.Performance parametersareshowninTable1.
Table1. Simulationparameters
Parameters
Values
RoutingProtocols AODV
TransmissionControlProtocol UDP,TCP
SimulationPeriod 300Seconds
CBRPacketSize 200Bytes
CBRRate 5000000MilliSec
DataRate 1,2,3,10Sec
SimulationTime
Routingprotocolidenti iedtheshortestpath betweensourceanddestination.Inthisworkthebase stationandsubscriberstationsarethesenderandthe receiver.Italsofunctionsasanetworkswitchand employsself‐de inedprotocolsforinterstationcom‐munication.Theroutingalgorithmusedinthisstudy iscalledAd‐hocOnDemandDistanceVectorRouting (AODV),whichisusedtocreateroutesonlywhenthey arerequested.Topologyhastwodifferentkindsof situations.Thescenariousedinthiscaseallowspacket datatransferbetweensourceanddestination,whichis responsibleforend‐to‐enddelivery.Followingarethe de inedvaluesusedforsimulationstudy.
CBRPacketsize: Inter‐arrivalpacketsize
CBRRate: Constantbitrateisatermdescribingthe behaviorofaTCPtraf icgenerator.
DataRate: Thetimedurationinsecondsthedatais transmitted
Simulationduration: Thedurationofdatatrans‐mission.
QoS: Itincludesrealtimepollingserviceswhich includesaudio,videoandmultimediaservices.
Thethreemetricsareusedtoestimatetheperfor‐manceofnetwork. ‐ Throughput:Therawbytessentbetweenthesender andthereceiver
Figure2. ThroughputinuplinkwithandwithoutrelaystationinRTPS
Figure3. ThroughputindownlinkwithandwithoutrelaystationinRTPS
Figure4. GoodputinuplinkwithandwithoutrelaystationinRTPS
Figure5. GoodputindownlinkwithandwithoutrelaystationsinRTPS
‐ Goodput:Successfullyreceivedbytesatthedestina‐tion.
‐ PacketsDrop:PacketDrop:Totalpacketsdropped duringthecommunicationduration.
6.ResultsandDiscussions
Thissectionshowstheresultsobtainedbysim‐ulationandrepresentedusinggraphswithjusti ica‐tion.Theperformanceofwithrelaystationandwith‐outrelaystationismeasuredusingthroughputand goodputinuploadinganddownloadingboth.Also, theresultsshowtheperformancewithmultiplesub‐scriberstationsandsinglesubscriberstation.
Thethroughputwithrateinuplinktransmissions isshownwithandwithoutrelaystationinFigure 2 Theresultshowsthatthewithandwithoutrelaysta‐tionsprovidethebestuplinktransmissionthroughput of0.16Mbps,itisalsoobservedthatthethroughput obtainedwiththemultiplesubscriberstationsand singlesubscriberstationispoorerthanwithandwith‐outrelaystation.
Thegraphfurtherexaminestherelationship betweenrateandthroughput:thehigherthroughput isseenwhenusingarelayandwithoutrelaystation, andthisisbecausethechannelisbeingusedtoits fullestpotential.Throughputrisesasmorepackets cantraveloveragivendistanceinagivenamountof timeinbothsituationsofdatapackets.
Figure 3 depictsdownlinkthroughputwithand withoutrelaystations.Thegraphcomparesthefour scenarios:singlesubscriberstation,multiplesub‐scriberstations,withoutrelaystation,andwithrelay stations.Theresultshowsthatincaseofdownload‐ingconditions,highestthroughputisobtainedinwith multiplesubscriberstationsasrateincreases.The resultsalsoshowthataftermultiplesubscribersta‐tions,bestresultsareobtainedinwithandwith‐outrelaystationsindownlinkconnectionsasrate increases.Theperformanceisincreasedasrate increasesduetothehighestchannelutilizations.
Figure 4 depictsuplinkdatatransmissionsin termsofpacketsreceivedpersecond.Sincedata isreceiveddirectlyfrombasestationswithmax‐imumpowerandwithfullbandwidthutilization, itisobservedfromtheanalysisthathighergood‐putisobservedwithmultiplesubscriberstationsas rateincreases.Furthermore,itisalsoobservedthat onincreasingrategoodputwithoutrelaystationis observedsecondhighestoutputsthangoodputwith relaystationsandlastlyobservediningoodputwith onesubscriberstation.Asthetimeperiodincreases inallthefourcases,goodputisdiscoveredtohave increasedasaresultofincreasedratesincemaximum packetsarereceivedonincreasingrates.
Figure 5 showsthatincaseofdownlinkconnec‐tionsbestresultsareobservedincaseofmultiplesub‐scriberstationsasrateincreases.Afterthatisgoodput withoutrelaystationgivesbetterresultsthanwithone subscriberstations.Inallthefourcasesitisobserved thatasrateincreasesthenumberofpacketsreceived persecondalsoincreasesincaseofdownloadinglinks. Theanalysisdemonstratesthatasraterises,greater goodputisseenintermsofmultiplesubscribersta‐tions:0.05Mbpsfor1secondand0.43Mbpsfor 10seconds.Thisworkdepictsthatasrateincreases, goodputalsoincreases.
Bothuplinkanddownlinkconnectionsareusedto evaluatetheRTPSperformanceofWiMAXnetworksin thiswork.Whencomparingthisworkwiththeprevi‐ousresearchworks,itisobservedfromtheFigure 6 thatthethroughputincaseofwithrelaystationin uplinkconnectionsperformsmuchbetter,whichis observedtobe0.16Mbps.
Inallthescenarios,itisobservedthatuplink transmissionswithrelaystation,withoutrelaystation, withsinglesubscriberstationperformedmuchbetter thanthepreviousworksanddownlinkconnections withmultiplesubscriberstationsintheWiMAXnet‐works.Itisobservedfromtheanalysisthatevery timetherateincreasestheperformanceincreases. Withoutrelaystations,goodputinanuplinkcon‐nectionproducesbetteroutcomes.Incaseofdown‐linkconnections,withmultiplesubscriberstations goodputperformsbetter.Throughputandgoodput bothincreaseastherateincreases.Thecompara‐tiveresultsshowthattheproposedRTPSservice gives68%bettergoodputthan[6]and91.33%bet‐tergoodputthan[2],whileitshows90.66%bet‐tergoodputthan[16]and100%bettergoodput than[3].
Theresultsarecalculatedwithvariouscasescon‐sideringonesubscriberstationandmultiplesub‐scriberstationsbutnotlimitedtoRTPSonly.The resultscanbecarriedoutwithotherqualityofservice parametersusedinWiMAXnetworkslikeUGS(Unso‐licitedGrantService),ERTPS(ExtendedRealTime PollingService)andNRTPS(Non‐RealTimePolling Services)atthelaterstageofwork.Theresultscan becalculatedwithotherparameterslikecyclicpre ix andwithdifferentbandwidthallocationalgorithms. Thewholeanalysiscouldalsobeusedincaseof otherWiMAXparametersforbetterperformancein future.
AUTHORS
MubeenAhmedKhan –DepartmentofComputerSci‐enceandEngineering,SangamUniversity,Bhilwara, Rajasthan,India,e‐mail:makkhan0786@gmail.com.
AwanitKumar∗ –DepartmentofComputer ScienceandEngineering,SangamUniver‐sity,Bhilwara,Rajasthan,India,e‐mail: awanit.kumar@sangamuniversity.ac.in.
KailashChandraBandhu∗ –Department ComputerScienceandEngineering,Medi‐Caps University,Indore,MadhyaPradesh,India,e‐mail: kailashchandra.bandhu@gmail.com.
∗Correspondingauthor
References
[1] “IEEEStandardforLocalandMetropolitanArea NetworksPart16:AirInterfaceforFixedBroad‐bandWirelessAccessSystems,” IEEEStd802.162004(RevisionofIEEEStd802.16-2001).pp.0_1‐857,2004.doi:10.1109/IEEESTD.2004.226664.
[2] T.Bohnert,Y.Koucheryavy,M.Katz,E.Borcoci, andE.Monteiro,“NetworkSimulationandPer‐formanceEvaluationofWiMAXExtensionsfor IsolatedResearchDataNetworks,” IEEEJ.Commun.Softw.Syst.,vol.4,p.n/a,Mar.2008,doi: 10.24138/jcomss.v4i1.238.
[3] E.M.CenkandA.Nail,“rtPSUplinkSchedul‐ingAlgorithmsforIEEE802.16Networks,”in ProceedingsofEighthInternationalSymposium onComputerNetworks(ISCN’08),ISBN:978-975518-295-7,Copyright©2008byBoğaziçiUniversity,2008,pp.141–147.
[4] S.Ghazal,L.Mokdad,andJ.Ben‐Othman,“Per‐formanceAnalysisofUGS,rtPS,nrtPSAdmission ControlinWiMAXNetworks,”in 2008IEEEInternationalConferenceonCommunications,2008, pp.2696–2701.doi:10.1109/ICC.2008.509.
[5] C.So‐In,R.Jain,andA.‐K.Tamimi,“Scheduling inIEEE802.16emobileWiMAXnetworks:key issuesandasurvey,” IEEEJ.Sel.AreasCommun.,vol.27,no.2,pp.156–171,2009,doi: 10.1109/JSAC.2009.090207.
[6] I.Adhicandra,R.Garroppo,andS.Giordano, “Con igurationofWiMAXNetworkssupporting DataandVoIPtraf ic,”Aug.2008.[Online].Avail‐able:https://www.researchgate.net/publicati on/299366342_Configuration_of_WiMAX_Netw orks_supporting_Data_and_VoIP_traffic#fullTe xtFileContent
[7] T.Raina,P.Gupta,B.Kumar,andB.L.Raina, “DownlinkSchedulingDelayAnalysisofrtpsto nrtpsandBEServicesinWiMAX,” Int.J.Adv.Res. ITEng.,vol.6,no.2,pp.47–63,2013,[Online]. Available:chrome‐extension://efaidnbmnnni bpcajpcglclefindmkaj/https://garph.co.uk/IJAR IE/June2013/6.pdf
[8] B.KharthikaandG.M.Vigneswari,“Improve WiMAXNetworkPerformanceUsingCross‐LayerFramework,” Int.J.Sci.Eng.Res.,vol.4,no. 1,2013,[Online].Available:chrome‐extension: //efaidnbmnnnibpcajpcglclefindmkaj/https: //www.ijser.org/researchpaper/Improve‐WiMAX‐Network‐Performance‐Using‐Cross‐Layer‐Framework.pdf
[9] A.Lygizou,S.Xergias,andN.Passas,“rtPS SchedulingwithQoEMetricsinJoint WiMAX/SatelliteNetworks,”P.Pillai,R.Shorey, andE.Ferro,Eds.Berlin,Heidelberg:Springer BerlinHeidelberg,2013,pp.1–8.doi:10.1007/ 978‐3‐642‐36787‐8_1.
[10] A.L.Yadav,P.D.Vyavahare,andP.P.Bansod, “ReviewofWiMAXSchedulingAlgorithmsand TheirClassi ication,” J.Inst.Eng.Ser.B,vol.96,no. 2,pp.197–208,2015,doi:10.1007/s40031‐014‐0145‐5.
[11] D.M.S.Madhuri,D.Reethu,C.K.Mani,andT.A. V.S.S.N.Raju,“ServicePrioritizedOpportunistic SchedulingAlgorithmforWiMAXMobileMulti‐HopRelayNetworks,” Int.J.Eng.Res.Technol., vol.03,no.02,pp.2274–2279,2014,[Online]. Available: https://www.ijert.org/research/s ervice‐prioritized‐opportunistic‐scheduling‐algorithm‐for‐wimax‐mobile‐multi‐hop‐relay‐networks‐IJERTV3IS21432.pdf.
[12] R.A.TalwalkarandM.Ilyas,“AnalysisofQual‐ityofService(QoS)inWiMAXnetworks,”in 200816thIEEEInternationalConferenceon Networks,2008,pp.1–8.doi:10.1109/ICON. 2008.4772615.
[13] P.SapnaandD.Priyanka,“OptimizingIEEE 802.16j:MultihopRelayinginWiMAX Networks,” Int.J.Eng.TrendsTechnol.,vol. 19,no.1,pp.24–28,2015,[Online].Available: https://ijettjournal.org/assets/volume/volum e‐19/number‐1/IJETT‐V19P206.pdf
[14] N.N.Alfaisaly,S.Q.Naeem,andA.H.Neama, “EnhancementofWiMAXNetworksusing OPNETModelerPlatform,” Indones.J.Electr.Eng. Comput.Sci.,vol.23,no.3,pp.1510–1519,2021, doi:http://doi.org/10.11591/ijeecs.v23.i3.pp1 510‐1519
[15] S.SB,S.MS,S.Pathak,S.Irfan,andR.H,“Analysis OfSchedulingAlgorithminWiMAXNetwork,” J. Emerg.Technol.Innov.Res.,vol.6,no.6,pp.414–418,2019,[Online].Available:https://www.jeti r.org/papers/JETIR1906A64.pdf.
[16] C.‐Y.Chang,M.‐H.Li,W.‐C.Huang,andC.‐C.Chen, “AnEf icientSchedulingAlgorithmforMaximiz‐ingThroughputinWiMAXMeshNetworks,”in Proceedingsofthe2009InternationalConference onWirelessCommunicationsandMobileComputing:ConnectingtheWorldWirelessly,2009,pp. 542–546.doi:10.1145/1582379.1582497.
[17] K.J.KimandB.D.Choi,“PerformanceAnaly‐sisofExtendedRtPSAlgorithmforVoIPSer‐vicebyMatrixAnalyticMethodinIEEE802.16e withAdaptiveModulationandCoding,”2009. doi:10.1145/1626553.1626564.
[18] B.‐J.Chang,Y.‐H.Liang,andS.‐S.Su,“Analy‐sesofQoS‐basedrelaydeploymentin4GLTE‐Awirelessmobilerelaynetworks,”in 2015 21stAsia-Paci icConferenceonCommunications (APCC),2015,pp.62–67.doi:10.1109/APCC. 2015.7412581.
[19] J.M.M rquez‐Barja,C.T.Calafate,J.‐C.Cano, andP.Manzoni,“EvaluatingthePerformance BoundariesofWI‐FI,WiMAXandUMTSUsing theNetworkSimulator(Ns‐2),”in Proceedings ofthe5thACMWorkshoponPerformanceMonitoringandMeasurementofHeterogeneousWirelessandWiredNetworks,2010,pp.25–30.doi: 10.1145/1868612.1868618.
[20] H.M.IsmailandM.I.Ashour,“Analysisand DesignofIEEE802.16UplinkSchedulingAlgo‐rithmsandProposingtheIRAAlgorithmfor RtPSQoSClass,”in Proceedingsofthe6th ACMWorkshoponWirelessMultimediaNetworkingandComputing,2011,pp.49–54.doi: 10.1145/2069117.2069127.
[21] B.Al‐Madani,M.Al‐Saeedi,andA.A.Al‐Roubaiey,“ScalableWirelessVideoStreaming overReal‐TimePublishSubscribeProtocol (RTPS),”in 2013IEEE/ACM17thInternational SymposiumonDistributedSimulationandReal TimeApplications,2013,pp.221–230.doi: 10.1109/DS‐RT.2013.32.
[22] R.C.Garcia,B.S.ReyesDaza,andO.J.Sal‐cedo,“EvaluationofQualityServiceVoiceover InternetProtocolinWiMAXNetworksBasedon IP/MPLSEnvironment,”in Proceedingsofthe 11thACMSymposiumonQoSandSecurityfor WirelessandMobileNetworks,2015,pp.59–66. doi:10.1145/2815317.2815322.
[23] R.Underwood,J.Anderson,andA.Apon, “MeasuringNetworkLatencyVariation ImpactstoHighPerformanceComputing ApplicationPerformance,”in Proceedingsofthe 2018ACM/SPECInternationalConferenceon PerformanceEngineering,2018,pp.68–79.doi: 10.1145/3184407.3184427.
[24] A.BuschandM.Kammerer,“NetworkPerfor‐manceIn luencesofSoftware‐De inedNetworks onMicro‐ServiceArchitectures,”in Proceedings oftheACM/SPECInternationalConferenceonPerformanceEngineering,2021,pp.153–163.doi: 10.1145/3427921.3450236.
[25] “TheNetworkSimulator‐Ns‐2,”2022. https: //www.isi.edu/nsnam/ns/ (accessedJun.18, 2022).