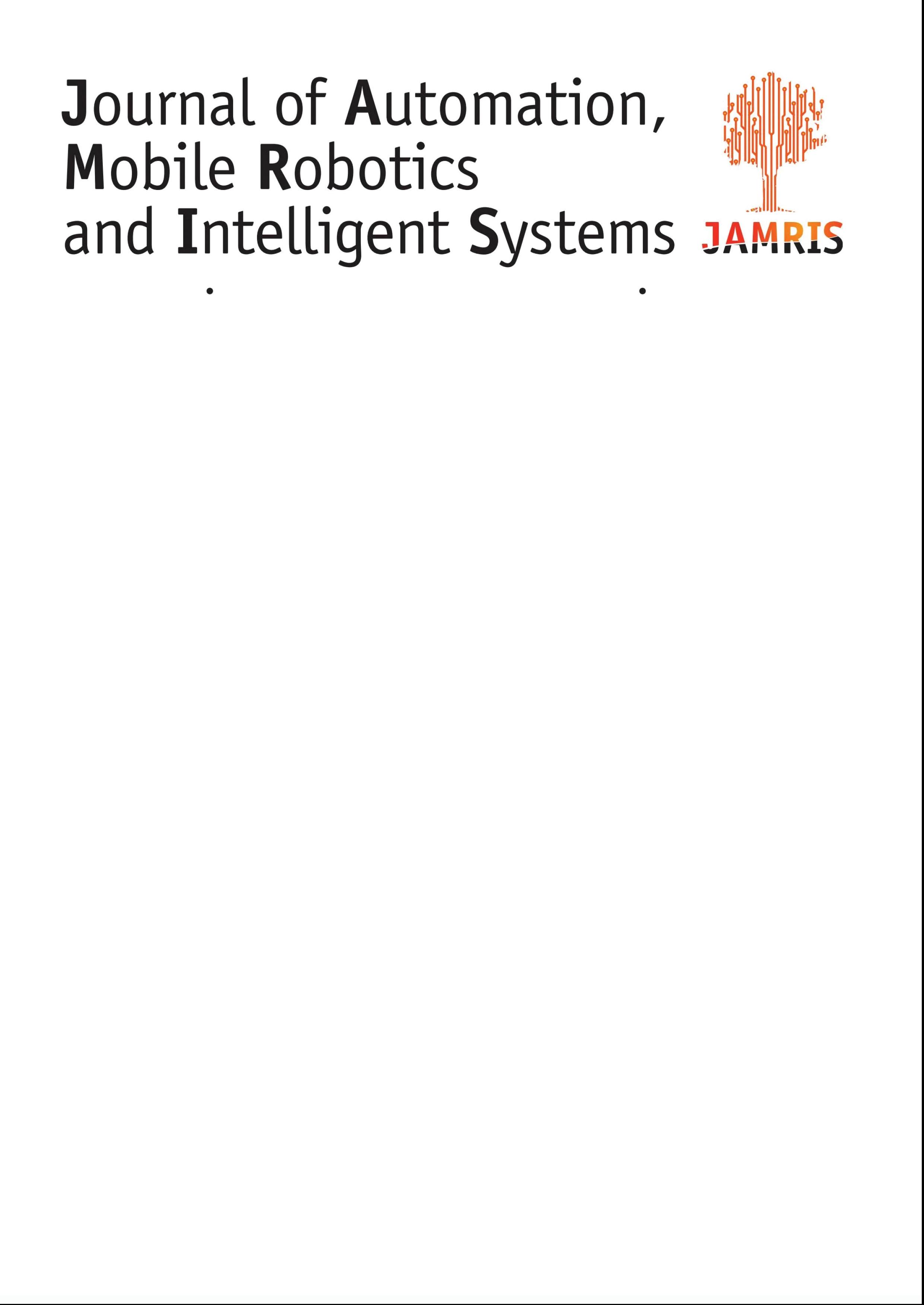
WWW.JAMRIS.ORG pISSN 1897-8649 (PRINT)/eISSN 2080-2145 (ONLINE) VOLUME 18, N° 4, 2024
Indexed in SCOPUS
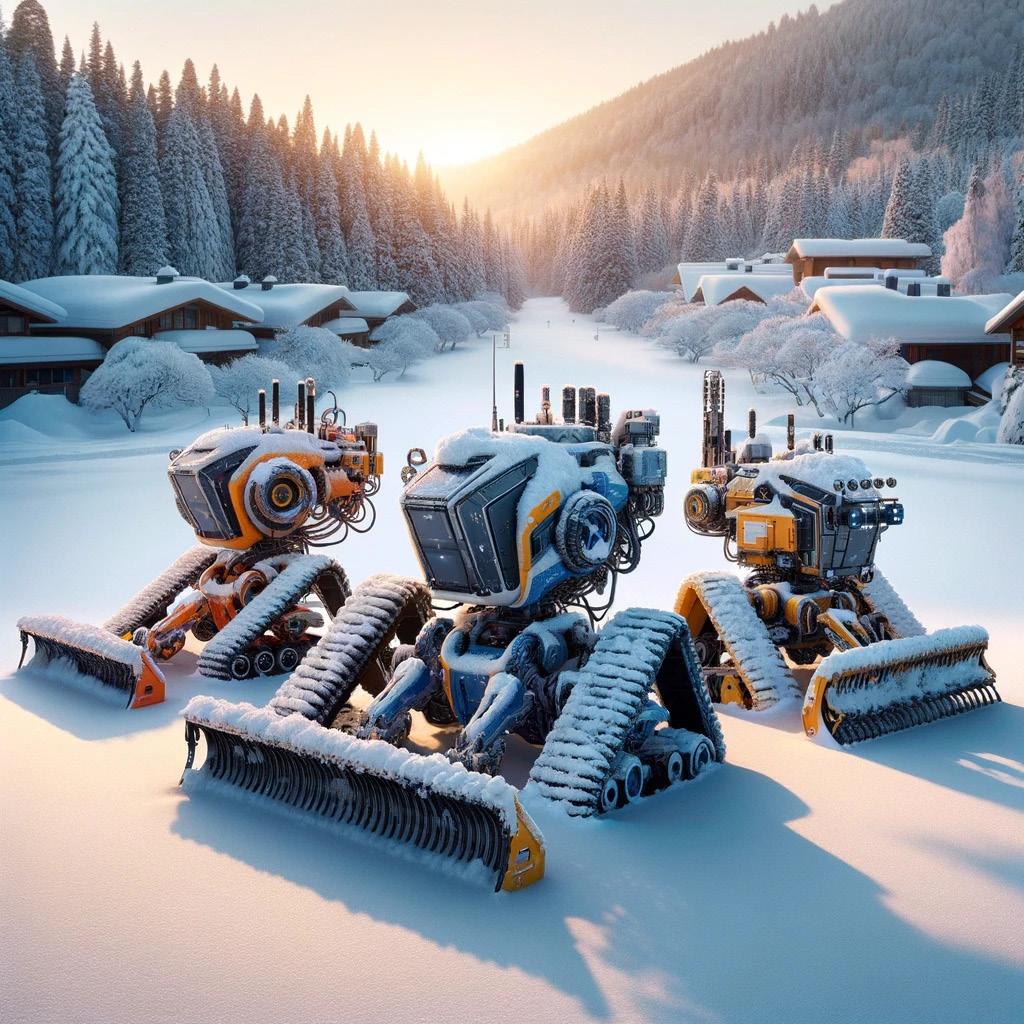
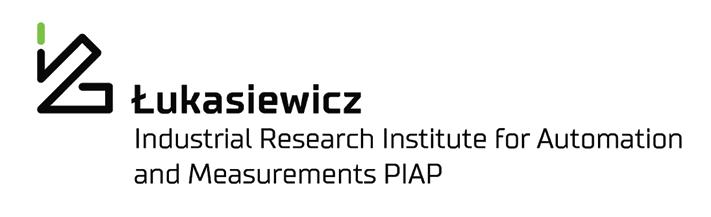
WWW.JAMRIS.ORG pISSN 1897-8649 (PRINT)/eISSN 2080-2145 (ONLINE) VOLUME 18, N° 4, 2024
Indexed in SCOPUS
A peer-reviewed quarterly focusing on new achievements in the following fields: • automation • systems and control • autonomous systems • multiagent systems • decision-making and decision support • • robotics • mechatronics • data sciences • new computing paradigms •
Editor-in-Chief
Janusz Kacprzyk (Polish Academy of Sciences, Łukasiewicz-PIAP, Poland)
Advisory Board
Dimitar Filev (Research & Advenced Engineering, Ford Motor Company, USA)
Kaoru Hirota (Tokyo Institute of Technology, Japan)
Witold Pedrycz (ECERF, University of Alberta, Canada)
Co-Editors
Roman Szewczyk (Łukasiewicz-PIAP, Warsaw University of Technology, Poland)
Oscar Castillo (Tijuana Institute of Technology, Mexico)
Marek Zaremba (University of Quebec, Canada)
Executive Editor
Katarzyna Rzeplinska-Rykała, e-mail: office@jamris.org (Łukasiewicz-PIAP, Poland)
Associate Editor
Piotr Skrzypczyński (Poznań University of Technology, Poland)
Statistical Editor
Małgorzata Kaliczyńska (Łukasiewicz-PIAP, Poland)
Editorial Board:
Chairman – Janusz Kacprzyk (Polish Academy of Sciences, Łukasiewicz-PIAP, Poland)
Plamen Angelov (Lancaster University, UK)
Adam Borkowski (Polish Academy of Sciences, Poland)
Wolfgang Borutzky (Fachhochschule Bonn-Rhein-Sieg, Germany)
Bice Cavallo (University of Naples Federico II, Italy)
Chin Chen Chang (Feng Chia University, Taiwan)
Jorge Manuel Miranda Dias (University of Coimbra, Portugal)
Andries Engelbrecht ( University of Stellenbosch, Republic of South Africa)
Pablo Estévez (University of Chile)
Bogdan Gabrys (Bournemouth University, UK)
Fernando Gomide (University of Campinas, Brazil)
Aboul Ella Hassanien (Cairo University, Egypt)
Joachim Hertzberg (Osnabrück University, Germany)
Tadeusz Kaczorek (Białystok University of Technology, Poland)
Nikola Kasabov (Auckland University of Technology, New Zealand)
Marian P. Kaźmierkowski (Warsaw University of Technology, Poland)
Laszlo T. Kóczy (Szechenyi Istvan University, Gyor and Budapest University of Technology and Economics, Hungary)
Józef Korbicz (University of Zielona Góra, Poland)
Eckart Kramer (Fachhochschule Eberswalde, Germany)
Rudolf Kruse (Otto-von-Guericke-Universität, Germany)
Ching-Teng Lin (National Chiao-Tung University, Taiwan)
Piotr Kulczycki (AGH University of Science and Technology, Poland)
Andrew Kusiak (University of Iowa, USA)
Mark Last (Ben-Gurion University, Israel)
Anthony Maciejewski (Colorado State University, USA)
Typesetting
SCIENDO, www.sciendo.com
Webmaster TOMP, www.tomp.pl
Editorial Office
ŁUKASIEWICZ Research Network
– Industrial Research Institute for Automation and Measurements PIAP
Al. Jerozolimskie 202, 02-486 Warsaw, Poland (www.jamris.org) tel. +48-22-8740109, e-mail: office@jamris.org
The reference version of the journal is e-version. Printed in 100 copies.
Articles are reviewed, excluding advertisements and descriptions of products.
Papers published currently are available for non-commercial use under the Creative Commons Attribution-NonCommercial-NoDerivs 4.0 (CC BY-NC-ND 4.0) license. Details are available at: https://www.jamris.org/index.php/JAMRIS/ LicenseToPublish
Open Access.
Krzysztof Malinowski (Warsaw University of Technology, Poland)
Andrzej Masłowski (Warsaw University of Technology, Poland)
Patricia Melin (Tijuana Institute of Technology, Mexico)
Fazel Naghdy (University of Wollongong, Australia)
Zbigniew Nahorski (Polish Academy of Sciences, Poland)
Nadia Nedjah (State University of Rio de Janeiro, Brazil)
Dmitry A. Novikov (Institute of Control Sciences, Russian Academy of Sciences, Russia)
Duc Truong Pham (Birmingham University, UK)
Lech Polkowski (University of Warmia and Mazury, Poland)
Alain Pruski (University of Metz, France)
Rita Ribeiro (UNINOVA, Instituto de Desenvolvimento de Novas Tecnologias, Portugal)
Imre Rudas (Óbuda University, Hungary)
Leszek Rutkowski (Czestochowa University of Technology, Poland)
Alessandro Saffiotti (Örebro University, Sweden)
Klaus Schilling (Julius-Maximilians-University Wuerzburg, Germany)
Vassil Sgurev (Bulgarian Academy of Sciences, Department of Intelligent Systems, Bulgaria)
Helena Szczerbicka (Leibniz Universität, Germany)
Ryszard Tadeusiewicz (AGH University of Science and Technology, Poland)
Stanisław Tarasiewicz (University of Laval, Canada)
Piotr Tatjewski (Warsaw University of Technology, Poland)
Rene Wamkeue (University of Quebec, Canada)
Janusz Zalewski (Florida Gulf Coast University, USA)
Teresa Zielińska (Warsaw University of Technology, Poland)
Publisher:
1
VOLUME 18, N˚4, 2024
DOI: 10.14313/JAMRIS/4-2024
Influence of Migration on Efficacy and Efficiency of Parallel Evolutionary Computing
Sylwia Biełaszek, Leszek Rutkowski, Aleksander Byrski
DOI: 10.14313/JAMRIS/4‐2024/27
13
Mutual Inductance Model of the Mechanical Stress Sensitivity of a Power Transformer’s Functional Parameters
Paweł Rękas, Roman Szewczyk, Tadeusz Szumiata, Michał Nowicki
DOI: 10.14313/JAMRIS/4‐2024/28
22
Nonlinear Optimal and Multi‐Loop Flatness‐Based Control of Omnidirectional 3‐Wheel Mobile Robots
Gerasimos Rigatos, Masoud Abbaszadeh
DOI: 10.14313/JAMRIS/4‐2024/29
47
Autonomous Underwater Vehicle Design and Development: Methodology and Performance Evaluation
Ismail Bogrekci, Pinar Demircioglu, Goktug Ozer
DOI: 10.14313/JAMRIS/4‐2024/30
62
Comparison of Open Source SDN Controllers and Cloud Platforms in Terms of Performance, Stability, and Infrastructure Flexibility
Andrzej Mycek
DOI: 10.14313/JAMRIS/4‐2024/31
Implementation and Performance Evaluation of Intelligent Techniques for Controlling a Pressurized Water Reactor
Ahmed J. Abougarair, Abdulhamid A. Oun, Widd B. Guma, Shada E. Elwefati
DOI: 10.14313/JAMRIS/4‐2024/32
Efficient Vehicle Detection and Classification Algorithm Using Faster R‐CNN Models
Imad EL Mallahi, Jamal RIFFI, Hamid Tairi, Mohamed Adnane Mahraz
DOI: 10.14313/JAMRIS/4‐2024/33
Submitted:22nd September2023;accepted:10th April2024
SylwiaBiełaszek,LeszekRutkowski,AleksanderByrski DOI:10.14313/JAMRIS/4‐2024/27
Abstract:
Metaheuristics,suchasevolutionaryalgorithms(EAs), havebeenproventobe(alsotheoretically,see,forexam‐ple,theworksofMichaelVose[1])universaloptimization methods.Previousworks(ZbigniewSkolickiandKenneth DeJong[2])investigatedimpactofmigrationintervalson islandmodelsofEAsintheirworks.Hereweexploredif‐ferentmigrationintervalsandamountsofmigratingindi‐viduals,complementingSkolickiandDeJong’sresearch. Inourexperiments,weusedifferentwaysofselecting migrantsandpavethewayforfurtherresearch,e.g., involvingdifferenttopologiesandneighborhoods.We presenttheideaofthealgorithm,showexperimental results.
Keywords: parallelevolutionarycomputing,metaheuris‐tics,migration
1.Introduction
SinceseminalworkofWolpertandMacReady[3] andformulationofNoFreeLunchtheoremweknow thateachmetaheuristicalgorithmsmustbeproperly parameterizedinordertomakeitfeasibleforapar‐ticularproblem.ResearcherssuchasSudholt,Cantu‐PazorSkolnickiandDeJongworkedontheparal‐lelmodelofanevolutionaryalgorithm(EA)[4][5] arguingthatdecompositionofpopulationincreases diversityandtheef icacyofthewholealgorithm.We havebeenexaminingthisproblemandthispaperis devotedactuallytoextendtheresultsdescribedby SkolnickiandDeJongdescribedin[2].
Whilestudyingthein luenceofvariousmigra‐tionssizes(migrationrate)andmigrationintervals onislandmodels,researchersnoticedthatthemigra‐tionintervalseemedtobeadominatingfactortothe bestsolutionfound,toofrequentlymigrationscause islandstodominateothersandloseglobaldiversity, toorarelymirationsperformdegradedperformance duetoslowconvergence,butevensmallmigrations alreadymakeasigni icantimpactontheresultofan islandmodel.
Therefore,wefocusedonfurtherparametrization oftheparallelEAstrivingtowardscheckingwhatkind ofcon igurationswillhelpusreachingbetteref icacy, usingthepopularmultimodalbenchmarksascase studies.
Thepaperisorganizedasfollows:Inthenextchap‐terweintroduceparallelmodelsofEAs.Inthethird chapterwedescribeconsideredalgorithm.Inchapter fourwepresenttheresultsanddiscussthem.Inthe ifthchapterwecometotheconclusion.
2.ParallelModelsofEvolutionaryAlgorithms
EAsarewellknownandwidelydecribedinthe literature[6–8]asprobabilisticoptimizationmethods inspiredbybiologicalanalogies(naturalevolution). TheessenceofEAsistocombinethephenomenonof random(undirected)genotypechangeswithstrictly directedenvironmentalpressureonthephenotype.It isapowerfulmethodforsolvingalargescaleofprob‐lemsthatcanbedescribedinanappropriateform. Itconsistsofchoosingtherighttypeofalgorithm, designingthemethodofcodingsolutions(creatinga solutionspaceoftheproblem)andconstructingthe objectivefunction.To indasolution,weneedtoknow almostnothingaboutthefunctionbeingoptimized (“blackbox”).Theremayevenbenoobjectivefunction atall:wecanuseevolutionaryalgorithmsevenwhen theonlythingwecansayaboutthepointsinthestate spaceiswhichofthetwopointsisbetter(tournament selection).
Theschemeoftheclassicalevolutionaryalgorithm includesthecreationofaninitialpopulationconsist‐ingofrandomindividuals,theuseofgeneticopera‐tors(i.e.certaintransformationsofthegeneticcode ofindividuals),calculatingthevalueoftheobjec‐tivefunctionofindividuals,selection.Theoperations describedabovetakesplaceinacyclethatendswhen thespeci iedterminationconditionismet.The inal populationineachcyclebecomesthecurrentfornext oneandevolutioncontinues.Thealgorithmstopsat theuser’srequest,afteracertaintime,certainnum‐berofsolutionevaluationsorwhenacertainsolution qualitythresholdisreached.Thealgorithmisnon‐deterministic(randomactionofmutation,crossing andselection),wehavenoguaranteethatthesolution foundisoptimal,buttheygiveahighprobabilitythat theresultwillbeclosetotheoptimaloneandwewill getitinatimethatsatis iesus.Thegeneticoperators: mutation,crossoverandselectioncanbeusedindif‐ferentvariants.
ThebasicandthenecessaryelementsofanEAare:
‐ Individual–anexemplarysolutionoftheproblem–placedinacertainenvironmenttowhichhemaybe betterorworseadapted.The“goal”ofevolutionisto createanindividualthatisaswelladaptedtoagiven environmentaspossible.
‐ Phenotype–characteristicsofagivenindividual.In thecaseofEAs,thesearetheparameters(features)of thesolutionthataresubjecttoevaluation.
‐ Genotype–acompleteandunambiguousdescription ofanindividualcontainedinitsgenes.
‐ Chromosome–theplacewherethegenotypeofan individualisstored.
‐ Population–agroupofindividualslivinginacommonenvironmentandcompetingforitsresources.
‐ Solutioncoding–awayofstoringanyacceptable solutiontoaproblemintheformofanindividual’s genotype(e.g.astringofbits).
‐ Functionofadaptation( itness)–afunctionthat allowstodetermineitsqualityforagivenindividual (fromthepointofviewoftheproblembeingsolved). Itsvaluesarerealnon-negativeandahighervalueof thefunctionalwaysmeansthatagivenindividualis better.Inthecaseofnaturalevolution,theequivalent ofsuchafunctionisthegeneralassessmentofan individual’sadaptationtoagivenenvironment.In practice,thisfunctionisusuallyaslightmodi ication oftheobjectivefunctionoftheproblembeingsolved.
‐ Geneticoperators:mutation,crossoverandselection canbeusedindifferentvariants
2.1.MainkindsofPEA’s
Asigni icantimprovementintheoperationofthe EAisobtainedbyusingaparallelEAmodel(PEA).The basicideaistodivideataskintosubtasks,andtosolve themsimultaneouslyusingmultipleprocessors.
Realizationtakesplaceasworkonsinglepopu‐lationoronseveralrelativelyisolatedpopulations, usingmassivelyparallelcomputerarchitecturesor multicomputerswithfewerandmorepowerfulpro‐cessingelements.
TherearethreemaintypesofparallelEAs;there areglobalsingle‐populationmaster‐slaveEAs,single‐population ine‐grainedEAs,andmultiple‐population coarse‐grainedEAs.
The irsttypeofparallelmodelsisthemaster slave[9].It’saneasytoimplementandveryef i‐cientmethodofparallelisationwhereweuseasin‐glepanmiticpopulation,justlikeinasimpleEA,but evaluationoftheindividualsandgeneticoperatorsis parallel.Thismodeldoesnotassumeanythingabout theunderlyingcomputerarchitecture.Eachindividual maycompeteandmatewithanyother(thusselec‐tionandmatingareglobal).Selectionandcrossover considertheentirepopulation.Inthismodelmaster storesthepopulation,andslavesevaluatethe itness ofanindividualwhichisindependentfromtherest ofthepopulation,andassigningafractionofthepop‐ulationtoeachoftheprocessorsavailable.Commu‐nicationbetweenmasterandslaveoccursonlywhen
eachslavereceivesitssubsetofindividualstoevaluate andwhentheslavesreturnthe itnessvalues.The algorithmisusuallysynchronous.
Thesecondtype, ine‐grainedparallelEAs[10] consistofonespatially‐structuredpopulationthat limitstheinteractionsbetweenindividuals.Selection andmatingarerestrictedtoasmallneighborhood,but neighborhoodsoverlappermittingsomeinteraction amongalltheindividuals.Theidealcaseistohaveonly oneindividualforeveryprocessingelementavailable. Themostpopularstructuresusedforthismodelare ring,torus,cubeorhypercube.Thismodelissuitedfor massivelyparallelcomputers.
Third,themostpopularmethodofparallelimple‐mentationofEAsismultiple‐populationEAs[4].It consistinfewrelativelylargesubpopulationswhich exchangeindividualsoccasionallyinprocessnamed migration,controlledbyseveralparameters.
Theyareknownas“distributed”EAs,because theyareusuallyimplementedondistributed‐memory MIMDcomputers(possiblyalsousingVLSIcircuitsyn‐thesis,GPGPUorHPC)orthe“islandmodel”because relativelyisolateddemeswecancall“islands”.They arealsocalledcoarse‐grainedEAs,sincethecompu‐tationtocommunicationratioisusuallyhigh.
Themainideaisthatcopyofthebestindividual foundineachdemeissenttoalloroneofitsneighbors aftereverygeneration.
Itispossibletousedifferentapproachestosolve thisproblem[11],suchasworkwithisolateddemes andwitha“delayed”migrationschemeinwhichcom‐municationsbeganonlyafterthedemeswerenear convergence(veryhighmigrationrate).Inthiscase thesolutionfoundbyisolateddemeswasmuchlower thanthatreachedwithasinglelargepopulation,how‐ever,thisdelayedschemefoundsolutionsofthesame qualityasthepanmicticpopulationandasmultiple demeswithfrequentmigrations.Wecanalsomigrate solutionsbetweendemesafterthedemesconverged completely[12,13].
Sometimesmigrationhappensatregularinter‐vals,andsometimes[13]migrationoccursafterthe demesconvergedcompletely(theauthorusedthe term“degenerate”)withthepurposeofrestoring diversityintothedemestopreventprematureconver‐gencetoalow‐qualitysolution.
Inpracticewetakeafewconventional(serial)EAs, runeachofthemonanodeofaparallelcomputer,and atsomepredeterminedtimes,ornumberofcarried evaluationsinanEA,exchangeafewindividuals.
Mostofthetime,populationsareinequilibrium (i.e.,therearenosigni icantchangesinitsgenetic composition),butthatchangesontheenvironment canstartarapidevolutionarychange.Therefore,the arrivalofindividualsfromotherpopulationscan punctuatetheequilibriumandtriggerevolutionary changes.
2.2.PEA’sMainParameters
Mainparametersofmigrationarethetopology thatde inestheconnectionsbetweenthesubpopula‐tions,migrationratethatcontrolshowmanyindivid‐ualsmigrate,andmigrationintervalthataffectsthe frequencyofmigrations,thenumberofislands,and thepopulationssizes.
Researchers[11, 14]aretryingto indrelation‐shipsbetweenimportantPEAparameters.Itisdif i‐cultanddiffersfordifferentmethodsofemigration andimmigrationandtheproblembeingsolved.
Findingthesedependencieswouldbealsohelpful inestimatingtheoptimalnumberofprocessorsfor solvingproblemsintheparallelmodel.
Soitisworthtotestitformanzdifferentsettings.
Alsodifferentwaysofcreating itnessandmuta‐tionandcrossoverfunctions,usingasinglepopulation ormultiplesubpopulations(dems),differentwaysof exchangingmigrantsandhowselectionisapplied (globallyorlocally)wereinvestigated[15].
2.3.Topologies
Manyresearchershavestruggledwiththetopicof islandmodelcommunicationtopology.Itisamajor factorinthecostofmigration.Denselyconnected topologymaypromoteabettermixingofindividuals, butitalsoentailshighercommunicationcosts.The generaltrendistousestatictopologiesthatarespec‐i iedatthebeginning,butsomeanalyse[4]ofthe designandexpectedoptimizationtimesdepending onthetopologyleadtochangesduringtheexecution ofthealgorithmaccordingtothesettings.Wecall itdynamictopologyschemes.Itspeedsuptheopti‐mization.Migrantsaresenttodemesthatmeetsome criteria.
[16]alsostudiedthesizeoftheconnectiontopol‐ogyimpactandtheappropriatetopologychoicesfor differentapplications.Migrationtopologyrankings (moreprecisely,preorders)werebuiltforadifferent numberofislands,differentoptimizationproblems anddifferentbasicalgorithms.
Therearesomeunresolvedquestionsinthis model,suchas:
‐ whatisthelevelofcommunicationnecessarytomake aparallelEAbehavelikeapanmicticEA?
‐ whatisthecostofthiscommunication?
‐ isthecommunicationcostsmallenoughtomakethis aviablealternativeforthedesignofparallelEAs?
2.4.EA’sSequentialContraParallelVersions
SequentialEAsareveryeffectiveinmanyapplica‐tions.However,thereare[15]problemsintheiruse thatcanbesolvedwithPEA.
ItalsohappensthatsequentialEAscangettrapped inasub‐optimalregionofthesearchspace.
PEAscansearchdifferentsubspacesofthesearch spaceinparallel,thusreducingthelikelihoodofbeing trappedbylow‐qualitysubspaces.
Migrationofindividualsbetweenpopulationsmay increasetheselectionpressure[5].Thishasthedesir‐ableconsequenceofspeedingupconvergence,butit mayresultinanexcessivelyrapidlossofvariationthat maycausethesearchtofail.
Forexample,sometimesproblemsrequirethe useofverylargepopulations[17],andthememory neededtostoreeachindividualcanbesigni icant.In somecases,thispreventsanapplicationfromrunning ef icientlyonasinglemachine,sosomeparallelform ofEAisnecessary.Afterdividingitinsub‐population, asdifferentislandsretainadegreeofindependence andthusexploredifferentregionsofthesearchspace, theprobabilityofanimprovedscoreincreases.When theperformanceofasplitevolutionaryalgorithmis similartothatofalargepopulation,theuseofmigra‐tionmakestheperformanceequaltoorexceedthatof alargepopulation.
2.5.DynamicsofPEA’s
SkolickianddeJong[18]describedthemechanism ofimprovingtheresultsofpeaoperation,usingthe two‐leveldynamicsoftheislandmodel,dividingit intolevels:localoneachislandandinter‐islandinter‐actions.Thesetwoevolutionaryprocessesinteract andmaycontributetotheoveralloutcometovarying degrees.Butitisimportanttochoosethenumberof islands(global)andpopulationsize(locallevel)on theseislandsaccordingly.Andtochooseamigrant attherightmoment,sothatheisgoodenoughand admittedattherighttimesothathedoesnotdisrupt theprocessestakingplacethere.
In[2],researchershaveexperimentallystudied thein luenceofvariousmigrationssizes(migration rate)andintervalsonislandmodelsusingasetof specialfunctions.Theynoticethatthemigrationinter‐valseemstobeadominatingfactor,withmigration sizegenerallyplayingaminorrolewithregardto thebestsolutionfound.Toofrequentlymigrations causeislandstodominateothersandloseglobaldiver‐sity,evensmallmigrationsalreadymakeasigni icant impactonthebehaviorofanislandmodelbutrare migrationscauseadegradedperformanceduetothe slowconvergence.
Whileexaminingthebehavioroftheislandmodel, weidenti iedtheneedtostudytheimpactofmigrant selectionstrategiesonthequalityofthesolution.In ourapproach,wecopyselectedmigrants(notrelo‐cate,likemostofresearchersbefore)andjointhe populationonthetargetislandwheretheytakepart ingeneticoperations.Attheend,theyaresubjected toselectiontogetherwiththerestofthepopulation, i.e.,weallowthemtotakepartingeneticoperations togetherwiththeresidentsoftheisland.
Usingdifferentmigrantselectionstrategies,we foundthattheyhadanoticeableimpactontheresults obtained.Weusedtwostrategiestoselectthebestand mostdistantmigrants.Thecontrolstrategyofrandom selectiondidnotgivesuchsigni icantimprovement. Therehasbeenabigprogresswithmanysettings(dif‐ferentmigrationintervalsandnumberofmigrants) regardingthemigrationintervalandthesizeofthe migrantgroup.
Figure1. Threewaysofselectingmigrantsstudied.The colorsusedarereferencedtobarresultscharts
Intheislandmodel,weusethreemigrantselec‐tionstrategies:“best”and“maxdistance”(“mDist”) withoutrepeats,and“random”.Thosestrategieswill bediscussedindetaillater.
Inourapproachmigrationsconsistofcopying(not moving)selectedindividualsfromthesourceislandon destinationisland.Fourmigrationintervalswereused every5th,15th,25thand35thevaluation,andthere werefourmigrantgroupsizesettings:1,4,9and12 individuals.
Migrantadmissionstrategywastojointhemtothe populationondestinationisland,thenusingcrossover andmutationoperators,thenselection.
Threemigrantselectionstrategieswithoutrepeti‐tions(showninFig.1)wereused:
‐ “best”–nindividualswereselectedinorderof itness values,startingfromthebestone
‐ “random”–nrandomindividualswererandomly selected
‐ “mDist”–Euclideandistancesofallindividualswere testedinpairsandthenthefarthestoneswere selectedinpairs(ifpossible)untilthenumberof migrantssetintheparameterswasexhausted Eachofthealgorithmsettingsweretested10times andtheresultswereaveraged.
AllcomputationswereperformedonaPCwork‐stationwithWindows10IntelCorei5‐2520M2.50 GHz,8GBRAMmemoryandusingIntelHDGraphics 3000graphiccard.
ThealgorithmwascreatedusingthejMetalPy framework,andthemovementofmigrantsbetween theislandswascreatedusingrabbitMQ,Dockerand Pika.WeinvestigateRastriginandSphere(DeJong) problemsindimension200.Thetopologyconsistsof 5islandsconnectedinaringwithtwo‐waytraf ic.The populationsizeoneachislandis16andtheoffsetis4. Westudytheresultsofthealgorithmforoneislandby reference.Thenthepopulationhasacardinalityof80 andanoffsetof20.
Whencomparingevolutionaryalgorithmsrunning ondifferenthardware,itisimportanttohaveanequal numberofevaluationsperformedinthealgorithms. Thisisbecauseenvironmentparametersaredifferent, e.g.,thesizeoftheoperatingmemory,whichaffects thespeedofthecomputer.
Similarly,thisistruewhencomparinganalgorithm runningononeislandtoonerunningon iveislands. Butit’snotonlythenumberofevaluationsthatmat‐ters.Itisalsoimportantthatthenumberofcyclesof thealgorithmisequalononeislandandeachofthe iveislands.Weachievedthisbyequalizingthepopu‐lationandoffsetononeislandandthetotalnumber onmanyislands,aswedescribedabove.
Theendcriterionwasthemaximumnumberof evaluations.Forthe iveislandsmodel:15,000for theSphereand20,000fortheRastrigin,andforone island,75,000fortheSphereproblemand100,000for theRastriginproblem.Thismeansthatnotonlythe stopcondition,butalsothesizeofthepopulationare thesameinthecomparedstudies.
InFigures 2 and 3 (fortheRastriginandSphere problemswithdifferentmigrationstrategies,respec‐tively),thecolorscale(fromtheworstresult–dark redtothebestresult–darkgreen)showstheaverage resultsofvarioussettings:themigrationintervaland thesizeofthemigrantgroup.Wecanseethatthered colorvaluesaregroupedinthesameareaandthe greencolorvaluesinapproximateareas.
Thesamething,butintheformofa3Dspatial graph,canbeseenincharts4and5(fortheRastrigin andSphereproblemswiththeexaminedsettingsof themigrationintervalandthenumberofmigrants, respectively).Thebestresultsarethosewiththelow‐estpositioninthedrawings,andtheworstarethose placedhighest.Theworstresultsareinthesameplace inallthedrawings,andthebestonesoccupysimilar areas.Wecanseeasimilarinclinationandshapeof theplanescreatedforthesesamples–whichindicates thatthesizeofthemigrantgroupsandtheinter‐valsworksimilarlyforthesedifferentproblemsand strategies.
InFigures 6 and 7 (fortheRastriginandSphere problems,respectively),thebargraphsshowthecom‐parisonofaverage inalresultsofthe ive‐islandand one‐islandsettings.Herewecanclearlyobservehow manyattemptsofthetestson iveislands(interval, sizeofthegroupofmigrants)ledtosuccesswith agivenmigrantselectionstrategy,i.e.improvedthe resultofthesingle‐islandmodel(itsgraphistothe leftofthebarofoneisland).Forexample,thestrategy “best”and“mDist”inmanysettings(interval,sizeof thegroupofmigrants)ledtoanimprovementinthe resultinrelationtothesingle‐islandmodel.Andinthe “random”strategy–comparative,becausethemigrat‐ingindividualsweresimplydrawnrandomly,itdidnot improvetheresultofoneislandatall(forSphere)or almostatall(forRastrigin).
(a)Rastriginwith“best”migrationstrategy(left)andwith“mDist”(right)
(b)Rastriginwith“random”migrationstrategy
Figure2. AverageresultsachievedforRastriginproblemwithdifferentmigrationstrategies.Resultstabulatedby migrationintervalsandnumbersofmigrants.Colorscaleandintensity–worstresult–darkred,bestresult–darkgreen
(a)Spherewith“best”migrationstrategy(left)andwith“mDist”(right)
(b)Spherewith“random”migrationstrategy
Figure3. AverageresultsachievedforSphereproblemwithdifferentmigrationstrategies.Resultstabulatedbydifferent migrationintervalsandnumbersofmigrants.Colorscaleandintensity–worstresult–darkred,bestresult–darkgreen
TableinFigure 8 describes(foreachproblem, eachwayofselectingmigrants,differentmigration intervalsandnumbersofmigrants)ranking(inplus orminus)oftheresultsobtainedinrelationtothe correspondingresultobtainedononeisland.
Thefollowingsymbolshavebeenusedinthetable Figure8:
‐ 0–referencepoint–resultforamodelwith1island
‐ positivenumbers–positionof iveislandmodelwith speci icparametersinrankingofresultsbetterthan appropriate“reference”ononeisland
‐ negativenumbers–positionof iveislandmodelwith speci icparametersintherankingofresultsworse thantheresultofappropriate“reference”onone island
The irstthreecolumnsintheTable 8 describe algorithmstartparameters:thenumberofislands, themigrationintervalandthenumberofmigrating individuals.
Figures 12 and 13 showtherunsofthe10‐trial averageforeachsetting(interval,migrantgroupsize) inthewinningstrategiesforagivenproblem.
ForeasierunderstandingofFigure8,pleasecom‐pareFigure13showingalllineresultsofSphere200in “best”version,it’svaluesshowninFigure3aandthe forthcolumnoftheResultstableTable8,i.e.,Sphere 200/“best”column.Findtheresultobtainedonone islandinthegraphandnoticethatsubsequentresults havethesamenumberinthetableasthedistanceof theirgraphfromthegraphobtainedononeisland.
Similarly,whenconsideringRastriginproblem, Figure12,Figure2aandTable8(theseventhcolumn, ie.Rastr200/“best”column)shouldbeconsidered.
Thebest,takingintoaccountmigrantsselection strategies,were:“best”,then“mDist”and inally“ran‐dom”.
(a)“best”(left)and“mDist”(right)migrantselectingstrategy
(b)“random”migrantselectingstrategy
Figure4. ComparativeresultsofRastriginproblem,withtheexaminedsettingsofthemigrationintervalandthenumber ofmigrants
(a)“best”(left)and“mDist”(right)migrantselectingstrategy
(b)“random”migrantselectingstrategy
Figure5. ComparativeresultsofSphereproblem,withtheexaminedsettingsofthemigrationintervalandthenumberof migrants
(a)“best”(left)and“mDist”(right)migrantselectionstrategy
(b)“random”migrantselectionstrategy
Figure6. GraphsofresultsofRastriginproblem,obtainedwithdifferentsettingsofthemigrationintervalandthenumber ofmigrantsonthefiveislands.Forcomparison–redbar–theresultachievedononeislandwithcomparablesettings
(a)“best”(left)and“mDist”(right)migrantselectionstrategy
(b)“random”migrantselectionstrategy
Figure7. Graphsofresultsofsphereproblem,obtainedwithdifferentsettingsofthemigrationintervalandthenumber ofmigrantsonthefiveislands.Forcomparison–redbar–theresultachievedononeislandwithcomparablesettings
Figure8. Ranking,foreachoftheexaminedmigrationintervalsandthenumberofmigrants,(inplusorminus)theresults obtainedinthePEAwithfiveislandsinrelationtotheEAresultobtainedononeislandforthesameproblemandthe methodofselectingmigrants
Figure9. Comparingthebestandworstresultsinfiveislandmodeltooneislandmodelfordifferentmigrantsselection strategies.Rastriginproblem)
InSphere200/random,nosettingsconnectedwith iveislandsgaveresultsbetterthantheoneisland score,andinRastr200/randomonlyonesetting (co5ile4)gavebetterresultswhencomparinganalo‐gously.
Intheotherversionsofthemigrationstrategy (“mdist”and“best”),forbothproblems,thereare alwayssome iveislandresultsthatperformbetter thanoneisland.
Thismeansthatourselectiveselectionofmigrants onsourceislandisworking.Weachievethebest resultswhenweselectthebestormorediverseindi‐viduals(themostdistantinourcase),theresultsare thensigni icantlybetterthanwhenweselectmigrants randomly.
ThebestresultsintheSphereproblemwere achievedwiththe“best”strategy(settings–interval5, groupof4migrants),worsewiththe“mDist”strategy (interval15,groupof9migrants),andallsettingsof “random”strategyachievedworseresultsthanresults ofoneisland.
Figure10. Comparingthebestandworstresultsinfiveislandmodeltooneislandmodelfordifferentmigrantsselection strategies.Sphereproblem
Figure11. Comparingtheimprovedresults(persixsettings=2problems*3strategies)ofPEAwithfiveislandsin relationtotheresultofEAononeisland,obtainedfortheexaminedmigrationintervalsandnumbersofmigrants
ThebestresultsoftheRastriginproblemwere achievedwith“best”strategy(settings–interval5, groupof4migrants),followedby“mDist”(settings–interval15,groupof4migrants)andonlyone“ran‐dom”strategysetting(settings–interval5,groupof4 migrants)wasbetterfor iveislandsthantheresults ofoneisland.
Inthe“random”strategy,individualsofdiffer‐entqualitywererandomlyselected.Therefore,their resultsareworsethaninstrategieswherecarefully selectedindividuals–thebestorthemostdiverse–migratequalitativelyorimprovethediversityonthe destinationisland.
Foreveryissueandmigrationstrategy,thesettings withinterval5andsizeofmigrationgroup9or12–alwaysperformedsigni icantlyworsethanallothers. Ithappensbecauseinsuchcasesmigrantsarrivingin largenumbersonthedestinationislandhinderthe evolutiononthisisland,almostreplacingitsexisting populationThesearecasesofshortmigrationinter‐valswithabignumberofmigrants(closetothesizeof thepopulation)atthesametime.
TheadditionalinformationinFigure 9 (forRast‐rigin)andFigure10(forSphere)showthenumerical valuesachievedon iveislands(bestandworstresult) andononeislandandshowthenumericaldifferences betweentheseresults.
Let’slookatthisdifferences.Aswecanseethe greatestvaluedifferencesbetweenresultsachieved on iveislandsandbiggestimprovementon ive islandsoverthescoresofasingleislandwereachieved for“best”migrantselectionstrategyinbothproblems. InRastrigin(Fig. 9)“best”surpassedtheoneisland scoreby8,13,“mDist”by5,72,anda“random”by0,66. InSphere(Fig.10)“best”surpassedoneislandscore by0,43,“mDist”by0,14,and“random”by0,05.
Figure11summarizespositivevaluesinFigure8 byrows.Ineach ieldattheintersectionoftheappro‐priatemigrationintervalandthesizeofthemigrant group,thenumberofresultsfrom iveislandsthat achievedsuccessappears,i.e.,theywerebetterthan theresultononeisland(outofsixpossible–two problems*threestrategies).
Figure12. Averagedrunsofparallelcalculationsonfiveislandsandreferencecalculationsobtainedononeislandfora winningmigrantselectionstrategy(“best”)fortheRastriginproblemwithdimension200
Figure13. Averagedrunsofparallelcalculationsonfiveislandsandreferencecalculationsobtainedononeislandfora winningmigrantselectionstrategy(“best”)fortheSphereproblemwithdimension200
Aswecanseethebestresultsweachievedforlow numberofmigrants(one,fourornine)andmorefre‐quentmigrations(afterevery5thorevery15theval‐uation).Thisisbecausetheseareshortintervalsand smallgroupsofmigrants–thatiswhytheyhavethe opportunitytoimprovethequalityofthepopulation onthetarget,andnotdisruptthecourseofprocesses onit.
Ifwefocusontheworstsettingsoftheinterval andthenumberofmigrantgroups,wecanseethat, asmentionedabove–largemigrationsdestroythe populationofthedestinationislandbyreplacingit, bothwhentheyarefrequent(parameters–interval5, migrantgroupsize:9,12),whentheyareverydisturb‐ing,andrare(parameters–interval35,migrantgroup size:9,12).
Anothergroupoftheworstattemptswereinwhich migrationswerefewandrare(parameters–interval–25,35,migrantgroupsize:1).
InspiredbytheworksofDeJongandSkolicki,we performedanexperimentforRastriginandSphere problems.Weusedfourmigrationintervalsandfour sizesofmigrantgroups,expandingtheexperiment withastudyfordifferentmigrationstrategies.
Weobservedthatthestrategiesweusedforselect‐ingmigrantsinmanycasesledtoimprovedresults comparedtoourreference,i.e.,theEAmodelworking asoneisland.
Inthe“best”strategy,wesentgroupofbestindi‐vidualsfromthesourceisland.Sotherewasapossibil‐itythatonthedestinationislandtheywouldbegood materialforthefurtheroperationoftheevolutionary algorithm.
Usingthe“mDist”strategy,wesentagroupof individualsthatwereasdistantaspossiblefromeach otherintermsofgenotype.Thus,theyhadachanceto increasediversityonthedestinationisland,orevento restorediversityifthepopulationonthedestination islandwastooconvergent.
Weintendtocontinueourresearchonthisimpor‐tanttopicinthefuture.Wewillconductourresearch usingHPConalargescale.Inaddition,weintendto studythebehaviorofaparallelizedEAinanenviron‐mentwithdelays,assumingthepossibilityofdesyn‐chronizationandtakingcareofscalability.Thiswill makeitpossibletocomparetheoperationofthealgo‐rithmincontrasttocoherentlyandsynchronously operatingmaster‐slavemodelsandtheclassicisland model.
AUTHORS
SylwiaBiełaszek –AGHUniversityofScienceand Technology,Al.Mickiewicza30,Krakow30‐059, Poland,e‐mail:bielsyl@agh.edu.pl.
LeszekRutkowski –AGHUniversityofScience andTechnology,Al.Mickiewicza30,Krakow30‐059, Poland;InstituteofSystemsSciencesPolishAcademy
ofSciences,ul.Newelska6,Warsaw01‐447,Poland, e‐mail:rutkowski@agh.edu.pl.
AleksanderByrski∗ –AGHUniversityofScience andTechnology,Al.Mickiewicza30,Krakow30‐059, Poland,e‐mail:olekb@agh.edu.pl.
∗Correspondingauthor
ACKNOWLEDGEMENTS
ThisworkwassupportedbyNCNUMO‐2019/35/O/ST6/00571andthefundsassigned bythePolishMinistryofEducationandScienceto AGHUniversityofScienceandTechnology.
[1] L.D.Davis,K.DeJong,M.D.Vose,L.D.Whit‐ley,W.Miller,Eds.,EvolutionaryAlgorithms,Vol. 111ofTheIMAVolumesinMathematicsand itsApplications,NewYork,NY,Springer,1999, [Online].Available:doi:10.1007/978‐1‐4612‐1542‐4.
[2] Z.Skolicki,K.DeJong,Thein luenceofmigra‐tionintervalsonislandmodels,2005,pp.1295–1302,doi:10.1145/1068009.1068219.
[3] D.Wolpert,W.Macready,“NoFreeLunchThe‐oremsforOptimization”,IEEETransactionson EvolutionaryComputation,vol.1,no.1,pp.67–82,1997.
[4] D.Sudholt,ParallelEvolutionaryAlgorithms, Berlin,Heidelberg,SpringerHandbooks, Springer,2015,doi:10.1007/978‐3‐662‐43505‐2‐46.
[5] E.Cantu‐Paz,“OntheEffectsofMigrationon theFitnessDistributionofParallelEvolutionary Algorithms”,no.UCRL‐JC‐138729,2000,URLht tps://www.osti.gov/biblio/791479.
[6] D.E.Goldberg,GeneticAlgorithmsinSearch, Optimization,andMachineLearning,Addison‐Wesley,1989,google‐Books‐ID:2IIJAAAACAAJ.
[7] R.Chiong,T.Weise,Z.Michalewicz,Variantsof EvolutionaryAlgorithmsforReal‐WorldApplica‐tions,2012,doi:10.1007/978‐3‐642‐23424‐8.
[8] Z.Michalewicz,GeneticAlgorithms+DataStruc‐tures=EvolutionPrograms,SpringerScience &BusinessMedia,1996,google‐Books‐ID:vlh‐LAobsK68C.
[9] E.Cantú‐Paz,Master‐SlaveParallelGeneticAlgo‐rithms,GeneticAlgorithmsandEvolutionary Computation,Boston,MA,Springer,2001,doi: 10.1007/978‐1‐4615‐4369‐5‐3.
[10] E.Cantú‐Paz,Fine‐GrainedandHierarchicalPar‐allelGeneticAlgorithms,GeneticAlgorithms andEvolutionaryComputation,Boston,MA, Springer,2001,doi:10.1007/978‐1‐4615‐43 69‐5‐8.
[11] E.Cantú‐Paz,D.E.Goldberg,“Ef icientparallel geneticalgorithms:theoryandpractice”,Com‐puterMethodsinAppliedMechanicsandEngi‐neering,vol.186,no.2–4,pp.221–238,2000, doi:10.1016/S0045‐7825(99)00385‐0.
[12] Y.Sato,Y.Takai,M.Munetomo,“Anef icient migrationschemeforsubpopulation‐based asynchronouslyparallelgeneticalgorithms.”, Material:Proceedingsofthe ifthinternational conferenceongeneticalgorithms,S.F.Ed., SanMateo,CA:MorganKaufmann,1993, pp.649.
[13] H.Braun,“Onsolvingtravellingsalesmanprob‐lemsbygeneticalgorithms”,ParallelProblem SolvingfromNature,LectureNotesinComputer Science,H.P.SchwefelandR.Manner,Eds.Berlin, Heidelberg:Springer,1991,pp.129–133,doi:10 .1007/BFb0029743.
[14] E.Cantú‐Paz,D.E.Goldberg,OntheScalability ofParallelGeneticAlgorithms,vol.7,1999,pp. 429–449,doi:10.1162/evco.1999.7.4.429.
[15] M.Nowostawski,R.Poli,“Parallelgeneticalgo‐rithmtaxonomy”,1999ThirdInternationalCon‐ferenceonKnowledge‐BasedIntelligentInfor‐mationEngineeringSystems.Proceedings(Cat. No.99TH8410),1999,pp.88‐92,doi:10.1109/ KES.1999.820127.
[16] M.Ruciński,D.Izzo,F.Biscani,“Ontheimpact ofthemigrationtopologyontheislandmodel”, ParallelComputing,Issues,vol.36,no.10–11,pp. 555–571,1993,doi:10.1016/j.parco.2010.04. 002.
[17] D.Whitley,S.Rana,R.Heckendorn,“TheIsland ModelGeneticAlgorithm:OnSeparability,Pop‐ulationSizeandConvergence”,JournalofCom‐putingandInformationTechnology,vol.7,Dec. 1998.
[18] Z.Skolicki,K.DeJong,“Theimportanceofatwo‐levelperspectiveforislandmodeldesign”,Con‐ference:EvolutionaryComputation,2007.CEC 2007.IEEECongresson,IEEEXplore,2007,doi: 10.1109/CEC.2007.4425078.
Submitted:21st May2024;accepted:26th September2024
PawełRękas,RomanSzewczyk,TadeuszSzumiata,MichałNowicki
DOI:10.14313/JAMRIS/4‐2024/28
Abstract:
Themutualinductancemodelenablestestingofthe behaviorofpowertransformersunderdifferentopera‐tionconditions,especiallyundertheenvironmentalinflu‐encesonthetransformer’score.Thispaperpresentsthe resultsofaninvestigationofthestressdependenceofthe magneticrelativepermeabilityofapowertransformer. Itwasobservedthatundertensilemechanicalstresses upto98MPaappliedtothecore,thetransformerinput currentamplitudeincreasesbyalmost67%,whereas thetransformer’sreactivepowerincreasesby53%.In industrialsystems,suchchangescanpotentiallyleadto unwantedpowersystemshutdownsduetooverloading. Thiseffectshouldbeconsideredduringthedevelopment ofcriticalpowersystems.
Keywords: mutualinductancemodel,magnetoelastic effect,electricsteel
1.Introduction
Specializedpowersuppliesoftenoperatewithin theirdesignconstraints,whereevenminorchanges cansigni icantlyaffectperformanceandsafety[1], includingtheriskofoverheating[2,3].Understanding theselimitationsiscrucialforensuringreliabilityand performanceindemandingapplications,suchasthe military[4],aerospace[5],andautomotivesectors[6].
Themechanicalstressdependenceofthemag‐neticfunctionalcharacteristicsofelectricsteelsheets hasbeenwidelytested,bothfornon‐oriented[7,8] andgrain‐orientedelectricsteels[9,10].Thispaper presentsatechnical‐orientedapproachandexamines theimpactofchangesinthemagneticpermeability oftransformercoressubjectedtomechanicalstress ontheirfunctionalperformance.Thestudyutilizeda speciallydesignedexperimentalsetup[11]toinvesti‐gatehowmechanicalstressaffectsthemagneticper‐meabilityofatransformercore.Thehypothesisposits thatvariationsinthecore’smagneticpermeability resultinaproportionaldecreaseininductance,acrit‐icalparameterfortransformerfunctionality.
The indingsofthisresearchareparticularlyrel‐evantfordesignersofthetransformersusedinhigh‐overloadapplicationswheremechanicalandthermal stressesaresubstantial.Designersmustthoroughly assesstheimpactofmechanicalstressontransformer performancetopreventpotentialfailures.
Additionally,attentionmustbepaidtoheatdis‐sipationduringinstallationtomitigatetheeffectsof stress‐inducedchanges.Thisconsiderationiscritical inspecializedinstallations,suchasaerospaceandmil‐itaryapplications,whereoverloadscanreachextreme levels.
Thearticlefocusesonstudyingtheimpactof mechanicalstressonthefunctionalparametersof apowertransformer.Intheinitialstage,exper‐imentswereconductedusingthreemeasurement yokesbasedontheEpsteinframe,withframesmadeof sheetmetalcutatdifferentanglesrelativetothestrip axisandtherollingdirection.Thisresearchaimedto verifytheaccuracyofthetransformermodelselec‐tionimplementedinMatlabSimulink.Inthesubse‐quentpartofthearticle,theeffectofmechanicalstress onthemagneticpermeabilityofM120‐27ssteelwas analyzed.Theresultswereusedtoassesschangesin thepermeabilityofthecoreofthesimulatedtrans‐formerundermechanicalstress.Theparametersofa samplesingle‐phasetransformerwereadoptedasthe initialvalues,re lectingtheunstressedcondition.The transformermodelfromtheMatlabSimulinkpackage, whichwasselectedasthebasisforthesimulation, mettheassumptionsveri iedbyEpsteinyoke‐based measurementsregardingtheconstancyofvoltagein thesecondarywindingandtheincreaseincurrentin theprimarywindingwithchanginginductancesofthe primaryandsecondarywindings.Inthe inalphase ofthestudy,theimpactofmechanicalstressonthe parametersofthesimulatedpowertransformerwas determined.
2.InitialTestingoftheModelUsingData ObtainedFromYokesBasedontheRescaled EpsteinFramewithNon‐stressedMaterials Tovalidatetheassumptionsregardingthetrans‐former’sresponsetochangesinthemagneticparam‐etersofthetransformercore,testswereconducted usingmeasurementyokesbasedontherescaled Epsteinframe[12, 13].Threemeasurementyokes wereemployed,utilizingstripsoftransformersheet metalcutat0∘,55∘,and90∘ anglesrelativetotheaxis ofthesheetstrip(magnetizationdirection)andthe rollingdirection.Themeasurementsystemwascon‐iguredaccordingtotheschematicshowninFigure1.
Table1. ResultsofthemeasurementyokesbasedontheEpsteinframe
Figure1. Wiringdiagramofthemeasuringyokebased ontheEpsteinframe
Initialinductancemeasurementsweretakenfor boththeprimaryLPri andsecondaryLSec windings ofthetransformer.Theinductancesweremeasured usingaHandheldLCRMeterTH2822A,manufactured byTonghui.Theresultsofthetransformerresponse andinitialinductancemeasurementsarepresentedin Table1
Theexperimentalsetupbeganwithafunctiongen‐eratorproducingasinusoidalwaveformat50Hzwith apeak‐to‐peakvoltage(Vpp)of20VAC.Thissignalwas ampli iedusingaKepcoBOP100‐2Mampli ier.The ampli iedsignalpassedthroughanammeter(Appa 207)anda0.1 Ω shuntresistorbeforereachingthe magnetizingwindingsofthemeasurementyoke.A voltmeter(Appa207)wasconnectedinparallelwith themagnetizingwindingtomeasurethevoltage.An oscilloscopemonitoredthevoltageacrosstheshunt resistorandthemagnetizingwinding.
Themeasurementwindingoftheyokewastested undertwoconditions:noloadcondition,wherethe windingwasnotconnectedtoanyexternalload, andloadedcondition,whereanoutputpowermeter (PWT‐5A)wasconnectedwithaloadsetat15 Ω. The15 Ω loadwasselected,underwhichthemost signi icantpoweroutputwasobservedfromthemea‐surementwinding.Themeasurementwindingwas connectedtothisloadthroughanammeter(Appa 207)anda0.1Ωshuntresistor.Avoltmeter(Tonghui TH1951)measuredthevoltageacrossthemeasure‐mentwinding,whiletheoscilloscopecapturedboth thevoltageacrosstheshuntresistorandthemeasure‐mentwindingvoltage.
Figure2. Wiringdiagramofthemeasurementsetup
Datacollectedincludedpowermeterreadings fromthemeasurementwinding,voltageacrossthe magnetizingwinding,currentthroughthemagnetiz‐ingwinding,andvoltageacrossthemeasurement winding.ThesevaluesaresummarizedinTable 1, demonstratingtheimpactofcoremagneticperme‐abilityonthetransformer’selectricalparameters.
Theresultsunequivocallyindicatethatasthemag‐neticpermeabilityofthecorematerialdecreases, thevoltageandpoweronthesecondarywindingof thetransformerremainlargelyunchangedwithinthe testedrange.However,asigni icantincreaseinthe currentontheprimarywindingisobserved.
3.TheMethodofMeasuringStressDepen‐denceof2DMagnetizationCharacteristics inElectricalSteelSheets
Aspecializedmeasurementsetupbasedonamag‐neticyokewasdevelopedtoinvestigatetheeffectof themechanicalstressdependenceonthemagnetic propertiesofelectricalsteels[11].Themechanical partofthesetupwaspreparedwithafocusonthe stressapplicationdevice[14],andaspeciallydesigned specimenshapewascreatedtoensureoptimalstress distribution[15].Anautomaticsystemforpositioning theyokethroughoutthetestingcyclewasalsodevel‐oped.Thetestingprocesscontrolsystem,signalanaly‐sis,anddataprocessingsystemwereestablished.This setupisdescribedindetailin[11].Thewiringdiagram isshowninFigure2
Schematicblockdiagramofthemeasurement setup
Theinformation lowdiagramispresentedinFig‐ure3.Thesetupiscontrolledbyacomputerequipped withLabViewsoftwareandaDAQDeviceNIUSB‐6341 dataacquisitioncard.Themagnetic luxintheyoke ismeasuredusingaLakeShoremodel480 luxmeter. Theactuatorsaremanagedbyanembeddedsystem basedonamicrocontrollerthatcontrolstherotation andlinearmotionoftheyokesystemhead.
3.1.TheExperimentalSetup
Thedevelopedsetupoperatesautomaticallyand performsacomplexseriesofmeasurements.Byusing specimenscutatvariousanglesbetweenthedirection oftheappliedstress(specimenaxis)andtherolling directionofthesheet,itispossibletostudychangesin magneticpermeabilityasafunctionofvaryingstress, theanglebetweenthestressdirectionandrolling direction,andtheanglebetweenthespecimenaxis andthemagnetizationdirection.Combinedwithan automaticallyrotatingyoke[11],thisfacilitatesawide rangeofpossibleresearch.
Themeasurementprocess,withtheexperimen‐talsetuppresentedinFigure 4,beginsbymount‐ingthespecimeninamechanicalmoduletoapply stresses(7).Themechanicalmoduleispositioned onamechanicalpress,whichappliestheappropri‐atestressestotheclampedsample.Theyokesystem head(5)withtheattachedmeasuringyokeispressed againstthesampleduringthemeasurement.Theforce appliedremainsconstantthroughoutasinglecycle duetotheuseofalinearoverloadclutchandthelock‐ingofthelinearactuatorinthemeasuringposition. Uponcompletingthemeasuringcycle,theyokesystem headretractsandperformsacontrolledrotarymotion tothenextmeasuringpositionusingtherotatorstep‐permotor(3).Theentireassembly,coupledtothe linearactuator,movesalongthelinearguide(4).
Figure4. Photographyofthemeasurementsetup:(a) testedsheetsample,(b)generalviewofthe measurementsystem,and(c)measuringheadduring themeasurementprocess:1–linearactuator,2–cushioningmechanism,3–rotatorwithsteppermotor, 4–linearrollingguide,5–measuringhead,6–tested sheetsample,7–mechanicalmoduleformountingand applyingstressestothetestedsample
M120‐27selectricalsteel[16]sheetsampleswith aspeciallydesignedshapewereusedforthemeasure‐ments.Thespecimencomprisesthreesections:the irstandthirdsectionswereusedsolelyformount‐ingthespecimensinthemechanicalmoduleanddid notparticipateinthestudyofmagneticproperties, whilethesecondsectionwassubjectedtostress,and itsmagneticpropertieswerestudied.Duringthetest, themeasuringyokeispressedagainstthemeasuring section.
Figure5. Themeasuringhead(5),madeof non‐magneticmaterials,featuresa2DCardan gyroscopicmechanismandameasuringyoke(8)
Thespecimen’sshapeisoptimizedusingthe inite elementmethodtoensureauniformstressdistri‐bution[11].Thespecimensareclampedinanon‐magneticmechanism.Onlytensilestressesareapplied duetothelowforcerequiredtobucklethespecimens.
3.2.AutomaticallyAdjustingtheYokeSystem Magneticyokesprovidethebestresultswhen measuringthedirectionalpropertiesoftransformer sheets.However,whenstudyingtheangularproper‐tiesofthesheetsoverafull360‐degreerangewith high‐resolutionyokepositioning,themanualopera‐tionbecomesinef icientduetothelengthymeasure‐menttimesinvolved.Thesolutiontothisproblem isimplementinganautomaticmeasurementsystem basedonamechatronicsetupthatpositionstheyoke accurately.
Automatedmeasurementsrequireensuringthat theyokeispressedagainstthesampleinthemost effectiveand,crucially,repeatablemanner.Thiscon‐sistencywasachievedbysuspendingtheyokeona Cardanjoint.Thedevelopedsystemallowstheyoketo maneuverwithfourdegreesoffreedom.Theposition‐ingoftheyokeitselfusestwodegreesoffreedom:XY andXZrotationoftheyokeinthehead.Additionally, theYZrotationoftheentireheadandlinearmovement alongtheX‐axisenablecomplete360‐degreetesting. AphotographoftheyokeholderwithCardansuspen‐sionandaxisdescriptionisshowninFigure5
3.3.UncertaintyAnalysisandErrorMitigationinthe MeasurementConfiguration
Thepresentedteststandwasdesignedtomeasure relativemagneticpermeability,withparticularatten‐tiontothedependenceofthispermeabilityonthe anglesbetweenthedirectionofexternalmechanical stresses,therollingdirectionofthetransformersheet, andthedirectionofmagnetization.Adetaileddescrip‐tionoftheuncertaintydeterminationmethodcanbe foundin[11].
Figure6. Theinfluenceofmechanicaltensilestresses �� ontheshapeofthemagnetichysteresisloopB(H)of M120‐27sgrain‐orientedelectricalsteel
Themainfactorin luencingchangesinrelative permeability(����)wasthevaryingintensityofthe magnetizing ield.Therefore,theA‐typeuncertainty, denotedas ��(����),wasestimatedbasedonthecal‐culatedvaluesof ���� witha95%con idencelevel, dependingontheappliedmagnetizing ieldstrength H.Itisworthnotingthatbeyondtheinitiallevelof H,wheresigni icantinteractionbetweenremanent magnetizationanddemagnetizing ieldsoccurs,theA‐typeuncertaintyoftheproposedmeasurementsys‐temdoesnotexceed0.5%.
Withthemaximumresolutionofpositioningthe measurementyokerelativetothesampleundertest— 200positionsovertheentire360‐degreerange—and consideringtheresponsetodifferentmagnetization ields,measurementscantakemanyhours.Under suchconditions,thedriftoftheLakeShore luxmeter model480canbeobserved.Thedriftaffectingthe measuredvalueswasaddressedbyautomaticcali‐brationofthe luxmeter,performedaftereachyoke rotation.
Mechanicalstressesareappliedusingahydraulic actuatorequippedwithapressurecontrolsystem, ensuringthestabilityoftheappliedmechanical stressesthroughoutthetest.
TheeffectofmechanicalstressesonM120‐27s grain‐orientedelectricalsteelwasmeasured,withten‐silestressesappliedintherollingdirection.Thespec‐imenwassubjectedto ivelevelsofstress:s1 =0 MPa,s2 =19.6 MPa,s3 =45.8 MPa,s4 =71.9 MPa,s5 =98.1MPa.Thesteelsheetwasmagnetized witha ieldstrengthof750A/minalignmentwiththe rollingdirection.Themeasuredhysteresisloopsare presentedinFigure6
Therelativemagneticpermeabilityofthesample duringeachstresswasdeterminedusingthefollowing relationship:
Figure7. TheMatlabSimulinkmodeloftheelectricaltransformersystemincludesamutualinductor.Theload,whichis switchedonhalfwaythroughthesimulation,ishighlightedwitharedbox
Itwasobservedthatasthestressappliedtothe sampleincreases,therelativemagneticpermeability decreases.Sincetheinductanceoftransformerwind‐ingsispartiallydependentonthemagneticperme‐abilityofthetransformercore,itisassumedthata decreaseinthemagneticpermeabilityofthestressed transformercorewillresultinaproportionaldecrease intheinductanceofboththeprimaryandsecondary windings.
5.ModelingtheImpactofMechanicalStress ontheFunctionalParametersofPower Transformers
Toinvestigatetheimpactofmechanicalstresson thefunctionalparametersofpowertransformers,the MutualInductormodelfromtheSimscapelibrarywas used.Themodelisgovernedbythefollowingequa‐tions[17]:
ThevoltageV1 ontheprimarywindingwasset to230VACRMSat50Hz.Initially,thevoltageV2 on thesecondarywindingwasano‐loadvoltagefromthe starttothemidpoint(t = 0.25s)ofthesimulation. Fromthemidpointtotheendofthesimulation,the secondarywindingwasautomaticallyloadedwitha resistorofR=8?.Forthepurposesofthesimula‐tion,thecoef icientofcoupling,whichdeterminesthe mutualinductanceM,wasassumedtobek = 0.9. Simulationswereconductedinthetimedomaint,with atotalsimulationtimeof0.5seconds.The igures illustratetheperiodfromt1 =0.2stot2 =0.3s.
V1 representstheinducedvoltageacrosstheprimary winding,whileV2 istheinducedvoltageacrossthe secondarywinding.I1 isthecurrententeringthepos‐itiveterminaloftheprimarywinding,andI2 isthe currentleavingthepositiveterminalofthesecondary winding.L1 andL2 aretheself‐inductancesofthe windings,Misthemutualinductance,kisthecoupling coef icient,andtistime.
Othertransformermodelsavailablein Simscape[18]werealsoevaluated,butnoneyielded satisfactoryresultsconsistentwiththevalidateddata. The inalcon igurationofthemodelusedforthe simulationsisdepictedinFigure7.
Theinitialparametersforthemodelwerederived fromarealtransformer,modelTE‐4812167,manu‐facturedbyHuaJiaElectricApplianceCo.,Ltd.The transformeroperateswithaprimarywindingvolt‐ageof230–240Vat50Hz,asecondarywinding voltageof12V,andamaximumcurrentof1.67A. Thepowerratingofthetransformeris20VA.The inductancesweremeasuredusingaHandheldLCR Meter,modelTH2822A,manufacturedbyTonghui. TheinductanceLPri oftheprimarywindingwasmea‐suredtobe11.836H,andtheinductanceLSec ofthe secondarywindingwas35.323mH.Basedonthese valuesandthereductioninmagneticpermeabilityof thetransformersheetundermechanicalstress,the decreaseininductanceofboththeprimaryandsec‐ondarywindingsofthesimulatedtransformerwas calculated.Itwasassumedthatthereisalinearrela‐tionshipbetweenstressandmagneticpermeabilityin theinitialrangesofmechanicalstress.However,for higherstressvalues,thisrelationshipbecomesnonlin‐ear.Thevaluesusedinthesimulationarepresentedin Table2.
Inordertothoroughlyanalyzeandinvestigatethe impactofmechanicalstressonthefunctionalparam‐etersofpowertransformers,theactivepowerPand reactivepowerQwerecalculated.Thesevalueswere obtainedusingadedicatedSimulinkblock.
Table2. InductancesLPri andLSec oftransformer windingsasafunctionofsimulatedtensilestresses appliedtothetransformercore
Figure8. Themodelofanelectricaltransformersystem inMatlabSimulink—thesectionofthemodel responsibleforcalculatingactivepowerP,reactive powerQ,andpowerfactorPF
Table3. Measuredcurrentamplitude(I)intheprimary winding
ThepowerfactorPFwascalculatedastheratioof realpowertoapparentpowerinagivenmoment,as showninEquation(5).
ThecomplexpowerSwasdeterminedasthevec‐torsumofactiveandreactivepower,expressedas:
Thesectionofthemodelresponsibleforcalculat‐ingpowerisdepictedinFigure8
6.ResultsofSimulationStudiesonMechanical StressEffectsinPowerTransformers
Simulationswereperformedfor5different stresses.Theresultsofthesimulationsareshownin Figures9,10,and11andsummarizedinTables3–7
Table 3 showsthecurrentamplitudeinthepri‐marywindingofthetransformerfordifferentlevelsof stress��expressedinMPa,measuredattwomoments intime,t1 andt2.Increasingthestresscausesan increaseintheamplitudeofthecurrentinthepri‐marywinding.Themostsigni icantincreaseincur‐rentamplitudeisobservedwhenthetensionrises from0to19.6MPa.
Figure9. Resultsofthesimulations—oscillogramsof currentandvoltageonthewindingsofthesimulated transformer:(a)measuredcurrentintheprimary windingasafunctionoftime,(b)measuredcurrentin thesecondarywindingasafunctionoftime,and(c) measuredvoltageinthesecondarywindingasa functionoftime
Table4. Measuredactivepower(P)intheprimary winding
Table4presentstheamplitudeandaveragevalue ofactivepowerintheprimarywindingofthetrans‐formeratdifferentstresslevels,measuredattwo momentsintime.Theamplitudeandtheaveragevalue ofactivepowerincreasewithincreasingstress.
Table5showstheamplitudeandaveragevalueof activepowerinthesecondarywindingofthetrans‐formerfordifferentstresslevels.
Figure10. Resultsofthesimulations—oscillogramsof poweronthewindingsofthetestedtransformer:(a) activepowerPintheprimarywinding,(b)activepower Pinthesecondarywinding,and(c)reactivepowerQin theprimarywinding
Figure11. Resultsofthesimulations—powerfactorPF oscillogramintheprimarywinding
Theamplitudeofactivepowerinthesecondary windingisrelativelystable,suggestingthatchanges instresshavelesseffectonthiswinding.Theaverage activepowervaluesarealmostconstantregardlessof thestresslevel,whichmayindicatethetransformer’s ef iciencyinmaintainingpowerinthesecondarycir‐cuit.
Table5. Measuredactivepower(P)inthesecondary winding
Table6. Measuredreactivepower(Q)intheprimary winding
Table7. Measuredpowerfactor(PF)intheprimary winding
Table 6 illustratestheaveragevaluesofreactive powerintheprimarywindingofthetransformerat differentstresslevels.Reactivepowerincreasesas stressincreases,suggestingmoresigni icantinef i‐ciencyathigherstresslevels.Reactivepowervalues arehighestforthemostcriticalstresses,whichmay requirecompensationtoimprovetransformeref i‐ciencyoroverloadreactivepowercompensationsys‐temsforsystemsthatdonotanticipatereactivepower increasingwithcorestress.
Table7presentstheamplitudeandaveragevalue ofthepowerfactorintheprimarywindingfordiffer‐entstresslevels.Thepowerfactortendstodecrease withincreasingstress,indicatingadecreaseinpower ef iciency.Theneedtoimprovethepowerfactormay requireadditionalmeasures,suchastheuseofreac‐tivepowercompensation.
Applyingmechanicalstresstothetransformer coresigni icantlychangesitselectricalparameters. Itisworthnotingthatwithinthetestedranges, theoutputparameters,suchascurrentandvolt‐age,remainlargelyunchanged.However,signi icant changesoccurintheprimarywinding,wherethe currentamplitudeincreasesbyalmost67%under extremestressconditions.Thesearecriticalchanges, astheyhaveaprofoundeffectonthekeyoperational parametersofthetransformer.
Thisphenomenoncanbeextremelydangerous becausethesechangesarenotdetectableontheload sideofthetransformer.Thechangesinoperating parametersalsoaffectthepowersystem,asthetrans‐former’sreactivepowerisseentoincreasebyalmost 53%whenoperatingunderload,potentiallylead‐ingtounwantedpowersystemshutdownsdueto overloading.
Itshouldbehighlightedthataquantitative descriptionofthein luenceofmechanicalstresseson thefunctionalpropertiesofthepowertransformer playsavitalroleinboththeef icientdevelopment ofthepowertransformersystemaswellasduring itssafeexploitation.Onthebasisoftheproposed quantitativemodelandtheproposedmethod ofexperimentalmeasurementsofthestress‐dependenceof2Drelativemagneticpermeability,it ispossibletopredicttheoperatingconditionsand properlyselectthemagneticmaterialforthecoreof thepowertransformer.
Thesimulationresultswerecomparedwithmea‐surementsofthematerial’spropertiesanditseffecton thetransformer.However,fullvalidationoftheimpact ofstressonthetransformerrequiresthepreparation ofateststandundernaturalconditionsthataccurately re lectthedevice’sactualoperatingconditions.
AUTHORS
PawełRękas –FacultyofMechatronics,Warsaw UniversityofTechnology,Poland,e‐mail: pawel.rekas.dokt@pw.edu.pl.
RomanSzewczyk∗ –ŁukasiewiczResearch Network–IndustrialResearchInstitutefor AutomationandMeasurementsPIAP,Poland,e‐mail: roman.szewczyk@pw.edu.pl.
TadeuszSzumiata –FacultyofMechanicalEngineer‐ing,DepartmentofPhysics,KazimierzPulaskiRadom University,Poland,e‐mail:t.szumiata@uthrad.pl.
MichałNowicki –DepartmentofMechatronics, RoboticsandDigitalManufacturing,Facultyof Mechanics,VilniusGediminasTechnicalUniversity, Lithuania,e‐mail:michal.nowicki@pw.edu.pl.
∗Correspondingauthor
References
[1] L.Cesky,F.Janicek,andJ.Kubicaetal.,“Over‐heatingofPrimaryandSecondaryCoilsofVolt‐ageInstrumentTransformers,” 201718thInternationalScienti icConferenceonElectricPower Engineering(EPE),May2017.doi:10.1109/ep e.2017.7967359.
[2] K.Liu,“IntelligentIdenti icationMethodof TransformerOverheatFaultinDistributionSub‐stationBasedonDeepLearningAlgorithmand InfraredTemperatureMeasurementTechnol‐ogy,” 20233rdInternationalConferenceonElectricalEngineeringandControlScience(IC2ECS), Dec.2023.doi:10.1109/ic2ecs60824.2023.104 93404.
[3] K.S.Kassi,I.Fofana,andF.Meghne ietal., “ImpactofLocalOverheatingonConventional andHybridInsulationsforPowerTransformers,” IEEETransactionsonDielectricsandElectrical Insulation,vol.22,no.5,pp.2543–2553,Oct. 2015.doi:10.1109/tdei.2015.005065.
[4] C.Qingsongetal.,“AnalysisofTransformer AbnormalHeatingBasedonInfraredThermal ImagingTechnology,” 20182ndIEEEConference onEnergyInternetandEnergySystemIntegration(EI2),Oct.2018.doi:10.1109/ei2.2018.8 582496.
[5] K.FurmanczykandM.Stefanich,“Overview ofMultiphasePowerConvertersforAerospace Applications,” SAETechnicalPaperSeries,Nov. 2008.doi:10.4271/2008‐01‐2878.
[6] M.Yamamoto,T.Kakisaka,andJ.Imaoka,“Tech‐nicalTrendofPowerElectronicsSystemsfor AutomotiveApplications,” JapaneseJournalof AppliedPhysics,vol.59,no.SG,Apr.2020.doi: 10.35848/1347‐4065/ab75b9.
[7] N.Leuning,S.Steentjes,andK.Hameyer,“Effect ofMagneticAnisotropyonVillariEffectin Non‐orientedFESIElectricalSteel,” International JournalofAppliedElectromagneticsandMechanics,vol.55,pp.23–31,Oct.2017.doi:10.3233/ja e‐172254.
[8] M.Yamagashira,S.Ueno,andD.Wakabayashi etal.,“VectorMagneticPropertiesandTwo‐DimensionalMagnetostrictionofVariousSoft MagneticMaterials,” InternationalJournalof AppliedElectromagneticsandMechanics,vol.44, nos.3–4,pp.387–400,Mar.2014.doi:10.3233/ jae‐141801.
[9] E.Beyer,L.Lahn,andC.Schepersetal.,“The In luenceofCompressiveStressAppliedbyHard CoatingsonthePowerLossofGrainOriented ElectricalSteelSheet,” JournalofMagnetismand MagneticMaterials,vol.323,no.15,pp.1985–1991,Aug.2011.doi:10.1016/j.jmmm.2011. 02.044.
[10] K.Fonteyn,A.Belahcen,andA.Arkkio,“Prop‐ertiesofElectricalSteelSheetsunderStrong MechanicalStress,” PollackPeriodica,vol.1,no. 1,pp.93–104,Apr.2006.doi:10.1556/pollack. 1.2006.1.7.
[11] P.Rȩkasetal.,“AMeasuringSetupforTest‐ingtheMechanicalStressDependenceofMag‐neticPropertiesofElectricalSteels,” Journalof MagnetismandMagneticMaterials,vol.577,p. 170791,Jul.2023.doi:10.1016/j.jmmm.2023. 170791.
[12] P.Marketos,S.Zurek,andA.J.Moses,“AMethod forDe iningtheMeanPathLengthoftheEpstein Frame,”IEEETransactionsonMagnetics,vol.43, no.6,pp.2755–2757,Jun.2007.doi:10.1109/ tmag.2007.894124.
[13] D.‐X.ChenandY.‐H.Zhu,“EffectiveMagneticPath LengthinEpsteinFrameTestofElectricalSteels,”
ReviewofScienti icInstruments,vol.93,no.5, May2022.doi:10.1063/5.0084859.
[14] P.Rękas,T.Szumiata,andR.Szewczyketal., “In luenceofMechanicalStressesNoncoaxial withtheMagnetizingFieldontheRelative MagneticPermeabilityofElectricalSteel,” IEEE TransactionsonMagnetics,vol.60,no.8,pp.1–9, Aug.2024.doi:10.1109/tmag.2024.3418668.
[15] T.Szumiata,P.Rekas,andM.Gzik‐Szumiataet al.,“TheTwo‐DomainModelUtilizingtheEffec‐tivePinningEnergyforModelingtheStrain‐DependentMagneticPermeabilityinAnisotropic Grain‐OrientedElectricalSteels,” Materials,vol. 17,no.2,p.369,Jan.2024.doi:10.3390/ma17 020369.
[16] EN10107:2022Grain‐OrientedElectricalSteel SheetandStripDeliveredintheFullyProcessed State.doi:10.3403/30092684.
[17] “MutualInductor,” MutualInductorinElectrical Systems–MATLAB, https://www.mathworks. com/help/simscape/ref/mutualinductor.html (accessedAug.11,2024).
[18] “SimscapeElectrical,” SimscapeElectricalDocumentation,https://www.mathworks.com/help /sps/index.html(accessedAug.11,2024).
NONLINEAROPTIMALANDMULTI‐LOOPFLATNESS‐BASEDCONTROLOF
NONLINEAROPTIMALANDMULTI‐LOOPFLATNESS‐BASEDCONTROLOF
Submitted:25th October2023;accepted:24th April2024
GerasimosRigatos,MasoudAbbaszadeh DOI:10.14313/JAMRIS/4‐2024/29
Abstract:
Inthisarticle,thecontrolproblemforomnidirectional3‐wheelautonomousmobilerobotsissolvedwiththeuse of(i)anonlinearoptimalcontrolmethod(ii)aflatness‐basedcontrolapproachwhichisimplementedinsucces‐siveloops.Toapplymethod(i)thatisnonlinearopti‐malcontrol,thedynamicmodeloftheomnidirectional 3‐wheelautonomousmobilerobotsundergoesapprox‐imatelinearizationateachsamplinginstantwiththe useoffirst‐orderTaylorseriesexpansionandthrough thecomputationoftheassociatedJacobianmatrix.The linearizationpointisdefinedbythepresentvalueofthe system’sstatevectorandbythelastsampledvalueof thecontrolinputsvector.Tocomputethefeedbackgains oftheoptimalcontrolleranalgebraicRiccatiequation isrepetitivelysolvedateachtime‐stepofthecontrol algorithm.Theglobalstabilitypropertiesofthenon‐linearoptimalcontrolmethodareproventhroughLya‐punovanalysis.Toimplementcontrolmethod(ii),that isflatness‐basedcontrolinsuccessiveloops,thestate‐spacemodeloftheomnidirectional3‐wheelautonomous mobilerobotisseparatedintochainedsubsystems,which areconnectedincascadingloops.Eachoneofthese subsystemscanbeviewedindependentlyasadifferen‐tiallyflatsystemandcontrolaboutitcanbeperformed withinversionofitsdynamicsasinthecaseofinput‐outputlinearizedflatsystems.Thestatevariablesof thepreceding(i‐th)subsystembecomevirtualcontrol inputsforthesubsequent(i+1‐th)subsystem.Inturn, exogenouscontrolinputsareappliedtothelastsubsys‐tem.Thewholecontrolmethodisimplementedinsuc‐cessiveloopsanditsglobalstabilitypropertiesarealso proventhroughLyapunovstabilityanalysis.Theproposed methodachievestrajectorytrackingandautonomous navigationfortheomnidirectional3‐wheelautonomous mobilerobotswithouttheneedofdiffeomorphismsand complicatedstate‐spacemodeltransformations.
Keywords: omnidirectional3‐wheelautonomousmobile robots,autonomousnavigation,differentialflatness properties,nonlinearoptimalcontrol,flatness‐based controlinsuccessiveloops,globalstability
The3‐wheelomnidirectionalmobilerobotisaspe‐cialtypeofroboticvehiclethatiscapableofperform‐ingmotioninalldirectionsofthehorizontalplane withoutnonholonomicconstraints[1‐4].
Ithasthreedegreesoffreedomassociatedwiththe cartesiancoordinatesofitscenterofgravityinthehor‐izontalplaneandwithitsorientation(heading)angle withrespecttothehorizontalaxisofaninertialcoor‐dinatesframe[5–7].Itsactuationcomesfromthree DCmotorswhichmakethewheelsofthemobilerobot turnatthespeci iedspeed[8–10].Suchmobilerobots canbedirectedpreciselyalongcomplicatedpathsand haveimprovedmaneuverability[11–14].Toachieve controlofsuchrobotsandtheirautonomousnaviga‐tionalongspeci ictrajectories,suitablevaluesforthe turnspeedofthewheelshavetobereachedwhich inturnareassociatedwithspeci icvoltageinputsof itsmotors[15–17].Omnidirectional3‐wheelmobile robotsaremetinseveralapplicationsassociatedwith patrollingandsecuritytasks,cleaninganddisinfecting tasks,withthetransferofproductsinwarehousesand withautomateddeliverytasks,aswellaswithseveral agriculturaltasksforinstancespraying,weedingor harvesting[18–20].Omnidirectional2‐wheelmobile robotshavenonlinearandmulti‐variabledynamics andso‐farseveralnonlinearcontrolmethodshave beenproposedaboutthem[21–25].Incertaincases autonomousnavigationof3‐wheelomnidirectional mobilerobotshasbeenbasedonstateestimation‐basedcontrolorvisualservoing[26–29].Thepresent articledevelopsandteststwonewcontrolmethods forthedynamicmodelof3‐wheelomnidirectional mobilerobots.Thesemethodsare(i)anovelnonlinear optimalcontrolapproach,(ii)a latness‐basedcontrol approachwhichisimplementedinsuccessiveloops. Bothcontrolschemesavoidchangesofstatevariables inthedynamicmodelofthe3‐wheelmobilerobotas wellascomplicatedtransformationsofthestatespace modelofthissystem.
Inthe irstcontrolmethodwhichisproposedby thepresentarticle,thatisnonlinearoptimalcontrol, thedynamicmodeloftheomnidirectional3‐wheel mobilerobotundergoesapproximatelinearization through irst‐orderTaylorseriesexpansion[30–31]. Thelinearizationprocesstakesplaceateachsampling instanceandcomputestherobot’sJacobianmatrices aroundatemporaryoperatingpointwhichisde ined bythepresentvalueofitsstatevectorandbythelast sampledvalueofthecontrolinputsvector[32–34].
Themodellingerrorwhichisduetotruncationof higher‐ordertermsintheTaylorseriesexpansionis consideredtobeaperturbationwhichisasymptot‐icallycompensatedbytherobustnessofthecontrol algorithm.Fortheapproximatelylinearizedmodelof theomnidirectionalrobotanH‐in initycontrolleris de ined.Thiscontrollerrepresentsamin‐maxdiffer‐entialgametakingplacebetween(i)therobot’scon‐trolinputswhichtrytominimizeacostfunctionthat containsaquadratictermofthestatevector’strack‐ingerrorand(ii)themodeluncertaintyandexoge‐nousperturbationtermswhichtrytomaximizethis costfunction.Tocomputethecontroller’sfeedback gainsanalgebraicRiccatiequationhastoberepeti‐tivelysolvedateachtime‐stepofthecontrolalgorithm [35–36].Theglobalstabilitypropertiesofthiscon‐trolschemeareproventhroughLyapunovanalysis. Ata irststagetheH‐in initytrackingperformance criterionisshowntoholdwhichsigni iesrobustness tomodeluncertaintyandperturbations.Moreover, undermoderateconditions,globalasymptoticstabil‐ityisproven[37–38].Thenonlinearoptimalcontrol methodachievesfastandaccuratetrackingofrefer‐encesetpointsbythestatevariablesofthemobile robotundermoderatevariationsofitscontrolinputs. Besides,itachievesminimizationofenergydispersion bytheactuatorsoftheomnidirectionalautonomous vehicleandtheimprovementofthisrobot’sautonomy andoperationalcapacity.
Inthesecondcontrolmethodwhichisproposed bythepresentarticle,thatis latness‐basedcontrolin successiveloops,itisshownthatthedynamicmodel ofthe3‐wheelomnidirectionalmobilerobotcanbe decomposedintosubsystemswhichareconnectedin chainedform[36].Inthischainedstate‐spacedescrip‐tionthestatevariablesofthesubsequent(i+1‐th)sub‐systembecomevirtualcontrolinputsinthepreced‐ing(i‐th)subsystem.Additionally,thevirtualcontrol inputsofthepreceding(i‐th)subsystembecomeset‐pointsforthesubsequent(i+1‐th)subsystem[39–40]. Itisalsoproventhateachoneofthesesubsystems, ifviewedindependently,isdifferentially lat.Thusit canbeconcludedthattheindividualsubsystemsare input‐outputlinearizableandastabilizing latness‐basedcontrollercanbedesignedforeachoneofthem throughinversionoftheirdynamics,asitiscommonly doneforinput‐outputlinearizedsystems.Thereal controlinputswhichareappliedtothemobilerobot arecomputedfromthelastsubsystem.Startingfrom it,thechainedstate‐spacedescriptionofthemobile robotistracedbackwards,thatisfromthelastto the irstsubsystem,withineachsamplingperiod.The globalstabilitypropertiesofthiscontrolmethodare proventhroughLyapunovanalysis.The latness‐based controlmethodissuboptimalinthesensethatitdoes nottargetexplicitlyattheminimizationofthevari‐ationsofthecontrolinputsoftherobot,howeverit alsoachievesprecisetrackingofreferencetrajecto‐riesinthe2Dplanebythe3‐wheelomnidirectional autonomousvehicle.
Thestructureofthepaperisasfollows:(i) inSection 2 thedynamicmodelofthe3‐wheel omnidirectionalmobilerobotisanalyzedandthe associatedstate‐spacemodelisformulated.In Section 3 anonlinearoptimalcontrollerisdesigned forthedynamicmodelofthe3‐wheelomnidirectional mobilerobotbasedonthecomputationofthesystem’s Jacobianmatrices.InSection4amulti‐loop latness‐basedcontrollerisdesignedforthedynamicmodel oftheautonomousroboticvehicleafterdecomposing itsstate‐spacemodelintosubsystenswhichare connectedinchainedformandwhichindependently satisfydifferential latnessproperties.InSection 5 simulationtestsareperformedtofurthercon irmthe ineperformanceofthetwoaforementionedcontrol methods.Finally,inSection6concludingremarksare stated.
2.DynamicModelofthe3‐wheelOmnidirec‐tionalMobileRobot
2.1.State‐spaceDescriptionofthe3‐wheelOmnidirec‐tionalRobot
Thediagramofthe3‐wheelomnidirectional mobilerobotisgiveninFigure1.Thediagramdepicts theposition(��,��)ofthecenterofgravityofthemobile robotinthehorizontalplaneandtherobot’sheading angle ��.Besides,thetwocoordinateframeswhich areusedinthede initionoftherobot’skinematic anddynamicmodelaregiven,namelythebody‐ ixed referenceframe ������������ andtheinertialreference frame ������������.Theparametersofthedynamic modeloftherobotareoutlinedinTable1[1],[3]:
Thedynamicmodelofthemobilerobotisgivenby [1],[3]:
(1)
Thedisturbancesvector ��=[����,����,����]�� com‐prisesfrictionforcesthustakingtheform ��= [��1̇��,�� 2̇��,�� 3��]��.Thecontrolinputsvectoris ��= [��1,��2,��3]��.Theinertiamatrix��isgivenby
TheCoriolismatrix��isgivenby
Thecontrolinputsgainmatrix��isgivenby
Figure1. Diagramofthe3‐wheelomnidirectionalmobilerobotandtheassociatedinertialandbody‐fixedreference frames
Table1. Dynamicmodelof3‐wheelomnidirectionalrobot
Parameter
De inition ��=[��,��,��]�� robot’spositionandorientationininertialframe ̇��=[̇��,̇��, ��]�� robot’slinearandangularvelocityininertialframe ̈��=[̈��,̈��, ��]�� robot’slinearandangularaccelerationininertialframe �� gearsreductionratiobetweenthemotorsandthewheels �� radiusofthewheelsoftheomnidirectionalrobot �� massoftheomnidirectionalrobot ��
momentofinertiaofwheel,gearandmotor
motors’torqueandbackEMFconstants
momentofinertiaforrobot’srotationaroundtheverticalaxis ���� radiusoftherobot’scylindricalsection ���� viscousfrictioncoef icientofwheel,gearandrotorshaft ��
wherecoef icients��0,��1,��2 arede inedas
Theinverseoftheinertiamatrix��isgivenby
resistanceofthemotors’armature
ThenfromEq.(1)onehasthefollowingstate‐spacedescription:
Afterintermediateoperations,thestate‐space descriptionofthe3‐wheelomnidirectionalrobot
Thestatevectorofthe3‐wheelomnidirectional robotisde inednextas
��3 (11)
andusingthisnotation,thedynamicmodelofthe mobilerobotiswrittenas
Thedynamicmodeloftheomnidirectional3‐wheelmobilerobotcanbealsowritteninthenonlin‐earaf ine‐in‐the‐inputstate‐spaceform ̇��=��(��)+�� 1(��)��1 +��2(��)��2 +��3(��)��3 (12) where��∈��6×1 ,��(��)∈��6×1 ,����(��)∈��6×1 and����∈�� for ��=1,2,3.Aboutvectors��(��)and����(��)��=1,2,3one hasthat
2.2.DifferentialFlatnessofthe3‐wheelOmnidirec‐tionalMobileRobot
Thedynamicmodelofthe3‐wheelomnidirec‐tionalmobilerobotthatwasgiveninEq.(11)isdiffer‐entially lat,with latoutputsvector��=[��1,��2,��3]�� or��=[��,��,��]��.Indeedfromthe irstthreerowsof thestate‐spacedescriptionofEq.(11)oneobtainsthat
(15)
whichsigni iesthatstatevariables��4,��5,��6 arediffer‐entialfunctionsofthe latoutputsvector��.Moreover, fromthelastthreerowsofthestate‐spacemodelof thesystemonehasthat
Themodellingerrorwhichisduetothetruncation ofhigher‐ordertermsfromtheTaylorseriesisconsid‐eredtobeaperturbationwhichisasymptoticallycom‐pensatedbytherobustnessofthecontrolalgorithm. Theinitialnonlinearstate‐spacemodelofthesystem ofEq.(14)intheforṁ��=��(��)+��(��)�� ,isturnedinto theequivalentlinearizedstate‐spaceform
�� (17) where�� isthecumulativedisturbancesvectorwhich maycomprise(i)modellingerrorduetothetrunca‐tionofhigher‐ordertermsfromtheTaylorseries,(ii) exogenousperturbations,(iii)sensormeasurement noiseofanydistribution.TheJacobianmatricesofthe systemaregivenby:
Thelinearizationapproachwhichhasbeenfol‐lowedforimplementingthenonlinearoptimalcontrol schemeresultsintoaquiteaccuratemodelofthesys‐tem’sdynamics.Considerforinstancethefollowing af ine‐in‐the‐inputstate‐spacemodel
wherealltermsthatappearintherightpartofEq. (16)aredifferentialfunctionsofthe latoutputsvec‐tor,consequentlythecontrolinputs ��1, ��2, ��3 are alsodifferentialfunctionsofthe latoutputsvector�� Therefore,theentiredynamicmodelofthe3‐wheel omnidirectionalmobilerobotisadifferentially lat system.
Thedifferential latnesspropertyisanimplicit proofofthesystem’scontrollability,aswellasofits input‐outputlinearizability.Italsoallowsforsolving thesetpointsde initionproblem.
3.1.ApproximateLinearizationoftheDynamicsofthe 3‐wheelOmnidirectionalRobot
Thedynamicmodelofthe3‐wheelomnidirec‐tionalmobilerobotundergoesapproximatelineariza‐tionaroundthetemporaryoperatingpoint (��∗,��∗) whichisde inedateachsamplinginstantbythe presentvalueofthesystem’sstatevector ��∗ andby thelastsampledvalueofthecontrolinputsvector ��∗.Thelinearizationprocessisbasedon irst‐order Taylorseriesexpansionandonthecomputationofthe associatedJacobianmatrices.
(20) where ��1 isthemodellingerrorduetotruncationof higherordertermsintheTaylorseriesexpansionof ��(��) and ��(��).Next,byde ining ��=[∇����(��)∣��
Moreoverbydenoting ��=−����
)��
+��1 aboutthecumulativemodellingerror termintheTaylorseriesexpansionprocedureonehas ̇��=����+����+ �� (22) whichistheapproximatelylinearizedmodelofthe dynamicsofthesystemofEq.(14).Theterm��(��∗)+ ��(��∗)��∗ isthederivativeofthestatevectorat(��∗,��∗) whichisalmostannihilatedby−����∗ . TheJacobianmatricesofthelinearizedmobile robotarecomputedasfollows:
ComputationoftheJacobianmatrix
ComputationoftheJacobianmatrix
Ateverytimeinstantthecontrolinput ��∗ is assumedtodifferfromthecontrolinput��appearing inEq.(27)byanamountequalto Δ��,thatis ��∗ = ��+Δ�� �� =������ +����∗ +��2 (28)
Thedynamicsofthecontrolledsystemdescribed inEq.(27)canbealsowrittenas ̇��=����+����+���� ∗ −����∗ +��1 (29)
andbydenoting ��3 =−����∗ +��1 asanaggregate disturbancetermoneobtains
∗ +��3 (30)
BysubtractingEq.(28)fromEq.(30)onehas
(31)
Bydenotingthetrackingerroras��=��−���� and theaggregatedisturbancetermas����=��3 −��2,the trackingerrordynamicsbecomes
(32)
ComputationoftheJacobianmatrix
where��isthedisturbanceinputgainmatrix.Forthe approximatelylinearizedmodelofthesystemastabi‐lizingfeedbackcontrollerisdeveloped.Thecontroller hastheform
(33)
with ��= 1 �� ������ where �� isapositivede initesym‐metricmatrixwhichisobtainedfromthesolutionof theRiccatiequation[30]
(25)
ComputationoftheJacobianmatrix
00 000000
(26)
3.2.StabilizingFeedbackControl
Afterlinearizationarounditscurrentoperating point(��∗,��∗),thedynamicmodelofthe3‐wheelomni‐directionalmobilerobotiswrittenas[30]
1 (27)
Parameter ��1 standsforthelinearizationerror inthe3‐wheelomnidirectionalmobilerobot’smodel appearingpreviouslyinEq.(27).Thereferenceset‐pointsforthe3‐wheelomnidirectionalmobilerobot’s statevectoraredenotedby���� =[���� 1,⋯,���� 6].Tracking ofthistrajectoryisachievedafterapplyingthecontrol input��∗
Thepreviouslyanalyzedconceptaboutthenonlin‐earoptimalcontrolloopforthethree‐wheelomnidi‐rectionalmobilerobotisgiveninFigure2 where��isapositivesemi‐de initesymmetricmatrix. WhereastheLinearQuadraticRegulator(LQR)isthe solutionofthequadraticoptimalcontrolproblem usingBellman’soptimalityprinciple,H‐in initycon‐trolisthesolutionoftheoptimalcontrolproblem undermodeluncertaintyandexternalperturbations. Thecostfunctionthatissubjecttominimizationinthe caseofLQRcomprisesaquadratictermofthestate vector’strackingerror,aswellasaquadraticterm ofthevariationsofthecontrolinputs.Inthecaseof H‐in initycontrolthecostfunctionisextendedwith theinclusionofaquadratictermofthecumulative disturbanceandmodeluncertaintyinputsthataffect themodelofthecontrolledsystem.
Inthecaseofthe3‐wheelomnidirectionalmobile robot,thevehicle’sdynamicmodelisnonlinearand isalsoaffectedbyuncertaintyandexternalperturba‐tions.Byapplyingapproximatelinearizationtothe3‐wheelomnidirectionalmobilerobot’sdynamicsone obtainsalinearstate‐spacedescriptionwhichissub‐jecttomodellingimprecisionandexogenousdistur‐bances.Therefore,onecanarriveatasolutionofthe relatedoptimalcontrolproblemonlybyapplyingthe H‐in initycontrolapproach.
Figure2. Diagramofthenonlinearoptimalcontrolschemefortheomnidirectionalthree‐wheelmobilerobot[Source: Authors’ownwork]
ItisalsonotedthatthesolutionoftheH‐in inity feedbackcontrolproblemforthe3‐wheelomnidi‐rectionalmobilerobotandthecomputationofthe worstcasedisturbancethatthiscontrollercansustain, comesfromsuperpositionofBellman’soptimality principlewhenconsideringthattherobotisaffected bytwoseparateinputs(i)thecontrolinput��(ii)the cumulativedisturbanceinput ��(��).Solvingtheopti‐malcontrolproblemfor �� thatisfortheminimum variation(optimal)controlinputthatachieveselimi‐nationofthestatevector’strackingerrorgives ��= 1 �� ��������.Equivalently,solvingtheoptimalcontrol problemfor ��,thatisfortheworstcasedisturbance thatthecontrolloopcansustaingives��= 1 ��2��������.
3.3.LyapunovStabilityAnalysis
ThroughLyapunovstabilityanalysisitwillbe shownthattheproposednonlinearcontrolscheme assures ��∞ trackingperformanceforthe3‐wheel omnidirectionalmobilerobot,andthatincaseof boundeddisturbancetermsasymptoticconvergence tothereferencesetpointsisachieved.Thetrack‐ingerrordynamicsforthe3‐wheelomnidirectional mobilerobotiswrittenintheform ̇��=����+����+�� �� (35) whereinthe3‐wheelomnidirectionalmobilerobot’s case ��=��∈��6×6 with �� beingtheidentitymatrix. Variable �� denotesmodeluncertaintiesandexternal disturbancesofthe3‐wheelomnidirectionalmobile robot’smodel.ThefollowingLyapunovequationis considered
(36)
where��=��−���� isthetrackingerror.Bydifferenti‐atingwithrespecttotimeoneobtains
Thepreviousequationisrewrittenas
Assumption:Forgivenpositivede initematrix �� andcoef icients��and��thereexistsapositivede inite matrix��,whichisthesolutionofthefollowingmatrix equation
Moreover,thefollowingfeedbackcontrollawis appliedtothesystem
BysubstitutingEq.(39)andEq.(40)intoEq. (38)andbyperformingintermediateoperationsone obtains
Lemma:Thefollowinginequalityholds
Proof :Thebinomial (����−
(43)
Thefollowingsubstitutionsarecarriedout:��=�� and��=��������andthepreviousrelationbecomes
Eq.(44)issubstitutedinthelastrowofEq.(41) andtheinequalityisenforced,thusgiving
(45)
Eq.(45)showsthatthe��∞ trackingperformance criterionissatis ied.Theintegrationof��from0to�� gives
Byfollowingthestagesofthestabilityproofone arrivesatEq.(45)whichshowsthattheH‐in inity trackingperformancecriterionholds.Byselectingthe attenuationcoef icient �� tobesuf icientlysmalland inparticulartosatisfy��2 <||��||2 ��/||��||2 onehasthat the irstderivativeoftheLyapunovfunctionisupper boundedby0.Thisconditionholdsateachsampling instanceandconsequentlyglobalstabilityforthecon‐trolloopcanbeconcluded.
Thenonlinearoptimalcontrolapproachexhibits advantagesagainstothercontrolschemesonecould haveconsideredforthedynamicsofthe3‐wheel omnidirectionalmobilerobot.Forinstance:(1)Com‐paredtofeedbacklinearizationwiththecomputed‐torquemethodthearticle’sapproachachievesmini‐mizationofthevariationsofthecontrolinputsand eliminationofenergydispersionbytheactuatorsof themobilerobot.Thearticle’snonlinearoptimalcon‐trolavoidsalsoinversetransformationswhichmay comeagainstsingularityproblems(2)comparedto thegloballinearization‐basedcontrolschemes(such asLiealgebra‐basedcontrolor latness‐basedcontrol withtransformationintocanonicalforms)itdoesnot requirecomplicatedchangesofstatevariables(diffeo‐morphisms)andtransformationofthesystem’sstate‐spacedescription.(3)comparedtoNonlinearModel PredictiveControl,theproposednonlinearoptimal controlmethodisofprovenglobalstabilityandthe convergenceofitsiterativesearchforanoptimum doesnotdependoninitializationandcontroller’s parametrization,(4)comparedtosliding‐modecon‐trolandbacksteppingcontroltheapplicationofthe nonlinearoptimalcontrolmethodisnotconstrained intodynamicalsystemsofaspeci icstate‐spaceform. Itisknownthatunlessthecontrolledsystemisfound intheinput‐outputlinearizedformthede initionof theassociatedslidingsurfacesisanempiricalproce‐dure.Besides,unlessthecontrolledsystemisfound inthebacksteppingintegral(triangular)form,the applicationofbacksteppingcontrolisnotpossible(5) comparedtoPIDcontrolthenonlinearoptimalcontrol methodisofprovenglobalstabilityanditsperfor‐manceisnotdependantonheuristics‐basedselec‐tionofparametersofthecontroller,(6)comparedto multiple‐modelsbasedoptimalcontrol,thenonlinear optimalcontrolmethodrequiresthecomputationof onlyonelinearizationpointandthesolutionofonly oneRiccatiequation.
(46)
Moreover,ifthereexistsapositiveconstant���� > 0suchthat∫∞ 0 ||��||2����≤��
thenonegets
(47)
Thus,theintegral ∫∞ 0 ||��||2 ������ isbounded.More‐over, ��(��) isboundedandfromthede initionofthe Lyapunovfunction��inEq.(36)itbecomesclearthat ��(��) willbealsoboundedsince ��(��)∈Ω�� = {��|��������≤2��(0)+��2����}.Accordingtotheabove andwiththeuseofBarbalat’sLemmaoneobtains ��������→∞��(��)=0.
4.DesignofaMulti‐loopFlatness‐based Controllerforthe3‐wheelRobot
Thedynamicmodelofthe3‐wheelomnidirec‐tionalmobilerobotcanbedecomposedintotwo chainedsubsystems Σ1 and Σ2,whileitcanbealso demonstratedthatforeachoneofthesesubsystems differential latnesspropertieshold.First,subsystem Σ1 isde ined,whichcomprisesthe irstthreerowsof thestate‐spacemodelofEq.(11).
Thefollowingsubvectorsandsubmatricesare de ined:
where ��1 isavirtualcontrolinputcomprisingthe statevariablesofthelastthreerowsofthestate‐spacemodelofEq.(11).Next,subsystem Σ2 iscon‐sideredandthefollowingsubvectorsandsubmatrices arede ined
Theproofofdifferential latnessforsubsystems Σ1 and Σ2 showsalsothatthesesubsystemsare input‐outputlinearizableandthattheycanbecon‐trolledthrough latness‐basedcontrolthatinverts theirdynamicsasitiscommonlydoneforinput‐outputlinearizedsystems.
Indeed,forsubsystemΣ1 ofEq.(51)thestabilizing feedbackcontrolistakentobe:
��1 =��1,3(��1,3)−1
(55)
where���� 1,3 isthereferencesetpointforsubsystemΣ1 andmatrix��1 >0isadiagonalmatrix��1∈��3×3 with positivediagonalelements��1,���� >0for��=1,2,3.The virtualcontrol��1 =��4,6 becomesreferencesetpoint ���� 4,6 forsubsystemΣ2.
ForsubsystemΣ2 ofEq.(52)thestabilizingfeed‐backcontrolistakentobe: ��=��4,6(��1,3,��4,6)−1 [̇���� 4,6 −��4,6(��1,3,��4,6)−��2(��4,6 −���� 4,6))] (56) where���� 4,6 isthereferencesetpointforsubsystemΣ2 andmatrix��2 >0isadiagonalmatrix��2∈��3×3 with positivediagonalelements��2,���� >0for��=1,2,3.The followingtrackingerrorvariablesarede inednext: ��
BysubstitutingthecontrolofEq.(55)intoEq.(51) oneobtains
where �� isthevectorofrealcontrolinputs.Asa resultoftheabove,thedynamicsoftheomnidirec‐tionalrobotcanbewrittenintheformoftwochained subsystems:
BysubstitutingthecontrolofEq.(56)intoEq.(52) oneobtains
=��4,6
Itcanbeproventhateachoneofsubsystems Σ1 andΣ2 isdifferentially lat.ForsubsystemΣ1 the lat outputis��1 =��1,3.Solvingforthevirtualcontrolinput ��1 onehas
��1 =��1,3(��1,3)−1[̇��1,3 −��1,3(��1,3)] (53)
whichsigni iesthat��1 =��4,6 isadifferentialfunction of ��1 =��1,3 (indeeditholds ��1 =��4,6 =̇��1,3). Consequently,subsystemΣ1 isdifferentially lat. ForsubsystemΣ2 the latoutputis��2 =��4,6.Con‐sideringthat��1,3isacoef icientsvectorandsolvingfor therealcontrolinput��onehas
��=��4,6(��1,3,��4,6)−1[̇��4,6 −��4,6(��1,3,��4,6)] (54) whichsigni iesthat��isadifferentialfunctionof��2 = ��4,6.Consequently,subsystemΣ2 isdifferentially lat.
Consequently,allstatevariablesofthedynamic modelofthe3‐wheelomnidirectionalmobilerobot convergetotheassociatedsetpointsandthesystem isgloballyasymptoticallystable.
Theglobalstabilitypropertiesof latness‐based controlinsuccessiveloopsforthedynamicmodel ofthe3‐wheelomnidirectionalmobilerobotcanbe alsoproventhroughLyapunovanalysis.Thefollowing Lyapunovfunctionisde ined:
BydifferentiatingtheLyapunovfunctionofEq. (59)intimeoneobtains
] (60)
BysubstitutinginEq.(60)thetrackingerror dynamicsproveninEq.(57)andEq.(58)oneobtains
(61)
Therefore,itholdsthat
(62)
while��=0ifandonlyif��1,3 =0and��4,6 =0.Thus, �� remainsnegativeand �� isastrictlydiminishing functionwhichconvergesasymptoticallyto0.Thusit holds
(63)
Itisalsoproventhattheconvergenceofthetrack‐ingerrortothezeroequilibriumisexponential.The Lyapunovfunctionofthecontrolloopiswrittenas:
(64)
where ���� ��=1,⋯,3 arethetrackingerrorsforthe statevariablesofthemobilerobotassociatedwith positionandorientationand ���� ��=4,⋯,6 arethe trackingerrorsforthestatevariablesofthemobile robotassociatedwithlinearandangularvelocities. Equivalently,the irst‐ordertime‐derivativeofthe Lyapunovfunctioniswrittenas
(65)
Oncethestate‐spacemodelofthesystemhas beenseparatedintochainedsubsystemswhichindi‐viduallysatisfythedifferential latnessproperty, latness‐basedcontrolisimplementedateachtime‐stepthroughthefollowingsteps:(i)thestatevector ofthesubsequent(��+1)‐thsubsystembecomesvir‐tualcontrolinputtothepreceding��‐thsubsystem,(ii) Equivalently,thevirtualcontrolinputofthepreceding ��‐thsubsystembecomessetpointforthesubsequent (��+1)‐thsubsystem,(iii)thevalueofthevirtualcon‐trolinputforeachsubsystemiscomputedbyinverting thedynamicsofthissubsystemandbyselectingfeed‐backgainswhichallowforeliminatingtheassociated localtrackingerror,(iv)therealcontrolinputiscom‐putedfromthelastsubsystembyinvertingagainthe dynamicsofthissubsystem(v)therealcontrolinput makesimplicitlyuseofthevirtualcontrolinputsof allprecedingsubsystems.Itsstabilizingeffectsappear bytracingthesubsystemsofthestate‐spacemodel backwardsfromthelasttothe irstone.
5.1.ResultsonNonlinearOptimalControlforthe3‐DOF OmnidirectionalRobot
Theproposednonlinearoptimalcontrolmethod forthe3‐wheelomnidirectionalmobilerobothasbeen testedanditsperformancehasbeenfurthercon‐irmedthroughsimulationtests.Toimplementthe nonlinearoptimalcontrolmethodthealgebraicRic‐catiequationofEq.(39)hadtoberepetitivelysolved ateachtime‐stepofthecontrolalgorithm,withtheuse ofMatlab’sfunction aresolv().TheJacobianmatrices ofthesystemwerealsoupdatedateachsampling instance.Indicativevaluesabouttheparametersofthe mobilerobotwereasfollows:
where��1,�� >0��=1,⋯,3arethediagonalelements ofgainmatrix ��1 and ��2,�� >0��=4,⋯,6 arethe diagonalelementsofgainmatrix��2.Bydenotingthe minimumoftheabove‐notedelementsofthefeedback gainmatricesas��������,thatis �������� =������{��1,�� ∶��=1,⋯,3and��2,�� ∶��=4,⋯,6} (66) andusingEq.(65)oneobtainsthat ��≤−��������[∑4 ��=1��2 �� +∑6 ��=4��2 �� ] ⇒��≤−2����������⇒��+2��
(67)
FromEq.(67)onecandemonstratetheexponen‐tialconvergenceoftheLyapunovfunction��to0.
Finally,adiagramwiththeimplementationstages ofmulti‐loop latness‐basedcontrolmethodisgivenin Figure3
=0.02.Theobtainedresultsare depictedinFigure 4 toFigure 19.Themeasurement unitsforthecartesiancoordinatesoftheroboticvehi‐clewereinmeters(m)andforitsrotationangleswere inradians(rad).Themeasurementunitsforlinear velocityvariableswerem/secwhileangularvelocity variablesweremeasuredinrad/sec.Therealvalues ofthestatevariablesofthe3‐wheelomnidirectional mobilerobotaredepictedinblue,theirestimated valueswhichhavebeenprovidedbytheH‐in inity KalmanFilterareplottedingreenwhiletheassociated referencesetpointsareprintedinred.Thesimula‐tionresultshaveshownthatthenonlinearoptimal controlschemeachievesfastandaccuratetracking ofsetpointsundermoderatevariationsofthecontrol inputs.Theobtainedresultscontain2Ddiagramsare givenaboutthetrackingofreferencetrajectoriesby thecenter‐of‐gravityoftheroboticvehicle.
Figure3. Diagramwiththeimplementationstagesofmulti‐loopflatness‐basedcontrolofthethree‐wheel omnidirectionalmobilerobot[Source:Authors’ownwork]
0
Figure4. Nonlinearoptimalcontrol‐Trackingofsetpoint1forthe3‐wheelomnidirectionalmobilerobot(a)convergence ofstatevariables ��1 to ��3 totheirreferencesetpoints(redline:setpoint,blueline:realvalue,greenline:estimated value),(b)convergenceofstatevariables ��4 to ��6 totheirreferencesetpoints(redline:setpoint,blueline:realvalue, greenline:estimatedvalue)[Source:Authors’ownwork]
Thetransientperformanceofthecontrolmethod dependsmainlyofgains ��, �� andongainmatrix �� whichappearintheRiccatiequationofEq.(39).Actu‐ally,byassigningsmallvaluesto �� onecanachieve eliminationofthestatevector’strackingerror,while byassigningrelativelylargevaluestothediagonal elementsofmatrix��onecanachievefastconvergence ofstatevariablestosetpoints.Moreover,thesmallest valueof �� forwhichonecanobtainavalidsolution fortheabove‐notedRiccatiequation(intheformof positive‐de initeandsymmetricmatrix ��)istheone thatprovidesthecontrolloopwithmaximumrobust‐ness.Thenonlinearoptimalcontrolmethodresults intominimizationofenergydispersionbytheactua‐torsofthe3‐wheelomnidirectionalmobilerobot,thus increasingtheautonomyandoperationalcapacityof theautonomousvehicle.Themethodexhibitsglobal (andnotlocal)stabilitypropertiesasitiscon irmedby itsabilitytotracktime‐varyingandabruptlychanging setpoints.
Figure5. Nonlinearoptimalcontrol‐Trackingofsetpoint1forthe3‐wheelomnidirectionalmobilerobot(a)variationsof thecontrolinputs ��1 to ��3 (b)trackingofthereferencetrajectorybythecenterofgravityofthemobilerobotinthe2D xyplane[Source:Authors’ownwork]
Figure6. Nonlinearoptimalcontrol‐Trackingofsetpoint2forthe3‐wheelomnidirectionalmobilerobot(a)convergence ofstatevariables ��1 to ��3 totheirreferencesetpoints(redline:setpoint,blueline:realvalue,greenline:estimated value),(b)convergenceofstatevariables ��4 to ��6 totheirreferencesetpoints(redline:setpoint,blueline:realvalue, greenline:estimatedvalue)[Source:Authors’ownwork]
Figure7. Nonlinearoptimalcontrol‐Trackingofsetpoint2forthe3‐wheelomnidirectionalmobilerobot(a)variationsof thecontrolinputs ��1 to ��3 (b)trackingofthereferencetrajectorybythecenterofgravityofthemobilerobotinthe2D xyplane[Source:Authors’ownwork]
Figure8. Nonlinearoptimalcontrol‐Trackingofsetpoint3forthe3‐wheelomnidirectionalmobilerobot(a)convergence ofstatevariables ��1 to ��3 totheirreferencesetpoints(redline:setpoint,blueline:realvalue,greenline:estimated value),(b)convergenceofstatevariables ��4 to ��6 totheirreferencesetpoints(redline:setpoint,blueline:realvalue, greenline:estimatedvalue)[Source:Authors’ownwork]
Figure9. Nonlinearoptimalcontrol‐Trackingofsetpoint3forthe3‐wheelomnidirectionalmobilerobot(a)variationsof thecontrolinputs ��1 to ��3 (b)trackingofthereferencetrajectorybythecenterofgravityofthemobilerobotinthe2D xyplane[Source:Authors’ownwork]
Figure10. Nonlinearoptimalcontrol‐Trackingofsetpoint4forthe3‐wheelomnidirectionalmobilerobot(a) convergenceofstatevariables ��1 to ��3 totheirreferencesetpoints(redline:setpoint,blueline:realvalue,greenline: estimatedvalue),(b)convergenceofstatevariables ��4 to ��6 totheirreferencesetpoints(redline:setpoint,blueline:real value,greenline:estimatedvalue)[Source:Authors’ownwork]
Figure11. Nonlinearoptimalcontrol‐Trackingofsetpoint4forthe3‐wheelomnidirectionalmobilerobot(a)variations ofthecontrolinputs ��1 to ��3 (b)trackingofthereferencetrajectorybythecenterofgravityofthemobilerobotinthe 2Dxyplane[Source:Authors’ownwork]
Figure12. Nonlinearoptimalcontrol‐Trackingofsetpoint5forthe3‐wheelomnidirectionalmobilerobot(a) convergenceofstatevariables ��1 to ��3 totheirreferencesetpoints(redline:setpoint,blueline:realvalue,greenline: estimatedvalue),(b)convergenceofstatevariables ��4 to ��6 totheirreferencesetpoints(redline:setpoint,blueline:real value,greenline:estimatedvalue)[Source:Authors’ownwork]
Figure13. Nonlinearoptimalcontrol‐Trackingofsetpoint5forthe3‐wheelomnidirectionalmobilerobot(a)variations ofthecontrolinputs ��1 to ��3 (b)trackingofthereferencetrajectorybythecenterofgravityofthemobilerobotinthe 2Dxyplane[Source:Authors’ownwork]
Figure14. Nonlinearoptimalcontrol‐Trackingofsetpoint6forthe3‐wheelomnidirectionalmobilerobot(a) convergenceofstatevariables ��1 to ��3 totheirreferencesetpoints(redline:setpoint,blueline:realvalue,greenline: estimatedvalue),(b)convergenceofstatevariables ��4 to ��6 totheirreferencesetpoints(redline:setpoint,blueline:real value,greenline:estimatedvalue)[Source:Authors’ownwork]
Figure15. Nonlinearoptimalcontrol‐Trackingofsetpoint6forthe3‐wheelomnidirectionalmobilerobot(a)variations ofthecontrolinputs ��1 to ��3 (b)trackingofthereferencetrajectorybythecenterofgravityofthemobilerobotinthe 2Dxyplane[Source:Authors’ownwork]
Figure16. Nonlinearoptimalcontrol‐Trackingofsetpoint7forthe3‐wheelomnidirectionalmobilerobot(a) convergenceofstatevariables ��1 to ��3 totheirreferencesetpoints(redline:setpoint,blueline:realvalue,greenline: estimatedvalue),(b)convergenceofstatevariables ��4 to ��6 totheirreferencesetpoints(redline:setpoint,blueline:real value,greenline:estimatedvalue)[Source:Authors’ownwork]
Figure17. Nonlinearoptimalcontrol‐Trackingofsetpoint7forthe3‐wheelomnidirectionalmobilerobot(a)variations ofthecontrolinputs ��1 to ��3 (b)trackingofthereferencetrajectorybythecenterofgravityofthemobilerobotinthe 2Dxyplane[Source:Authors’ownwork]
Figure18. Nonlinearoptimalcontrol‐Trackingofsetpoint8forthe3‐wheelomnidirectionalmobilerobot(a) convergenceofstatevariables ��1 to ��3 totheirreferencesetpoints(redline:setpoint,blueline:realvalue,greenline: estimatedvalue),(b)convergenceofstatevariables ��4 to ��6 totheirreferencesetpoints(redline:setpoint,blueline:real value,greenline:estimatedvalue)[Source:Authors’ownwork]
Figure19. Nonlinearoptimalcontrol‐Trackingofsetpoint8forthe3‐wheelomnidirectionalmobilerobot(a)variations ofthecontrolinputs ��1 to ��3 (b)trackingofthereferencetrajectorybythecenterofgravityofthemobilerobotinthe 2Dxyplane[Source:Authors’ownwork]
Figure20. Multi‐loopflatness‐basedcontrol‐Trackingofsetpoint1forthe3‐wheelomnidirectionalmobilerobot(a) convergenceofstatevariables ��1 to ��3 totheirreferencesetpoints(redline:setpoint,blueline:realvalue,greenline: estimatedvalue),(b)convergenceofstatevariables ��4 to ��6 totheirreferencesetpoints(redline:setpoint,blueline:real value,greenline:estimatedvalue)[Source:Authors’ownwork]
Figure21. Multi‐loopflatness‐basedcontrol‐Trackingofsetpoint1forthe3‐wheelomnidirectionalmobilerobot(a) variationsofthecontrolinputs ��1 to ��3 (b)trackingofthereferencetrajectorybythecenterofgravityofthemobile robotinthe2Dxyplane[Source:Authors’ownwork]
Figure22. Multi‐loopflatness‐basedcontrol‐Trackingofsetpoint2forthe3‐wheelomnidirectionalmobilerobot(a) convergenceofstatevariables ��1 to ��3 totheirreferencesetpoints(redline:setpoint,blueline:realvalue,greenline: estimatedvalue),(b)convergenceofstatevariables ��4 to ��6 totheirreferencesetpoints(redline:setpoint,blueline:real value,greenline:estimatedvalue)[Source:Authors’ownwork]
Figure23. Multi‐loopflatness‐basedcontrol‐Trackingofsetpoint2forthe3‐wheelomnidirectionalmobilerobot(a) variationsofthecontrolinputs ��1 to ��3 (b)trackingofthereferencetrajectorybythecenterofgravityofthemobile robotinthe2Dxyplane[Source:Authors’ownwork]
Figure24. Multi‐loopflatness‐basedcontrol‐Trackingofsetpoint3forthe3‐wheelomnidirectionalmobilerobot(a) convergenceofstatevariables ��1 to ��3 totheirreferencesetpoints(redline:setpoint,blueline:realvalue,greenline: estimatedvalue),(b)convergenceofstatevariables ��4 to ��6 totheirreferencesetpoints(redline:setpoint,blueline:real value,greenline:estimatedvalue)[Source:Authors’ownwork]
Figure25. Multi‐loopflatness‐basedcontrol‐Trackingofsetpoint3forthe3‐wheelomnidirectionalmobilerobot(a) variationsofthecontrolinputs ��1 to ��3 (b)trackingofthereferencetrajectorybythecenterofgravityofthemobile robotinthe2Dxyplane[Source:Authors’ownwork]
Figure26. Multi‐loopflatness‐basedcontrol‐Trackingofsetpoint4forthe3‐wheelomnidirectionalmobilerobot(a) convergenceofstatevariables ��1 to ��3 totheirreferencesetpoints(redline:setpoint,blueline:realvalue,greenline: estimatedvalue),(b)convergenceofstatevariables ��4 to ��6 totheirreferencesetpoints(redline:setpoint,blueline:real value,greenline:estimatedvalue)[Source:Authors’ownwork]
Figure27. Multi‐loopflatness‐basedcontrol‐Trackingofsetpoint4forthe3‐wheelomnidirectionalmobilerobot(a) variationsofthecontrolinputs ��1 to ��3 (b)trackingofthereferencetrajectorybythecenterofgravityofthemobile robotinthe2Dxyplane[Source:Authors’ownwork]
Figure28. Multi‐loopflatness‐basedcontrol‐Trackingofsetpoint5forthe3‐wheelomnidirectionalmobilerobot(a) convergenceofstatevariables ��1 to ��3 totheirreferencesetpoints(redline:setpoint,blueline:realvalue,greenline: estimatedvalue),(b)convergenceofstatevariables ��4 to ��6 totheirreferencesetpoints(redline:setpoint,blueline:real value,greenline:estimatedvalue)[Source:Authors’ownwork]
Figure29. Multi‐loopflatness‐basedcontrol‐Trackingofsetpoint5forthe3‐wheelomnidirectionalmobilerobot(a) variationsofthecontrolinputs ��1 to ��3 (b)trackingofthereferencetrajectorybythecenterofgravityofthemobile robotinthe2Dxyplane[Source:Authors’ownwork]
Figure30. Multi‐loopflatness‐basedcontrol‐Trackingofsetpoint6forthe3‐wheelomnidirectionalmobilerobot(a) convergenceofstatevariables ��1 to ��3 totheirreferencesetpoints(redline:setpoint,blueline:realvalue,greenline: estimatedvalue),(b)convergenceofstatevariables ��4 to ��6 totheirreferencesetpoints(redline:setpoint,blueline:real value,greenline:estimatedvalue)[Source:Authors’ownwork]
Figure31. Multi‐loopflatness‐basedcontrol‐Trackingofsetpoint6forthe3‐wheelomnidirectionalmobilerobot(a) variationsofthecontrolinputs ��1 to ��3 (b)trackingofthereferencetrajectorybythecenterofgravityofthemobile robotinthe2Dxyplane[Source:Authors’ownwork]
Figure32. Multi‐loopflatness‐basedcontrol‐Trackingofsetpoint7forthe3‐wheelomnidirectionalmobilerobot(a) convergenceofstatevariables ��1 to ��3 totheirreferencesetpoints(redline:setpoint,blueline:realvalue,greenline: estimatedvalue),(b)convergenceofstatevariables ��4 to ��6 totheirreferencesetpoints(redline:setpoint,blueline:real value,greenline:estimatedvalue)[Source:Authors’ownwork]
Figure33. Multi‐loopflatness‐basedcontrol‐Trackingofsetpoint7forthe3‐wheelomnidirectionalmobilerobot(a) variationsofthecontrolinputs ��1 to ��3 (b)trackingofthereferencetrajectorybythecenterofgravityofthemobile robotinthe2Dxyplane[Source:Authors’ownwork]
Figure34. Multi‐loopflatness‐basedcontrol‐Trackingofsetpoint8forthe3‐wheelomnidirectionalmobilerobot(a) convergenceofstatevariables ��1 to ��3 totheirreferencesetpoints(redline:setpoint,blueline:realvalue,greenline: estimatedvalue),(b)convergenceofstatevariables ��4 to ��6 totheirreferencesetpoints(redline:setpoint,blueline:real value,greenline:estimatedvalue)[Source:Authors’ownwork]
Figure35. Multi‐loopflatness‐basedcontrol‐Trackingofsetpoint8forthe3‐wheelomnidirectionalmobilerobot(a) variationsofthecontrolinputs ��1 to ��3 (b)trackingofthereferencetrajectorybythecenterofgravityofthemobile robotinthe2Dxyplane[Source:Gerasimosownwork]
5.2.ResultsonMulti‐loopFlatness‐basedControlfor the3‐DOFOmnidirectionalRobot
Resultsaboutthetrackingaccuracyandthespeed ofconvergencetosetpointsofthe latness‐basedcon‐trolmethodinsuccessive‐loops,inthecaseofthe 3‐wheelomnidirectionalmobilerobot,areshownin Figure20toFigure35.Themeasurementunitsforthe cartesiancoordinatesoftheroboticvehiclewerein meters(m)andforitsorientationanglewereinradi‐ans(rad).Themeasurementunitsforlinearvelocity variableswerem/secwhileangularvelocityvariables weremeasuredinrad/sec.
Therealvaluesofthestatevariablesofthe autonomousroboticvehiclearedepictedinblue,their estimatedvalueswhichhavebeenprovidedbythe H‐in inityKalmanFilterareplottedingreenwhile theassociatedreferencesetpointsareprintedinred. Thesimulationresultshaveshownthat latness‐based controlinsuccessiveloopsachievesprecisetracking ofsetpointsundermoderatevariationsofthecon‐trolinputs.Onceagain,2Ddiagramsaregivenabout thetrackingofreferencetrajectoriesbythecenter‐of‐gravityofthemobilerobot.
Itcanbenoticedagain,thatunderthiscontrol schemeoneachievesfastandprecisetrackingofref‐erencesetpointsforallstatevariablesofthedynamic modelofthe3‐wheelomnidirectionalmobilerobot withoutchangesofstatevariablesandwithoutstate space‐modeltransformations.Itisnoteworthy,that throughthestagesofthismethodonesolvesalsothe setpointsde initionproblemforallstatevariablesof theautonomousroboticvehicle.Actually,theselection ofsetpointsforthestatevariablesofthe irstsubsys‐teminthechainedstate‐spaceformisunconstrained.
Ontheothersidebytakingthestatevariablesof thesubsequentsubsystemtobevirtualcontrolinputs fortheprecedingsubsystemonecan indalsothe setpointsofthesubsequentsubsystem(thestatevari‐ablesofthesubsequentsubsystemshouldconvergeto thevirtualcontrolinputthatstabilizesitspreceding subsystem).Thespeedofconvergenceofthestate variablesofthedynamicmodeloftheroboticvehicle under latness‐basedcontrolimplementedinsucces‐siveloopsisdeterminedbytheselectionofvaluesfor thediagonalgainmatrices��1,��2
Theaimofthepresentarticlehasbeentodevelop novelnonlinearcontrolmethodsforthedynamic modelofthe3‐wheelomnidirectionalmobilerobot whichdonotneedchangesofstatevariablesorcom‐plicatedstate‐spacemodeltransformations.Tothis endthefollowingtwocontrolmethodshavebeen developedandtested(i)nonlinearoptimalcontrol, (ii) latness‐basedcontrolinsuccessiveloops.Inthe nonlinearoptimalcontrolmethodthedynamicmodel oftheomnidirectionalmobilerobothasundergone irstapproximatelinearizationwiththeuseof irst‐orderTaylorseriesexpansionandthroughthecom‐putationoftheassociatedJacobianmatrices.This linearizationprocesswastakingplaceateachsam‐plinginstantaroundatime‐varyingoperatingpoint whichwasde inedbythepresentvalueofthesys‐tem’sstatevectorandbythelastsampledvalueof thecontrolinputsvector.Forthelinearizedmodelof themobilerobotanH‐in inityfeedbackcontrollerhas beendesigned.TheH‐in initycontrolmethodsigni‐iesamin‐maxdifferentialgamebetweenthecontrol andthedisturbanceinputsandachievessolutionof theassociatedoptimalcontrolproblemundermodel uncertaintyandexogenousperturbations.
TocomputethegainsoftheH‐in initycontroller analgebraicRiccatiequationhadtoberepetitively solvedateachsamplingperiod.Theglobalstability propertiesofthenonlinearoptimalcontrolmethod havebeenproventhroughLyapunovanalysis.
Inthemulti‐loop latness‐basedcontrolmethod, thedynamicmodelofthe3‐wheelomnidirectional mobilerobotwasseparatedintosubsystemscon‐nectedinchainedform.Thestatevectorofthesubse‐quent(i+1‐th)subsystemwasavirtualcontrolinput forthepreceding(i‐th)subsystem.Moreover,thevir‐tualcontrolinputforthepreceding(i‐th)subsystem wasthesetpointforthesubsequent(i+1‐th)subsys‐tem.Itwasproventhatallsubsystemsinthischained state‐spacestructureweredifferentially lat.Thusit wascon irmedthattheywereinput‐outputlineariz‐ableandthatastabilizing latness‐basedcontroller couldbedesignedforeachoneofthembyinverting theirdynamics,asitiscommonlydoneforinput‐outputlinearizedsystems.Therealcontrolinputs oftheomnidirectionalmobilerobotwerecomputed fromthelastsubsysteminthischainedstate‐space structure.Inthiscomputationthevirtualcontrol inputsoftheprecedingsubsystemshadtobetraced backwards,fromthelasttothe irstsubsystem,within eachsamplingperiod.Theglobalstabilityproperties ofthemulti‐loop latness‐basedcontrolschemewere alsoproventhroughLyapunovanalysis.
AUTHORS
GerasimosRigatos –UnitofIndustrialAutoma‐tion,IndustrialSystemsInstitute,26504,RionPatras, Greece,e‐mail:grigat@ieee.org.
MasoudAbbaszadeh –Dept.ofECSEngineering, RensselaerPolytechnicInstitute,12065,Troy,New YorkUSA,e‐mail:masouda@ualberta.ca.
References
[1] C.Ren,X.Li,X.YiangandS.Ma, Extendedstate observer-basedsliding-modecontrolofanomnidirectionalmobilerobotwithfrictioncompensation,IEEETransactionsonIndustrialElectron‐ics,vol.66,no.17,pp.9580‐9489,2019.
[2] H.KimandB.K.Kim, Onlineminimumenergy trajectoryplanningandcontrolonastraightlinepathforthree-wheeledomnidirectional mobilerobots,IEEETransactionsonIndustrial Electronics,vol.61,no.9,pp.4721‐4779,2014.
[3] C.Ren,Y.DingandS.Ma, Astructureimproved extendedstateobserver-basedcontrolwith applicationtoanomnidirectionalmobile robot,ISATransactions,Elsevier,vol.101,pp. 335‐345,2020.
[4] J.C.LinsBarreto,A.G.ScolariConceicao,C.E.T. Dorea,L.MartinezandE.R.dePieri, Design andimplementationofModelPredictiveControlwithfrictioncompensationofanomnidirectionalmobilerobot,IEEE/ASMETransactions
onMechatronics,vol.19,no.2,pp.462‐476, 2014.
[5] K.B.KimandB.K.Kim, Minimum-timetrajectory ofthree-wheelomnidirectionalmobilerobots followingaboundedcurvaturepathwithareferencedheadingpro ile,IEEETransactionson Robotics,vol.27,no.6,pp.800‐808,2011.
[6] M.El‐Sayyah,M.E.SaadandM.Saad, Enhanced MPCforomnidirectionalrobotmotiontracking usingLaguerrefunctionsandnon-iterativelinearization,IEEEAccess,vol.10,pp.118290‐118302,2022.
[7] H.C.Huang, SoPCbasedparallelACOalgorithm anditsapplicationtooptimalmotioncontroller designforintelligentomnidirectionalmobile robot,IEEETransactionsonIndustrialInfor‐matics,vol.9,no.4,pp.1828‐1835,2013.
[8] M.Hamaguchi, Dampingandtransfercontrol systemwithparallellinkagemechanism-based activevibrationreducerforomnidirectional wheeledrobots,IEEE/ASMETransactionson Mechatronis,vol.23,no.4,pp.2424‐2435, 2019.
[9] T.Kalmar‐Nagy,R.D.AndreuandPGanguly, Near-optimaldynamictrajectorygeneration andcontrolofanomnidirectionalvehicle, RoboticsandAutonomousSystems,Elsevier, vol.46,pp.47‐64,2004.
[10] F.Dong,D.Jin,X.Zhou,andJ.Han, Adaptive robustconstraintfollowingcontrolforomnidirectionalmobilerobot:Anindirectapproach, IEEEAccess,vol.9,pp.8877=8889,2021.
[11] J.Liu,J.Zhu,R.L.WilliamsandJ.Wu, Omnidirectionalmobilerobotcontrollerbasedontrajectorylinearization,RoboticsandAutonomous Systems,Elsevier,vol.56,pp.461‐479,2005.
[12] V.T.Dinh,H.Nguyen,S.M.Shin,M.K,Kim,S.B. KimandG.S.Bynn, Trackingcontrolofomnidirectionalmobileplatformwithdisturbance usingdiffeentialsliding-modecontroller,Journal ofPrecisionEngineeringSpringer,vol,19,no,5, pp.39‐48,2012.
[13] N.HacenandB.Merdal, Motionanalysisand controlofomnidirectionalmobilerobot,Journal ofControl,AutomationandElectricalSystems, Springer,vol.30,pp.194‐213,2019.
[14] D.J.Balkom,P.A.KavathekarandM.T.Mason, Time-optimaltrajectoryforanomnidirectional vehicle,InternationalJournalofRobotics Research,SagePublications,vol.25,no.10,pp. 985‐999,2006.
[15] C.A.Huang,H.M.WuandW.H.Hung, Software/Hardware-basedhierarchical initetimesliding-modecontrolwithinput-saturation foranomnidirectionalautonomousmobile robot,IEEEAccess,vol.7,pp.90254‐90267, 2019.
[16] C.Ren,Y.Ding,S.Ma,L.HuandX.Zhu, Passivitybasedtrackingcontrolofanomnidirectional mobilerobotusingonegeometricalparameter, ControlEngineeringPractice,Elsevier,vol.90, pp.160‐168,2019.
[17] H.M.WuandM.Karkouh, Frictionalforcesand torquecompensation-basedcascadingslidingmodetrackingcontrolforanuncertainomnidirectionalmobilerobot,MeasurementandCon‐trol,SagePublications,vol.55,no.3,pp.178‐188,2022.
[18] C.Ren,H.Jiang,C.MaandS.Ma, Conditional disturbancerejection-basedcontrolforanomnidirectionalmobilerobot:Anenergyperspective, IEEERoboticsandAutomationLetters,vol.7, no.4,pp.11641‐11647,2022.
[19] C.RenandS.Ma, Dynamicmodellingandanalysisofanomnidirectionalmobilerobot,IEEE IROS2013,Intl.Conf.onIntelligentRobotsand Systems,Tokyo,Japan,Nov.2013.
[20] H.Vellasco‐Villa,H.Rodriguez‐Castro,J. Estrada‐Sanchez,H.Sira‐RamirezandI.A. Vasquez, Dynamictrajectorytrackingcontrol ofanomnidirectionalmobilerobotbased onapassiveapproach,AdvancesinRobot Manipulators,InTechPublications,2010.
[21] K.Watanabe,Y.Shiraishi,S.Tzafestas,J,Tung andT.Fukuda, Feedbackcontrolofanomnidirectionalautonomousplatformformobileservicerobots,JournalofIntelligentandRobotic Systems,Springer,vol.22,pp.315‐330,1998.
[22] M.Diehl,H.G.Bock,M.DiedemandP.B.Wieber, Fastdirectmultipleshootingalgorithmsforoptimalrobotcontrol,In:Fastmotioninbiome‐chanicsandrobotics,LectureNotesinControl andInformationSciences,LNCISvol.40,pp.65‐93,2006.
[23] J.T.Huang,T.V.HungandM.L.Tseng, Smooth switchingrobustadaptivecontrolforomnidirectionalmobilerobots,IEEETransactionson ControlSystemsTechnology,vol.23,no.5,pp. 1986‐1993,2015.
[24] A.S.Lafmajani,H.FarivamejadandS.Berman, ��∞ optimaltrackingcontrollerforthreewheeledomnidirectionalmobilerobotswith uncertaindynamics,IEEEIROS2020,IEEEIntl. Conf.onIntelligentRobotsandSystems,Las Vegas,USA,Oct.2020.
[25] J.Lafay,C.ColletteandP.B.Wieber, Modelpredictivecontrolfortiltrecoveryofanomnidirectionalwheeledhumanoidrobot,IEEEICRA 2015,IEEE2015IntlConf.onRoboticsand Automation,Seattle,USA,May2015.
[26] C.RenandS.Ma, Generalizedproportionalintegralobserver-basedcontrolofanomnidirectionalmobilerobot,Mechatronics,Elsevier,vol. 26,pp.36‐44,2015.
[27] M.Sira‐Ramirez,C.Lopez‐UribaandM.Velasco‐Villa, Linearobserver-basedactivedisturbance rejectioncontroloftheomnidirectionalmobile robot,AsianJournalofControl,J.Wiley,vol.15, no.1,pp.51‐63,2013.
[28] S.LeeandD.Chwa, Dynamicimage-based visualservoingonmonocularcameramounted omnidirectionalmobilerobotsconsideringactuatorsandtargetmotionviafuzzyintegral sliding-modecontrol,IEEETransactionson FuzzySystems,vol.29,no.7,pp.2063‐2076, 2022.
[29] G.Makonnen,S.KumarandP.M.Pathak, Wirelesshybridvisualservoingof omnidirectionalwheeledmobilerobots,Robotics andAutonomousSystems,Elsevier,vol.75,pp. 450‐462,2020.
[30] G.RigatosandK.Busawon, Roboticmanipulatorsandvehicles:Control,estimationand iltering,Springer,2018
[31] G.RigatosandE.Karapanou,Advancesin appliednonlinearoptimalcontrol,Cambridge ScholarsPublishers,2020
[32] G.G.RigatosandS.G.Tzafestas, Extended KalmanFilteringforFuzzyModellingandMultiSensorFusion,MathematicalandComputer ModellingofDynamicalSystems,Taylor& Francisvol.13,pp.251‐266,2007.
[33] M.BassevilleandI.Nikiforov, Detectionof abruptchanges:TheoryandApplications, Prentice-Hall,1993.
[34] G.RigatosandQ.Zhang, Fuzzymodelvalidation usingthelocalstatisticalapproach,FuzzySets andSystems,Elsevier,vol.60,no.7,pp.882‐904,2009.
[35] G.Rigatos,M.AbbaszadehandM.A.Hamida, Intelligentcontrolforelectricpowersystemsand electricvehicles,TaylorandFrancis/CRCPub‐lications,2024.
[36] G.Rigatos, Nonlinearcontroland ilteringusing differential latnessstheoryapproaches:Applicationstoelectromechanicalsystems,Springer, 2016
[37] G.J.Toussaint,T.BasarandF.Bullo, ��∞ optimaltrackingcontroltechniquesfornonlinear underactuatedsystems,inProc.IEEECDC2000, 39thIEEEConferenceonDecisionandControl, SydneyAustralia,2000.
[38] G.Rigatos,M.Abbaszadeh,P.Siano, Controland estimationofdynamicalnonlinearandpartial differentialequationsystems:TheoryandAppli‐cations,IETPublications,2022
[39] G.Rigatos,M.AbbaszadehandJ.Pomares, Flatness-basedcontrolinsuccessiveloopsfor electropneumaticactuatorsandrobots,IFAC JournalofSystemsandControl,Elsevier,vol. 25,pp.10222,2023.
[40] G.Rigatos,P.Wira,M.AbbaszadehandJ. Pomares, Flatness-basedcontrolinsuccessive loopsforindustrialandmobilerobots,IEEE
IECON2022,IEEE2022Intl.Conf.onIndustrial Electronics,Brussels,Belgium,Oct.2022.
Abstract:
Submitted:23rd July2023;accepted:30th November2023
DOI:10.14313/JAMRIS/4‐2024/30
IsmailBogrekci,PinarDemircioglu,GoktugOzer
Thisstudyfocusedonthedevelopmentofan AutonomousUnderwaterVehicle(AUV)acrossfour keydomains:MechanicalDesign,Software,Electronics, andSecurity.TheMechanicalDesignphaseinvolved utilizingcomputer‐aideddrawingprogramstocreatethe AUVmodel.Importantdesignconsiderationsencompass manufacturability,cost,power,weight,anddurability. Comparisonswithnominalvaluesofexistingmarket productsvalidatedtheprecisionoftheproduceddesigns. Thisstudyplacedparticularemphasisontheoptimization ofmodelweight,withafocusonensuringtheAUV’s efficiency,lightness,andexceptionalmaneuverability withinunderwaterenvironments.TheSoftwarestage entailedthedevelopmentofAUVsoftwaretoenable sensitiveandeffectivevehicleoperations.Efficient functioning,devoidoferrorsorcomplications,was imperativetoensureoptimalautonomousdrivingand operationalcapabilities.Imageprocessingalgorithms wereincorporatedintothesoftwaretomaintainhigh accuracyandprovidedimensionalandgeometric informationfromtargetedareas.Furthermore,the softwarephaseinvolvedthedevelopmentofanimage processingalgorithmbasedoncoloranalysis,further augmentingaccuracy.Theselectionofelectronic componentsfortheAUVwasalsoavitalconsideration, alongsideensuringsafetymeasuresateverystageofthe UAV’sdevelopment.
Keywords: autonomousunderwater,imageprocess,3D designandproduction,self‐controlled
1.Introduction
Autonomousunderwatervehicles(AUVs)are advancedsystemsthatintegratesensordetection, informationfusion,andvehiclecontroltechnologies. AkeyapplicationofAUVsisunderwatermulti‐target search.Toimprovesearchef iciency,accuracy,and speed,ateamofAUVscancollaborate.Thisapproach enhancessystemperformance,recon igurability, and lexibilitywhilereducingcostsandmission duration.Multi‐AUVsystems indutilityingathering environmentalgradientdataandconductinghabitat mappingacrossdiverse ields[1].
Two‐thirdsofourplanet’ssurfaceiscoveredby waterintheformofoceans,seas,andrivers,which remainslargelyunexplored.Thisstudyismotivatedby thisfact.Withunderwatercommunicationsinthecon‐textoftheInternetofThings(IoT),anintelligentinter‐connectioncanbeestablishedbetweennodesand vehicles,providingremarkableservicesforunderwa‐terapplications.Thus,theconceptoftheInternetof UnderwaterThings(IoUT)encompassescommunica‐tionforboththeUnderwaterWirelessSensorNet‐work(UWSN)andAUVsfacilitatedbyInternetser‐vices[2].TheIoUTnetworkenablesthedeploymentof variousunderwaterapplications,includingenviron‐mentalmonitoring,surveying,militarypurposes,and more[3].
Scienti icAUVshavediverseapplications,includ‐ingservingasatestbedfornewtechnologies[4];col‐lectingbathymetricdataandmappingthesea loor[5]; conductingarchaeologicalsurveys[6];monitoringthe environment[7];studyingphysical,biological,and chemicalprocesses[8];performinggeologicalsur‐veys[9];andexploringextremeenvironments[10].
CommercialAUVsareutilizedinseveralappli‐cations,includingunderwaterinfrastructureinspec‐tion[11],intheoilandgasindustry[12],andinrepair andmaintenanceoperations[13].
MilitaryAUVsservearangeofapplications,includ‐ingantisubmarinewarfare[14],minecountermea‐sures[15],targetlocalization[16],andsearchand rescueoperations[17].
Inthebibliometricanalysisconductedforthis study,three‐ ieldplotsandco‐occurrencenetworks ofauthorkeywordswereemployedtoyieldvalu‐ableinsightsintotheinterconnectionsandpatterns withinacademicliterature.Thethree‐ ieldplot(Fig.1) providesavisualrepresentationofaf iliations,key‐words,andco‐authors’countries,wheretheheight oftheboxesservesasanindicatorofresearchout‐put.Furthermore,co‐occurrencenetworks(Fig. 2) visuallydepictrelationshipsbetweenkeywordsin researchpublications,uncoveringthematicinterplay. Theseanalyticaltoolsarecriticalforgraspingaca‐demicliteratureandresearchpatterns,providinga detailedunderstandingofcollaborativenetworksand currenttrends.Forinstance,Figure 1 illustratesthat theUnitedStates,Spain,China,andJapanprominently featureintheAUVdomain,whileFigure2focuseson 50nodesassociatedwithAUVkeywords.
Figure1. Three‐fieldplotanalysis(AU_UN(Authors’University)—DE(Keywords)—AU_CO(Authors’Country)
Figure2. Co‐occurrencenetworkofauthorkeywords
Theprimaryaimofthisstudywastoadvancethe evolutionofanAUVbyconductingathoroughinves‐tigationintofourkeydomains:MechanicalDesign, Software,Electronics,andSecurity.Thepivotalobjec‐tivewastoguaranteetheAUV’sef iciency,achieve alightweightstructuralcomposition,andfacilitate superiormaneuverabilitywithinunderwaterenviron‐ments.Throughaconcentratedexaminationofthese foundationaldomains,thisresearchendeavorstoaug‐menttheoverallperformanceandoperationalcapa‐bilitiesoftheAUV.
2.1.MechanicalDesignProcessUsedintheDevelop‐mentoftheAUV
Asastartingpoint,theCADprogramSolidWorks wasselectedtocreateandvisuallyrepresentdesigns onacomputer.Duringthedesignprocessforthe mechanicalbody,themaincriteriaforselectionare cost,weight,symmetryofthemainbody,security, timesavings,andeaseofmanufacturing.Designing forunderwaterconditionspresentsadditionalchal‐lengescomparedtodesigningforland‐basedappli‐cations.Factorssuchasvehiclestabilization,system steadiness,communication,andpressureparameters aremoredif iculttoadjustand inalizeinthedesign.
Toaddresstheseparameters,variousacademicstud‐ies,drawingprograms,andanalysistoolswereuti‐lized.Theoverallmechanicaldesignencompassesthe mainchassis,thrusters,propellers,aleak‐proofplex‐iglasstube,andtube‐stabilizingcuffs.Followingthor‐oughworkbasedontheaforementionedcriteria,the inaldesignofthevehiclewasachieved,asshownin Figure3.
Instreamliningthemanufacturingprocess,the primarychassisoftheAutonomousUnderwaterVehi‐cle(AUV)wasmeticulouslydesignedtoundergo 3Dprinting,comprisingsixdistinctcomponents. Thismodularapproachfacilitateseaseofproduction, allowingeachcomponenttobeprintedseparatelyand subsequentlyassembledtoformtheintegralchassis structure.Forvisualclarityandreference,Figure 4 providesadetaileddepictionofthesixmaincon‐stituentpartsthatcollectivelyconstitutetheAUV’s chassis.Thismethodnotonlyenhancestheef iciency ofthemanufacturingprocessbutalsooffers lexibil‐ityandscalabilityinadaptingthedesigntospeci ic requirementsandmodi ications.
Theholderstaskedwithstabilizingthethrusters onthemainchassisunderwentrigorousstresstest‐ingandwerepurposefullydesignedtowithstandthe considerableforcesencounteredinunderwaterenvi‐ronments.Inparticular,thehalf‐circlesegmentof thechassisdesignatedformotorplacementunder‐wentreinforcementtomitigatetheriskofpotential breakage.
Utilizingadvanced3Dprintingtechnology,thesec‐tionswherethethrusterswillbepositionedwere intricatelycrafted.Thesesectionsfeaturetwinfemale andmaleconnections,augmentedwithM4boltsto ensurearobustandsecureattachmentmechanism. Thedesignandstructuraldetailsofthesefemale‐male chassispartsarevividlyillustratedinFigure5,provid‐ingavisualreferenceforthemeticulousengineering anddurabilityconsiderationsincorporatedintothe AUV’sthrusterstabilizationsystem.
Toaccommodatetheinstallationofacamera insidethevehicle,agapwasdesignedonthelower partsofthechassis.Theassemblyofthechassisis inalizedusingM4boltstoconnectthefemale‐male parts.Inordertoensurestabilitywhileontheground, smallstandsinstripswereaddedtothelowersection ofthechassis.PleaserefertoFigure 6 foravisual representationofthelowerpartofthechassis.
Inordertomaintainweightbalanceandsym‐metry,whileenablingsmoothforward‐backwardand right‐leftmovement,fourpropulsionmotorswere strategicallyplacedatthefourcornersofthemain chassis,angledat45degrees.Additionally,toachieve verticalmovement,anotherfourpropulsionmotors weresymmetricallypositionedatthecenterofthe chassis.Thiscon igurationensuresbothaesthetic appealandoptimalweightdistribution.Consequently, atotalofeight(8)BrushlessDirectCurrent(BLDC) motorsareutilizedinthevehicle.
Themotorsresponsibleforgeneratingvertical movementaresecuredusingM3bolts,whilethe remainingmotors,whichfacilitatehorizontalmove‐ment,aretightly ixedusingpins.Eachmotoris equippedwithalidcoverforitshousing.Thesecovers notonlyprovideprotectionbutalsoenablethemotors tobesealedwithepoxyattheirconnectionpoints, ensuringtheyarewatertight.Thissafeguardallows themotorstooperatesafelyunderwaterforextended periodsoftime(Fig.7).
Thepropellers,asshowninFigure 8,designed inSolidWorksand3Dprinted,underwenttestingvia CFDanalysisandreal‐worldconditions.Theobser‐vationrevealedthatasingleboltwasinsuf icientfor secureattachment,promptingtheadditionofanother strategicallypositionedbolt.CFDanalysishighlighted substantialthrust,primarilyduetoahighpitchangle, butposedachallengeofthecontrolcardoverheat‐ing.Thisunderscorestheimportanceofadoptinga balancedoptimizationapproachthataddressesnot onlypropellerperformanceenhancementsbutalso thecriticalaspectofthermalmanagement.Suchan approachhighlightstheinterconnectedconsidera‐tionsintegraltotheprocessesofdesign,analysis,and practicalapplication,ensuringacomprehensiveand effectivesolutiontothechallengesencountered.
Topreventanycontactbetweentheelectronic componentsandwater,arobustsolutionwas implemented.Allelectroniccomponentsaresecurely housedwithinaleak‐proofplexiglasstube.This tubefeatureslidsatboththefrontandbackends. Theuseofaleak‐proofplexiglasstubetohouse theelectroniccomponentsisarobustsolutionfor preventingcontactbetweenthecomponentsand water.Byenclosingtheelectronicswithinthetube, onecreatesaphysicalbarrierthatshieldsthemfrom anypotentialwaterdamageormoistureintrusion.
Thefrontallidincorporatesacircularplexiglass materialwithaninnerdiameterof114mm,allow‐ingforcameraviewing.Ontherearlid,thereare leak‐proofconnectorholesspeci icallydesignedfor themotorcablestopassthrough.Tomaintainthe integrityofthesystemandpreventanyleakage,the motorcablespassingthroughtheconnectorsare enclosedwithinaseparatetubethatis illedwith epoxy.Thisadditionaltubemeasures120mmindiam‐eterand400mminlength.Boththelidsofthistube werereinforcedwithO‐ringstoensureacompletely leak‐proofsystem.
Figure9illustratestheCADassemblyandlid designoftheplexiglasstube,showcasingthemeticu‐lousattentiongiventomaintainingawatertightenclo‐surefortheelectroniccomponents.
Thestabilityoftheplexiglasstubeisofutmost importance,anditiscrucialtosecurelyfastenthe tubetothechassis.Toaddressboththevibrations generatedbythemotorsandthe luidmovements causedbywater,supporting ixingcuffswerestrate‐gicallypositionedandconnectedtothechassisusing M4bolts.Theuseofsupporting ixingcuffsandM4 boltstosecuretheplexiglasstubetothechassisis aneffectiveapproachtoensurestability.Thevibra‐tionsgeneratedbythemotorsandthe luidmove‐mentscausedbywatercanpotentiallycreatestress andstrainonthetube,whichmayleadtoinstability orevendamageifnotproperlyaddressed.Figure10 presentsthedesignofthetube ixingcuffs,offeringa visualrepresentationoftheircon iguration.The“Tube FixingCuffDesign”referstothespeci icengineering andstructuralarrangementcreatedtosecurelyfas‐tentheplexiglasstubetothechassisofthevehicle, therebyensuringstabilityduringitsoperation.
Isometric(a),frontal(b),andtop(c)viewsof thedesign
Furthermore,Figures11a–cpresenttheisometric (a),frontal(b),andtop(c)viewsofthedesign,provid‐ingacomprehensiveoverviewofthe inalizedvehicle designanditsoverallappearanceandfeatures.
InordertoenhancetheAUV’sfunctionalityand enableittoperformadditionaltasks,agripperarm designwasmeticulouslycrafted.Thiscomponent servesasamechanicalmovingpartthatcansecurely graspandholdobjectswithoutexertingexcessive pressurethatcouldpotentiallydamagethem.The developmentprocessinvolvedmultipleiterationsand redesigns,asthegripperarmprovedtobeamore complexandchallengingcomponenttomanufacture.
The inaldesignofthegripperarm(Fig. 12)was intentionallykeptassimpleaspossibletoful illits intendedtaskwithoutintroducingunnecessarycom‐plexitytootheraspectsoftheAUV.Tofacilitatethe grippingmotion,awaterproofservomotorwasuti‐lized.Thegripperitselfispositionedatthefrontof thesub‐frameandiscapableofachievinganopen diameterofupto80mmandacloseddiameterofupto 25mm,allowingittoeffectivelycarryoutitsobjective.
Theplexiglasstubeservesasaprotectiveenclo‐sure,effectivelycontainingalltheelectroniccompo‐nentsandensuringaleakproofenvironment.Within thistube,theheaviestcomponentofthesystem,the Lithium‐Polymer(Li‐Po)battery,issecurelyhoused.
Toenablethecameraplacedinsidetheacrylictube tocaptureimages,awindowisprovidedtoconnect itwiththeoutsideworld.Thisisachievedbyincor‐poratinganacryliccircleonthefrontalsideofthe tube,whichallowsthecameratoperformitsfunction effectively.Theacryliccircle(Fig.13)hasadiameter of114mmandathicknessof3mm,providingaclear andtransparentviewforthecamera’simagingcapa‐bilities.
Figure14. Frontal(a)andrear(b)sidelids
Figure15. O‐rings
Thetubessituatedonboththefrontal(Fig. 14a) andrear(Fig.14b)sidesoftheacrylictubearecrafted fromaluminummaterial.Forthefrontallids,the designincorporatesmortisestoaccommodateO‐rings (Fig.15),ensuringasecureandleakproofseal.Incon‐trast,therearlidsareequippedwith ivepassholes toallowthecablesfromoutsidetopassthrough.Once thecablepassagesarecompleted,thelidsaresealed usingepoxyandbrassmaterialtofurtherenhancethe leakproofintegrityofthesystem.
2.2.1.PLA+Filament
The ilamentemployedin3Dprintingforproduc‐ingmaterialsisPolyacticAcid+(PLA+).Thisorganic biopolymerandthermoplasticexhibitadensityof 1.24g/cm3 andatensilestrengthof43MPa.The optimalprintingtemperaturerangesfrom190∘Cto 220∘C,whiletherecommendedbedtemperaturefalls between60∘Cand80∘C.
Comparedtoothermanufacturingoptions,3D printingusingPLA+ ilamentprovestobecost‐effective.Oncethetemperatureandprintingsettings areappropriatelycon igured,itallowsforprinting withoutencounteringsigni icantissues.Moreover, PLA ilamentiseasiertoworkwithcomparedto other ilamenttypes,offeringtheaddedadvantageof abrighterappearance.Thiscontributestoanaesthet‐icallypleasing inishedproduct.Additionally,PLAis considerednon‐harmfultohumans.
Table1. Materialsusedformechanicalparts[18]
MechanicalPartName
2.2.2.Aluminum
Thematerialchosenforthelidsofthetubesis aluminumduetoitsfavorablepropertiesandsuitabil‐ityfortheintendedpurpose.Aluminumwasselected becauseofitseaseofprocessing,lowmanufactur‐ingtolerance,andexcellentresistancetocorrosion, whichisparticularlyimportantsincethelidswillbe predominantlyunderwater.Thesurfaceofaluminum naturallyformsathinyetdurableoxide ilm,pro‐vidingenhancedcorrosionresistance.Additionally, aluminumisnon‐magnetic,possessesgoodelectrical conductivity,andhasadensityof2.70g/cm3.Ithasa relativelylowmeltingtemperatureof660.32∘Canda tensilestrengthof49MPa.
2.2.3.MaterialsUsedforMechanicalParts
Thedesignprocessforthephysicalpartswas focusedonminimizingtheoverallweightofthevehi‐cle,ensuringitsmaneuverabilityonwater.The inal designachievedatotalweightof3357g,accompa‐niedbyavolumeof9,002,562.75mm3.Thechassis dimensionsmeasure473.57mminlength,437.43mm inwidth,and133mminheight.Belowisatablelisting allthemechanicalpartsincorporatedintothevehicle:
2.3.MethodsUsedintheDevelopmentofAUV
2.3.1.3DPrinting
Alltheparts,exceptforthefrontcoverglass, tubelids,andO‐rings,weremanufacturedusinga 3Dprinter.Thechoiceofa3Dprinterwasdriven bytheconvenienceitoffersinthemanufacturing process.Additionally,theiterativenatureofdesign changes,whichrequiredminimaltonomodi ications inthemanufacturingprocess,andtheoverallcost‐effectivenessof3Dprintingwerekeyfactorsinthe decision.
Toensurerobustnessandpreventanyissuesdur‐ingbolttightening,allthecomponentswereprinted withahighin illpercentageof85%.Thisincreased in illpercentagecontributedtotheoverallstrength andstructuralintegrityoftheprintedparts.
Thealuminumtubelids,utilizedonboththe frontalandrearsidesoftheAUVtoensurealeak‐proof sealfortheacrylictube,weremanufacturedthrough machiningprocesses.Thismethodwaschosenasthe mostappropriatemeansoffabrication,asitenables preciseprocessingwhilemaintainingalowtolerance rate.
Afterevaluatingthediverserangeoffunctions expectedfromthevehicle,includingimageprocess‐ing,motorcontrol,sensorintegration,dataprocess‐ing,andautomation,powerconsumptioncalcula‐tionsdeterminedthatthemostsuitablepowersup‐plyoptionforthesystemwastheLithiumPolymer ProfuseBattery6S25C(22.2V22000mAh).ThisLi‐Pobatteryprovidessuf icientpowertorunallelec‐troniccomponentswithoutencounteringanypower shortages.
Toensuresmoothandef icientmovementunder‐water,theselectedmotorsandelectronicspeedcon‐trollers(ESCs)werecarefullychosentoworkinhar‐monywiththeLi‐Pobattery.Themotorsare6Scom‐patiblewitharotationspeedof30,000RPMand requireapproximately30Aofcurrent,whiletheESCs haveacurrentresistanceof40A.
Fordataprocessing,whichincludesinformation fromsensorssuchasultrasonic,depth,pressure,and thecameraforimagecapture,aRaspberryPi4was incorporated.Additionally,aPixhawkPX4 lightcon‐trolcardwasemployedforvehicleautomation.Topre‐ventoverheating,a5Vvoltageregulatorwaschosen topowerthesensorsandcamerainsteadofdirectly relyingontheRaspberryPi4andPixhawkPX4.
Safetymeasureswerealsoimplemented,including anemergencystopbuttonandapowerdistribution cardcapableofhandlingupto400A,servingassafe‐guardsforunexpectedhighvoltageoroverheating situations.
ThesensorsandcameraareconnectedtotheRasp‐berryPi4whichhasBroadcomBCM2711central processingunit(CPU)witha4‐coreadvancedRISC machines(ARM)architectureCortex–A72toprocess thedatareceivedandgivethevehicleautonomous abilities.HavingaCPUof1.5GHzworkfrequencywas selectedasitis%10fasterthanthepreviousmodel.
Beingahighlyadvanced lightcontrolcardthat worksfortheautomationofthevehicleitself,the Pixhawkiswidelyusedbothinairvehiclessuchas multirotorplanesandhelicopterswhilealsobeing usedinremote‐controlledvehicles.Itwasselectedas anideal lightcontrolcardwitha32‐bitprocessor. ThePixhawkreceivesthenecessarydatatoprop‐erlycontrolthespeedofthemotors.Theconnection betweentheRaspberryPi4andthePixhawkwas madepossiblewithatelemetrydevicethatsecuresa universalasynchronousreceiver/transmitter(UART) communication.Thepowerrequiredtorunthe light controlcardisreceivedfromtheRaspberryPi4.
Ananalogpowermodulewasintegratedtopro‐videastablepowersupplytothePixhawk lightcon‐trolcardandthemini‐computerRaspberryPi4which alsosupportsthemeasurementofthebatteryvoltage andthecurrentconsumption.Thepreferredpower modulehasamaximuminputof30V90Aandamaxi‐mumoutputof5.3V3A.
Toprovidetheconnectionbetweenthemotorsand thePixhawkandtocontrolthemotorsthemselves, eight40AESCscompatiblewith2‐6Spowerinput wereused.WiththeseESCs,controlofthemotorswas achievedwiththehelpofthesignalscomingfromthe lightcontrolcard.
Topreventdamagetotheelectricalcomponents ofthesystemfromthehighvoltagecomingfromthe powersupply,aprocesscontrolblock(PCB)regulator wasintegratedintothesystemtoregulatetheincom‐ingvoltage.
Tocaptureandprocesstheimagesduringthework activityofthevehicle,a5MPcamerawitha isheye lensthatiscompatiblewiththeRaspberryPi4Model Bwasselected.
Todetectthepositionofobjectsunderwaterand avoidacollisionwiththem, iveunitsofleak‐proof ultrasonicdistancesensorswereplacedontheedges andthebottomofthechassis.
Forthepurposeofmeasuringthedistancefrom thesurfaceandthedepthofthevehicleitself,andto knowitspositionalinformation,depthandpressure sensorswereincludedinthesystem.
Tochecktheelectricalcomponentsfortemper‐aturecontrolandcutthepowertothesystemin thecaseofoverheating,atemperatureandhumid‐itysensorwasincludedinthesystem.TheDHT21 Temperature‐Humiditysensorwaschosenasitis highlyreliableandcanworkstablyeveninlong‐termworkingconditions,inadditiontohavingafast responserateasithasan8‐bitmicroprocessor.
Theboarditselfwashandmadewithcopper cables,wheretheplatewithblackcoloredcablesrep‐resents“ ”whiletheplatewiththeredcoloredcables represents“+”.Itisacrucialpartofthesystemas itallowsthepowercomingfromthesourcetoreach everypartofthesystem.
Incaseofasystemoverloademergency,acurrent sensorwasintegratedtoproactivelyshutdownthe electricitysupplybeforeexternalintervention.Addi‐tionally,aleakageemergencystopbuttonwasadded tothechassisforimmediatedeactivationinunfore‐seencircumstances,enhancingdevicesafetyproto‐cols.Figure 16 providesavisualrepresentationof the inalplacementoftheelectroniccomponentsin theacrylictube,aidingoperationalunderstandingand facilitatingpotentialtroubleshootingscenarios.
2.5.ElectronicalDesignProcessUsedintheDevelop‐mentofAUV
AsthecomplexityofanAUV’sperformedjobforit toaccomplishincreases,sodoesthedecision‐making processofsaidjobtoo.InROVs(RemotelyOperated Vehicle),thisproblemissolvedbyhighlytrainedindi‐vidualspersonallymonitoringthesituationduring workandgivingthecorrectandnecessaryinputsto themachinetosolveoravoidanyproblemsthatcould occur.ButforanAUVtoautomateitself,algorithms comeintoreplacethishumanrequirement.
AlgorithmsareusedforanAUVtomovefrompoint AtoB,avoidobstacles,performcommunications,col‐lectdata,facilitatetargetandcolordetection,give instructions,andsolveanyproblemthatmightoccur withperformingacomputation.
TheunderwaterenvironmentinwhichanAUV operatesisnotastaticlocationlikeagroundenviron‐ment.Comparedtotheground,underwatersurround‐ingsaremoredynamicandmorepronetochange.It isnotalwayspossibleforAUVpathplanningopera‐tions,suchasobstacleavoidanceanddetection,tobe donebeforehandastherearemanydynamicobstacles toconsidersuchasmarinelife,reefs,ships,oreven various‐sized loatingobjectssuchasgarbage.Articles intheliteraturewerestudiedtoexaminethedifferent waysofpath indingofAUVsforstaticanddynamic obstaclesituations.
Allthedatacollectedfromtheoutsidebythe vehicleweretransferredbytheRaspberryPi4viaa radiofrequency(RF)communicationmoduletothe maincomputer.Onthesystembesidethe lightcon‐trolcard,agroundcontrolstationalongsideacom‐panioncomputer,suchasRaspberryPi4,wasused. PythonsoftwarelanguageontheRaspberryPiwas selected.Alltheoperationsrequiredfromthevehicle werecodedwithPythonviatheRaspberryPi.For thegroundcontrolstationsoftware,theQGroundCon‐trolprogramwasused.ArduSubsoftware,developed besideArduPilot,wasusedforauto‐pilotingsoftware. ArduSubworksalongsidetheQGroundControlinter‐faceasagroundcontrolstationtogiveanadvantage andeaseofusefordepth ixation,stabilization,and manualdrivingmode.
Thealgorithmwaspreparedfortheautonomous softwareofthevehicle.Withthisdesign,an autonomousmotionsystem,targetdetectionand passing,colordetectionandpositioning,target detection,anddestructionalgorithmswerecreated fortheirtasks.
Theprocessofwritinganalgorithmstartedwith thecommunicationssystem.Analgorithmwaswrit‐tentodealwithbasiccommunicationsbetweenthe serialandthesensoraccordingtothedatatrans‐ferandreturnsfeedbackwithinformationaboutthe motoranditsposition.Thenextprocesswastode ine thestartingpreparationstepsforthemovementby checkingthedatatheAUVreceiveswithitssensors. Thevehiclestartsitsmovementfromarandomdrop pointtotheclosestcornerviaitsimplementedalgo‐rithmandsoftware.AftertheAUVstartsthezigzag motion,thevehiclebeginstosearchforthechosen speci iedtargetobject,whichisdesignatedasasub‐marine,andcenteritselffacingthelocatedsubmarine withtheinformationreceivedfromthecamera.Then, throughthedesignatedalgorithm,thevehiclestarts scanningforthedesignatedtargetwhichisacircle. Thedatareceivedthroughscanningthepoolandthe circlesarekeptinmini‐PCforstorage.Thenextstep afterlocatingandidentifyingthetargetobjectthrough itscolors,isthedestructionofsaidtarget.
Thetargetobject’spingfrequencyisdetectedand thenthevehiclemovestothetargetobjecttodirectly impactitanddestroyit.Anotheralgorithmwaswrit‐tenfortheAUVtogivethevehicletheabilitytodetect andpassthroughadoorwhichwasshapedasarectan‐gularhollowobject,bycenteringitselfwiththeinfor‐mationgainedviaitssensors.
Hereweoutlinetheprocessofwritingautoma‐tioncodesfortheAUVtoautomateitselfandful ill itsobjectivessuccessfully.Pythonsoftwarelanguage programwaschosenasithasanopen‐sourcedlibrary whilealsobeingadequatesoftwareforimageprocess‐ing.Python3.9wastheversionofthePythonpro‐gramchosen.Itisasuperbchoiceasitcanbeused forprototypedevelopmentsuchasanAUVwithout havingproblemswithmaintainability.OpenCVlibrary wasusedfortheimageprocessinglibrary.Theimage processingtoolperformsthetasksgiventothevehicle suchasidenti icationofsurroundings,targetdetec‐tionofthedoorandforpassingthroughit,andcolor detectionforpositioninganddestructionofthedesig‐natedtarget.
Theevaluationoftheimagesobtainedthrough thesensorswasdonebyimageprocessingalgo‐rithmssothattheESCsandthemotorsthatarecon‐nectedtothemcanworkinunison.Thecommu‐nicationbetweentheRaspberryPi4andPixhawk wasprovidedwithtelemetryandgeneral‐purpose input/output(GPIO)pins.
Imageoftherectangularshaperecognition andtaskbars
ThePixhawkreceivesthecommandsthatare transmittedfromRaspberryPi4withthehelpofthe “Pymovlink”libraryinthecommunicationpart,thus enablingthePixhawktocheckuponthemotorsto startthevehicle.
Totesttheimageprocessingsystems,a rectangular‐shapedcalendarwasdisplayedto thecamerafromadistanceof30cm.Throughits observations,thesystemrecognizedfourdifferent rectangles.Duringadditionaltestsatvarious brightnesslevels,itwasobservedthatindarker environmentswithalesserdegreeoflight,especially insituationsofinsuf icientlightlevelsatcloserange, objectdetectionofrectangularshapeswasnot consistentenough.Assuch,itwasconcludedthat thesystemcandetectanobject’srectangularshape accuratelygiventhattheenvironmenthassuf icient brightnesslevelsortheobjectitselfisundersome lightsource.
Tocircumventtheissuesthatoccurredinthe rectangularshapedetectionsystem(Fig. 17)under insuf icientbrightnesslevels,slidersthatcanadjust thesettingsofthecamerawereaddedtoperforma calibrationtoadjusttocurrentlightlevelsforbetter imageprocessing.
Throughtheresultoftheliteraturereviewforthe taskofpassingthroughthedoor,itwasfoundthat theYouOnlyLookOnce(YOLO)modelwasthemost ef icientmethod.YOLOisareal‐timeobjectdetection algorithmthatoperatesbydividingtheinputimage intoagridandpredictingboundingboxesandclass probabilitiesforeachgridcell.Ithassmallyetsimple structuralfeatures.Withitsneuralnetwork,itcan quicklygiveade initerecognitionoutput;therefore, ithasafaircomputationspeed.Thespeedofthe algorithmtorecognizetheobjectwithafastresponse timeisapositiveabilityfortheAUV.Themodelof therectangular‐shapeddoorwhichtheAUVwillpass throughisshowninFigure18.
GatheringadatasetfortrainingtheYOLOmodel isanessentialstepintheprocess.Wedesigneda modeloftherectangulardoorusingSolidWorksand captured100screenshotsandphotosfromvarious angles.Thisdatasetwillhelpthemodellearntorecog‐nizeandlocalizethedoorindifferentorientationsand perspectives.Dividingthedatasetintotrainingand testingsetsisacommonpracticeinmachinelearn‐ing.Using80photosfortrainingandreservingthe remaining20fortestingallowsonetoevaluatethe model’sperformanceonunseendataandassessits generalizationabilities.
DuringthedevelopmentoftheYOLOmodelin Python,theterm“weightingdocuments”wasmen‐tioned.However,itisimportanttoclarifythat“weight‐ingdocuments”isnotastandardpartoftheYOLO trainingprocess.InYOLO,theprimaryobjectiveisto optimizethemodel’sperformancebyminimizinga designatedlossfunction,whichmeasuresthediscrep‐ancybetweenthepredictedboundingboxesandthe groundtruthannotations.Thisoptimizationprocess reliesonbackpropagation,wherethemodel’sweights areiterativelyupdatedtominimizethelossfunction.
The ine‐tuningofthemodel’sparametersoccursover multipleepochsuntilitachievessatisfactoryaccuracy onthetrainingdata.Foreffectivetraining,itiscru‐cialtostrictlyadheretothestandardYOLOtraining procedureandavoidanyconfusionormisconceptions arisingfromunconventionaltermssuchas“weighting documents.”Byfollowingtheestablishedtrainingpro‐cess,theYOLOmodelcaneffectivelylearnanddemon‐strateimprovedprecisionandreliabilityindetecting andlocalizingobjectsinreal‐worldscenarios.
Toachievethetargetdetectionanddestruction objectives,circledetectionwasinitiallytestedusing Pythonwithacomputercamera(Fig. 19).However, thisapproachprovedinadequateduetothecamera’s poorprecisionandinsuf icientlightingconditionsin thetestingenvironment.
Insubsequenttests,aswitchwasimplemented, transitioningtotheRaspberryPicamera.Thischange resultedinnoticeableenhancementsinimagepro‐cessingandcolordetectioncapabilities,particularly forobjectswithred,blue,andgreencolors(Figs.20–22).Thecodesforimageprocessingwerespeci i‐callytailoredtotheRaspberryPiplatform,leveraging existingcolorcodelibraries.Thedetectionofcolors wasaccomplishedusinghuesaturationvalues(HSV) asafundamentalcomponentoftheimageprocessing algorithm.TheutilizationoftheRaspberryPicamera, coupledwithHSV‐basedcolordetection,provedtobe asuccessfulcombination,signi icantlyimprovingthe accuracyandef iciencyofidentifyingobjectsbasedon theirdistinctcolorsignatures.Thisadvancementin imageprocessingpavedthewayformorereliableand precisedetectionandlocalizationofobjectsinreal‐worldscenarios.
ThroughtheintegrationoftheRaspberryPicam‐eraandemployingcolordetectiontechniquesbased onHSVvalues,thesystem’sabilitytodetectcircles fortargetidenti icationandotherpurposeswassig‐ni icantlyimproved.Theenhancedprecisionofthe RaspberryPicamera,alongwithaccesstospecialized colorcodelibraries,allowedformoreaccuratecircle detection,enablingthesystemtoidentifycirclesof speci iccolors.Thiscapabilityisvaluableforvari‐ousapplications,suchastargetedtasksorachieving
speci icobjectives.However,itisimportanttonote thatthetechnology’spotentialusesextendfarbeyond destructivepurposesandcanhavediverseapplica‐tionsinawiderangeof ields.
Inthecontextofunderwaterexploration,the identi icationofthesubmarineandtheaccurate positioningoftheAUVrelativetoitarecriticaltasks. Thesetasksareaccomplishedthroughsophisticated calculationsperformedwiththeaidofthepowerful computervisionlibrary,OpenCV.Thesystememploys colorprocessingtechniquesforobjectrecognition duringimageprocessing.Thegoalistodetectand distinguishobjectsintheunderwaterenvironment basedontheiruniquecolorcharacteristics.Tofacil‐itatethis,pre‐de inedcolorcodesandHSVvalues fromtherovpylibrary,speci icallydesignedforROV tools,wereutilized.Byharnessingthecapabilitiesof OpenCV,thesystemcarriesoutcalculationstodetect andidentifythesubmarine(Figs.23–24).
Colorprocessingplaysapivotalroleinobject recognition,enablingthesystemtodistinguishobjects basedontheirrespectivecolors.Therovpylibrary’s colorcodesandHSVvaluesarespeci icallytailoredfor ROVapplications,consideringfactorssuchaswater clarity,lightingconditions,andtypicalunderwater objectappearances.Thisspecializedlibrarystream‐linestheprocessofobjectrecognitionasitprovides asetofready‐to‐usecolorcodesthatmatchthepreva‐lentunderwatercolorschemes.
Codesusedincolordetectionsoftware applications
IntegratingOpenCVwiththerovpylibrary’scolor codesandHSVvaluesenabledthesystemtosuccess‐fullyaccomplishitsmissionofidentifyingthesub‐marineandaccuratelydeterminingtheAUV’sposi‐tionrelativetoit.Thisapproachenhancedthesys‐tem’simageprocessingcapabilities,facilitatedpre‐ciseobjectrecognition,andeliminatedtheneedfor extensivemanualcalibrationorcustomcolorcoding, streamliningtheoverallprocess.
Theimageprocessingsystemsplayacrucialrole inprovidingvaluabledatafortheAUV’soperations. TheyenabletheAUVtodetectcoloredringsplaced aroundthesubmarine.Byanalyzingthecaptured images,thesystemcanidentifyandlocatethesecol‐oredringsaccurately.Thesystem’ssophisticatedalgo‐rithmsallowittocalculatethediametersoftherings, providingessentialmeasurementsforprecisenaviga‐tion.Withthedataacquiredfromtheimageprocess‐ing,theAUVusesthisinformationtopositionitselfin theclosestproximitytothesubmarine.
Thereweretheoreticalplanstoincorporatean underwatermicrophoneintothevehicleforthe
propeller
purposeofdetectingatargetpingingball.Thepro‐posedsystemwouldhaveincludedadditionalcompo‐nentssuchasatransmittingandreceivingtransistor, aswellasasignalgeneratorcapableoftransmittinga 45kHzacousticwave.Theintentionwastosynchro‐nizethefrequencyrangeofthebuzzerimplantedon thevehiclewiththe45kHzfrequency.Thiswould enablethevehicle’smotorstobedirectedbasedon thefrequencydirectionusingtheassistanceofthe buzzer.However,theseideasandapplicationswere notimplementedinthecurrentiterationandwereleft forfuturerevisions.
2.5.3.ExternalInterface
Thesystem’sexternalinterfaceintegratescontrol parametersformotorsettings,underwaterlighting systems,andsensorcalibrationsettings.Effectivedata lowwithinthesystemisenabledbytheQGroundCon‐trolsoftware,facilitatingcommunicationandman‐agement.Motorcontrolsandsensordataprocessing wereimplementedusingthePixhawkToolchain,a robustsoftwareframeworkdesignedspeci icallyfor autonomousvehicles.
Imageprocessingtaskswereexecutedusingthe PythonprogramminglanguageontheRaspberryPi 4throughoutallstages.Python’sextensivelibraries anduser‐friendlysyntaxprovidea lexibleandef i‐cientplatformforimageprocessingwithinthesys‐tem.ByharnessingPython’scapabilitiesontheRasp‐berryPi4,thesystemef icientlyprocessesandana‐lyzesimagedata,supportingvariousfunctionalities andobjectives.
3.1.FlowSimulationofPropellerAUV
Thepropeller,initiallydesignedusingtheSolid‐Worksprogram,underwentacomprehensiveanal‐ysistoassessitsthrustforceandtheadjustability ofthepropellerbladeangleusingacomputer‐aided lowsimulationprogram.Thesimulationinvolvedthe adjustmentofvariousparameterstoinvestigatethe propeller’sperformance.
Figure 25 presentsimagescapturedduringthe analysis,depictingtheexaminationofambientpres‐sureandtheanalysisofinlet‐outlet lowinthespeci ic sectionwiththedesignated lowdirection.Thesevisu‐alizationsoffervaluableinsightsintothebehaviorof thepropelleranditsinteractionwiththesurrounding luidthroughoutthesimulationprocess.
SGInput Mass Flow 1Velocity Avg1985,71m/sn done (IT=154) 23,9215m/s1989,76m/sn
SGInlet Mass Flow 1Total Pressure Avg5,39235e+09Pa done (IT=154) 2,04633e+07Pa5,39381e+09Pa
SG Heat Transfer Rate (Convec on)200W done (IT=154) 0W0W
SG Heat Transfer Rate 190W done (IT=154) 5e-06W0W
SGForce (X) 24-16431,6N done (IT=181) 652,471N-16391,7N
SGForce (Y) 2521069,9N done (IT=197) 3069,88N22092,8N
SGForce (Z)26-46907,3N done (IT=187) 3723,87N-44965,9N
SG Maximum Peripheral Speed147872,77m/sn done (IT=154) 7,87277e-05m/sn7872,77m/sn
SGMax Speed (X)8503,617m/sn done (IT=192) 8,55082m/sn501,421m/sn
SGMax Speed (Y) 108957,24m/sn done (IT=154) 8,95724-05m/sn8957,24m/sn
SGMaximum Speed (Z) 128732,87m/sn done (IT=154) 8,73287e-05m/sn8732,87m/sn
SGMaximum Turbulence Intensity 181000% done (IT=154) 1e-05%1000%
SGMaximum Turbulent Viscosity 16212,154Pa*sn done (IT=154) 17,2252Pa*sn213,129Pa*sn
SGMinimum Peripheral Speed 13-6994,64m/sn done (IT=154) 6,99464-05m/sn-6994,64m/sn
SGMinimum Speed (X)7-789,288m/sn done (IT=154) 16,2098m/sn-788,564m/sn
SGMinimum Speed (Y)9-8922,42m/sn done (IT=154) 8,92242e-05m/sn-8922,42m/sn
SGMinimum Speed (Z)11-8948,37m/sn done (IT=154) 8,94837e-05m/sn-8948,37m/sn
SGMinimum Turbulence Intensity 171,116412% done (IT=154) 0,0334869%1,11642%
SGMinimum Turbulent Viscosity 150Pa*sn done (IT=154) 0Pa*sn0Pa*sn
SGNormal Force (X) 21-16413,8N done (IT=181) 650,159N-16374N
SGNormal Force (Y) 2221059N done (IT=192) 3067,95N22082N
SGNormal Force(Z) 23-46761,9N done (IT=187) 3715,46N-44822,3N
SGAverage Dynamic Pressure 42,28142e+09Pa done (IT=154) 1,01983e+07Pa2,28293e+09Pa
SGAverage Total Pressure 32,09627e+09Pa done (IT=193) 9,07887e+06Pa2,09534e+09Pa
SGFric on Force (X) 28-17,7643N done (IT=186) 7,74142N-17,6834N
SGFric on Force (Y) 2910,9151N done (IT=194) 13,9573N10,8454N
SGFric on Force (Z) 30-145,471N done (IT=186) 8,78955N-143,647N
SGFric on Force 27146,958N done (IT=179) 13,3315N145,185N
Figure26. Outputimageofflowsimulationresults‐1
Flowsimulationiteration‐basedgraphic results‐1
Thegathereddataanalysiswassuccessfullyexe‐cuted.However,uponfurtherexamination,itwas observedthattheoutputresultsoftheanalysiswere derivedfromconstantvaluesusedattheoutsetof theprocess.Asaconsequence,theobtainedmea‐sureddatawerefoundtobe10timeshigherthanthe expectednominalvalues.Thisdiscrepancyledtoan inaccuraterepresentationintheschematicvisualiza‐tionofthegraph.
Theresultinggraphschematicdidnotalignwith theinitiallyanticipatedpattern,whichwasexpected todisplayalinearincreasetransitioningintoacon‐stant lowafterreachingthemaximumlevel.Theissue aroseduetotheutilizationofincorrectvaluesinthe analysis.Toaddressthis,theanalysisneedstobe reevaluated,andthecorrectvaluesshouldbeutilized inthecalculations.Rectifyingtheinputvalueswill enablesubsequentanalysisandgraphgenerationto provideamoreaccuraterepresentationofthedata. Thiswillfacilitateaproperinterpretationofthe low behavioranditsrelationshiptothemaximumlevel, allowingforamorereliableassessmentofthesys‐tem’sperformance.Conductingtheanalysiswithpre‐ciseandaccuratevalueswillensurethatthegraph exhibitstheexpectedlinearincreaseandtransition intoaconstant lowatthemaximumlevel.Thiscor‐rectionwillenhancethereliabilityandvalidityofthe analysis,enablingmeaningfulconclusionstobedrawn fromthegraph(Figs.26–27).
Figure29. Flowsimulationiteration‐basedgraphic results‐2
Furtheranalysiswasconductedonthepropeller usinga lowsimulationprogramtodeterminethe rotationdirectionofthepropeller(Fig.28)andinves‐tigateanypotentialturbulencegeneratedduringits rotation.Thisanalysisaimedtogaininsightsintothe propeller’sbehaviorandunderstandthe lowdynam‐icsassociatedwithitsrotation.
Byutilizingthe lowsimulationprogram,detailed examinationswerecarriedouttovisualizeandstudy thepropeller’srotationcharacteristics.Thisincluded assessingthedirectionofrotationandstudyingthe lowpatternsandturbulencesthatmightariseduring propelleroperation.
Theanalysisprovidedvaluableinformation regardingthepropeller’sperformanceand low behavior.Theseinsightscanbeusedtooptimizethe propellerdesign,improveef iciency,andminimize anyundesirableeffectssuchasturbulenceor cavitation.
Duringtheanalysis,theoutputvaluesdemon‐stratedanotabledecreaseafterreachingapeakvalue, followedbylineardecreasesinsubsequentiterations. Thistrend,alongwiththeprolongedrundurations, con irmedtheaccuracyoftheanalysisresults.Since themotorsusedinthesystemprovideunidirectional rotation,thepropellerdirectionwasassumedtobe clockwisefortheanalysis.Thisassumptionwasbased onthefactthatthepropellersintheAUVworkin bothclockwiseandcounterclockwisedirections.The analysisresultsaredepictedinFigs.29–30
Descrip onValueCriteriaMean
GG Force (Z)1123,578N22,1985I-39,8686N
GG Average Dynamic Pressure 261828,1Pa1247,511I66462,2Pa
GG Average Speed (X) 4-7,17387m/sn0,129199-7,51505m/sn
GG Average Speed 38,66918m/sn0,0618679,04822m/sn
GG Average Total Pressure 1144056Pa9549,05I1517440Pa
GG Average Turbulent Intensity10,8485%0,97883510,6876%
GG Average Turbulent Distributed133,361W/Kg66,3415I149,113W/Kg
GG Average Turbulent Energy0,623994J/ Kg0,2050830,694007J/Kg
Figure30. Outputimageofflowsimulationresults‐2
3.2.ResultsoftheAUV
Followingthecompletionoftheelectronic, mechanical,andalgorithmpreliminarydesigns,the implementationandtestingphaseswereinitiated. The irststepinvolved3Dprintingofthepropellers responsibleformotormovementandwater lowin thepool.Subsequently,theassemblyofthemotors, propellers,andthrusterwascarriedout.However, itwasdiscoveredthatthenutusedtoconnectthe propellertothemotorshaftcauseddamagetothe propellerskirts.
Inthenextstage,theassemblybetweenthepower unit,ESC,andthemotorwasestablishedandsub‐jectedtotesting.Duringthistestingphase,itbecame evidentthattheESCsandconnectioncableswitha resistanceof30Awerepronetooverheating,which couldpotentiallyleadtomalfunctionsinthevehicle.
Furthermore,underwatertestingwasconducted toassesstheperformanceofthemotorsandpro‐pellers.Unfortunately,thedesiredef iciencywasnot achievedduetoaweakconnectionbetweenthemotor andthepropeller.Itwasalsoobservedthattheblade angleofthepropellerbladescontributedtotheinabil‐itytoattainthedesiredef iciency.
Basedonthese indings,modi icationsweremade tothepropellerdesigntoaddresstheidenti iedissues andimproveperformance.
Toaddresstheissuesencounteredduringthetest‐ingphases,severalmodi icationsandadjustments weremadetothedesignandcomponentsofthe vehicle.
First,anutslotwasaddedtothepropellerdesign toensureaproperconnectionbetweenthemotorand thepropeller.Thismodi icationresolvedtheprevious issuesrelatedtotheconnection.
Next,thebladeangleofthepropellerwasreduced toenhanceitsef iciencywhileoperatingunderwa‐ter.Thisadjustmentimprovedthepropeller’sperfor‐manceandcontributedtomoreef icientpropulsion.
TomitigatetheheatgeneratedbytheESCand cables,anESCwith40Aresistanceandthickercables wereintegratedintothevehicleinsteadofthe30A ESC.Thisupgradehelpedpreventoverheatingand potentialmalfunctions.
Intheelectronicdesignofthevehicle,twocopper cableswereinitiallyemployedforpowerdistribution. However,itwasdiscoveredthatthesecables,like theESCconnectioncables,wereoverheatingbeyond acceptablelevels.Additionally,theRaspberryPimini‐computerexperiencedthesameoverheatingissue. Measuresweretakentoaddresstheseproblemsand ensureproperheatdissipationwithintheelectronic components.
Duringthesensortestingphase,itwasfoundthat thesonarsensor’sacousticwavescouldnotpass throughthefrontcoverframeinthetube,resulting ininadequatedata.Subsequently,aconnectionwas establishedbetweentheRaspberryPiandPixhawk viathePixhawk’sUSBport.Unfortunately,thiscon‐nectionmethodledtodelayeddataprocessingand inef icienciesinhandlingtherequesteddata.
Finally,itwasdeterminedthatthebuzzersen‐sorsintendedfordetectingthepingerballunderwater werenotoperatingef icientlywithinthetube.Fur‐therimprovementsoralternativeapproacheswere exploredtoaddressthislimitation.
Byimplementingthesemodi icationsandadjust‐ments,effortsweremadetoenhancetheoverallfunc‐tionalityandef iciencyofthevehicleanditscompo‐nents.
Inresponsetotheencounteredsituations,several changesandadjustmentsweremadetoimprovethe system’sperformanceandef iciency.
Toaddresstheoverheatingandpowerdistribution issues,coppercableswerereplacedwithmorerobust copperplateswithastrengthratingof400A.This upgradeensuredreliablepowerdistributionwithin thesystem.Additionally,ametalcoolerwasintegrated topreventoverheatingoftheRaspberryPiminicom‐puterandenhancetheef iciencyoftheprocessor.
TheconnectionbetweentheRaspberryPiandPix‐hawkwasestablishedviathetelemetryport,allow‐ingforsmootherandmorereliablecommunication betweenthetwodevices.
Toenhancethedatacollectioncapabilitiesofthe sonarandbuzzersensors,water‐resistantmodelsof thesesensorswereordered.Thisupgradeaimedto providemoreef icientandreliabledataacquisitionin underwaterconditions.
Inthealgorithmdesignprocess,aninitial approachinvolvedasinglealgorithmtohandleall tasks.However,itwasrecognizedthatthisapproach mightleadtochallengesincompletingthetasks effectively.Asaresult,arevisedstrategywasadopted, whereinalgorithmsweredevelopedindividuallyfor eachspeci ictask.Thisiterativeapproachensures thateachtaskisaddressedeffectivelyandimproves theoverallperformanceofthesystem.
Byimplementingthesechangesandre ining thealgorithms,effortsweremadetoovercomethe encounteredissuesandenhancethesystem’sef i‐ciencyandperformance.
Withthegrowingdemandsandadvancementsin technology,thereisanoticeableincreaseininter‐estsurroundingunmannedandautonomousvehicles. Recognizingthistrend,thedeploymentofunderwa‐tervehicleswithsuchcapabilitiesholdssigni icant promiseforourcoastalnation.Thepotentialcontribu‐tionsofthesevehiclesspanvariousdomains,encom‐passingscienti icresearchandenvironmentalpreser‐vation.
Notably,autonomousunderwatervehiclestudies areinfrequentinourcountry.Againstthisbackdrop,a researchprojecthasbeenundertakentoprovideasig‐ni icantadditiontothecurrentliterature.Theobjec‐tiveistoexploreandadvanceautonomousunder‐watervehicles,therebyenrichingtheknowledgeand comprehensionwithinthisdomain.Thisinitiative, byaddressingtheresearchgap,aimstouncoverthe potentialbene itsandapplicationsofautonomous underwatervehiclestailoredtoourspeci iccontext.
Thisstudyconductedacomprehensiveinvesti‐gationintothemechanical,electrical‐electronic,and softwareprerequisitesessentialforthecreationof anunmannedandautonomousunderwatervehicle. Emphasiswasplacedonidentifyingcrucialmateri‐alsindispensableforthevehicle’sproduction,accom‐paniedbyadetailedexplorationoftheproduction methodsandequipmentintegraltothemanufacturing process.
Themechanicalrequirementsencompassthe structuraldesign,propulsionsystem,buoyancy control,andothermechanicalcomponentscrucial forunderwatervehicleoperation.Theelectrical‐electronicaspectscoverthenecessarysensors, communicationsystems,powerdistribution,and controlmechanismsrequiredforautonomous functionality.
Additionally,thearticleexploredthesoftware requirementsinvolvedinprogrammingthe vehicle’sautonomousbehavior,includingnavigation algorithms,sensorfusiontechniques,andmission planning.
Furthermore,attentionwasgiventothemateri‐alsandmanufacturingtechniquesemployedinthe constructionoftheautonomousunderwatervehi‐cle.Thearticleprovidedinsightsintotheselection ofsuitablematerials,fabricationmethods,assembly procedures,andqualitycontrolmeasurestoensure thevehicle’srobustnessandreliabilityinunderwater environments.
Byaddressingthesekeyaspects,thearticleaimed toofferacomprehensiveoverviewoftherequire‐ments,materials,productionmethods,andequipment involvedinthedevelopmentofanunmannedand autonomousunderwatervehicle.
Afterconductingathoroughliteraturereview,the minimumrequirementsforautonomousunderwater vehicleswereestablished.Basedonthese indings,a mechanicaldesignwascreatedusingSolidWorks,a 3Ddrawingprogram,andtheproductionwasrealized usinga3Dprinter.Furthermore,calculationswere performedtoensuresuitabilityforunderwatercondi‐tions,andappropriatematerialssuchasmotors,ESCs, andpowerunitswerecarefullyselectedandprocured.
Toenableautonomouscapabilities,acombina‐tionofsensors,aRaspberryPiminicomputer,and aPixhawk lightcontrollerboardwereincorpo‐rated.Essentialdrivingandimageprocessingsoft‐warewasdevelopedtofacilitateautonomousoper‐ations.Enhancementsintheutilizationofelectronic sensorscangreatlycontributetofuturestudies,offer‐ingimprovedef iciencyandguidance.Moreover,a moreef icientintegrationofsensorselectionandsoft‐wareforunderwatersounddetectioncanenhancethe vehicle’sadaptabilitytounderwaterconditions.
Recommendationsforenhancingtheperformance andreliabilityoftheAUVincludeexploringintegrated solutionsforwaterproo ingthevehicle’smotorsand conducting lowandstrengthanalysesusingdiverse materials.Thesemeasuresaredeemedessentialfor extendingthelongevityandensuringthereliability ofthevehicle,particularlyinchallengingunderwa‐terenvironments.The indingsunderscorethesignif‐icanceofongoingresearchanddevelopmentefforts aimedatoptimizingtheperformanceandcapabili‐tiesofautonomousunderwatervehicles.Incorporat‐ingthesuggestedimprovementsinsensorintegration, softwaredevelopment,andmaterialanalysisiscrucial forfurtherenhancingthevehicle’sfunctionalityand adaptabilityinunderwaterscenarios.
AUTHORS
IsmailBogrekci –DepartmentofMechanical Engineering,FacultyofEngineering,AydinAdnan MenderesUniversity,Efeler,Aydin,Türkiye/ AlproboticsInc.,Co‐founder,Aydin,Türkiye,e‐mail: ibogrekci@adu.edu.tr.
PinarDemircioglu∗ –InstituteofMaterialsSci‐ence,TUMSchoolofEngineeringandDesign,Tech‐nicalUniversityofMunich(TUM),Garching,Munich, 85748,Germany/Dept.ofMechanicalEngineering, FacultyofEngineering,AydinAdnanMenderesUni‐versity,Aydin,Turkey/AlproboticsInc.,Co‐founder, Aydin,Türkiye,e‐mail:pinar.demircioglu@tum.de, pinar.demircioglu@adu.edu.tr.
GoktugOzer –AlplerAgriculturalMachinery, R&DManager,Aydin,Türkiye,e‐mail: goktug.ozer@alpler.com.tr.
∗Correspondingauthor
WewouldliketothankADUBAPResearchInfras‐tructureProject(projectnumberMF‐22005)forsup‐portingthisstudy.AlproboticsandAlplerAgricultural Machineryhavecontributedtotheexperiments.
[1] W.Linling,Z.Daqi,P.Wen,andZ.Youmin,“A SurveyofUnderwaterSearchforMulti‐Target usingMulti‐AUV:TaskAllocation,PathPlanning, andFormationControl,” OceanEngineering,vol. 278,2023,114393;doi:10.1016/j.oceaneng.2 023.114393.
[2] E.S.Alietal.,“ASystematicReviewonEnergy Ef iciencyintheInternetofUnderwaterThings (IoUT):RecentApproachesandResearchGaps,” JournalofNetworkandComputerApplications, vol.213,2023,pp.1–22,103594;doi:10.1016/ j.jnca.2023.103594.
[3] B.Zhangetal.,“AutonomousUnderwaterVehicle Navigation:AReview,” OceanEngineering,vol. 273,2023,113861,pp.1–29;doi:10.1016/j.oc eaneng.2023.113861.
[4] A.Bahr,J.J.Leonard,andA.Martinoli,“Dynamic PositioningofBeaconVehiclesforCoopera‐tiveUnderwaterNavigation,”In: 2012IEEE/RSJ InternationalConferenceonIntelligentRobots andSystems,2012,pp.3760–3767;doi:10.110 9/IROS.2012.6386168.
[5] C.Germanetal.,“HydrothermalExplorationwith theAutonomousBenthicExplorer,” DeepSea ResearchPartI:OceanographicResearchPapers, vol.55,2008,pp.203–219;doi:10.1016/j.dsr. 2007.11.004.
[6] B.Allottaetal.,“TheARROWSProject: RoboticTechnologiesforUnderwater Archaeology,” IFAC-PapersOnLine;vol.48, no.2,2015,pp.194–199,ISSN2405‐8963;doi: 10.1016/j.ifacol.2015.06.032.
[7] F.Schill,A.Bahr,andA.Martinoli,“Vertex: ANewDistributedUnderwaterRoboticPlat‐formforEnvironmentalMonitoring,”In: DistributedAutonomousRoboticSystems.Springer ProceedingsinAdvancedRobotics,vol.6,Cham: Springer,2018;doi:10.1007/978‐3‐319‐7300 8‐0_47.
[8] R.Katzschmannetal.,“ExplorationofUnder‐waterLifewithanAcousticallyControlledSoft RoboticFish,” ScienceRobotics,vol.3no.16, 2018,eaar3449;doi:10.1126/scirobotics.aar3 449.
[9] T.Wuetal.,“AHydrothermalInvestigationSys‐temfortheQianlong‐IIAutonomousUnderwa‐terVehicle,” ActaOceanologicaSinica;vol.38,
2019,pp.159–165;doi:10.1007/s13131‐019‐1408‐4.
[10] G.Lietal.,“Self‐PoweredSoftRobotintheMar‐ianaTrench,” Nature,vol.591,2021,pp.66–71; doi:10.1038/s41586‐020‐03153‐z.
[11] A.Palomer,P.Ridao,andD.Ribas,“Multibeam 3DUnderwaterSLAMwithProbabilisticRegis‐tration,” Sensors,vol.16,no.4,2016:560;doi: 10.3390/s16040560.
[12] R.Kimuraetal.,“GuidanceandControlofan AutonomousUnderwaterRobotforTracking andMonitoringSpilledPlumesofOilandGas fromSeabed,”In: TheTwenty-ThirdInternationalOffshoreandPolarEngineeringConference OnePetro,2013,pp.366–371.
[13] G.Marani,S.K.Choi,andJ.Yuh,“Underwater AutonomousManipulationforInterventionMis‐sionsAUVs,” OceanEngineering,vol.36,no.1, 2009,pp.15–23;doi:10.1016/j.oceaneng.200 8.08.007.
[14] M.J.Hamilton,S.Kemna,andD.Hughes, “AntisubmarineWarfareApplicationsfor AutonomousUnderwaterVehicles:TheGLINT09 SeaTrialResults,” J.FieldRobotics;vol.27,2010, pp.890–902;doi:10.1002/rob.20362.
[15] D.P.Williams,“OnOptimalAUVTrack‐Spacing forUnderwaterMineDetection,”In: 2010 IEEEInternationalConferenceonRoboticsand Automation,IEEE,2010,pp.4755–4762;doi: 10.1109/ROBOT.2010.5509435.
[16] M.Pratsetal.,“Recon igurableAUVforInter‐ventionMissions:ACaseStudyonUnderwater ObjectRecovery,” IntelligentServiceRobotics,vol. 5,2012,pp.19–31;doi:10.1007/s11370‐011‐0101‐z.
[17] G.Casalinoetal.,“UnderwaterIntervention Robotics:AnOutlineoftheItalianNational ProjectMARIS,” MarineTechnologySocietyJournal,vol.50,2016,pp.98–107;doi:10.4031/MT SJ.50.4.7.
[18] G.Ozer,“DevelopmentofAutonomousUnder‐waterVehicle,”UnpublishedM.Sc.Thesis,2023‐M.Sc.‐032.AydınAdnanMenderesUniversity, Türkiye,2023.
Abstract:
COMPARISONOFOPENSOURCESDNCONTROLLERSANDCLOUDPLATFORMSIN
COMPARISONOFOPENSOURCESDNCONTROLLERSANDCLOUDPLATFORMSIN
COMPARISONOFOPENSOURCESDNCONTROLLERSANDCLOUDPLATFORMSIN TERMSOFPERFORMANCE,STABILITY,ANDINFRASTRUCTUREFLEXIBILITY
Submitted:27th March2024;accepted:16th April2024
AndrzejMycek
DOI:10.14313/JAMRIS/4‐2024/31
TheITindustryisadvancingrapidly,withvirtuallyevery branchofmoderncomputingexperiencingswiftdevelop‐ment.ConceptssuchasCloudComputingandArtificial Intelligencenolongersurpriseanyone.Recently,Soft‐wareDefinedNetworks(SDN)havebeengainingsignif‐icantpopularity.Thisinnovativeapproachtocomputer networksallowsforgreaterflexibilityandis,therefore, muchmorewell‐knownintheworldofcloudcomputing thanintraditionalnetworkimplementations. ThispaperintroducestheconceptofSDNandNetwork FunctionsVirtualization(NFV)andoutlinesallthechal‐lengesandsecurityissuesassociatedwiththecloud environment.ThedynamicnatureoftheITlandscape requiresconstantadaptationtoemergingtechnologies, andSDNrepresentsanoteworthyevolutionintherealm ofcomputernetworking.PlatformssuchasSDNand open‐sourcetoolsenablingthecreationofprivatecloud environmentssuchasOpenStackorOpenNebulawere compared.Atthesametime,aspectslikesecurity,net‐workperformance,flexibility,andscalabilitywereana‐lyzed.Basedontheprioranalysis,acomprehensivecloud environmentwasbuiltusingtheOpenStacksolutionand SDN‐OpenDaylightwasdeployed.Additionaltestscon‐ductedontheOpenStackcloud,bothwithandwithout SDN,demonstratedthesuperiorityofSDNimplementa‐tioninthecloud.
Keywords: Software‐definednetwork,NFV,cloudcom‐puting,OpenDaylight,OpenStack,OpenNebula
1.Introduction
TheconceptofSoftware‐De inedNetworking (SDN)positsthatnetworksshouldbedesignedto beeasilymanagedthroughspecializedsoftware. Thefoundationofthisapproachundoubtedlylies intheseparationofindividualcomponentsthat communicatewitheachotherthroughinterfaces. Controlfunctionsmustbecentralizedonthe controller,whilethedevicesareresponsiblesolelyfor datatransmissionandexecutinginstructionsreceived fromthecontroller.
ThisentailsaclassicdivisionintotheControlPlane (controller)andDataPlane(packetforwarding)[11]. EquallyimportantistheApplicationPlane.Onthis level,applicationsaredeveloped,enablingcommuni‐cationandinteractionwiththeentirearchitecture. TheApplicationPlaneisatypicalabstractlayer[4].
TheSDNtechnologyoriginatedfromtheresearch projectCleanSlateatStanfordUniversity,partly inspiredbytheOpenFlowprotocol.Theoperational conceptofOpenFlowwasinitiallyintroducedinthe papertitled’OpenFlow:EnablingInnovationinCam‐pusNetworks’byStanfordUniversityin2008,align‐ingwiththeideaofNetworkFunctionsVirtualization (NFV)[15].
Ayearlater,ProfessorNickMcKeownformally presentedtheconceptofSDN.In2012,theAmerican companyGooglesuccessfullyimplementedSDNtech‐nologyinitsbackbonenetwork,signi icantlyincreas‐ingGoogle’sSDNutilizationtoover70%.Thisevent markedabreakthroughinthedevelopmentandfuture ofSDNnetworks,promptingmajorglobaltechnology companiestointroducetheirSDNsolutionstothe marketprogressively[31].
SDNnetworksarecommonlydividedintoUnder‐layandOverlaynetworks.Whilethesetermsmay soundmysterious,itisinterestingthatwell‐known technologies,suchasIPsecandMPLS(Multiprotocol LabelSwitching),involveOverlaynetworks.TheOver‐laylayerinSDNnetworksiswhereintelligentfunc‐tionssuchassegmentationandvariouspoliciesare implemented.Despitevariationsincommunication protocolsattheOverlaylayer,dependingontheman‐ufacturer,theVXLAN(VirtualExtensibleLAN)pro‐tocoliscommonlyusedforencapsulationtoaddress scalabilityissues[31,32].
Ontheotherhand,theUnderlaynetworkis designedformaximumsimplicity,servingas aroutableIPnetwork.ThisiswhysomeSDN solutionsmayinvolvedevicesofbotholderand newergenerations,withcriticalOverlaynetwork functionsimplemented.TheUnderlaynetworkhas fewertasksandisintendedtoprovidestraightforward routing.Overall,SDNtechnologyoffersanuanced architecturethatcombinessimplicityintheUnderlay networkwithintelligentfunctionalityintheOverlay network.
Additionally,threeofthemostinvolvedcompanies intheproject,namelyCisco,JuniperNetworks,and ExtremeNetworks,havedisclosedtheirdevelopment plansfortheNFVarchitectureproposedbytheEuro‐peanTelecommunicationsStandardsInstitute(ETSI) [31].InadditiontotheITmentionedaboveindustry giants,thereistheOpenDaylightinitiative,whosetask istopromoteimplementations.Duetocompatibility requirements,OpenDaylightistailoredtothestan‐dardSDNONNarchitecturealongwithintegration withNFV.
TheSDNnetwork’sstructureinOpenDaylightis dividedintothreeparts:
‐ Networkapplicationsandbusinessprocesses
‐ Thecontrolplatform
‐ Virtualandphysicalnetworkdevices
OpenDaylightprovidesaJavaVirtualMachine (JVM),andtoenhancecompatibilitywithmodules fromothercompanies,OpenDaylightalsofeatures plug‐inmodulesthatallowforadditionalextensionof SDNfunctionality[5].
2.SDNNetworkRequirements
TheimplementationofSDNatanappropriatelevel involvesmeetingaseriesofrequirements.Asmen‐tionedearlier,oneofthemostcrucial,ifnotthemost critical,requirementsisthevirtualizationoftheentire network(overlayandunderlaynetworks).NFV(Net‐workFunctionsVirtualization)providesbothinternal andexternalvirtualization.Internalvirtualizationis used,forexample,forvirtualizingnetworkdevices thatserveasnetworkelements.Ontheotherhand, externalvirtualizationconnectsnetworks,thuscreat‐ingauni iedvirtualnetwork[23].Equallyimportant istheApplicationPlane.Onthislevel,applicationsare developed,enablingcommunicationandinteraction withtheentirearchitecture.TheApplicationPlaneis atypicalabstractlayer[32].
SDNnetworksarealsocharacterizedbyautoma‐tion.Byeliminatingrepetitivetasksoftenperformed intraditionalnetworks,wecanrapidlycreateenvi‐ronmentsandautomaticallysetnetworkparameters relatedtosecurityorQualityofService(QoS)poli‐cies.Anotherelementthatperfectlyencapsulatesthe ideaofSoftware‐De inedNetworksisprogrammabil‐ity.SDNaddresseschallengestraditionalnetworks strugglewithbecausetheirconceptreliessolelyon packetexchangebetweenhosts.
However,thevastamountofdatatransmitted overtheInternettoday,coupledwiththeevolu‐tionoftechnologieslikeInternetProtocolTelevision (IPTV),videoconferencing,VoiceoverInternetPro‐tocol(VoIP),therapidincreaseinnetwork‐connected devices,andonlinegaming,ledtothequestfora newparadigm.TheprogrammabilityofSDNnetworks makesthemresemblemobileapplicationsonsmart‐phonesratherthantraditionalnetworks.Network Engineerscanseamlesslyprogramandmanagethese networksthroughApplicationProgrammingInter‐faces(APIs).Additionally,openAPIsfacilitateintegra‐tionwithothertools[19].
SDNcontinuestogainpopularity.Wepreviously facedtechnologicalgapsinnumerousareas,andSDN hasbeen illingthesegaps.Currently,themostcru‐cialdomainwhereSDNissuccessfullyutilizedisin DataCenters.ThehistoryofSDN(Software‐De ined Networking)canbetracedbacktoDataCenters.Some evenrefertoSDNnetworksasSoftware‐De inedData Centers(SDDC).Thisdesignationisnotsurprising, giventhatimplementingSDNinDataCentersallows forautomation,arevolutionaryapproachtonetwork management,andtheabilitytocreatenewenviron‐ments.SDNisanexcellentideainenvironmentsgrap‐plingwithsecurityissuesandcomplexinfrastructure. Inhomogenousenvironments,deployingSDNona largescalemaynotbepractical.
Currently,themostpopularcommercialSDNsolu‐tioninthemarketisundoubtedlyCiscoACI.Itserves asanSDNcontroller,offeringacomprehensivesetof IPsolutionswithfullintegrationfromthedatalink layertotheapplicationlayerwithintheSDNnetwork. Additionally,itsupportstheVXLANprotocolandNFV. Oneoftheadvantagesofthissolutionisitscapabil‐ityforclouddeployment,andthetoolitselfsupport AWSCloud.AlsohighlypopularareVMwareNSXand JuniperContrailNetworking.Anessentialdistinction betweenCiscoACIandVMwareNSXisthatCiscoACI isasolutionencompassingsoftwareandhardware components.Itsimplementationrequiresbuildingthe infrastructurearoundNexus9000switchesoperating inaSpine‐Leafarchitecture.Incontrast,VMwareNSX operatesexclusivelyattheoverlaylayeranddoesnot directlyimpactthephysicalnetworklayer.However, anundeniableadvantageisthatitcanfunctionin almostanyinfrastructure[12].
Amongopen‐sourcesolutions,ONOSSDNcon‐trollerandOpenDaylighttakethelead.Inthelater partofthepaper,OpenDaylightwasutilized.This solutionisthefoundationforcommercialcontrollers likeFujitsuVirtuoraandEricssonSDNController. OpenDaylight’sstrengthsinclude,amongothers,sup‐portformultipleprotocolsintheServiceAbstraction Layer(SBI),encompassingprotocolssuchasBorder GatewayProtocol(BGP),OpenFlow,NETCONF,Sim‐pleNetworkManagementProtocol(SNMP),andOpen vSwitchDatabase(OVSDB)[2].
AnotherareawhereSDNisgainingpopularityis campusnetworks,withusersbeingtheprimaryfocus inthiscase.SDNleveragesthetechnologyofnumer‐icalcontrolseparationtoachievecentralizedlogic managementandenhancetheprogrammabilityofthe controlplane.Thissigni icantlyfacilitatesthesimpli‐icationofnetworkmanagement.Additionally,itpos‐itivelyimpactsperformanceandsecurity,whichisa signi icantadvantagebecause,asweknow,traditional campusnetworkslack lexibility,makingchanges challengingandsigni icantlyaffectingresponsetimes incaseoffailuresandassociatedcosts.Thethirdarea wheretheutilizationofSDNtechnologyisexperi‐encingsigni icantgrowthisinWideAreaNetworks (WANs).
Figure1. OpenDaylightArchitecture[3]
TheSoftware‐De inedWideAreaNetwork(SD‐WAN)isparticularlywell‐knownincomputernet‐works.Thistechnologyenablesprogrammaticcontrol ofloadbalancingandrouting,aligningseamlesslywith theconceptknownasApplication‐drivennetwork‐ing.Theexpectationfromthisapproachisthatthe networkwillcatertotheneedsofbothcustomers andservices,aligningwithvariousapplications[27]. Themostpopularprojectshowinghowimportant thistechnologyisGoogle’sproject,whichbuilttheB4 structurebasedonSDN[31].
Despiteitsnumerousadvantages,SDNarchitec‐turealsocomeswithsecuritychallenges.Beyondthe classicalthreatvectorssuchasDenialofService(DoS), dataleaks,orunauthorizedaccess,SDNintroduces speci icthreatsuniquetoitsarchitecture.Examples ofthesethreatsincludeattackstargetingthecon‐trollersoftwareandattemptstodisruptcommunica‐tionbetweenthecontrolplaneandthedataplane,as wellasbetweenthecontrolplaneandtheapplication plane[1].Additionally,controllerswithvulnerabili‐tiescancausesigni icantharmtoournetwork.An attackergainingaccesstothecontrollercanmanage ournetworkbyprogrammingswitches.
IssuesrelatedtoMan‐in‐the‐Middle(MITM) attacksareundoubtedlyoneofSDN’smostcritical securityconcerns.AsuccessfullyexecutedMITM attackallowseavesdroppingandmodifyingtraf ic lowbetweenroutersandthenetworkendpoint. SecuringthecommunicationchannelinSDNinvolves usingtheTransportLayerSecurity(TLS)protocol, whichissupportedbyOpenFlowbydefault.However, itisessentialtonotethattheTLSprotocolandPublic KeyInfrastructure(PKI)willnotprotectusifthe attackergainsaccesstothecontrolplane.Atthis stage,theattackercaneasilyleverageswitchesto launchDistributedDenialofService(DDoS)attacks. AnotheroverlookedpointinSDNsecurityisthe computersconnectedtothecontroller.Computers areoftenoverlooked,andtheissueofsecurityis downplayed.Inthecaseofvulnerabilitiesthrough computers,gainingaccesstothecontrollercanbe easilyaccomplished[26].
Despiteitsincrediblecapabilities,SDNisunfortu‐natelysusceptibletoattacksandfailures.Forinstance, intheeventofacontrollerfailure,itisadvisableto havereplicatedcontrollersprepared.Itisalsorecom‐mendedtousedifferentcontrollers,ashavingidenti‐caloneseverywhereintroducestheriskofencounter‐ingthesamesoftwarebugsacrosstheentirenetwork [13].
Accesstothecontrollermustbesecurelyguarded, andcommunicationwiththecontrolplaneshould onlybeallowedfortrustedsystemsandadminis‐trators.InthecontextofSDN,itiscrucialtorec‐ognizetheAAAmethod(Authentication,Authoriza‐tion,andAccounting).Authenticationmechanisms suchascerti icates,passwords,ortokensshould beimplemented.Theauthorizationprocessshould clearlyde inethepermissionsandaccesstospeci ic resourcesthatanindividualhasonthecontrolplane. CriticaltosecuritycontextsareIntrusionDetec‐tionSystems(IDS).IDSisutilizedinbothtraditional infrastructureandcloudinfrastructure.Theroleof IDSistoanalyzeandidentifysuspiciousactivities, promptlynotifyingITsecurityteams[17].
SpeakingaboutSDN,wemustrememberthe OpenFlowprotocol.Unfortunately,inthiscase,we encounteradditionalsecuritychallenges.Acrucial matteristheprotectionofthecontrolplaneagainst DoS/DDoSattacks.Aspecializedframeworkcalled AVANT‐GUARDhasbeendevelopedtoaddresssuch threatsinOpenFlow‐basednetworks.Thisframework consistsoftwomodulesandislocatedinthedata plane[28].
Intoday’sITindustry,twosolutionsstandoutin popularity.Undoubtedly,theseareArti icialIntelli‐genceandCloudComputing.Inthisdiscussion,we willfocusonthelatter,namelycloudcomputing.Asis customaryintheITindustry,everybranchofmodern computingevolvesalmostdaily,andthesameholds forcloudcomputing.Theconceptofcloudcomputing isnotnew—ithasbeenaknownmodelfordeliver‐ingservicesovertheInternetforseveralyears.Cloud computingoffersvariousservices,withthemostpop‐ularmodelsundoubtedlybeingInfrastructureasa Service(IaaS),PlatformasaService(PaaS),andSoft‐wareasaService(SaaS).
IntheIaaSmodel,theserviceprovidermakes theirinfrastructureavailabletous(suchasvirtual machinesandloadbalancers).PaaSallowsusto deployourapplicationsusingtheprovider’sinfras‐tructure,whileSaaSissimplypre‐builtsoftwarefrom thecloudproviderthatwecanuseonthecloudplat‐form[18].
Itisinconceivabletoenvisiontheoperationofeven asmallorganizationwithoutleveragingthebene itsof cloudcomputing.However,withthedevelopmentof thisserviceandthesimultaneousincreaseinitspop‐ularity,itbecomesmorevulnerabletocybercriminal attacks.Hence,oneoftheprimaryelementsconstantly addressedbycloudserviceprovidersisimproving theoverallsecurityoftheirservices.Traditionalnet‐works,eveninthecaseofcloudserviceproviders,are nowbeingreplacedbySDNtoenhancesecurity[23]. Becauseeverysecondseesamassiveamountoftraf ic generatedbycloudclients,notonlyissecuritycrucial inthecaseofcloudnetworks,butsoisreliability. OptingforaSDNinsteadoftraditionalnetworkscan bene itusascloudserviceproviders[9].Thereare plentyofexamplesofsuchadvantages,andamongthe mostsigni icantarethe“programmable”approachto networkmanagement,theabilitytoexperimentwith networkswithoutimpactingtheproductionenviron‐ment,thepotentialfornetworkgranularity,andthe enhancementofitsresponsiveness.Traditionalclouds areincrediblyreliableandsecure,butthereisand willneverbeanytechnologythatis100%secure. TheAchilles’heelofcloudscanbeDoS/DDoSattacks, sotomitigatethisthreat,itisadvisabletoabandon traditionalnetworksinfavourofSDN,whichfeatures anisolatedcontrollayerfromthedatalayer[23].
AsforSDNcontrollers,numerousoptionsare availableinthemarket,butOpenDaylighthasbeen selectedforthisstudy.BothOpenDaylightandFlood‐lightareopen‐sourceSDNmanagementtools,provid‐ingsigni icant lexibility,whichallowsthemtobeused invariousenvironments.Undoubtedly,theadvantage ofOpenDaylightliesinitscomplexity,makingitsuit‐ableformoreadvancedprojects,andthefactthat asigni icantlylargercommunitydevelopsit.Inarti‐cle[24],theauthorscomparedcontrollers,analyz‐ingnetworkperformance,architecture,andQoS.The researchwasconductedwithparticularemphasison delaysanddatalossinvariousnetworktopologiesand usagescenariossuchascloudcomputingormultime‐diaprocessing.Theresultsofthetestsconductedby theauthorsindicatedthatinasmanyas95%ofcases, OpenDaylightoutperformedFloodlight’squality.
TheauthorscomparedOpenDaylightwithFlood‐lightacrossthreedifferentnetworktopologies,con‐sideringthreescenarios:onewithnocross‐traf ic, anotherwherenearlyhalfofthebandwidthwascross traf ic,andathirdwherethewholebandwidthwas illedwithcrosstraf ic.
Table1. Bestcontrollerindifferentsituations[24]
Topology Load Latency
Single Lowload OpenDaylight (2.5timesbetter)
Single Midload Same
Single Heavyload Same
Linear Lowload OpenDaylight (4.4timesbetter)
Linear Midload Same
Linear Heavyload Floodlight (1.2timesbetter)
Tree Lowload OpenDaylight (2.1timesbetter)
Tree Midload OpenDaylight (4.5timesbetter)
Tree Heavyload Same
Inthetests,emphasiswasplacedonlatency,jitter, packetloss,andthroughput.Theauthors’researchhas shownthatOpenDaylightperformssigni icantlybet‐terinenvironmentswheretreetopologiesarepreva‐lent,suchasinDataCentersandcloudcomputing environments(see:Table1).
Hence,thechoicefellontheOpenDaylightplat‐form.
Asigni icantfactorwasalsothatOpenDaylight issigni icantlymore lexiblethanFloodlight.Flood‐lightmainlyfocusesontheOpenFlowprotocol,while OpenDaylightsupportsOpenFlow,Netconf,andYANG. OpenDaylightisanopen‐sourceSDNcontrollerunder theauspicesoftheLinuxFoundation.Thefactthatit isanopen‐sourceprojectissigni icant,asanopen‐sourceproductwaschosentoimplementcloudcom‐putinginthiswork.OpenDaylightisfullyvendor‐independentandcansupportvariousnetworktech‐nologiesanddevices,providing lexibility.Withits modulararchitecture,itishighly lexibleandscalable. Moreover,thetoolisimplementedinJava,allowingit tobeinstalledonvirtuallyanyoperatingsystemwith aJavaVirtualMachine(JVM)[3].
OpenDaylightis,therefore,anexcellentchoice whenitcomestointegrationwiththeOpenStackplat‐form.Thisisparticularlyimportantbecauseanother dilemmawasthecloudplatform.
6.1.TheChoiceBetweenOpenStackandOpenNebula Twomainoptionswereconsidered:OpenStack andOpenNebula.Theseareopen‐sourcesoftware designedforcreatingbothprivateandpublicclouds.
OpenStackisreleasedundertheApachelicense andconsistsofvariousmodules.Itoriginatedin 2010asacollaborativeprojectbetweenNASAand RackspaceHosting.Overtime,theprojectsawincreas‐ingcontributionsfrommultiplecompaniesandorga‐nizations,includingmajorplayerssuchasRedHat, Huawei,Ericsson,Intel,Dell,T‐Mobile,Canonical, Fujitsu,HP,Cisco,andBloomberg.
Currently,theprojectismanagedbytheOpen InfrastructureFoundation(formerlyknownasthe OpenStackFoundation),bringingtogetherover500 companies[10].
ThelatestversionofOpenStack,2023.2Bobcat, consistsofover40componentsandmodules,manyof whichareoptional[21].
Thekeycomponentsinclude:
‐ Nova(Compute): amoduleformanagingvirtual machines(VMs)andoverseeingtheentirelifecycle ofinstantiatedinstances;
‐ Neutron(Networking): responsiblefornetwork‐ingandconnectionmanagementbetweenvarious instancesandresources;
‐ Cinder(BlockStorage): enablesblock‐leveldata storageinthecloud,whichiscrucialforvirtual machineinstances;
‐ Swift(ObjectStorage): amoduleresponsiblefor storingandprovidingaccesstoobjects,suchasmul‐timedia iles;
‐ Glance(Imageservice): amodulethatincludesdis‐covering,registering,andretrievingvirtualmachine images;
‐ Keystone(Identity): facilitatesauthenticationand identitymanagementforallOpenStackcomponents. Amongotherpopularandavailablecomponents ontheOpenStackplatformare,forinstance,Ironic (BareMetalProvisioningService),Manila(providing ilesharingservicesinthecloud),Octavia(Loadbal‐ancer),Designate(DNSService),Barbican(keyand certi icatemanagement),Heat(Orchestration),Mag‐num(ContainerOrchestrationEngineProvisioning), Trove(DatabaseasaService),Horizon(Dashboard), andthemodernSkyline(Nextgenerationdashboard) [10,21].
OpenNebula,muchlikeOpenStack,isalsoanopen‐sourceprojectenablingthecreationofone’scloud computinginfrastructure.ItwasinitiatedbySpan‐ishresearchersIgnacioMartínLlorenteandRubénS. Montero.Theprojectaimedtocreateaplatformwell‐suitedformanagingvirtualmachinesindistributed infrastructures.
Figure4. SupportforresiliencyinOpenStackand OpenNebula[30]
Astheprojectevolved,theauthorsestablished C12GLabs,nowknownasOpenNebulaSystems, focusingondesigning,consulting,andimplement‐ingservicesbasedontheOpenNebulasolution.The softwaresupportsvirtualizationtechnologiessuch asVMware,KVM,LXD,Kubernetes,andDocker. Thankstoahighlyuser‐friendlyinterface,itallowsfor easyinfrastructurescalabilityandenablesswiftand straightforwarddeploymentanddeliveryofservices inthecloud[14,16].
OpenNebulaisafullyplatform‐independent Enterprise‐classproductwithanopen, lexible,and easilyextensiblearchitecture,makingitsuitable foruseasaprivatecloud,publiccloud,orin conjunctionwithanothercloudasahybridcloud. Thisplatformhasbeenimplementedinthemost popularprogramminglanguages,suchasJava,C, C++,andRuby.Itallowscentralizedmanagement throughdatastorage,monitoring,andnetwork virtualizationtechnologies.Additionally,itallows forintegrationwithOpenCloud,AmazonEC2, OpenStack,orOpenShift[14, 25].Whencreatinga cloudenvironmentbasedonOpenNebula,amachine mustbecon iguredasthefront‐end,whichwillhost thecloudmanager,whiletheremainingmachines mustbecon iguredasslavenodes[29].
Table2. IaaStoolssupportforresiliencyinOpenStackandOpenNebula[30]
Support OpenStack OpenNebula FullandBareMetalvirtualization VMware,Hyper‐V,KVM,Xen,VirtualBox VMware,KVM,Xen OS RHEL,Fedora,Debian,Ubuntu,SLES RHEL,Ubuntu,Debian,SLES,CentOS Network Neutron,OVS,NSX,PLUMgrid dummy,ebtables,OVS,VLAN,VMware Storage NFS,Ceph,LVM,Gluster,ZFS,Sheepdog NFS,Ceph ObjectStorage Ceph,Cinder Ceph Diskformats QCOW2,RAw,VHD,LVM,VMDK,VDI QCOW2,VMFS,LVM,RAW,DEV,Ceph ContainerVirtualization LXC LXC
InfavourofOpenStackcomparedtoOpenNeb‐ula,ithasamuchlargernumberoffunctionsand popularity,whichautomaticallytranslatesintomuch bettersupportintermsofthetool’screatorsand theinfrastructureprovidersthemselves.However,the inaldecisiontochooseaclouddevelopmenttool wasmadebasedon[30]’swork.Intheabovework, theauthorscomparedthe lexibility,performance, andresilienceofthemostpopularopen‐sourcetools fordeployingcloudcomputing:OpenStack,OpenNeb‐ula,andCloudStack.Theteststhatwereconducted showedthatOpenStackisthemostresilient.Tothor‐oughlyinvestigatetheseissues,theauthorsexpanded thetaxonomyprovidedin[8].Sincestabilityisthe primaryandmostimportantfactorincloudcomput‐ing,itwasthekeycriterionwhenchoosingatoolto createacloudcomputingenvironmentforresearch ontheimpactofSDNoncloudcomputing.System resilienceisnothingbuttheabilitytoadapttofailures andcontinuouschangeswithoutcompromisingthe levelofserviceavailability.Thisaspect’sdiversityof infrastructuretechnologiespositivelyaffectstheenvi‐ronment’sresilience.Table 2 presentsacomparison ofIaaStoolssupportingresiliency.Basedonthisdata, thesupportforresiliencywascalculatedconsidering thenumberofsupportedtechnologiesbythecloud creationtool.OpenStackeasilyoutperformedOpen‐Nebulahereduetoitsexcellentsupportforstorage, networking,containers,hypervisors,andoperating systems(see:Fig.4).
Anothercriticalcriterionwhenchoosingacloud computingtoolwasperformance.In[30],theauthors conductedperformancetestsofOpenStack,OpenNeb‐ula,andCloudStack.Theenvironmentconsistedoftwo components:thecloudcontrollerandthenode.Per‐formancewascalculatedbasedon40samplesineach micro‐testfollowing[22].Thetestswereconducted usingtoolssuchasLINPACK,STREAM,andIPerf,along withscienti icapplications[6, 7],onfouridentical Supermicroblades,eachequippedwith24GBRAM at1333MHz,IntelXeonX5560(4Cores,8Threads, MaxTurboFrequency3.20GHz,ProcessorBaseFre‐quency2.80GHz,Cache8MB),and3SATAII7200RPM physicaldisksinRAID5con igurationconnectedviaa gigabitnetwork[30].Intheperformancetestunder intensiveloads,OpenNebulaexhibitedapoordisk throughput,particularlyinwriteandrewriteoper‐ations.OpenStackoutperformedthecompetitionin thisaspect,andadditionally,theresultsofinstances createdinOpenStackshowedlessvariability.
Furthermore,inmemorytests,OpenStackdemon‐strateditssuperiorityovercompetitors.Considering theoften‐encounteredpoorperformanceofinstances createdinOpenNebulaandthefactthatOpenStack performedmostconsistentlyinthetestsconductedby theauthors,itwasdecidedtousetheOpenStacktool forSDNtestsinthecloud.
6.2.InstallationandIntegrationofTools
The irststepwasthepreparationofthelaboratory environment.Open‐sourcecloudcomputinginfras‐tructuresoftwarewasinstalledonaserveroperating underthecontrolofUbuntuServer22.04.3.Thissoft‐warewasOpenStack2024.1Caracalinthedeveloper version.Afterasuccessfulinstallation,basicadminis‐trativetaskswerecarriedout,suchasaddingimages withLinuxoperatingsystemstothecloud.
Thankstotheseactions,wehadapreliminary cloudenvironmentready.Toconducttests,creatinga virtualnetworkwithinthecloudprojectanddeploy‐inginstancesinitwasonlynecessary.Thetesting utilizedtheiperf3software,anopen‐sourcetoolcom‐monlyusedfornetworktesting(bandwidthmeasure‐ment)[6].
Aftercompletingtheinitialpartofthetests(as discussedinthenextsection),theOpenDaylightBoron softwarewasinstalledandintegratedwiththeOpen‐Stackcloudtocomparetheresultsoftestsbetweenthe ‘pure’cloudandwhenourcloudisintegratedwiththe SDNplatform.
6.3.TestswithoutSDN
Initially,bandwidthwasmeasuredusingtheiperf toolinthecloudenvironment(withoutSDN)for 10seconds.Subsequently,additionalmeasurements wereconductedfor20‐secondintervals.Inthe irst case,thesenderandreceiver’saveragebitratewas 146Mbits/secand145Mbits/sec,respectively.These numbersweresigni icantlybetterduringtheini‐tial20‐secondmeasurement,astheaveragedbitrate resultsindicatedathroughputof4Mbits/sechigher forboththesenderandreceiver.However,thesec‐ondmeasurementwitha20‐secondintervalyielded decidedlypoorerresults.Asimilarsituationoccurred inthecaseofmeasurementswithSDN,butthatwillbe discussedinthelaterpartofthearticle.
Thesecondpartofthecloudenvironmenttest withoutSDNinvolvedconductinganalogoustests exclusivelyfortheUDPprotocol.Twotests,eachlast‐ing20seconds,werecarriedout,revealingdifferences inthedeliverytimeofindividualpacketsaveraging 4.773and4.757milliseconds.
6.4.TestswithSDN
Toperformthispartoftheexperiment,installing andintegratingOpenDaylightwiththecloudwasnec‐essary.Afterasuccessfuldeployment,testswerecon‐ductedusingthesamemethodology,butthistime inanSDNenvironment.Theinitial10‐secondtest alreadydemonstratedthesuperiorityoftheSDN environment,showinganincreaseinbitrateby6 Mbits/sec.Inthesubsequenttwotestswitha20‐secondinterval,thebitrateincreasehoveredaround 15Mbits/sec.
Toconcludetheexperiment,verifyingtheresults oftheUDPprotocolwasessential.Here,too,the advantageofSDNwereevident,withlowerjitterval‐uescomparedtothenon‐SDNenvironment.
Betterresultswereconsistentlyachievedfor OpenStackintegratedwithOpenDaylightineverytest case—regardlessoftheprotocoltype,with10‐second and20‐secondintervals.Inthecaseofa10‐second interval,SDN‐basedresultswereover4%superior comparedtotheenvironmentwithoutOpenDaylight.
Table3. Transferandthroughputmeasurementsfora cloudnetworkwithoutSDN
Type Interval TotalTransfer ABR
sender (0‐10sec) 175MBytes 146Mbps
receiver (0‐10sec) 174MBytes 146Mbps
sender (0‐20sec) 357MBytes 150Mbps
receiver (0‐20sec) 357MBytes 149Mbps
sender (0‐20sec) 298MBytes 121Mbps
receiver (0‐20sec) 298MBytes 120Mbps
Table4. Transferandthroughputmeasurementsfora cloudnetworkwithSDN
Type Interval TotalTransfer ABR
sender (0‐10sec) 181MBytes 152Mbps
receiver (0‐10sec) 181MBytes 151Mbps
sender (0‐20sec) 393MBytes 165Mbps
receiver (0‐20sec) 393MBytes 164Mbps
sender (0‐20sec) 325MBytes 136Mbps
receiver (0‐20sec) 324MBytes 136Mbps
Figure7. Comparisonofthebesttransferresults obtainedforanenvironmentwithoutandwithSDN
For20‐secondintervalsinthetraditionalenvi‐ronment,theaveragebitrateforbothsenderand receiverhoveredaround150Mbits/secforthe irst andapproximately120Mbits/secforthesecondtests (see:Table3).
Inthe irsttestscenariofortheOpenStackenviron‐mentwithintegratedSDN,resultswereobtainedwith abitratelevelof152Mbits/sec.Asmentionedearlier, thisisover4%betterthaninthecaseofatraditional cloudenvironment.
Testsfor20‐secondintervalsalsoshowedthat OpenStackwithintegratedSDNismoreef icientand optimal.Inthe irsttestofthesecondtestscenario,an averagebitrateresultof165Mbits/secwasachieved (anincreaseof10%comparedtothetraditionalenvi‐ronment).
Anothertestonceagaindemonstratedtheadvan‐tageoftheSDNenvironment,whereabitrateincrease ofover13%wasrecordedcomparedtostandard OpenStack(see:Table4).
Table5. LatencyandthroughputmeasurementsforUDP inanon‐SDNenvironment
Type Interval ABR Jitter
sender (0‐20sec) 1.05Mbps 0.000ms
receiver (0‐20sec) 1.05Mbps 4.757ms
sender (0‐20sec) 1.05Mbps 0.000ms
receiver (0‐20sec) 1.05Mbps 4.506ms
7.1.TestsfortheUDPProtocol
The inalelementoftheexperimentinvolvedcon‐ductingtestsfortheUDPprotocol.Testsusingthe iperf3toolinUDPmodehelpidentifynetworkdelays andpacketlossandareextremelyvaluableformea‐suringbandwidth.Suchinformationisbene icial,for example,whenprovidingservicesrelatedtoreal‐time streaming.InUDPmode,theOpenStackenvironment withintegratedOpenDaylightperformedbetter.In bothtestcases,jitter(ameasureofthetemporalvari‐abilityofdelaybetweenpackets)waslowerintheSDN cloudenvironmentthaninthecaseofthetraditional environment.ThisindicatesthatOpenStackwithinte‐gratedSDNallowsformorestabledatatransmission inthenetwork.
Table6. LatencyandthroughputmeasurementsforUDP inanon‐SDNenvironment
Itisworthconsideringhowtheseresultswill translateinthecaseofavastandextensivelydevel‐opedcloudinfrastructure.SDNisadditionallyscalable and lexible,allowingfordynamicmanagementofnet‐workresources.Thankstoacentralizedapproach,it facilitatesresourcemanagementfromasinglepoint. Thecentralizedapproachisalsoanadditionaladvan‐tageintermsofsecurity,makingiteasiertoensure consistencyinnetworksecurity,whichcannotbesaid fortraditionalnetworks,asmentionedatthebegin‐ningofthestudy.Withtheconstantdevelopmentand popularityofcloudcomputing,SDNwillcontinueto evolveasthecharacteristicsofthissolutionaretai‐loredtosuchlarge‐scaleprojects.
AUTHOR
AndrzejMycek∗ –CracowUniversityofTechnol‐ogy,FacultyofComputerScienceandTelecom‐munications,DepartmentofComputerScienceul. Warszawska24,31‐155Cracow,Poland,e‐mail: andrzej.mycek@pk.edu.pl.
∗Correspondingauthor
References
[1] M.Akbaş,E.Karaarslan,andC.Güngör,“A PreliminarySurveyontheSecurityofSoftware‐De inedNetworks”, InternationalJournalof AppliedMathematics,ElectronicsandComputers, vol.4,pp.184–189,2016.
8.Conclusion
Currently,wearewitnessingcontinuousgrowthin cloudcomputing.However,thisextendsbeyondgiants likeAWS,Azure,orGoogleCloudPlatform.Thesame appliestoopensourceprojects,whicharedeveloping dailywiththesupportofnumerousITenthusiasts andwell‐knowncompanies.Currently,forourown needsorevenfortheneedsofourorganization,wecan createourcloudusingcloudcomputingtoolssuchas OpenStack,OpenNebula,ApacheCloudStack,orEuca‐lyptus.Ourcloudgivesusmorecustomizationoptions andcontroloveritsoperation.
Additionally,wecanin luenceitsperformance improvementthankstovariousadditionalmecha‐nisms.Thispaperdemonstratesthebene itsandpos‐sibilitiesgainedthroughSoftware‐De inedNetwork‐ing(SDN)intheOpenStackcloudenvironment.We canenhancenetworkperformance,streamlinethe managementprocess,andsigni icantlyelevatesecu‐ritythroughintegration.SDNcandynamicallycon‐troltraf ic,contributingtotheoptimizationofdata lowandminimizingdelays.Thebene itsofSDNin thecloudenvironmentwereobservedthroughexper‐imentsconducted.
[2] F.BadaroNetoetal.,“SDNControllers‐ACom‐parativeapproachtoMarketTrends”, 9thInternationalWorkshoponADVANCEsinICTInfrastructuresandServices(ADVANCE2021),pp.48–51,2021.
[3] S.BadotraandJ.Singh,“OpenDaylightasaCon‐trollerforSoftwareDe inedNetworking”, InternationalJournalofAdvancedComputerResearch, vol.8,pp.1105–1111,2017.
[4] M.BarreraPérezetal.,“Stateoftheartinsoft‐warede inednetworking(SDN)”, Visiónelectrónica,vol.13,pp.178–194,2019.
[5] B.Barrosetal.,“ApplyingSoftware‐de inedNet‐workstoCloudComputing”, 33rdBrazilianSymposiumonComputerNetworksandDistributed Systems,pp.54–107,2015.
[6] D.Bourilkovetal.,“UsingvirtualLustreclients ontheWANforanalysisofdatafromhighenergy physicsexperiments”, JournalofPhysics:ConferenceSeries,vol.396,no.3,2012.
[7] J.Dongarra,P.Luszczek,andA.Petitet,“TheLIN‐PACKBenchmark:past,presentandfuture”, ConcurrencyandComputation:PracticeandExperience,vol.15,pp.803–820,2015.
[8] R.DukaricandM.B.Juric,“Towardsauni iedtax‐onomyandarchitectureofcloudframeworks”, FutureGenerationComputerSystems,vol.29,no. 5,pp.1196–1210,2013.
[9] A.FressancourtandM.Gagnaire,“ASDN‐based networkarchitectureforcloudresiliency”, 2015 12thAnnualIEEEConsumerCommunicationsand NetworkingConference(CCNC),pp.479–484, 2015.
[10] D.Grzonka,“TheAnalysisofOpenStack CloudComputingPlatform:Featuresand Performance”, JournalofTelecommunications andInformationTechnology,vol.3,no.3,pp. 52–57,2015.
[11] A.Hakirietal.,“Software‐De inedNetwork‐ing:Challengesandresearchopportunitiesfor FutureInternet”, ComputerNetworks,vol.75,pp. 453–471,2014.
[12] P.Ijari,“ComparisonbetweenCiscoACIand VMWARENSX”, IOSRJournalofComputerEngineering,vol.19,pp.70–72,2017.
[13] D.Kreutz,F.Ramos,andP.Veríssimo,“Towards secureanddependablesoftware‐de inednet‐works”, SecondACMSIGCOMMWorkshoponHot TopicsinSoftwareDe inedNetworking,pp.55–60,2013.
[14] R.Kumaretal.,“OpenNebula:OpenSourceIaaS CloudComputingSoftwarePlatforms”, National ConferenceonComputationalandMathematical Sciences(COMPUTATIA-IV),2014.
[15] N.McKeownetal.,“OpenFlow:EnablingInno‐vationinCampusNetworks”, SIGCOMMComput. Commun.Rev.,vol.38,no.2,pp.69–74,2008.
[16] D.Milojičić,I.Llorente,andR.Montero,“Open‐Nebula:ACloudManagementTool”, IEEEInternetComputing,vol.15,no.2,pp.11–14,2011.
[17] A.Mycek,“Monitoring,Management,andAnal‐ysisofSecurityAspectsofIaaSEnvironments”, JournalofTelecommunicationsandInformation Technology,vol.4,no.4,pp.108–116,2023.
[18] A.Mycek,D.Grzonka,andJ.Tchórzewski,“Agent‐basedSimulationandAnalysisofInfrastructure‐as‐CodeProcesstoBuildandManageCloud Environment”, ECMS2023:Proceedingsofthe 37thECMSInternationalConferenceonModelling andSimulation,pp.513–520,2023.
[19] B.OmaymaandY.Laaziz,“SoftwareDe ined Networks(SDN):thenewEraofNetworking”, AdvancedInformationTechnology,Servicesand Systems(AIT2S-15),2015.
[20] OpenNebula.“OpenNebulaDocs:6.8”.
[21] OpenStack.“OpenStackDocs:2023.2”.
[22] A.Paradowski,L.Liu,andB.Yuan,“Benchmark‐ingthePerformanceofOpenStackandCloud‐Stack”, 2014IEEE17thInternationalSymposium onObject/Component-OrientedReal-TimeDistributedComputing,pp.405–412,2014.
[23] P.Patel,V.Tiwari,andM.Abhishek,“SDNand NFVintegrationinopenstackcloudtoimprove networkservicesandsecurity”, 2016InternationalConferenceonAdvancedCommunication ControlandComputingTechnologies(ICACCCT), pp.655–660,2016.
[24] S.Rowshanrad,V.Abdi,andM.Keshtgari,“Per‐formanceevaluationofsdncontrollers:Flood‐lightandOpenDaylight”, IIUMEngineeringJournal,vol.17,no.11,pp.47–57,2016.
[25] G.Rycaj,“Comparisonofvirtualizationper‐formanceofProxmox,OpenVZ,OpenNebula, VmwareESXandXenServer”, JournalofComputerSciencesInstitute,vol.12,pp.214–219, 2019.
[26] S.Scott‐Hayward,S.Natarajan,andS.Sezer, “ASurveyofSecurityinSoftwareDe inedNet‐works”, IEEECommunicationsSurveys&Tutorials,vol.18,no.1,pp.623–654,2016.
[27] P.Segečetal.,“SD‐WAN‐architecture,functions andbene its”, 202018thInternationalConferenceonEmergingeLearningTechnologiesand Applications(ICETA),pp.593–599,2020.
[28] S.Shinetal.,“AVANT‐GUARD:ScalableandVig‐ilantSwitchFlowManagementinSoftware‐De inedNetworks”, Proceedingsofthe2013ACM SIGSACConferenceonComputer&CommunicationsSecurity,pp.413–424,2013.
[29] G.Toraldo, OpenNebula3CloudComputing,Com‐munityexperiencedistilled,PacktPublishing, 2012.
[30] A.Vogeletal.,“PrivateIaaSClouds:ACompar‐ativeAnalysisofOpenNebula,CloudStackand OpenStack”, 201624thEuromicroInternational ConferenceonParallel,Distributed,andNetworkBasedProcessing(PDP),pp.672–679,2016.
[31] Z.Zhangetal.,“SoftwareDe inedNetwork‐ing(SDN)ResearchReview”, Proceedingsofthe 2018InternationalConferenceonMechanical, Electronic,ControlandAutomationEngineering (MECAE2018),pp.291–300,2018.
[32] Z.Zhao,F.Hong,andR.Li,“SDNBasedVxLAN OptimizationinCloudComputingNetworks”, IEEEAccess,vol.5,pp.23312–23319,2017.
Submitted:11th October2023;accepted:3rd April2024
AhmedJ.Abougarair,AbdulhamidA.Oun,WiddB.Guma,ShadaE.Elwefati DOI:10.14313/JAMRIS/4‐2024/32
Abstract:
Pressurizedwaterreactors(PWRs)arethemostcommon andwidelyusedtypeofreactor,andensuringthestability ofthereactorisofutmostimportance.Thechallengeslie ineffectivelymanagingpowerfluctuationsandsudden changesinreactivitythatcouldresultinunsafesitua‐tions.Reactorpowerfluctuationscancausechangesin behavior.Atthesametime,thetransferofheatfromthe fueltothecoolantandreactivitychangesresultingfrom differencesinfuelandcoolanttemperaturescanalso makethesystemunpredictable.Theprimarygoalofa powercontrollerusedinanuclearreactoristosustainthe specifiedpowerlevel,whichguaranteesthesecurityof thepowerplant.Toaddressthesechallenges,thispaper presentsadynamicmodelofaPWRandappliesseveral controltechniquestothesystemforpowerlevelcontrol. Specifically,atraditionalPIDcontroller,aneuralnetwork controller,afuzzyself‐tunedPIDcontroller,andaneuro‐fuzzyself‐tunedPIDcontrollerwereindividuallydesigned andevaluatedtoenhancetheperformanceofthereac‐torpowercontrolsystemunderconstantandvariable referencepower.Inaddition,therobustnessofeach controllerwasassessedagainsttimedelaysandexternal disturbances.Thesystemwastestedwithvariousinitial powervaluestoevaluateitsperformanceunderdifferent conditions.Theresultsdemonstratethattheneuro‐fuzzy self‐tunedPIDcontrollerhasthebestperformance,as wellasthefastestresponsetimecomparedtotheother controllers.
Furthermore,theintelligentcontrollerswerefoundto exhibitgoodrobustnessagainsttimedelaysandexternal disturbances.Thesystem’sstabilitywasnotsignificantly affectedbychangesintheinitialpowervalue,althoughit hadaminoreffectonthetransientresponse.Overall,the findingsofthisstudycaninformthedesignandoptimiza‐tionofcontrolsystemsforPWRs,withtheultimategoal ofimprovingtheirsafety,reliability,andperformance.
Keywords: fuzzyself‐tuned,pressurizedwaterreactor, neuralnetwork,neuro‐fuzzyself‐tuned
Nuclearenergy,despitenotbeingarenewable energysource,isrecyclableandisthesecond‐largest sourceoflow‐carbonenergyintheworld[1].Itplays acrucialroleinmeetingthedemandforelectricity andaddressingclimatechangebyreliablysupplying poweraroundtheclock.Infact,upto95%ofspent
nuclearfuelcanberecycled[2].Therehasbeenan increasinginterestintheuseofnuclearenergytopro‐duceelectricity,withcurrentnuclearpowerreactors contributingtoabout10.3%oftheworld’selectricity generation[3].
Nuclearenergyisproducedthroughnuclear is‐sion,wheretheatom’snucleusisdividedintotwo ormoresmallernuclei,releasingenergyinthepro‐cess.Forexample,thenucleusofanatomofuranium dividesintotwosmallernucleiwhenitisstruckby aneutron,releasingheatandradiation[4].Nuclear powerplantsusenuclearreactorsandassociated equipmenttocontrolandmanagechainreactions thatproduceheatthrough ission,primarilydriven byuranium.Theheatisusedtowarmupacool‐ingagent,usuallywater,whichproducessteamthat spinsturbinestogeneratelow‐carbonpower[5].Var‐ioustypesofreactors,includingHTGR,FNR,PWR, BWR,PHWR,GCR,andLWGR,areusedgloballyto produceelectricity[6].PWRsarethemostpopu‐lartypeofnuclearreactorusedforenergygenera‐tionduetotheirself‐regulatingandself‐stabilizing characteristics,whichenablethemtoexecuteload‐followingoperations.However,fastpowermaneuver‐ingisachallengingtaskduetotheinherentnon‐linearityofreactors,wherethebehaviorvarieswith changesinreactorpower.Additionally,thesystem isunpredictableduetoheattransfersfromthefuel tothecoolantandreactivitychangesresultingfrom temperaturevariationsinthefuelandcoolant.Con‐sequently,designingareliablecontrollerforpower‐followingoperationinPWRshaslongbeenatopicof intenseresearchinterest[6,7].Resultshaveshown thatafuzzyPIDcontrollerissuperiortoatraditional PIDcontrollerintermsofcontrollingandmaintaining systemdesignpressure[8,9].Sabrietal.[10]also appliedastatespaceMPCforloadtrackinginPWRs basedonalinearizedsystemmodel.Linetal.[11] developedarobustpowercontrolstrategyforPWR usingthe��∞ onalinearizedsystem.Abdelfattah[12] employedanadaptiveneuralnetworkalgorithmto computetherecommendedpowercorrectionvalue thatminimizesthesteady‐stateerror.Mousakazemi [13]employedageneticalgorithm(GA)totuneand schedulePIDcontrollergainsforPWRpowercontrol whileemphasizingtherobustnessofthecontroller. Vajpayeeetal.[14],designedafuzzylogiccontroller (FLC)forcontrollingthePWRpower.
Althoughmanyofthesestudiesusedthecon‐trollersonthelinearizedsystem,theaccuracyofthe linearapproximationofanonlinearsystemislim‐itedtoasmallregionaroundtheoperatingpoint. Thus,itiscrucialtodesignarobustcontrollerthat canbeappliedtothenonlinearsystemandensure powerleveltrackingatalloperatingpoints.Intelligent controllers,whichdonotrequireknowledgeofthe system’sphysics,appeartobeasuitablesolutionfor manyresearchers.WhileANFISself‐tunedPIDcon‐trollershavenotbeenusedinnuclearcontrolsys‐tems,theyhavedemonstratedsuperiorityinmany applications[15,16].Thispaperwilldesigndifferent intelligentcontrolmethods,whichareneuralnetwork control,fuzzyself‐tunedPIDcontroller,andneuro‐fuzzyself‐tunedPID,toregulatereactorcorepower. Theperformancesofthesecontrollerswillbecom‐paredwitheachotherandwiththeconventionalPID controllerundervariouscircumstances.Thispaper consistsof ivesections:abriefdescriptionofthePWR systemaccompaniedbyitsmathematicalmodelin Section 2,thedesignofthecontrollersinSection 3, simulationresultsinSection 4,andaconclusionin Section5
Apressurizedwaterreactor(PWR)isacommonly usednuclearpowerreactordesignwherehigh‐purity waterisheatedby issionreactionstoaveryhigh temperature,maintainedunderhighpressuretopre‐ventboiling,andthenconvertedtosteaminasteam generator.Thissteamisutilizedtodriveturbines, which,inturn,activategeneratorstoproduceelectri‐calpower[16].APWRplantconsistsoftwomainsys‐tems,namely,theprimarysystemandthesecondary system,eachservingspeci icfunctionswithdedicated componentsasdescribedbelow:Theprimarysys‐tem,alsoknownastheNuclearSteamSupplySystem, includesareactorcore,pressurizer,andthreeorfour loops,eachequippedwithasteamgeneratoranda coolantpump.Thesecondarysystem,oftenreferred toas“theBalanceofPlant,”consistsofaturbine,a maincondenser,feedwaterpumps,andfeedwater heaters[17].Thefollowingstepsprovideasummary
Figure2. Reactormodeldiagram[17]
ofthePWR’scycleandwater low,asdepictedin Figure1
Thenuclearfuelelement,moderator,coolant,and controlrodsareallcontainedinacylindricaltank thatservesasthereactorvesselinthesimulation.In PWR,uraniumisusedasafuelelementtogenerate heatfromatom issionsandislocatedinthecenter ofthevessel.Further,lightwaterisusedasamod‐eratoraswellasthereactorcoolanttotransferthe heat,andinorderforthe issionchainreactiontobe moreef icient,itisnecessarytoslowdowntherapid neutrons(generatedbybreakingatoms).Thereactor modelnodalizationisshowninFigure 2,including threenodesforthefuelelementandsixnodesfor thecoolant,aswellasadditionalnodesfortheupper plenum,lowerplenum,hotleg,andcoldleginthe reactorcoolantsystem,accordingtoreference[17]. Coolantentersthereactoratroomtemperatureand lowsupthroughthecore,whereitstemperature increasesasitpassesthroughthefuelrods.
Then,thehotterreactorcoolantreachestheupper reactorregion,whereitisdirectedouttheoutlet nozzleintothehotlegsoftheprimaryloopand continuestothesteamgenerators.Alargecoolant pumptransfersthecoolantfromthesteamgener‐atortotheprimaryloop.Thisreactorcanbecon‐trolled,improvingtheef iciencyandsecurityofthe nuclearpowersystem.The issionprocessmaybe controlledinrealtimebythecontrolrods,which makesthemessentialformanagingreactorpower. Acontrolrodstopsmore issionsfromoccurringby absorbingneutrons[18].Thereactorcoremathemat‐icalmodel,whichdescribesboththekineticsand thermalhydraulicsofthereactorcoreundersteady stateandtransientconditions,iscomposedof15ordi‐narydifferentialequationsderivedfromthephysi‐calmodel[13].Thefollowingshowshowthereactor modelequationsareexpressed[17],andTable1hasa de initionforeachoftheparameters.
‐ Pointreactorkineticsequations:
‐ Coldlegtemperatureequation:
Thelowerplenumtemperatureequation:
‐ Theupperplenumtemperatureequation:
‐ Hotlegtemperatureequation:
‐ Reactorcoreheattransferequations: Firstnode:
‐ Constitutiveequations[17]:
Secondnode:
Externalreactivityisthereactivityduetocontrol rods[4]:
Inthisstudy,theexternalreactivityandoutlettem‐peratureareconsideredforcingfunctionsontheiso‐latedmodelofthesteamgenerator.Thereactorpower systemhastwooutputs,namely,thehotlegtemper‐atureandthereactorpower,bothofwhichcanbe maintainedbycontrollingeitherofthem[11].Forthe purposesofthispaper,theseoutputsareassumedto beconstant,whilethevariablethatwillbecontrolled usingthecontrolrodsisthereactorpower.Thedesign parametersutilizedinthisstudyareobtainedfrom varioussources,includingreferences[18,19].
Table1. Parametersofareactormodel[17]
P Powerdistributionacrossnodesinthe reactorcore
��
Reactornominaloutputpower
C Precursorconcentration
��
Totaldelayedneutrongroupfraction
�� Averageofsixgroupdecayconstants
Λ Timeofneutrongeneration
Totalreactivity
Externalreactivity
Reactivitycoef icientofthefuel
Reactivitycoef icientofthecoolant
Mass lowofthecoolant
Coolantmassintwo luidnodes ��
Proportionofpowergeneratedinthefuel
Fuelmassineachnode
Heatcapacityofthefuel
ℎ Averageoverallheattransfercoef icient
Coldlegwatermass
Lowerplenumwatermass
Upperplenumwatermass
Hotlegwatermass �� Effectiveheattransfersurfaceareabetween fuelandcoolant
Fueltemperaturesinnodes(1–3)
Moderatortemperaturesinnodes(1‐6)
Fluidtemperatureinlowerandupper plenums,respectively
Heatcapacityofthecoolant
3.ControlSystemAlgorithms
Controlsystemalgorithmsforpressurizedwater reactors(PWRs)arecrucialformaintainingsafety, ef iciency,andstabilityinnuclearpowergeneration.
3.1.PIDController
Duetotheirabilitytodeliveradequateperfor‐mancewithastraightforwardalgorithmforvarious processes,PIDcontrollersareextensivelyemployed intheprocessindustries[20, 21].Itispopularly knownasa“three‐term”controller,theproportional (����),integral(����),andderivative(����),sinceitcon‐trolsaprocessthroughthesethreeparameters.These parameterscanbetunedtoadjusttheirimpactonthe process.Theformulaofthecontrolsignal u(t)forthe PIDcontrollerisdescribedas[22,23]:
(18)
Therearemanymethodsandapproachesfortuning aPIDcontroller,whichcanprovideimprovedperfor‐manceandreducetheerrorsignal e(t)[24,25].The parametersofthePIDcontrollerweretunedusing theZiegler‐Nicholastuningmethodtoobtaintheini‐tial/estimatedsetofworkingPIDparametersforthe system[26,27].
InthecontextofaPWRmodel,thePIDcontroller isemployedtocontrolimportantvariableslikethe coolant lowrate,reactorpower,andreactorcoolant temperature.TheactualapplicationofPIDcontrolto aPWRmodelwillbedeterminedbyfactorssuchasthe characteristicsofthesystem,controlgoals,andsafety considerations[28].
Controlrodreactivity
Controlrodspeed
Outlettemperatureoftheprimarywater leavingsteamgeneratorU‐tubes
Coldandhotlegtemperatures
Timeconstantsofmoderatornodes,cold leg,upperplenum,lowerplenum,andhot leg,respectively
3.2.NeuralNetworkController
Aneuralnetworkcontroller(NNC)wasusedto implementthecontrollerdirectly.TheNNCmustbe trained irstaccordingtosomecriteria,usingeither numericalI/Odataoramathematicalmodel[29]. Throughneuralnetworktrainingandlearning,the weightsareadjustedtoreduceerrors.Theprocedure continuesuntiltheresultissatisfactoryorthedesig‐natedamountoflearningtimehasbeencompleted. Theparameters(weightandbias)ofaneuralnet‐worksystemmaybedeterminedusingavarietyof techniques[30,31].Thesystemidenti icationmethod utilizesobservedinput–outputdatatoapproximate theoriginalcontroller.Priortodesigningtheneuro controller,systemidenti icationisacrucialaspectto consider.Theproposedapproachutilizesabackprop‐agationneuralnetworktopredictthesystem’soutput fornewinputsbasedonpastinputsandoutputs[32].
Theadvantagesofneuralnetworks,suchasadap‐tivelearning,self‐organization,andfaulttolerance, makethemsuitableforsystemidenti icationapplica‐tions.Figure3illustratesarecurrentneuralnetwork with n inputs, t outputs,and m hiddenlayernodes.The network’sinput,internalstate,andoutputvectorsare representedby u, z,and y,respectively[33,34].
��(��)= [��1 (��) …���� (��)]�� ��(��)= [��1 (��) …���� (��)]��
̂�� (��)= [̂�� 1 (��) …̂���� (��)]�� (19)
Theequationsofthenetworkare
{��(��)=��(����(��)+����(��−1)) ̂��(��)=����(��) } (20)
��isthetangenthyperbolicfunctionandistheactiva‐tionfunctionoftheinteriorlayerneurons.
��(��)=������ℎ������ℎ (��) = ���� −��−�� ���� +��−�� (21)
where����×��,����×��,and����×��areweightmatri‐ces.Theweightmatricesofthenetworkaredeter‐minedthroughtraining.Themeansquareerror(MSE) isutilizedasthecostfunction,whichiscalculatedfor eachtrainingsample.Here,��representsthenumber oftrainingsamples, y denotestheactualoutput,and representsthepredictedoutputoftheneuralnetwork.
‐ ControlActionGeneration:Oncetheneuralnetwork modelistrained,itcangeneratecontrolactions basedonthecurrentstateandinputdataofthePWR system.Theneuralnetworkprocessestheinput variablesusingitstrainednetworkarchitectureand producesanoutputcontrolsignal.Thiscontrolsig‐nalisthenappliedtothereactorcontrolsystemto regulatethebehaviorofthesystem.
TheNNCholdsadvantagesindealingwiththe complexandnonlineardynamicsofthePWRsystem. Byleveragingneuralnetworks,itcanmakeprecise controldecisionsandadapttochangingconditionsin real‐time.
Insummary,anNNCforaPWRsystemutilizes arti icialneuralnetworkstomodelandregulatethe behaviorofthereactor.Bylearningfromtrainingdata, itimprovesitscontrolperformance,makingitavalu‐abletoolformanagingtheoperationofaPWR.
��.(̂��(��)−��(��)) (22)
AnNNCdesignedforaPWRisacontrollerthatutilizes arti icialneuralnetworkstoregulatetheoperationof thePWRsystem.
Thiscontrollerharnessesthecapabilitiesofneu‐ralnetworkstomodeltheintricaterelationshipsand dynamicsofthereactorandmakecontroldecisions basedoninputdata.
ThetypicalcomponentsofanNNCareasfol‐lows[35]:
‐ NeuralNetworkModel:Theneuralnetworkmodel isspeci icallydesignedtocapturethebehaviorand characteristicsofthePWRsystem.Ittakesvarious inputvariables,suchascoolant lowrate,reactor power,temperature,andpressure,andemployshid‐denlayersandactivationfunctionstoprocessthis informationandgenerateanoutputcontrolsignal.
‐ TrainingData:TotraintheNNC,adatasetisneces‐sary.Thisdatasetcontainshistoricaldataorsim‐ulatedoutputsofthePWRsystem,alongwiththe correspondingdesiredcontrolactions.Bylearning fromthesedata,theneuralnetworkadjustsitsinter‐nalparametersandenhancesitspredictivecapabil‐ities.
Fuzzylogiccontrolhasbeeneffectivelyutilized innumerouscontrolissuesinrecentyearsbecause itdoesnotrequireprecisemathematicalmodelsof theuncertainnonlinearsystemsbeingcontrolled.A languagecontrolapproachwasdevelopedbyhumans andtransformedintoanautomatedcontrolmethod viaFLC[36].Intheself‐tuningfuzzyPIDcontroller, thePIDcontrollerparameters(����, ����,and ����)are adjustedusingfuzzylogicrules,which,inturn,makes thecontrolsystemcapabletomodifyitsownoper‐ationforchangingprocessconditionstoachievethe bestpossiblemodeofoperationandexcellentperfor‐mance.Anadaptivefuzzyself‐tunedPIDcontrolleris designedspeci icallyforregulatingtheoperationsof PWRs.Itcombinesprinciplesfromfuzzylogic,adap‐tivecontrol,andPIDcontroltooptimizecontrolper‐formanceinrealtime.
Thiscontrollercomprisesthreeprimarycompo‐nents:
PIDController:ThePIDcontrollerisawell‐known controlalgorithmthatemploysproportional,integral, andderivativetermstocalculatecontrolactions.It ensuresstability,accuracyinsteadystate,andrespon‐sivenesswithinthecontrolloop.
FLC:TheFLCutilizeslinguisticrulesandmem‐bershipfunctionstohandlecomplexandnonlinear behaviorsobservedinthePWRsystem.Byemploying fuzzyinferencerules,itdeterminestheappropriate controlactionsbasedonthesystem’scurrentstate anderrorsignals.
AdaptiveMechanism:Theadaptivemechanism continuouslymonitorsthesystem’sresponseand adjuststheparametersofboththePIDandFLCsto optimizecontrolperformance.Itdynamicallytunes thegainsofthePIDcontrollerormodi iesthefuzzy logicrulesandmembershipfunctionsbasedonreal‐timesystembehavior.
Theintegrationofthesethreecomponentsallows thecontrollertoadapttochangesinreactorcondi‐tions,disturbances,andotherfactorsastheyoccur. Throughitsadaptivefuzzyself‐tuningapproach,the controllerensurescontrolperformanceandstability withinthePWRsystem.
Insummary,theadaptivefuzzyself‐tunedPIDcon‐trollerforPWRaimstodeliverrobustandef icient controlbyleveragingtheadvantagesofPIDcontrol, fuzzylogic,andadaptivemechanisms.
Atwo‐input–three‐outputFLCisshowninFig‐ure4.Theinputsare��(��)(errorbetweenthereference inputandthecontrolledvariable)andΔ��(��)(change oferrorwithrespecttotime).Thegains����1,����1,and ����1 aretheFLCoutputs[37].
Thesegainsareusedtoadjusttheparametersof thePIDcontrolleronline.ThePIDcontrollercontrol signalcanbeexpressedas:
(23) where����1,����1,and����1 aretheFLCoutputsthatare usedtotunethePIDcontrolleronline.Thedescription ofeachinputcomprisesarangeofexpressionssuchas NB(representingnegativebig),NS(negativesmall), Z(zero),PS(positivesmall),andPB(positivebig). Additionally,eachoutputisdenotedbyarangeof expressions:Z(zero),MS(mediumsmall),S(small), M(medium),B(big),MB(mediumbig),andVB(very big).Asetof25rulesforthefuzzyrulebasecanbe derivedfromTable2[38].
ThePIDcontrollernowhasthecapacitytomod‐ifyitssettingsonlinethankstoacombinationofthe bene itsoftheFLCandPIDcontroller.Choosingthe parametersofthefuzzysystem,suchasinputand outputmembershipfunctions,isadif iculttaskand oneofthedrawbacksofdesigningafuzzycontroller thatreliesonexpertknowledge.Toovercomethis challenge,someresearchershaveproposedaneu‐ralfuzzyself‐tunedPIDcontroller,whichallowsfor themodi icationofmembershipfunctionparameters usingfuzzylogic[38].Theself‐tuningneuro‐fuzzy PIDcontrollercombinesafuzzylogicmethodwitha ive‐layerANNstructure.The irstlayerinthis ive‐layerstructureisforinputs,thesecondlayerisfor fuzzi ication,thethirdandfourthlayersareforeval‐uatingfuzzyrules,andthe ifthlayerisfordefuzzi‐ication[39].Anadaptiveneuro‐fuzzyself‐tunedPID controllerdesignedforaPWRisacontrollerthat utilizesadaptivecontrol,fuzzylogic,neuralnetworks, andPIDcontrolprinciplestoregulatetheoperationof aPWRsystem.Thiscontrollercontinuouslyadjustsits parametersinresponsetoreal‐timeinformationand systemfeedback,aimingtooptimizecontrolperfor‐mance[40,41].
Thecontrollercomprisesfourkeycomponents:
‐ PIDController:ThePIDcontrollerisawell‐establishedcontrolalgorithmthatcalculates controlactionsusingproportional,integral,and derivativeterms.Itensuresstability,steady‐state accuracy,andresponsivenesswithinthecontrol loop.
‐ FLC:TheFLCemployslinguisticrulesandmem‐bershipfunctionstohandlecomplexandnonlinear systembehavior.Byutilizingfuzzyinferencerules, itdeterminestheappropriatecontrolactionbased onthesystem’scurrentstateanderrorsignals.
‐ NeuralNetwork:Theneuralnetworkcomponent employsarti icialneuralnetworkstomodeland capturetheintricaterelationshipsandnonlinear dynamicsofthePWRsystem.Bylearningfrom historicaldataorsimulationoutputs,theneu‐ralnetworkenhancesitspredictivecapabilityand improvescontrolperformance[42].
‐ AdaptiveMechanism:Theadaptivemechanismcon‐tinuallymonitorsthesystem’sresponseandadjusts theparametersofthePIDcontroller,FLC,andneu‐ralnetworkcomponenttooptimizecontrolperfor‐mance.ItcanadaptivelytunethegainsofthePID controller,modifythefuzzylogicrulesandmember‐shipfunctions,andupdatetheinternalparameters oftheneuralnetworkbasedonreal‐timesystem behavior[43–45].
Thecombinationofthesefourcomponents empowersthecontrollertoadapttochangesin reactorconditions,disturbances,andotherfactors inrealtime.Theadaptiveneuro‐fuzzyself‐tuning approachenablesthecontrollertomaintaincontrol performanceandstabilityinthePWRsystem.
Insummary,theadaptiveneuro‐fuzzyself‐tuned PIDcontrollerforaPWRsystemaimstodeliverrobust andef icientcontrolbyintegratingtheadvantagesof PIDcontrol,fuzzylogic,neuralnetworks,andadap‐tivemechanisms.Theblockdiagramoftheproposed neuro‐fuzzycontrollerhastwoinputsandthreeout‐puts,aspresentedinFigure5.Thetwoinputsforthe systemare e(t),whichisthedifferencebetweenthe referenceinputandthecontrolledvariable,andΔ��(��), whichisthechangeinerrorovertime,andtheoutputs are ����1, ����1,and ����1,whichareusedtoadaptthe parametersofthePIDcontroller.
Theneuro‐fuzzycontrollerhasbeencreatedto produceanappropriatecontrolsignaltomodifythe PIDcontroller’ssettings.Itwastrainedwithinput andoutputdataobtainedafterthestudyofsystem responseinthenominalcase,dividingitintoregions, andtestingthesystemresponseatseveralproposed valuesforeachregion.Thestructureoftheneuro‐fuzzymodelforeachoutputisdescribedinFigure6 Thealgorithmusedfortrainingisthehybridlearning algorithm(HLA)withsevenandsixtriangularmem‐bershipfunctionsforerrorandchangeoferrorinputs, respectively,andthefuzzyrulebasecontains42rules.
Figure4. Fuzzyself‐tuningPIDcontrollerstructure
Figure5. Blockdiagramofanadaptiveneurofuzzyself‐tunedPIDcontrollerwiththesystem
Table2. Rulebaseofthefuzzyself‐tunedPIDcontroller
4.ResultsandDiscussion
ThissectionpresentsthesimulinkmodelofPWR withdifferentcontrollersasshowninFigure 7 and simulationresultsofthePWRpowercontrolsystem usingMATLABSimulink.Thesectionisdividedinto iveparts.Firstly,thesimulationresultsundercon‐stantandvariablereferencepowerarepresentedin Section4.1
Theoutputofthefuzzycontrollercanberepre‐sentedasfollows:
Rulei:If(eisinputmfx)and(ceisinputmfy),then ��
=����(��)+����(����)+����
��,����,and���� arethedesignparametersdetermined duringtheperiodoftrainingphase. (1≤��≤42),(1≤��≤7),and(1≤��≤6) ���� represents����1,����1,or����1.
Secondly,theresponseofthecontrollersagainst timedelayisdiscussedinSection4.2Sections4.3and 4.4assesshowresilientthecontrollersaretoexternal disturbancesintroducedatboththeinputandoutput respectivelyforthereactorsystem.Lastly,theeffect ofthechangeintheinitialvalueoftherelativeoutput powerisanalyzedinSection4.5.
4.1.HardAlterationofReferencePower
Thesimulationsarecarriedoutwithaninitial relativepowerofzero.The irstsetofsimulationsis conductedwithaconstantreferencerelativepower (��/����)of1.Theresponseofthereactorwiththe neuro‐fuzzyself‐tunedPIDcontrolleriscomparedto theresponsesofthePID,neuralnetwork,andfuzzy self‐tunedPIDcontrollers,asshowninFigure8.
Structureofanadaptiveneurofuzzysystem
Next,avariablereferencerelativepower(��/����) isappliedtothesystem,startingat1anddropping to0.3atthe60thsecond.Itremainsat0.3untilthe 140thsecondwhenitrisesto0.9.Theresponseofthe reactorwiththeneuro‐fuzzyself‐tunedPIDcontroller iscomparedtotheresponsesoftheothercontrollers undervariablereferencepowerinFigure9.
Thereactoroutputrelativepowerisexamined inthreeintervals(0–15s,60–85s,and140–155s) tocomparethecontrollers.Thesettlingtimeand overshootforthefourcontrollersineachregionare summarizedinTable 3.Itisevidentfromtheprevi‐oussimulationresultsthatthethreeintelligentcon‐trollers,neuralnetwork,fuzzyself‐tunedPID,and neuro‐fuzzyself‐tunedPID,arecapableofensuring goodreferencetrackingperformancesintermsof minimalovershootsandsettlingtime.Theresults demonstratethattheneuro‐fuzzyself‐tunedPIDcon‐trollerhasthesmallesterror‐integralperformance indexesISEandIAE,ascomparedtotheothercon‐trollersaspresentedinFigure10
Toevaluatethesystem’srobustnessagainsttime delay,a10‐msdelaywasintroducedandtheresponse ofeachcontrollerwasanalyzedundervariableref‐erencerelativepower.AsdepictedinFigure 11,all designedcontrollerswereabletomaintaintherefer‐ence,indicatingtheirrobustnessagainsttimedelay. Despiteaslightovershootintheresponseofthe fuzzyself‐tunedPIDandneuro‐fuzzyself‐tunedPID controllers,themoderator’stemperatureremained withinthepermissiblerange[46].
Toobservetheresponseofthedesignedcon‐trollerstoexternaldisturbances,theinputtempera‐turefromthesteamgeneratorsystem,represented by ������,wasconsideredasanexternaldisturbanceto thereactorcoresystem.Theinputtemperaturewas increasedby6∘Fatthe35thsecondofthesimula‐tion,withatotalsimulationtimeof200s,andasthe inputtemperature������ increased,thereactor’spower decreasedrapidlyduetothenegativetemperature feedbackrelationship.However,thecontrollerswere abletoregulatethereactor’spowerandmaintainthe referencepowerbyincreasingthecontrolaction.As showninFigure12,allthecontrollersexhibitedgood disturbancerejectioncapabilities[47].
Thecontrollers’robustnessisevaluatedbyintro‐ducinganexternaldisturbanceintheoutputofthe reactorsystem,asshowninFigure 13.Thedistur‐bancesignal,witharelativepoweramplitudeof0.01 (approximately11,453kW),wasinjectedevery20s withapulsewidthof25%ofitsperiod.Asdemon‐stratedfromtheresult,whentherelativeoutput powerincreasedduetothedisturbancesignal,the controlrodswereinsertedintothereactorcoreto reducetherelativepower.Theintelligentcontrollers demonstratetheirrobustnessastheywereableto returntotrackingthereferenceafterapproximately 1s[48].
Figure7. SimulinkmodelofPWRwithdiffrentcontrollers
Figure8. Systemresponseunderconstantreferencepower
Figure9. Systemresponseundervariablereferencepower
Figure10. IAEandISEversustime
Table3. Settlingtimeandovershootforthefourcontrollersineachregion
4.5.DifferentInitialConditions
Toevaluatethestabilityofthenonlinearsystem, thereactorcore‐controlledsystemwastestedwith multipleinitialrelativepowervalues.Astheinitial conditionhasasigni icantimpactonsystemstability, theinitialrelativepowervaluesof0.5wereexam‐ined.Theresponseofthesystemforeachcontroller isshowninFigure14.
Eachcontrolmethodcanhelpreducetheimpact oftimedelayanddisturbancesinaPWRsystem. Theeffectivenessofeachcontrollerdependsonfac‐torssuchasthedurationofthetimedelayordis‐turbances,theaccuracyofthesystemmodel,andthe optimizationofcontrolparameters.Whenselecting
andimplementingthemostappropriatecontrolstrat‐egy,itiscrucialtoconsidertheuniquecharacteristics andrequirementsofthePWRsystem.ThePIDcon‐trollercontinuouslyadjustscontrolactionsbymoni‐toringtheerrorbetweenthedesiredsetpointandthe measuredprocessvariable.Thisminimizesthein lu‐enceofuncertainty.Theproportionaltermresponds promptlytosuddenchangesandprovidesimmediate correctiveaction.Theintegraltermaddressessteady‐stateerrorscausedbydisturbances,graduallyadjust‐ingthecontrolactiontocounteracttheireffects.
Figure11. Systemresponseduetotimedelay
Figure12. Systemresponseduetoexternalinputdisturbance
Figure13. Systemresponseduetoexternaloutputdisturbance
Systemresponsewithdifferentinitialoutputrelativepowervalues
Thederivativetermenablesarapidresponse totherateofchangeofthedisturbances,assisting instabilizingthesystem’sbehavior.TheNNCeffec‐tivelyhandlesbothexternalinputandoutputdis‐turbancesinaPWRsystem.Bylearningtherela‐tionshipsbetweenthedisturbancesandthereactor’s behavior,theneuralnetworkcanpredictandmodel theireffects.Itadjuststhecontrolactionsbasedon thislearnedinformationtominimizetheimpactof thedisturbancesonthereactor’soutput.Theadap‐tivefuzzyself‐tunedPIDcontrollercombinesfuzzy logicandPIDcontrolwithadaptivecapabilities.It continuouslymonitorsthesystem’sresponseand theeffectsofbothexternalinputandoutputdistur‐bances,adaptivelyadjustingthePIDcontrolparame‐ters.Thisadaptivemechanismenablesthecontroller torespondtoandmitigatetheimpactofdisturbances onbothsysteminputsandoutputsinrealtime.The adaptiveneuro‐fuzzyself‐tunedPIDcontrollerfurther improvescontrolperformancebyintegratingneural networksandfuzzylogic.Theneuralnetworkcom‐ponentmodelsthesystemanddisturbances,while thefuzzylogiccomponenthandlesuncertaintyand linguisticrules.Thecontroller’sadaptivemechanism dynamicallyadjuststhePIDcontrolparameters,fuzzy logicrules,andneuralnetwork’sinternalparameters tooptimizecontrolperformanceandeffectivelycoun‐teracttheeffectsofbothexternalinputandoutput disturbances.
Nuclearreactorsarecriticalcomponentsof nuclearenergysystems,withthecontrolofreactor powerbeingofparamountimportance.Themain objectiveofthepowercontrollerinnuclearreactors istomaintainthereferencepowerlevel,which ensuressafeandreliableoperationofthepower station.PWRsarethemostcommonandwidelyused typeofreactor,andfastpowermaneuveringisa challengingtaskduetotheinherentnonlinearityof thesystem.Reactorpower luctuationscancause
changesinbehavior,whilethetransferofheatfrom thefueltothecoolantandreactivitychangesresulting fromdifferencesinfuelandcoolanttemperaturescan alsomakethesystemunpredictable.Toaddressthese challenges,thispaperpresentsadynamicmodel ofaPWRandappliesseveralcontroltechniquesto thesystemforpowerlevelcontrol.Speci ically,a traditionalPIDcontroller,anNNC,afuzzyself‐tuned PIDcontroller,andaneuro‐fuzzyself‐tunedPID controllerwereindividuallydesignedandevaluated toenhancetheperformanceofthereactorpower controlsystemunderconstantandvariablereference power.
Inaddition,therobustnessofeachcontrollerwas assessedagainsttimedelaysandexternaldistur‐bances.Thesystemwasalsotestedwithdifferent initialpowervaluestoensurestability.Theresults demonstratethattheneuro‐fuzzyself‐tunedPIDcon‐trollerhasthefastestresponsetimecomparedtothe othercontrollers.Furthermore,theintelligentcon‐trollerswerefoundtoexhibitgoodrobustnessagainst timedelaysandexternaldisturbances.Thesystem’s stabilitywasnotsigni icantlyaffectedbychangesin theinitialpowervalue,althoughithadaminoreffect onthetransientresponse.Overall,the indingsof thisstudycaninformthedesignandoptimizationof controlsystemsforPWRs,withtheultimategoalof improvingtheirsafety,reliability,andperformance. Asapotentialfuturedirection,ratherthantrainingthe neuralnetworkandadaptiveneurofuzzycontrollers usinginput/outputdataselectedafteranalyzingthe system’sresponseinnominalconditionsandchoos‐ingsuitableoutputsignals,analternativeapproachto enhancingtheperformanceofthesecontrollersisto traintheirparametersusinganoptimalPIDcontroller. ThisoptimalPIDcontrollercanbedesignedusingan optimizationalgorithm,therebyofferingthepotential forimprovedcontrollerperformance.
AUTHORS
AhmedJ.Abougarair∗ –ElectricalandElectron‐icsEngineering,UniversityofTripoli,Libya,e‐mail: a.abougarair@uot.edu.ly.
AbdulhamidA.Oun –ElectricalandElectronics Engineering,UniversityofTripoli,Libya,e‐mail: a.oun@uot.edu.ly.
WiddB.Guma –ElectricalandElectronics Engineering,UniversityofTripoli,Libya,e‐mail: w.gu@uot.edu.ly.
ShadaE.Elwefati –BiomedicalEngineering,Univer‐sityofTripoli,Libya,e‐mail:s.elwefati@uot.edu.ly.
∗Correspondingauthor
References
[1] J.Hao,L.Chen,andN.Zhang,“AStatisticalReview ofConsiderationsontheImplementationPathof China’sDoubleCarbonGoal,” Sustainability,vol. 14,no.18,Sep.2022,pp.11274,doi:10.3390/ su141811274.
[2] W.ToandA.Chung,“Carbon‐NeutralityResearch inChina—TrendsandEmergingThemes,” World, vol.4,no.3,Sep.2023,pp.490–508;doi:10.339 0/world4030031.
[3] N.Alietal.,“Carbon‐BasedNano luidsandTheir AdvancestowardsHeatTransferApplications— AReview,” Nanomaterials,vol.11,no.6,Jun. 2021,pp.1628,doi:10.3390/nano11061628.
[4] B.Saleh,etal.,“UsingDirectSolarEnergyCon‐versioninDistillationviaEvacuatedSolarTube withandwithoutNanomaterials,” Processes,vol. 11,no.6,Jun.2023,pp.1734,doi:10.3390/pr11 061734.
[5] Y.Wang,etal.,“Carbonpeakandcarbonneu‐tralityinChina:Goals,implementationpath,and prospects,” ChinaGeology,vol.4,no.4,2021,pp. 720–746,doi:10.31035/cg2021083.
[6] W.Liu,etal.,“CarbonEmissionIn luencingFac‐torsandScenarioPredictionforConstruction IndustryinBeijing–Tianjin–Hebei,” Advancesin CivilEngineering,2023,ArticleID2286573,doi: 10.1155/2023/2286573.
[7] Y.Wu,etal.,“Nuclearsafetyintheunexpected secondnuclearera,” ProceedingsoftheNational AcademyofSciences,vol.116,no.36,Aug.2019, pp.17673–17682,doi:10.1073/pnas.1820007 116.
[8] G.KiruiandJ.Wang,“DesignofaFuzzyFOPID ControllerforPowerLevelControlofaPres‐surizedWaterReactor,”ICMLC’20:Proceed‐ingsofthe202012thInternationalConfer‐enceonMachineLearningandComputing,2020, pp.549–553.doi:10.1145/3383972.3384004.
[9] N.SutarnaandB.Purwanti,“MetodeTuning OperatingRangeFuzzyPIDControllerpadaSis‐temOrdeTiga,” JurnalTeknikElektro,vol.12,no.
1,Jun.2020,pp.33–39,doi:10.15294/jte.v12i1 .24050.
[10] M.Sabri,etal.,“ModelPredictiveandFuzzyLogic ControllersforReactorPowerControlatReactor TRIGAPUSPATI,” IOPConferenceSeries:Materials ScienceandEngineering,vol.1231,no.1,Feb. 2022,012001,doi:10.1088/1757899x/1231/ 1/012001.
[11] Y.Lin,etal.,“RobustPowerControlDesignfor aSmallPressurizedWaterReactorUsinganH In inityMixedSensitivityMethod,” NuclearEngineeringandTechnology,vol.52,no.7,Jul.2020, pp.1443–1451,doi:10.1016/j.net.2019.12.031.
[12] H.Abdelfattah,“AdaptiveNeuro‐FuzzySelf Tuned‐PIDControllerforStabilizationof CorePowerinaPressurizedWaterReactor,” InternationalJournalofRoboticsandControl Systems,vol.3,no.1,Nov.2022,pp.1–18,doi: 10.31763/ijrcs.v3i1.710.
[13] S.Mousakazemi,“ControlofaPWRNuclear ReactorCorePowerUsingScheduledPIDCon‐trollerwithGA,BasedonTwo‐PointKinetics ModelandAdaptiveDisturbanceRejectionSys‐tem,” AnnalsofNuclearEnergy,vol.129,2019, pp.487–502,doi:10.1016/j.anucene.2019.02. 019.
[14] V.Vajpayee,etal.,“LQGI/LTRBasedRobustCon‐trolTechniqueforaPressurizedWaterNuclear PowerPlant,” AnnalsofNuclearEnergy,vol.154, May2021,pp.108105,doi:10.1016/j.anucene. 2020.108105.
[15] A.MwauraandY.Liu,“AdaptiveNeuro‐Fuzzy InferenceSystem(ANFIS)BasedModelingof IncipientSteamGeneratorTubeRuptureDiag‐nosis,” AnnalsofNuclearEnergy,vol.157,2021, pp.108262,doi:10.1016/j.anucene.2021.108 262.
[16] A.Sabo,etal.,“ApplicationofaNeuro‐Fuzzy ControllerforSingleMachineIn initeBusPower SystemtoDampLow‐FrequencyOscillations,” TransactionsoftheInstituteofMeasurementand Control,vol.43,no.16,Sep.2021,pp.3633–3646,doi:10.1177/01423312211042781.
[17] V.Vajpayee,etal.,“DynamicModeling,Simula‐tion,andControlDesignofaPressurizedWater‐TypeNuclearPowerPlant,” NuclearEngineering andDesign,vol.370,Dec.2020,pp.110901,doi: 10.1016/j.nucengdes.2020.110901.
[18] C.Tucker, HowtoDriveaNuclearReactor,Cham: SpringerInternationalPublishing,2019,doi:10 .1007/978‐3‐030‐33876‐3.
[19] N.Osman,“LinearizedMathematicalModelfor PWRDynamicsSimulation,” ArabJournalof NuclearSciencesandApplications,vol.53,no.2, 2020,pp.78–86;doi:10.21608/ajnsa.2019.18 780.1290.
[20] X.Zhang,etal.,“DesignandVeri icationofReac‐torPowerControlBasedonSteppedDynamic
MatrixController,” ScienceandTechnologyof NuclearInstallations,2019,pp.1–11,doi:10.115 5/2019/4973120.
[21] S.Mousakazemi,N.Ayoobian,andG.Ansarifar, “ControlofthePressurizedWaterNuclear ReactorsPowerUsingOptimizedProportional‐Integral‐DerivativeControllerwithParticle SwarmOptimizationAlgorithm,” Nuclear EngineeringandTechnology,vol.50,no.6,2018, pp.877–885,doi:10.1016/j.net.2018.04.016.
[22] A.Amhathib,etal.,“SupervisoryControland SimulationofBoilerDrumWaterLevel,” 2023 IEEEInternationalConferenceonAdvancedSystemsandEmergentTechnologies(IC_ASET’2023), Tunisia,2023,doi:10.1109/IC_ASET58101.202 3.10150778.
[23] J.Wan,“DesignofaTwo‐Degree‐of‐Freedom ControllerforNuclearReactorPowerControlof PressurizedWaterReactor,” AnnalsofNuclear Energy,vol.144,Sep.2020,pp.107583,doi:10 .1016/j.anucene.2020.107583.
[24] A.Oun,etal.,“IntelligentControlDesignfor LinearModelofActiveSuspensionSystem,” 30thInternationalConferenceonMicroelectronics(IEEE),Tunisia,2018.doi:10.1109/ICM.20 18.8703995.
[25] A.Zrigan,etal.,“OptimizedPIDController andGeneralizedInvertedDecouplingDesignfor MIMOSystem,” 2023IEEEInternationalConferenceonAdvancedSystemsandEmergentTechnologies(IC_ASET’2023),Tunisia,2023,doi:10.1 109/IC_ASET58101.2023.10150957.
[26] I.Buzkhar,“ModelingandControlofaTwo‐WheeledRobotMachinewithaHandlingMech‐anism,” 2023IEEE3rdInternationalMaghreb MeetingoftheConferenceonSciencesandTechniquesofAutomaticControlandComputerEngineering),Libya,2023,doi:10.1109/MI‐STA575 75.2023.10169424.
[27] V.Vajpayee,etal.,“L1‐AdaptiveRobustControl DesignforaPressurizedWater‐TypeNuclear PowerPlant,” IEEETransactionsonNuclearScience,vol.68,no.7,July2021,pp.1381–1398.doi: 10.1109/TNS.2021.3090526.
[28] A.Abougarair,“NeuralNetworksIdenti ication andControlofMobileRobotUsingAdaptive NeuroFuzzyInferenceSystem,” Proceedingsof the6thInternationalConferenceonEngineering &MIS,2020,doi:10.1145/3410352.3410734.
[29] X.Shi,H.Zhao,andF.Zheng,“ParameterOpti‐mizationofNonlinearPIDControllerUsingRBF NeuralNetworkforContinuousStirredTank Reactor,” Measurement&Control,2023,doi:10 .1177/00202940231189307.
[30] A.Naimi,etal.,“DynamicNeuralNetwork‐Based SystemIdenti icationofaPressurizedWater Reactor,” 20208thInternationalConferenceon Control,MechatronicsandAutomation(ICCMA), 2020,doi:10.1109/iccma51325.2020.9301483.
[31] M.Edardar,etal.,“AdaptiveNeuralNetworks BasedRobustOutputFeedbackControllersfor NonlinearSystems,” InternationalJournalof RoboticsandControlSystems,vol.2,2022,p. 109513,doi:10.31763/ijrcs.v2i1.523.
[32] G.ZhouandD.Tan,“ReviewofNuclearPower PlantControlResearch:NeuralNetwork‐Based Methods,” AnnalsofNuclearEnergy,vol.181, 2023,pp.109513,doi:10.1016/j.anucene.20 22.109513.
[33] A.Abougarair,“LyapunovRedesignofPiezo ActuatorforPositioningControl,” 9thInternationalConferenceonSystemsandControl,France, 2021,doi:10.1109/ICSC50472.2021.9666594.
[34] M.Lot i,etal.,“ADesignofSwitching SupervisoryControlBasedonFuzzy‐PID ControllersforVVER‐1000PressurizerSystem withRELAP5andMATLABCoupling,” Annalsof NuclearEnergy,vol.147,Nov.2020,p.107625; doi:10.1016/j.anucene.2020.107625.
[35] M.Abdelghany,A.Okasha,andS.Ossama,“Opti‐mumPIDControllerwithFuzzySelf‐Tuningfor DCServoMotor,” JournalofRoboticsandControl (JRC),vol.4,no.4,2023,pp.500–508,doi:10.1 8196/jrc.v4i4.18676.
[36] S.Elwefati,etal.,ControlofEpidemicDis‐easeBasedOptimizationTechnique, 2021IEEE 1stInternationalMaghrebMeetingoftheCon‐ferenceonSciencesandTechniquesofAuto‐maticControlandComputerEngineeringMI‐STA,Libya,2021,doi:10.1109/MISTA52233.2 021.9464453.
[37] X.Luan,J.Wang,Z.Yang,andJ.Zhou,“Load‐FollowingControlofNuclearReactorsBasedon FuzzyInput‐OutputModel,” AnnalsofNuclear Energy,vol.151,Feb.2021,p.107857,doi:10.1 016/j.anucene.2020.107857.
[38] N.Zare,etal.,“RobustnessofOptimizedFPID ControllerAgainstUncertaintyandDisturbance byFractionalNonlinearModelforResearch NuclearReactor,” NuclearEngineeringandTechnology,vol.52,no.9,Sep.2020,pp.2017–2024, doi:10.1016/j.net.2020.03.002.
[39] Z.Chen,etal.,“AdaptiveResilientNeuralControl ofUncertainTime‐DelayNonlinearCPSswith Full‐StateConstraintsunderDeceptionAttacks,” Entropy,vol.25,no.6,2023,pp.900,doi:10.339 0/e25060900.
[40] S.Han,J.Dong,J.Zhou,andY.Chen,“Adaptive FuzzyPIDControlStrategyforVehicleActive SuspensionBasedonRoadEvaluation,” Electronics,vol.11,no.6,2022,pp.921,doi:10.3390/el ectronics11060921.
[41] H.Abdelfattah,M.Mosaad,andN.Ibrahim, “AdaptiveNeuroFuzzyTechniqueforSpeedCon‐trolofSix‐StepBrushlessDCMotor,” Indonesian JournalofElectricalEngineeringandInformatics (IJEEI),vol.9,no.2,Jun.2021,doi:10.52549/ije ei.v9i2.2614.
[42] S.Jović,“AdaptiveNeuro‐FuzzyPredictionof FlowPatternandGasHold‐UpinBubbleCol‐umnReactors,” EngineeringwithComputers,Jan. 2020,doi:10.1007/s00366‐019‐00905‐y.
[43] A.Marjani,M.Babanezhad,andS.Shirazian, “ApplicationofAdaptiveNetwork‐BasedFuzzy InferenceSystem(ANFIS)intheNumerical InvestigationofCu/WaterNano luidConvective Flow,” CaseStudiesinThermalEngineering,vol. 22,Dec.2020,p.100793;doi:10.1016/j.csite.20 20.100793.
[44] M.Aburakhis,etal.,“PerformanceofAnti‐LockBrakingSystemsBasedonAdaptiveand IntelligentControlMethodologies,” Indonesian JournalofElectricalEngineeringandInformatics (IJEEI),vol.10,no.3,2023.doi:10.52549/ijeei.v 10i3.3794.
[45] A.AbougarairandN.Shashoa,“IntegratedCon‐trollerDesignforUnderactuatedNonlinearSys‐tem,”2022SecondInternationalConferenceon Power,ControlandComputingTechnologies (ICPC2T),doi:10.1109/ICPC2T53885.2022.9 776984.
[46] A.Elmulhi,etal.,“SlidingModeControlforthe SatellitewiththeIn luenceofTimeDelay,”2023 IEEE3rdInternationalMaghrebMeetingofthe ConferenceonSciencesandTechniquesofAuto‐maticControlandComputerEngineering(MI‐STA),Libya,2023,doi:10.1109/MI‐STA57575 .2023.10169804.
[47] M.Aboud,andA.Emhemmed,“RobustH‐In inity ControllerSynthesisApproachforUncertainties System,” 2023IEEE11thInternationalConferenceonSystemsandControl(ICSC),doi:10.110 9/ICSC58660.2023.10449693.
[48] A.AbougarairandN.Shashoa,“ModelReference AdaptiveControlforTemperatureRegulationof ContinuousStirredTankReactor,” 2021IEEE2nd InternationalConferenceonSignal,Controland Communication(SCC),doi:10.1109/SCC53769 .2021.9768396.
Submitted:16th March2022;accepted:3rd April2024
ImadELMallahi,JamalRIFFI,HamidTairi,MohamedAdnaneMahraz DOI:10.14313/JAMRIS/4‐2024/33
Abstract:
Thisstudyproposesanintegratedframeworkforeffi‐cienttrafficobjectdetectionandclassificationbyleverag‐ingadvanceddeep‐learningtechniques.Theframework beginswiththeinputofvideosurveillance,followedbyan image‐acquisitionprocesstoextracttherelevantframes. Subsequently,aFasterR‐CNN(ResNet‐152)architecture wasemployedforpreciseobjectdetectionwithinthe extractedframes.Thedetectedobjectsarethenclassified usingdeepreinforcementlearning,specificallytrained toidentifydistincttrafficentities,suchasbuses,cars, trams,trolleybuses,andvans.TheUA‐DETRACdataset servedastheprimarydatasourcefortrainingandeval‐uation,ensuringthemodel’sadaptabilitytoreal‐world trafficscenarios.Finally,theperformanceoftheframe‐workwasassessedusingkeymetrics,includingprecision, recall,andF1score,providinginsightsintoitseffec‐tivenessinaccuratelydetectingandclassifyingtraffic objects.Thisintegratedapproachoffersapromisingsolu‐tiontoenhancetrafficsurveillancesystemsandfacilitate improvedtrafficmanagementandsafetymeasuresin urbanenvironments.
Keywords: classification,deeplearning,vehicledetection videosurveillance,trafficestimation,efficientR‐CNN, trafficdetection,convolutionalneuralnetwork
1.Introduction
Inrecentyears,bothindustryandacademia havewitnessedsigni icantadvancementsinvehicle‐detectiontechnologies[1].However,manystate‐of‐the‐art‐imageidenti icationalgorithmshavestrug‐gledtomeetstringentstandardsforvehicledetection. Theprimarychallengesinautomobileidenti ication includesubstantialdifferencesinobjectsize,signi i‐cantocclusions,and luctuationsinlightingconditions [2–5].
Sensor‐basedalgorithmshavebeenemployedto addresscertainsurveillancetasksinurbantraf icsys‐tems,includingvehiclecounting,licenseplateiden‐ti ication,incidentdetection,driverfacialemotion identi ication,andInternetofThings(IoT)source locationandidenti ication.Conversely,vision‐based approachesoffertheadvantageofleveragingvisual patternstodistinguishtargetobjectsinamannersim‐ilartohumanperception.
Whileradar‐sensor‐basedmethodsmaybelim‐itedtorelativelysmallareas,vision‐basedsystems utilizingcamerascandetectvehiclesacrossvast regionsandprovideadditionalinformationabout eachdetectedvehiclesimultaneously[6].
Consequently,researchershaveextensively exploredvariouscomputervisionandmachine learningmodelstoaddressarangeofchallengesin intelligenttransportationsystems.Classicvehicle detectionalgorithms,fromearlydevelopmentsto contemporaryapproaches,haveoftenreliedon handcraftedfeaturessuchasHaar‐likefeatures andhistogramoforientedgradient(HOG)features. Notably,thecascadeddetectorhasemergedas apioneeringreal‐timedetectionsystem,while methodssuchasSupportVectorMachines(SVM)and deformablepart‐basedmodels(DPM)haveaddressed issuessuchasheavyocclusionandsigni icant variationsinobjectsizes[7–13].
Toaddresschallenges,suchaslightvariance, occlusion,andsizevariations,researchershavepro‐posedinnovativesolutions.Forinstance,astrong CNNmodelwasdevelopedfortraf iclightrecognition, andstrategiesforhandlingheavyocclusionscaused by ixedsurveillancecamerasweredevised[14–16]. Inaddition,ascale‐awareRegionProposalNetwork (RPN)hasbeenintroducedtoeffectivelyidentifyvehi‐clesofvarioussizeseffectively[17,18].
Amongthevarioustechniquesexplored,region‐basedconvolutionalneuralnetworks(R‐CNNs)have demonstratedpromisingperformanceinvehicleiden‐ti ication.TheFasterR‐CNN(Resnet‐152),whichinte‐gratesaRegionProposalNetwork(RPN),hasshown competitiveperformanceinobjectrecognitiontasks. Notably,recentworkshavereportedimpressivemean averageaccuracyonbenchmarkdatasetssuchas KITTIandCOCO,showcasingtheeffectivenessof FasterR‐CNN(Resnet‐152)invehicledetection[19–22].
Despiteitscompetitiveperformance,there remainsroomforimprovementinFasterR‐CNN (Resnet‐152),particularlyinaddressingwide‐scale varianceinvehicledetection.Accurateclassi ication ofvehiclesintodistinctcategoriesiscrucialnot onlyfortransportationmanagementbutalsofor ef icientdamagedetectionininsurancesolutions. Therefore,thereisapressingneedforadvancements inautomaticdamageassessmentproceduresto
mitigateworkaccidentsandensureacomprehensive vehicleassessment[23–25].
Currently,mostcitiesworldwidehaveseveral videosurveillancesystems[26–30].Theyhavegrown rapidly,andnowtheyhaveheterogeneouscameras withvariousresolutions[1].Today,closed‐circuittele‐visionworksalltimesadayandweektoproducea hugeamountofdata,mainlybigandhugeframesof videos.ThedatavisualizationpresentedinFigure 1 offersacomprehensiveviewoftheinputfromvideo surveillancesystems,enablinganalyststoeffectively monitor,analyze,andinterpretactivitiescaptured withinthemonitoredenvironment.
FasterR‐CNN,anabbreviationfor“Region‐based ConvolutionalNeuralNetwork,”marksasigni icant advancementincomputervision’sobjectdetection capabilities.Itintroducesastreamlinedapproach, condensingobjectlocalizationandclassi icationinto asingle‐stepprocess.Thisbreakthroughsimpli ies detectiontasks,enablingaFasterR‐CNNtoswiftly analyzeimagesandvideoswithexceptionalaccuracy. Additionally,theevolutionofthistechnology,exem‐pli iedbyYOLOv8,continuestopushtheboundaries betweenobjectdetectionandimagesegmentation. YOLO’sreal‐timeperformancedistinguishesitasthe topchoiceforapplicationsdemandingbothrapidand preciseobjectidenti ication.
Theprimaryfocusofthisresearchisthedetection andclassi icationoftraf ic,whichisapivotalaspectof urbantraf icmanagementsystems.Theobjectiveisto preciselycategorizevehicleswithinde inedregionsin everyframetoaccuratelyevaluatetraf icdensity.This criticalinformationplaysakeyroleinrecognizing peaktraf ictimesandcongestedareasandcontributes signi icantlytourbanplanningefforts.Throughthis endeavor,ouraimistoconstructanextensivetoolkit capableofofferingnuancedanalysesoftraf ic low andtrends,therebybolsteringtraf icmanagement strategiesandurbanplanninginitiatives.Figure 2 showsthestructuredclassi icationandcategorization ofdeeplearningmethodsemployedinvehicledetec‐tionandclassi icationtasks.Themethodsareorga‐nizedintoseveralcategoriesbasedontheirunderly‐ingtechniquesandapproaches.
Moreover,thisvideodatacanbeusedasaprin‐cipalforautomatedvehiclecontrolsystems.There aremanyissueswhenworkingwithBigDatatraf ic surveillance.Torealizeanintelligentsystemfortraf ic vehiclesurveillance,wehaveanef icientharddisk systemforstoring,movingforwardandbackandana‐lyzingvideos.Inthisstudy,wefocusedonanalyzing videosfortraf icsurveillance,whichremainslimited intermsofreal‐timedataanalysis.
Somerepresentativepapersinthisthemeused heterogeneouslow‐resolutiondatatoestimatetraf ic densityandcountvehicles.
Manyeffortshavebeenmadetoprovidevehicle analysis,counting,classi ication,detection,andsatis‐factoryresultsinspeci ictasks.Theremainderofthis paperisorganizedasfollows.
Section 1 introducesrelatedstudiesontraf ic classif icationanddominantfeatureselectionmeth‐ods.InSection 2,wediscusstraf icclassif ication anddominantfeatureselectionmethods.Section 3 presentsthemethodologyusedinthisstudy.Section4 presentsexperimentalresultsandperformanceeval‐uation.Finally,inSection 5,weprovideconcluding remarks.
2.1.RelatedWorks
Detectingandcategorizingobjectswithinvideo footagerepresentsacriticalchallengeinthedevelop‐mentofautonomoussurveillancesystems.Numerous algorithmshavebeenproposedtoaddressthischal‐lenge,rangingfrombackground‐subtraction‐based techniquestoclassi ier‐basedmethodsforobject detectionandclassi icationinvideos.Eachapproach tothisproblempresentsitsownsetofadvantagesand drawbacks,necessitatingcarefulconsiderationofthe algorithmtypebestsuitedtothespeci ictaskathand [31,32].
Backgroundsubtractionmethodsinvolvethe detectionofnewobjectsinanimagethatare absentfromareferencebackgroundimage.The fundamentalprincipleinvolvessubtractinganew imagecontainingmultipleobjectstobedetectedfrom thereferenceimagetoyieldadifference‐encoded image.Athresholdvaluewasappliedtoenhance thebackgroundsubtraction’stolerancetopotential noisewithinthevideo.Subsequently,ablobdetector wasemployedtoidentifyandcountobjects.Each identi iedblobwasthentreatedasasingleobjectand subjectedtoclassi icationalgorithmsforre inement. Amoreintricatebackgroundsubtractionmethod basedonamixtureofGaussian(MoG)models, capableofdetectingnotonlyforegroundobjectpixels butalsotheshadowstheycast,hasbeenproposed inpreviousstudies.ThisMoG‐basedapproachhas alsobeenutilizedtodetecthumanmovementsin videos[33–36].
Thedetectionofspeci icobjectswithinimages poseschallengesduetovariationsinobjectsize,orien‐tation,andinstancesofoverlappingobjectsthatcause occlusions.Addressingthesechallengesrequiresa detectionalgorithmthatpossessescertainproperties includingtranslation,rotation,andscaleinvariance. Typically,machinelearningmethodsareemployed tolearnrepresentationsdirectlyfromavailabledata totrainmodels.Popularapproachesinvolveutiliz‐inglow‐levelfeatures,suchasScale‐InvariantFea‐tureTransform(SIFT),HistogramofOrientedGradi‐ents(HOG),andHaar‐likefeatures,combinedwith machinelearningtechniquesforobjectclassi ication. Thismethodologyiscommonlyreferredtoasthe “Feature+Classi ier”approach.Fortrackingorobject detectioninvideosurveillance,ef icientconvolutional neuralnetworks(FCNN),aredividedintotwoprinci‐palclasses:single‐phasecaptorsandsecondphase.
Figure1. Datavisualization
Classification andCategorizationofDeepLearning Methods for VehicleDetection andClassificationTasks
Benchmark Datasetsand Metricsfor Performance Functionsfor Activationand Loss Techniquesfor DetectingVehicles
Conventional Detection Techniques
Two-step Algorithms Basedon Convolutio nalNeural Histogramof Oriented Gradient
Haar
Localbinary Pattern
Benchmark Datasets Performance Evaluation R-CNN FastR-CNN
Activation
Refinementand Optimization
GradientDescent (GD)
Stochastic Gradient Descent(SGD)
Nesterov Momentum
Utilizationof DCNNfor VehicleDetection andClassification
Difficultiesand challenges
DL. inVehicle detection
DL. inVehicle Classification
FasterRCNN (Resnet-152) R-FCN
Adagrad
Adadelta
Single-stepAlgorithmsBasedon ConvolutionalNeuralNetworks
RetinaNet Algorithm
SSDAlgorithm
YOLO Algorithm
RMSProp
Adaptive Momentum Estimation
Adapg
RMSProp
YOLOv5 Algorithm YOLOv3 Algorithm
YOLOv4 Algorithm
YOLOv8 Algorithm
2.2.ObjectsDetectioninVideoSurveillance
Theprimary‐phasecaptoristypicallyveryfast andcanbeusedtopredictobjectsinvideoskipping
Prospective Avenuesand Tasksfor Future Dl
Multi-taskjoint Optimization andMulti-model information combination
Scale andsize alteration Spatial Correlations andContextual Modelling
Cascade Architecture Weakly supervised and Unsupervisd Learning
Model Optimizationn Lightweight Classification orDetection
Detectionor Classification inVideos
Figure2. Classificationandcategorizationofdeeplearningmethodsforvehicledetectionandclassificationtasks
boxeswithclasseswithinasimplenetwork.Thecon‐ventionalexperimentsofthe irstcaptorwereSSD andYOLO.Inaddition,theapplicationofgoodspeed‐precisiontrade‐ofisversion2fortheYOLOmethod,
particularlyforvehicledetectionviaanchorcluster‐ing,multi‐layerfeaturefusionstrategy,andlossnor‐malization.TheR‐CNNfamilyofcaptorsisusedin manyrepresentativetwo‐stagecaptormethods[37].
Thecomparisonbetweenthe irst‐andsecond‐stagecaptorsbeganwiththepredictionofregions,and thenclassi iedandre inedeachofthemduringthetwo stages.
The irststudyonR‐CNNemployedasimple approach:aregionwasgeneratedwithmanyselec‐tiveresearchalgorithmsandthentherecognitionthe objectwasimplemented.TheglobalVitesseofthe ef icientR‐CNNisfeasibleforcomputingthetimefor eachregion.Toresolvethisissue,weproposeaFast R‐CNN.InsteadofexecutingaCNNforeachregion. ModifyingselectiveresearchintheproposedEf icient R‐CNNwiththismethod,calledregion‐suggestednet‐work,alsocomputestheaccuracyandVitesseofthe captor[38,39].
Theprocesscommenceswiththeextractionof framesfromtheinputvideostreamandissubse‐quentlyforwardedtotheFasterR‐CNNmodelfor detection.FasterR‐CNNencompassestwodistinct modelswithinitsarchitecture.Initially,aregionpro‐posal(RP)augmentedwithDeformableConvolutional Networks(DCN)isexecutedtogeneratepotential objectregions.Thisstageef icientlyidenti iescandi‐dateregionswithinframesthatarelikelytocontain theobjectsofinterest.Subsequently,inthesecond model,aFastR‐CNNwasemployedtoconductobject detectionwithintheproposedregions,leveragingthe re inedregionproposalsobtainedfromthepreceding stage.ThearchitectureofFasterR‐CNN,delineating theintegrationofregionproposalandobjectdetection stages,isshowninFigure3,offeringavisualrepresen‐tationofthemodel’sintricatedesignandoperational low.
Thework lowofthedetectionandclassi ication modelsisshowninFigure3.Thediagramillustrates theinitialconversionofinputsurveillancevideosinto asequenceofframes,followedbytheapplicationof theFasterR‐CNNmodelfordetectionofeachframe individually.
3.1.DatasetofDetectionoftheVideoSurveillance
Inthisapplication,thenumberofsurveillance camerasismorethanfortysurveillancecameras,and mostsurveillancecamerasare ixed.Inthisdataset, mostcamerashad25framespersecond,witha resolutionof960 × 540.Moreover,thestreamsof thesevideosarenotverygoodbecauseofhardware faults,blurring,andcompressionartifacts.Figure 1 showsthevideofromoneofthesecameras,and Figure 4 showssomeexperimentationscamerasof UA‐DETRACdataset.Algorithm1leveragestheef i‐ciencyoftheFasterR‐CNNframework,enhancedby theResNet‐152backbone,toachievestate‐of‐the‐art performanceinobject‐detectiontasks.Owingtoits
intricatearchitectureandfeatureextractioncapabil‐ities,itenablestheaccurateandef icientdetectionof objectsindiversereal‐worldscenarios.
WefocusedondatasetssuchasUA‐DETRACand KITTI,notingkeydifferencesacrossvariousaspects: thetotaloccurrencesofthesamegrid,signi icant scalevariations,viewingangles,andocclusionlev‐els.Theprimarychallengewiththisdatasetliesin theimagesthemselves,asillustratedinFigure4.Our studyutilizedasmallcameramonitoringoneofthe manytraf ictypes,aimingtoachieveoptimaldetec‐tionaccuracywhileprovidingaviablealternativeto ixedcameras.Tothisend,wedevelopedspeci icsolu‐tionsandannotatedover60,000polygonsacross982 imagesfromtheselectedcamera,usingtheCOCO Annotatorutility[14].Annotatingvideosequences canbehighlytime‐consuming,especiallyincrowded scenes;therefore,wefocusedontraf icscenarios, weatherconditions,anddifferenttimesoftheday. Additionally,wemeticulouslyannotatedindividual vehicleswithahighdegreeofcon idence,particu‐larlyindensetraf icconditions.Table 1 providesan overviewofthedatasetdistribution.
Algorithm1. ObjectdetectionusingFasterR‐CNN (ResNet‐152).
1. InputVideoSurveillanceData:
• Receiveinputfromvideosurveillancesystemsthatcapture traf icscenes.
2. ImageAcquisitionandFrameExtraction:
• Extracttherelevantframesfromtheinputvideo.
3. ObjectDetectionUsingFasterR-CNN(ResNet-152):
• EmployFasterR‐CNN(ResNet‐152)architectureforprecise objectdetectionwithinextractedframes.
• Detectandlocalizetraf icobjects,suchasbuses,cars,trams, trolleybuses,andvans.
4. ObjectClassi icationusingDeepReinforcement Learning:
• Utilizedeepreinforcementlearningtechniquesforobject classi ication.
• Trainthemodeltoidentifydistincttraf icentitiesincluding buses,cars,trams,trolleybuses,andvans.
5. DatasetUtilization:
• UtilizetheUA‐DETRACdatasetastheprimarydatasource fortrainingandevaluation.
• Ensureadaptabilityofthemodeltovariousreal‐world traf icscenariospresentinthedataset.
6. PerformanceEvaluation:
• Assesstheperformanceoftheframeworkusingkeymetrics suchasprecision,recall,andF1score.
• Evaluatetheeffectivenessoftheframeworkinaccurately detectingandclassifyingtraf icobjects.
Output:
• Generateinsightsintotheeffectivenessoftheintegrated approachfortraf icsurveillance.
• Provideapromisingsolutionforenhancingtraf ic managementandsafetymeasuresinurbanenvironments.
IntelligentvideoanomalydetectionandclassificationusingFasterR‐CNN
Figure4. Someexperimentationscamerasof UA‐DETRACdataset
Table1. Distributionofdataset
4.1.NumericalResultandExperimentations
Toensurerobustevaluation,weutilizedthepub‐liclyavailableUA‐DETRACdatasettoconstructour trainingdataset.Thisdatasethasbeenextensively employedinpreviousstudiesfocusingontraf icclas‐si icationtasks,thusfacilitatingcomparabilitywith existingresearchoutcomes.Despitetherichdiversity ofimagescontainedwithintheUA‐DETRACdataset, thenumberoflabeledinstancesperframe,whichis crucialfortrainingdeep‐learningmodels,isinsuf i‐cient.
Inresponsetothislimitation,weaugmented thetrainingdatabygatheringsupplementaryUA‐DETRACdata,therebyenrichingourdatasetwitha morecomprehensiverepresentationoftraf ic lows andscenarios.
Inthisexperiment,asshowninFigure5,weper‐formedobjectdetectionusingFasterR‐CNNonan HPEliteBook ×3601130G5runningWindows11. ThesetupwasbasedonaPython3.8environment, utilizinganIntelCorei7—84002.80GHzCPUandan NvidiaGeForceRTX2160GPUwith8GBofmemory. WeemployedDarknet‐53astheconvolutionalneural networkwithinthedeeplearningframework.
Figure 6 illustratesseveralexperimentsinobject detectionusingFasterR‐CNN.Theconfusionmatrix, depictedinthe igure,isanessentialtoolforevalu‐atingclassi icationperformance,offeringinsightsinto theaccuracyofthemodel’spredictions.Itquanti ies correctandincorrectclassi icationsacrossvarious classesandenablesthecalculationofkeymetrics, includingtruepositives,truenegatives,falsepositives, andfalsenegatives.AsillustratedinFigure7,thecon‐fusionmatrixdelineatestheclassi icationoutcomes foreachclass.
Figure6. Someexperimentationsofobjectdetection usingFasterR‐CNN
Figure7. Trafficclassconfusionmatrix:buses,cars, trams,trolleybuses,andvans
Notably,themodelachievedanimpressiveover‐allclassi icationaccuracyofapproximately96.67%. Furthermore,byleveragingtheinformationfromthe confusionmatrix,metricssuchasprecision,recall, andF1‐scoreforeachservicecanbemeticulously calculated,asshowninFigure8.Thesemetricsoffer acomprehensiveevaluationofthemodel’sperfor‐mance,capturingitseffectivenessincorrectlyidenti‐fyinginstancesbelongingtospeci icclasses.
Thisstudypresentsanintegratedframework thatcombinesFasterR‐CNNanddeepreinforcement learningfortraf icobjectdetectionandclassi ication. Bysequentiallyprocessingvideosurveillancedata, extractingframes,andutilizingadvanceddeeplearn‐ingtechniques,theframeworkdemonstratedpromis‐ingresultsinaccuratelyidentifyingandcategorizing varioustraf icentities.
TheutilizationofFasterR‐CNN(ResNet‐152) ensuresrobustobjectdetectioncapabilities,enabling thesystemtolocalizeandidentifytraf icobjects withhighprecision.Subsequently,deepreinforce‐mentlearningenhancestheclassi icationprocess,
Figure8. Precision,recall,andF1‐scoreanalysisfor trafficclasses:buses,cars,trams,trolleybuses,andvans
enablingthemodeltoautonomouslylearnandclassify objects,suchasbuses,cars,trams,trolleybuses,and vans.
Theframework’sperformancewasevaluated usingkeymetricssuchasprecision,recall,and F1score,providingcomprehensiveinsightsinto itseffectiveness.Throughexperimentationwith theUA‐DETRACdataset,theframeworkshowsits adaptabilitytodiversetraf icscenarios,highlighting itspotentialforreal‐worldapplications.
ImadELMallahi∗ –https://orcid.org/0000‐0001‐9735‐6799SidiMohamedBenAbdellahUniversity, FacultyofSciences,DepartmentofComputerSciences, Fez,Morocco,e‐mail:imade.elmallahi@usmba.ac.ma. JamalRIFFI –https://orcid.org/0000‐0003‐0818‐7706SidiMohamedBenAbdellahUniversity,Faculty ofSciences,DepartmentofComputerSciences,Fez, Morocco,e‐mail:jamal.rif i@usmba.ac.ma.
HamidTairi –https://orcid.org/0000‐0002‐5445‐0037SidiMohamedBenAbdellahUniversity,Faculty ofSciences,DepartmentofComputerSciences,Fez, Morocco,e‐mail:hamid.tahiri@usmba.ac.ma.
MohamedAdnaneMahraz –https://orcid.org/00 00‐0002‐0966‐9654SidiMohamedBenAbdellahUni‐versity,FacultyofSciences,DepartmentofComputer Sciences,Fez,Morocco,e‐mail:hamid.tairi@usmba. ac.ma.
∗Correspondingauthor
Wegratefullyacknowledgethesupportprovidedby theLISACLaboratory,DepartmentofComputerSci‐ences,FacultyofScience,SidiMohamedBenAbdellah
UniversityofFez,Morocco,fortheirassistanceinfacil‐itatingthisstudy.Theirsupportwasinstrumentalin thesuccessfulcompletionofthiswork.
References
[1] R.Sha iqueetal.,“AdvancingAutonomousVehi‐cleSafety:MachineLearningtoPredictSensor‐RelatedAccidentSeverity”, IEEEAccess,vol.12, 2024,pp.25933–25948.
[2] S.Ahnetal.,“ExplainingDeepLearning‐Based Traf icClassi icationUsingaGeneticAlgorithm,” IEEEAccess,vol.9,2021,pp.4738–4751;doi: 10.1109/ACCESS.2020.3048348.
[3] M.A.Berwoetal.,“DeepLearningTechniques forVehicleDetectionandClassi icationfrom Images/Videos:ASurvey” Sensors,vol.23,no.10, 2023:4832;doi:10.3390/s23104832.
[4] Y.Ghasemietal.,“DeepLearning‐BasedObject DetectionInAugmentedReality:ASystematic Review,” ComputersinIndustry,vol.139,2022, p.103661,ISSN0166‐3615;doi:10.1016/j.co mpind.2022.103661.
[5] X.Zhangetal.,“VideoAnomalyDetectionAnd LocalizationUsingMotion‐FieldShapeDescrip‐tionAndHomogeneityTesting,” PatternRecognition,vol.105,2020,p.107394.
[6] SVeluchamy,LRKarlmarx,KMichaelMahesh, DetectionandLocalizationofAbnormalitiesin SurveillanceVideoUsingTimerider‐BasedNeu‐ralNetwork,TheComputerJournal,Volume64, Issue12,December2021,Pages1886–1906,ht tps://doi.org/10.1093/comjnl/bxab002
[7] Y.Fanetal.,“VideoAnomalyDetectionandLocal‐izationviaGaussianMixtureFullyConvolutional VariationalAutoencoder,” ComputerVisionand ImageUnderstanding,vol.195,2020,p.102920.
[8] A.Alametal.,“Intellibvr‐IntelligentLarge‐Scale VideoRetrievalforObjectsandEventsUtiliz‐ingDistributedDeep‐LearningandSemantic Approaches,” 2020IEEEInternationalConference onBigDataandSmartComputing(BigComp), IEEE,2020,February,pp.28–35.
[9] S.LiuandJ.Tang,“Modi iedDeepReinforce‐mentLearningwithEf icientConvolutionFea‐tureforSmallTargetDetectioninVhrRemote SensingImagery,” ISPRSInternationalJournalof Geo-Information,vol.10,no.3,2021,p.170.
[10] S.K.Lakshmanaprabuetal.,“OnlineClinicalDeci‐sionSupportSystemusingOptimalDeepNeural Networks,” AppliedSoftComputing,vol.81,2019, p.105487.
[11] Uthayakumar,J.,Metawa,N.,Shankar,K.etal. RETRACTEDARTICLE:Intelligenthybridmodel for inancialcrisispredictionusingmachine learningtechniques.InfSystE‐BusManage18, 617–645(2020).https://doi.org/10.1007/s102 57‐018‐0388‐9.
[12] R.Hinami,T.Mei,andS.Satoh,“JointDetection andRecountingofAbnormalEventsbyLearn‐ingDeepGenericKnowledge,” IEEEInternational ConferenceonComputerVision(ICCV),2017.
[13] R.T.Ionescuetal.,“Object‐CentricAuto‐Encoders andDummyAnomaliesforAbnormalEvent DetectioninVideo,” TheIEEEConferenceon ComputerVisionandPatternRecognition(CVPR), 2019.
[14] W.Luo,W.Liu,andS.Gao,“ARevisitofSparse CodingBasedAnomalyDetectioninStacked RNNFramework,” 2017IEEEInternationalConferenceonComputerVision(ICCV),2017,pp. 341–349.
[15] M.Sabokrou,M.Fathy,andM.Hoseini,“Video AnomalyDetectionandLocalizationBasedon theSparsityandReconstructionErrorofAuto‐Encoder,” ElectronicsLetters,vol.52,no.13, 2016,pp.1122–1124.
[16] M.Ravanbakhshetal.,“Plug‐and‐PlayCNN forCrowdMotionAnalysis:AnApplicationin AnomalousEventDetection,” WACV,2017.
[17] M.Sabokrouetal.,“Deepanomaly:FullyConvolu‐tionalNeuralNetworkforFastAnomalyDetec‐tioninCrowdedScenes,” ComputerVisionand ImageUnderstanding,vol.172,2018,pp.88–97.
[18] M.Hasanetal.,“LearningTemporalRegularityin VideoSequences,” CVPR,2016.
[19] D.Xuetal.,“LearningDeepRepresentationsof AppearanceandMotionforAnomalousEvent Detection,” BMVC,2015,pp.1–12.
[20] M.Bellveretal.,“HierarchicalObjectDetection withDeepReinforcementLearning,” Proceedings oftheConferenceonNeuralInformationProcessingSystems,Barcelona,Spain,December2016, pp.5–20.
[21] X.Kongetal.,“CollaborativeDeepReinforcement LearningforJointObjectSearch,” Proceedings oftheIEEEConferenceonComputerVisionand PatternRecognition,SanFrancisco,CA,USA21–26July2017,pp.7072–7081.
[22] B.Uzkent,C.Yeh,andS.Ermon,“Ef icientObject DetectioninLargeImagesusingDeepReinforce‐mentLearning,” Proceedingsofthe2020IEEE WinterConferenceonApplicationsofComputer Vision,SnowmassVillage,CO,USA1–5March 2020,pp.1824–1833.
[23] S.Liu,D.Huang,andY.Wang,“PayAttention toThem:DeepReinforcementLearning‐Based CascadeObjectDetection,” IEEETransactionson NeuralNetworksandLearningSystems,vol.31, 2020,pp.2544–2556.
[24] S.Renetal.,“FasterRCNN(Resnet‐152): TowardsReal‐TimeObjectDetectionwith RegionProposalNetworks,” IEEETransactions onPatternAnalysisandMachineIntelligence,vol. 39,no.6,2015,pp.1137–1149.
[25] A.A.MichealandK.Vani,“AutomaticObject TrackinginOptimizedUAVVideo,” Journalof Supercomputing,vol.75,no.8,2019,pp.4986–4999.
[26] X.LeiandZ.Sui,“IntelligentFaultDetectionof HighVoltageLineBasedontheFasterRCNN,” Measurement,vol.138,2019,pp.379–385.
[27] Y.Dingetal.,“IntelligentFaultDiagnosisfor RotatingMachineryusingDeepQ‐Network BasedHealthStateClassi ication:ADeep ReinforcementLearningApproach,” Advanced EngineeringInformatics,vol.42,2019,p. 100977.
[28] B.S.Muruganetal.,“Region‐BasedScalable SmartSystemforAnomalyDetectioninPedes‐trianWalkways,” ComputersandElectricalEngineering,vol.75,2019,pp.146–160.
[29] L.Zadeh,“ProbabilityMeasuresofFuzzyEvents”, JournalofMathematicalAnalysisandApplications,vol.23,no.2,1968,pp.421–427.
[30] I.ElMallahietal.,“ADistributedBigDataAna‐lyticsModelsforTraf icAccidentsClassi ication andRecognitionBasedSparkMlLibCores,” JournalofAutomation,MobileRoboticsandIntelligent Systems,vol.16,no.4,2023,pp.62–71.doi:10.1 4313/JAMRIS/4‐2022/34.
[31] R.Sha iqueetal.,“AdvancingAutonomousVehi‐cleSafety:MachineLearningtoPredictSensor‐RelatedAccidentSeverity”, IEEEAccess,vol.12, 2024,pp.25933–25948.
[32] N.SohaeeandS.Bohluli,“NonlinearAnalysis oftheEffectsofSocioeconomic,Demographic, andTechnologicalFactorsontheNumberof FatalTraf icAccidents”, Safety,vol.10,no.1,2024, p.11.
[33] I.E.Mallahietal.,“PredictionofTraf icAccidents usingRandomForestModel,” 2022International ConferenceonIntelligentSystemsandComputer Vision(ISCV),Fez,Morocco,2022,pp.1–7;doi: 10.1109/ISCV54655.2022.9806099.
[34] A.Nasry,A.Ezzahout,andF.Omary,“People TrackinginVideoSurveillanceSystemsBased
onArti icialIntelligence,” JournalofAutomation, MobileRoboticsandIntelligentSystems,vol.17, no.1,2023,59–68;doi:10.14313/JAMRIS/1‐2023/8.
[35] A.Ndayikengurukiyeetal.,“ResourceOptimi‐sationinCloudComputing:ComparativeStudy ofAlgorithmsAppliedtoRecommendationsin aBigDataAnalysisArchitecture,”Journalof Automation,MobileRoboticsandIntelligentSys‐tems,vol.15,no.4,2022,pp.65–75;doi:10.143 13/JAMRIS/4‐2021/28.
[36] A.Nasry,A.Ezzahout,andF.Omary,“People TrackinginVideoSurveillanceSystemsBased onArti icialIntelligence,” JournalofAutomation, MobileRoboticsandIntelligentSystems,vol.17, no.1,2023,pp.59–68;doi:10.14313/JAMRIS/ 1‐2023/8.
[37] N.Ferhaneetal.,“DataDrivenDecisionMak‐ingwithIntelligentCCTV,”In:M.Ezziyyani,J. Kacprzyk,V.E.Balas,eds., InternationalConferenceonAdvancedIntelligentSystemsforSustainableDevelopment(AI2SD’2023).AI2SD2023. LectureNotesinNetworksandSystems,vol.905. Cham:Springer,2024;doi:10.1007/978‐3‐031‐52385‐4_154.
[38] A.Ezzahoutz,H.M.YoussefandR.O.H.Thami, “DetectionEvaluationandTestingRegionIncom‐ingPeople’sinaSimpleCameraView,” Second InternationalConferenceontheInnovativeComputingTechnology(INTECH2012),Casablanca, Morocco,2012,pp.179–183;doi:10.1109/IN TECH.2012.6457804.
[39] A.Nasry,A.Ezzahout,andF.Omary,“People TrackinginVideoSurveillanceSystemsBased onArti icialIntelligence,” JournalofAutomation, MobileRoboticsandIntelligentSystems,vol.17, no.1,2023,pp.59–68;doi:10.14313/JAMRIS/ 1‐2023/8.