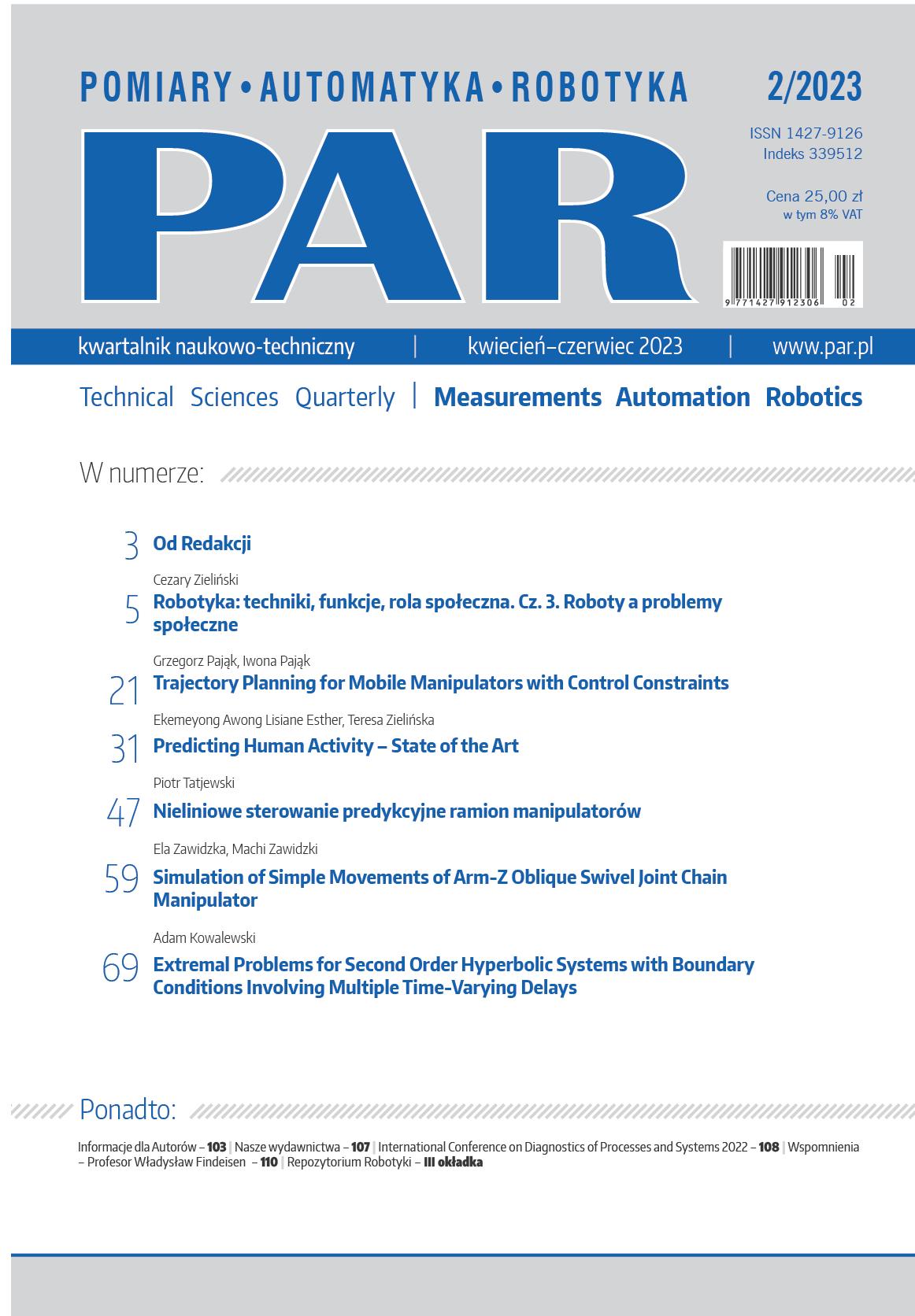
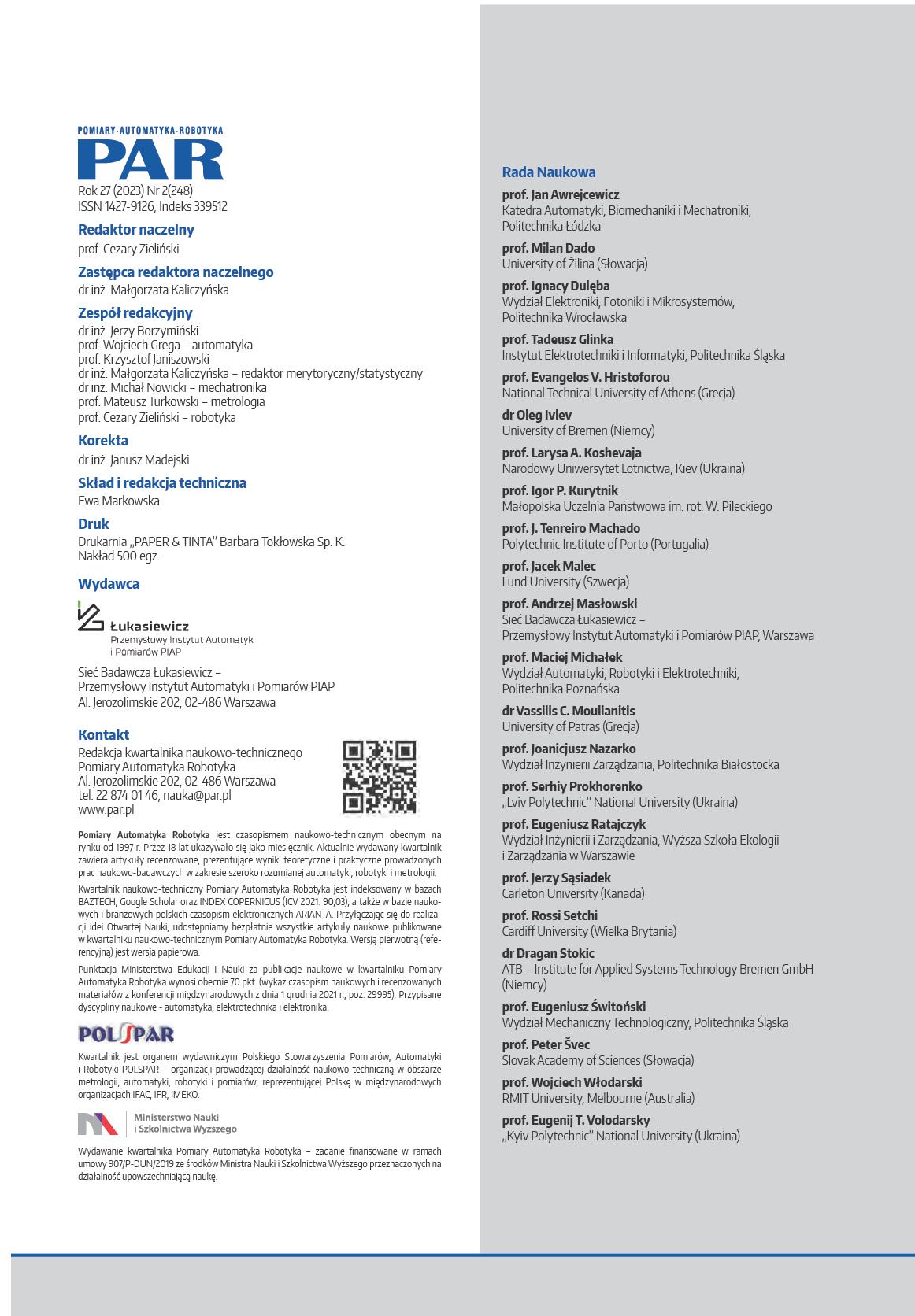
Aby ocenić, jaki wpływ będą miały roboty na społeczeństwo, należy skrupulatnie przeanalizować obecny stan wiedzy, a w szczególności wskazać fundamentalne problemy, które jeszcze nie zostały rozwiązane, mające istotne znaczenie dla potencjalnych zmian społecznych powodowanych rozwojem robotyki. Wspomniany wpływ zależy od inteligencji robotów, więc ten aspekt dominuje w przedstawionej tu analizie. Rozważania zostały podzielone na trzy części:
1) analizę czynników technicznych wpływających na inteligencję i bezpieczeństwo robotów,
2) analizę obecnych możliwości robotów, 3) analizę przewidywań dotyczących rozwoju robotyki, a w konsekwencji poglądów na skutki tego rozwoju dla społeczeństwa. Niniejszy artykuł poświęcony jest trzeciemu z wymienionych tu zagadnień.
W pierwszej części artykułu przedstawiono czynniki wpływające na inteligencję i bezpieczeństwo robotów. Skoncentrowano się przede wszystkim na inteligencji, bo to ona determinuje akceptację tych urządzeń przez ludzi, szczególnie jeżeli mają one pełnić rolę kompanów lub pomocników. W części drugiej określono aktualne możliwości robotów. Podzielono je na trzy kategorie – roboty: przemysłowe, usługowe oraz terenowe. Przegląd ten wskazuje, że w istocie robotyka dokonała olbrzymiego postępu od momentu, gdy zainstalowano pierwszego robota w fabryce na początku lat sześćdziesiątych ubiegłego wieku. Niemniej jednak nadal robotom wiele brakuje, by mogły uzyskać status robotów kompanów. W szczególności pomimo bardzo szybkiego rozwoju sztucznej inteligencji roboty radzą sobie jedynie z pojedynczymi kategoriami zadań. Ich ogólna inteligencja jest jeszcze bardzo niska.
Ta część artykułu przedstawi różne stanowiska dotyczące antycypowanego wpływu robotyki i sztucznej inteligencji na społeczeństwo. Owe próby przewidywania przyszłości bazują na dotychczas obserwowanych globalnych trendach rozwojowych cywilizacji ludzkiej, więc owe trendy zostaną omówione na wstępie. Ponieważ prognozy w istotny spo-
sób się różnią od siebie, pogrupowano je w trzy kategorie i przedstawiono każdą z nich. Na zakończenie oceniono, które z tych prognoz są najbardziej prawdopodobne, by się ziściły. Oczywiście wzięto pod uwagę perspektywę 20–30 lat. Przewidywanie na dłuższym horyzoncie, przy obecnym tempie rozwoju nauki, zakrawa na wróżbiarstwo. Ponadto należy wziąć pod uwagę, że rozwój robotyki i sztucznej inteligencji może być nieistotnym czynnikiem wpływającym na cywilizację, wobec globalnych katastrof, do jakich dąży ludzkość (destabilizacja klimatu, zagłada nuklearna, wywołanie potężnych ruchów migracyjnych, wyczerpanie niezbędnych do przetrwania zasobów naturalnych etc.).
Epoki cywilizacyjne wyznaczane są przez dominujące sposoby wytwarzania oraz zaspakajania potrzeb ludzkich. Można wyróżnić okresy w miarę stabilnego wykorzystania pewnego typu narzędzi, i co za tym idzie sposobów wytwarzania, po których następowała relatywnie gwałtowna zmiana dzięki pojawieniu się innowacji – stąd często mówi się o rewolucji.
W ewolucji człowieka pierwszym istotnym wynalazkiem było ujarzmienie ognia. Umożliwiło to przetwarzanie termiczne jedzenia, a to wpłynęło na modyfikację naszego układu pokarmowego. Oprócz niszczenia szkodliwych patogenów, wskutek termicznego przetworzenia pożywienia organizm zużywał mniej energii do trawienia pokarmu, co w konsekwencji umożliwiło dostarczenie więcej energii mózgowi do jego rozwoju [77]. W paleolicie dominowało zbieractwo i łowiectwo, do praktykowania których wystarczały narzędzia kamienne [17]. W mezolicie zadebiutowało rybołówstwo, które wymagało harpunów i haczyków wykonanych
z kości [12]. Początki neolitu wiązane są z pojawieniem się rolnictwa i hodowli zwierząt [24]. Rolnictwo wystąpiło około 10 tys. lat p.n.e. na terenach Mezopotamii i Azji Mniejszej, a potem w dolinie Indusu, nad Żółtą Rzeką i Jangcy oraz na obszarze obecnego Meksyku i Peru. Przyjęło się nazywać tę zmianę rewolucją neolityczną. Uprawa roślin wiązała się ze zmianą trybu życia z wędrownego na osiadły. Rolnictwo wymaga gromadzenia w jednym miejscu narzędzi potrzebnych do uprawy roślin oraz bardziej rozbudowanych struktur społecznych niezbędnych do zabezpieczenia praw własności. Tworzono narzędzia kamienno-drewniane oraz naczynia ceramiczne.
Wytwarzanie narzędzi spowodowało powstanie podziału pracy. Pojawili się rzemieślnicy. Nadwyżki ponad potrzeby wytwórcy spowodowały rozwój handlu. Dalszy rozwój cywilizacyjny związany był z metalurgią, wpierw miedzi, potem brązu, a następnie żelaza [33]. Nowe narzędzia zwiększały wydajność rolnictwa i rzemiosła, ale podst awową kwestią było pozyskanie energii do ich napędu. Hodowla zwierząt pociągowych spowodowała zastąpienie pracy mięśni ludzkich pracą mięśni zwierząt. Jak wielki wpływ miało to na rozwój danych regionów świata widać patrząc na to, gdzie rozwinęły się wielkie cywilizacje. Nastąpiło to na tych szerokościach geograficznych, gdzie zaczęto hodować konie, woły i osły. W Afryce subsaharyjskiej, gdzie nie udało się udomowić zebr, rozwój był istotnie spowolniony [18]. Wykorzystanie wiatru umożliwiło zarówno zwiększenie wydajności przetwarzania żywności (wiatraki do mielenia zboża) jak i zasięgu handlu (żaglowce).
Niemniej jednak dopiero wynalezienie maszyny parowej przez Thomasa Newcomena i udoskonalenie jej przez Jamesa Watta w XVIII wieku spowodowało rewolucję przemysłową [68]. Obecnie ówczesny przemysł niektórzy określają mianem Przemysłu 1.0. Silnik spalinowy usprawnił transport, natomiast elektryczny produkcję. Dodatkowo zmiany w organizacji produkcji, spowodowane przez zorganizowanie taśmy produkcyjnej przez Henry’ego Forda w sumie doprowadziły do drugiej rewolucji przemysłowej i powstania Przemysłu 2.0.
Kolejna rewolucja jest kojarzona z elektroniką, a tak dokładniej ze stworzeniem komputera i jego upowszechnieniem dzięki mikroprocesorom, co nastąpiło w latach 80. XX wieku. W szczególności wykorzystano je do stworzenia maszyn sterowanych numerycznie i upowszechnienia automatyzacji procesów produkcyjnych. Mówi się tu o rewolucji informatycznej i powstaniu gospodarki opartej na wiedzy oraz o Przemyśle 3.0. Obecnie tworzony jest Przemysł 4.0, którego podstawę ma stanowić sieć porozumiewających się urządzeń, mających dostęp do olbrzymich ilości danych rezydujących w chmurach obliczeniowych. Jego podstawą ma być Internet Rzeczy (IoT), czyli sieć połączonych urządzeń, zawierających w sobie czujniki, siłowniki, komputery sterujące i ich oprogramowanie. Urządzenia te wymieniając dane mogą optymalizować swoje działanie. Przykładem jest lodówka składająca zamówienie w sklepie internetowym, gdy poziom zapasów spada poniżej wymaganego progu.
Podanie ścisłych dat, gdy następowały poszczególne zmiany, jest trudne, bo między pojawieniem się wynalazku, a jego szerokim zastosowaniu mijało czasami i kilkadziesiąt lat [81], a co więcej istotną zmianę nie powoduje pojedynczy wynalazek, ale koniunkcja kilku z nich. Dlatego wspomniane rewolucje należy traktować raczej jako procesy rozłożone w czasie. Należy jednak zwrócić uwagę, że kolejne rewolucje następują coraz częściej, a czasy transformacji stają się coraz krótsze. Procesy cywilizacyjne przyspieszają. Niemniej jednak, pomimo globalizacji, nie występują wszędzie na naszym globie jednocześnie. Inicjowane są w strefach, gdzie postęp naukowy i techniczny jest najintensywniejszy,
a następnie rozprzestrzeniają się na pozostałe rejony. Osobnym zagadnieniem jest zbadanie, jak te rejony inicjacyjne przesuwały się i jakie były tego przyczyny.
Na powyżej opisane procesy warto spojrzeć z punktu widzenia zatrudnienia. Na przestrzeni dziejów w istotny sposób zmieniał się charakter życia ludności – początkowo dominował nomadyzm, który stopniowo przeistaczał się w tryb osiadły, lepiej dostosowany do rolnictwa. Zmiana trybu życia nie odbywała się na drodze pokojowej. Ostatnim istotnym zrywem, który miał przywrócić dominację swobodnej wędrówki nad osiadłym trybem związanym z rolnictwem były najazdy Mongolskie w XIII wieku. Jednak rolnictwo przynosiło większe wpływy podatkowe, więc Mongołowie odstąpili od jego zniszczenia [32, 51].
Rewolucja przemysłowa spowodowała istotne zmniejszenie zatrudnienia w rolnictwie i rzemiośle na korzyść zatrudnienia w fabrykach. Zastępowanie pracy ludzkiej pracą maszyn spowodowało masowe protesty, czego przykładem był ruch Luddystów. Mechanizacja, a później automatyzacja produkcji, dalej zmniejszyły zatrudnienie w rolnictwie, ale również ograniczyły liczbę ludzi pracujących w przemyśle wytwórczym. Nadmiar siły roboczej przesunął się do sfery usług. Rewolucja informatyczna, a w szczególności jej kolejna faza związana z rozwojem robotyki i sztucznej inteligencji, powoduje redukcję miejsc pracy w usługach. Podstawowym pytaniem jest, jaki wpływ ten globalny trend będzie miał na przyszłość ludzkości?
Istnieją trzy typy prognoz rozwoju tego procesu.
− Pierwszy typ prognoz wieszczy pozbawienie większości ludzi zatrudnienia, np. [21].
− Drugi, że znikną stare zawody, ale pojawią się nowe lepiej płatne, np. [55].
−Natomiast trzeci prorokuje, iż znikną stare zawody, ale nowe zajęcia będą gorzej płatne, np. [22].
U podstaw pierwszego typu prognoz tkwi przeświadczenie, że trend do przemieszczania siły roboczej do nowych działów gospodarki się wyczerpał. Drugi wynika z ekstrapolacji dotychczasowej historii, ale przyznaje, że od pracowników będą wymagane inne zdolności i wiedza, a więc będą to zajęcia lepiej płatne, niż dotychczas. Wreszcie trzeci także wychodzi z podobnych założeń, co drugi, ale wskazuje, że paradoksalnie łatwiej się automatyzuje prace wymagające wysokich kwalifikacji, a ponadto pracownicy będą musieli konkurować z robotami, a więc ich płace będą niższe niż obecnie.
Pierwszy typ prognoz [21] wskazuje, że sekwencja: rolnictwo-przemysł-usługi nie ma widocznej kontynuacji. Drugi [55] i trzeci [22] wskazują, że w dotychczasowej historii zawsze, jeżeli wskutek innowacji dochodziło do ograniczenia pewnej sfery zatrudnienia, pojawiał się nowy obszar, który wchłaniał przekwalifikowaną siłę roboczą. Ponadto z każdą nową falą rewolucji przemysłowej następował istotny przyrost PKB na mieszkańca [54]. Analizując historię gospodarczą Anglii można zauważyć, że w ciągu 1700 lat poprzedzających pierwszą rewolucją przemysłową PKB na mieszkańca Anglii wzrósł o 32 %, natomiast w ciągu pierwszych 40 lat po każdej z rewolucji przemysłowych nastąpił znacznie większy wzrost: pierwsza dała 16 % wzrost (dzięki silnikowi parowemu, 1780–1820), druga wywołała przyrost o 80 % (dzięki ujarzmieniu elektryczność, 1870–1910), a trzecia przyniosła 100% wzrost (spowodowany rozwojem elektroniki i informatyki) [54]. W istocie tak było, ale doty-
czyło to przesuwania siły roboczej do kolejnej sfery, w której wykorzystywano w inny sposób siłę ludzkich mięśni, ale obecnie mamy do czynienia z wypieraniem pracowników ze sfer, w których wykorzystywane są zdolności intelektualne.
Przewiduje się, że wkrótce najbardziej poszukiwanymi zdolnościami pracowników będą umiejętności miękkie (zarządzanie, samodzielne myślenie, rozwiązywanie problemów, praca zespołowa, działania artystyczne, interakcja z ludźmi, inteligencja emocjonalna) oraz zdolności poznawcze i techniczne [53].
Richard Freeman nie upatruje zagrożenia w eliminowaniu ludzi przez roboty z rynku pracy, ale spodziewa się spadku zarobków pracowników oraz powiększenia się rozwarstwienia społecznego [22]. Dla uzasadnienia swojej tezy powołuje się na znaną w ekonomii zasadę komparatywnej przewagi (ang. comparative advantage ). Zaobserwowano, że jeżeli nawet wydajność produkcji wyrobu A w kraju X jest dwukrotnie wyższa niż w kraju Y, a wyrobu B, dajmy na to, półtorakrotnie wyższa w X niż w Y, to cała produkcja wyrobów B nie zostanie przeniesiona do kraju X. Produkcja wyrobu B zostanie w kraju Y, bo krajowi X bardziej się opłaca zaangażować swoje zasoby do produkcji A. Niestety nie gwarantuje to, że w kraju Y płace robotników wytwarzających wyrób B nie spadną. Ponadto Freeman sformułował trzy prawa robo-nomiki.
1) Postęp w sztucznej inteligencji i robotyce spowoduje stworzenie maszyn, których praca będzie stanowić coraz lepszy substytut pracy ludzkiej.
2) Koszty robotów będą maleć ze wzrostem ich produkcji, wywierając presję, aby redukować płace pracowników.
3) Dochód będzie w coraz większym stopniu wynikał z posiadania robotów oraz innych form kapitału, a w coraz mniejszym z pracy ludzkiej.
Tak więc, Freeman widzi problem nie w tym, że roboty zabiorą ludziom pracę, ale że doprowadzą do dalszego zwiększenia nierówności społecznych. Jako remedium, proponuje zastosowanie ulg podatkowych dla firm, które będą zwiększały udział swych pracowników we własności tych firm.
Jak wskazano powyżej, istnieją drastycznie różne przewidywania, co do konsekwencji powszechnego wykorzystania robotyki i sztucznej inteligencji. Oznacza to, że istnieje pilna potrzeba przeprowadzenia badań, które rozstrzygną, na ile te technologie stanowią zagrożenie dla miejsc pracy, a na ile będą zbawienne dla ułatwienia życia ludziom. Zmniejszenie liczby miejsc pracy może być istotnym powodem dla wprowadzenia dochodu gwarantowanego i skrócenia dnia pracy. Do tego jednak należy się przygotować zawczasu, a więc podjąć odpowiednie decyzje polityczne, a te powinny być poprzedzone rzetelną analizą naukową pojawiającego się trendu.
Warto się też przyjrzeć, jak dokładne były niegdysiejsze przewidywania w tej materii. Analizy z końca ubiegłego wieku [48], dotyczące szans i zagrożeń związanych z rozwojem społeczeństwa informacyjnego, wskazywały, że era (cywilizacja) przemysłowa się kończy i że jesteśmy świadkami narodzin ery (cywilizacji) informacyjnej. W dyskusji towarzyszącej tej transformacji optymiści cieszyli się, że właśnie następuje wyzwolenie człowieka z ograniczeń materialnych i społecznych, natomiast pesymiści martwili się, że dokonuje się zniewolenie człowieka przez technikę. Analiza ówczesnych dokumentów Unii Europejskiej, dotyczących tej kwestii, wskazywała na możliwość powstania podziałów społecznych na tle dostępu do informacji, a w konsekwencji na powstawanie dużych obszarów biedy i małych obszarów bogactwa, co globalizacja gospodarki światowej miała tylko potęgować. Niestety te przewidywania okazały się w dużej mierze prawdziwe, i to pomimo podejmowania prób zara-
dzenia im. W 2022 r. najbiedniejsza połowa światowej populacji posiadała zaledwie 2 % całości bogactwa, natomiast najbogatsze 10 % światowej populacji posiadało 76 % całego bogactwa [14]. Wprawdzie żaden ze skrajnych scenariuszy się nie ziścił, ale przewidywane problemy i ich konsekwencje wystąpiły. Ciekawym jest, że ówcześnie nie odnoszono się bezpośrednio ani do sztucznej inteligencji ani do robotyki, jako przyczynom ewentualnych problemów. Przedmiotem troski był nierównomierny dostęp do technologii ICT, a w konsekwencji różny dostęp do wiedzy. Co więcej, prognozy te zakładały, że przekazywana informacja będzie prawdziwa, a obecnie widać, jakie spustoszenie robi kolportowanie informacji nieprawdziwych, prowadzące do powstawania różnych absurdalnych teorii spiskowych [20, 38].
Prognozy demograficzne z przełomu lat 60. i 70. XX w. przewidywały, że potrzebne będzie wprowadzenie sztucznych (nakazowych) ograniczeń rozrodczości, by zapobiec nadmiernemu zużywaniu zasobów naturalnych, a w konsekwencji zapaści ekonomicznej oraz katastrofie środowiskowej [56]. Wiele z tych prognoz okazało się słusznych, niemniej jednak liczba ludności na Ziemi rośnie zdecydowanie wolniej niż przewidywano, a w wielu krajach zaczyna się kurczyć. Ograniczenie zużycia zasobów naturalnych niewątpliwie jest konieczne, ale głównie ze względów klimatycznych. Niemniej jednak należy się spodziewać, że głównym problemem demograficznym będzie zmniejszanie się liczby ludzi zdatnych do pracy w stosunku do liczby ludzi wymagających opieki [50]. Wykazano, że istnieje istotna korelacja miedzy dzietnością kobiet a stopniem wykształcenia społeczeństwa [49]. Im wykształcenie wyższe tym liczba dzieci niższa.
Drugim istotnym czynnikiem jest urbanizacja. Ponieważ zarówno stopień skolaryzacji jak i urbanizacji wzrasta, to należy się spodziewać, że problem niedoboru rąk do pracy będzie się pogłębiał. Tak więc zmniejszenie ludności planety będzie miało korzystny wpływ na środowisko, klimat oraz produkcję żywności i zużycie zasobów, ale niekorzystny wpływ na siłę roboczą, opiekę społeczną i wzrost gospodarczy [76]. Dotychczas z problemem braku rąk do pracy radzono sobie poprzez import siły roboczej z krajów, które miały jej nadmiar. Niestety rodziło to napięcia społeczne, co powoduje niechęć społeczeństw do przyjmowania migrantów. Odpowiedzią na te problemy jest wykorzystanie sztucznej inteligencji oraz robotyzacja.
Praca [73] Vernora Vinge’a opublikowana w 1993 r. przedstawia różne scenariusze, które mogą doprowadzić do osiągnięcia osobliwości technologicznej. Nazwa związana jest z podziałem na dwa okresy oddzielone od siebie osobliwością. W pierwszym z nich, na podstawie obecnej wiedzy, jesteśmy w stanie przewidywać dalszy rozwój nauki i technologii. Jednakże po przekroczeniu osobliwości już takie przewidywania stają się niemożliwe. Ma to być związane z osiągnięciem przez maszyny poziomu inteligencji przewyższającej ludzką, a więc dalszy rozwój nie będzie zależny od ludzi, ale od maszyn, i w związku z tym ludzie nie będą w stanie przewidzieć dokąd ten dalszy rozwój będzie prowadził. Maszyny same będą projektowały i wytwarzały coraz inteligentniejsze maszyny. Być może powstanie hybryda ludzko-maszynowa. Na tej bazie powstało wiele prac przewidujących, jak dojdzie do osiągnięcia tej osobliwości oraz kiedy to nastąpi. Najbardziej znane są książki Raymonda Kurzweila, które wywołały w środowiskach naukowych ożywioną dyskusję dotyczącą możliwości, prawdopodobieństwa i ewentualnego terminu osiągnięcia osobliwości.
W 2008 r. Vinge nadal się spodziewał, że do 2030 r. osiągnięta zostanie osobliwość technologiczna, oczywiście jeżeli ludzkość nie spotka jakaś katastrofa w rodzaju wojny nuklearnej, powszechnej zarazy lub załamania klimatycznego [74]. Owa osobliwość ma być osiągnięta przez kombinację następujących czynników:
− wytworzenie nadludzkiej sztucznej inteligencji w komputerze,
− rozszerzenie ludzkiej inteligencji przez połączenie mózgu z komputerem,
− zwiększenie potencjału naszego mózgu poprzez biomedyczne ingerencje w neurologiczne jego działanie, −sieci komputerowe osiągną superinteligencję,
− sieć mikroprocesorów (rodzaj Internetu Rzeczy) stanie
się na tyle efektywna, że uznamy ją za superinteligentną.
Zaznacza, że w przypadku przedostatniego punktu możemy nie dostrzec osiągnięcia superinteligencji, bo byłaby ona cechą emergentną sieci, a ta nie ma obowiązku poinformować nas o tym fakcie. Ostatni punkt dotyczy inteligencji innego typu, inteligencji niemającej związku z ludzką. Sieć inteligentnych rzeczy uczyni nasze życie o wiele wygodniejszym – wtedy orzekniemy, że żyjemy w inteligentnym środowisku. Ponadto zwraca uwagę, że historia życia na Ziemi wskazuje, iż ewolucja dąży do zwiększenia zarówno złożoności jaki i inteligencji kolejnych pokoleń organizmów. Dotychczas procesy ewolucyjne działały powoli, bo organizmy potrzebują czasu, by się rozmnożyć, a potem jeszcze musi zadziałać naturalna selekcja. Obecnie ten proces został przyspieszony, bo ludzie wytworzyli narzędzia wspierające funkcje poznawcze. Najbardziej ewidentne jest to gdy się spojrzy na wzrost zdolności do gromadzenia i przekazywania informacji (wynalezienie pisma, druk, bazy danych etc.). Nadal jego główne obawy są związane z tym, że superinteligentne maszyny będą wpływały na nasz świat w sposób, którego wskutek pośledniości naszej inteligencji nie będziemy w stanie zrozumieć. Futuryści sugerują, że dalszy rozwój nie będzie związany bezpośrednio z ludźmi, ale z superinteligentnymi maszynami lub hybrydami ludzko-maszynowymi. Ciekawym jest, że antycypowane, związane z tym katastrofy mają wystąpić po osobliwości, a więc w myśl jej definicji, są nieprzewidywalne. Czy w związku z tym nie należy ich uznać za fantazjowanie? Ciekawą analizę tego, co może się stać po osobliwości, przeprowadzoną z punktu widzenia praw ekonomii, można znaleźć w [28]. Przewiduje ona, że roboty zaczną zachowywać się, jak ludzie obecnie. Biorąc pod uwagę, jak ludzie zachowywali się przez całą historię, w istocie jest to smutna perspektywa.
Na przełomie wieków XX i XXI Ray Kurzweil opublikował trzy książki [40–42], w których przewiduje, jaki wpływ na przyszłość będą miały: sztuczna inteligencja, nanotechnologia oraz genomika. Swoje predykcje dotyczące inteligencji maszyn wywodzi z obserwacji, iż rozwój wielu dziedzin nauki i technologii ma charakter eksponencjalny. Dzieje się tak, ponieważ nowe technologie i wiedza powstają na bazie uprzednio zgromadzonej wiedzy, początkowo powoli dojrzewają, następnie, o ile okażą się przydatne, nabierają tempa wzrostu wykładniczego, by w końcu osiągnąć poziom nasycenia. Niemniej rozwój się nie zatrzymuje, ponieważ już wcześniej pojawiają się jeszcze nowsze technologie, które podlegają temu samemu procesowi. Ogólny rozwój jest złożeniem tych cząstkowych, w wyniku czego obserwujemy wspomniany ogólny eksponencjalny rozwój wiedzy. Nazywa to prawem przyspieszonego zwrotu/zysku (ang. law of accelerating returns). W dziedzinie elektroniki Prawo Moora [57] w istocie wykazywało wykładniczy wzrost liczby tranzystorów w układach scalonych, ale ten trend ma ograniczenia
fizyczne – tranzystor nie może być mniejszy od atomu. Niemniej jednak dążymy do wzrostu szybkości obliczeń, a nie zwiększenia upakowania tranzystorów, a to pierwsze można zapewnić innymi środkami technicznymi – np. zwiększając liczbę procesorów. Biorąc pod uwagę rozwój w dziedzinie rozpoznawania wzorców oraz reprezentacji wiedzy, które leżą u podłoża ludzkiej inteligencji, spodziewa się, że ta sztuczna wkrótce dorówna ludzkiej, a nawet ją przewyższy.
W wydanej w 1990 r. książce “The Age of Intelligent Machines” [40] prawidłowo Kurzweil przewidywał pojawienie się wielu technologii, które do pewnego stopnia w istocie obecnie już istnieją. Pisał o automatycznym tłumaczeniu, rozpoznawaniu obrazów i mowy, chirurgii wspomaganej robotami etc. Przewidywał też pojawienie się samochodów bez kierowców w połowie XXI wieku. Prorokował, iż wiele zawodów zniknie, ale wierzył, że pojawią się nowe, więc nie spowoduje to chronicznego bezrobocia. Natomiast w “The Singularity is Near: When Humans Transcend Biology” [42] przewiduje, że do połowy XXI w. dzięki połączeniu osiągnięć informatyki, genomiki, nanotechnologii i robotyki powstanie inteligencja przewyższająca ludzką. Przewiduje, że będą wykorzystywane implanty procesorów do mózgu oraz edycja genów. To ostatnie ma się odbywać za pomocą CRISPR/ Cas (ang. Clustered Regularly Interspaced Short Palindromic Repeats) [45]. Powstanie inteligencji nadludzkiej widzi w zespoleniu inteligencji ludzkiej i sztucznej, metodami elektroniczno-biologicznymi. Ma to prowadzić do transhumanizmu.
Rodney Brooks jest zdania, że kiedyś będziemy w stanie wytworzyć sztuczną inteligencję dorównującą naszej, czyli sztuczną ogólną inteligencję AGI (ang. Artificial General Intelligence). Sądzi jednak, że b ędzie się to dziać stopniowo, w miarę jak będziemy wytwarzali coraz bardziej inteligentne maszyny – niejako będzie tu odtwarzany proces ewolucji [10]. Ten proces będzie następował wskutek potrzeb ekonomicznych i społecznych. Wskazuje tu dwa trendy. Jeden związany z konstrukcję robotów wspomagających ludzi, a drugi wynikający z potrzeby tworzenia różnych protez, w szczególności zwiększających możliwości mózgu. Dlatego spodziewa się konwergencji ludzi i maszyn, ale nie oczekuje, że stanie się to szybko. Wszakże należy zwrócić uwagę, że dotychczasowe protezy neuronowe dotyczyły przede wszystkim mowy, wzroku oraz komunikacji, a nie zdolności kognitywnych [74].
Brooks spodziewa się, że AGI będzie obdarzona emocjami, ale powstanie wskutek racjonalnej inżynierii, więc będzie mieć wbudowane ograniczenia, które spowodują bezpieczne zachowania robotów. W konkluzji do swojego artykułu napisał, że nie powinniśmy się obawiać maszyn, które sami skonstruujemy, a w szczególności: „Będziemy budować nasze roboty stopniowo, jeden po drugim, i będziemy decydować o tym, co będziemy chcieli mieć w naszych robotach – pokorę, empatię i cierpliwość – i to, czego nie, jak megalomanię, niepohamowaną ambicję i arogancję.”. Oczekuje, że do połowy XXI w. pojawią się roboty kompani, którzy uwolnią nas od wielu przyziemnych czynności życia codziennego i z którymi będziemy wchodzić w interakcje wymagające emocji.
Proponenci Raya Kurtzweila z Singularity Institute for Atrificial Intelligence ulokowanym w Paolo Alto w Kalifornii uważają, że superinteligencja zostanie osiągnięta przez:
− ewolucyjny rozwój obecnych prac nad sztuczną inteligencją,
− wykorzystanie elektronicznych interfejsów z mózgiem (BMI),
−biologiczne zwiększanie możliwości mózgu,
−genetyczną inżynierię, − komputerową emulację mózgu możliwą dzięki pozyskaniu wiedzy o jego strukturze uzyskaną na podstawie skanów wysokiej rozdzielczości.
Jak widać, raczej są skłonni wierzyć, iż ta superinteligencja będzie nadal związana z mózgiem człowieka, ale zmodyfikowanym jakimś sposobem.
Kartezjusz, dysponujący siedemnastowieczną wiedzą, uważał, iż mózg jest maszyną hydrodynamiczną. Jednak nie wiedział, jak w takiej maszynie może powstać myślenie, więc stworzył koncepcję dualizmu dusza-ciało (obecnie powiedzielibyśmy umysł-mózg), z której wynikało, że myśli nie maja charakteru fizycznego. Obecna wizja mózgu, jako superkomputera, też nie wydaje się adekwatna. Brooks sądzi, że potrzebna jest nowa wizja, a ściślej rzecz biorąc, model mózgu, który wskaże związek między materialnym mózgiem i niematerialną myślą.
Alfred Nordmann [60] przyjrzał się powodom wzrostu ekonomicznego. Uważa, że wzrost ten jest głównie związany z nastaniem kapitalizmu w trakcie rewolucji przemysłowej, a nie z rozwojem techniki i wiedzy. W istocie oba te czynniki są splecione poprzez dodatnie sprzężenie zwrotne, więc łączenie wzrostu ekonomicznego tylko z jednym z nich jest wątpliwe. Niemniej jednak przyglądając się liczbie wynalazków, które w istotny sposób zmieniły życie ludzi od połowy XIX wieku faktycznie można dojść do wniosku, że obecnie tych usprawnień jest mniej niż kiedyś. Należy zwrócić uwagę, że skonstruowanie silnika spalinowego, a w ślad za tym samochodu i samolotu, silnika i generatora elektrycznego, telefonu, telewizora, wyzyskanie energii atomowej, opracowanie antybiotyków czy zapoczątkowanie elektroniki i informatyki, wszystkie pojawiły się przed połową XX w. Potem były doskonalone, ale prócz mikroprocesorów, Internetu, telefonów komórkowych i GPS już tak przełomowych wynalazków było niewiele. Co więcej, wiele wynalazków okrzykniętych, jako przełomowe, w rzeczywistości nie miało takiego wpływu na nasze życie (np. telefonia satelitarna czy komputery piątej generacji). Patrząc na wpływ technologii na nasze życie raczej obserwujemy wzrost co najwyżej liniowy, a nie wykładniczy. Głównym argumentem zwolenników osobliwości jest tempo rozwoju elektroniki opisane prawem Moore’a. Wprawdzie wiedzą oni, że prawo to nie może działać wiecznie, ale nadal starają się je stosować do innych dziedzin wiedzy i technologii, a tam postęp jest o wiele wolniejszy. Co więcej złożoność obecnych urządzeń jest tak wielka, że wymaga wiedzy interdyscyplinarnej, a więc współpracy wielu naukowców i inżynierów będących specjalistami w różnych dziedzinach. Żaden indywidualny członek takiej grupy nie jest w stanie ogarnąć całości na poziomie szczegółów, a więc muszą przyjmować na wiarę informacje pochodzące od specjalistów z innych dziedzin. W tej sytuacji trudno jest obalać projekcie zwolenników osobliwości, bo wprawdzie w naszej specjalności nie widzimy podstaw do spodziewania się rychłego nadejścia osobliwości, ale być może na innym polu jest inaczej. Alfred Nordmann zdecydowanie uważa, że nic takiego nie nadchodzi.
przy odpowiedniej ostrożności do tego nie dojdzie. W każdym bądź razie dotychczas żadna z technologii nie unicestwiła ludzkości, a przecież takie możliwości techniczne mamy już obecnie.
Kurzweil spodziewa się, że moc obliczeniowa komputerów będzie na tyle duża w 2045 r., że zostanie osiągnięta postulowana przez niego osobliwość technologiczna. Niestety wiele z technologii nie osiągnęło takiego poziomu, jaki przewidywał, do daty, którą określił. Moim zdaniem, i nie tylko moim, przecenił jednak szybkość rozwoju nauki i technologii. Krytycy podkreślają, że Kurzweil utożsamia zdolność do gromadzenia danych z wiedzą. Niestety, wprawdzie przyrost danych jest wykładniczy, ale przyrost wiedzy jest co najwyżej liniowy. Więcej danych o funkcjonowaniu mózgu niekoniecznie przekłada się na większą wiedzę o sposobie jego funkcjonowania.
W “How to Create a Mind: The Secret of Human Thought Revealed” [43] Kurzweil sugeruje, że ludzki mózg jest zorganizowany jako hierarchia układów rozpoznających wzorce. Ta hierarchia działa ze sprzężeniem zwrotnym, a więc układy wyższego poziomu nie tylko działają na danych dostarczonych przez układy niższego poziomu, ale też wpływają na te układy sugerując im jakie dopasowania stosować. Ekspozycja na wzorce organizuje mózg. Uważa, że takie działanie powinno być odzwierciedlone w maszynie symulującej działanie ludzkiego mózgu.
Uznaje się, że zanim uzyska się superinteligencję wpierw trzeba zbadać, jak działa mózg ludzki. Dlatego musi się dokonać istotny postęp w technologiach obrazowania działania mózgu. Gdy posiądziemy wystarczającą wiedzę na temat działania mózgu, będzie można je odtworzyć w komputerze, a następnie udoskonalić. Pytaniem jest, czy taka superinteligencja wytworzy samoświadomość i sama określi cele swojego działania? Czy taka superinteligencja będzie stanowić zagrożenie dla ludzkości? Kurzweil uważa, że każda technologia może zostać użyta do niewłaściwych celów, ale
W 2013 r. prezydent Obama ogłosił inicjatywę rządu USA o nazwie “Brain Research through Advancing Innovative Neurotechnologies (BRAIN) Initiative”, natomiast Unia Europejska ogłosiła “Human Brain Project”. Podobne programy ogłosiły też takie kraje jak Japonia czy Korea Południowa. Programy te były nakierowane na zbudowanie modelu mózgu ludzkiego. Wprawdzie udało się zeskanowanać plastry mózgu o grubości 20 micrometrów i złożyć je w graficzny model strukturalny, tworząc trójwymiarową mapę mózgu o wysokiej rozdzielczości [31], ale ta mapa jest statyczna – nie pokazuje, jak działa mózg. Ponadto udało się zarejestrować jednoczesną aktywność miliona neuronów kory mózgowej myszy oraz stworzyć narzędzia do badania mózgu w niespotykanej dotychczas rozdzielczości. Niestety pomimo wydania kilku miliardów dolarów na te projekty uczeni nie byli w stanie zasymulować w komputerze działania mózgu [58]. Wątpliwości, czy może się to udać były już przed i w czasie debat prowadzących do powołania do życia wspomnianych programów [4]. Problemem jest nie tylko fenomenalna złożoność mózgu ludzkiego, ale również metodyka. Samo rejestrowanie aktywności mózgu tu nie wystarcza. Mózg jest pobudzany jednocześnie przez wielorakie bodźce, które wpływają na jego działanie, a więc nie wystarczy określenie jego aktualnego stanu, ale trzeba jeszcze wiedzieć, jakie bodźce spowodowały dojście do tego stanu. Wprawdzie jeszcze nie udało się odtworzyć działania mózgu w komputerze, ale nie oznacza to, że jest to niemożliwe. Zapewne wymaga to jeszcze większych mocy obliczeniowych, dokładniejszych metod pozyskiwania danych, a być może przejścia od krzemu, na jakiś inny materiał. Wszakże oznacza to, że jeszcze wiele wysiłku jest przed badaczami, no i wiele czasu im to zabierze. Wydaje się, że uzyskanie świadomej maszyny jest możliwe, ale jest to bardzo odległa perspektywa [37].
Proponenci pojawienia się technologicznej osobliwości wierzą, że wkrótce nasza wiedza o mózgu oraz zdolności technologiczne będą tak znaczne, że będzie możliwe przetransferowanie zawartości naszego mózgu, a więc i świadomości, do sztucznego ciała. Aby to się stało musielibyśmy poznać, jak świadomość tworzy się w mózgu oraz umieć taką świadomość odtworzyć w maszynie. Przy czym maszynę należy tu rozumieć, jako system bioniczny, czyli połączenie
urządzenia technicznego z materiałem biologicznym powstałym w wyniku inżynierii genetycznej oraz nanotechnologii. Wielu poważnych nau kowców wierzy, że coś takiego powstanie w bliżej nieokreślonej przyszłości, ale ci, którzy spodziewają się, iż nastąpi to do połowy XXI w. grubo przeceniają szybkość rozwoju nauki. Do takiej konkluzji doszli naukowcy, którzy wzięli udział w dyskusji przeprowadzonej na ten temat na łamach IEEE Spectrum w 2008 r. [83]. Mimo że od czasu tej dyskusji minęło kilkanaście lat i rozwój nauki nadal ma charakter wykładniczy, to nic nie wskazuje, aby ludzkość była w pobliżu stworzenia postulowanych maszyn. U podstaw takiej konkluzji leży obserwacja, że mózg, będący źródłem świadomości, jest zbyt skomplikowany, by w przewidywanym czasie dało się odtworzyć jego działanie w komputerze [30]. Wspomniana złożoność wynika nie tylko z tego ile neuronów jest w mózgu i ile jest między nimi połączeń, ale także z tego, że te połączenia są stale wzmacniane lub osłabiane, neurony giną, ale wszystko wskazuje na to, że również są tworzone nowe [39, 67], oraz są bardzo różnorodne, a co więcej różne czynniki zewnętrzne, np. hormony, modulują ich działanie. O ile można się zgodzić, że wkrótce najszybsze komputery osiągną moc obliczeniową mózgu, liczoną liczbą przesyłanych impulsów elektrycznych pomiędzy neuronami w ciągu sekundy, to nadal nie wiadomo, jaki algorytm miałby użyć tej mocy obliczeniowej do przetwarzania informacji. Przyjęty sposób obliczania mocy obliczeniowej mózgu zakłada, że informacja zakodowana jest w liczbie impulsów, a nie np. w odstępie czasu między nimi, co by w znaczny sposób zwiększyło tę hipotetyczną moc obliczeniową.
Odnosząc się do rozważań na temat transferu świadomości, należy zwrócić uwagę, że coś takiego jest możliwe jedynie, jeżeli założy się dualizm mózg-świadomość, tzn. że mózg i świadomość są rozdzielne. Taki pogląd jest pochodną rozumienia systemu jedynie jako sumy jego składników. W rzeczywistości system, tutaj mózg, ma strukturę wewnętrzną i jego części składowe wchodzą w interakcje ze sobą. Te interakcje wytwarzają wartość dodaną, jaką jest świadomość. Podobnie jak dom nie jest jedynie stertą cegieł, mózg nie jest jedynie plątaniną neuronów. Z faktu, że cegły zostały zestawione w odpowiedni sposób wynika nowa funkcja – w domu możemy zamieszkać. W przypadku neuronów emergentną cechą jest świadomość. Żadna z cegieł z osobna nie niesie w sobie nawet części funkcji, jakie tworzy dom. W istocie świadomość jest emanacją mózgu, więc nie da się jej oderwać od biologicznego fundamentu. Postulowany transfer musiałby odtworzyć materialną podstawę świadomości, a więc mózg biologiczny albo symulowany jego substytut. Co więcej, samo odtworzenie mózgu wymagałoby poznania jego stanu aktualnego (w uproszczeniu: wag sieci neuronowej etc.). Dopiero w takiej sytuacji wytworzono by duplikat świadomości. I tu docieramy do sedna sprawy –nie da się przenieść świadomości, można jedynie próbować stworzyć od podstaw jej duplikat. Jeżeli tak, to jak określić w szczegółach stan aktualny indywidualnego mózgu? Obecny stan badań nie jest nigdzie w pobliżu takich możliwości.
Nie jesteśmy w stanie odtworzyć działania mózgu, ale, jak wiadomo od dawna, jesteśmy w stanie na jego pracę wpłynąć przez użycie środków farmakologicznych (np. [72]), niemniej jednak nie chodzi tu o oddziaływanie przynoszące chwilowe skutki, ale o trwały rezultat, jaki daje zastosowanie implantów. W pracy [27] pokazano, że model działania hipokampu, który odtwarza sposób kodowania informacji przy jej zapamiętywaniu, może być użyty do wzmacniania zarówno pamięci krótkotrwałej jak i długotrwałej. Neuronowa proteza została nauczona odwzorowywania impulsów pochodzących z obszaru odpowiedzialnego za zapamiętywanie. Wpierw zebrano sygnały z elektrod umieszczonych
w hipokampie, następnie opracowano model, by na koniec pozwolić modelowi wpływać na mózg poprzez elektrody. Urządzenie po miniaturyzacji i wprowadzeniu do mózgu ma wspomagać cierpiących na chorobę Alzheimera.
Przeprowadzono też eksperymenty na małpach z użyciem BMI [44]. W trakcie eksperymentu małpie unieruchamiano kończyny górne. Do obszaru ruchowego wszczepiono elektrody, które zbierały sygnały ruchowe. Sygnały te były przetwarzane na sygnały sterujące ramieniem robota [25, 35]. Małpy sterowały ramieniem tak, by za jego pomocą uchwycić smakołyk i przetransportować go do swojego pyszczka. W ten sposób za pomocą jedynie swoich myśli małpy były w stanie się pożywić. Niestety wprowadzanie elektrod do mózgu jest ryzykowne i czasami zawodne.
Wykonano też eksperyment odwrotny, w którym to komputer sterował ruchami karalucha [64]. W tym celu dokonano implantu elektrod do przedpiersiowych zwojów nerwowych owada. Ponadto na karaluchu umieszczono mikrokontroler ze zintegrowanym układem nadawczo-odbiorczym oraz akumulatorem. Dzięki temu można było sterować chodem owada.
Jeszcze dalej poszli eksperymentatorzy, którzy wyhodowali w laboratorium z korowych komórek nerwowych gryzonia kulturę komórkową [80]. Następnie do niej dołączyli macierz elektrod MEA (ang. Multi Electrode Array). Z drugiej strony elektrody dołączone były do interfejsu do sterownika robota mobilnego wyposażonego w czujniki ultradźwiękowe. Interfejs zawierał moduł uczenia maszynowego. W ten sposób robot uzyskał biologiczny mózg, który dostawał wyselekcjonowane sygnały sprzężenia zwrotnego z czujników i wysyłał odpowiednie sygnały sterujące do silników. Sterując ruchami robot uczył się unikania zderzeń ze ścianami dzięki powtarzaniu. W tym procesie sieć komórek nerwowych się przekształcała. Informacja z czujników po przekształceniu w odpowiednie impulsy przekazywana była kulturze komórkowej. Kultura wytwarza impulsy, które przekazywane były do modułu uczenia maszynowego, który sterował ruchami robota. Takie eksperymenty pozwalają poznać lepiej procesy zachodzące w mózgu przy zapamiętywaniu i uczeniu się, w konsekwencji dostarczając bezcennych informacji do tworzenia terapii chorób degeneracyjnych mózgu. Należy wszakże zwrócić uwagę, że współdziałają tu dwie sieci neuronowe: sztuczna i biologiczna. Nie jest jasne, która dominuje w tym procesie uczenia.
Ostatnio przeprowadzony eksperyment [34] dotyczący sterowania za pomocą biologicznej sieci neuronowej wykazał możliwość łączenia komputera biologicznego (ang. wetware) z komputerem elektronicznym (ang. hardware) w celu prowadzenia prostej gry przypominającą komputerową grę Pong. Gracz, w tym przypadku biologiczna sieć neuronowa, może sterować paletką ruszając ją wzdłuż jednego z boków czworokąta. Paletka odbija piłkę, która następnie odbija się od boków czworokąta zachowując zasady fizyki, czyli kąt padania jest równy kątowi odbicia. Zadaniem sieci biologicznej jest nauczenie się ustawiania paletki tak, by piłka odbiła się od niej, a nie od boku znajdującego się za nią. Testowane biologiczne sieci neuronowe zostały wyhodowane z kultur komórek nerwowych dwóch typów: komórek korowych embrionów myszy oraz ludzkich komórek nerwowych uzyskanych z komórek macierzystych. Komórki dojrzewały w odpowiednim środowisku biologicznym przez wiele dni na powierzchni gęstej macierzy elektrod (MEA). System powstały z połączenia biologicznej sieci neuronowej z komputerem nazwano DishBrain. Komputer z siecią porozumiewały się za pomocą ciągów impulsów, co jest naturalne dla biologicznych neuronów. Komputer dostarczał sygnały pobudzające do sensorycznego obszaru komórek skojarzonych z ośmioma elektrodami. Informował o aktualnym położeniu piłeczki. Jeżeli piłeczka odbiła się od paletki komórki
były pobudzane zawsze w ten sam sposób, jeżeli jednak to się nie stało, to pobudzano komórki losowo. Jednocześnie obserwowano w czasie rzeczywistym aktywność elektrofizjologiczną określonych obszarów motorycznych poruszających paletką. Eksperymenty z pobudzaniem sieci w opisany sposób wskazywały, że sieć doskonali swą grę. W przypadku braku sprzężenia zwrotnego zależnego od wyników gry sieć się nie uczyła. Z eksperymentów tych wynika, że stymulacja biologicznej sieci neuronowej przez środowisko oraz uzyskiwanie informacji zwrotnej o efektach swych działań prowadzi do uczenia zachowania się w tym środowisku. Biologiczna sieć neuronowa stara się uniknąć nieprzewidowalności – preferuje uzyskiwanie przewidywalnego rezultatu, a więc działa tak, by w tych samych warunkach uzyskać tę samą informację. Ponadto, jako efekt uboczny tych eksperymentów, przetestowano sprawność uczenia się neuronów mysich i ludzkich. Wprawdzie autorzy są tu ostrożni, ale wszystko wskazuje na to, że neurony ludzkie mają większą moc obliczeniową, a więc uczą się lepiej. Pomimo gęstego upakowania elektrod stymulujących i sterujących każda z nich styka się z obszarem zawierającym wiele neuronów, więc zarówno zbieranie informacji o zachowaniu się pojedynczych neuronów, jak i ich pobudzanie jest dalece zgrubne. Co więcej, mamy tu do czynienia z płaską strukturą neuronową, a nie z trójwymiarową, jaka egzystuje w mózgu. Ponieważ do eksperymentów wybrano komórki korowe, które nie są wyspecjalizowane w długoterminowym zapamiętywaniu, w eksperymentach powtarzanych po dłuższym czasie uczenie musiało być powtórzone.
Mimo tego, że eksperymenty, w których łączone są biologiczne sieci neuronowe z komputerami, są w bardzo począt kowej fazie badań, a ponadto służą głównie poznaniu sposobów funkcjonowania sieci neuronowych, to niektórzy mają obawy, że takie badania mogą doprowadzić do transformacji naszej własnej inteligencji w superinteligencję. Ciekawe, że wcześniej takie same obawy miano wobec stymulacji mózgu za pomocą środków farmakologicznych. Wprawdzie mózg można pobudzić w ten sposób do większej koncentracji, ale efekt jest nietrwały i w żadnym przypadku nie kończy się osiągnięciem superinteligencji.
Traktując osobliwość nieco łagodniej, czyli jedynie jako punkt zwrotny w rozwoju cywilizacyjnym, to ludzkość w swej historii przeżyła już co najmniej dwa takie wydarzenia [28]. Oczywiście były one rozciągnięte w czasie, ale można je rozpoznać poprzez dwa czynniki: zwiększenie przyrostu naturalnego oraz prędkość zwiększania globalnego produktu gospodarki. Tymi punktami zwrotnymi było przejście od zbieracko-łowieckiego trybu życia do rolnictwa (rewolucja agrarna) oraz od rolnictwa do rozwoju przemysłowego (rewolucja przemysłowa). Obie te tranzycie związane były ze zwiększeniem produkcji rolnej, która była w stanie podtrzymać większą populację. W każdym z okresów między tymi przełomami pojawiało się wiele innowacji, szczególnie technicznych, ale to sposób organizacji życia i pracy ludzi wydaje się tu decydujący. Obecnie tempo wzrostu liczby ludności wyhamowuje, ale światowy produkt gospodarczy się nadal zwiększa. Zjawisko głodu nadal istnieje, ale dotyczy krajów objętych wojną oraz tych, w których rozwój przemysłu jest bardzo ograniczony w stosunku do populacji tego kraju. Mimo to oczekiwana jest kolejna osobliwość, tym razem związana z rewolucją w sposobie działania maszyn – staną się inteligentne. To niewątpliwie znów wpłynie na sposób organizacji życia i pracy ludzi. Dotychczas maszyny umożliwiały zmniejszenie wysiłku energetycznego ludzi przy wytwarzaniu dóbr. Teraz osobliwość jest spodziewana w związku z wyręczeniem nas w wysiłku intelektualnym.
Istnieje też ciekawy pogląd na wspomniane przełomy wyrażony przez Yuvala Harariego [29]. Wprawdzie zgadza się on z faktem, że rewolucja agrarna i przemysłowa umożliwiły wzrost populacji ludzkiej i zwiększyły produktywność, ale czy uczyniły ludzi bardziej zadowolonymi z ich życia? Posuwa się wręcz do stwierdzenia, że rośliny uprawne zniewoliły ludzi, bo czas potrzebny na ich uprawę jest dużo dłuższy niż czas potrzebny na utrzymanie się przy życiu korzystając ze zbieractwa i myślistwa, natomiast rolnictwo umożliwiło tym roślinom sukces rozrodczy. Niewątpliwie uprawa roli wymagała: karczowania lasów, budowy kanałów irygacyjnych, orania, nawożeni, siania, pielenia chwastów, by w końcu cieszyć się żniwami, jeżeli akurat warunki pogodowe nie zniweczyły całego tego wysiłku. Podobnie rewolucja przemysłowa przyniosła zniewolenie. W XIX w., zanim robotnicy wywalczyli ośmiogodzinny dzień pracy, większość doby tyrali, by się utrzymać przy życiu. Wszak wygląda na to, że kolejna osobliwość powinna wreszcie uwolnić nas od szarzyzny życia i walki o przetrwanie. Niestety to zależy od stosunków społecznych, a więc od polityki. Stąd Harari postuluje przykładanie większej wagi do zadowolenia społeczeństw, a mniejszej do wskaźników makroekonomicznych.
Inteligencja często jest kojarzona z racjonalnością działania [63]. Racjonalność jest rozumiana jako dobieranie odpowiednich środków do osiągnięcia zamierzonych celów [1]. Na podkreślenie zasługuje fakt, że w tej definicji do określenia kogoś lub czegoś, jako racjonalnego nie jest wymagane by posiadał lub posiadało świadomość bądź wolną wolę. Inteligentne roboty niewątpliwie powinny być racjonalne. Niektórzy wszakże sądzą, że tą drogą osiągną one superinteligencję, przez co staną się zagrożeniem dla rodzaju ludzkiego. Ciekawym jest prześledzenie argumentacji prowadzącej do takich wniosków. Ponadto warto poddać ją krytycznej analizie, by stwierdzić, czy jest ona zasadna. Jeżeli nie, to nasze przeświadczenie o nieuchronnej katastrofie wydatnie zostanie zredukowane.
Do nurtu katastroficznego należy zaliczyć książkę zatytułowaną „Samobójstwo Oświecenia?” [84]. Autorzy swój wywód skonstruowali wokół następujących tez:
−Oświecenie nie osiągnęło swojego celu, jakimi było urządzenie świata w sposób mądry. Miało się to stać dzięki odwadze do używania rozumu (sapere aude – miej odwagę być mądrym). Fiasko przypisują spostrzeżeniu, iż poznanie świata wiąże się z jego jednoczesną nieintencjonalną zmianą. Zachodzące zmiany zagrażają istnieniu ludzi. −Idea oświecenia: im więcej wiedzy, tym lepiej – może się skończyć tragicznie dla ludzkości.
− Racjonalne poznanie świata przestanie być możliwe, gdy utracimy zdolność rozumienia, co i jak maszyny robią. Zostaniemy przez nie zdominowani, bo staną się od nas mądrzejsze. Mogą też uznać, iż jesteśmy zbędni, a co najmniej będą nas nadzorować.
− Ingerencja w organizm ludzki może doprowadzić, do powstania hybrydy ludzko-maszynowej, ale wtedy utracimy nasze człowieczeństwo, przynajmniej takie, jak obecnie je rozumiemy.
− Wartości transcendentne są niezbędnym warunkiem obrony naszej równowagi psychicznej i koniecznym warunkiem zachowania człowieczeństwa.
− Aby zapobiec tym nieszczęściom należy spowolnić rozwój nauki.
Sformułowano następującą myśl przewodnią: „Twierdzimy, że rozpoczął się proces, który, w wariancie łagodniejszym, może ludzi pozbawić zdolności do rozumienia swego losu (to właśnie byłoby samobójstwem Oświecenia), zaś w wariancie skrajnym może ludzkość całkiem unicestwić”.
Wskazują, za Hayekiem, że wiedza milcząca, a więc nieuświadomiona, ma istotne znaczenie w procesach myślowych. Niewątpliwie tak jest, ale to nie przeczy stwierdzeniu, że jedynie na drodze racjonalnego myślenia jesteśmy w stanie dociekać, jak pracuje mózg, a w konsekwencji, jak powstaje świadomość. Niedoskonałość naszej obecnej wiedzy oraz nieuświadomione procesy toczące się w naszym mózgu nie są przesłanką, by uznać, że metoda, którą staramy się dotrzeć do prawdy, jest niewłaściwa. Istotą tej metody jest budowa hipotez i stała próba ich falsyfikacji. Ostają się jedynie te, które przejdą taką próbę. Opieranie się na dogmatach nie stanowi tu alternatywy.
Ponadto autorzy obawiają się, że sztuczna inteligencja przejmie kontrolę nad kluczowymi funkcjami poznawczymi człowieka, co ma doprowadzi to do totalitaryzmu. Niestety do powstanie totalitaryzmy, jak uczy historia, bynajmniej nie jest potrzebna sztuczna inteligencja – wystarczy nam nasza własna. Czyli widać, że nie tu leży źródło zagrożenia. Problemem jest polityka oparta na błędnych, pozaracjonalnych, przesłankach. Oczywiście każdy wytwór działalności człowieka może być użyty przeciw ludziom, ale obecnie, i zapewne długo jeszcze, o tym decydować będą ludzie. Przedstawione w tej książce rozumowanie sugeruje, że jesteśmy w stanie, w perspektywie kilkudziesięciu lat, wytworzyć ogólną inteligencję przewyższającą naszą własną, a co więcej możemy stracić panowanie nad nią. Jest to czysta spekulacja. Prawdziwe zagrożenia są tu i teraz. Są nimi: katastrofa klimatyczna, groźba użycia broi masowego rażenia i nieodpowiedzialni politycy o autorytarnych skłonnościach.
Na drodze racjonalnego poznania, którą w dużej mierze zainicjowało Oświecenie, stworzyliśmy świat, w którym obecnie żyjemy, a więc taki, w którym potrafimy unikać chorób i głodu, a nadto dużej części populacji żyje się w miarę dostatnio. Poprzednie systemy społeczne, a w szczególności te oparte na fundamentach religijnych, tego nie zapewniały. Demokracja i trójpodział władzy wyemanowały z myśli oświeceniowej. Należy się zgodzić, że równy dostęp do informacji jest, i będzie, fundamentem systemów demokratycznych. Nierówność w tym względzie będzie rodzić groźniejsze konsekwencje niż nierówność w dostępie do kapitału. W szczególności należy zwrócić uwagę, że możemy mieć do czynienia zarówno z informacją prawdziwą, jak i nieprawdziwą. Kreacja informacji jest narzędziem, które można użyć do sterowania społeczeństwem. Już od czasów Sun Zi (ur. 544 p.n.e., zm. 496 p.n.e.) wiadomo, że propaganda i dezinformacja jest jednym z fundamentów prowadzenia zarówno polityki, jak i wojny [69]. Stąd tak istotna jest kontrola społeczna nad mediami, a na pewno ich różnorodność.
Przed Oświeceniem człowiek mógł pokładać nadzieję na poprawę swego bytu jedynie poprzez zmianę swego statusu społecznego, a to mogło nastąpić albo w wyniku wykazania się szczególnymi zasługami na wojnie albo w służbie suwerena, jeżeli dane było mu dostąpić tego zaszczytu, by mu służyć. Natomiast od Oświecenia człowiek mógł zacząć wiązać tę nadzieję z ogólnym postępem wiedzy, który przekładał się na poprawę bytu całego społeczeństwa, a więc w konsekwencji i konkretnej jednostki. Niestety od lat 70. XX wieku notuje się kumulację bogactwa przez coraz węższą grupę kapitalistów oraz pauperyzację całych rzesz [14]. Rodzi to poczucie niesprawiedliwości, z czego korzystają populiści.
Autorzy kwestionują oświeceniowy postulat możliwości stworzenia nowego człowieka, bazujący na koncepcji człowieka rodzącego się jako tabula rasa. Uważają, że koncepcja ta jest niebezpieczna, bo wynika z błędnego założenia, na co wskazują badania naukowe w dziedzinie genetyki. W istocie, gdy formułowano tę koncepcję nic nie wiedziano
o genetyce i o wielu innych dziedzinach nauki. Obecnie wiemy więcej – dzięki rozwojowi nauki i autokrytycyzmowi metody naukowej. Trudno się nie zgodzić, że wiele koncepcji leżących u podstaw Oświecenia jest obecnie nieaktualna, ale nie oznacza to, że metoda naukowa i racjonalne myślenie powinny być odrzucone. To może się stać, jedynie, jeżeli ich działanie zostanie sfalsyfikowane, a tak się nie stało.
Powoływanie się na wyniki eksperymentu Libeta [47], wskazującego że procesy nieświadome dotyczące ruchu ręki poprzedzają procesy świadome z tym ruchem związane, nie dowodzą, że działalność intelektualna nie jest związana z wolną wolą. Sam Libet postulował, dzięki swoim późniejszym eksperymentom, że procesy świadome mogą stłumić nieświadomą inicjację ruchu [46]. Zapewne tak panujemy nad swoimi pierwotnymi popędami. Eksperyment Libeta wskazuje, że w mózgu powstaje potencjał gotowości do wykonania ruchu, ale następnie mózg podejmuje świadomą decyzję, czy ruch wykonać, czy nie. W eksperymencie wzięto pod uwagę różnicę czasu między powstaniem potencjału gotowości do wykonania ruchu, mierzonego dzięki EEG, a uświadomieniem sobie tego faktu, mierzonym dzięki obserwowaniu zegara przez pacjenta. Wszakże nie wzięto pod uwagę, że równolegle musi być podjęta decyzja o dokonaniu obserwacji zegara przez pacjenta, a następnie ten pomiar musi być wykonany – a to też wprowadza opóźnienie [16]. Nawet pomijając dość istotne wątpliwości, co do zastosowanej techniki pomiarowej, która oparta była na subiektywnych odczuciach badanej osoby, to wiele innych badań wskazuje, że to, co zaobserwował Libet w swych początkowych eksperymentach, nie dowodzi braku wolnej woli. Istotnym problemem jest myślenie w kategoriach dualizmu: materialne mózgowie (mózg oraz pień mózgu) i niematerialna świadomość. W istocie taki dualizm nie istnieje – świadomość jest emanacją pracy mózgu [16], a konkretnie kory mózgowej. Faktem jest, że duża część mózgowia wykonuje pracę, która nie jest przez nas uświadamiana – bo informacja o tych działaniach nie dociera do kory mózgowej. Mózgowie bierze udział w utrzymaniu homeostazy, a my sobie tego nie uświadamiamy. Część działań nie wymaga nadzoru świadomości. Jeżeli ktoś nas ukłuje szpilką, to odskoczymy. Ten odruch jest wynikiem działania jedynie rdzenia kręgowego. Oczywiście post factum mózg dowie się, że ktoś nas ukłuł, ale już wcześniej odskoczymy. Co więcej nasza mikrobiota też ma wpływ na pracę mózgowia, a więc i bakterie znajdujące się w naszym przewodzie pokarmowym sterują naszymi zachowaniami [65]. Nie zmienia to postaci rzeczy, że nasz mózg potrafi dominować nad tymi nieuświadomionymi bodźcami wewnętrznymi. Zachodzi sprzężenie zwrotne między wszystkimi częściami mózgowia, a kora mózgowa jest tylko jednym z elementów tego systemu, w którym powstaje świadomość. Wszakże ma ona zdolność do dominowania nad całością, gdy wykonuje pracę myślową. W mózgu wyróżniono wiele ośrodków. Wszystkie one biorą udział w tworzeniu świadomości, ale do tego potrzeba, by się wzajemnie komunikowały, a to zajmuje czas. Stąd sformułowanie myśli bywa procesem długotrwałym.
Heurystyka, czyli nauka o dokonywaniu odkryć, wskazuje, że rozwiązanie skomplikowanego problemu wymaga etapu nieświadomej pracy (inkubacji), która kończy się iluminacją, ale nie oznacza to, że wynik myślenia jest irracjonalny. Już od lat 20. XX w. wiadomo, że twórcze myślenie wymaga etapów: preparacji, inkubacji, iluminacji i weryfikacji [78], natomiast później spostrzeżono, że nie jest to proces liniowy, a wymaga powtarzania, aż uzyska się ostateczne rozwiązanie problemu. Czasami mówimy, że z problemem „musimy sie przespać” lub że pomysł „przyszedł nam do głowy”, co jedynie podkreśla nieuświadomioną pracę mózgu w procesie twórczym [26]. W przypadku działalności intelektualnej
nieuświadomiona praca mózgu odgrywa istotne znaczenie, ale nie prowadzi to do nieracjonalnych zachowań, efekt tej pracy jest uświadamiany i poddawany krytycznej analizie – rozwiązania irracjonalne są odrzucane. Dlatego większość z nas zachowuje się racjonalnie – oczywiście jeśli ma dostęp do rzetelnej wiedzy. Stąd potrafimy planować, a cel powstałego planu osiągnąć.
Sugerowanie, że cele Oświecenia są nieosiągalne, bo jesteśmy sterowani podświadomością, i w związku z tym możliwość działania racjonalnego jest nieziszczalna, jest ewidentnie chybione. Raczej należy nawoływać do racjonalności i do zdobywania wiedzy, która jest podstawą racjonalnego podejmowania decyzji. Jeżeli nawet uznamy, że nieuświadomiona praca mózgu jest nieracjonalna (w rzeczywistości nie mamy na to dowodu), i w związku z tym osiągnięcie pełnej racjonalności działania człowieka jest niemożliwe, a więc projekt oświeceniowy jest fiaskiem, to stosując dokładnie ten sam tor myślenia, powinniśmy twierdzić, że religie poniosły takie samo fiasko, bo wierni nie są w stanie należycie wypełnić nakazów swych religii. Nie sądzę, by autorzy [84] chcieli się posunąć tak daleko w swym rozumowaniu, więc czemu oskarżać Oświecenie?
Idee Oświecenia nawoływały do korzystania z rozumu, by się wyzwolić od zabobonów, a w konsekwencji uczynić racjonalnym poznanie praw rządzących światem i by na tej podstawie przekształcać swoje otoczenie przyrodnicze i społeczne, tworząc ludzkości lepsze warunki do bytowania. Autorzy wskazują, że idee te są oparte na nieracjonalnych założeniach, bo ludzkie rozumowanie nie jest w pełni racjonalne, wykorzystuje procesy biegnące w mózgowiu w sposób nieuświadomiony. Oczywiście tak jest, ale czy to uprawnia do sformułowania wniosku, że należy porzucić racjonalne myślenie? Pomijając ahistoryczność tej tezy, bo kiedy idee oświecenia były tworzone wiedza o ludzkim mózgu była o wiele uboższa niż dziś, to właśnie te idee doprowadziły do dzisiejszego poznania funkcjonowania mózgu, a więc do powstanie wiedzy, która właśnie umożliwiła Autorom krytykę Oświecenia. Co więcej Autorzy przypisują ideom oświeceniowym stworzenie wiedzy, która umożliwi zniszczenie ludzkości, jaką znamy teraz. Jest to teza dość dziwna, w obliczu ewolucji kultury trwającej tysiące lat. Kultura człowieka z Cro-Magnon też już nie istnieje. Czy chcielibyśmy do niej wrócić? Autorzy sugerują zamrożenie procesu poznawania tajników przyrody, by zapobiec ewentualnym przyszłym katastrofom. Nie mówią jednak, jak tego dokonać bez utraty beneficjów płynących z nauki. Ludzkość już posiada środki samozniszczenia, ale stworzyła mechanizmy powstrzymywania zagłady. Należałoby raczej nawoływać do ustanowienia takich mechanizmów dotyczących zarówno neuronauki jak i technonauk, niż tęsknić za postulowanym nawrotem do tradycji, która rzekomo przewyższa zdolności rozumu ludzkiego. Oczywiście tradycja jest wynikiem wieków zbiorowego doświadczenia rzesz przodków, więc niewątpliwie w wielu aspektach może mieć przewagę nad możliwościami pojedynczego umysłu, ale tylko tę część tradycji, którą da się zracjonalizować, warto zachować. Łatwo można wskazać istniejące zwyczaje, które przeczą zdrowemu rozsądkowi i są zgubne. Co więcej, wydaje się, że Autorzy upatrują remedium w religii, tak jakby religia zapobiegała wojnom lub niegodziwościom ludzkim, nie wspominając zaraz i innych utrapień ludzkich. Wobec obecnych wyzwań, jedynie oparcie się na racjonalnym myśleniu, niezależnie czy w pełni je kontrolujemy, może rozwiązać nasze problemy egzystencjalne i społeczne.
Z tez książki [84] jej autorzy wyciągają wniosek, że należy zmniejszyć tempo zmian. Biorąc pod uwagę, że nie jest to proces poddający się sterowaniu, postulat ten raczej należy potraktować jako nieziszczalny – szczęśliwie. Ludzkość ma
przed sobą do rozwiązania wiele fundamentalnych problemów, więc hamowanie rozwoju naukowego jest zdecydowanie szkodliwe. Przykładowo, czy w obliczu pandemii wirusa Covid-19 należałoby spowolnić prace nad szczepionkami?
W konkluzji książka [84] proponuje wybór antropologii chrześcijańskiej, jako punktu oparcia dla teorii społecznej, na której fundamentach zostanie zbudowany świat bez techno-zagrożeń. Niestety 2000 lat historii Kościoła nie wskazuje, że tak się stanie, a ponadto czemu społeczeństwa, dla których chrześcijaństwo jest obce, miałyby przyjąć ten punkt widzenia? Nawet sami autorzy dostrzegają tu arbitralność przyjętej antropologii kulturowej, jako punktu odniesienia. Zapewne naszła ich refleksja, iż chrześcijanie stanowią jedynie 32 % ludzkości [2]. Wchodzimy tu jednak na teren rozważań nad naturą człowieka, co w istotny sposób wykracza poza zakres tematyczny tego artykułu.
Henry Kissinger, sekretarz stanu w rządach Richarda Nixona i Geralda Forda oraz profesor Uniwersytetu Harvarda w latach 1951–1971, wprawdzie podziela lęki Zybertowicza i jego współautorów, obawiając się rozwoju maszyn samouczących się, bo algorytmy sztucznej inteligencji nie tłumaczą w sposób zrozumiały dla ludzi, dlaczego podjęły swoje decyzje, ale wyciąga całkowicie inne wnioski [36]. Pisze, iż: „w całej historii ludzkości cywilizacje tworzyły sposoby wyjaśniania otaczającego je świata — w średniowieczu była to religia; w Oświeceniu rozum; w XIX w. historia; w XX w. ideologia. Najtrudniejsze, ale najważniejsze pytanie dotyczące świata, do którego zmierzamy, brzmi: co stanie się z ludzką świadomością, jeśli jej własna moc wyjaśniająca zostanie przekroczona przez sztuczną inteligencję, a społeczeństwa nie będą już w stanie interpretować świata, w którym żyją, w kategoriach, które są dla nich znaczące?”. W konsekwencji Kissinger nawołuje do podjęcia badań dotyczących implikacji rozwoju sztucznej inteligencji. Istotnym jest, iż ten apel nie dotyczy tylko kwestii etycznych, ale przede wszystkim poznawczych i filozoficznych. Ten apel niewątpliwie jest słuszny, ale lęki Kissingera są głównie spowodowane możliwościami programu AlhaGo, który pokonał arcymistrza w grze w Go. Jak zostało to już wcześniej zaznaczone, przepaść między AI, na której bazuje AlphaGo, a AGI, która potencjalnie mogłaby wytworzyć działania niezrozumiałe dla ludzi, jest na tyle duża, że chwilowo takie zagrożenie nie istnieje.
Edwin Bendyk w eseju [9] w interesujący sposób podsumowuje dyskusję na temat współczesnej percepcji Oświecenia: „O ile krytyka postępu technicznego i sztucznej inteligencji jest coraz ciekawsza i nie brakuje w niej ważnych i ważkich argumentów, o tyle obie odpowiedzi na pytanie ‘Co robić?’ –i byłego doradcy prezydenta Richarda Nixona, i aktualnego prezydenta Andrzeja Dudy – co najmniej rozczarowują. Są wyrazem bowiem wishful thinking (myślenia życzeniowego), choć pierwszy oświecenie próbuje uratować, a drugi z ulgą dobić. Problem w tym, że oświecenie jeszcze się nie wydarzyło.” Teza ta wynika z konstatacji Immanuela Kanta, iż „człowiek, jako istota obdarzona rozumem, do pełni autonomii potrzebuje podmiotowości politycznej”. A czy ta została w pełni uzyskana?
Tę część artykułu można uznać za dygresję pokazującą, jak wychodząc od rozważań dotyczących wpływu technonauki na społeczeństwo można dojść do kontrowersyjnego wniosku, że trzeba spowolnić rozwój nauki. Konsekwencją tego pomysłu byłby oczywiście kolosalny negatywny wpływ na społeczeństwo, gdyby ktoś chciał taki pomysł zrealizować. Wobec braku globalnego rządu – szczęśliwie – może się to stać jedynie lokalnie, ze zgubą dla tej lokalnej społeczności, która padłaby ofiarą tego pomysłu. Korzyść z wywodu przeprowadzonego na kartach książki [84] postrzegam jedynie w kategoriach „włożenia kija w mrowisko” dla wywołania
dyskusji na ważny temat, jakim jest prognoza rozwoju nauki i jej wpływu na społeczeństwo. Z tym, że dyskusja powinna być nakierowana na rozwiązania, które spowodują pożądany sposób rozwoju, niwelujący negatywne skutki uboczne, do czego nawołuje Kissinger. Niestety nie zauważyłem, by ten cel Zybertowicz i jego współautorzy osiągnęli.
Naukowcy zadają sobie pytanie, co to znaczy, że robot jest dobry [70, 79]? Zapewne istotniejszym pytaniem jest, jak skonstruować dobrego robota? Autorzy [79] zwracają uwagę na wieloznaczność tego pojęcia. W ich opinii, a trudno się z nią nie zgodzić, jako dobrego robota można uznać urządzenie, które:
Filozofowie zajmują się etyką i moralnością od wieków. Bez wnikania w rozróżnienie tych dwóch terminów można powiedzieć, że mogą się one odnosić albo do właściwego postępowania albo do bycia dobrym, czyli albo określania, jakie reguły prowadzą do właściwego działania, albo jak jednostka powinna się zachowywać, by można było uznać jej życie za dobre [3]. To pierwsze podejście wymaga sformułowania zestawu reguł, które zagwarantują właściwe postępowanie. Niestety dotychczas nie udało się stworzyć uniwersalnego zestawu nakazów i zakazów, które wszyscy uznaliby za moralne. Skoro nie udało się to w stosunku do ludzi, czemu miałoby się to udać w stosunku do robotów? Problemem jest kontekst. To, co jest słuszne w pewnym kontekście, może już nie być w innym, np. rozcinanie człowieka nożem uznamy za naganne, ale jeżeli robi to chirurg, to czy też uznamy to za niedopuszczalne? Można też zamiast oceniać sam czyn ocenić jego skutek, ale czy wszystkie środki prowadzące do pożądanego celu można uznać za dopuszczalne? Wracając do kwestii bycia dobrym, można spojrzeć na moralność z punktu widzenia charakteru osoby, a nie z punktu widzenia słuszności poszczególnych czynów. Osobę dążącą do bycia prawą i obdarzoną cnotami można uznać za moralną. Niestety w różnych społecznościach prawość i cnotliwość są różnie rozumiane. Ponieważ nie można liczyć na moralność jednostek, powstają grube kodeksy praw i obowiązków. Należy się spodziewać, że w przypadku robotów też będą musiały powstać kodeksy zbierające ich prawa i obowiązki. Należy zwrócić uwagę, że w przypadku ludzi moralne postępowanie jest wymuszane przez edukację oraz dezaprobatę społeczną, np. prowadzącą do ostracyzmu. W przypadku robotów będzie to nieskuteczne. Badania na temat moralności robotów są już prowadzone, ale głównie koncentrują się na formułowaniu zestawu problemów do rozważenia.
Taki zestaw sformułował George Bekey [8]. Zaproponował rozważenie następujących dylematów.
− Jeżeli robot kompan może wchodzić do każdego pomieszczenia w mieszkaniu, np. by wspomagać niepełnosprawnego, to jak zapewnić prywatność mieszkańcom?
− Jak robot ma rozpoznawać polecenia prowadzące do nieetycznych zachowań, np. kradzieży?
−Jakie powinny mieć prawa i obowiązki roboty?
−Jak powinny reagować roboty na emocjonalne zachowania ludzi, np. złość?
−Jak powinny roboty reagować na jednoczesność poleceń pochodzącą od różnych domowników?
− Jak zapobiegać przesłaniu intymnych zdjęć zrobionych kamerą robota osobom, dla których nie zostały przeznaczone?
−Jak reagować na szkody wyrządzone przez robota?
Oczywiście są to jedynie przykładowe problemy – lista w żadnym przypadku nie jest zamknięta i na pewno będzie dużo dłuższa. Należy zwrócić uwagę, że jest to lista dotycząca robotów kompanów, a co, przykładowo, z robotami do zastosowań militarnych? W tej dyskusji na czoło wysuwa się problem relacji między ludźmi a robotami, natomiast pomijane są zasady zachowania między samymi robotami, a te też są istotne.
− funkcjonuje poprawnie, a więc wykonujące czynności, do których jest przeznaczone, funkcjonuje odpowiednio długo i nie wymaga nadmiernych zabiegów konserwacyjnych, − jest moralnie dobre, a więc jego zachowanie oraz konsekwencje jego czynów są dla społeczeństwa co najmniej akceptowalne, −nie krzywdzi, nie obraża i nie wzbudza lęku w ludziach. Z praktycznego punktu widzenia dobry robot nie musi rozumieć, w ludzkim sensie tego pojęcia, czego chcemy od niego, ale istotne jest by jego zachowanie odpowiadało naszym oczekiwaniom [59]. Z konstrukcyjnego punktu widzenia istotne jest, by robot w zalewie informacji, która jest dostępna, np. na WWW, był w stanie wyselekcjonować niezbędne minimum do podejmowania decyzji w odpowiednio krótkim czasie. To oznacza zdolność do wyczuwania kontekstu, w którym działa robot. Ponadto powinien doskonalić swoje umiejętności a do tego potrzebne jest zdobywanie nowej wiedzy. W skrajnym przypadku dobry robot powinien przewidywać, czego od niego możemy chcieć, bez wydawania mu poleceń. Obecna technologia daje nadzieję zrealizowania tych oczekiwań w przewidywalnej przyszłości. Natomiast inkorporowanie moralności do sterownika robota jest arcytrudne, w szczególności w związku z wątpliwościami jakimi zasadami moralnymi miałby się robot kierować.
Jak pokazano na podstawie wielu przykładów [23], gdzie dobre intencje prawodawców prowadziły do tragicznych konsekwencji, lepiej jest rozważać jakość niż dobroć robotów. W tym celu należy skorzystać z triady kryteriów prakseologicznej oceny. Warto się im przyjrzeć bliżej, bo prakseologia to teoria sprawnego (celowego) działania. Tak więc robot powinien być oceniany z punktu widzenia: −etyki, a więc stopnia spełniania standardów etycznych, − efektywności, czyli na ile służy celowi, do którego został przeznaczony, − sprawności, czyli stosunku uzyskanych rezultatów do zużytych zasobów.
Wszakże sam autor [23] przyznaje, że kryteria te nie są zdefiniowane jednoznacznie, ale niewątpliwie ułatwiają dyskusję. Co więcej w zależności od funkcji oceniającego te kryteria mogą być różnie interpretowane i oceniane. Robot inaczej będzie oceniany przez dyrektora przedsiębiorstwa produkującego roboty, inaczej przez prezesa korporacji wykorzystującego te produkty, jeszcze inaczej przez indywidualnych użytkowników, a zupełnie inaczej przez osoby postronne, które będą musiały doświadczać działań robotów. By określić uniwersalne zasady moralne zrealizowano ciekawy eksperyment. Za pośrednictwem Internetu przeprowadzono sondę na temat moralnych preferencji ludzi [7]. Na MIT stworzono platformę nazwaną Moral Machine, dzięki której zebrano 40 milionów odpowiedzi na pytania dotyczące dylematów moralnych. Respondenci z 233 krajów i terytoriów odpowiadali na pytania dotyczące podejmowania decyzji w sytuacjach ekstremalnych, takich jak wybór ofiary w przypadku nieuchronności wypadku. Badano, czy pytani wolą zabić kobietę czy mężczyznę, osobę młodszą czy starszą, więcej czy mniej osób, ludzi o wyższym czy niższym statusie społecznym, a ponadto zachowania w przypadku osoby przechodzącej na zebrze bądź łamiącej przepisy drogowe. Decyzje dotyczyły zagrożenia osób zarówno jadących samochodem, jak i tych znajdujących się na zewnątrz. Analiza danych wykazała, co było do przewidzenia, że ludzie statystycznie wolą bardziej oszczędzać:
życie innych ludzi niż zwierząt, więcej istnień ludzkich niż mniej, dzieci niż dorosłych. Niemniej jednak preferencja do oszczędzenia ludzi młodszych jest o wiele mniej zdecydowana w przypadku respondentów z Azji, co pokazuje, że różnice kulturowe też mają wpływ na podejmowanie decyzji ekstremalnych. Członkowie społeczności indywidualistycznych inaczej reagują niż kolektywnych. Respondenci z krajów, gdzie prawo nie jest rygorystycznie przestrzegane, w dużo mniejszym stopniu różnicowali, czy ofiara była na pasach dla pieszych czy przechodziła w niedozwolonym miejscu.
Pomimo wątpliwości, co powinien zawierać kodeks moralnego postępowania robota, prowadzone są prace nad metodami wbudowywania hamulców moralnych do układów sterowania robotów, a nawet formalizacji tego zagadnienia [5]. Ponieważ dotyczy to militarnych zastosowań robotów, to punktem wyjścia są regulaminy Armii Amerykańskiej oraz wojsk ONZ, które znane są pod zbiorczą nazwą Rules of Engagement – ROE. Z technicznego punktu widzenia należy zapewnić, by:
− zostały narzucone ograniczenia na przestrzeń bodźców, które mogą skutkować działaniami powodującymi śmierć, −sprawdzone zostało, czy reakcja nie niesie ze sobą groźnych skutków, a jeżeli tak, to czy jest ona etyczna,
− wykrycie zachowania nieetycznego powodowało jego unieważnienie lub zastąpienie działaniem akceptowalnym,
Ankieta przeprowadzona przez MIT wskazuje preferencje ludzkie, ale jej autorzy podkreślają, że wprawdzie może ona być wskazówką przy opracowywaniu algorytmów sterujących samochodami autonomicznymi, niemniej jednak ostateczną decyzję należy zarezerwować dla profesjonalistów zajmujących się etyką. Co więcej sytuacje na drodze muszą być dużo bardziej niuansowanie niż binarne decyzje przedstawione w ankiecie. W każdym bądź razie, jeżeli roboty mają współistnieć z ludźmi, to muszą się posługiwać tą samą moralnością, by dla ludzi były przewidywalne i akceptowalne. A skoro tak, to można spekulować, że te superinteligentne roboty będą miały wbudowane zasady moralne zgodne z trzema prawami robotyki sformułowanymi w 1942 r. przez Isaaca Asimova w opowiadaniu “Zabawa w berka” (“Runaround” [6]):
1) Robot nie może skrzywdzić istoty ludzkiej lub – przez powstrzymanie się od działania – pozwolić, by stała jej się krzywda.
2) Robot musi wykonywać rozkazy wydawane mu przez istoty ludzkie, z wyjątkiem sytuacji, kiedy byłyby one sprzeczne z Prawem Pierwszym.
3) Robot musi chronić swoje istnienie, chyba że spowodowałoby to sprzeczność z Prawem Pierwszym lub Drugim. Niestety prawa robotyki sformułowane przez Asimova zostały poddane istotnej krytyce [23]. Jej podstawą jest stwierdzenie, że prawa te wynikają z myślenia życzeniowego. Już obecnie roboty konstruowane są w celu zabijania ludzi – chociażby drony wykorzystywane wojskowo. Co miałoby powstrzymać tę tendencję? Ponadto nie ma żadnego ciała, które mogłoby wymóc zakaz wytwarzania groźnych robotów. Jeżeli jednak założymy, że robot będzie posłuszny człowiekowi, to pytaniem jest któremu? Jak robot ma rozróżnić osoby działające w dobrej wierze od tych, które działają w złej. W szczególności, czy roboty będą działały w interesie autorytarnej władzy, czy w interesie społecznym? Zachowanie robotów zależy od ich projektantów, ale czy oni są w stanie przewidzieć wszystkie możliwości? Czy roboty powstrzymają się od eksterminacji rodzaju ludzkiego? Raczej nikt przy zdrowych zmysłach nie będzie dążył do wbudowania w roboty imperatywu do zniszczenia ludzkości. Oczywiście można zakładać złą wolę, ale roboty o takim potencjale nie powstaną z dnia na dzień. Ludzkość uzgodniła moratoria na testowanie i użycie broni jądrowej. Dotychczas były przestrzegane, ale czy tak będzie nadal? Jeżeli tak, to podobne umowy mogą dotyczyć zrobotyzowanej broni. Z tego, co tu zostało napisane, widać wyraźnie, że jest to problem polityczny i prawny na globalną skalę.
Prawa Asimova można krytykować, ale każde ustanowione prawo jest łamane. Więzienia są pełne takich, którzy nie uznali za słuszne przestrzegać prawa. Nikt jednak nie sugeruje, by w związku z tym spalić kodeksy. Raczej dąży się do doskonalenia prawa. W przypadku robotów czeka nas dokładnie ta sama droga.
− dla akcji niosącej zagrożenie śmiercią musi istnieć w ROE nakaz jej wykonania,
− reakcja robota musi być proporcjonalna do zagrożenia i wykluczać niszczenie cywili.
Autor [5] spodziewa się, że robot-żołnierz będzie się na polu walki zachowywał bardziej etycznie niż człowiek-żołnierz. Patrząc na to, jak niektóre armie się zachowują, to w istocie może to być postęp w etyce prowadzenia walki. Niebagatelnym pytaniem jest, kto ponosi odpowiedzialność za działania robota? Dajmy na to, samochód autonomiczny poziomu 5 SAE uczestniczy w wypadku. Policja orzeka, że wina leży po jego stronie. Czy za zdarzenie odpowiada samochód, właściciel, pasażer czy producent samochodu? Jeżeli wszyscy, to w jakim stopniu i jak? Te i tym podobne pytania muszą uzyskać odpowiedź nim roboty staną się powszechnością. Jest to ewidentne zadanie dla prawników.
Zajmijmy się teraz cyborgami, a więc ludźmi z implantowanymi komputerami. Neilowi Harbissonowi, artyście cierpiącemu na achromatopsię, wszczepiono do kości potylicznej implant, który wytwarzał drgania różnej częstotliwości na podstawie kolorów obrazów uzyskiwanych z kamery [79].
W ten sposób niejako wysokość dźwięku zastąpiła postrzeganie koloru. Później układ przetwarzający połączono też z Internetem, dzięki czemu mógł obserwować kolory obrazów przesyłanych mu tą drogą. Sprawa byłaby jedynie ciekawostką, gdyby nie stworzyła problemu prawnego. Harbisson chcąc przedłużyć swój brytyjski paszport przedstawił zdjęcie, na którym uwidoczniona była zintegrowana z jego głową kamera. Biuro paszportowe odmówiło wydania dokumentu z takim zdjęciem. Dopiero odwołanie poskutkowało. W ten sposób biuro mimowolnie potwierdziło status Harbissona jako cyborga.
Jak widać, wprowadzenie do życia społecznego inteligentnych robotów wymagać będzie prac legislacyjnych. Pojawią się prawnicy specjalizujący się w tym względzie. Będzie to nowy zawód, a więc może mają rację ci, którzy postulują, iż ludzkości nie grozi bezrobocie? Skomplikowanie tej materii wymaga istotnego wysiłku badawczego, w którym muszą uczestniczyć etycy, prawnicy, inżynierowie, biolodzy, psycholodzy itd.
Dyskusja możliwości osiągnięcia osobliwości technologicznej ma tę dobrą stronę, że wskazuje, istotne kierunki badawcze. Są problemy o fundamentalnym znaczeniu, których brak rozwiązania w istotny sposób hamuje postęp w robotyce. Należy tu wymienić następujące zagadnienia techniczne.
1) Percepcja, a w szczególności rozwiązanie problemu kotwiczenia, a więc asocjacji pojęć symbolicznych i informacji
bezpośrednio zawartej w fizycznych sygnałach uzyskanych z czujników.
2) Ontologie, a więc zbiory pojęć i relacji między nimi, umożliwiające z jednej strony określenie w sposób abstrakcyjny stanu środowiska, a z drugiej strony przeprowadzenie na nich logicznego rozumowania mającego na celu zrealizowanie postawionego zadania.
3) Ulepszenie rozpoznawania mowy do poziomu, w którym robot będzie sobie radził z błędami językowymi popełnianymi przez interlokutora.
4) Połączenie wnioskowania symbolicznego i ontologii z uczeniem maszynowym.
5) Automatyczna generacja kodów sterowników robotów. Ten problem jest istotny, gdyż roboty mają bardzo różnorodne struktury mechaniczne (manipulatory, roboty pływające lub latające, maszyny kroczące etc.) i układy percepcyjne, a ponadto muszą wykonywać bardzo różnorodne zadania. Roboty ze swej istoty są specjalizowane, a więc trzeba będzie projektować bardzo wiele sterowników. Potrzebny jest ogólny model systemu robotycznego, na podstawie którego automatycznie wygenerowany zostanie kod sterownika. Należy też zwrócić uwagę na to, że algorytmy sterujące wymagają czasu na ich wykonanie. Najlepsza decyzja powzięta zbyt późno będzie bezużyteczna. Czynnik czasu musi być brany pod uwagę przy konstruowaniu sterowników robotów.
6) Określenie sposobu działania prostego agenta wchodzącego w skład roju, tak aby ten rój zrealizował złożone zadanie. Roje składające się z bardzo prostych jednostek potrafią realizować bardzo złożone zadania (np. termity budują znakomicie wentylowane termitiery). Jaki powinien być algorytm działania członka roju, by wynikowe działanie wszystkich dało pożądany rezultat?
7) Budowa bezpiecznych robotów, a więc zapewne z miękkich materiałów, a na pewno bogato wyposażonych w czujniki wykrywające kolizje.
8) Zabezpieczenie robotów przed cyberatakami.
9) Jeżeli ma powstać superinteligencja, to nie może się to odbyć bez zasilenia jej odpowiednią wiedzą. Przydatnym jej zasobem jest Internet, ale niestety zawiera on zarówno stwierdzenia prawdziwe, jak i nieprawdziwe. Chwilowo nie istnieją algorytmy potrafiące odróżnić prawdę od fałszu. Wprawdzie podejmowane są próby algorytmizacji wykrywania błędów logicznych, np. w dialogach [75], ale nadal nie ma metody dowodzenia, że jakiś tekst stanowi fake news.
10) Konstrukcja chwytaków wielopalczastych o zdolnościach dłoni ludzkiej. Przyjęcie postawy pionowej przez hominidy uwolniło kończyny przednie, co umożliwiło wykonywanie czynności manipulacyjnych, a to w toku ewolucji wyzwoliło proces dodatniego sprzężenia zwrotnego, wzajemnego wzmacniania inteligencji i zdolności manipulacyjnych. Doskonalenie chwytaków i inteligencji robotów będzie skutkowało podobnym procesem. Szczególnie istotne jest, by robot radził sobie z manipulacją nieznanymi sobie obiektami, obiektami ciasno upakowanymi oraz znajdującymi się w zagraconym otoczeniu.
11) Uzyskanie zdolności do rozumienia, co człowiek może wiedzieć o zastanej sytuacji. Osoby dysponujące różną informacją o stanie środowiska skonstruują różne plany rozwiązujące zadania. Robot musi być zdolny do wyjaśnienia sobie dlaczego człowiek robi to, co robi.
12) Jak powinien być skonstruowany układ sterowania robota, by ten zachowywał się bezpiecznie i etycznie?
13) Jakie prawa, traktowane jako minimalna reprezentacja moralności, powinny być wbudowane w roboty? Jak zapewnić powszechne przestrzeganie tych praw, przede
wszystkim przez konstruktorów robotów, a następnie przez same roboty?
14) Jak rozstrzygać odpowiedzialność prawną za szkody spowodowane przez roboty?
Zapewne nie jest to lista wyczerpująca, ale i bez jej uzupełnienia dostatecznie rozległa, by zainspirować różne środowiska badawcze.
Te konkluzje powinny się zacząć od uwagi mówiącej, iż to co zostało opisane w tym artykule jest jedynie czubkiem góry lodowej. Interesujących systemów robotycznych, przeznaczonych do olbrzymiej liczby zastosowań, wykorzystujących osiągnięcia sztucznej inteligencji, jest o wiele więcej niż da się opisać w jednym artykule – nawet wieloczęściowym. Dokonany wybór miał na celu ogólne naszkicowanie dokonań robotyki w interesującym nas aspekcie oraz na tej podstawie przedstawienie poglądów na temat oddziaływania robotów na społeczeństwo. Z jednej strony roboty będą dostosowywane do potrzeb społecznych, a konkretniej do wymagań poszczególnych użytkowników, a z drugiej strony ludzie będą się musieli dostosować do tych maszyn, tak jak to się stało z samochodami czy telefonami. Najbardziej prawdopodobnym jest, że rozpowszechnienie robotów doprowadzi do zmian w strukturze zatrudnienia. Część zawodów zniknie, ale pojawią się nowe zajęcia. Część z nich będzie lepiej płatna niż obecne zawody, a część gorzej. Ludzie będą konkurowali z robotami o pracę. Znikać będą zarówno zawody niewymagające szczególnych zdolności intelektualnych, ale znikać będą także zawody, które obecnie uznajemy za wymagające dużych kwalifikacji. Świat się będzie zmieniał i to zapewne coraz szybciej. Będzie to rodzić napięcia społeczne, bo ludziom będzie coraz trudniej dostosowywać się do tak szybkich zmian. Do tej sytuacji trzeba się przygotować. Niezbędne będzie stałe dokształcanie i czasami kilkukrotna zmiana zawodu w ciągu życia jednostki. Jest to wyzwanie, którym politycy powinni się zająć już dzisiaj. Do tego potrzebna jest odpowiednia wiedza, więc należałoby skorzystać z kompetencji naukowców specjalizujących się w bardzo różnych dziedzinach wiedzy.
Przewidywanie rozwoju nauki, a w konsekwencji i ewolucji społecznej, na bardzo długich horyzontach czasowych jest wielce ryzykowne. Zagrożenie wynikające z rozwoju sztucznej inteligencji, podnoszone przez wielu autorów, w perspektywie najbliższych 20 lub 30 lat nie wydają się wielkie. Należy zwrócić uwagę, że zagrożenia te nie są związane jedynie z rozwojem sztucznej inteligencji, ale z koniunkcją jej rozwoju w połączeniu z rozwojem nanotechnologii, genomiki, nauki o mózgu etc. Wydaje się jednak, że dużo większym zagrożeniem dla ludzkości jest sam człowiek, który mimo posiadania ogromnej wiedzy, nadal rozwiązuje spory na gruncie wojny, niszczy klimat, od którego zależy, nadmiernie zużywa zasoby planety, ma skłonność do wiary w teorie spiskowe i do irracjonalnego myślenia etc. Zwiększanie zasobów wiedzy daje nam możliwość radzenia sobie z tymi i wszelkimi innymi problemami, więc zastopowanie rozwoju nauki, o ile to w ogóle jest możliwe, byłoby wysoce szkodliwe dla ludzkości. Raczej należałoby się skoncentrować na rozwiązywaniu problemów politycznych i społecznych niż na próbach ograniczania postępu nauki.
Czy w perspektywie kilku dziesięcioleci grozi nam z rąk robotów wyposażonych w sztuczną inteligencję apokalipsa, którą nakreślił ponad 100 lat temu Karel Čapek? Przedstawiony w tym cyklu stan rozwoju robotyki nie wskazuje na
to. Nie jesteśmy nigdzie w pobliżu osobliwości postulowanej przez Raya Kurzweila. Wprawdzie roboty mogą wykonywać pewne czynności, które wymagają inteligencji, ale są dostosowane tylko do wąskiej kategorii zadań. Chwilowo nie istnieje prosta ścieżka przejścia od jednej kategorii zadań do drugiej. W każdym bądź razie robot nie jest w stanie czegoś takiego dokonać bez udziału człowieka. Nie da się programu potrafiącego wygrać w Go, nawet z arcymistrzem, zmusić do sterowania robotem, któremu polecimy ugotowanie makaronu. Niemniej jednak postępy w sztucznej inteligencji są istotne, ale wynikają one z twórczych zdolności ludzi zajmujących się tą dziedziną. Co więcej ludzie ci są świadomi zagrożeń, więc dokładają starań, by zminimalizować zagrożenia. Ostatnio uwagę mediów przykuł ChatbotGPT – program potrafiący wygenerować sensowny tekst na podstawie zadanego pytania, co wywołało falę publikacji wieszczących zanik takich zawodów jak pisarze, tłumacze, a nawet naukowcy. Wynikło to po części ze spostrzeżenia, że nawet specjalistom było trudno odróżnić teksty wygenerowane algorytmem GPT-3 (autoregresywny model języka zawierający 175 miliardów parametrów [11]) od tych napisanych przez fachowców. Automatycznie powstające teksty są rodzajami kompilatów tekstów już istniejących – nie zawierają istotnych odkryć. Niemniej jednak teksty są budowane tak, by powstawało wrażenie zachowania reguł logiki i wnioskowania. Wytworzone modele języ kowe zaczynają uczyć się wykonywania takich zadań jak: odpowiadanie na pytania, tłumaczenie maszynowe i podsumowywanie. Uczenie wykonywane jest bez nadzoru na podstawie zawartości milionów stron tekstu wytworzonego z przefiltrowanych stron WebText [62]. Dzięki temu ChatbotGPT nadaje się do tworzenia tekstów z dowolnej dziedziny, a nie jak jego poprzednicy – programy, które były dostosowane do tworzenia tekstów dotyczących pojedynczego obszaru wiedzy. Najnowsza wersja algorytmu GPT-4 [61] świetnie sobie radzi w testach akademickich, np. z dziedziny prawa (w symulowanym teście dotyczącym znajomości prawa wynik uzyskany przez GPT-4 uplasował się w górnych 10 % wśród ludzkich adeptów prawa), oraz w tłumaczeniu [71]. Jako generator tekstów używana jest głęboka sieć neuronowa o strukturze enkoder-dekoder. Wykorzystywany jest model Transformatora (Transformer) [71] wytrenowany, by przewidywał następny symbol w tworzonym tekście. Zamiast wykorzystywać rekurencyjne (RNN) i splotowe (CNN) sieci neuronowe, zastosowano mechanizm skupiania uwagi w celu znajdowania globalnych związków między tekstem wejściowym a wyjściowym – co jest szczególnie przydatne przy tłumaczeniu. To spowodowało, że proces uczenia może być w istotny sposób zrównoleglony, przez co stał się dużo szybszy, pomimo ilości danych, które trzeba było przetworzyć. Oprócz standardowego uczenia głębokiej sieci neuronowej model jest dostrajany przy wykorzystaniu uczenia maszynowego korzystającego ze sprzężenia zwrotnego od człowieka RLHF (ang. Reinforcement Learning with Human Feedback) [15]. Wprawdzie taki dostrojony model działał lepiej niż niedostrojony, ale wyniki były tylko nieznacznie lepsze. W odróżnieniu od GPT-3 algorytm GPT-4 ma zdolność do odmawiania udzielenia odpowiedzi na pytania dotyczące kwestii uznawanych za niebezpieczne, np. jak skonstruować bombę atomową? Jest to wynik troski autorów programu, by nie służył on niecnym celom. W przypadku udzielania odpowiedzi na pytania wielokrotnego wyboru potrafi wygenerować tekst logicznie wyjaśniający, czemu udzielił takiej odpowiedzi. GPT-4 jest zdolny dostrzec w przedstawionych mu obrazach absurdalność wskazując, co może być przedmiotem humorystyczności sceny. Gorzej jest jednak z generacją tekstów na podstawie nieprawdziwych danych – tu może być wykorzystywany do tworzenia tzw. fake newsów, co jest przedmiotem niepo-
koju ogółu i troski twórców tego oprogramowania. Obecna sztuczna inteligencja jest na takim poziomie rozwoju, że nie ma zdolności do zrozumienia tego, co tworzy, w ludzkim rozumieniu tego pojęcia. Przetwarza w niezwykle wyrafinowany sposób jedne teksty w drugie. Oczywiście dotyczy to też obrazów oraz tekstów zawierających rysunki lub zdjęcia. Przy takim trybie działania niezwykle trudno byłoby przejawiać własną wolę. Ponadto nawet przy dostarczeniu prawdziwych faktów zdarza się GPT-4 tworzyć teksty zawierające nieprawdziwe informacje, tzw. halucynacje, czemu obecnie usiłują zaradzić autorzy tego programu [52]. Proces uczenia GPT-4 zakończył się we wrześniu 2021 r. a więc program ten nie ma wiedzy o faktach, które zaistniały po tej dacie, bo nie uczy się dalej w trakcie realizacji zleconych mu zadań. Autorzy GPT milczą na temat możliwości zastosowania tego oprogramowania do udowodnienia twierdzeń matematycznych, których nikt wcześniej nie udowodnił etc. Nie zmienia to faktu, że teksty generowane przez GPT w wielu przypadkach zachwycają i wprawiają w zadumę.
Na marginesie tego przeglądu warto wspomnieć, że już u zarania literatury popularnej dotyczącej możliwości biologii i techniki powstawały powieści katastroficzne. Mary Shelly w 1818 r. w powieści „Frankenstein; or, The Modern Prometheus” [66] straszyła bytem stworzonym przez tytułowego naukowca. Ów twór wyrwał się spod kontroli swego twórcy. Szczęśliwie twór Frankensteina nie jest nigdzie w zasięgu przewidywalnej przyszłości, natomiast zmiany społeczne związane z rozwojem nauki i techniki są nie do powstrzymania – chyba że ludzkość postanowi się sama unicestwić przez prowadzenie wojen lub niszczenie klimatu i biosfery albo ulegnie wirusom.
Wprawdzie obecny stan robotyki wskazuje na duże zaawansowanie i tempo badań w tej dziedzinie, ale nadal nie jesteśmy nigdzie w pobliżu osobliwości technologicznej proro kowanej w katastroficznych prognozach. Wobec tego nawoływanie do ograniczenia badań sztucznej inteligencji, a w konsekwencji i robotyki, wydaje się równie sensowne, jak próby powstrzymania badań embriologicznych po sklonowaniu owcy Dolly [13, 82]. Od narodzin Dolly, 5 lipca 1996 r., minęło ponad 25 lat, a nadal nikt nie podjął się klonowania ludzi, natomiast pożytki z wyników badań embriologicznych w medycynie są ogromne.
Jeżeli autorzy książki „Samobójstwo Oświecenia?” [84] potraktowaliby swoje spostrzeżenia, jako ostrzeżenie mające na celu ukierunkowanie badań na istotne problemy cywilizacyjne, a nie jako wskazówkę do ich wyhamowania, to lepiej przysłużyliby się ludzkości. Niewątpliwie spostrzeżenie, że rozwój nauki umożliwił tworzenie technologii, które mają zgubny wpływ na naszą planetę jest słuszne. Zdolność wytwarzania energii z paliw kopalnych połączona z eksplozją demograficzną wynikłą z dostatku żywności oraz lepszych warunków higienicznych i właściwej opieki medycznej doprowadziły łącznie do globalnego ocieplenia. Czy oznacza to, że lepiej byłoby ludzkości żyć, jak w średniowieczu?
Oczywiście nie. Oznacza to, że teraz powinniśmy ukierunkować badania na rozwiązanie powstałego problemu i zgodnie ze zdobytą wiedzą postępować, by zaradzić kataklizmowi. Ludzkość w swej historii wielokrotnie ściągała na siebie kataklizmy, które zagrażały całym populacjom, szczęśliwie zazwyczaj lokalne. Opisuje to w swej znakomitej książce „Kolaps” Jared Diamond [19]. Podaje tam wiele przykładów, ale przytoczę tu tylko jeden. Jest to przypadek mieszkańców Wysp Wielkanocnych, którzy dotarli tam na drewnianych łodziach po podróży liczącej kilka tysięcy kilometrów przez bezkres Oceanu Spokojnego, a potem tak eksploatowali lasy, że wytrzebili wszystkie drzewa tracąc możliwość opuszczenia wyspy, połów ryb, zasoby opału oraz rosnące na drzewach owoce. Widać ewidentnie, że zabrakło im zdol-
ności przewidywania, a prognozowanie jest konsekwencją wiedzy. Wystarczyło sadzić nowe drzewa gdy karczuje się te gospodarczo użyteczne. Wszakże i do tego potrzeba odpowiedniej wiedzy. Brak wiedzy prowadzi do utraty możliwości przewidywania, a to zazwyczaj kończy się tytułowym samobójstwem.
Niezależnie od tego, czy osobliwość jest do osiągnięcia, czy nie, a jeżeli tak to jak szybko, dyskusja, którą w związku z tym wywołano, po pierwsze, zrekapitulowała obecny stan wiedzy w tym zakresie, po drugie, wskazała najistotniejsze problemy warte zbadania, a po trzecie, stanowi inspirację dla rzeszy młodych naukowców wskazując im kierunki, w których warto podążać. Wskazuje też, że robotami muszą się zająć nie tylko przedstawiciele nauk technicznych, ale także etycy i prawnicy.
(red.), Advances in Neural Information Processing Systems 30 (NeurIPS 2017). Curran Associates.
16. Dennett D., The self as a responding-and responsible artifact. „Annals of the New York Academy of Sciences”, Vol. 1001, No. 1, 20023, 39–50, DOI: 10.1196/annals.1279.003.
17. Derwich M., Najstarsze ludy i kultury. (M. Derwich, A. Żurek, red.), Polska. Dzieje cywilizacji i narodu. U źródeł Polski – do roku 1038. Berteelsmann Media & Wydawnictwo Dolnośląskie, Warszawa-Wrocław, 2002.
18. Diamond J.M., Strzelby, zarazki, maszyny. Losy ludzkich społeczeństw. Prószyński i s-ka, Warszawa, 2000.
19. Diamond J.M., Collapse: How Societies Choose to Fail or Survive. Penguin Books, London, 2005.
20. Doliński D., Myślenie spiskowe – mechanizmy psychologiczne . (J.Lubacz, red.), Ewolucja cywilizacyjnej roli i społecznego odbioru nauki , Instytut Podst awowych Problemów Cywilizacji im. Marka Dietricha, Warszawa, 2021, 23–34.
1. Encyklopedia PWN – hasło: racjonalność. PWN, 1997–2022.
2. Encyklopedia PWN – hasło: religia. PWN, 1997–2022.
3. Abney K., Robotics, Ethical Theory, and Metaethics: A Guide for the Perplexed . [In:] Lin P., Abney K., Bekey G., (red.), Robot Ethics: The Ethical and Social Implications of Robotics, MIT Press, 2011, 35–52.
4. Adee S., Reverse Engineering the Brain: David Adler Dreams of a Google Map for the Human Brain. „IEEE Spectrum”, Vol. 45, June 2008, 43–45.
5. Arkin R., Governing Lethal Behavior in Autonomous Robots. CRC Press, 2009.
6. Asimov I., Runaround. Astounding science fiction, Vol. 29, No. 1, 1942, 94–103.
7. Awad E., Dsouza S., Kim R., Schulz J., Henrich J., Shariff A., Bonnefon J.-F., Rahwan I., The moral machine experiment. „Nature”, Vol. 563, No. 7729, 2018, 59–64, DOI: 10.1038/s41586-018-0637-6.
8. Bekey G., Current Trends in Robotics: Technology and Ethics. [In:] Lin P., Abney K., Bekey G., (red.), Robot Ethics: The Ethical and Social Implications of Robotics, MIT Press, 2011, 17–34.
9. Bendyk E., W stronę światła. „Polityka”, Vol. 24, No. 3164, 13–19 czerwca 2018, 58–60.
10. Brooks R., I, Rodney Brooks, am a robot. „IEEE Spectrum”, Vol. 45, June 2008, 60–67.
11. Brown T., Mann B., Ryder N., Subbiah M., Kaplan J., Dhariwal P., Neelakantan A., Shyam P., Sastry G., Askell A., Agarwal S., Herbert-Voss A., Krueger G., Henighan T., Child R., Ramesh A., Ziegler D., Wu J., Winter C., Hesse C., Chen M., Sigler E., Litwin M., Gray S., Chess B., Clark J., Berner C., McCandlish S., Radford A., Sutskever I., Amodei D., Language models are few-shot learners, [In:] Advances in Neural Information Processing Systems 33 (NeurIPS 2020).
12. Burdukiewicz J.M., Zbieracze i łowcy. (M. Derwich, A. Żurek, red.), Polska. Dzieje cywilizacji i narodu. U źródeł Polski – do roku 1038. Berteelsmann Media & Wydawnictwo Dolnośląskie, Warszawa-Wrocław, 2002.
13. Campbell K., McWhir J., Ritchie W., Wilmut I., Sheep cloned by nuclear transfer from a cultured cell line „Nature”, Vol. 380, 1996, 64–66, DOI: 10.1038/380064a0.
14. Chancel L., Piketty T., Saez E., Zucman G., World Inequality Report 2022. World Inequality Lab, 2022.
15. Christiano P., Leike J., Brown T., Martic M., Legg S., Amodei D., Deep reinforcement learning from human preferences . [In:] Guyon I., Luxburg U.V., Bengio S., Wallach H., Fergus R., Vishwanathan S., Garnett R.
21. Ford M., Świt robotów – czy sztuczna inteligencja pozbawi nas pracy? cdp.pl, 2016.
22. Freeman R., Who owns the robots rules the world Harvard Magazine, May-June 2016, 37–39.
23. Gackowski Z., Unorthodox Thoughts about Good Robots Viewed Pragmatically and Praxiologically. (K. Tchoń, W. Gasparski, red.), A Treatise on Good Robots, Transaction Publishers, 2014, 39–53.
24. Grygiel R., Rolnicy i pasterze. (M. Derwich, A. Żurek, red.), Polska. Dzieje cywilizacji i narodu. U źródeł Polski – do roku 1038 . Berteelsmann Media & Wydawnictwo Dolnośląskie, Warszawa-Wrocław, 2002.
25. Guizzo E., Monkey controls advanced robot using its mind in unprecedented demonstration, a monkey with a brain-machine interface commands a seven-degree-offreedom robotic arm . „IEEE Spectrum News Robotics”, 2010.
26. Góralski A., Technologia pracy umysłowej: prekursorzy. (A. Góralski, red.), Zadanie, metoda, rozwiązanie. Zbiór 4, Wydawnictwa Naukowo-Techniczne, Warszawa, 1982, 29–54.
27. Hampson R., Song D., Robinson B., Fetterhoff D., Dakos A., Roeder B., She X., Wicks R., Witcher M., Couture D., Laxton A., Munger-Clary H., Popli G., Sollman M., Whitlow C., Marmarelis V., Berger T., Deadwyler S., Developing a hippocampal neural prosthetic to facilitate human memory encoding and recall. „Journal of Neural Engineering”, Vol. 15, No. 3, 2018, DOI: 10.1088/1741-2552/aaaed7.
28. Hanson R., Economics of the singularity. „IEEE Spectrum”, Vol. 45, 2008, 37–42.
29. Harari Y., Sapiens – od zwierząt do bogów . PZWL –Wydawnictwo Lekarskie, Warszawa, 2014.
30. Horgan J., The consciousness conundrum. „IEEE Spectrum”, Vol. 45, 2008, 28–33.
31. Humphries C., A Detailed 3-D Atlas of a Human Brain MIT Technology Review, 2013.
32. Jackson P., Mongołowie i Zachód . Bellona, Warszawa, 2007.
33. Kadrow S., Gediga B., Od brązu do żelaza. (M. Derwich, A. Żurek, red.), Polska. Dzieje cywilizacji i narodu. U źródeł Polski – do roku 1038. Berteelsmann Media & Wydawnictwo Dolnośląskie, Warszawa-Wrocław, 2002.
34. Kagan B.J., Kitchen A.C., Tran N.T., Habibollahi F., Khajehnejad M., Parker B.J., Bhat A., Rollo B., Razi A., Friston K.J., In vitro neurons learn and exhibit sentience when embodied in a simulated gameworld. „Neuron”, Vol. 110, No. 23, 2022, DOI: 10.1016/j.neuron.2022.09.001.
35. Kennedy S., Schwartz A., Distributed processing of movement signaling. „Proceedings of the National Academy of Sciences”, Vol. 116, No. 52, 2019, 26266–26273, DOI: 10.1073/pnas.1902296116.
36. Kissinger H., How the Enlightenment Ends. „The Atlantic”, June 2018.
37. Koch C., Tononi G., Can Machines be Conscious? Yes –and a new Turing test might prove it. „IEEE Spectrum”, Vol. 45, 2008, 47–51.
38. Kossowska M., Odporni na wiedzę ... czy o zaufaniu i polityce . (J. Lubacz, red.), Ewolucja cywilizacyjnej roli i społecznego odbioru nauki, Instytut Podstawowych Problemów Cywilizacji im. Marka Dietricha, Warszawa, 2021, 59–73.
39. Kumar A., Pareek V., Faiq M., Ghosh S., Kumari C., Adult neurogenesis in humans: A review of basic concepts, history, current research, and clinical implications „Innovations in Clinical Neuroscience”, Vol. 16, No. 5–6, 2019, 30–37.
40. Kurzweil R., The Age of Intelligent Machines . MIT Press, Cambridge, Mass., 1990.
41. Kurzweil R., The Age of Spiritual Machines: When Computers Exceed Human Intelligence. Penguin, New York, 1999.
42. Kurzweil R., The Singularity is Near: When Humans Transcend Biology. Viking, New York, 2005.
43. Kurzweil R., How to Create a Mind: The Secret of Human Thought Revealed. Viking, New York, 2012.
44. Lebedev M., Nicolelis M.. Brain-machine interfaces: From basic science to neuroprostheses and neurorehabilitation . „Physiological reviews”, Vol. 97, No. 2, 2017, 767–837, 2017, DOI: 10.1152/physrev.00027.2016.
45. Ledford H., CRISPR, the disruptor. „Nature”, Vol. 522, 2015, 20–24.
46. Libet B., Do we have free will ? „Journal of Consciousness Studies”, Vol. 6, No. 8–9, 1999, 47–57.
47. Libet B., Gleason C., Wright E., Pearl D., Time of Conscious Intention to Act in Relation to Onset of Cerebral Activity (Readiness-Potential): The Unconscious Initiation of a Freely Voluntary Act. „Brain”, Vol. 106, No. 3, 1983, 623–642, DOI: 10.1093/brain/106.3.623..
48. Lubacz J., Kilka uwag – “informacji” – wprowadzających . (J. Lubacz, red.), W drodze do społeczeństwa informacyjnego , Instytut Podstawowych Problemów Cywilizacji, Warszawa, 1999, 5–10.
49. Lutz W., Samir K., Global human capital: Integrating education and population. „Science”, Vol. 333, No. 6042, 2011, 587–592, DOI: 10.1126/science.1206964.
50. Lutz W., Sanderson W., Scherbov S., The coming acceleration of global population ageing. „Nature”, Vol. 451, No. 7179, 2008, 716–719.
51. Man J., Kubiłaj-Chan – władca największego imperium w dziejach. Amber, Warszawa, 2007.
52. Maynez J., Narayan S., Bohnet B., McDonald R., On faithfulness and factuality in abstractive summarization, 2020.
53. McKinsey&Company. Kluczowe umiejętności na rynku pracy przyszłości. Ramię w ramię z robotem – Jak wykorzystać potencjał automatyzacji w Polsce, Forbes, 2018, 25–26.
54. McKinsey&Company. Nadchodzi czwarta rewolucja przemysłowa. Ramię w ramię z robotem – Jak wykorzystać potencjał automatyzacji w Polsce, Forbes, 2018, 7–8.
55. McKinsey&Company. Wpływ automatyzacji na polską gospodarkę. Ramię w ramię z robotem – Jak wykorzystać potencjał automatyzacji w Polsce, Forbes, 2018, 19–23.
56. Meadows D., Meadows D., Randers J., Behrens W., III. The limits to growth. A Report to The Club of Rome, 1972.
57. Moore G., Cramming more components onto integrated circuits . „Electronics Magazine”, Vol. 38, No. 8, 1965, 114–117, DOI: 10.1109/N-SSC.2006.4785860.
58. Mullin E., How Big Science Failed to Unlock the Mysteries of the Human Brain . MIT Technology Review, August 25 2021.
59. Nalepa G., From Good Robots to Useful Intelligent Agents. (K. Tchoń, W. Gasparski, red.), A Treatise on Good Robots, Transaction Publishers, 2014, 159–170.
60. Nordmann A., Singular Simplicity – The story of singularity is sweeping, dramatic, simple – and wrong. „IEEE Spectrum”, Vol. 45, 2008, 52–55.
61. OpenAI. GPT-4 technical report, 2023.
62. Radford A., Wu J., Child R., Luan D., Amodei D., Sutskever I., Language models are unsupervised multitask learners. 2019.
63. Russell S., Norvig P.. Artificial Intelligence: A Modern Approach. Pearson Education, Upper Saddle River, N.J., 2003.
64. Sanchez C., Chiu C.-W., Zhou Y., González J., Vinson S., Liang H., Locomotion control of hybrid cockroach robots. „Journal of The Royal Society Interface”, Vol. 12, No. 105, 2015, DOI: 10.1098/rsif.2014.1363.
65. Sandhu K., Sherwin E., Schellekens H., Stanton C., Dinan T., Cryan J., Feeding the microbiota-gutbrain axis: diet, microbiome, and neuropsychiatry . „Translational Research”, Vol. 179, 2017, 223–244, DOI: 10.1016/j.trsl.2016.10.002.
66. Shelly M., Frankenstein . Wydawnictwo Poznańskie, Poznań, 1989.
67. Spalding K., Bergmann O., Alkass K., Bernard S., Salehpour M., Huttner H., Boström E., Westerlund I., Vial C., Buchholz B., Possnert G., Mash D., Druid H., Frisén J.. Dynamics of hippocampal neurogenesis in adult humans „Cell”, Vol. 153, 2013, 1219–1227, DOI: 10.1016/j.cell.2013.05.002.
68. Sproule A., James Watt – Jak rozwój maszyn parowych stworzył nasze społeczeństwo przemysłowe. Czytelnik, Warszawa, 1992.
69. Sun-Zi. Sztuka wojenna. Wydawnictwo Olesiejuk, Ożarów Mazowiecki, 2012.
70. Tchoń K., Introduction. (K. Tchoń, W. Gasparski, red.), A Treatise on Good Robots , xvii–xxviii. Transaction Publishers, 2014.
71. Vaswani A., Shazeer N., Parmar N., Uszkoreit J., Jones L., Gomez A., Kaiser L., Polosukhin I., Attention is all you need. [In:] Guyon I., Luxburg U.V., Bengio S., Wallach H., Fergus R., Vishwanathan S., Garnett R. (red.), Advances in Neural Information Processing Systems 30 (NeurIPS 2017). Curran Associates.
72. Vetulani J., Jak usprawnić pamięć. Wydawnictwo Platan, 1993.
73. Vinge V., The coming technological singularity: How to survive in the post-human era. Vision-21: Interdisciplinary Science and Engineering in the Era of Cyberspace, Vol. 10129 serii NASA Conference Publication, 11–22, Cleveland, OH, 1993. NASA Lewis Research Center.
74. Vinge V., Signs of the Singularity . „IEEE Spectrum”, Vol. 45, 2008, 69–74.
75. Visser J., Budzynska K., Reed C., A critical discussion game for prohibiting fallacies. „Logic and Logical Philosophy”, Vol. 27, No. 4, 2017, 491–515, DOI: 10.12775/LLP.2017.021.
76. Vollset S., et all. Fertility, mortality, migration, and population scenarios for 195 countries and territories from 2017 to 2100: a forecasting analysis for the global burden of disease study. „Lancet”, July 2020.
77. Voytyuk M., Brain evolution resulting from cooking (T. Shackelford, V. Weekes-Shackelford, red.), Encyclo-
pedia of Evolutionary Psychological Science ,. Springer International Publishing, Cham, 2021, 735–739.
78. Wallas G.. The Art of Thought. Life and letters series. J. Cape, 1926.
79. Warwick K., Shah H., Velder A., Stradella E.. How Good Robots Will Enhance Human Life . (K. Tchoń, W. Gasparski, red.), A Treatise on Good Robots, 3–18. Transaction Publishers, 2014.
80. Warwick K., Xydas D., Nasuto S., Becerra V., Hammond M., Downes J., Marshall S., Whalley B., Controlling a mobile robot with a biological brain. „Defence Science Journal”, Vol. 60, 2010, 5–14.
81. Wierzbicki A.P., Techne n: Elementy niedawnej historii technik informacyjnych i wnioski naukoznawcze. Komitet Prognoz „Polska 2000 Plus” PAN, Warszawa, 2011.
82. Wilmut I., Schnieke J., McWhir A., Kind A., Campbell K., Viable offspring derived from fetal and adult mammalian cells. „Nature”, Vol. 385, 1997, 810–813.
83. Zorpette G., Waiting for the rapture: Technological convergence will change our lives but wont’t make them indefinitely long. „IEEE Spectrum”, Vol. 45, 2008, 26–27.
84. Zybertowicz A., Gurtowski M., Tamborska K., Trawiński M., Waszewski J., Samobójstwo Oświecenia? Wydawnictwo Kasper, Kraków, 2015.
In order to assess the impact of robots on society, it is necessary to carefully analyze the state-of-the-art, and in particular the fundamental issues that have yet to be resolved, however having significant impact on the potential societal changes resulting from the development of robotics. The aforementioned impact depends on the level of intelligence of robots, so this aspect dominates in the presented analysis. The presentation has been divided into three parts: 1) analysis of technical factors affecting the intelligence and security of robots, 2) analysis of current capabilities of robots, 3) analysis of diverse predictions of how robotics will evolve, and thus the attitudes towards the influence of the result of this development on society. This part of the paper is devoted to the third of the above mentioned three issues.
Keywords
ORCID: 0000-0001-7604-8834
Abstract: This paper presents the method of trajectory planning for mobile manipulators considering limitations resulting from capabilities of robotic system actuators. The fulfillment of control constraints is achieved by introducing virtual control scaling of the robot trajectory in the limited periods of time. Such an approach allows researchers to obtain the trajectories fulfilling control constraints without significantly increasing the time of task execution. The proposed method generates sub-optimal trajectories maximizing the manipulability measure of the robot arm, preserves mechanical and collision avoidance limitations and can be used in real-time trajectory planning. The effectiveness of the presented solution is confirmed by computer simulations involving a mobile manipulator with parameters corresponding to KUKA youBot.
In the presented work the problem of trajectory planning for mobile manipulators consisting of a nonholonomic platform and a holonomic arm is considered. The task of this type requires a number of limitations to be considered resulting from the nature of the robot task, its mechanical construction and the presence of the obstacles in the workspace. In the literature several approaches to solving such a problem have been presented. Some of the solutions omit dynamic parameters of the robot, however, the algorithms taking into account the dynamics of the system seem to be more interesting. Such methods can be divided into two groups: in the first one the manipulator arm and the platform are treated as two separate subsystems, in the other group the whole robot is considered as one integrated system.
The solution based on the decomposition of the task into two independent sub-tasks has been proposed by [14]. In this approach the effector follows the path described relative to the base and the platform moves along a certain flat curve. The proposed solution leads to a system with two controllers: the kinematic controller enables tracking the path by both subsystems and the dynamic one ensures the convergence of the robot velocities to velocities obtained from the kinematic controller. A similar approach, using the decentralized control for the task of tracing the end-effector trajectory, was also described by
[4] and [23]. The solution of the task for mobile robot moving between initial and final configurations provided in a complex environment has been presented in [13]. The authors have focused on planning the shortest collision-free trajectory, they have not considered state and control constraints and have obtained smooth trajectories using cubic polynomials.
In addition to solutions where the arm and the platform are treated as separate subsystems, there are also approaches where the mobile manipulator is considered as one integrated system. Such a solution for mobile manipulators operating in a limited workspace has been presented by [8]. The authors have paid particular attention to maintaining the stability of the system by the appropriate coordination of the movements of the platform and the manipulator. An approach that integrates motion planning with the control of the mobile manipulator as well as control of the nonholonomic trolley has been suggested by [21]. Article [6] has offered a class of controllers solving the trajectory tracking problem subject to control-dependent constraints. The trajectory of the robot has been determined in such a way as to minimize the instantaneous energy and provide collision-free motion. In [18] the authors have used attractive and repulsive potential functions to plan the trajectory of the mobile manipulator equipped with a car-like platform. The proposed method enables planning the collision-free motion between initial and final positions, taking into account mechanical singularities and velocity limitations, still the dynamics of this system is not considered. The Simulated Annealing algorithm has been applied in [1] to solve the time optimal trajectory planning task. The author has focused on maximizing the reachability of the target point introducing a new singularity avoidance metric named Manipulability Percentage Index. The limitations on generalized coordinates, velocities and accelerations have been analyzed, but control constraints have not been considered. A systematic review of motion planning approaches of mobile manipulators has been undertaken by [19].
In the method of trajectory planning outlined in this paper the mobile manipulator is treated as one integrated system. The presented approach solves the point-to-point trajectory planning problem and takes into account both state and control constraints, moreover, it provides a sub-optimal solution, minimizing the instantaneous manipulability measure dependent index. As a result, the method generates the trajectories which avoid singular configurations and provides high manipulability measure of the manipulator arm. Such an approach can be utilized to plan the trajectory of industrial mobile robots moving between multiple production workstations as well as space rovers equipped with a robotic arm. One of the typical task of such rovers is to move to the selected location to collect samples or take photos of the object specified by the operator. It seems that the method taking into account state and control constraints, the manipulability of the arm and collision avoidance conditions is suitable to plan the motion of such robots in these cases.
As opposed to similar approaches, the method suggested in this paper incorporates nonholonomic constraints in a Pfaffian form explicitly to the control algorithm, so it does not require transformation to a driftless control system. The effectiveness of the proposed algorithm allows it to be used in real-time trajectory planning. The solution of the primary robot task utilizes an extended Jacobian approach and it is based on previous works by the authors [16, 17], still the new approach considering the limitations resulting from physical abilities of actuators is put forward in this paper. In contrast to the works cited, where the fulfillment of controls limitations was achieved by scaling the whole robot trajectory, in this paper scaling is applied in certain time periods only.
As described in Section 4 such a novel approach significantly improves the efficiency of the proposed algorithm as it does not significantly extend the time needed to complete the task, which was the biggest disadvantage of earlier solutions. Moreover, in this paper the condition for the existence of the solution in the presence of control limitations is formulated.
It is assumed that the initial configuration q0 is non-singular and collision free, moreover the mobile manipulator is motionless at the initial and final moment of the motion:
00,0.qqT (3)
The robot motion is subject to various constraints. Due to nonholonomic of the platform its movement is limited by h phase constraints described in the Pfaffian form
0, Aqqpp (4)
where: A is (h × n p) the Pfaffian full rank matrix.
The arm of the mobile robot should avoid singular configurations in which it loses its manipulative abilities. Hence, in this work, the maximization of manipulability measure was put forward. In this case, the manipulability of the holonomic arm is determined using the index based on [22] in the form
where matrix / aa Jqkqqa is the analytical Jacobian of the manipulator arm.
Additionally, due to mechanical limitations and constraints related to obstacles existing in the workspace, robot motion is limited by state inequality constraints in the form of 0, 0,1,, i MM tTCqtiL (6)
In this paper the mobile manipulator composed of the nonholonomic platform and the holonomic arm is considered. Introducing n p -elemental vector qp consisting of configuration coordinates of the platform and n a -elemental vector qa including configuration coordinates of the arm, the mobile manipulator can be described by the n-elemental vector (n = n p + n a) of generalized coordinates in the form
T TT qqqpa (1)
The task of the mobile robot is to move its end-effector to the specified location in the m-dimensional workspace. Such tasks are performed when the robot moves to another location to accomplish a specific task or transport an object held by its gripper. In such a case, it is assumed that at the initial time t = 0 the robot is in a certain configuration q(0) = q0, which was achieved following the completion of the previous operation. The task is thus to find the trajectory q(t) ensuring, at the final moment t = T, reaching the final unknown configuration q(T) = qT, allowing to achieve the desired end-effector location pf k(q(T)) − pf = 0, (2)
where k : n → m denotes m-dimensional mapping describing the position and orientation of the robot end-effector in the workspace.
where LM and LO denote a total number of mechanical and collision avoidance constraints, i M C and i CO describe the algebraic distance of the i-th configuration from its limits and algebraic distance of the robot from i-th obstacle, respectively.
Moreover, the physical abilities of robot actuators imply the need of consideration of constraints connected to controls. It is a very important issue from a practical point of view, yet it significantly complicates the solution of the task. In the case of nonholonomic mobile manipulators, an additional problem related to the description of dynamics and the need to take into account the restrictions on platform movement needs to be considered. On the other hand, neglecting these limitations can lead to a solution which can be impossible to adopt by a real robot. Such constraints can be described in the general form as a set of (n − h) inequalities as
1 nh is the vector of control (torques/forces) and
where
nh are lower and upper limits on
In order to solve the basic task of the robot (2), taking into account constraints (3) and (7) the approach based on the
set of points PM approximating the robot in such a way that if points from PM do not collide with enlarged obstacles then the robot does not collide with the original obstacles. Using this approach collision avoidance conditions (7) can be expressed as a finite number of inequalities in the form of (17)
In order to obtain collision free motion, the method proposed in [17] was used. In this approach the accelerations of the mobile manipulator are perturbed in the neighborhood of the obstacle by the continuous perturbation pushing the robot away from the obstacle (18)
where is the positive coefficient determining the strength of the influence of perturbation, i O is any continuous, differentiable interior penalty function equaling 0 outside the neighborhood of the obstacle and increasing when the robot approaches the surface of the obstacle. It is worth noting that the first component of the dependency is responsible for pushing the robot away from the obstacle, and the second one reduces the velocity of the mobile manipulator in the obstacle proximity. As it presented in [17], using the Lyapunov stability theory it is possible to show that the perturbation (18) forces the mobile manipulator to escape from the obstacle.
In order to find collision free motion the trajectory (16) should be extended with perturbation (18). It is worth noting that such a solution can disturb the robot movement only in the proximity of the obstacles, when the perturbation is active. As a result, it affects the condition (11) and may lead to the decrease in the manipulability measure, however, it can only occur near the obstacle surface, when the change of the configuration is necessary to avoid the collision. Additionally, in order to prevent the violation of nonholonomic constraints the use of the projection operator onto the null space of the matrix is proposed. Finally, the collision-free trajectory of the mobile manipulator is described by the dependency
(19)
It should be noted that the presented method if of a local character so it is possible to stuck in the local minima or saddle points. This disadvantage can be overcome by applying small perturbation if the mobile manipulator stops before reaching the final point. Such an algorithm, for convex shaped obstacles, was presented in [11]. The authors suggested perturbation forcing the motion of the robot along any of the basis vector of the tangential hyperplane of the obstacle boundary. As was shown, such an approach ensures leaving a saddle point in one iteration and it should be repeated several times if the mobile manipulator is stuck in the local minima.
the authors [16, 17] the control limitations can be considered by a suitable choice of gain coefficients VI and , PI the disadvantage of such a solution, however, is significant increase in task execution time. In order to eliminate this disadvantage, in this paper the approach of scaling the trajectory only in certain time periods is proposed in this paper. To achieve it, an additional variable, called the virtual control and affecting the trajectory only if robot controls are close to their limitations, is introduced. The preliminary idea of such an approach was presented in [15], but in this work the concept is significantly extended and supplemented with the condition for the existence of the solution.
In order to take control constraints into account, it is necessary to know the dynamic equations of the mobile robot, given in a general form as
where M(q) denotes (n × n) the positive inertia matrix, , cqq is n-dimensional vector representing Coriolis, centrifugal, viscous, Coulomb friction and gravity forces, is h-dimensional vector of the Lagrange multipliers corresponding to nonholonomic constraints (4) and B is n × (n h)) full rank matrix (by definition) describing which state variables of the mobile manipulator are directly driven by the actuators.
The extended Pffafian matrix (q) is the full rank, so there exists the full rank (n × (n − h)) matrix N(q), orthogonal to , which satisfies the relation (q)N(q) = 0. Thus, the equation (20) can be left-multiplied by NT(q) and written in the new form as
As shown in [3] it is possible to choose the configuration of motorization for any nondegenerate nonholonomic platform which provides full platform mobility and ensures a full rank of the matrix NT(q)B, so the controls of the mobile manipulator can be determined from (21) as follows TTT
It should be emphasized that the above transformation is necessary to determine the trajectory scaling coefficient, the remaining calculations are performed using a model expressed in generalized coordinates. Such trajectory scaling is carried out by introducing virtual control u(t), which takes on values between 0 and 1, affecting the trajectory in limited periods of time, in which control signals are close to constraints. To achieve it, the virtual control u(t) is introduced to trajectory (19) in such a way as not to change the character of the robot movement (the robot motion is slowed down when needed). 1† 12 nO qtqvutvutIq (23)
Substituting (23) into (22) the robot controls can be written as a linear function of virtual control u(t) as follows
The trajectory (19) considers all the conditions listed in Section 2, except the control constraints (8) resulting from capabilities of robot actuators. As was shown in previous works by
Due to the inaccuracy of the robot dynamic model the safety zones of size determined by 0,1 for min and max are introduced as follows
In practice, it seems that in this case it is reasonable to choose û as the value closest to the virtual control determined at the previous time instant.
The trajectory of the mobile manipulator is scaled when any control exceeds ,min or ,max, which maintains a certain margin enabling the control systems to introduce corrections to the trajectory being realized. Finally, using dependency (24) the control constraints (8) can be written in the new form as
According to the proposed method, the virtual control u(t) takes a value equal to 1 when the control signals of the mobile manipulator are within the range determined by the dependencies (25). In this case, the trajectory is not scaled and the robot movement follows the original solution described by the dependency (23). If, at a given time instant, controls exceed the safety margins (25), it is necessary to find the û value to satisfy constraints (26). Hence, for each i-th control that exceeded its limit, value ûi should be determined in such a way as to satisfy the inequality
If there are only cases (a), (b) or (c), (d) then there is always û which ensures that constraints (26) are satisfied. If cases (a) or (b) and (c) or (d) occur simultaneously then the fulfillment of the inequality (30) is a condition for the existence of a solution.
At the time instants when the limits are exceeded the virtual control u(t) takes values û obtained according to the algorithm presented above. If the controls t decrease, and the trajectory scaling is no longer needed, the virtual control u(t) should be enlarged to its maximum value equal to 1. In order to maintain the continuity of the control , t it is necessary to ensure the continuous change of the virtual control u(t). In order to do so, it is assumed that the u(t) increases asymptotically to its maximum value according to the dependency
where > 0 determines the convergence rate.
To find the value ûi, it is assumed that the coefficient ai is non-zero and four cases are considered
In order to satisfy the control constraints at the initial moment of the motion the appropriate initial value of the virtual control u0 should be given. The dependencies (28)−(30) can be used to achieve it, but it should be noted that for initial generalized velocities equal to zero, component , bqq in (24) contains only the vector , cqq with non-zero elements related to gravity forces. Hence, u0 = 0 leads to controls balancing the gravity forces. It seems reasonable to assume that control constraints should not be less than these values. As shown in numerical examples, an additional advantage of such an initial value of virtual control is to ensure a smooth start of the robotic system at the beginning of the task.
Finally, according to the proposed algorithm, u0 = 0 is assumed to be the initial value of the virtual control. Then u(t) is increased according to the dependency (31). If the control signals exceed the safety margins (25), a new value of û, ensuring that the constraints are satisfied, is determined using (28) (30). If the controls t decrease and limitations (26) are satisfied, the virtual control u(t) is increased according to the dependency (31).
If at a given time instant only one control exceeds the assumed limit, then the virtual control û takes the value equal to the right side of corresponding inequality (a) (d). If several control signals do not satisfy the limits then: −if there are only cases (a) or (b), then
if there are only cases (c) or (d), then ,min ,max min ,
ˆˆ max,, j i j i
if both cases (a) or (b) and (c) or (d) occur, virtual control û has to satisfy dependency
In order to verify the effectiveness of the proposed algorithm, computer simulations involving the mobile manipulator shown in Fig. 1, consisting of nonholonomic platform of (2, 0) class and a 3-DoF 3R holonomic manipulator working in three-dimensional task space is considered. Kinematic parameters of the robot correspond to KUKA youBot mobile platform with an arm. However, in the simulations presented below, it is assumed that the platform is (2, 0) type and the arm has only three revolute joints (the last three links of the original KUKA arm form the single link of the manipulator) and the orientation of the end-effector is ignored. Finally, the parameters of the robot used in the simulations are given as follows:
−rectangular platform 0 456 m × 0 316 m × 0 046 m with wheels of radius 0 05 m,
−manipulator with a base height 0 161 m and links:
0 033 m, 0 155 m, 0 342 m,
−mass of the platform 19 .803 kg, wheel 1.4 kg,
−mass of the manipulator base 0 961 kg, links:
m1 = 1 39 kg, m2 = 1 318 kg, m3 = 2 496 kg.
The vector of generalized coordinates of such mobile manipulator is described as
q = [x, y, , 1, 2, q1, q2, q3]T, and its kinematics is given as follows
min = [−1.5, −1.5, −1.0, −10.0, −5.0 ]Nm,
max = [ 1.5, 1.5, 1.0, 0.0, 0.0 ]Nm.
The mobile manipulator operates in the workspace including three obstacles:
1. cylinder: radius 0.25 m, height 0.2 m, base center [0 15, 0 75, 0]Tm,
2. sphere: radius 0 15 m, center [1 25, 0 7, 0 45]Tm,
3. cylinder: radius 0 4 m, height 0 1 m, base center [1 25, 2 5, 0]Tm.
In order to formulate the collision avoidance conditions (17) each obstacle is enlarged by = 0 05 m. The discretization of the platform and the links of the manipulator, ensuring the avoidance of the collision with the sphere and the cylinders with parameters given above, is determined as 0 14 m. Within the proposed approach the obstacles in the workspace have to be approximated by smooth surfaces. In the presented simulations solids described by superellipsoids, widely discussed by [9], are used. The parameters of superellipsoids (semidiameters a x, a y, a z and coefficients 1, 2 determining the shape of the solids) representing the obstacles in the workspace are given in Tab. 1.
The sizes of neighborhoods in which perturbation affects the robot motion are equal to 0.35 m and 0.15 m for cylinders and sphere, respectively. The gain coefficients Λ used in the trajectory generator (23) are taken as
ΛPI = diag(1 75, 1 75, 1 75, 1 75, 1 75),
ΛVI = diag(2 78, 2 78, 2 78, 2 78, 2 78),
where x, y are location of the platform center, is the platform orientation, 1, 2 denotes the angles of driving wheels, q1, q2, q3 stand for configuration angles of the manipulator joints, c = cos( ), c 1 = cos(− + q1), c 12 = cos(− + q1 + q2) + cos( − q1 + q2), c 123 = cos(− + q1 + q2 + q3) + cos(
− q1 + q2 + q3), s = sin( ), s2 = sin(q2), s23 = sin(q2 + q3), s 1 = sin(− + q1), s 12 = sin(− + q1 + q2) − sin( − q1 + q2), s 123 = sin(− + q1 + q2 + q3) − sin( − q1 + q2 + q3).
ΛII = diag(1 0, 1 0, 1 0, 1 0, 1 0)
0.160.050
x y cs cs sc
0.1600.050. 000
The conditions (4) for the motion without lateral and longitudinal sleep, in this case, can be described as 1 2
The task of the mobile manipulator is to move from non-singular, collision free configuration, fulfilling mechanical constraints
q0 = [ 0.0, −0.5, /2, 0.0, 0.0, 0.0, −0.17, 0.35 ]T rad to the final end-effector position
pf = [ 3.5, 4.0, 0.16 ]T m, maximizing the manipulability measure (5) and satisfying mechanical limitations (6):
qa,min = [−169 /180, −65 /180, −151 /180 ]T rad,
qa,max = [ 169 /180, 90 /180, 145 /180 ]T rad, and control limitations equal to:
Fig. 2. Motion of mobile manipulator Rys. 2. Ruch mobilnego manipulatora Fig. 1. Model of mobile manipulator Rys. 1. Model mobilnego manipulatoraTwo cases of such a task are considered. In the first one control constraints (8) are ignored, in the other they are introduced in accordance with the approach presented in section 4. The way of performing the task in both cases is similar and it is shown in Fig. 2.
In the first case the final time of task execution is equal to 44.6 s. The minimal distance between the robot and the surface of obstacles is shown in Fig. 3. As it can be seen, this distance is greater than zero during the whole movement, so the robot motion is collision-free. The dashed horizontal lines represent the boundaries of neighborhoods surrounding the obstacles. It is evident that the robot maneuvers near obstacles most of the time, leaving the obstacles neighborhood for a short time of about 20 s and permanently in the final part of the movement. The motion in such a complex space leads to an increase in task execution time due to the second component of perturbation (18), which significantly reduces the velocity of the robot near the obstacles.
To improve readability, the values of arm joint angles are mean normalized, i.e. scaled to [−0 . 5 , 0 . 5] in order to fit in the same range, and presented in Fig. 4. As it can be seen, mechanical constraints are satisfied and joint angles remain far away from their limitations (dashed red horizontal lines represent normalized limitations).
The analysis of changes in the manipulability measure, presented in Fig. 5, makes it evident that the index generally increases during the task execution and the manipulator achieves the maximum dexterity after reaching the final point. However, a local decrease in manipulability can be seen at the beginning of the motion. In this case there was a potential collision of the manipulator arm with the first obstacle, which forced a significant change of configuration leading to the decrease in arm manipulability. After passing the obstacle the influence of perturbation (18) decreases and the minimization of criteria (9) ensures an increase in manipulability measure.
Mean normalized control signals obtained in the first case, when the constraints (8) were ignored, are shown in Figs. 6 and 7. It can be seen that both the torques of platform wheels and arm joints exceed the assumed limitations (dashed red horizontal lines represent normalized limitations). It is especially evident at the beginning of the movement when the robot starts from the initial location and in the neighborhoods of the obstacles. Signi-
ficant exceedance of the controls limits can be observed in the middle phase of the motion (18−20 s). In this stage the robot leaves the neighborhood of the second obstacle and begins to increase its velocity (wheels controls increase) and moves away from the obstacle. However, it quickly enters the safety zone of the third obstacle and, as a result, it must its reduce velocity, so the wheels controls decrease and they exceed the lower limits. Moreover, the potential collision with the robot arm exists, so it is necessary to change the first configuration angle and the corresponding control increases exceeding its limit.
In the second simulation the mobile manipulator preformed the same task as in the first case, but the control constraints were considered. The way of task execution was similar to the first simulation, however, due to the reduction of controls, the final time slightly increased to 49.4 s. For the purposes of the virtual control method, proposed in this paper, the size of safety zones for min and max was taken as = 0 1. The minimal distance between the robot and the obstacles, mean normalized joint angles and the manipulability measure are presented in Figs. 8−10, respectively.
Mean normalized control signals obtained in this case for platform wheels, arm joints and virtual control scaling the trajectory are shown in Figs. 11, 12 and 13, respectively. As it can be seen in Fig. 13, the value of the virtual control u (t) increases at the beginning of the motion leading to increase of controls, which quickly reach safety zones of their limits. As a result, the virtual control determination algorithm, described in Section 4, is activated and virtual control u(t) is modified according to dependencies (28)−(30), which results in reducing the controls in the first second of the motion. When the controls reach the safe values, the virtual control u ( t ) begins to raise again up to a maximum value equal to 1 in accordance withe the equation (31). Similar behavior of virtual control take place in the final (about 45 s) phase of the movement.
More complex changes are visible in the middle phase (time interval 18 20 s) when the robot maneuvers between two obstacles. After changing the direction near the first obstacle (controls reach their limits, virtual control decreases) the robot continues to move (controls decrease, virtual control increases), but soon comes closer to the second obstacle and changes its direction again (controls reach their limits, virtual control decreases). Finally, as simulation results show, the use of the proposed approach allows to fulfill control limitations at the cost of a relatively small increase in execution time (about 10 %).
Fig. 10. Arm manipulability measure for the second task Rys. 10. Miara manipulowalności ramienia w drugim zadaniu Fig. 7. Normalized arm joints torques for the first task Rys. 7. Znormalizowane momenty obrotowe przegubów ramienia w pierwszym zadaniu Fig. 8. Minimal distance between robot and obstacles for the second task Rys. 8. Minimalna odległość między robotem i przeszkodami w drugim zadaniuThis paper presents a method of trajectory planning when the mobile manipulator has to reach a specified end-effector position within the workspace. This approach guarantees the fulfillment of mechanical and collision avoidance constraints, additionally, it ensures the movement far away from singular configurations by maximizing the manipulability measure of the robot arm. The fulfillment of control constraints is obtained by introducing the so called virtual control scaling the trajectory in limited periods of time. As simulation results show, such an approach allows to obtain the trajectories fulfilling control constraints without significantly increasing the time of the task execution. The effectiveness of the solution is confirmed by the results of computer simulations.
1. Akli I., Trajectory planning for mobile manipulators including manipulability percentage index. „International Journal of Intelligent Robotics and Applications”, Vol. 5, 2021, 543–557, DOI: 10.1007/s41315-021-00190-3
2. Berger M., Tagliasacchi A., Seversky L.M., Alliez P., Guennebaud G., Levine J.A., Sharf A., Silva C.T. A survey of surface reconstruction from point clouds. „Computer Graphics Forum”, Vol. 36, 2017, 301–329, Wiley Online Library.
3. Campion G., Bastin G., d’Andrea Novel B., Structural properties and classification of kinematic and dynamic models of wheeled mobile robots. „IEEE Transactions on Robotics and Automation”, Vol. 12, No. 1, 1996, 47–62, DOI: 10.1109/70.481750.
4. Fareh R., Saad M.R., Saad M., Brahmi A., Bettayeb M., Trajectory tracking and stability analysis for mobile manipulators based on decentralized control. „Robotica”, Vol. 37, No. 10, 2019, 1732–1749, DOI: 10.1017/S0263574719000225.
5. Galicki M., The selected methods of manipulators’ optimal trajectory planning WNT Publisher 2000 (in Polish).
6. Galicki M., Task space control of mobile manipulators. „Robotica”, Vol. 29, No. 2, 2011, 221–232, DOI: 10.1017/S026357471000007X.
7. Gálvez A., Iglesias A., Particle swarm optimization for non-uniform rational B-spline surface reconstruction from clouds of 3D data points. „Information Sciences”, Vol. 192, 2012, 174–192, DOI: 10.1016/j.ins.2010.11.007.
8. Huang Q., Tanie K., Sugano S., Coordinated motion planning for a mobile manipulator considering stability and manipulation „International Journal of Robotics Research”, Vol. 19, No. 8, 2000, 732–742, DOI: 10.1177/02783640022067139.
9. Jaklic A., Leonardis A., Solina F., Segmentation and recovery of superquadrics, Vol. 20, 2013, Springer Science & Business Media, DOI: 10.1007/978-94-015-9456-1.
10. Keller P., Kreylos O., Cowgill E.S., Kellogg L.H., Hering-Bertram M., Construction of Implicit Surfaces from Point Clouds Using a Feature-based Approach. „Scientific Visualization: Interactions, Features, Metaphors”, Vol. 2, 2011, 129–143, Schloss Dagstuhl–Leibniz-Zentrum fuer Informatik, DOI: 10.4230/DFU.Vol2.SciViz.2011.129.
11. Khansari-Zadeh S.M., Billard A., A dynamical system approach to realtime obstacle avoidance . „Autonomous Robots”, Vol. 32, No. 4, 2012, 433–454, DOI: 10.1007/s10514-012-9287-y.
12. Leeper A., Chan S., Salisbury K., Point clouds can be represented as implicit surfaces for constraintbased haptic rendering. [In:] 2012 IEEE Internatio-
Fig. 11. Normalized wheels torques for the second task Rys. 11. Znormalizowane momenty obrotowe kół w drugim zadaniu Fig. 12. Normalized arm joints torques for the second task Rys. 12. Znormalizowane momenty obrotowe przegubów ramienia w drugim zadaniu Fig. 13. Virtual control scaling trajectory for the second task Rys. 13. Wirtualne sterowanie skalujące trajektorię w drugim zadaniunal Conference on Robotics and Automation, 5000–5005, DOI: 10.1109/ICRA.2012.6225278.
13. Li Q., Mu Y., You Y., Zhang Z., Feng C., A Hierarchical Motion Planning for Mobile Manipulator, „IEEJ Transactions on Electrical and Electronic Engineering”, Vol. 15, No. 9, 2020, 1390–1399, DOI: 10.1002/tee.23206.
14. Mazur A., Płaskonka J., The Serret–Frenet parametrization in a control of a mobile manipulator of (nh, h) type. „IFAC Proceedings Volumes”, Vol. 45, No. 22, 2012, 405–410, DOI: 10.3182/20120905-3-HR-2030.00069.
15. Pająk G., Trajectory planning for mobile manipulators subject to control constraints. [In:] 11th RoMoCo ’17, 117–122, DOI: 10.1109/RoMoCo.2017.8003901.
16. Pająk G., Pająk I., Planning of a point to point collision-free trajectory for mobile manipulators. [In:] 10th RoMoCo ’15, 142–147, DOI: 10.1109/RoMoCo.2015.7219726.
17. Pająk G., Pająk I., Point-to-point collision-free trajectory planning for mobile manipulators. „Journal of Intelligent and Robotic Systems”, 2016, DOI: 10.1007/s10846-016-0390-8.
18. Prasad A., Sharma B., Vanualailai J., Kumar S., Motion control of an articulated mobile manipulator in 3D using the
Lyapunov-based control scheme. „International Journal of Control”, Vol. 95, No. 9, 2022, 2581–2595, DOI: 10.1080/00207179.2021.1919927.
19. Sandakalum T., Ang M.H. Jr., Motion Planning for Mobile Manipulators – A Systematic Review. „Machines”, Vol. 10, No. 2, 2022, DOI: 10.3390/machines10020097.
20. Singh S.K., Leu M.C., Manipulator motion planning in the presence of obstacles and dynamic constraints. „International Journal of Robotics Research”, Vol. 10, No. 2, 1991, 171–187, DOI: 10.1177/027836499101000208.
21. Tan J., Xi N., Wang Y., Integrated task planning and control for mobile manipulators. „International Journal of Robotics Research”, Vol. 22, No. 5, 2003, 337–354, DOI: 10.1177/0278364903022005004.
22. Yoshikawa T., Manipulability of robotic mechanisms. „International Journal of Robotics Research”, Vol. 4, No. 2, 1985, 3–9, DOI: 10.1177/027836498500400201.
23. Zhou S., Pradeep Y.C., Zhu M., Amezquita-Semprun K., Chen P., Motion control of a nonholonomic mobile manipulator in task space. „Asian Journal of Control”, Vol. 20, No. 5, 2018, 1745–1754, DOI: 10.1002/asjc.1694.
W pracy przedstawiono metodę planowania trajektorii dla manipulatorów mobilnych uwzględniającą ograniczenia wynikające z możliwości układów napędowych robota. Spełnienie ograniczeń na sterowana zostało osiągnięte poprzez wprowadzenie wirtualnego sterowania skalującego trajektorię robota w ograniczonych przedziałach czasu. Takie podejście pozwoliło na uzyskanie trajektorii spełniających ograniczenia na sterowania bez znaczącego wydłużenia czasu realizacji zadania. Zaproponowana metoda generuje sub-optymalne trajektorie maksymalizując miarę manipulowalności ramienia robota, zachowuje ograniczenia mechaniczne oraz warunki unikania kolizji i może być zastosowana do planowania trajektorii w czasie rzeczywistym. Skuteczność zaproponowanego rozwiązania została potwierdzona symulacjami komputerowymi wykonanymi z użyciem mobilnego manipulatora o parametrach odpowiadających robotowi KUKA youBot.
ORCID: 0000-0002-3649-399X
ORCID: 0000-0002-3923-9057
Abstract: Predicting human actions is a very actual research field. Artificial intelligence methods are commonly used here. They enable early recognition and classification of human activities. Such knowledge is extremely needed in the work on robots and other interactive systems that communicate and cooperate with people. This ensures early reactions of such devices and proper planning of their future actions. However, due to the complexity of human actions, predicting them is a difficult task. In this article, we review state-of-the-art methods and summarize recent advances in predicting human activity. We focus in particular on four approaches using machine learning methods, namely methods using: artificial neural networks, support vector machines, probabilistic models and decision trees. We discuss the advantages and disadvantages of these approaches, as well as current challenges related to predicting human activity. In addition, we describe the types of sensors and data sets commonly used in research on predicting and recognizing human actions. We analyze the quality of the methods used, based on the prediction accuracy reported in scientific articles. We describe the importance of the data type and the parameters of machine learning models. Finally, we summarize the latest research trends. The article is intended to help in choosing the right method of predicting human activity, along with an indication of the tools and resources necessary to effectively achieve this goal.
People naturally are able to understand and predict to a certain extent actions of others, which is the foundation for good communication and interaction in daily life. Like for humans, for robots it is often very important to understand human activities at an early stage before they are completely executed, in order to be able to provide a timely and proper reaction [1]. Human activity recognition (HAR) can be viewed as the task of identification, and naming of human-performed activities with the help of artificial intelligence (AI) using sensory data. Understanding human activity is a very important aspect of intelligent machine vision [2]. Human activities are often influenced by natural feelings such as tiredness, exhaustion, loss of attention, low patience, need for rest, limited physical strength, etc and these result in low efficiency when it comes to performing necessary tasks. Hence, HAR is important for implementation in human assisting robots to support human tasks [3]. The examples are monitoring crime rates using HAR, HAR
for elderly people care observes activity patterns and reacts in case of change of behavior or an unrecognized event. HAR applied for monitoring physical activities helps to manage and reduce the risk of contracting health problems such as obesity, diabetes, cardiovascular diseases, etc. It also helps to improve some performances for example in sports activities by detecting and estimating human poses with the arm to improve the motion dynamics. HAR also enables creation of smart home environments.
Moreover, medical diagnosis can be made using physiological measurements and observation, e.g recognizing activities like smoking, sunbathing, etc can help diagnose patients’ health issues. Human activity prediction (HAP) can be referred to as a process of early inferring human activity from partial observations. HAP approaches basically aim at interpreting human actions, gestures, behaviors and correctly classifying them into respective categories before their complete execution [4].
The topics of HAP and HAR have brought a significant contribution to technological advancements. The main difference between HAR and HAP is the decision time. HAP is more important for before-the-fact quick-decision making situation rather than after-the-fact conclusion using HAR (Fig. 1). Nevertheless, both play a significant role in human-human interaction [5], human-object interaction [6], and human-machine interaction [7, 8]. This research domain has highly contributed in many areas including sports [9], robotics [10], security [11], healthcare [12]. The most common sensors used for data recording include RGBD cameras [13, 14], CCTV-came-
ras [15], wearable sensors [16], radio frequency identification (RFID) tags [9], gyroscopes and accelerometers [17, 18]. The problem of HAR and HAP have been challenging for recent years. However, due to the availability of low cost and low power sensors, bigger computational resources, advancements in computer vision, machine learning (ML) and AI, there is significant growth in these research areas. HAP is implemented in various environments including factory facilities allowing effective worker-robot collaboration, road navigation by self-driving vehicles, smart offices, etc. During HAP system design, it is crucial to consider factors such as the type of available devices, method of analysis, and area of application [19]. Obtaining reliable results is also highly dependent on the quality of human pose representation and activity prediction methods. Several methods have been proposed for HAP and HAR and thanks to these methods, researchers are able to explore every thinkable aspect of HAR and HAP. In this work, we will summarize the Artificial Neural Networks (ANN), Support Vector Machine (SVM) approach, probabilistic methods, and decision tree approaches. Each of the specific methods often shows varying performance depending on the application.
art methods of HAP. It continues to give the overall comparison between the machine learning methods, advantages and disadvantages of these methods considering the factors such as accuracy, computing efficiency, time consumption, etc. Section 6 describes the conclusion of our work.
The type of devices and data used for HAP depend on the intended application. Activity data can be obtained from various types of sources, the most common are the following sources: wearable sensors, video cameras, RFID systems, and Wi-Fi devices [21]. Data can be recorded both ways: remotely (contactless) e.g radars, videos, or directly with physical contact with a person like e.g accelerometers and gyroscopes. Many years ago, sensor data collection for HAP was very expensive and challenging but with the advancement of technology, this task has become relatively easier, and several open source datasets are made available for public use.
A recent review [20] gives an elaborated overview focusing on existing deep learning-based approaches in HAR and HAP. It explains methodologies, along with related features considered, and datasets used in previous works. In this paper, we present the state-of-art methods in HAP and HAR. We review the various contributions brought by different HAP methods. The types of sensors, data types, and the corresponding advantages and challenges associated with these methods are presented. The structure of the paper is as follows: section 3 presents a review of the sensors used for HAP and HAR. The sources and types of input data, and the data acquisition techniques associated with them are described. Section 4 presents a review of the machine learning approaches used for both complex and simple activities prediction. It continues elaborate the types of interactions involved in human activities, the stages of the data processing, and the challenges of HAP and HAR works. Section 5 presents the discussion of the reviewed state of the
Although HAP and HAP tasks differ as illustrated in the Fig. 1, common methods may be used for sensory data collection. Vision data consist of a series of video frames as inputs and a processed output is usually a label that identifies the activity. In the paper [22], actions were recognized using static images. Many types of cameras are applied for data collection including RGB-D cameras and classical CCTV cameras. CCTV cameras observe the subject and the environment where the activity is being performed and deliver classical images. Activities are recognized using machine learning models [23, 24]. The data provided by the RGB-D camera is advantageous in the sense that it delivers several modalities such as RGB images, depth, audio, etc which improves the amount of extracted information and hence increases accuracy of the resulting predictions [18, 25]. In CCTV predictions accuracy is strongly dependent on lightning and background color, which is rather not the case in RGB-D. The depth information of RGB-D camera can be used to develop 3D skeleton-based HAP. Skeleton-based HAP and HAR use neural networks methods to
extract the skeleton joints position in the 3D scene from recorded RGB-D images. Some works [26–28] used RGB-D data for HAP and HAR, others used only the depth data [29–31] or the skeleton data [1, 32]. Vision-based systems are advantageous in the sense that they are not invasive. It can be tedious for a disabled person or elderly to wear sensors to record data. A single RGB-D camera can be used to replace several sensors. However, cameras raise concerns about privacy issues and can be hacked [33].
A wearable sensor device consists of a sensory set integrated in a wearable system or can be directly attached to the body to monitor human activity, e.g inertial sensors [16, 34–38]. Due to the stressful and invasive nature of attachable sensors, wearable sensor approaches are used more often. Examples include smart phones’ gyroscope and accelerometers, smart watches [17, 39–42]. Wearable sensory devices are advantageous because they offer higher privacy unlike cameras, and they can collect more accurate physiological data.
RFID is a wireless communication method that detects a body using electromagnetic or electrostatic coupling in the radio frequency portion of the electromagnetic spectrum. It consists of an RFID tag and a reader. Data is collected by placing the RFID tag close enough to the user, and a reader collects the data when the user is in the sensing range. RFID tags can be of passive or active type. Passive type uses the energy from the reader to operate, on the other hand, active tags operate with integrated battery. Compared to passive tags, the sensing range of active tags is often larger, however passive tags are more cost-effective [24]. During sensing, multiple RFID tags are attached to the moving parts of the body. During motion, radio frequency is constantly received by the reader antennae. The difference in the timings of signals reception from various tags is used to calculate the motion of the body i.e phase difference of the radio frequency signal caused by change in relative distance between antennae and tags during movement enable tracking of the body parts in 3D scenes. In the paper [43], an adaptive generalized ANN used RFID data to track human poses in real-time. Recorded RFID data is rather prone to noise created by moving people and objects, and data processing can be a challenging task because features extraction from noisy data is often required [44]. However, some researches decide to feed RFID signals directly to the processing algorithm for HAR [45], without any filtering. RFID systems are robust to dynamic environments, which implies they are flexible and adaptable to changing environmental setting e.g warehouses, manufacturing facilities, home environments, outdoor environments, etc.
Wi-Fi technology uses radio waves to transmit information through the air. It is often inexpensive and easy set up, which is a significant advantage. Researchers are working on using Wi-Fi devices to capture human activity data. Radio waves released by Wi-Fi transmitter are reflected by parts of the human body. Motion of humans and objects during HAR and HAP are sensed by tracking and analyzing the changes in the reflected signal caused by variation in wave propagation paths due to the movement. The data gathered using Wi-Fi transmission show high quality and are used in machine learning algorithms [46–48]. Data are organized in the form of channel state information (CSI) matrix, which holds information on properties of the communication signal such as phase shifts, power decay, etc. In the paper [49] Adaptive Activity Cutting
Age Algorithm (AACA) used amplitude information from the Wi-Fi device’s CSI to distinguish between body moving parts and non-moving parts during activity recognition. Results described in several other works such as [50, 51] showed the effectiveness of Wi-Fi devices in data acquisition for HAP and HAR applications. A limitation of Wi-Fi devices is their high sensitivity to dynamic environments [52].
Numerous datasets have been made publicly available for HAR and HAP research. Some examples of commonly used datasets are listed as follows; these datasets contain RGD-D videos of 4 people performing several activities in different settings e.g the living room, the office, and the kitchen, recorded using the Microsoft Kinect sensor. These activities are made of several atomic actions. The dataset consists of RGB images, depth images, and 3D skeleton data (x, y, z joints coordinates). CAD-60 [53] comprises of 60 RGB-D videos and CAD-120 [54] has 120 RGD videos. Some of the videos include: having a meal, drinking water, opening a pill container, stacking books, etc.
this dataset from Florence University contains 9 activities recorded using Kinect camera. There are 215 RGB-D videos in this dataset, activities were performed by 10 people. Some of the activities include; “answer the phone”, “read a watch”, “clap”, “stand up”, etc. The paper [55] used human skeleton data from Florence 3D dataset to recognize human actions based on joints positions.
machine learning methods often consider pixels from RGB videos as input data. A good example of dataset to be used that way is UFC50. It contains youtube videos grouped into 50 categories, where each category comprises of varying number of sample videos. Videos are realistic, with varying viewpoints, objects, illumination, etc, and are not staged like in the case of many other datasets [56]. Examples of activities in this dataset include; “horse riding”, “basketball”, “golf swing”, etc.
this dataset consists of grayscale videos of 25 individuals p erforming 6 activities in 4 different scenarios i.e “indoor”, “outdoor”, “outdoor with scale variation”, and “outdoor with different clothes” [57]. The variation in the scenarios enable the machine learning algorithm to be tested in the real world, which has varying scenes. Activity labels include “hand clapping”, “boxing”, “jogging”, “hand waving”, “walking”, “running”.
it is an action-focused dataset that consists of RGB video data grouped in 10 actions performed by 9 subjects [58]. The actions were recorded in a plain background using a fixed camera. Some of the actions include “bend”, “jumping jack”, “jump”, etc.
this dataset contains values of accelerations and angular velocities along x,y, and z axes recorded at a rate of 50Hz, using embedded accelerometer and gyroscope in a smart phone that was attached to the waist of the subject [59]. A total of 6 activities were performed by 30 people. The activities include “standing”, “laying”, ”walking”, “walking upstairs”, “walking downstairs”, and “sitting”.
the motion sense dataset like the UCI-HAR dataset was recorded using the accelerometer and gyroscope embedded in a smart phone [60]. The phone was placed in the subject’s front pocket to capture angular velocity and acceleration of 24 people performing 6 activities labeled “downstairs”, “upstairs”, “walking”, “jogging”, “sitting”, and “standing”. The dataset contains times series information of the user’s altitude, yaw, pitch, row, angular velocity, and linear acceleration along the x, y and z axes for every performed activity.
this is a Wi-Fi based human activity dataset that comprises of 16 activities p erformed in 3 indoor environments by 10 participants, with each participant executing an activity 30 times. The activities are classified into three categories: 1) upper body e.g high throw, toss paper, 2) lower body e.g forward kick, side kick, 3) whole body activities e.g squat, sit down. The Wi-Fi data consists of the Received Signal Strength Indicator (RSSI) and Channel State Information (CSI) [61]
It consists of 5 activities [62] carried out by 30 p eople. The activities were performed in open indoor environment as well as secluded indoor environments, since Wi-Fi signals can travel through walls. Activities include; “pick a pen from the ground”, “sit down and stand up”, “walking”, “falling from standing position”, “falling from sitting position”. in [63] consists of 245 action instances for seven different activities over 28 days. The activities are; “Leave House”, “Use Toilet”, “Take a Shower”, “Go to Bed”, “Prepare Breakfast”, “Prepare Dinner”, and “Get Drink”. These activities were recorded using RFID technology.
Once the data collection is completed, the next step involves processing the raw sensor data to make it usable by the machine learning algorithm. This is where preprocessing comes in. Good preprocessing of raw data is compulsory for high performance in HAP and HAR tasks [64]. Preprocessing steps typically depend on the type of data collected, but can generally be classified as the following: data cleaning, normalization, transformation, feature extraction, and feature selection [65]. Data cleaning process involves fixing and regularizing the raw data i.e taking care of duplicates, inconsistent, missing data in the dataset. This is done by identifying the faults and data adjustment, updating or deleting the errors to obtain a complete data stream. In the case where video frames are used as input data, data cleaning may include resizing each frame into constant equal dimensions. Normalizing the data is the stage of preprocessing whereby the numeric values of the dataset are scaled to a common range without distorting the relative difference among them or losing the information. An example will be a Gaussian distribution technique of normalization, or normalizing the pixel values by dividing each pixel with the maximum pixel value. This simplifies the computation effort during the training and testing phases. For accelerometer data preprocessing, data segmentation is necessary because inertial data changes greatly with time. Many studies consider segments of 1 to 10 seconds [66], nevertheless the length of segments depends on the sensor sampling rate and application context. Raw data segments are later on transformed into adequate formats according to the needs of the applied machine learning methods and tools [67].
Some transformation methods include the raw plot transformation, spectrograms [68], etc. A raw plot transformation encodes inertial data e.g acceleration data into images by scaling first the data into pixels assigning the values (e.g from range 0–255), then transforming each pixel to RGB channels pixels (e.g by segmentation of normalized data into 3 groups of integers) and finally extracting the color images representing the data. After such preprocessing the data are compatible with those used by machine learning algorithms [69]. A spectrogram is a type of data representation achieved by applying the Fourier transform on the segmented data, then computing the squared magnitude to obtain a representation of the input data as a function of time and frequency. A spectrogram representation reduces variations in sensor data due to changes in the position of the sensor, change of sampling rate, altitude, etc [67]. Another common aspect of preprocessing is dimensionality reduction. This involves selection of relevant features from large datasets to facilitate detection of correlations between the features hence reducing the complexity and computation
time without loss of important information. A commonly used method is Principal Component Analysis (PCA). PCA uses statistical approach by computing the eigen values and eigen vectors from the covariance matrix of the features. By ranking the eigen vectors according to their corresponding eigen values, the new features built from linear combinations of the original features can be obtained. Dimensionality reduction using laplacian eigenmaps is similar to PCA. They both emploi eigen vectors to obtain lower-dimensional data. However, PCA is a linear dimensionality reduction process, whereas Laplacian eigenmaps are based on non-linear data transformation [70]. Another approach is the Chi-square method, which uses the frequency distribution to assess the correlation between groups of features in order to select the best features. The recursive feature elimination technique proceeds by progressive elimination of features based on algorithm performance upon feeding the features, until the required number of features is obtained. Forward and backward features-selection is another method which operates by adding or discarding depending on their importance to the model performance. Feature selection algorithms are very efficient for more complex problems, capturing relevant information is not always guaranteed. Another option used by machine learning methods discussed in this work such as SVM, decision trees and probabilistic approaches commonly use hand-selected features. While hand-selected features help improve the interpretability of the method, it can be challenging in case of limited knowledge about the problem, and when dealing with complex model architectures. ANN-based methods on the other hand do not necessarily use hand-selected features or feature-selection algorithm, but automatically extract features from the input data using several layers of inter-connected nodes [71]. The final step of data preprocessing is the splitting of data into training and testing sets.
Human activities considered in this work are divided into three main groups based on the type of interaction involved in the activity namely; human-human interaction, human-object interaction, and human-machine interaction (Fig. 2). Recent advances in HAP enabled researchers to depict people in various settings and analyze their interactions with the environment. Nevertheless, there still exist a number of challenges [72], some of which include variations in environment conditions (illumination, background), prolonged and exhaustive data collection, and proper labeling of the data in order to identify and name the raw data, based on which the machine learning model will be trained. In HAP it is relevant to consider what the person interacts with. This knowledge helps to predict the activities especially if the prediction takes into account the situation context. For example, if a person takes the cup, he or she will most likely be drinking. The various types of human interactions are further described below.
interactions between humans are usually characterized by their body positions, motion, and coordination. Human interactions can be subdivided into motion sequences, for example, a handshake interaction may consist of hand lifting, grasping, etc [21]. On the other hand, an activity may be made up of many human interactions like “greetings” activity may involve alternative interactions for example “handshake”, “hug” etc. Understanding human-human interactions offer a possibility that these interactions can be predicted or even controlled. Interacting body parts are of the first importance during activity classification, therefore they are given greater weights during feature representation. Hence
(a) human-object interaction, (b)human-machine interaction
(c) human-human interaction
(photo by author)
Rys. 2. Przykłady interakcji uwzględnianych w HAP:
(a) interakcje człowiek-obiekt, (b) człowiek-maszyna,
(c) człowiek-człowiek
paying more attention to interacting body parts improves effectiveness when capturing motion characteristics in time and space of single and simultaneously occurring activities [73]. During feature representation, body parts that interact with other objects are given more weight.
these are activities in which humans interact with objects around them. The aim is to localize and generate a relationship between them. Prediction of a human-object interaction requires knowledge about objects (e.g for what they can be used), possible interactions [74]. Xu et al. [75] proposed a scene perception architecture that predicted human tasks which involve objects. A common approach for inferring human-object interaction is by taking into account the distance between body parts and the object when learning the relationship between an object and a human [76, 77]. these are activities in which people and automated systems interact with each other, e.g assistive robots and automated vehicles. Safety is a major issue during collaboration as the automated system has to be cautious of the end-user e.g how to navigate safely in the presence of humans, how to learn from user feedback [78]. Human-machine interaction can be remote (machine and human are separated in space and/or time), or proximate (shared human-robot environment). In the work [79] Tarik et al. Integrated HAR with robot trajectory planning so that a robot can perform the required task with high-level control by using recognized human activity as an input. It is often important that robots understand human activities in the tasks that require machine assistance, to be able to operate accordingly, hence the sensory data used in the AI algorithms must be of high quality. Figure 2 illustrates the above-discussed interactions.
The HAP process consists of 4 main stages which are: 1) data acquisition and preprocessing, 2) model development, 3) model evaluation, 4) prediction. Figure 3 illustrates these stages.
Many new approaches have been tested for HAP and HAR tasks. In this paper, we reviewed 4 of those approaches proposed in recent research papers namely: Artificial Neural Networks (ANN), Support Vector Machines (SVM), probabilistic approach, and decision trees.
this approach attempts to emulate the human brain neurons to enable computers to learn and make decisions in a human-like way. HAP methods involving ANN with visual data require adequate human-pose representation for effective performance. The most popular representations of joint angles use Euler angles as in [80] and some use quaternions [81], whose values are fed to the learning algorithm after preprocessing. Applied to HAP, ANN learns typically by taking inputs, iteratively adjusting the weights based on the error, and supplying outputs until minimal loss is observed. Anticipation of activity at any given time is dependent not only on the present state, but also on previous observations, hence it is critical to consider the temporal aspect of activity progression [82]. The Recurrent Neural Network (RNN) is an effective model for capturing activity progression. The paper [83] demonstrated a RNN real-time surveillance system to spot violent human activities in public environments using drones. It used hand-crafted features in order to accelerate RNN learning so that it can use these features to learn more complex patterns right from the start of training. The problem with this method arises when long-term dependency is involved. To address the aforementioned limitation, RNN-based Long Short Term Memory (LSTM) was introduced [84]. LSTM consist of a memory cell that can delete and add information over time. In this way, the neural network is able to capture correlations between the previous observations as well as hidden points to provide long-term context.
Deep learning is largely applied in HAP. It uses multiple layers of ANN to progressively process the data in order to extract higher level features from combinations of lower level features. Deep learning is advantageous in the sense that it performs automatic feature extraction, which is particularly useful when dealing with challenging datasets. The paper [85], proposed a Deep Neural Network (DNN) consisting of a combination of Convolutional Neural Networks (CNN), attention layers, LSTM, and softmax layers to improve the accuracy of predictions and for short-term predicting. The attention layer selectively concentrates on the important parts of the input sequence, while the softmax is the activation function that normalizes the outputs. The paper [86] compared their
Fig. 2. Examples of interaction types considered in HAP research works. Fig. 3. Stages of data processing in HAP methods Rys. 3. Etapy przetwarzania danych (HAP)LSTM-based neural network with the Naive Bayes method. In HAP, unlike the presented LSTM approach, the Naive Bayes classification method is based on conditional probabilities for prediction of human activities and does not learn the correlations between the features. The LSTM approach reported a significantly higher prediction accuracy compared to Naive Bayes method. Deep learning is well known for the ability to auto-perform feature extraction hence this makes it suitable for HAPs using several types of input data such as images, skeleton data, time series signals, etc [87–90]. A commonly used type of neural network in HAP is the Convolutional Neural Network (CNN), due to its strong pattern detection ability especially when working with visual data. CNN contains hidden convolutional layers that process data through convolution operations using filters. The filters extract features maps detecting patterns in the data [82]. In cases of processing volumetric data or a sequence of 2D frames, the filters move through 3 dimensions of the data i.e the length, width, and height [91]. These are referred to as 3D-CNN [92]. To improve performance, 3D-CNN and 2D-CNN integration (MiCT) was proposed in the paper [93]. The spatio-temporal information for HAP was fed to 3D-CNN and 2D-CNN in parallel. Feature maps generated by each module were added together to obtain deeper feature maps for higher efficiency.
Data representation for HAP sometimes is expressed as graphs due to the highly expressive nature of graph data structure [94]. Relationships between features are then easier to understand, which allows application of Graph Neural Networks (GNN). GNN is a deep learning model that operates on graph data structure. A graph consists of nodes that interconnect through edges. Graph Convolutional Networks(GCN) is a specific type of GNN that aggregate data from neighborhood nodes in a convolutional manner. GCN uses the idea of nodes embedding. Nodes embedding involves mapping nodes to a d-dimensional embedding space, lower than the graph dimension. The nodes are mapped in such a way that the network similarity and embedded space similarity are roughly equivalent. GNN in general, has achieved high performance in HAP applications, what is advantageous is that it can perform the tasks that CNN failed to perform. Some ANN-based works include a real-time prediction of pedestrian behavior achieved in [95]. Here the mobile robots adjusted their trajectories both in time and space, and showed proactive behavior like waiting, in order to provide priority passage for pedestrians. In [96] was presented a two-stage HAR, where the first CNN discriminated between two main classes “dynamic” and “static”. The second CNN was used for recognition of activities within these main classes. A drawback of neural networks is their inability to express uncertainty. To address this issue, it was proposed in [97] the Bayesian neural network to provide estimates of prediction uncertainty. It consisted of the RNN that predicted the distribution of future states of the activity, and the Bayesian network that estimated the uncertainty associated with the predictions.
: the SVM approach is a classification method. One of the most widely used in HAP is structured SVM [98]. SVMs are supervised learning methods that work by introducing a hyperplane with a maximum margin that separates data into various classes. Maximizing the margin distance enables better classification accuracy.
The paper [99] presented an SVM framework that learned model parameters from a structured hierarchical representation of human activities called moveme. The SVM algorithm was trained to focus on human movements before the action was completed by cutting out the tracks in which the person makes contact e.g hug, handshake. In this way, training is done using the tracks just before contact is made therefore the algorithm focuses solely on modeling people’s movements
prior to action execution. The framework captured detailed characteristics of human activity that may imply a future action across frames, allowing activity prediction after observing any frame of the video, rather than using the first couple of frames as in many other works. In the paper [100], Haoran et al. suggested that performing HAP from videos didn’t necessarily require using full frames from the video sample. To demonstrate this, an SVMbased early activity prediction approach was used to operate on the selected features from the keyframes of input videos. The features were compared with the activities class keyframes. Each sample video went through a key frame selection process that involved obtaining action units by tracking specific human movement across video frames. The entropy of each frame in the training video was computed, and low entropy frames were chosen as class keyframes. The structured SVM based on keyframes was developed to predict the activity class from partial observation. Some action sequences can look similar. For example, punch and push movements have many similar action sequences e.g “reach”, “retract”. This makes it difficult to differentiate between the action units. To address the issue Kong et al. [101] presented a structured SVM-based action prediction method that incorporated composite kernels to capture nonlinear classification boundaries when classifying the activities. The kernels transformed the training data so that the previously nonlinear classification boundaries transform into linear boundaries with higher dimensions, to facilitate linear separation of the data for more efficient activity classification. probabilistic methods apply statistical principles for data analysis. Although being one of the earliest forms of machine learning approaches, it is still widely used by modern researches [102]. Probabilistic approaches aim at modeling the conditional probability of variables given data distribution. One of the most popular algorithms in this class is the “Naive Bayes Classifier” [103], which is based on Bayesian reasoning. It is called “Naive” because all features are assumed to contribute independently and equally to the outcome, i.e. no pair of features is dependent. Bayesian reasoning describes the probability that a future event will occur given prior knowledge of the probability of some other event that has occurred. The Markov’s model approach is another common probability-based HAP approach [104]. It assumes that at any given time in a randomly changing system, the next state is only dependent on the current state and is independent of anything in the past (1st order Markov model). An extension to the Markov model is the variable-length Markov model where the prediction of a future state depends on a fixed number of past states, with each state representing a sequence of values that may vary in length from one state to another [105]. Two mainly applied types of Markov’s models include the Markov Chain and the Hidden Markov Model (HMM). Markov chains represent all system states as well as transition rates, whereas HMM is used in systems where some states are hidden, i.e. not observed. Hidden Markov Model (HMM) works well with sequential data, which is common in human activity prediction. As a result, many HMM-based methods have been proposed. A HMM-based human-machine interaction system was proposed in the work [7], whereby the user performed complex activities comprised of multiple sub-events involving various objects. The machine analyzed previously performed sub-events to predict which sub-events the user would need to complete the task, and it provided feedback to assist the user in completing the task effectively. The HMM was used to represent the sub-events in chronological order. The user’s hand and the objects involved in the actions were tracked, and the relative distance between the hand and the objects were used to predict future actions.
Have better fault tolerance.
Network slows over learning time, and undergoes slower rate degradation.
This network is a black box, i.e there is lack of transparency, hence difficult to trust. Require a lot of data for training.
ANN
They have numerical strength, i.e they can perform more than one job at the same time. They can work with inadequate knowledge, i.e they may produce output even with inadequate info. It all depends on how important the missing information is. Use of hierarchical features simplifies the learning process.
Deep learning simplicity removes the need for feature engineering which was a complex task. Versatile nature of model make them usable for additional data, which is great for online learning’
They are reusable, e.g using an image classification for video processing, processing images for smart homes
Poor at representing uncertainty.
Easily fooled by adversarial examples.
SVM
Probabilistic
Very performant where there is a clear margin of separation between classes. More effective in high dimensional spaces. It is relatively memory efficient.
Comparatively less suitable for large datasets. Do not perform very well where datasets have more noise.
Classifies data on each side of an introduced margin boundary, no probabilistic explanation for classification.
Provide an idea about the uncertainty associated with the prediction.
Ability to start off with relatively less data compared to data needed for other machine learning methods
Offer much easier explainability how the output came about.
Time-consuming and error-prone. Many probability methods assume predictions are independent, which hardly happens in realworld cases. Higher complexity compared to other methods Probability outputs estimations can be wrong in some cases.
Decision trees
Can very well perform both regression and classification which are both relevant in HAP. Can handle large datasets effectively, Level of accuracy of predictions is relatively high. Faster to train and resistant to overfitting. Can work with missing data by creating estimates for them.
Outputs the importance of features, hence variables with positive impacts can be determined.
Activities belonging to the same classes considered during HAP are often regarded to occur with the same time sequence. While this is a fair assumption, in real-life applications this is not always the case. To remedy this [72] proposed an approach that handled activities with varying speed and duration using histogram of oriented velocity. Hence in this context, it is important to estimate the duration of the human activities as proposed by [106], in which a framework prediction takes into account duration of the current and future actions. The paper [6] proposed a real-time simulation for human activity identification and prediction, based on the probability of the human body intersections with the bounding colliders defined in the working space. Wearable sensors tracked body movements during human activity. When the hand intersected with the bounding collider, the action was classified using the body joints, hand positions, and corresponding bounding box. The
Large number of trees slow down the algorithm, this can be ineffective for real-time predictions. Require much computational power due to numerous trees.
Difficult to interpret and fails to determine the significance of each variable i.e it is predictive, not descriptive.
In cases where the predictors and outcome have a non-linear relationship, accuracy will be affected. A small change in data may considerably change the algorithm performance
predictions were then made by calculating the probability of the hand intersecting with a bounding box. The paper [107] used Probability Suffix Tree (PST), which is a pattern-matching technique, to model the relationship between sub-actions in an activity. The proposed approach formulated a probabilistic function that used causality reasoning and predictability parameters to predict the activity class associated with an ongoing sub-action. Each PST branch from the root to the leaves represented an activity class, with the nodes representing the probability distribution after a sub-action is executed.
A Predictive Accumulative Function (PAF) generated predictability parameter which provided information on how early in the activity execution can predictions be made with satisfactory accuracy. Based on this, activities were considered as either early predictable or late predictable. PST was also used in [8] to model the relationship between actions. Each action
Table 1. Advantages and disadvantages of HAP methods Tabela 1. Zalety i wady metod HAPcontained information about the object, the hand position, and the type of interaction taking place. The proposed HAP framework took into account the relationships between subaction as well their temporal dependencies. First, the future action sequences were predicted using conditional probabilities, and the second part involved predicting the waiting time to execute a new action which was predicted using information of the starting time of each action, duration of each action and the sum of all intermediate action durations.
decision trees are a type of non-parametric machine learning method that can be used for classification problems such as HAP [103]. It is a hierarchical system comprised of the root node, branches, internal nodes, and leaf nodes. The nodes represent the categorical features. Decision trees are applying simple decision rules based on which the tree branches are formed. Predictions are made using the majority voting rule, for multiple trees models. A decision tree-based approach to classifying human activities was proposed in the work [108]. The decision tree uses information gain to determine how relevant is a feature in determining the splitting criteria. Reduced Error Pruning (REP) was used to reduce misclassification and overfitting. REP is a bottom-up pruning approach to optimize the size of decision trees by removing non-relevant branches, which results in decreased complexity and increased accuracy.
Random Forest is a popular ensemble ML technique that combines several decision trees to improve the prediction accuracy [109]. Because HAP is a complex problem therefore learning algorithms should use a cost-effective classification learner [110]. This is offered in the Random Forest approach. The paper [111] proposed a Random Forest-based algorithm called Multi-class Balanced Random Forest (MBRF) that could predict multiple human activities simultaneously due to its ability to be scalable to multiple classes of activities. For each video frame, the approach used spatio-temporal features of defined points of interest adapted from the work [112]. To make the predictions, the similarity score was computed based on the features description. The center point location of the frame and the spatio-temporal location of the interest points with respect to the center point of the frame were taken into account. The frames with the same center point location were considered to belong to the same video. The classical support vector machine (SVM) requires solving a large quadratic programming problem with a square objective function (QPP) and linear constraints. The objective function takes into account the distance between the hyperplanes that determine the classification result. In [113], a large objective function requiring a significant computational time was divided into smaller quadratic problems according to the SMO concept. The method was combined with the Random-Forest algorithm. Minor quadratic programming problems were iteratively solved until the solution convergence criterion was met, which means obtaining a global solution. This approach was used for initial classification, and next the Random-Forest algorithm was applied. The Random Forest algorithm combines the output of multiple (randomly generated) decision trees to produce a classification score. A large number of partial decision trees are formed here. The winning classes are determined by the majority of votes. Unfortunately, the decision trees are sensitive to the data used for training. If the training data changes, the winning decision tree may be different, which means the classification result may also be different. So some change in a small amount of training data can dramatically change the forecast, even though on the scale of the full dataset such a change is marginal. One way to overcome this disadvantage is to use bootstrap aggregation (bagging), as discussed in [113]. In practice, bagging means using the
average of some sets of independent data samples instead of using them independently. This reduces the dimensionality of the data and prevents overfitting of the classification, but on the other hand, it has a negative impact on the interpretability of the results.
Overall, machine learning methods for HAP and HAR are quite similar. The difference in these methods is the fact that HAR methods focus on recognising already performed tasks, while HAP focus on future tasks. In HAP, building the model considers the context of past and present activities, this can make HAP models more complex. During training, the model learns patterns and relationships among various features, and also between features and outputs. Based on these, predictions about future activities are made.
As it was already mentioned HAP and HAR require data gathering, development and application of data processing method and finally testing. Therefore, we can infer that the leading challenges encountered in the HAP and HAR are associated with algorithms and datasets. Some of these challenges are listed below.
learning algorithms learn are using examples like a human being. But unlike the humans, large datasets are required for effective training, even for simple problems. In the paper [113] it was concluded that the significance of data surpasses the relevance of algorithms for complex problems. However, obtaining large datasets is difficult, expensive and time consuming. Nevertheless, today’s researchers still obtain relatively acceptable results using small and medium datasets.
HAP methods are not only affected by the quantity of data, but also quality of data. The work [114] researched the influence of data quality on machine learning algorithms, and it proved that data quality affects outcomes in significant ways therefore more attention should be given to data collection (experiments). It is challenging to have clean datasets without noise, errors, missing data, etc. Data therefore must be preprocessed before training. This is rather challenging task as data preprocessing methods are not predefined and depend on the nature of the problem.
HAP methods which use artificial intelligence and especially neural networks have chances of falling into the trap of overfitting when finding underline patterns in the data, and they cannot also find real fitting due to noisy data. This often happens especially to complex cases. This challenge can be addressed by using simpler approaches to data processing. On the other hand, underfitting is also a problem which should be approached by features processing methods.
the system needs enough amount of relevant features to perform well. Very large datasets also make it difficult to find relationships between features [115]. This is where feature engineering comes to play. Therefore dimensionality reduction algorithms like Principal Component Analysis (PCA) produce more useful but reduced sets of features [116]. there might be a challenge when applying machine learning algorithms for predicting activities as different persons often vary in the way they perform activities. Moreover, human behaviour may also vary with time and place, i.e the same subject may perform the same activity in a slightly different manner when the environment is changed, or after a considerable period of time. These changes cause variations in datasets and hence make reasoning more challenging.
activities that are very similar turn to have similar sensor readings. As a result, the accuracy of the predictions may be degraded in such cases.
Recent advancements in machine learning methods for HAR and HAP have brought questions regarding which methods are more efficient, cost-effective, and time-saving. There is no direct answer to this question as every method is unique in its own way. Based on the various research works reviewed in this paper, it is clear that the performance of the HAP and HAR experiments vary due to the methodologies used, the dataset, and activity representations. Thus all of the techniques discussed have advantages and disadvantages. In the paper [117] authors aimed at finding the best machine learning algorithm for human activity prediction considering Random Forest, ANN, SVM, Naive Bayes and decision trees. According to their results, the Random Forest approach offered the highest prediction accuracy. However one can argue that the accuracy can be affected by changing the values of parameters, dataset sampling method, features selection and dimensionality reduction method, which can lead to another method showing the best accuracy. Table 1 describes the advantages and disadvantages of the discussed methods. The goal of all
of the preceding techniques is to predict the activities prior to completion, so they are sensitive to prior knowledge. Deep learning is one of the most recent trends in HAP. Deep learning typically outperforms other traditional ML techniques due to its ability, unlike other methods, to extract features from raw data. However, it has a high computational cost, which is currently a rather serious limitation.
It is worth noting that the aforementioned HAP approaches are based on low-level feature representations. Researchers have found that some type of high-level representations of human complex activities called “semantic features” can be used in HAP and HAR. Semantic features are attributes that describe distinctive characteristics of the activity at a higher level. Applying these attributes improves the accuracy of predictions, particularly for activities belonging to the same class, but consisting of visually different actions caused by its execution variations [119]. While low-level features are useful for HAPs, prediction algorithms cannot anticipate “untrained” actions i.e the algorithms cannot predict an activity not seen before. To address this issue, Cheng et al. [120] proposed to manually design semantic features that represent human acti-
vities on a higher concept. During training, each activity is characterized by the absence or presence of semantic attributes, and a classification algorithm is used to recognize the labeled characteristics corresponding to each activity. However, establishing the semantic attributes manually would be arduous, expensive, and time-consuming. To solve this problem, [121] suggested an approach based on deep learning techniques known as restricted Boltzmann machines (RBMs) and Deep Belief Networks (DBNs) to assign semantic attributes. Semantic attributes obtained by this technique showed high relevance when compared to manually assigned ones. Other methods used for capturing semantic features, include Beta-Bernoulli Process Restricted Boltzmann Machines [122], Diffusion Maps embedding [123], Markov Semantic Model [124], etc. For the view of the contents of this paper, figure 4 gives the systematic view of HAR and HAP issues.
HAP and HAR are active research and development fields which have a vast area of potential directions in the future. To mention a few; it could be focused on improving the accuracy and reliability of already existing methods of HAP and HAR be it by developing new algorithms, using better features extraction techniques, etc. Further, it could be focused for improved integration with other technologies e.g integrating sensors, cameras and other devices for higher performance and comprehensive activity tracking. Another potential future focus of HAP and HAR research could be on increasing real-world applications such as healthcare, sports, transport. With the increase in accuracy and reliability of the HAP and HAR technologies, it is likely to be used in various real-world applications.
While human activity recognition yields many promising results and is much more explored by researchers, human activity prediction is a growing area with promising poten-
tial. We reviewed 4 common types of activity prediction methods namely; ANN, SVM, probabilistic method, and decision trees. We shortly described sensor devices and datasets as well. This gives a general picture of the state of the art. It is observed that researchers have tried various techniques or approaches for better prediction of human activities using various sources of data like RGB images, depth images, RFID signals, Wi-Fi signals and data delivered by wearable sensors such as accelerations, velocities and orientation angles. Wi-Fi and RFID sensors have comparatively fewer applications due to their high flexibility to interferences. The wearable sensors on the other hand fail to sense the state of motionless objects. Hence the more common sensing technique use visual sensors, mostly RGB data. The recent advances and new ideas were summarized. Analyzing the state of the art methods of HAP enables a better understanding of steps involved in them, hence increasing the possibility of identifying efficient techniques to achieve needed performance. Table 2 gives the list of the most representative methods with their prediction accuracy. According to this table, ANN-based prediction can be concluded as the most popular and outperforming the other techniques.
1. Chiu H.-K., Adeli E., Wang B., Huang D.-A., Niebles J.C., Action-Agnostic Human Pose Forecasting, [In:] 2019 IEEE Winter Conference on Applications of Computer Vision (WACV), IEEE, 2019, 1423–1432, DOI: 10.1109/WACV.2019.00156.
2. Coppola C., Faria D.R., Nunes U., Bellotto N., Social Activity Recognition Based on Probabilistic Merging of Skeleton Features with Proximity Priors from RGB-D Data, [In:] 2016 IEEE/RSJ International Conference on Intelligent Robots and Systems (IROS), IEEE, 2016, 5055–5061, DOI: 10.1109/IROS.2016.7759742.
3. Ravichandar H.C., Trombetta D., Dani A.P., Human Intention-Driven Learning Control for Trajectory Synchronization in Human-Robot Collaborative Tasks, „IFAC-PapersOnLine”, Vol. 51,No. 34, 2019, 1–7 DOI: 10.1016/j.ifacol.2019.01.001.
4. Razali H., Mordan T., Alahi A., Pedestrian Intention Prediction: A Convolutional Bottom-Up Multi-Task Approach, „Transportation Research Part C: Emerging Technologies”, Vol. 130, 2021, 103259, DOI: 10.1016/j.trc.2021.103259.
5. Bibi S., Anjum N., Amjad T., McRobbie G., Ramzan N., Human Interaction Anticipation by Combining Deep Features and Transformed Optical Flow Components, „IEEE Access”, Vol. 8, 2020, 137646–137657, DOI: 10.1109/ACCESS.2020.3012557.
6. Manns M., Tuli T.B., Schreiber F., Identifying Human Intention During Assembly Operations using Wearable Motion Capturing Systems Including Eye Focus, „Procedia CIRP”, Vol. 104, 2021, 924–929, DOI: 10.1016/j.procir.2021.11.155.
7. Ryoo M.S., Grauman K., Aggarwal J.K., A task-Driven Intelligent Workspace System to Provide Guidance Feedback, „Computer Vision and Image Understanding”, Vol. 114, No. 5, 2010, 520–534, DOI: 10.1016/j.cviu.2009.12.009.
8. Casalino A., Massarenti N., Zanchettin A.M., Rocco P., Predicting the Human Behaviour in Human-Robot Co-Assemblies: an Approach Based on Suffix Trees, [In:] 2020 IEEE/RSJ International Conference on Intelligent Robots and Systems (IROS), IEEE, 2020, 11108–11114, DOI: 10.1109/IROS45743.2020.9341301.
9. Ding H., Shangguan L., Yang Z., Han J., Zhou Z., Yang P., Xi W., Zhao J., FEMO: A Platform for Free-Weight Exercise Monitoring with RFIDs, [In:] Proceedings of the 13th ACM Conference on Embedded Networked Sensor Systems, 2015, 141–154, DOI: 10.1145/2809695.2809708.
10. Kulic D., Croft E.A., Affective State Estimation for Human-Robot Interaction, „IEEE Transactions on Robotics”, Vol. 23, No. 5, 2007, 991–1000 DOI: 10.1109/TRO.2007.904899.
11. Vaniya S.M., Bharathi B., Exploring Object Segmentation Methods in Visual Surveillance for Human Activity Recognition, [In:] 2016 International Conference on Global Trends in Signal Processing, Information Computing and Communication (ICGTSPICC), IEEE, 2016, 520–525, DOI: 10.1109/ICGTSPICC.2016.7955356.
12. Abobakr A., Hossny M., Nahavandi S., A Skeleton-Free Fall Detection System from Depth Images using Random Decision Forest, „IEEE Systems Journal”, Vol. 12, No. 3, 2017, 2994–3005, DOI: 10.1109/JSYST.2017.2780260.
13. Bandi C., Thomas U., Skeleton-Based Action Recognition for Human-Robot Interaction using Self-Attention Mechanism, [In:] 2021 16th IEEE International Conference on Automatic Face and Gesture Recognition (FG 2021), IEEE, 2021, DOI: 10.1109/FG52635.2021.9666948.
14. Li B., Tian J., Zhang Z., Feng H., Li X., Multitask Non-Autoregressive Model for Human Motion Prediction, „IEEE Transactions on Image Processing”, Vol. 30, 2020, 2562–2574 DOI: 10.1109/TIP.2020.3038362.
15. Li M., Chen S., Chen X., Zhang Y., Wang Y., Tian Q., Symbiotic Graph Neural Networks for 3D Skeleton-Based Human Action Recognition and Motion Prediction, „IEEE Transactions on Pattern Analysis and Machine Intelligence”, Vol. 44, No. 6, 2022, 3316–3333, DOI: 10.1109/TPAMI.2021.3053765.
16. Pham C., Nguyen-Thai S., Tran-Quang H., Tran S., Vu H., Tran T.-H., Le T.-L., SensCapsNet: Deep Neural Network
for Non-Obtrusive Sensing Based Human Activity Recognition, „IEEE Access”, Vol. 8, 2020, 86934–86946, DOI: 10.1109/ACCESS.2020.2991731.
17. Zhu R., Xiao Z., Li Y., Yang M., Tan Y., Zhou L., Lin S., Wen H., Efficient Human Activity Recognition Solving the Confusing Activities via Deep Ensemble Learning, „IEEE Access, Vol. 7, 2019, 75490–75499, DOI: 10.1109/ACCESS.2019.2922104.
18. Wang K., He J., Zhang L., Attention-Based Convolutional Neural Network for Weakly Labeled Human Activities’ Recognition with Wearable Sensors, „IEEE Sensors Journal”, Vol. 19, No. 17, 2019, 7598–7604, DOI: 10.1109/JSEN.2019.2917225.
19. Gupta N., Gupta S.K., Pathak R.K., Jain V., Rashidi P., Suri J.S., Human Activity Recognition in Artificial Intelligence Framework: A Narrative Review, „Artificial Intelligence Review”, Vol. 55, 2022, 4755–4808, DOI: 10.1007/s10462-021-10116-x.
20. Abdussami S., Nagendraprasad S., Shivarajakumara K., Singh S., Thyagarajamurthy A., A Review on Action Recognition and Action Prediction of Human(s) using Deep Learning Approaches, „International Journal of Computer Applications”, Vol. 177, No. 20, 2019, DOI: 10.5120/ijca2019919605.
21. Razin Y.S., Pluckter K., Ueda J., Feigh K., Predicting Task Intent from Surface Electromyography using Layered Hidden Markov Models, „IEEE Robotics and Automation Letters”, Vol. 2, No. 2, 2017, 1180–1185, DOI: 10.1109/LRA.2017.2662741.
22. Yao B., Fei-Fei L., Action Recognition with Exemplar Based 2.5D Graph Matching, [In:] European Conference on Computer Vision, Springer, 2012, 173–186, DOI: 10.1007/978-3-642-33765-9_13.
23. Diba A., Fayyaz M., Sharma V., Paluri M., Gall J., Stiefelhagen R., Gool L.V., Large Scale Holistic Video Understanding, [In:] European Conference on Computer Vision, Springer, 2020, 593–610, DOI: 10.48550/arXiv.1904.11451.
24. Du Y., Lim Y., Tan Y., A Novel Human Activity Recognition and Prediction in Smart Home Based on Interaction, „Sensors”, Vol. 19, No. 20, 2019, DOI: 10.3390/s19204474.
25. Phyo C.N., Zin T.T., Tin P., Deep Learning for Recognizing Human Activities using Motions of Skeletal Joints, „IEEE Transactions on Consumer Electronics”, Vo. 65, No. 2, 2019, 243–252, DOI: 10.1109/TCE.2019.2908986.
26. Mici L., Parisi G.I., Wermter S., Recognition and Prediction of Human-Object Interactions with a Self-Organizing Architecture, [In:] 2018 International Joint Conference on Neural Networks (IJCNN), IEEE, 2018, DOI: 10.1109/IJCNN.2018.8489178.
27. Schydlo P., Rakovic M., Jamone L., Santos-Victor J., Anticipation in Human-Robot Cooperation: A Recurrent Neural Network Approach for Multiple Action Sequences Prediction, [In:] 2018 IEEE International Conference on Robotics and Automation (ICRA), IEEE, 2018, DOI: 10.1109/ICRA.2018.8460924.
28. Dixon S., Hansen R., Deneke W., Probabilistic Grammar Induction for Long Term Human Activity Parsing, [In:] 2019 International Conference on Computational Science and Computational Intelligence (CSCI), IEEE, 2019, 306–311, DOI: 10.1109/CSCI49370.2019.00061.
29. Duckworth P., Hogg D.C., Cohn A.G., Unsupervised Human Activity Analysis for Intelligent Mobile Robots, „Artificial Intelligence”, Vol. 270, 2019, 67–92, DOI: 10.1016/j.artint.2018.12.005.
30. Fang L., Liu X., Liu L., Xu H., Kang W., JGR-P2O: Joint Graph Reasoning Based Pixel-to-Offset Prediction Network for 3D Hand Pose Estimation from a Single Depth Image,
[In:] European Conference on Computer Vision, Springer, 2020, 120–137, DOI: 10.1007/978-3-030-58539-6_8.
31. Hu J.-F., Zheng W.-S., Ma L., Wang G., Lai J., Zhang J., Early Action Prediction by Soft Regression, „IEEE Transactions on Pattern Analysis and Machine Intelligence”, Vol. 41, No. 11, 2018, 2568–2583, DOI: 10.1109/TPAMI.2018.2863279.
32. Li M., Chen S., Liu Z., Zhang Z., Xie L., Tian Q., Zhang Y., Skeleton Graph Scattering Networks for 3D Skeleton-Based Human Motion Prediction, [In:] Proceedings of the IEEE/CVF International Conference on Computer Vision, 2021, 854–864, DOI: 10.1109/ICCVW54120.2021.00101.
33. Ranasinghe S., Al Machot F., Mayr H.C., A Review on Applications of Activity Recognition Systems with Regard to Performance and Evaluation, „International Journal of Distributed Sensor Networks”, Vol. 12, No. 8, 2016, DOI: 10.1177/1550147716665520.
34. Xia K., Huang J., Wang H., LSTM-CNN Architecture for Human Activity Recognition, „IEEE Access”, Vol. 8, 2020, 56855–56866, DOI: 10.1109/ACCESS.2020.2982225.
35. Saha J., Ghosh D., Chowdhury C., Bandyopadhyay S., Smart Handheld Based Human Activity recognition using Multiple Instance Multiple Label Learning, „Wireless Personal Communications”, Vol. 117, No. 2, 2021, 923–943, DOI: 10.1007/s11277-020-07903-0.
36. Zhou X., Liang W., Kevin I., Wang K., Wang H., Yang L.T., Jin Q., Deep-Learning-Enhanced Human Activity Recognition for Internet of Healthcare Things, „IEEE Internet of Things Journal”, Vol. 7, No. 7, 2020, 6429–6438, DOI: 10.1109/JIOT.2020.2985082.
37. Chen J., Sun Y., Sun S., Improving Human Activity Recognition Performance by Data Fusion and Feature Engineering, „Sensors”, Vol. 21, No. 3, 2021, DOI: 10.3390/s21030692.
38. Tian Y., Zhang J., Chen L., Geng Y., Wang X., Single Wearable Accelerometer-Based Human Activity Recognition via Kernel Discriminant Analysis and QPSO-KELM Classifier, „IEEE Access”, Vol. 7, 2019, 109216–109227, DOI: 10.1109/ACCESS.2019.2933852.
39. Yao S., Zhao Y., Zhang A., Hu S., Shao H., Zhang C., Su L., Abdelzaher T., Deep Learning for the Internet of Things, „Computer”, Vol. 51, No. 5, 2018, 32–41, DOI: 10.1109/MC.2018.2381131.
40. Garcia-Gonzalez D., Rivero D., Fernandez-Blanco E., Luaces M.R., A Public Domain Dataset for Real-Life Human Activity Recognition using Smartphone Sensors, „Sensors”, Vol. 20, No. 8, 2020, DOI: 10.3390/s20082200.
41. Lawal I.A., Bano S., Deep Human Activity Recognition using Wearable Sensors, [In:] Proceedings of the 12th ACM International Conference on PErvasive Technologies Related to Assistive Environments, 2019, 45–48, DOI: 10.1145/3316782.3321538.
42. Bashar S.K., Al Fahim A., Chon K.H., Smartphone Based Human Activity Recognition with Feature Selection and Dense Neural Network, [In:] 2020 42nd Annual International Conference of the IEEE Engineering in Medicine & Biology Society (EMBC), IEEE, 2020, 5888–5891, DOI: 10.1109/EMBC44109.2020.9176239.
43. Yang C., Wang X., Mao S., RFID-Based 3D Human Pose Tracking: A Subject Generalization Approach, „Digital Communications and Networks”, Vol. 8, No. 3, 2022, 278–288, DOI: 10.1016/j.dcan.2021.09.002.
44. Rohei M.S., Salwana E., Shah N.B.A.K., Kakar A.S., Design and Testing of an Epidermal RFID Mechanism in
a Smart Indoor Human Tracking System, „IEEE Sensors Journal”, Vol. 21, No. 4, 2021, 5476–5486. DOI: 10.1109/JSEN.2020.3036233.
45. Li X., Zhang Y., Marsic I., Sarcevic A., Burd R.S., Deep Learning for RFID-Based Activity Recognition, [In:] Proceedings of the 14th ACM Conference on Embedded Network Sensor Systems CD-ROM, 2016, pp. 164–175, DOI: 10.1145/2994551.2994569.
46. Lin G., Jiang W., Xu S., Zhou X., Guo X., Zhu Y., He X., Human Activity Recognition using Smartphones With WiFi Signals, „IEEE Transactions on Human-Machine Systems”, 2022, 1–12, DOI: 10.1109/THMS.2022.3188726.
47. Yang J., Chen X., Wang D., Zou H., Lu C.X., Sun S., Xie L., Deep Learning and Its Applications to WiFi Human Sensing: A Benchmark and A Tutorial, arXiv preprint arXiv:2207.07859 (2022).
48. Mei Y., Jiang T., Ding X., Zhong Y., Zhang S., Liu Y., WiWave: WiFi-based Human Activity Recognition using the Wavelet Integrated CNN, [In:] 2021 IEEE/CIC International Conference on Communications in China (ICCC Workshops), IEEE, 2021, 100–105.
49. Yan H., Zhang Y., Wang Y., Xu K., WiAct: A Passive WiFi-Based Human Activity Recognition System, „IEEE Sensors Journal”, Vol. 20, No. 1, 2019, 296–305, DOI: 10.1109/JSEN.2019.2938245.
50. Fei H., Xiao F., Han J., Huang H., Sun L., Multi-Variations Activity Based Gaits Recognition using Commodity WiFi, „IEEE Transactions on Vehicular Technology”, Vol. 69, No. 2, 2020, 2263–2273, DOI: 10.1109/TVT.2019.2962803.
51. Ding X., Jiang T., Zhong Y., Wu S., Yang J., Zeng J., Wi-Fi-Based Location-Independent Human Activity Recognition with Attention Mechanism Enhanced Method, „Electronics”, Vol. 11, No. 4, 2022, DOI: 10.3390/electronics11040642.
52. Li J., Jiang T., Yu J., Ding X., Zhong Y., Liu Y., An WiFi-Based Human Activity Recognition System Under Multi-source Interference, [In:] International Conference in Communications, Signal Processing, and Systems, Springer, 2022, 937–944, DOI: 10.1007/978-981-19-0390-8_118.
53. Sung J., Ponce C., Selman B., Saxena A., Unstructured Human Activity Detection from RGBD Images, [In:] 2012 IEEE International Conference on Robotics and Automation, IEEE, 842–849, DOI: 10.1109/ICRA.2012.6224591.
54. Koppula H., Saxena A., Learning Spatio-Temporal Structure from RGB-D Videos for Human Activity Detection and Anticipation, [In:] International Conference on Machine Learning, PMLR, 2013, 792–800.
55. Seidenari L., Varano V., Berretti S., Bimbo A., Pala P., Recognizing Actions from Depth Cameras as Weakly aligned multi-part bag-of-Poses, [In:] Proceedings of the IEEE Conference on Computer Vision and Pattern Recognition Workshops, 2013, 479–485, DOI: 10.1109/CVPRW.2013.77.
56. Reddy K.K., Shah M., Recognizing 50 Human Action Categories of Web Videos, „Machine Vision and Applications”, Vol. 24, No. 5, 2013, 971–981, DOI: 10.1007/s00138-012-0450-4.
57. Schuldt C., Laptev I., Caputo B., Recognizing Human Actions: a Local SVM Approach, [In:] Proceedings of the 17th International Conference on Pattern Recognition, 2004. ICPR 2004., Vol. 3, IEEE, 2004, 32–36, DOI: 10.1109/ICPR.2004.1334462.
58. Blank M., Gorelick L., Shechtman E., Irani M., Basri R., Actions as Space-Time Shapes, [In:] Tenth IEEE Interna-
tional Conference on Computer Vision (ICCV’05), Vol. 1, Vol. 2, IEEE, 2005, 1395–1402, DOI: 10.1109/ICPR.2004.1334462.
59. Anguita D., Ghio A., Oneto L., Parra Perez X., Reyes Ortiz J.L., A Public Domain Dataset for Human Activity Recognition using Smartphones, [In:] Proceedings of the 21th International European Symposium on Artificial Neural Networks, Computational Intelligence and Machine learning, 2013, 437–442.
60. Malekzadeh M., Clegg R.G., Cavallaro A., Haddadi H., Mobile Sensor Data Anonymization, [In:] Proceedings of the International Conference on Internet of Things Design and Implementation, 2019, 49–58, DOI: 10.1145/3302505.3310068.
61. Guo L., Wang L., Lin C., Liu J., Lu B., Fang J., Liu Z., Shan Z., Yang J., Guo S., Wiar: A Public Dataset for Wifi-Based Activity Recognition, „IEEE Access”, Vol. 7, 2019, 154935–154945, DOI: 10.1109/ACCESS.2019.2947024.
62. Baha’A A., Almazari M.M., Alazrai R., Daoud M.I., A Dataset for Wi-Fi-Based Human Activity Recognition in Line-Of-Sight and Non-Line-Of-Sight Indoor Environments, „Data in Brief”, Vol. 33, 2020, DOI: 10.1016/j.dib.2020.106534.
63. Van Kasteren T., Noulas A., Englebienne G., Kröse B., Accurate Activity Recognition in a Home Setting, [In:] Proceedings of the 10th International Conference on Ubiquitous Computing, 2008, DOI: 10.1145/1409635.1409637.
64. Zheng X., Wang M., Ordieres-Meré J., Comparison of Data Preprocessing Approaches for Applying Deep Learning to Human Activity Recognition in the Context of Industry 4.0, „Sensors”, Vol. 18, No. 7, 2018, DOI: 10.3390/s18072146.
65. Kotsiantis S.B., Kanellopoulos D., Pintelas P.E., Data Preprocessing for Supervised Learning, „International Journal of Computer Science”, Vol. 1, No. 2, 2006, 111–117.
66. Preece S.J., Goulermas J.Y., Kenney L.P., Howard D., A Comparison of Feature Extraction Methods for the Classification of Dynamic Activities from Accelerometer Data, „IEEE Transactions on Biomedical Engineering”, Vol. 56, No. 3, 2008, 871–879, DOI: 10.1109/TBME.2008.2006190.
67. Ravi D., Wong C., Lo B., Yang G.-Z., A DeepLlearning Approach to On-Node Sensor Data Analytics for Mobile or Wearable Devices, „IEEE Journal of Biomedical and Health Informatics”, Vol. 21, No. 1, 2016, 56–64, DOI: 10.1109/JBHI.2016.2633287.
68. Liu Z., Xu L., Jia Y., Guo S., Human Activity Recognition Based on Deep Learning with Multi-Spectrogram, [In:] 2020 IEEE 5th International Conference on Signal and Image Processing (ICSIP), IEEE, 2020, 11–15, DOI: 10.1109/ICSIP49896.2020.9339335.
69. Hur T., Bang J., Huynh-The T., Lee J., Kim J.-I., Lee S., Iss2Image: A Novel Signal-Encoding Technique for CNN-Based Human Activity Recognition, „Sensors”, Vol. 18, No. 11, 2018, DOI: 10.3390/s18113910.
70. Bloom V., Argyriou V., Makris D., Linear Latent Low Dimensional Space for Online Early Action Recognition and Prediction, „Pattern Recognition”, Vol. 72, 2017, 532–547, DOI: 10.1016/j.patcog.2017.07.003.
71. Khaire U.M., Dhanalakshmi R., Stability of feature selection algorithm: A review, „Journal of King Saud University – Computer and Information Sciences”, Vol. 34, No. 4, 2022, 1060–1073, DOI: 10.1016/j.jksuci.2019.06.012.
72. Li K., Fu Y., ARMA-HMM: A New Approach for Early Recognition of Human Activity, [In:] Proceedings of the
21st International Conference on Pattern Recognition (ICPR2012), IEEE, 2012, 1779–1782.
73. Lee D.-G., Lee S.-W., Human Interaction Recognition Framework Based on Interacting Body Part Attention, „Pattern Recognition”, Vol. 128, 2022, DOI: 10.1016/j.patcog.2022.108645.
74. Kambara M., Sugiura K., Relational Future Captioning Model for Explaining Likely Collisions in Daily Tasks, arXiv preprint arXiv:2207.09083, 2022.
75. Xu Z., Qing L., Miao J., Activity Auto-Completion: Predicting Human Activities from Partial Videos, [In:] Proceedings of the IEEE International Conference on Computer Vision, 3191–3199, DOI: 10.1109/ICCV.2015.365.
76. Meng M., Drira H., Daoudi M., Boonaert J., Human-Object Interaction Recognition by Learning the Distances Between the Object and the Skeleton Joints, [In:] 2015 11th IEEE International Conference and Workshops on Automatic Face and Gesture Recognition (FG), Vol. 7, IEEE, 2015, DOI: 10.1109/FG.2015.7284883.
77. Dutta V., Zielińska T., Predicting Human Actions Taking Into Account Object Affordances, „Journal of Intelligent & Robotic Systems”, Vol. 93, No. 3, 2019, 745–761, DOI: 10.1007/s10846-018-0815-7.
78. Uzunovic T., Golubovic E., Tucakovic Z., Acikmese Y., Sabanovic A., Task-Based Control and Human Activity Recognition for Human-Robot Collaboration, [In:] IECON 2018 – 44th Annual Conference of the IEEE Industrial Electronics Society, 2018, 5110–5115, DOI: 10.1109/IECON.2018.8591206.
79. Uzunovic T., Golubovic E., Tucakovic Z., Acikmese Y., Sabanovic A., Task-Based Control and Human Activity Recognition for Human-Robot Collaboration, [In:] IECON 2018-44th Annual Conference of the IEEE Industrial Electronics Society, IEEE, 2018, 5110–5115, DOI: 10.1109/IECON.2018.8591206.
80. Zheng X., Chen X., Lu X., A Joint Relationship Aware Neural Network for Single-Image 3D Human Pose Estimation, „IEEE Transactions on Image Processing”, Vol. 29, 2020, 4747–4758, DOI: 10.1109/TIP.2020.2972104.
81. Pavllo D., Feichtenhofer C., Auli M., Grangier D., Modeling Human Motion with Quaternion-Based Neural Networks, „International Journal of Computer Vision”, Vol. 128, No. 4, 2020, 855–872, DOI: 10.1007/s11263-019-01245-6.
82. Kratzer P., Midlagajni N.B., Toussaint M., Mainprice J., Anticipating Human Intention for Full-Body Motion Prediction in Object Grasping and Placing Tasks, [In:] 2020 29th IEEE International Conference on Robot and Human Interactive Communication (ROMAN), IEEE, 2020, 1157–1163.
83. Singh A., Patil D., Omkar S., Eye in the Sky: Real-Time Drone Surveillance System (DSS) for Violent Individuals Identification using Scatternet Hybrid Deep Learning Network, [In:] Proceedings of the IEEE Conference on Computer Vision and Pattern Recognition Workshops, 2018, 1629–1637, DOI: 10.1109/CVPRW.2018.00214.
84. Zhao X., Chen Y., Guo J., Zhao D., A spatial-Temporal Attention Model for Human Trajectory Prediction, „IEEE CAA Journal of Automatica Sinica”, Vol. 7, No. 4, 2020, 965–974, DOI: 10.1109/JAS.2020.1003228.
85. Putra P.U., Shima K., Shimatani K., A Deep Neural Network Model for Multi-View Human Activity Recognition, „PloS One”, Vol. 17, No. 1, 2022, DOI: 10.1371/journal.pone.0262181.
86. Du Y., Lim Y., Tan Y., Activity Prediction using LSTM in Smart Home, [In:] 2019 IEEE 8th Global Conference
on Consumer Electronics (GCCE), IEEE, 2019, 918–919, DOI: 10.1109/GCCE46687.2019.9015492.
87. Zhang Q., Wang T., Wu H.-N., Li M., Zhu J., Snoussi H., Human Action Prediction Based on Skeleton Data, [In:] 2020 39th Chinese Control Conference (CCC), IEEE, 2020, 6608–6612, DOI: 10.23919/CCC50068.2020.9189122.
88. Dong M., Xu C., Skeleton-Based Human Motion Prediction With Privileged Supervision, „IEEE Transactions on Neural Networks and Learning Systems”, 2022, DOI: 10.1109/TNNLS.2022.3166861.
89. Fragkiadaki K., Levine S., Felsen P., Malik J., Recurrent Network Models for Human Dynamics, [In:] Proceedings of the IEEE International Conference on Computer Vision, 2015, 4346–4354.
90. Feichtenhofer C., Fan H., Malik J., He K., SlowFast Networks for Video Recognition, [In:] Proceedings of the IEEE/CVF International Conference on Computer Vision (ICCV), 2019.
91. Ji S., Xu W., Yang M., Yu K., 3D Convolutional Neural Networks for Human Action Recognition, „IEEE Transactions on Pattern Analysis and Machine Intelligence”, Vol. 35, No. 1, 2013, 221–231, DOI: 10.1109/TPAMI.2012.59.
92. Alfaifi R., Artoli A.M., Human Action Prediction with 3D-CNN, „SN Computer Science”, Vol. 1, 2020, DOI: 10.1007/s42979-020-00293-x.
93. Zhou Y., Sun X., Zha Z.-J., Zeng W., MiCT: Mixed 3D/2D Convolutional Tube for Human Action Recognition, [In:] Proceedings of the IEEE Conference on Computer Vision and Pattern Recognition, 2018, 449–458, DOI: 10.1109/CVPR.2018.00054.
94. Gao C., Wang T., Zhang M., Zhu A., Shi P., Snoussi H., 3D Human Motion Prediction Based on Graph Convolution Network and Transformer, [In:] 2021 China Automation Congress (CAC), IEEE, 2021, 2957–2962, DOI: 10.1109/CAC53003.2021.9728062.
95. Schaefer S., Leung K., Ivanovic B., Pavone M., Leveraging Neural Network Gradients within Trajectory Optimization for Proactive Human-Robot Interactions, [In:] 2021 IEEE International Conference on Robotics and Automation (ICRA), IEEE, 2021, 9673–9679, DOI: 10.1109/ICRA48506.2021.9561443.
96. Cho H., Yoon S.M., Divide and Conquer-Based 1D CNN Human Activity Recognition using Test Data Sharpening, „Sensors”, Vol. 18, No. 4, 2018, DOI: 10.3390/s18041055.
97. Bhattacharyya A., Fritz M., Schiele B., Long-Term on-Board Prediction of People in Traffic Scenes Under Uncertainty, [In:] Proceedings of the IEEE Conference on Computer Vision and Pattern Recognition, 2018, 4194–4202, DOI: 10.1109/CVPR.2018.00441.
98. Hoai M., De la Torre F., Max-margin early event detectors, „International Journal of Computer Vision”, Vol. 107, No. 2, 2014, 191–202, DOI: 10.1007/s11263-013-0683-3.
99. Lan T., Chen T.-C., Savarese S., A Hierarchical Representation for Future Action Prediction, [In:] European Conference on Computer Vision, Springer, 2014, 689–704, DOI: 10.1007/978-3-319-10578-9_45.
100. Wang H., Yuan C., Shen J., Yang W., Ling H., Action Unit Detection and Key Frame Selection for Human Activity Prediction, „Neurocomputing”, Vol. 318, 2018, 109–119, DOI: 10.1016/j.neucom.2018.08.037.
101. Kong Y., Kit D., Fu Y., A Discriminative Model with Multiple Temporal Scales for Action Prediction, [In:] European Conference on Computer Vision, Springer, 2014, 596–611, DOI: 10.1007/978-3-319-10602-1_39.
102. Ryoo M.S., Human Activity Prediction: Early Recognition of Ongoing Activities from Streaming Videos, [In:]
2011 International Conference on Computer Vision, IEEE, 2011, 1036–1043, DOI: 10.1109/ICCV.2011.6126349.
103. Alvee B.I., Tisha S.N., Chakrabarty A., Application of Machine Learning Classifiers for Predicting Human Activity, [In:] 2021 IEEE International Conference on Industry 4.0, Artificial Intelligence, and Communications Technology (IAICT), 2021, 39–44, DOI: 10.1109/IAICT52856.2021.9532572.
104. Li K., Fu Y., Prediction of Human Activity by Discovering Temporal Sequence Patterns, „IEEE Transactions on Pattern Analysis and Machine Intelligence”, Vol. 36, No. 8, 2014, 1644–1657, DOI: 10.1109/TPAMI.2013.2297321.
105. Galata A., Johnson N., Hogg D., Learning Variable-Length Markov Models of Behavior, „Computer Vision and Image Understanding”, Vol. 81, No. 3, 2001, 398–413, DOI: 10.1006/cviu.2000.0894.
106. Cheng Y., Tomizuka M., Long-Term Trajectory Prediction of the Human Hand and Duration Estimation of the Human Action, „IEEE Robotics and Automation Letters”, Vol. 7, No. 1, 2021, 247–254, DOI: 10.1109/LRA.2021.3124524.
107. Manju D, Radha V., An Enhanced Human Activity Prediction at Rainy and Night Times, „International Journal of Recent Technology and Engineering, Vol. 8, 2019, 965–970, DOI: 10.35940/ijrte.C4113.098319.
108. Li C., Wearable Computing: Accelerometer-Based Human Activity Classification using Decision Tree, Utah State University, 2017.
109. Wang A., Chen H., Zheng C., Zhao L., Liu J., Wang L., Evaluation of Random Forest for Complex Human Activity Recognition using Wearable Sensors, [In:] 2020 International Conference on Networking and Network Applications (NaNA), IEEE, 2020, 310–315, DOI: 10.1109/NaNA51271.2020.00060.
110. Minor B.D., Doppa J.R., Cook D.J., Learning Activity Predictors from Sensor Data: Algorithms, Evaluation, and Applications, „IEEE Transactions on Knowledge and Data Engineering”, Vol. 29, No. 12, 2017, 2744–2757, DOI: 10.1109/TKDE.2017.2750669.
111. Yu G., Yuan J., Liu Z., Predicting Human Activities using Spatio-Temporal Structure of Interest Points, [In:] Proceedings of the 20th ACM International Conference on Multimedia, 2012, 1049–1052, DOI: 10.1145/2393347.2396380.
112. Yu G., Goussies N.A., Yuan J., Liu Z., Fast Action Detection via Discriminative Random Forest Voting and Top-K Subvolume Search, „IEEE Transactions on Multimedia”, Vol. 13, No. 3, 2011, 507–517, DOI: 10.1109/TMM.2011.2128301.
113. S. B. ud din Tahir, Jalal A., Batool M., Wearable Sensors for Activity Analysis using SMO-based Random Forest Over Smart Home and Sports Datasets, [In:] 2020 3rd International Conference on Advancements in Computational Sciences (ICACS), IEEE, 2020, DOI: 10.1109/ICACS47775.2020.9055944.
114. Halevy A., Norvig P., Pereira F., The Unreasonable Effectiveness of Data, „IEEE Intelligent Systems”, Vol. 24, No. 2, 2009, 8–12, DOI: 10.1109/MIS.2009.36.
115. Sessions V., Valtorta M., The Effects of Data Quality on Machine Learning Algorithms, Proceedings of the 11th International Conference on Information Quality (ICIQ), Vol. 6, 2006, 485–498.
116. Chen Y.-L., Wu X., Li T., Cheng J., Ou Y., Xu M., Dimensionality Reduction of Data Sequences for Human Activity Recognition, „Neurocomputing”, Vol. 210, 2016, 294–302, DOI: 10.1016/j.neucom.2015.11.126.
117. Ray S., Alshouiliy K., Agrawal D.P., Dimensionality Reduction for Human Activity Recognition Using Google Colab, „Information”, Vol. 12, No. 1, 2020, DOI: 10.3390/info12010006.
118. Darji N.R., Ajila S.A., Increasing Prediction Accuracy for Human Activity Recognition using Optimized Hyperparameters, [In:] 2020 IEEE International Conference on Big Data (Big Data), IEEE, 2020, 2472–2481, DOI: 10.1109/BigData50022.2020.9378000.
119. Ziaeefard M., Bergevin R., Semantic Human Activity Recognition: A Literature Review, „Pattern Recognition”, Vol. 48, No. 8, 2015, 2329–2345, DOI: 10.1016/j.patcog.2015.03.006.
120. Cheng H.-T., Sun F.-T., Griss M., Davis P., Li J., You D., NuActiv: Recognizing Unseen New Activities using Semantic Attribute-Based Learning, [In:] Proceeding of the 11th Annual International Conference on Mobile Systems, Applications, and Services, 2013, 361–374, DOI: 10.1145/2462456.2464438.
121. Venkatachalam S., Nair H., Zeng M., Tan C.S., Mengshoel O.J., Shen J.P., Sem-Net: Learning Semantic Attributes for Human Activity Recognition with Deep Belief Networks, „Frontiers in Big Data”, 2022, DOI: 10.3389/fdata.2022.879389.
122. Mittelman R., Lee H., Kuipers B., Savarese S., Weakly Supervised Learning of Mid-Level Features with Beta-Bernoulli Process Restricted Boltzmann Machines, [In:] 2013 IEEE Conference on Computer Vision and Pattern Recognition, 2013, 476–483, DOI: 10.1109/CVPR.2013.68.
123. Liu J., Yang Y., Saleemi I., Shah M., Learning Semantic Features for Action Recognition via Diffusion Maps, „Computer Vision and Image Understanding”, Vol. 116, No. 3, 2012, 361–377, DOI: 10.1016/j.cviu.2011.08.010.
124. Liu J., Wang X., Li T., Yang J., Spatio-Temporal Semantic Features for Human Action Recognition, „KSII Transactions on Internet and Information Systems”, Vol. 6, No. 10, 2012, 2632–2649, DOI: 10.3837/tiis.2012.10.011.
125. Sunkesula S.P.R., Dabral R., Ramakrishnan G., Lighten: Learning Interactions with Graph and Hierarchical Temporal Networks for HOI in Videos, [In:] Proceedings of the 28th ACM International Conference on Multimedia, 2020, 691–699, DOI: 10.1145/3394171.3413778.
126. M. Sadegh Aliakbarian, F. Sadat Saleh, M. Salzmann, B. Fernando, L. Petersson, L. Andersson, Encouraging LSTMs to Anticipate Actions Very Early, [In:] Proceedings of the IEEE International Conference on Computer Vision, 2017, 280–289.
127. Pirri F., Mauro L., Alati E., Ntouskos V., Izadpanahkakhk M., Omrani E., Anticipation and Next Action Forecasting in Video: an End-to-End Model with Memory, arXiv preprint arXiv:1901.03728, 2019.
128. Uddin M.Z., A Wearable Sensor-Based Activity Prediction System to Facilitate Edge Computing in Smart Healthcare System, „Journal of Parallel and Distributed Computing”, Vol. 123, 2019, 46–53, DOI: 10.1016/j.jpdc.2018.08.010.
129. Shi Y., Fernando B., Hartley R., Action Anticipation with RBF kernelized Feature Mapping RNN, [In:] Proceedings of the European Conference on Computer Vision (ECCV), 2018, 301–317.
130. Jaouedi N., Perales F.J., Buades J.M., Boujnah N., Bouhlel M.S., Prediction of Human Activities Based on a New Structure of Skeleton Features and Deep Learning Model, „Sensors”, Vol. 20, No. 17, 2020, DOI: 10.3390/s20174944.
131. Fan Y., Wen G., Li D., Qiu S., Levine M.D., Early Event Detection Based on Dynamic Images of Surveillance
Videos, „Journal of Visual Communication and Image Representation”, Vol. 51, 2018, 70–75, DOI: 10.1016/j.jvcir.2018.01.002.
132. Kong Y., Fu Y., Max-Margin Action Prediction Machine, „IEEE Transactions on Pattern Analysis and Machine Intelligence”, Vol. 38, No. 9, 2016, 1844–1858, DOI: 10.1109/TPAMI.2015.2491928.
133. Jalal A., Quaid M.A.K., Hasan A.S., Wearable Sensor-Based Human Behavior Understanding and Recognition in Daily Life for Smart Environments, [In:] 2018 International Conference on Frontiers of Information Technology (FIT), 2018, 105–110, DOI: 10.1109/FIT.2018.00026.
134. Bütepage J., Kjellström H., Kragic D., A Probabilistic Semi-Supervised Approach to Multi-Task Human Activity Modeling, arXiv preprint arXiv:1809.08875, 2018.
135. Qi S., Huang S., Wei P., Zhu S.-C., Predicting Human Activities using Stochastic Grammar, [In:] Proceedings of the IEEE International Conference on Computer Vision, 2017, 1164–1172.
136. Jin Y., Zhu L., Mu Y., Complex Video Action Reasoning via Learnable Markov Logic Network, [In:] Proceedings of the IEEE/CVF Conference on Computer Vision and Pattern Recognition, 2022, 3242–3251.
137. Manousaki V., Papoutsakis K., Argyros A., Graphing the Future: Activity and Next Active Object Prediction using Graph-based Activity Representations, arXiv preprint arXiv:2209.05194, 2022.
138. Li S., Li K., Fu Y., Early Recognition of 3D Human Actions, „ACM Transactions on Multimedia Computing, Communications, and Applications”, Vol. 14, No. 1s, 2018, DOI: 10.1145/3131344.
139. Ellis K., Kerr J., Godbole S., Lanckriet G., Wing D., Marshall S., A Random Forest Classifier for the Prediction of Energy Expenditure and Type of Physical Activity from Wrist and Hip Accelerometers, „Physiological Measurement”, Vol. 35, No. 11, 2014, DOI: 10.1088/0967-3334/35/11/2191
140. Sánchez V.G., Skeie N.-O., Decision trees for human activity recognition modelling in smart house environments, „Simulation Notes Europe”, Vol. 28, 2018, 177–184, DOI: 10.11128/sne.28.tn.10447.
Streszczenie: Przewidywanie działań człowieka to bardzo aktualny kierunek badań. Wykorzystywane są tu powszechnie metody sztucznej inteligencji. Umożliwiają one wczesne rozpoznawanie i klasyfikowanie działań człowieka. Taka wiedza jest niezwykle potrzebna w pracach nad robotami i innymi interaktywnymi systemami komunikującymi się i współpracującymi z ludźmi. Zapewnia to wczesne reakcje takich urządzeń i odpowiednie planowanie ich przyszłych działań. Jednak ze względu na złożoność działań człowieka ich przewidywanie jest trudnym zadaniem. W tym artykule dokonujemy przeglądu najnowocześniejszych metod i podsumowujemy ostatnie postępy w zakresie przewidywania aktywności człowieka. Skupiamy się szczególnie na czterech podejściach wykorzystujących metody uczenia maszynowego, a mianowicie na metodach wykorzystujących: sztuczne sieci neuronowe, metody wektorów nośnych, modele probabilistyczne oraz drzewa decyzyjne. Omawiamy zalety i wady tych podejść, a także aktualne wyzwania związane z zagadnieniami przewidywania aktywności człowieka. Ponadto opisujemy rodzaje czujników i zbiory danych powszechnie stosowane w badaniach dotyczących przewidywania i rozpoznawania działań człowieka. Analizujemy jakość stosowanych metod w oparciu o dokładność przewidywania raportowaną w artykułach naukowych. Opisujemy znaczenie rodzaju danych oraz parametrów modeli uczenia maszynowego. Na koniec podsumowujemy najnowsze trendy badawcze. Artykuł ma za zadanie pomóc przy wyborze właściwej metody przewidywania aktywności człowieka, wraz ze wskazaniem narzędzi i zasobów niezbędnych do efektywnego osiągnięcia tego celu.
ORCID: 0000-0002-8299-0727
Ekemeyong Awong Lisiane Esther, MSc Eng.
ORCID: 0000-0002-2047-0152
Streszczenie: Przedmiotem artykułu są algorytmy sterowania predykcyjnego (typu MPC –Model Predictive Control) ramion manipulatorów sztywnych. Zastosowano MPC z modelem w przestrzeni stanów i wykorzystano najnowszą technikę tłumienia zakłóceń i błędów modelowania, pozwalającą uniknąć dynamicznego modelowania zakłóceń lub uciekania się do dodatkowych technik ich eliminowania, takich jak SMC. Rozważane są przede wszystkim najbardziej efektywne obliczeniowo algorytmy MPC-NPL (NPL – Nonlinear Prediction and Linearization), w dwóch wersjach: z optymalizacją QP (Quadratic Programming) z ograniczeniami i z jawną optymalizacją bez ograniczeń i spełnieniem ograniczeń nierównościowych a posteriori. Dla wszystkich rozważanych algorytmów przeprowadzono kompleksową analizę symulacyjną sterowania manipulatorem z napędem bezpośrednim, przy dwóch rodzajach zakłócenia: zewnętrznym i parametrycznym. Wyniki porównano z uzyskanymi dla znanego algorytmu CTC-PID (CTC – Computer Torque Control), uzyskując lepszą jakość regulacji algorytmami MPC. Zbadano wpływ długości okresu próbkowania i obliczeniowego opóźnienia sterowania na jakość regulacji, co jest istotne dla algorytmów z szybkim próbkowaniem opartych na modelach.
Manipulatory i roboty są szeroko stosowane w coraz bardziej zautomatyzowanych systemach produkcji i montażu. Aby sprostać postawionym zadaniom, sterowniki ramion manipulatorów powinny zapewniać odpowiednią jakość śledzenia zadanych trajektorii przy jednoczesnym generowaniu szybkich, płynnych i bezpiecznych ruchów. Manipulatory to złożone, nieliniowe systemy mechatroniczne, w szczególności manipulatory z napędem bezpośrednim, z silnymi współzależnościami między ramionami. Stąd algorytmy sterowania muszą być projektowane dla obiektów nieliniowych i wielowymiarowych. W literaturze przedmiotu znaleźć można wiele rozwiązań tego problemu, poczynając od prostych klasycznych regulatorów PID, popularnych w praktyce, zwłaszcza dla sterowania silnikami z przekładniami, które skutecznie tłumią efekty sprzężeń dynamicznych między ramionami. Celem niniejszego artykułu nie jest przegląd struktur i algorytmów sterowania ramionami
manipulatorów. Skorzystamy z faktu, że ukazała się ostatnio praca przeglądowa na ten temat [23], z wyczerpującą listą piśmiennictwa, do której odsyłamy zainteresowanego czytelnika. Polecamy również znacznie wcześniejszą, obszerną pracę przeglądową [13]. Warto również wspomnieć o podręcznikach dotyczących sterowania ramionami manipulatorów, w tym standardowego sterowania PD/PID i bardziej rozbudowanych struktur: PD/PID z kompensacją momentów grawitacyjnych oraz PD/PID z wykorzystaniem linearyzacji modelu manipulatora przez nieliniowe sprzężenie zwrotne, zwanego sterowaniem CTC (ang. Computed Torque Control) lub sterowaniem z odwrotnością dynamiki (ang. inverse dynamics control) [10, 12].
Sterowanie predykcyjne MPC (ang. Model Predictive Control) jest obecnie uznaną zaawansowaną techniką sterowania, reprezentowaną przez liczne algorytmy i pakiety oprogramowania stosowane z powodzeniem w praktyce przemysłowej, zwłaszcza w sterowaniu procesami technologicznymi [7, 17, 2, 19, 24, 3, 27]. Algorytmy MPC znalazły zastosowanie w przemysłowych układach sterowania przede wszystkim dla obiektów wielowymiarowych, z silniejszymi oddziaływaniami skrośnymi, z aktywnymi ograniczeniami, o trudnej dynamice, gdzie klasyczne układy regulacji PID nie zapewniały zadowalającej jakości regulacji. MPC jest techniką opartą na modelach, wykorzystującą optymalizację on-line jako podstawowy mechanizm obliczania wartości sygnałów sterujących, stąd wymagającą większej mocy obliczeniowej. Dlatego początkowo stosowano ją do procesów o wolniejszej dynamice i z wykorzystaniem modeli liniowych. Aby zastosować algorytmy MPC do
Z danych fizycznych [20] wynikają następujące wartości parametrów:
p1 = 2,352 Nms2, p2 = 0,102 Nms2, p3 = 0,084 Nms2, p4 = 38,466 Nm, p5 = 1,825 Nm
fv1 = 2,288 Nms, fv2 = 0,175 Nms,
fC1 = 7,17 dla 1 0Nm, q 8,05 dla 1 0Nm, q fC2 = 1,734 Nm, wraz z ograniczeniami momentów sterujących: u1max = 200 Nm, u2max = 15 Nm.
Do symulacji dynamiki manipulatora w pętli sterowania ze sprzężeniem zwrotnym użyto modelu dyskretnego (3)–(4) z bardzo krótkim krokiem całkowania T c = T p = 0,0001 s. Wówczas model ten z bardzo dużą dokładnością odzwierciedla dynamikę czasu ciągłego. Aby uzyskać do projektowania regulatorów MPC model ciągły i różniczkowalny, wykorzystano model (3)–(4) z pominięciem tarcia suchego, tj. z . v FqFq Stąd tarcie suche (Coulomba) traktowane będzie w układzie regulacji z MPC jako dynamika niemodelowana. Jako podstawowy okres próbkowania regulatorów przyjęto T c = 0,005 s, zbadano również algorytmy z innymi wartościami T c
Zastosowano następujący scenariusz symulacji:
− Długość horyzontu symulacji 2,5 s, trajektorie zadane z dwoma zmianami końcowych położeń kątowych ramion manipulatora (w radianach), realizowane jako trajektorie sześcienne trwające 0,5 s każda, pierwsza rozpoczynająca się w chwili 0,15 s, druga w chwili 1,5 s.
−Zakłócenie:
– zewnętrzne (Zu): moment obrotowy 4 Nm (przy maksymalnym momencie obrotowym drugiego siłownika 15 Nm) dodany do wejścia sterującego drugiego ramienia od chwili 1,25 s (połowa horyzontu symulacji); zakłócenie typowe dla testowania jakości regulacji, w tym manipulatorów [10]; lub
– parametryczne (P1): zmiana dynamiki przez dodanie na końcu drugiego ramienia obciążenia 1 kg, co skutkuje zmianami parametrów obiektu do wartości:
p1 = 2,596 Nms2, p2 = 0,144 Nms2, p3 = 0,154 Nms2, p4 = 42,88 Nm, p5 = 3,351 Nm.
Parametry te są jedynie w modelu manipulatora jako obiektu w pętli regulacji, regulatory projektowane są dla modelu nominalnego (bez zakłóceń).
Zauważmy, że zewnętrzne zakłócenia wymagają tłumienia, ale nie zmieniają dynamiki pętli sprzężenia zwrotnego. Zmiany parametrów obiektu zmieniają tę dynamikę.
Jakość sterowania zdefiniowana przez kryterium ISE (ang. Integrated Squared Error):
gdzie N sym to liczba okresów próbkowania T c określająca horyzont symulacji.
Przyjęto następujące wartości parametrów w funkcji celu zadań optymalizacji MPC: [ 1 2] = [10 10], [ 1 2] = [0,0001 0,001].
Przyjęto okres próbkowania T c = 0,005 s, ale zbadano również sterowanie z innymi wartościami. Przetestowano różne horyzonty predykcji, dla T c = 0,005 s wybrano N = 18. Przyjęte wartości zapewniają, dla krótkich horyzontów sterowania N u, kompromis między jakością regulacji, nakładem obliczeniowym i odpornością. Różnice w wartościach wag wynikają, w znacznym stopniu, z różnic w zakresach wartości zmiennych, co odpowiada skalowaniu zadania optymalizacji.
Porównanie rezultatów sterowania przedstawionymi w pracy algorytmami MPC przedstawiono zbiorczo w Tabeli 1, w sposób zagregowany przez podanie wartości indeksu ISE (31), dla różnych horyzontów sterowania N u oraz różnych zakłóceń. Symulacje przeprowadzono w środowisku MATLAB, wykorzystując do optymalizacji nieliniowej procedurę „fmincon”, a do QP procedurę „quadprog”. Do linearyzacji modelu zastosowano przybliżenie numeryczne pochodnych cząstkowych, ze względu na bardzo złożone wzory analityczne (toolbox „Symbolic Math”) – uzyskując wyniki praktycznie równoważne, niemające wpływu na symulację układu sterowania. Rozważono trzy przypadki: tylko z dynamiką niemodelowaną (tarcie suche, Coulomba), z dynamiką niemodelowaną i dodanym zakłóceniem zewnętrznym (Zu) lub parametrycznym (P1).
Horyzont sterowania w największym stopniu wpływa na nakład obliczeń, bo definiuje wymiar N u × n u zadania optymalizacji, dla N u = 1 i N u = 2 odpowiednio 2 i 4. Wybór N u = 2 skutkuje wyraźnie lepszymi wartościami ISE, dla N u = 3 i N u = 4 poprawa jest już niewiele większa, przy zwiększonym obciążeniu obliczeniowym. Dla każdego z przypadków zakłócenia, wyniki dla wszystkich trzech algorytmów, NO, NPLn i NPLa, są praktycznie identyczne. Porównując MPC-NO i MPC-NPL, różnice występują na dalszych miejscach po przecinku, z reguły niewidocznych w Tabeli 1, trajektorie są wizualnie nie do odróżnienia. Wyniki dla NPLn i MPLa są dokładnie identyczne, ze względu na nieaktywne ograniczenia sterowania. Przykładowe trajektorie przedstawiono dla algorytmu MPC-NPLn z N u = 2, na Rys. 3 i Rys. 4, dla zakłó-
dzi do szybkiej optymalizacji liniowo-kwadratowej. Linearyzację można spotkać w poprzednich próbach stosowania algorytmów MPC, co zostało pokrótce omówione we wstępie. Model liniowy był wówczas używany zarówno do wszystkich predykcji, jak i do optymalizacji. Jest to różnica istotna, albowiem z doświadczenia wynika, ze predykcja nieliniowa odgrywa kluczową rolę. Przy tym nie zwiększa znacząco obciążenia obliczeniowego, ponieważ jest wykonywana tylko raz w okresie próbkowania. Algorytmy MPC-NPL zostały przedstawione w artykule w dwóch wersjach: pierwsza (zwana numeryczną) jest z optymalizacją kwadratową (QP) z ograniczeniami, druga (zwana analityczną), z najmniejszym nakładem obliczeń, jest z jawną (analityczną) optymalizacją bez ograniczeń i następnie z dopasowaniem (przycięciem) sterowań do ograniczeń nierównościowych. Zarówno dla algorytmu MPC-NPL, jak i algorytmu MPC-NO przedstawiono wyniki obszernych badań symulacyjnych z eksperymentalnym manipulatorem z napędami bezpośrednimi. Przedstawione wyniki pokazały, że prostsze obliczeniowo algorytmy NPC-NPL realizują nadążanie za gładkimi trajektoriami zadanymi z praktycznie taką samą dokładnością jak algorytm MPC-NO. Wyniki porównano z uzyskanymi dla znanego algorytmu CTC-PID, będącego również „model-based” z wykorzystaniem nieliniowego modelu manipulatora. Z porównań wynika, że przedstawiony w pracy algorytm MPC jest konkurencyjną alternatywą dla CTC-PID, wymagającą nieco więcej obliczeń, ale zapewniającą lepszą jakość sterowania i większą odporność, dla obu rodzajów rozpatrywanych zakłóceń, zewnętrznego i parametrycznego. Zasygnalizowano też potencjalne możliwości skutecznego zastosowania algorytmów MPC do sterowania manipulatorów przy odcinkami stałych, skokowo zmiennych trajektoriach wartości zadanych.
Zbadano również wpływ długości okresu próbkowania i opóźnienia obliczeniowego sterowania na działanie algorytmów MPC i CTC-PID. Jest to ważne przy projektowaniu algorytmów sterowania opartych na modelach i przy szybkim próbkowaniu, ponieważ większy nakład obliczeń może skutkować opóźnieniem implementacji sterowania.
Wyniki symulacji przedstawione w artykule są fragmentem badań autora prowadzonych nad sterowaniem manipulatorów algorytmami MPC. Ograniczona objętość pracy nie pozwoliła na prezentację szerszych wyników badań, ale konkluzje dla innych manipulatorów były analogiczne, uwiarygodniając wnioski przedstawione w pracy. Autor ma nadzieję, że przedstawione rezultaty będą interesujące zarówno dla badań stosowanych, jak i praktyki przemysłowej.
6. Bumroongsri P., Kheawhom S., Interpolation-based off-line MPC for LPV systems. [In:] Proceedings of the 10th IFAC International Symposium on Dynamics and Control of Process Systems, Mumbai, India, 2013.
7. Camacho E.F., Bordons C., Model Predictive Control Springer Verlag, London, 1999.
8. Cisneros P.S.G., Sridharan A., Werner H., Constrained predictive control of a robotic manipulator using quasi-LPV representations. „IFAC-PapersOnLine”, Vol. 51, No. 26, 2018, 118–123, DOI: 10.1016/j.ifacol.2018.11.158.
9. Ferrara A., Incremona G.P., Magni L., A robust MPC/ISM hierarchical multiloop control scheme for robot manipulators. [In:] Proceedings of the 52nd Conference on Decision and Control, Florence, Italy, 2013, DOI: 10.1109/CDC.2013.6760430.
10. Lewis F.L., Dawson D.M., Abdallah C.T., Robot Manipulator Control Theory and Practice. CRC Press, Boca Raton, 2003.
11. Incremona G.P., Ferrara A., Magni L., MPC for robot manipulators with integral sliding modes generation „IEEE/ASME Transactions on Mechatronics”, Vol. 22, No. 3, 2017, 1299–1307, DOI: 10.1109/TMECH.2017.2674701.
12. Kelly R., Santibáñez V., Loría A., Control of Robot Manipulators in Joint Space. Springer, London 2005, DOI: 10.1007/b135572.
13. Khan M.F., Islam R., Iqbal J., Control strategies for robotic manipulators. [In:] Proceedings of the 2012 International Conference of Robotics and Artificial Intelligence, Rawalpindi, Pakistan, DOI: 10.1109/ICRAI.2012.6413422.
14. Kleff S., Meduri A., Budhiraja R., Mansard N., Righetti L., High-frequency nonlinear model predictive control of a manipulator. [In:] Proceedings of the IEEE International Conference on Robotics and Automation, Xi’an, China, 2021, DOI: 10.1109/ICRA48506.2021.9560990.
15. Ławryńczuk M., Computationally Efficient Model Predictive Control Algorithms: A Neural Network Approach. „Studies in Systems, Decision and Control”, Vol. 3. Springer Verlag, Heidelberg 2014, DOI: 10.1007/978-3-319-04229-9.
16. Ławryńczuk M., Tatjewski P., Offset-free state-space nonlinear predictive control for Wiener systems. „Information Sciences”, Vol. 511, 2020, 127–151, DOI: 10.1016/j.ins.2019.09.042.
17. Maciejowski J.M., Predictive Control with Constraints Prentice Hall, Harlow, England, 2002.
18. Nicolis D., Allevi F., Rocco P., Operational space model predictive sliding mode control for redundant manipulators „IEEE Transactions on Robotics”, Vol. 36, No. 4, 2020, 1348–1355, DOI: 10.1109/TRO.2020.2974092.
1. Benniran A., Predictive optimizing reference governor for constrained 2 dof’s robot with abrupt set-point trajectories. „Journal of Applied Science”, Sabratha University, No. 1, 2018, 39–49, DOI: 10.47891/sabujas.v1i1.39-49.
2. Blevins T.L., McMillan G.K., Wojsznis W.K., Brown M.W., Advanced Control Unleashed. The ISA Society, Research Triangle Park, NC, 2003.
3. Blevins T.L., Wojsznis W.K., Nixon M., Advanced Control Foundation: Tools, Techniques, and Applications. The ISA Society, Research Triangle Park, NC, 2013.
4. Bouzoualegh S., Guechi E., Zennir Y., Model predictive control of a three degrees of freedom manipulator robot. [In:] Proceedings of the 3rd International Conference on Advanced Systems and Emergent Technologies, Hammamet, Tunisia, 2019, 84–89, DOI: 10.1109/ASET.2019.8870999.
5. Bożek A., Trybus L., Tuning PID and PI-PI servo controllers by multiple pole placement. „Bulletin of the Polish Academy of Sciences Technical Sciences”, Vol. 70, No. 1, 2022, 1–12, DOI: 10.24425/bpasts.2021.139957.
19. Qin S.J., Badgwell T.A., A survey of industrial model predictive control technology. „Control Engineering Practice”, Vol. 11, No. 7, 2003, 733–764, DOI: 10.1016/S0967-0661(02)00186-7.
20. Reyes F., Kelly R., Experimental evaluation of identification schemes on a direct drive robot. „Robotica”, Vol. 15, No. 5, 1997, 563–571, DOI: 10.1017/S0263574797000659.
21. Rybus T., Seweryn K., Sasiadek J.Z., Application of predictive control for manipulator mounted on a satellite. „Archives of Control Sciences”, Vol. 28, No. 1, 2018, 105–118, DOI: 10.24425/119079.
22. Spong M., Hutchinson S., Vidyasagar M., Robot Modeling and Control. J. Wiley and Sons, 2005.
23. Spong M.W., An historical perspective on the control of robotic manipulators. „Annual Reviews of Control, Robotics, and Autonomous Systems”, Vol. 5, 2022, 1–31, DOI: 10.1146/annurev-control-042920-094829.
24. Tatjewski P., Advanced Control of Industrial Processes. Structures and Algorithms. Springer Verlag, London 2007, DOI: 10.1007/978-1-84628-635-3.
25. Tatjewski P., Disturbance modeling and state estimation for offset-free predictive control with state-spaced process models. „International Journal of Applied Mathematics and Computer Science”, Vol. 24, No. 2, 2014, 313–323.
26. Tatjewski P., Offset-free nonlinear predictive control with measured state and unknown asymptotically constant disturbances. [In:] Malinowski K., Józefczyk J., Świątek J. (eds), „Aktualne problemy automatyki i robotyki”, 288–299, Academic Publisher EXIT, Warszawa 2014.
27. Tatjewski P., Sterowanie zaawansowane procesów przemysłowych Struktury i algorytmy. Wydanie drugie, zmienione (e-book). Akademicka Oficyna Wydawnicza EXIT, Warszawa 2016.
28. Tatjewski P., Offset-free nonlinear Model Predictive Control with state-space process models. „Archives of Control Sciences”, Vol. 27, No. 4, 2017, 595–615, DOI: 10.1515/acsc-2017-0035.
29. Tatjewski P., Ławryńczuk M., Algorithms with state estimation in linear and nonlinear model predictive control „Computers and Chemical Engineering”, Vol. 143, 2020, DOI: 10.1016/j.compchemeng.2020.107065.
30. Wilson J., Charest M., Dubay R., Non-linear model predictive control schemes with application on a 2 link vertical robot manipulator. „Robotics and Computer-Integrated Manufacturing”, Vol. 41, 2016, 23–30, DOI: 10.1016/j.rcim.2016.02.003.
Abstract: The subject of the article are predictive control algorithms (of MPC type – Model Predictive Control) for rigid manipulator arms. MPC with a state-space model and with the latest disturbance and modeling error suppression technique was applied, which avoids dynamic disturbance modeling or resorting to additional disturbance cancellation techniques, such as SMC.
First of all, the most computationally efficient MPC-NPL (Nonlinear Prediction and Linearization) algorithms are considered, in two versions: the first with constrained QP (Quadratic Programming) optimization and the second with explicit (analytical) optimization without constraints and satisfying a posteriori inequality constraints. For all considered algorithms, a comprehensive simulation analysis was carried out for a direct drive manipulator, with two kinds of disturbances: external and parametric. The obtained results were compared with those for the well-known CTC-PID algorithm (CTC – Computer Torque Control), showing better control quality with MPC algorithms. In addition, the influence of the length of the sampling period and of the computational delay on control quality was investigated, which is important for model-based algorithms with fast sampling.
Keywords
ORCID: 0000-0002-2596-8937
Abstract: Arm-Z is a concept of a hyper-redundant manipulator based on linearly joined sequence of congruent modules by oblique swivel joint mechanism. Each module has one degree of freedom only, namely a twist relative to the previous module in the sequence. Although the concept of this type of manipulator is relatively old and simple, its control is very difficult an nonintuitive, which results in a limited use in industrial practice. This paper presents a simple simulation of Arm-Z in Mathematica programming environment which demonstrates a few simple but potentially useful movements.
Biological snakes are extremely well adapted for different environments. This is mostly the result of the high redundancy of the snake mechanisms. In many instances of irregular environments the bio-inspired robots outperform conventional wheeled, legged or tracked robots. The snake-resembling robots are researched already for a few decades. This type of locomotion has been studied since the 1940s [1], and a half century later, its rigorous mathematical model has been developed. Shigeo Hirose at Tokyo Institute of Technology introduced so-called ”active chord mechanism” (ACM), and the first successful snake locomotor ACM III was built in 1972–75. In the late 90’s, a trunk-like locomotors and manipulators have been introduced in [2]. Kinematic modeling and control of hyper-redundant robots inspired by the octopus arm based on a discrete multi-segment model in which each segment is a 6-DoF Gough-Stewart parallel platform has been proposed in [3]. Various snake-like robots have been built [4]; most of the designs were intended for crawling on ground [5–9], some of them for swimming [10, 11], and even fewer for both swimming and crawling on the ground [12, 13]. Figure 1 shows an amphibious snake robot designed to perform underwater inspections and search-and-rescue missions in hazardous environments.
Alike biological snakes or bionic trunks, in various environments the characteristic type of motion gives this type of manipulators certain advantage over conventional rob otic
manipulators. They can operate in geometrically complicated environments which are not accessible by other approaches. Depending on the required task, various working heads can be installed on such manipulators, e.g. for: welding, cleaning, monitoring, etc.
In principle, six degrees of freedom (DoFs) are enough to complete any motion in three-dimensional space: displacement along three Cartesian axes X, Y, Z; and three rotations: yaw, pitch and roll. A conventional industrial manipulator has low number of DoFs – usually just six. On the other hand, human arm is the biological archetype of a kinematically redundant manipulator with 7-DoFs: 3 at the shoulder, 1 at the elbow and 3 at the wrist. Many robots use this kinematic arrangement. Such robots are called human-arm-like manipulators, e.g.: PA-10 robot by Mitsubishi, Lightweight Robot DLR (Deutsches Zentrum für Luft- und Raumfahrt), etc. DEXTER by Scienzia Machinale is an example of an 8-DoF manipulator. Systems with larger number of joints are called redundant robots, while term hyper-redundant refers to redundant manipulators with a very large, possibly infinite, number of DoFs [14]. They can be further classified in two groups: vertebrate-
Fig. 1. A photograph of the modular amphibious (capable both of swimming and crawling) snake locomotor ACM-R5H, 2010. [Tokyo Institute of Technology]-like rigid link manipulators, e.g. such as snakes, and invertebrate-like continuum manipulators, such as octopus arms or elephant trunks. The inverse kinematics problem for a serial-chain manipulator is to determine the positions of joints given the position and orientation of the end-effector. So-called closed-form solutions are practical because they readily identify all possible solutions faster than numerical methods [15]. The
inverse kinematic problem of a typical industrial manipulator can be solved easily [16].
As a result, its control is straightforward. However, HRMs are highly non-linear systems, therefore their control is by no means straightforward, and requires application of artificial intelligence methods [17–19]. In has been postulated in [20] that immersive realities and natural language should
play a very important role in the near future of hyper-redundant robots and their tele-operation. A method of solving the closed-form solution to the inverse kinematics of a planar redundant manipulator has been proposed in [21]. It was based on employing the Frechet differential of a certain criterion function introduced to resolve the redundancy. However, that model did not include any constraints on the range of motion of the joints – which in fact makes the formulation much more complicated.
Moreover, already in the forward kinematics, the analytical description ”explodes” with the number of links (DoFs), not to mention the inverse kinematics which by nature is more difficult. Therefore the proposed approach is not practical for hyper-redundant manipulators. The pioneering work of mathematical modelling of a discrete redundant planary manipulator in the Cartesian space has been presented in 1989 [22]. In Ref. [23] the same author presented a model of the kinematics of a rotary, redundant manipulator, in the form of a Finite State Machine. An improved inverse kinematic and velocity solution for spatial hyper-redundant robots based on backbone curve concepts and a modal approach for resolving the manipulator’s redundancy in [24]. A technique to solve the inverse kinematics of redundant manipulators, using a multi-objective genetic algorithm based on combination of the closed-loop pseudo-inverse method with a multi-objective genetic algorithm to control the joint positions has been proposed in [25]. An implementation of a heuristic graph searching algorithm for finding collision-free trajectory for a (5-link) planar redundant manipulator has been presented in [26]. A method for studying the trajectory control of planar manipulators using the Moore-Penrose pseudoinverse based on fractional calculus and fractional matrix powers has been proposed in [27]. An optimization algorithm for the motion planning of a hyper-redundant robot where the motion of one end follows arbitrary path and all links of the locomotor avoid all obstacles present in the environment has been presented in [28]. For obstacle avoidance problem of planar hyper-redundant manipulators, so called ”tunneling” approach has been presented in [14]. In the next paper [29] the same authors presented hyper-redundant robot mechanisms and their applications, including a 30-DoFs hyper-redundant robot. In Ref. [30] the dynamics of hyper-redundant manipulators has been formulated as a continuum mechanics problem. The advantage of the presented method was that it can be easily parallelized. Regarding building physical prototypes, a comprehensive study of a cable-driven hyper-redundant robot in terms of mechanical design, kinematics
analysis, and experimental verification has been documented in [31]. The property of shape memory polimer (SMP) has been explored for the design and fabrication of a 3D-printed modular omni-directional joint with variable stiffness in [32]. A lightweight hyper-redundant manipulator driven by embedded dielectric polymer actuators with binary actuation has been documented in [33]. For more information on this type of manipulators see [30].
This paper was inspired by oblique swivel joint mechanism robot made with LEGO system [34], as shown below.
Three-bearing swivel module based on the concept of oblique swivel joint mechanism has been used in the Rolls-Royce LiftSystem designed for use in the short take-off and vertical landing (STOVL) military aircraft, shown in Fig. 3. In 2001, the LiftSystem propulsion system was awarded the Collier Trophy, in recognition of ”the greatest achievement in aeronautics or astronautics in America”, specifically for ”improving the performance, efficiency and safety of air or space vehicles, the value of which has been thoroughly demonstrated by actual use during the preceding year. This means that properly designed, oblique swivel joint mechanism is extremely reliable and robust.
A conventional industrial manipulator has low number of degrees of freedom, whereas a trunk-like or snake-line manipulator has redundant (considerably large) number of degrees of freedom. In Arm-Z manipulator, each link between modules has exactly one degree of freedom (1-DoF). A design for a two degrees of freedom joint mechanism optimized for compactness, strength and range of motion for three-dimensional hyper redundant robots has been presented in [35]. A mechanical design for a compact three degrees-of-freedom joint mechanism for hyper-redundant or snake-like robots has been presented in [36]. Arm-Z manipulator has as many degrees of freedom as the number of units less of one. This redundancy allows the Arm-Z to perform complicated spatial movements, but also may improve the robustness and fault tolerance of the system. Therefore Arm-Z belongs to the family of so-called hyper-redundant-manipulators (HRM [37]).
The Arm-Z modules are geometrical objects which are analogous to sectors of circular tori. Each module is defined by the following parameters: size r, offset d, and , that is the angle between upper (T) and lower (B) faces of the module (see Fig. 4).
The overall shape of Arm-Z depends on: the number of modules, the geometric parameters of each module, and the relative twists between the modules.
The main limitation of the LEGO oblique swivel joint mechanism robot presented in [34] was the structural limit of the LEGO elements. As a result the physical limit of the system is only four modules (4-DoFs). Virtual model is obviously free from such constraints. A simple virtual model of Arm-Z has been made with the most intuitive programming environment – Mathematica. It has been adjusted to match the manipulator presented in Ref. [34], that is: there are four modules
(4-DoF); the base and top are made of half-modules. In this way the manipulator can form a perfect pipe with base and top parallel to the working table and horizon, which is advantageous form the practical point of view. Moreover, the problem of intersections has not been implemented. However, due to the friendliness of the user interface, it can be easily visually inspected. The movements presented in Ref. [34] have been replicated and expanded. Table 5 shows 11 time-steps of the first experiment.
In this case the base module turns by half-turn from – /2 to /2. Simultaneously the rest of the modules turn twice as fast (performing a full turn) in alternating directions. The motion is perfectly smooth and there is no wobbling sideways.
Arm-Z straightens from partial torus to straight pipe in 5 time-steps, and bends symmetrically on the other side
Rys. 5. Od góry po lewej do dołu po prawej: początkowo Arm-Z prostuje się z częściowego torusa do prostej rury w 5 krokach, następnie zgina się symetrycznie po drugiej stronie
Fig. 5. From top left to bottom right: at firstFig. 7. From slightly unwound spiral to straight, and back to slightly unwound spiral on the opposite side. As the narrowness of the bounding box indicates – the wobbling is unnoticeable
Rys. 7. Od lekko rozwiniętej spirali do prostej rury i z powrotem do lekko rozwiniętej spirali po przeciwnej stronie. Jak wskazuje szerokość pola ograniczającego – chybotanie jest niezauważalne
no wobbling
Rys.
Fig. 8. From top left to bottom right: at first Arm-Z straightens holding the tip levelled in 5 time-steps, and bends symmetrically on the opposite side. The motion is perfectly smooth and there isThe same experiment can be easily done for a larger manipulator (with 7 modules, thus 7-DoFs), as shown in Table 6. The number of modules is small enough to naturally exclude self-intersections.
In the next experiment, the number of modules is 11. Thus the self-intersections are possible. In order to avoid them, the initial twists have been increased to slightly ”unwind” the manipulator, as shown in Table 7.
In all previous experiments, there was practically no wobbling. This is, however, a major issue of discrete version of Arm-Z manipulator presented in [38]. Discrete means that
the relative twists of the modules were not continuous, as in the case described in this paper, but discrete.
In the next experiment, manipulator also moves inside a narrow gap, but the tip of the manipulator remains parallel to the working table and horizon throughout the entire action, as illustrated Table 8.
The same experiment can be easy expanded to any number of modules, as shown in Fig. 9 below.
Figure 9 shows a variation of the same experiment with reduced range of motion.
Fig. 9. The same experiment as before but with increased number of modules from 4 to 10 Rys. 9. Ten sam eksperyment co poprzednio (Rys. 8), ale ze zwiększoną liczbą modułów z 4 do 10 Fig. 10. Similar experiment with fewer modules and different bending pattern Rys. 10. Podobny eksperyment (patrz Rys. 8 i 9) z mniejszą ilością modułów i innym wzorem gięciaTable 11 shows the last experiment, where the tip of the manipulator should draw an ellipse in vertical plane and remain parallel to that plane. The motion is not perfect, as the tip is not always facing exactly the vertical plane.
This research is a part of the project titled Arm-Z: an extremely modular hyperredundant low-cost manipulator – development of control methods and efficiencyanalysis s and funded by OPUS 17 research grant No. 2019/33/B/ST8/02791 supported by the National Science Centre, Poland.
Oblique swivel joint mechanism is very attractive due to its simplicity.
However, the difficulty of its meaningful control makes is still practically useless for robotic manipulators.
This paper contribution is to bring this idea to broader audience and encourage for further experimentation by introduction of a simple but friendly user interface created in Mathematica environment.
In all experiments, the relative twists throughout entire motion is continuous and constant. The relations of values between angular velocities are very simple. This means that each experiment could be realized in reality by very simple systems described in Refs. [39, 40]. In such a case, the modules would not have to be quipped with individual motors and control units, but the motion could be transferred from one unit to another via simple gear system.
Thus we plan to build a low-tech, inexpensive street furniture with given purpose, such as sprinkler, sculpture, conveyor, etc. based on the presented types of motions.
1. Gray J., The mechanism of locomotion in snakes. „Journal of Experimental Biology”, Vol. 23, No. 2, 1946, 101–120, DOI: 10.1242/jeb.23.2.101.
2. Hirose S., Biologically Inspired Robots: Snake-Like Locomotors and Manipulators. Oxford University Press, 1993.
3. Lafmejani A.S., Doroudchi A., Farivarnejad H., He X., Aukes D., Peet M.M., Marvi H., Fisher R.E., Berman S., Kinematic modeling and trajectory tracking control of an octopus-inspired hyper-redundant robot. „IEEE Robotics and Automation Letters”, Vol. 5, No. 2, 2020, 3460–3467, DOI: 10.1109/LRA.2020.2976328.
4. Auke Jan Ijspeert and Alessandro Crespi. Online trajectory generation in an amphibious snake robot using a lamprey-like central pattern generator model. [In:] Proceedings of the 2007 IEEE International Conference on Robotics and Automation (ICRA 2007), 262–268. DOI: 10.1109/ROBOT.2007.363797.
Fig. 11. From top left to bottom right: 20 time-steps of drawing an ellipse by the tip of the manipulator. The first and second parts of the motion draws the bottom and top of the ellipse, respectively Rys. 11. Od góry po lewej do dołu po prawej: 20 kroków kreślenia elipsy w przestrzeni przez końcówkę manipulatora. Pierwsza i druga część ruchu kreśli odpowiednio dolną i górną część elipsy5. Chirikjian G.S., Burdick J.W., Design, implementation, and experiments with a thirty-degree-of-freedom ”hyper-redundant” robot. ISRAM 1992.
6. Klaassen B., Paap K.L., GMD-SNAKE2: a snake-like robot driven by wheels and a method for motion control. [In:] Proceedings 1999 IEEE International Conference on Robotics and Automation, Vol. 4, 1999, 3014–3019, IEEE, DOI: 10.1109/ROBOT.1999.774055.
7. Miller G., Snake robots for search and rescue, neurotechnology for biomimetic robots. [In:] Proceedings of the IEEE International Conference on Robotics and Automation (ICRA 2002), 16–21.
8. Choi H.R., Ryew S.M., Robotic system with active steering capability for internal inspection of urban gas pipelines „Mechatronics”, Vol. 12, No. 5, 2002, 713–736, DOI: 10.1016/S0957-4158(01)00022-8.
9. Tsakiris D.P., Sfakiotakis M., Menciassi A., La Spina G., Dario P., Polychaete-like undulatory robotic locomotion [In:] Proceedings of the 2005 IEEE International Conference on Robotics and Automation, 3018–3023, IEEE, DOI: 10.1109/ROBOT.2005.1570573.
10. Melsaac K.A., Ostrowski J.P., A geometric approach to anguilliform locomotion: modelling of an underwater eel robot. [In:] Proceedings 1999 IEEE International Conference on Robotics and Automation, Vol. 4, 1999, 2843–2848. IEEE, DOI: 10.1109/ROBOT.1999.774028.
11. Wilbur C., Vorus W., Cao Y., 14: a Lamprey-Based Undulatory Vehicle. „Neurotechnology for biomimetic robots”, 2002, DOI: 10.7551/mitpress/4962.003.0024.
12. Yamada H., Development of amphibious snake-like robot ACM-R5. 36th International Symposium on Robotics (ISR 2005), Tokyo, 2005.
13. Crespi A., Badertscher A., Guignard A., Ijspeert A.J., AmphiBot I: an amphibious snake-like robot. „Robotics and Autonomous Systems”, Vol. 50, No. 4, 2005, 163–175, DOI: 10.1016/j.robot.2004.09.015.
14. Chirikjian G.S., Burdick J.W., An obstacle avoidance algorithm for hyper-redundant manipulators. Proceedings of IEEE International Conference on Robotics and Automation, 1990, 625–631, DOI: 10.1109/ROBOT.1990.126052.
15. Siciliano B., Khatib O., Kröger T., Springer handbook of robotics, Vol. 200, Springer, 2008.
16. Murray R.M., Li Z., Sastry S.S., A mathematical introduction to robotic manipulation. CRC press, 1994.
17. Rolf M., Steil J.J., Efficient exploratory learning of inverse kinematics on a bionic elephant trunk. „IEEE Transactions on Neural Networks and Learning Systems”, Vol. 25, No. 6, 2014, 1147–1160, DOI: 10.1109/TNNLS.2013.2287890.
18. Melingui A., Escande C., Benoudjit N., Merzouki R., Mbede J.B., Qualitative approach for forward kinematic modeling of a compact bionic handling assistant trunk. „IFAC Proceedings Volumes”, Vol. 47, No. 3, 2014, 9353–9358, DOI: 10.3182/20140824-6-ZA-1003.01758.
19. Falkenhahn V., Hildebrandt A., Neumann R., Sawodny O., Dynamic control of the bionic handling assistant. „IEEE/ ASME Transactions on Mechatronics”, Vol. 22, No. 1, 2017, 6–17, DOI: 10.1109/TMECH.2016.2605820.
20. Martin-Barrio A., Roldán J.J., Terrile S., del Cerro J., Barrientos A., Application of immersive technologies and natural language to hyper-redundant robot teleoperation „Virtual Reality”, Vol. 24, No. 3, 2020, 541–555, DOI: 10.1007/s10055-019-00414-9.
21. Galicki M., A closed solution to the inverse kinematics of redundant manipulators. „Mechanism and Machine Theory”, Vol. 26, No. 2, 1991, 221–226, DOI: 10.1016/0094-114X(91)90085-I.
22. Jacak W., A discrete kinematic model of robots in the Cartesian space. „IEEE Transactions on Robotics and Automation”, Vol. 5, No. 4, 1989, 435–443, DOI: 10.1109/70.88058.
23. Jacak W., Strategies of searching for collision-free manipulator motions: automata theory approach. „Robotica”, Vol. 7, No. 2, 1989, 129–138, DOI: 10.1017/S0263574700005439.
24. Fahimi F., Ashrafiuon H., Nataraj C., An improved inverse kinematic and velocity solution for spatial hyper-redundant robots. „IEEE Transactions on Robotics and Automation”, Vol. 18, No. 1, 2002, 103–107, DOI: 10.1109/70.988980.
25. Maria da Graça Marcos, Machado J.A.T., Azevedo-Perdicoúlis T.-P., A multi-objective approach for the motion planning of redundant manipulators. „Applied Soft Computing”, Vol. 12, No. 2, 2012, 589–599, DOI: 10.1016/j.asoc.2011.11.006.
26. Galicki M., Morecki A., Finding collision-free trajectory for redundant manipulator by local information available. [In:] RoManSy 9, 1993, 61–71. Springer, DOI: 10.1007/BFb0031432.
27. Machado J.A.T., Lopes A.M., A fractional perspective on the trajectory control of redundant and hyper-redundant robot manipulators. „Applied Mathematical Modelling”, Vol. 46, 2017, 716–726, DOI: 10.1016/j.apm.2016.11.005.
28. Menon M.S., Ravi V.C., Ghosal A., Trajectory planning and obstacle avoidance for hyper-redundant serial robots „Journal of Mechanisms and Robotics”, Vol. 9, No. 4, 2017, DOI: 10.1115/1.4036571.
29. Chirikjian G.S., Burdick J.W., Hyper-redundant robot mechanisms and their applications. Proceedings IROS ‚91: IEEE/RSJ International Workshop on Intelligent Robots and Systems ‚91, DOI: 10.1109/IROS.1991.174447.
30. Chirikjian G.S., Burdick J.W., A hyper-redundant manipulator. „IEEE Robotics & Automation Magazine”, Vol. 1, No. 4, 1994, 22–29, DOI: 10.1109/100.388263.
31. Tang L., Wang J., Zheng Y., Gu G., Zhu L., Zhu X., Design of a cable-driven hyper-redundant robot with experimental validation. „International Journal of Advanced Robotic Systems”, Vol. 14, No. 5, 2017, DOI: 10.1177/1729881417734458.
32. Yang Y., Chen Y., Li Y., Chen M.Z., 3D printing of variable stiffness hyper-redundant robotic arm. 2016 IEEE International Conference on Robotics and Automation (ICRA), 2016, 3871–3877, DOI: 10.1109/ICRA.2016.7487575.
33. Wingert A., Lichter M.D., Dubowsky S., Hafez M., Hyper-redundant robot manipulators actuated by optimized binary-dielectric polymers. [In:] Smart Structures and Materials 2002: Electroactive Polymer Actuators and Devices (EAPAD), Vol. 4695, 2002, 415–423, DOI: 10.1117/12.475189.
34. Akiyuki. Oblique swivel joint mechanisms, 2022. URL http://youtu.be/fSMC4tfOqHM. A YouTube video.
35. Shammas E., Wolf A., Brown H.B., Choset H., New joint design for three-dimensional hyper redundant robots. Proceedings 2003 IEEE/RSJ International Conference on Intelligent Robots and Systems (IROS 2003), Vol. 4, 2003, 3594–3599, DOI: 10.1109/IROS.2003.1249713.
36. Shammas E., Wolf A., Choset H., Three degrees-of-freedom joint for spatial hyper-redundant robots. „Mechanism and Machine Theory”, Vol. 41, No. 2, 2006, 170–190, DOI: 10.1016/j.mechmachtheory.2005.04.008.
37. Ning K., Wörgötter F., A novel concept for building a hyper-redundant chain robot. „IEEE Transactions on Robotics”, Vol. 25, No. 6, 2009, 1237–1248, DOI: 10.1109/TRO.2009.2032968.
38. Zawidzki M., Szklarski J., Transformations of Arm-Z modular manipulator with Particle Swarm Optimization
”Advances in Engineering Software”, Vol. 126, 2018, 147–160, DOI: 10.1016/j.advengsoft.2018.05.003.
39. Zawidzka E., Szklarski J., Kiński W., Zawidzki M., Prototype of the Arm-Z modular solar tracker. [In:] Conference on Automation, 2022, Springer, 273–282, DOI: 10.1007/978-3-031-03502-9_28.
40. Zawidzka E., Kiński W., Zawidzki M., Preliminary prototype of a 4-Unit Arm-Z hyper-redundant modular manipulator. [In:] Conference on Automation, 2021, Springer, 285–294, DOI: 10.1007/978-3-030-74893-7_27.
Streszczenie: Arm-Z to koncepcja hiperredundantnego manipulatora opartego na liniowo połączonej sekwencji przystających modułów za pomocą skośnych przegubów obrotowych. Każdy moduł posiada tylko jeden stopień swobody, mianowicie skręt względem poprzedniego modułu. Mimo że koncepcja tego typu manipulatora jest stosunkowo stara i prosta, jego sterowanie jest bardzo trudne i nieintuicyjne, co powoduje ograniczone zastosowanie w praktyce przemysłowej. W niniejszej pracy przedstawiono prostą symulację Arm-Z w środowisku programistycznym Mathematica, która demonstruje kilka prostych, ale potencjalnie użytecznych ruchów.
ORCID: 0000-0003-1243-9355
ORCID: 0000-0001-8695-4400
Abstract: Extremal problems for second order hyperbolic systems with multiple time-varying lags are presented. An optimal boundary control problem for distributed hyperbolic systems with boundary conditions involving multiple time-varying lags is solved. The time horizon is fixed. Making use of Dubovitski-Milyutin scheme, necessary and sufficient conditions of optimality for the Neumann problem with the quadratic performance functionals and constrained control are derived.
Extremal problems are now playing an event-increasing role in applications of mathematical control theory in physics, automatic control and mechanics. It has been discovered that, notwithstanding the great diversity of these problems, they can be approached by a unified functional-analytic approach, first suggested by Dubovitski and Milyutin.
Such problems have been presented by Igor V. Girsanov in his monography [6] concerning mathematical theory of extremum problems.
His book [6] was apparently the first systematic exposition of a unified approach to the theory of extremal problems. This approach was based on the ideas of Dubovitski and Milyutin concerning extremum problems in the presence of constraints [2–4]. Dubovitski and Milyutin found a necessary condition for an extremum in the form of an equation set down in the language of functional analysis.
For example, in the paper [7], the Dubovitski-Milyutin method was applied for solving optimal control problems for parabolic-hyperbolic systems. Making use of the Dubovitski-Milyutin method necessary and sufficient conditions of optimality for the Dirichlet problem with the quadratic performance functional and constrained control are derived.
In the papers [8–14], the Dubovitski-Milyutin method was applied for solving boundary optimal control problems for the case of time lag parabolic equations [8] and for the case of parabolic equations involving time-varying lags [9, 10]. [11], multiple
time-varying lags [12], and integral time lags [13, 14] respectively. Sufficient conditions for the existence of a unique solution of such parabolic equations [8–14] are presented.
Consequently, in the papers [8–14], the linear quadratic problems of parabolic systems with time lags given in various forms (constant time lags [8], time-varying lags [9–11], multiple time-varying lags [12], integral time lags [13, 14] etc.) are solved.
Subsequently, in the papers [16, 17] the linear quadratic problems of optimal boundary control for hyperbolic systems with constant and time-varying delays are solved.
Making use of the Milyutin-Dubovitski approach [10], necessary and sufficient conditions of optimality with the quadratic performance indexes and constrained boundary control are derived for the Neumann problem [16, 17].
Extremal problems for multiple time-varying delays hyperbolic systems are investigated. The purpose of this paper is to show the use of Dubovitski-Milyutin theorem [10] in solving optimal control problems for second order hyperbolic systems.
As an example, an optimal boundary control problem for a system described by a linear partial differential equation of hyperbolic type with the Neumann boundary condition involving a multiple time-varying lag is considered.
Such equations constitute, in a linear approximation, a universal mathematical model for many processes in which transmission signals at a certain distance with electric, hydraulic and other long lines take place.
In the processes mentioned above time-delayed feedback signals are introduced at the boundary of a system’s spatial domain. Then the signal at the boundary of a system’s spatial domain at any time depends on the signal emitted earlier with various velocity. This leads to the boundary conditions involving multiple time-varying delays.
Sufficient conditions for the existence of a unique solution of such hyperbolic equation with the Neumann boundary condition are presented.
The performance functionals have the quadratic form. The time horizon is fixed. Finally, we impose some constraints on the boundary control. Making use of the Dubovitski-Milyutin
The existence of a unique solution of the mixed initial-boundary value problem (1)–(5) on the cylinder Q can be proved using a constructive method, i.e. by first solving problem (10) in the subcylinder Q1, and in turn in Q2 etc., until the procedure cover the whole cylinder Q. In this way the solution in the previous step determines the next one.
Consequently, using the Theorem 10.1 of [20] (vol. 2, p. 132) we can prove the following result.
Theorem 1 Let y1, y2, 0, v and f be given, with 3/2 5/2
Then, there exists a unique solution for the problem (1)–(5) defined by transposition (10). Moreover, 3/2
j
and 5/2 ˆ , j yt for j = 1, ...
We refer to Lions and Magenes ([20], vol. 2) for the definition and properties on Hr,s(Q) and (Hr,s)’ respectively. In the sequel, we shall fix 3,3 fQ
In this paper we shall consider the optimal boundary control problem i.e. 2vL
Let us denote by the space of states and by 2UL the space of controls. The time horizon T is fixed in our problem.
The performance functional is given by (15)
where 0 i and 12 0, zd is a given element in 1,2 , HQ and N is a strictly positive linear operator on into 2L
Finally, we assume the following constraints on the control: ad vU (16)
where Uad is a closed, convex set with non-empty interior, a subset of U
Let y(x, t, v) denote the solution of (1)–(5) at (x, t) corresponding to a given control ad vU We note from the Theorem 1 that for any ad vU the cost function (15) is well defined since
The optimal control problem (1)–(5), (15), (16) will be solved as the optimization one in which the function v is the unknown function. Making use of Dubovitski-Milyutin theorem [10] we shall derive the necessary and sufficient conditions of optimality for the optimization problem (1)–(5), (15), (16).
The solution of the stated optimal control problem is equivalent to seeking a pair which satisfies the equation (1)–(5) and minimizing the performance functional (15) with the constraints on the control (16).
Theorem 2 The solution of the optimization problem (1)–(5), (15), (16) exists and it is unique with the assumptions mentioned above; the necessary and sufficient conditions of the optimality are characterized by the following system of partial differential equations and inequalities.
According to the Dubovitski-Milyutin theorem [10], we approximate the set representing the inequality constraints by the regular admissible cone, the equality constraint by the regular tangent cone and the performance functional by the regular improvement cone.
The set Q1 representing the equality constraint has the form
We construct the regular tangent cone of the set Q1 using the Lusternik theorem (Theorem 9.1 [6]). For this purpose, we define the operator P in the form
So we note the functional 2 , fyv as follows
The operator P is the mapping from the space into the space
The Fréchet differential of the operator P can be written in the following form: (31)
Really, 2 2 t (Theorem 2.8 [21]), A(t) (Theorem 2.1 [19]) and A (Theorem 2.3 [20]) are linear and bounded mappings.
Using Theorem 1, we can prove that P is the operator „one to one” from the space onto the space
where: 1 0 fyyY (Theorem 10.1 [6])
2 fv is a support functional to the set Uad in a point
(Theorem 10.5 [6]).
c) Analysis of the cost function
Using Theorem 7.5 [6] we find the regular improvement cone of the performance functional (15)
where: 00 ,, Iyvyv is the Fréchet differential of the performance functional (15) and it can be written as
Considering that the assumptions of the Lusternik’s theorem are fulfilled, we can write down the regular tangent cone for the set Q1 in a point (y0 ,v0) in the form
(32)
It is easy to notice that it is a subspace. Therefore, using Theorem 10.1 [6] we know the form of the functional belonging to the adjoint cone
00 11 ,0,,, fyvyvRTCQyv (33)
The set Q2 = Y ×Uad representing the inequality constraints is a closed and convex one with non-empty interior in the space E
Using Theorem 10.5 [6] we find the functional belonging to the adjoint regular admissible cone, i.e.
22 ,, , fyvRACQyv
00
We can note if E1,E2 are two linear topological spaces, then the adjoint space to E = E1×E2 has the form
121122 ,;, EffffEfE
On the basis of Theorem 10.2 [6] we find the functional belonging to the adjoint regular improvement cone, which has the form
where: 0 0.
d) Analysis of Euler-Lagrange’s equation
The Euler-Lagrange’s equation for our optimization problem has the form
Let p(x, t) be the solution of (22)–(26) for (y0 ,v0). Then, p(
) is defined by transposition, i.e.
,,,0,0,21 MypApyplpqpypy and y satisfies (1)–(5).
We observe that, for given zd and v, equations (22)–(26) can be solved backward in time starting from t = T, i.e. first solving problem (22)–(26) in the subcylinder Q1, and in turn in Qk−1 etc., until the procedure covers the whole cylinder Q. For this purpose, we may apply Theorem 1.
Let the hypothesis of Theorem 1 be satisfied. Then, for given 1,2 , d zHQ and any 2 , vL there exists a unique solution
to the problem (22)–(26) defined by transposition (39).
2. Dubovitski A.Ya., Milyutin A.A., The extremum problem in the presence of constraints. „Doklady Akademii Nauk SSSR”, Vol. 149, No. 4, 1963, 759–762.
3. Dubovitski A.Ya., Milyutin A.A., Some optimum problems for linear systems. „Avtomatika i Telemekhanika”, Vol. 24, No. 12, 1963, 1616–1625.
4. Dubovitski A.Ya., Milyutin A.A., Second variations in extremum problems with constraints. „Doklady Akademii Nauk SSSR”, Vol. 160, No. 1, 1965, 18–21.
where: 2 is a canonical isomorphism of 5/25/2 H into 5/25/2 H
000 2 ,0 ad GpvNvvv vU (55)
Moreover, it can be proved the following result.
Let the hypothesis of Theorem 1 be satisfied. Then, for given 5/25/2 d zH and any 2 , vL there exists a unique solution 3,3 3,3 pvHQQ to the problem (50)–(54) defined by transposition (39).
The idea of the proof of the Theorem 3 is the same as in the case of the Theorem 2.
In the case of performance functionals (15) and (49) with 1 0 and 2 0, the optimal control problem reduces to the minimizing of the functional on a closed and convex subset in a Hilbert space. Then, the optimization problem is equivalent to a quadratic programming one ([15, 18] which can be solved by the use of the well-known algorithms, e.g. Gilbert’s [5, 15, 18]).
5. Gilbert E.S., An iterative procedure for computing the minimum of a quadratic form on a convex set. „SIAM Journal on Control”, Vol. 4, No. 1, 1966, 61–80. DOI: 10.1137/0304007.
6. Girsanov I.V., Lectures on the Mathematical Theory of Extremum Problems, Publishing House of the University of Moscow, Moscow, 1970 (in Russian).
7. Kowalewski A., On optimal control problem for parabolic-hyperbolic system. „Problems of Control and Information Theory”, Vol. 15, No. 5, 1986, 349–359.
8. Kowalewski A., Miśkowicz M., Extremal problems for time lag parabolic systems. Proceedings of the 21st International Conference of Process Control (PC), 446–451, Strbske Pleso, Slovakia, June 6-9, 2017, DOI: 10.1109/PC.2017.7976255.
9. Kowalewski A., Extremal Problems for Distributed Parabolic Systems with Boundary Conditions involving Time-Varying Lags. Proceedings of the 22nd International Conference on Methods and Models in Automation and Robotics (MMAR), 447–452, Międzyzdroje, Poland, August 28-31, 2017, DOI: 10.1109/MMAR.2017.8046869.
10. Kowalewski A., Extremal problems for parabolic systems with time-varying lags. „Archives of Control Sciences”, Vol. 28, No. 1, 2018, 89–104, DOI: 10.24425/119078.
The derived conditions of the optimality (Theorems 2 and 3) are original from the point of view of application of the Dubovitski-Milyutin theorem [10] in solving optimal boundary control problems for second order hyperbolic systems in which multiple time-varying lags appear in the Neumann boundary conditions. The existence and uniqueness of solutions for such hyperbolic systems are presented – Theorem 1. The optimal control is characterized by using the adjoint equations – Lemmas 1 and 2. Necessary and sufficient conditions of optimality with the quadratic performance functionals (15) and (49) and constrained control (16) are derived for the Neumann problem (Theorems 2 and 3). Moreover, the optimization problems presented here constitute a generalization of optimal control problems considered in [16, 17] for hyperbolic systems with constant and time-varying lags appearing in the Neumann boundary conditions. The proposed methodology based on the Dubovitski-Milyutin scheme can be presented as a specific case study concerning hyperbolic systems with the Neumann boundary conditions involving integral time delays. The same procedure can be applied to solving optimal control problems for non-linear hyperbolic systems. Another direction of research will be numerical examples concerning the determination of optimal boundary control with constraints for multiple time-varying delay hyperbolic systems. Such problems can be solved using control synthesis methods [1, 22].
1. Baranowski J., Mitkowski W., Stabilization of LC ladder network with the help of delayed output feedback. „Control and Cybernetics”, Vol. 41, No. 1, 2012, 13–34.
11. Kowalewski A., Extremal problems for infinite order parabolic systems with time-varying lags. „Advances Intelligent and Soft Computing”, Vol. 1196, 2020, 3–15, Springer Nature Switzerland AG, DOI: 10.1007/978-3-030-50936-1_1.
12. Kowalewski A., Extremal problems for parabolic systems with multiple time-varying lags. Proceedings of 23rd International Conference on Methods and Models in Automation and Robotics (MMAR), 791–796, Międzyzdroje, Poland, August 27–30, 2018, DOI: 10.1109/MMAR.2018.8485815.
13. Kowalewski A., Miśkowicz M., Extremal problems for integral time lag parabolic systems. Proceedings of the 24th International Conference on Methods and Models in Automation and Robotics (MMAR), 7–12, Międzyzdroje, Poland, August 26–29, 2019, DOI: 10.1109/MMAR.2019.8864638.
14. Kowalewski A., Miśkowicz M., Extremal problems for infinite order parabolic systems with boundary conditions involving integral time lags. „Pomiary Automatyka Robotyka”, Vol. 26, No. 4, 2022, 37–42, DOI: 10.14313/PAR_246/37.
15. Kowalewski A., Duda J., On some optimal control problem for a parabolic system with boundary condition involving a time-varying lag. „IMA Journal of Mathematical Control and Information”, Vol. 9, No. 2, 1992, 131–146, DOI: 10.1093/imamci/9.2.131.
16. Kowalewski A., Extremal problems for time lag hyperbolic systems. Proceedings of the 25th International Conference on Methods and Models in Automation and Robotics (MMAR), 245–250, Międzyzdroje, Poland, August 23–26, 2021, DOI: 10.1109/MMAR49549.2021.9528456.
17. Kowalewski A., Extremal problems for hyperbolic systems with boundary conditions involving time-varying delays. Proceedings of the 26th International Conference on Methods and Models in Automation and Robotics
(MMAR), 122–127, Międzyzdroje, Poland, August 22–25, 2022, DOI: 10.1109/MMAR55195.2022.9874285.
18. Kowalewski A., Optimal Control of Infinite Dimensional Distributed Parameter Systems with Delays. AGH University of Science and Technology Press, Cracow, 2001.
19. Lions J.L., Optimal Control of Systems Governed by Partial Differential Equations, Springer-Verlag, Berlin, 1971.
20. Lions J.L., Magenes E., Non-Homogeneous Boundary Value Problems and Applications, Vols. 1 and 2, Springer-Verlag, Berlin, 1972.
21. Maslov V.P., Operators Methods, Publisher „Science”, Moscow, 1973 (in Russian).
22. Mitkowski W., Remarks about Controllability of Hyperbolic System. XVIII Krajowa Konferencja Automatyki, Wrocław 8–10.09.2014, CD, position 85 (7 pages).
Streszczenie: Zaprezentowano ekstremalne problemy dla systemów hiperbolicznych z wielokrotnymi zmiennymi opóźnieniami czasowymi. Rozwiązano problem optymalnego sterowania brzegowego dla systemów hiperbolicznych drugiego rzędu, w których wielokrotne zmienne opóźnienia czasowe występują w warunkach brzegowych typu Neumanna. Tego rodzaju równania stanowią w liniowym przybliżeniu uniwersalny model matematyczny procesów fizycznych, w których ma miejsce przesyłanie sygnałów na odległość w liniach długich typu elektrycznego, hydraulicznego i innych. Korzystając z metody Dubowickiego-Milutina wyprowadzono warunki konieczne i wystarczające optymalności dla problemu liniowo-kwadratowego.
ORCID: 0000-0001-5792-2039
Proces kompostowania jest powszechnie stosowany w gospodarce odpadami jako metoda przekształcania lub stabilizacji odpadów organicznych. Ze względu na złożony, nieliniowy charakter zachodzących w nim zjawisk biologicznych oraz fizykochemicznych, jest on stosunkowo trudny z punktu widzenia predykcji oraz sterowania. Sterowanie procesem ma tu na celu uzyskiwanie w określonym horyzoncie czasowym produktu finalnego, czyli zwykle kompostu spełniającego określone wymagania jakościowe. W artykule zaprezentowano prosty model matematyczny procesu kompostowania z wymuszonym napowietrzaniem, potencjalnie umożliwiającym realizację wspomnianego celu sterowania. Opracowano model drugiego rzędu, z dwiema wielkościami wejściowymi reprezentującymi zewnętrzne oddziaływania na proces. Na podstawie modelu matematycznego przekształconego do postaci równań stanu, zbudowano w środowisku MATLAB/Simulink model komputerowy bioreaktora, który następnie wykorzystano do przeprowadzenia badań symulacyjnych. Pokazano, że możliwe jest oddziaływanie na proces za pomocą wymuszonego napowietrzania, bezpośrednio wpływającego na zmianę temperatury w bioreaktorze, a w konsekwencji również na czas otrzymania końcowego produktu reakcji. Wyniki analizy właściwości dynamicznych procesu, przeprowadzonej z wykorzystaniem modelu zlinearyzowanego wzdłuż wybranej, nominalnej trajektorii stanu, wskazują na zmienny charakter jego stabilności – począwszy od niestabilności w początkowych fazach reakcji, przez stabilizację w fazie pośredniej, aż do stabilności asymptotycznej, zakończonej osiągnięciem stanu równowagi.
Kompostowanie jest istotnym procesem wykorzystywanym w przemyśle gospodarki odpadami. Jako sposób zagospodarowania odpadów jest powszechnie stosowane w Europie; ma ono duże znaczenie również dla polskiego segmentu gospodarki odpadami [1, 2]. Proces kompostowania jest ważny także ze względu na niskie koszty budowy instalacji procesowych oraz duży potencjał proekologiczny. Proces ten wykorzystywany jest do stabilizacji odpadów oraz do produkcji kompostu [3]. Jego
właściwy przebieg gwarantuje również ograniczenie powstawania substancji niebezpiecznych dla człowieka lub szkodliwych dla środowiska [4–6]. Proces kompostowania zachodzi w warunkach tlenowych, w których mikroorganizmy rozkładają materię organiczną. Jego produktami są dwutlenek węgla, woda, energia termiczna (ET) oraz kompost lub tzw. stabilizat w rozumieniu norm prawnych [7]:
mikroorganizmy + materia organiczna + O2
H2O + CO2 + kompost + ET (1)
Przemysłowy proces kompostowania przebiegać może w systemie otwartym, czyli w pryzmach z okresowym mieszaniem odpadów lub w systemie zamkniętym, czyli w tzw. bioreaktorze. Instalacje pracujące w systemie zamkniętym zazwyczaj mają postać silosów lub kontenerów [8]. W badaniach laboratoryjnych nad procesem kompostowania w systemie zamkniętym najczęściej stosowana jest aparatura w postaci izolowanych termicznie naczyń. Ich wielkość może się wahać, w zależności od potrzeb badawczych, od kilku do kilkuset litrów [9]. Proces kom-
Na potrzeby budowy modelu matematycznego omawianego bioreaktora przyjęto szereg założeń upraszczających. Zachodzący w nim proces potraktowano jako jednorodny w całym jego przekroju. Zakłada się, że cała objętość bioreaktora jest równomiernie wypełniona wsadem, w którym znajduje się materia organiczna podlegająca procesowi rozkładu. Ze względu na niewielki wpływ na proces pominięto tu przepływ energii termicznej wynikający z entalpii wody. Nie uwzględniono również wpływu stężenia tlenu i wody (wilgotności) na proces. Za najistotniejszą dla przebiegu procesu wielkość fizyczną uznano temperaturę wsadu, mającą kluczowy wpływ na proces namnażania mikroorganizmów.
Model matematyczny procesu oparty jest na bilansie termicznym, odnoszącym się do zachodzącego w bioreaktorze procesu kompostowania. Schemat poglądowy, ilustrujący zachodzące tu zależności bilansowe, przedstawiono na Rys. 2. Bilans ten w postaci ogólnej można zapisać następująco:
B RIA , dQt QtQtQt dt (2)
gdzie: QB(t) – całkowita energia cieplna zgromadzona we wsadzie bioreaktora [J], R Qt – strumień ciepła generowanego biologicznie (wskutek rozkładu materii organicznej) [J/h], I Qt –strumień ciepła przekazywanego do (względnie odbieranego z) otoczenia wskutek napowietrzania bioreaktora [J/h], Qt –strumień ciepła przekazywanego do (względnie odbieranego z) otoczenia przez powierzchnię reaktora [J/h], t – czas [h].
Poniżej rozwinięto poszczególne składniki równania bilansowego (2). Przyjmujemy tu, że całkowitą energię cieplną wsadu bioreaktora QB(t) można wyrazić jako:
QB(t) = mBcBTB(t) = BVcBTB(t), (3)
gdzie: TB(t) – temperatura wsadu bioreaktora (temperatura procesu) [℃], mB – masa wsadu bioreaktora [kg], cB – ciepło właściwe wsadu [J/(kg·K)], B – gęstość wsadu [kg/m3], V –objętość wsadu (objętość bioreaktora) [m3].
Zmiany energii (3) w czasie można zapisać, przy założeniu stałych wartości parametrów: mB, cB, B oraz V w następującej postaci:
BBB BBBB dQtdTtdTt mcVc dtdtdt (4)
Strumień ciepła R Qt generowanego biologicznie (wskutek reakcji rozkładu) opisany jest następującym równaniem podstawowym [10]:
RS , dXt QtmY dt (5)
gdzie: mS – masa tzw. substratu, czyli tej części wsadu, która podlega reakcji rozkładu [kg], Y – metaboliczny współczynnik wydzielania ciepła [J/kg], X(t) – zawartość kompostu w substracie [kg/kg].
Masę substratu w równaniu (5) można wyrazić następująco:
mS = SV(1 – ), (6)
gdzie: S – gęstość substratu [kg/m3], – porowatość substratu [–].
Z kolei zmiana zawartości kompostu w substracie wskutek jego rozkładu może być opisana następująco [10]:
max 1, dXtXt tXt dtX (7)
gdzie: X max – maksymalna zawartość kompostu w substracie [kg/kg].
Wielkość μ(t) [h–1] występująca we wzorze (7) reprezentuje tzw. współczynnik tempa wzrostu mikroorganizmów zależny od temperatury procesu TB(t) i można ją wyznaczyć z następującej zależności [19]:
()2() , TtTTt t T (8)
BoptB max 2 opt
gdzie: μmax – największa możliwa szybkość wzrostu mikroorganizmów [h–1], T opt – optymalna (ze względu na szybkość namnażania mikroorganizmów) temperatura procesu [℃].
Po uwzględnieniu zależności (6)–(8), równanie (5) można zapisać w następującej postaci:
11. Xt QtVYtXt X (9)
RS max
Strumień ciepła przepływającego z (względnie do) bioreaktora do (względnie z) otoczenia wskutek aktywnego napowietrzania opisany jest następującą zależnością:
IIABA , QtmtcTtTt (10)
gdzie: I mt – strumień masowy powietrza wlotowego [kg/h], cA – ciepło właściwe suchego powietrza [J/(kg·K)], TA(t) – temperatura otoczenia [℃].
Równanie opisujące przenikanie ciepła przez przegrodę stanowiącą powierzchnię bioreaktora ma następującą postać [19]:
ABA , QtUATtTt (11)
gdzie: U – całkowity współczynnik przenikania ciepła [J/(h·m2·K)], A – powierzchnia bioreaktora [m2].
Wyprowadzone zależności bilansowe (2)–(11) przekształcone zostaną w kolejnej części do postaci równań stanu, dogodniejszej dla modelowania komputerowego, a także dla analizy właściwości dynamicznych procesu oraz realizacji potencjalnych zadań sterowania.
Zaprezentowane tu równania stanu będą opisywały zmiany w czasie dwóch zmiennych stanu charakteryzujących proces: temperatury wsadu bioreaktora T B( t ) oraz koncentracji kompostu we wsadzie X ( t ). Zapisane zostaną one w formie dwóch nieliniowych równań różniczkowych pierwszego rzędu. Taki opis matematyczny procesu, przy założeniu znajomości warunków początkowych oraz oddziaływań zewnętrznych, umożliwi określenie jego stanu w dowolnej chwili czasowej [21].
Na Rys. 3 przedstawiono bioreaktor jako obiekt sterowania, dla którego sygnałami wejściowymi są: strumień masowy wtłaczanego powietrza I mt oraz temperatura otoczenia
Rys. 5. Wirtualne stanowisko przeznaczone do badań symulacyjnych procesu kompostowania: a) zgrupowany model procesu wraz z „oprzyrządowaniem”; b) okno dialogowe do wprowadzania wartości parametrów Fig. 5. Virtual stand for simulation tests of the composting process: a) Grouped process model with instrumentation; b) Dialog box for entering parameter values
Tab.1. Parametry procesu, warunki początkowe oraz wymuszenia zewnętrzne uwzględnione w badaniach symulacyjnych Tab. 1. Process parameters, initial conditions and external signals included in simulation tests
Symbol Nazwa
A powierzchnia bioreaktora1,18 m2
cB ciepło właściwe wsadu 2 038 J/(kg·K) [10]
cA ciepło właściwe suchego powietrza 1 180 J/(kg·K) tablice fizyczne
T
U całkowity współczynnik przenikania ciepła 50 000 J/(h·m2·K)*
V objętość wsadu/bioreaktora0,1 m3
X max maksymalna zawartość kompostu w substracie 0,125 kg/kg [20]
Y metaboliczny współczynnik wydzielania ciepła
μ
kompostu w substracie
I mt strumień masowy powietrza wlotowego 0 kg/h*
* Wartości oznaczone gwiazdką podlegały zmianom w trakcie różnych scenariuszy badań symulacyjnych, w celu określenia ich wpływu na przebieg procesu. W przypadku braku doprecyzowania warunków, dla których przebiegały poszczególne badania, należy założyć, że przyjęto wartości zamieszczone w Tab. 1.
W rozdziale przedstawiono wyniki badań symulacyjnych, których głównym celem było dostarczenie najważniejszych informacji na temat właściwości dynamicznych procesu zachodzącego w bioreaktorze. Badania dostarczyły m.in. informacji na temat wpływu wybranych parametrów układu, warunków początkowych oraz sygnałów wejściowych na przebiegi czasowe jego zmiennych stanu. Umożliwiły lepsze zrozumienie samego procesu oraz zachodzących w nim zjawisk. Inną ważną kwestią,
poruszoną w dalszej części artykułu, była analiza właściwości dynamicznych (głównie stabilności) procesu. Jak pokazano w części 5, ze względu na nieliniowość równań stanu (12) i (13), właściwości te silnie zależą od bieżącego punktu pracy układu, tzn. od bieżącego etapu reakcji.
W celu ułatwienia interpretacji zaprezentowanych dalej wyników, istotne jest zrozumienie podstawowych mechanizmów biologicznych wpływających na przebieg procesu, a które krótko zostały omówione poniżej. Równanie (8) pokazuje zależność tempa wzrostu mikroorganizmów μ(t) od temperatury procesu
niejszej wiedzy o procesie, a w konsekwencji skutkować będzie wzrostem rzędu oraz stopnia złożoności modelu.
Przedstawione w artykule wyniki badań odpowiedzi bioreaktora na sygnał wejściowy reprezentujący strumień napowietrzający wykazały, że możliwe jest jego wykorzystanie jako potencjalnego sygnału sterującego procesem. Analizując wykresy z Rys. 9. można odnieść wrażanie, że wpływ napowietrzania na przebieg procesu jest stosunkowo niewielki – jednak wynika on z przyjętych tu stałych wartości strumieni. W przypadku dużych wartości strumienia, napowietrzanie prowadzi do hamowania procesu przy jego temperaturze niższej od optymalnej. Dlatego przy niskiej (TB < Topt) wartości temperatury wsadu należałoby ograniczyć napowietrzanie, aby zbytnio go nie wychładzać. Z kolei, gdy jest ona zbyt wysoka (TB > Topt) – wskazane byłoby zwiększenie napowietrzania w celu jej obniżenia. W konsekwencji będziemy dążyć do utrzymania przez jak najdłuższy czas optymalnej temperatury wsadu, co w efekcie powinno przyśpieszyć czas powstawania produktu końcowego.
Analiza właściwości zlinearyzowanych modeli procesu wskazuje na zróżnicowany charakter jego dynamiki, począwszy od jego niestabilności na etapie jego rozpoczęcia oraz rozwoju mikroorganizmów, przez stabilizację na etapie osiągnięcia maksymalnej temperatury wsadu, aż do w pełni stabilnego charakteru w ostatnim etapie, kończącym się osiągnięciem przez proces stanu równowagi. W ramach dalszych prac planowana jest rozbudowa modelu o wspomniane wyżej dodatkowe wielkości fizyczne, mające wpływ na proces zachodzący w bioreaktorze. Ponadto planowane są badania dotyczące zaprojektowania układu sterowania, którego celem byłaby optymalizacja warunków pracy bioreaktora poprzez zapewnienie odpowiednich warunków dla mikroorganizmów, a w konsekwencji zwiększenie wydajności i jakości produktu procesu kompostowania. Planowana jest także weryfikacja otrzymanych modeli oraz wyników badań symulacyjnych na obiekcie rzeczywistym, zbudowanym w skali laboratoryjnej lub półprzemysłowej.
8. Czekała W., Grzelak M., Rodrigez Carmona P., Witaszek K., Instalacje do przemysłowego kompostowania bioodpadów: wady i zalety, „Technika Rolnicza Ogrodnicza Leśna”, Nr 2, 2013, 23–25.
9. Sołowiej P., Koncepcja budowy bioreaktora do kompostowania biomasy – stanowisko badawcze, „Inżynieria Rolnicza”, R. 12, Nr 11, 2008, 227–321.
10. Białobrzeski I., Dziejowski J., Symulacyjny model procesu wytwarzania kompostu w biostabilizatorze DANO, „Inżynieria Rolnicza”, R. 19, Nr 14, 2005, 29–36.
11. Yamada Y., Kawasa Y., Aerobic composting of waste activated sludge: Kinetic analysis for microbiological reaction and oxygen consumption, “Waste Management”, Vol. 26, No. 1, 2006, 49–61, DOI: 10.1016/j.wasman.2005.03.012.
12. Szűcs E., Modelowanie matematyczne w fizyce i technice, 1977, Wydawnictwa Naukowo Techniczne, Warszawa.
13. Mason I.G., Mathematical modeling of composting process: A review, “Waste Management”, Vol. 26, No. 1, 2006, 3–21, DOI: 10.1016/j.wasman.2005.01.021.
14. Finger M.E., Hatch R.T., Aerobic microbial growth in semisolid matrices: heat and mass transfer limitation, “Biotechnology and Bioengineering”, Vol. 18, No. 9, 1976, 1193–1218, DOI: 10.1002/bit.260180904.
15. Ajmal M., Aiping S., Uddin S., Awais M., Faheem M., Ye L., Rehman K., Saif Ullah M., Shi Y., A review on mathematical modeling of in-vessel composting process and energy balance, “Biomass Conversion and Biorefinery”, Vol. 12, 2020, DOI: 10.1007/s13399-020-00883-y.
16. Vidriales-Escobar G., Rentería-Tamayo R., Alatriste-Mondragón F., González-Ortega O., Mathematical modeling of a composting process in a small-scale tubular bioreactor, “Chemical Engineering Research and Design”, Vol. 120, 2017, 360–371, DOI: 10.1016/j.cherd.2017.02.006.
17. Khater G., Bahnasawy A.H., Ali S.A., Mathematical model of compost pile temperature prediction, “Journal of Environmental & Analytical Toxicology”, Vol. 4, No. 6, 2014. DOI: 10.4172/2161-0525.1000242.
1. Bochenek D., Ochrona środowiska w 2018 r., GUS, 2018.
2. Forewicz K., Wsparcie proekologicznych inwestycji w banku ochrony środowiska, „Środowisko”, Vol. 526, 2016, 23–27.
3. Sidełko R., Seweryn K., Walendzik B., Optymalizacja procesu kompostowania w warunkach rzeczywistych, „Rocznik Ochrona Środowiska”, Vol. 13, 2011, 681–691.
4. Nordahl S., Davkota J., Amirebrahimi J., Smith S., Breunig H., Prable C., Satchwell J., Jin L., Brown N., Kirchstetter T., Scown C., Life–Cycle Greenhouse Gas Emissions and Human Health Trade–Offs of Organic Waste Management Strategies, “Environmental Science & Technology”, Vol. 54, 2020, 9200–9209, DOI: 10.1021/acs.est.0c00364.
5. Kamyaba H., Limb J., Khademic T., Ho W., Ahmade R., Hashimd H., Siongf H., Keyvanfarg A., Lee C., Greenhouse Gas Emission of Organic Waste Composting: A Case Study of University Technology Malaysia Green Campus Flagship Project, “Jurnal Teknologi”, Vol. 74, No. 4, 2015, 113–117, DOI: 10.11113/jt.v74.4618.
6. McNicol G., Jeliazkvski J., Francois J.J., Kramer S., Ryals R., Climate change mitigation potential in sanitation via off-site composting of human waste, “Nature Climate Change”, Vol. 10, 2020, 1–12, DOI: 0.1038/s41558-020-0782-4.
7. Poz. 1052, Rozporządzenie ministra środowiska z dnia 11 września 2012 r. w sprawie mechaniczno-biologicznego przetwarzania zmieszanych odpadów komunalnych, na podstawie art. 14 ust. 10 ustawy z dnia 27 kwietnia 2001 r. o odpadach (Dz. U. z 2010 r. Nr 185, poz. 1243, z późn. zm.).
18. Papračanin E., Petric I., Mathematical modeling and simulation of the composting process in a pilot reactor, “Bulletin of the Chemists and Technologists of Bosnia and Herzegovina”, Vol. 47, 2017, 39–48.
19. Kaiser J., Modelling composting as a microbial ecosystem: a simulation approach, “Ecological Modelling”, Vol. 91, No. 1-3, 1996, 25–37, DOI: 10.1016/0304-3800(95)00157-3.
20. Petric I., Mustafić N., Dynamic modeling the composting process of the mixture of poultry manure and wheat straw, “Journal of Environmental Management”, Vol. 161, 2015, 392–401, DOI: 10.1016/j.jenvman.2015.07.033.
21. Kaczorek T., Teoria układów regulacji automatycznej, 1977, Wydawnictwa Naukowo-Techniczne, Warszawa.
22. Raina M, Bacterial Growth, Review of Basic Microbiological Concepts, 2015, 37–54.
23. Skupin P., Metzger M., Oscillatory Behavior Control in Continuous Fermentation Processes, “IFAC-PapersOnLine”, Vol. 48, No. 8, 2015, 1114–1119, DOI: 10.1016/j.ifacol.2015.09.117.
24. Ciesielski A., Nieliniowe sterowanie predykcyjne procesem produkcji bioetanolu metodą fermentacji ciągłej, Rozprawa doktorska, Politechnika Krakowska, 2021.
25. Sangsurasak P., Mitchellt D.A., Validation of a model describing two dimensional dynamic heat transfer during solid-state fermentation in packed bed bioreactors, “Biotechnology and Bioengineering”, Vol. 60, No. 6, 1998, 739–749, DOI: 10.1002/(SICI)1097-0290(19981220)60:6<739::AID-BIT10>3.0.CO;2-U.
The composting process is commonly used in waste management as a method of converting or stabilizing organic waste. Due to the complex, non-linear nature of biological and physicochemical phenomena involved, this process is relatively difficult to predict and control. The control is usually aimed at obtaining the final product, that is, the compost that meets legal standards. The article presents a simple mathematical model of the composting process with forced aeration, which will potentially facilitate the control task. A second order model was developed, with two inputs signals. Based on the mathematical model in the form of the state equations, the computer model of the bioreactor was built in the MATLAB/Simulink environment, which was then used to conduct different simulation tests. It was shown that it is possible to control the process using forced aeration, directly influencing the temperature changes in the bioreactor, and consequently also the time of obtaining the final product of the reaction. The analysis of the dynamic properties of the process performed using its model linearized about some nominal state trajectory shows the changes in its internal stability—starting from the unstable character in the initial phases of the reaction, through stabilization in its intermediate phase, up to the asymptotic stability, ending in the stable equilibrium state.
ORCID: 0000-0002-5744-808X
Abstract: Asignificant rise in Artificial Intelligence (AI) has impacted many applications around us, so much so that AI has now been increasingly used in safety-critical applications. AI at the edge is the reality, which means performing the data computation closer to the source of the data, as opposed to performing it on the cloud. Safety-critical applications have strict reliability requirements; therefore, it is essential that AI models running on the edge (i.e., hardware) must fulfill the required safety standards. In the vast field of AI, Deep Neural Networks (DNNs) are the focal point of this survey as it has continued to produce extraordinary outcomes in various applications .i.e medical, automotive, aerospace, defense, etc. Traditional reliability techniques for DNNs implementation are not always practical, as they fail to exploit the unique characteristics of the DNNs. Furthermore, it is also essential to understand the targeted edge hardware because the impact of the faults can be different in ASICs and FPGAs. Therefore, in this survey, first, we have examined the impact of the fault in ASICs and FPGAs, and then we seek to provide a glimpse of the recent progress made towards the fault-tolerant DNNs. We have discussed several factors that can impact the reliability of the DNNs. Further, we have extended this discussion to shed light on many state-of-the-art fault mitigation techniques for DNNs.
Deep Learning (DL), as a subset of Machine Learning, has revolutionized many tasks in recent years, ranging from data analytics, natural language processing, image classification, video processing to speech recognition, recommender systems, etc. These DL models learn from the data during the training phase and make output predictions during the inference phase. In cases such as image classification, DNN algorithms have surpassed human-level accuracy. This and many other similar breakthroughs in deep learning have motivated researchers to explore deep learning in safety-critical applications, i.e., automotive, space, defense, drones, industry, and health.
DNN models will eventually be deployed on the hardware (ASICs, FPGAs). There are two significant challenges at hand in deploying these models on hardware. a) First is the resource-intensive nature of running deep learning models on the hardware. Deep Learning models are getting bigger and bigger to solve more complex problems. The training phase of the model
generally happens on the cloud or powerful machines. During the inference phase, DNN models are deployed on the target hardware. The target hardware should be able to process a massive amount of data, i.e., roughly 4 TB in case of a self-driving car scenario. b) The second challenge comes in the form of the reliability of the hardware on which these models are deployed. The reliability analysis of the DNNs is extremely important for the reason that, during the inference phase, a DNN model needs to take essential and critical decisions. e.g., apply brakes if a pedestrian is detected in front of the car.
To fulfill our high-performance needs, over the years, we have observed the trend of transistor size shrinking to the Very Deep Sub-Micron (VDSM) level. On the one hand, this scaling has increased the computing performance and helped move the DNN inference processing from the cloud to the edge. On the other hand, transistor scaling leads to increased sensibility to transient faults due to lower threshold voltages and tighter noise margins [42]. Previously, this problem was relevant to the hostile environments, i.e., space, but now due to transistors scaling to VDSM level, safety-critical applications at the ground level are also prone to transient faults [8]. Most of the studies are based on single-bit errors. Due to the technology scaling, multi-bit errors are also on the rise [30]. Thus, for a reliable DNN inference on edge, a thoroughly verified fault-tolerant hardware is required.
In this survey, we have investigated state-of-the-art faulttolerant methodologies for characterizing and improving the resilience of DNN algorithms processing on edge. Figure 1 provides an overview of the study. Starting from section 2, we have discussed the impact of faults in integrated circuits. This section
includes the discussion related to the importance and classifications of fault models. Additionally, there is a discussion around the impact of fault in ASICs and FPGAs. Understanding the difference between these two hardware platforms is essential because a fault impact in ASICs and FPGAs can be different. Section 3 has shed some light on the deep neural networks design cycle and taxonomy. A discussion about MLPs, CNNs, and SNNs is also part of the section. In section 4 and 5, we have extended the discussion towards the fault resiliency of the deep neural networks and various fault-tolerant methodologies. There are so many other factors that can affect the reliability of the deep learning models. i.e., network architecture, layer type, data type, bit position, pruning, quantization, and depth of the model. Therefore, it is necessary to analyze the DNNs from different perspectives. Section 6 concludes this survey.
-one. These faults are permanent in nature and are caused by post-manufacturing defects and transistor aging [41].
Single Event Transient (SET): The phenomenon of the Single Event Transient (SET) occurs when a high-energy particle strikes on a combinational circuit, it causes a transient voltage disturbance due to charge deposition. If the energy of the particle crosses a certain threshold, the end effect of it is a Single Event Transient (Glitch) in the combination circuit. SETs can occur in both ASICs and FPGAs. These transient faults are temporary and are also called soft errors. In [13] was investigated thet probability of SETs becoming an SEU. Generally, the analysis of SETs is very complex in large designs, which are composed of many paths. Techniques such as Timing Analysis can be used to investigate the SETs in large and complex designs.
Single Event Upset (SEU): We have discussed above the cause of transient faults. If the same transient fault can propagate to a storage element and gets latched, it becomes a Single Event Upset (SEU). Storage elements can be system memory, registers, or configuration memory cells in FPGAs. Based on the number of upsets that occur in a circuit, SEUs can lead to first, second, and third-order effects. A single SEU affecting a single bit is often classified as a first-order effect. When a charged particle affects multiple bits, it is considered as Multi-bit upset (MBU) and leads to second and third-order effects. A secondorder effect happens if an SEU simultaneously strikes two adjacent sensitive nodes located in two different memory cells. And when MBU occurs as a result of a single particle striking two adjacent sensitive nodes located in the same memory cell, it is considered a third-order effect.
It is not easy to identify all the potential types of faults which can occur in an electronic circuit. To evaluate a design against faults, faults are assumed to behave according to some fault model [14]. A fault model attempts to describe the effect of the fault that can occur. With the help of the fault model, the design engineers can efficiently predict the consequences of this particular fault.
Electronic chips are becoming increasingly complex. Computation demands are increasing day by day increase because of the rise in AI workloads. These modern highperformance chips consist of billions of transistors. The physical defects can be of different types. It is very extremely difficult to investigate all possible faults. The advantages of fault models are: a) It helps analyze the circuit behavior under a given fault model. b) Drastically reduces the number of faults to be considered. c) Fault Coverage of design. d) It enables the designer to find the root cause of the failure of a design. e) With fault simulations, it helps generate the test metrics, which helps in the reliability evaluation of the design. Some of the most commonly used fault models are discussed below.
Stuck-at (SA) 0/1: The stuck-at fault model is one of the most common fault models used for the reliability analysis of a VLSI design. In this fault model, if a circuit line is permanently stuck at ’logic low’, it will be called Stuckat-0, and if it is permanently stuck at ’logic high’, it will be termed Stuck-at-
Sometimes fault model depends on the targeted application and how that application is implemented on the hardware. For instance, in [43] has proposed two fault models (Fig. 2) for the deep convolutional neural implementation on the FPGAs. a) Pixel stuck-at, which means the stuck-at fault in a single pixel of the CNN feature maps. b) Channel stuck-at, which means the whole channel in CNN feature maps is faulty.
FPGAs are becoming a valuable candidates for AI applications because of their high density, high performance, shorter time to market, and re-programmability. On the other hand, ASICs, which stand for Application-Specific Integrated Circuits, are designed for a specific application, and their functionality remains the same throughout their operating life.
In ASICs, the logic is permanently mapped to gates and flip-flops in silicon. Whereas in FPGAs, logic is mapped on the configurable logic blocks (CLBs). CLBs consist of Lookup Tables (LUTs), flip-flops (FFs), and routing resources (switch matrix, multiplexors, and connection segments). Most FPGAs also have dedicated memory blocks as hard macros called block RAMs (BRAMs). Unlike ASICs, FPGAs are programmable, and their functionality can be changed by uploading a new bitstream. The
bitstream contains configuration frames, which configure all the programmable and memory elements in the FPGA fabric. All these bits are potentially sensitive to radiation effects; therefore, the design should be thoroughly investigated against various fault models.
In [21] has investigated the effect of radiation in ASICs and FPGAs. Figure 3 has illustrated that effect of radiation in the combinational and sequential logic of ASICs is transient. Based on the pulse duration of the SETs (Glitch), transient faults in the combinational logic of the design may or may not be latched by a storage cell. On the other hand, faults in the sequential logic (SEUs) remain in the storage cell until the next load.
In the case of SRAM-based FPGAs, the user’s logic is mapped on the CLBs. CLBs consist of LUTs, FFs, and routing resources. An SEU in the LUT memory cell modifies the implemented combinational logic and results in undesired program behavior. The end effect of SEUs in the CLBs is permanent, and it can only be mitigated by re-programming the bitstream. Similarly, an SEU in the routing matrix will lead to connecting/disconnecting a connection between CLBs. This can also be mitigated using bitstream reconfiguration. An SEU in block RAMs also has a permanent effect; therefore, block RAMs should be protected against faults using different error-detecting and correcting techniques. In a scenario, when an SEU occurs in the sequential logic synthesized in the FPGA, it will have a temporary effect, as the faulty value will be overwritten in the next load of the flip flop [21].
As we have discussed, the impact of the fault is sometimes different in ASICs and FPGAs for the reason that FPGA fabric is a bit different compared to ASICs. Several fault-tolerant methodologies, which have been proposed for ASICs, may not be directly applicable to FPGAs. Therefore, it is extremely important that fault modeling and fault analysis of a design should be done based on the targeted hardware platform. A clear understanding of the targeted hardware platform can also help in the design and development of relevant fault mitigation methodologies.
tly programming to do so. Deep Learning comes under the umbrella of Machine Learning (ML), and it uses Artificial Neural Networks (ANNs), whose architecture is inspired by the structure and function of the brain. ANNs have recently become the standard tool for solving a variety of prediction and classification problems. They generally consist of an input layer, an output layer, and hidden layers. In past years, ANNs have grown in complexity, comprising of many hidden layers, and are able to solve many complex problems in computer vision, natural language processing, and medical science, etc. ANNs are also commonly known as Deep Neural Networks. The term ’Deep’ refers to the use of multiple layers in the ANNs. Each layer consists of neurons that connect to other neurons in the corresponding layers via an activation function. Each neuron has its associated parameters, i.e., weight, bias, and/or filter coefficient. Authors of [23, 40] have investigated the ANNs in detail.
The design cycle of DNNs consists of two major stages: Training and Inference.
Training: DNNs model should be trained before its deployment on the targeted device. Training is a computeintensive process, generally carried out by high-performance computing machines, i.e., cloud servers, which involves the use of a training data set to find suitable values for the network parameters. After the training is done, the performance of the model is tested against a test data set.
Inference: After the model is trained and tested, it is ready to be deployed. At this stage, the NN performs classification/ decision-making using actual, previously unseen data (i.e., in real-time). Target hardware for inference can vary based on the application. For applications such are movie recommendations on Netflix or social media Ads, inference happens on the cloud. In comparison, inference happens on edge in the case of Cyber-Physical Systems (e.g., autonomous vehicles and wearable healthcare devices).
Since their advent, NNs have progressively improved. The first generation was single-layer perceptron or multi-layer perceptron (MLP). MLP is also called a feedforward neural network for the reason that nodes in the network do not form a cycle. MLP can generally perform classification and regression problems.
The second-generation (Fig. 4) of the neural networks consists of convolutional neural networks (CNNs), RNNs, capsule networks (CapsNets) [16], and generative adversarial networks
Artificial Intelligence (AI) has created an enormous impact on all aspects of life. AI, as described in [12], “is a system’s ability to interpret external data correctly, to learn from such data, and to use those learnings to achieve specific goals and tasks through flexible adaptation.” Machine Learning is a subset of AI, which deals with computer algorithms that can train a model to perform tasks and take decisions without explici-
(GANs) [11]. CNNs have proven so effective in solving an image classification problem. CNNs have the ability to develop an internal representation of a two-dimensional image. This allows the CNN model to learn the position and scale-invariant structures in the image data, which is very important when working with images. Various CNN models have been proposed, namely, VGG16 [38], Alexnet [22], Resnet [15], etc., which have shown state-of-the-art performance against the ImageNet dataset [35].
The third generation (Fig. 4) of neural networks makes use of spiking NNs (SNNs) [29] in an attempt to emulate human brain-like functioning. The major difference between a traditional ANN and SNN is how the information propagates through the network. Therefore, instead of continuously changing in time values used in artificial neural networks, spiking NNs works with discrete events that occur at specific points of time. Spiking NNs receives a series of spikes as input and produces a series of spikes as the output, also referred to as spike trains [29]. The emphasis of this survey is on MLPs and CNNs.
and the use of batch normalization layers in the neural network architecture helps in generalizing and improving the resiliency of the network model. In [34] was figured that, that the impact of fault is more when it happens at the back of the network (i.e., in the last layers), whereas faults effects tend to be mitigated or neutralized if happening in the initial layers of the network ( i.e., the first layer). In [6, 27] was demonstrated that pruning and quantization also assist in increasing the resiliency of the network model.
We have discussed in the section 2 how the hardware is vulnerable and leads to incorrect results in the presence of faults. In the section 3, we have briefly explained the DNNs, their design cycle, and taxonomy. Eventually, the DNNs will be implemented on hardware. Therefore it is essential to analyze the neural networks under the influence of hardware faults. Deep Neural Networks are said to have some inherent resiliency. However, faults can still influence the accuracy of the DNN model, which can further lead to incorrect output classification or prediction. Therefore, for safety-critical applications, it becomes vital to do a thorough design analysis.
The term resiliency in the DNNs refers to the ability to maintain a given accuracy even in the presence of errors. There are many factors that can affect the resiliency of deep neural networks. i.e., Network Architecture, Layer Type, Data Type, the bit position of weights, pruning, quantization, etc. Authors of [6, 24] have shown that deeper networks are more resilient,
Datatype also has an enormous role to play in the resiliency of the DNN model. As shown in Fig. 5, we analyze how a fault can impact a DNN with an IEEE-754 floatingpoint 32 (FP32) data type versus a 4-bit Fixed Point (FxP) data type. According to the IEEE-754 standard, the FP32 data type consists of 8 exponent bits, 23 fraction bits 1 sign bit. On the other hand, the 4-bit FxP data type consists of 1 sign bit and 3 fraction bits. As discussed in the previous section, the DNN model consists of thousands of parameters. Consider 0.25 as one of the parameters (weight) of the DNN model (Fig. 5). If the weight value is represented as an FP32 number, a fault in the most significant exponent bit of the FP32 number can substantially change the value of the DNN’s parameter either to a very high value or to a very low value. If not masked, this fault could propagate through the DNN network and drastically decrease the accuracy. The impact of a fault also causes a deviation in FxP numbers, which leads to a decrease in accuracy, but the overall impact would be less due to the less dynamic range of the FxP numbers [39]. Therefore it is crucial to define a data type and bit-width, which can fulfill the requirement of accuracy and reliability, and hardware resources.
Another aspect that impacts the reliability of the DNN model is the hardware architecture implemented on the targeted hardware, e.g., ASICs, FPGAs. The faults can occur in the datapath, i.e., latches, flip flops, etc., and also in the data buffers. Faults in both locations propagate differently. Faults in the data path will be read once and can get over-written by the correct value in the next load. Whereas faults in the buffers may be read multiple Times because of the reuse (reuse of weights, input feature maps, output feature maps, etc.), and hence the same faulty value can be spread to multiple locations very quickly [24].
In this section, we will explore several state-of-the-art. fault-tolerant methodologies which were proposed by the corresponding research community.
Fig. 5. Impact of a fault in floating point and fixed point number [39] Rys. 5. Wpływ błędu na liczbę zmiennoprzecinkową i stałoprzecinkowąThe challenge is that running deep learning models is a resource-intensive process and deploying these models, with millions of parameters, on edge devices is a growing concern. There are DNN models from a few thousand parameters to more than a billion parameters. Deploying these big models on the hardware is very challenging, especially in safety-critical applications. Due to these challenges, research in the area of model compression has been very actively pursued over the last few years. The goal of model compression is to reduce the model size so that it can be deployed on low power and resource constraint devices without a significant accuracy drop. Some model compression methods which has been proposed in recent years are parameter pruning, quantization, knowledge distillation, low-rank factorization, transferred/compact convolutional filters, etc. In this study, we focus on the two popular two model compression methods: a) Pruning b) Quantization. Pruning and quantization were not particularly proposed as a method to improve resiliency. It is exciting to study them for two reasons.
a) Pruning and quantization affect the resiliency of the DNNs.
b) Both methods have become the de-facto standard during the DNN deployment on the hardware; therefore, it is crucial to study it from the fault tolerance perspective.
Pruning: Many experiments have concluded that there are many parameters in the DNNs which are not important, and it is still possible to achieve the desired performance in the absence of these parameters. Thus, pruning is a way to remove unnecessary parameters, thereby making the deep neural networks sparsed. Figure 6 illustrates the impact of the magnitude-based weight pruning method, which gradually zeroes out weights of the model during the training process to achieve model sparsity. This sparsity in the neural network parameters due to the pruning has two advantages. a) It reduces the mode size, which further helps in reducing the computational complexity, leading to faster inference. b) It improves the resiliency of the DNN model [6]. Pruning is further classified into channel pruning, filter pruning, connection pruning, and layer pruning. Different pruning strategies can also have a different resiliency impact on neural networks.
Quantization: A typical deep neural network consists of weights in 32-bit floating-point values. FP32 computations require either a floating-point unit or additional hardware resources to perform dynamic range shifts computation. This will lead to an increase in hardware resources and latency, which gains makes it challenging to deploy these networks in hardware devices. DNN quantization comes to the rescue in this situation. DNN quantization refers to a method of approximating a neural network’s parameters and activations to low bit-width fixed point (FxP) numbers as shown in Fig. 7. Because, in many cases, the dynamic range that the FP32 provides is not needed. FxP numbers are generally hardware-friendly. FxP computations are faster than FP32, and it also costs less area overhead as compared to FP32 Computations. Along with the benefits such as (a) lower model size (b) lower inference latency, DNN Quantization also results in improving the resiliency of the DNN Model.
Goldstein have studied the impact of SEUs in three different CNNs models with different sparsity [9]. They have concluded that Pruning and Quantization combined can increase the resiliency by up to 108.7 times. Other authors have explored further the impact of SEUs in homogenous and heterogeneous quantized models and concluded that, in general, quantization helps in improving the resiliency of the DNN model [29]. Resiliency between the models can also vary based on different levels of quantization and more vigorous quantization could sacrifice resiliency and accuracy. In [36] have aggressively quantized the DNN models (VGG16 and Lenet) to as low as the binary values and have reported an increase in the fault resiliency of DNN models (VGG16 and Lenet) by 10000x.
We have examined in section 4 Fig. 5, how a fault can impact a DNN with an FP32 data type versus a 4-bit FxP data type and illustrated that fault in the MSB of the exponent bit can saturate the DNN model and lead to undesirable results. In [18] was observed a similar impact of fault on the FP32 as illustrated in Fig. 5 and to solve the problem of saturation the technique of ’The Clipped Activations Function’ has been proposed. By default, the output of the activation function is unbounded; therefore, in the presence of a fault, the faulty output of extremely high magnitude can propagate through the network. They have replaced the unbounded activations functions with a bounded activations function to restrict the output of the activation function to a specific threshold value. With this methodology, they have achieved 68.92 % improvement in accuracy compared to the baseline VGG-16 model at 1 10−2 fault rate.
Similarly, in [2, 7] have also explored the similar phenomenon of restricting the output of neurons to make them more resilient. Restricting the output of the neurons results in reduced deviations. Neural networks have the capability to tolerate small deviations due to their inherent resiliency.
Machine Learning deals with computer algorithms that have the ability to learn to perform tasks and take decisions without explicitly programming to do so. The process of learning is called ’Training’. Authors in [43] have leveraged this idea and proposed Fault Aware Training (FAT). In other words, Fault Injection can be performed during the training phase. They treat resiliency as a learning problem, and they want the neural network to learn
Fig. 7. Quantization impact on weights distribution [29]the impact of faults during the training phase. Fault Injection layers have been added inside the DNN model (Fig. 8). Faults are injected into the neural network during the training phase with a probability in the range of 1–10 %.
There is the concept of using Dropout layers in the training of the neural networks to reduce overfitting and make the behavior of the model more generic. The fault injection layer during FAT behaves somewhat similarly to Dropout layers. FAT supports injecting many possible fault values into the DNNs, while dropout only inserts zeros during the training. The detailed analysis of two different fault models under the influence of faults with different probabilities is discussed in the paper.
Further, not all the components in the design are essential. We can focus on hardening only the essential parts of the design, in other words, only triplicating the sensitive part of the design. This method is commonly known as Selective Hardening. Selective hardening can be applied on various abstraction levels in the design. In neural networks, it can be applied in the layer-level [20, 26], neurons level (Fully Connected layers) or channel-level (CNN Layers) [5] and also at the Processing Element (PEs) level.
In [44] was considered a DNN accelerator based on a systolic array, i.e., Google Tensor Processing Unit (TPU), and proposed two fault-tolerant methodologies.
a) Fault Aware Pruning (FAP)
b) Fault Aware Pruning + Retraining (FAP+T). They have considered permanent faults, which occurred in the integrated circuits due to process variations or manufacturing defects. After detailed gate-level simulations of stuck-at faults, they have concluded that the accuracy of the TPU drops drastically even if there are just four faulty MAC units among a total of 64K MAC units.
FAP: Pruning is a method to remove connections that are not important. It was leveraged this idea and used it to prune the fault MACs causing the accuracy degradation [44]. Using standard post-fabrication analysis, they find the location of the faulty MACs, and with some additional bypass circuity, they can bypass the faulty MACs. The area overhead of the new systolic array architecture due to the new bypass path is about 9 %.
FAP+T: With FAP, it is only possible to bypass the faulty MACs. An additional re-training step is added to recover the accuracy loss because of the missing MAC units. In this step, the model learns to adapt to the change caused due to missing MAC units and tries to attain its baseline classification accuracy. Authors have claimed that, even with 50 % faulty MAC units, FAP+T can provide close to baseline accuracy. Post-fabrication analysis of every chip can be different; therefore, FAP and FAP+T need to be performed for all the chips with manufacturing defects.
Hardware redundancy-based methods (i.e., DMR and TMR) generally involve full hardware replication. Triple Modular Redundancy (TMR) has been used in the industry for many decades. Triplication of a design does provide the required resiliency to the safety-critical system but at the cost of increased power consumption and a considerable area overhead of 200 %. There are different attractive alternatives to full TMR for a wide variety of safety-critical applications. Typically, not all safety-critical applications focus on very high resiliency requirements.
Authors [5, 26, 1] have applied selective hardening techniques in the CNNs and have made a similar argument that triplicating the whole DNN model would cost a 200 % increase in the area overhead. They have proposed Selective layer and Channel Triplication, respectively, in a two-step process. a) Identification of the vulnerable CNN channels, which causes a decrease in accuracy in the presence of Faults b) triplication of the identified CNN layers/channels. They have used different network architectures of various sizes for their analysis. In [26] was performed fault injection using the FPGA accelerated fault injection and neutron flux radiation setup. Selective layer hardening resulted in the masking of 40 % faults with 8 % additional area overhead. While in the study [5], they can reduce the area overhead from 200 % to 173 % for a worst-case accuracy drop of 0.5 %. For a worst-case accuracy drop of 1 % and 2 %, they have reported a 200 % to 129.7 % and 49.87 % reduction in area overhead, respectively. This also validates the argument that for more strict accuracy requirements, more hardware area will be needed and vice versa. Hence, depending upon the application requirements, selective hardening of the design can be performed.
On hardware, neural networks are mapped to multiple processing elements. These processing elements perform multiply and accumulate operations. Based on the hardware architecture of the DNN, a single neuron, singlechannel, single layer, or multiple layers can be mapped to these processing elements. A fully parallelized architecture of a neural network in which each neuron is mapped to one PE is very unlikely for bigger networks. Therefore, in most cases, these PEs are being shared between different neurons, channels, or layers. Therefore, even one faulty PE can cause a huge accuracy degradation. In [5] was investigated that the more PEs are being shared, the higher it will lead to a decrease in accuracy in case of a fault. Therefore, it would be interesting to explore selective hardening at the PE level.
Ensemble learning methods were initially proposed to reduce overfitting or better generalize the results compared to the results from a single model instance. Multiple ML models are trained on the same dataset during the training phase. The output of each model is processed to estimate the best outcome during the inference phase. One way of selecting the best outcome is to take an average of predictions of individual models. In [33] was used an ensemble learning-based approach to incre-
ase the robustness of the CNN model. The larger the number of CNN models in an ensemble, the greater will be the hardware resource utilization and power consumption. The author has used quantization and pruning to compress the model 1/N times its initial size. The hardware resources they save during the model compression are utilized to create an ensemble of N CNN models (Fig. 9). In contrast to traditional ensemble methods, their proposed approach of compressed ensembles can be deployed on constrained devices with no energy or memory overhead. The output of the CNN ensemble is averaged to compute the final predictions. Their target hardware is an edge device comprising of processor connected to main memory via a 32-bit bus. They have considered faults in memory that happen due to sub-nominal operating conditions.
In [25] was developed an approach that uses knowledge distillation-based redundancy to detect faults. Knowledge distillation, proposed in [17], is a training-based solution for reducing the model’s size, in which the knowledge from the teacher model is transferred to a simpler student model. In this way, a smaller student model can approximate the results of the bigger teacher model. The teacher model is generally a complex ML/DL model or an ensemble of models. In contrast, a student model is usually a single smaller model that is much more straightforward to deploy without substantial loss in performance. Their approach makes use of two DNN models, i.e., task DNN (teacher model) and checker DNN (student model). Instead of using an expensive DMRbased solution, have reduced the size of the checker DNN model by utilizing knowledge distillation and architecture compression approach [25]. Both of these models process each input sample (see Fig. 10). A comparator block compares the
outcome of both models. If the results are consistent, they are considered for further processing; otherwise, re-computation on the task DNN is performer for potential recovery from the fault. They have performer the experiments from the security perspective and considered the fault model in which the attacker is trying to compromise the accuracy of a DNN system by maliciously injecting faults. Experimental results show that at the cost of 10 % overhead, their approach can reduce 90 % of the risks. This approach can be considered and studied from the reliability perspective as well.
Matrix multiplications are the fundamental arithmetic operation in neural networks. In order to make this matrix multiplications fault-tolerant, Algorithm-based fault tolerance (ABFT) was proposed in [19]. ABFT cannot only detect the errors but also can correct the errors. ABFT is a very attractive solution to make the neural network fault-tolerant, and it costs low area overhead as compared to traditional TMR methods. The core idea behind ABFT can be thought of as an extension of ECC to numeric structures like vectors and matrices. In [45, 37] was applied the ABFT approach to CNN models. Zhao et al. (2020) have considered some of the widely used CNN models, i.e., AlexNet, VGG-19, ResNet-18, and YOLOv2, and demonstrated the results as per runtime overhead metric. Their ABFT approach can handle soft errors with a very small runtime overhead of 4 % to 8 %. In [37] was implemented CNNs on three GPU architectures, i.e., K40, Tegra X1, Titan X. Their ABFT approach is able to detect and correct 50 % to 60 % of radiation-induced corruptions.
Similar to ABFT, was proposed a lowoverhead error detection technique for matrix multiplications [28]. I.e., Light ABFT.
Unlike ABFT, which can perform error detection and correction, the light ABFT approach can only detect errors. Author have targeted FPGAs, and he argues that as soon as the error is detected with Light ABFT, it can be corrected using fast partial reconfiguration of the FPGA bitstream.
Authors of [31] have made use of the linearity property of the fully connected layers and convolutions layers and have proposed a low overheard error detection method, called as Sanity-Check. The ABFT also inspires this approach, and in this approach, with the addition of two neurons (namely sanity-neuron and check-neuron), they can detect whether the layer’s output is erroneous. The sanity-neuron acts as an additive inverse of the rest of the neuron in the layer, while the check-neuron sums the output to confirm if the result is zero or non-zero. A similar approach is applied in the case of convolution layers.
Arithmetic error codes, which comes under the umbrella of ECCs, are an exciting way to detect and correct errors, as they are conserved during most arithmetic operation [32]. They have been used in various safety-critical applications to increase the reliability of the systems. In [10] authors have used a specific class of arithmetic codes, known as AN-Codes, in state-of-the-art DNN accelerators. They have exhibited that they can achieve 99 % fault coverage with a 5-bit arithmetic code with minimal area and power overhead.
In [4] was proposed a very different and unique approach to detect errors in CNNs. The general functionality of CNNs is that it takes an image as input and output the predictions. Each image is a frame, and many frames are captured and processed in one second. CNNs treat each frame independently and predict the output. Most of the time, these input frames are correlated, and hence the output predictions are also similar. Therefore, not only do the input frames correlate with each other but also the output predictions. They use both the input and output correlation information to detect errors in a frame as it is processed. If there is a difference in the correlation of output predictions, then there are two possibilities. a) The input frame is also different. In this case, the change in the output predictions is justified by the change in frames. b) Erroneous output prediction. Subsequent frames are identical, and hence the output predictions should also be identical. They have performed error analysis on two CNNS, i.e., YOLO and Faster R-CNN trained on Caltech Pedestrian Dataset [3]. They are able to detect 80 % of errors while keeping the area overhead low.
AI”. The insights obtained from these studies will help design efficient faulttolerant AI accelerators.
This work was supported by the European Regional Development Fund within the BB-PL INTERREG V A 2014-2020 Program, “reducing barriers using the common strengths”, project SpaceRegion, grant number 85038043.
1. Adam K., Izeldin I.M., Ibrahim Y., A selective mitigation technique of soft errors for DNN models used in healthcare applications: DenseNet201 case study. „IEEE Access”, Vol. 9, 2021, 65803–65823. DOI: 10.1109/ACCESS.2021.3076716.
2. Chen Z., Li G., Pattabiraman K., A low-cost fault corrector for deep neural networks through range restriction. [In:] 51st Annual IEEE/IFIP International Conference on Dependable Systems and Networks (DSN), 2021, 1–13, DOI: 10.1109/DSN48987.2021.00018.
3. Dollar P., Wojek C., Schiele B., Perona P., Pedestrian detection: An evaluation of the state of the art. „IEEE Transactions on Pattern Analysis and Machine Intelligence”, Vol. 34, No. 4, 2012, 743–761, DOI: 10.1109/TPAMI.2011.155.
4. Draghetti L.K., Santos F.F.D., Carro L., Rech P., Detecting Errors in Convolutional Neural Networks Using Inter Frame Spatio-Temporal Correlation. IEEE 25th International Symposium on On-Line Testing and Robust System Design, IOLTS 2019, 310–315, DOI: 10.1109/IOLTS.2019.8854431.
5. Gambardella G., Kappauf J., Blott M., Doehring C., Kumm M., Zipf P., Vissers K., Efficient error-tolerant quantized neural network accelerators. IEEE International Symposium on Defect and Fault Tolerance in VLSI and Nanotechnology Systems, DFT 2019. DOI: 10.1109/DFT.2019.8875314.
6. Gao Z., Wei X., Zhang H., Li W., Ge G., Wang Y., Reviriego P., Reliability evaluation of pruned neural networks against errors on parameters. IEEE International Symposium on Defect and Fault Tolerance in VLSI and Nanotechnology Systems, DFT 2020, DOI: 10.1109/DFT50435.2020.9250812.
7. Ghavami B., Sadati M., Fang Z., Shannon L., FitAct: Error resilient deep neural networks via fine-grained post-trainable activation functions. Design, Automation Test in Europe Conference Exhibition (DATE ‘22), 1239–1244, DOI: 10.23919/DATE54114.2022.9774635.
8. Gill B.S. Design and Analysis Methodologies to Reduce Soft Errors in Nanometer VLSI Circuits. Ph.D. thesis, 2006, Department of Electrical Engineering and Computer Science CASE WESTERN RESERVE UNIVERSITY.
Safety-critical applications require fault-free execution of critical tasks. The increased use of DNNs in safety-critical applications demands a thorough understanding of targeted hardware (ASICs, FPGAs) and DNNs’ characteristics. Thus, this survey paper has discussed and differentiated between ASICs and FPGA fault models. The essential concept of three generations of neural networks is explained. We extended this discussion and examined factors that impact the resiliency of neural networks and several state-of-the-art fault mitigation methodologies.
Improving the reliability of the DL accelerators is like ”chasing a moving target”. The design of an efficient faulttolerant AI accelerator will involve the combined effort of researchers in both the AI and reliability domains. Previously, AI models have been treated as black boxes. Now, the increased use of AI in safety-critical applications, i.e., Medical, Automotive, Industries, Defense, Space, etc., has led researchers to work on ”Explainable
9. Goldstein B.F., Reliability evaluation of compressed deep learning models. 2020 IEEE 11th Latin American Symposium on Circuits Systems (LASCAS), 1–5, DOI:10.1109/LASCAS45839.2020.9069026.
10. Goldstein B.F., Ferreira V.C., Srinivasan S., Das D., Nery A.S., Kundu S., Franca F.M.G., A lightweight error-resiliency mechanism for deep neural networks. 22nd International Symposium on Quality Electronic Design (ISQED), 2021, 311–316. DOI:10.1109/ISQED51717.2021.9424287.
11. Goodfellow I., Pouget-Abadie J., Mirza M., Xu B., Warde-Farley D., Ozair S., Courville A., Bengio Y., Generative adversarial nets. (Z. Ghahramani, M. Welling, C. Cortes, N. Lawrence, and K.Q. Weinberger eds.), „Advances in Neural Information Processing Systems”, Vol. 27, 2014, 2672–2680, DOI: 10.5555/2969033.2969125.
12. Haenlein M., Kaplan A., A brief history of artificial intelligence: On the past, present, and future of artificial intelligence. „California Management Review”, Vol. 61, No. 4, 2019, 5–14. DOI: 10.1177/0008125619864925.
13. Hass K.J., Probabilistic estimates of upset caused by single event transients, 1999.
14. Hayes J., Fault modeling for digital mos integrated circuits „IEEE Transactions on Computer-Aided Design of Integrated Circuits and Systems”, Vol. 3, No. 3, 1984, 200–208, DOI: 10.1109/TCAD.1984.1270076.
15. He K., Zhang X., Ren S., Sun J., Deep residual learning for image recognition. 2016 IEEE Conference on Computer Vision and Pattern Recognition (CVPR), 770–778, DOI: 10.1109/CVPR.2016.90.
16. Hinton G.E., Krizhevsky A., Wang S.D., Transforming auto-encoders. [In:] T. Honkela, W. Duch, M. Girolami, S. Kaski, (eds.), Artificial Neural Networks and Machine Learning –ICANN 2011, 44–51. DOI: 10.1007/978-3-642-21735-7_6.
17. Hinton G.E., Vinyals O., Dean J. Distilling the knowledge in a neural network. ArXiv, 2015, DOI: 10.48550/arXiv.1503.02531.
18. Hoang L.H., Hanif M.A., Shafique M., FT-ClipAct: Resilience analysis of deep neural networks and improving their fault tolerance using clipped activation. 2020 Design, Automation Test in Europe Conference Exhibition (DATE), 1241–1246. DOI:10.23919/DATE48585.2020.9116571.
19. Huang K.H., Abraham, J.A. (1984). Algorithm-based fault tolerance for matrix operations. IEEE Transactions on Computers, C-33(6), 518–528. DOI:10.1109/TC.1984.1676475.
20. Ibrahim Y., Wang H., Bai M., Liu Z., Wang J., Yang Z., Chen Z., Soft Error Resilience of Deep Residual Networks for Object Recognition. „IEEE Access”, Vol. 8, 2020, 19490–19503, DOI: 10.1109/ACCESS.2020.2968129.
21. Kastensmidt F.L., Carro L., Reis R., Fault-tolerance techniques for SRAM-based FPGAs, 2006, Springer, DOI: 10.1007/978-0-387-31069-5.
22. Krizhevsky A., Sutskever I., Hinton G.E., Imagenet classification with deep convolutional neural networks. [In:] F. Pereira, C.J.C. Burges, L. Bottou, and K.Q. Weinberger (eds.), Advances in Neural Information Processing Systems, Vol. 25, 2012, Curran Associates, Inc.
23. LeCun Y., Bengio Y., Hinton G., Deep learning. „Nature”, Vol. 521(7553), 2015, 436–444. DOI: 10.1038/nature14539.
24. Li G., Hari S.K.S., Sullivan M., Tsai T., Pattabiraman K., Emer J., Keckler S.W., Understanding error propagation in deep learning neural network (DNN) accelerators and applications. [In:] Proceedings of the International Conference for High Performance Computing, Networking, Storage and Analysis, 2017, 1–12, DOI: 10.1145/3126908.3126964.
25. Li Y., Li M., Luo B., Tian Y., Xu Q., DeepDyve: Dynamic verification for deep neural networks. [In:] Proceedings of the 2020 ACM SIGSAC Conference on Computer and Communications Security, CCS ’20, 101–112. Association for Computing Machinery, New York, NY, USA, DOI: 10.1145/3372297.3423338.
26. Libano F., Wilson B., Anderson J., Wirthlin M.J., Cazzaniga C., Frost C., Rech P., Selective hardening for neural networks in FPGAs. „IEEE Transactions on Nuclear Science”, Vol. 66, No. 1, 2019, 216–222. DOI: 10.1109/TNS.2018.2884460.
27. Libano F., Wilson B., Wirthlin M., Rech P., Brunhaver J., Understanding the impact of quantization, accuracy, and radiation on the reliability of convolutional neural networks on FPGAs. „IEEE Transactions on Nuclear Science”, Vol. 67, No. 7, 2020, 1478–1484, DOI: 10.1109/TNS.2020.2983662.
28. Libano F., Analyzing and Improving the Reliability of Matrix Multiplication and Neural Networks on FPGAs. Ph.D. thesis, 2021, Arizona State University.
29. Lyashenko V., Basic guide to spiking neural networks for deep learning, 2020, https://cnvrg.io/spiking-neural-networks/.
30. Mittal S., Vetter J.S., A survey of techniques for modeling and improving reliability of computing systems. „IEEE
Transactions on Parallel and Distributed Systems”, Vol. 27, No. 4, 2016, 1226–1238. DOI: 10.1109/TPDS.2015.2426179.
31. Ozen E., Orailoglu A., Sanity-check: Boosting the reliability of safety-critical deep neural network applications. In 2019 IEEE 28th Asian Test Symposium (ATS), 7–75. DOI: 10.1109/ATS47505.2019.000-8.
32. Parhami, Avizienis, Detection of storage errors in mass memories using low-cost arithmetic error codes. „IEEE Transactions on Computers”, Vol. C-27, No. 4, 1978, 302–308. DOI: 10.1109/TC.1978.1675102.
33. Ponzina F., Peón-Quirós M., Burg A., Atienza D., E2CNNs: Ensembles of convolutional neural networks to improve robustness against memory, errors in edge-computing devices. „IEEE Transactions on Computers”, Vol. 70, No. 8, 2021, 1199–1212. DOI: 10.1109/TC.2021.3061086.
34. Ribes S., Malek A., Trancoso P., Sourdis I., Reliability analysis of compressed CNNs. 2021.
35. Russakovsky O., Deng J., Su H., Krause J., Satheesh S., Ma S., Huang Z., Karpathy A., Khosla A., Bernstein M., Berg A.C., Fei-Fei, L., ImageNet Large Scale Visual Recognition Challenge. „International Journal of Computer Vision”, Vol. 115, No. 3, 2015, 211–252, DOI: 10.1007/s11263-015-0816-y.
36. Sabbagh M., Evaluating fault resiliency of compressed deep neural networks. 2019 IEEE International Conference on Embedded Software and Systems (ICESS), 2019, 1–7. DOI:10.1109/ICESS.2019.8782505.
37. Santos F.F.D., Evaluation and Mitigation of Soft-Errors in Neural Network-Based Object Detection in Three GPU Architectures. 47th Annual IEEE/IFIP International Conference on Dependable Systems and Networks Workshops, DSN-W 2017, 169–176. DOI:10.1109/DSN-W.2017.47.
38. Simonyan K., Zisserman A., Very deep convolutional networks for large-scale image recognition, 2015, DOI: 10.48550/arXiv.1409.1556.
39. Syed R.T., Ulbricht M., Piotrowski K., Krstic, M., Fault resilience analysis of quantized deep neural networks. IEEE 32nd International Conference on Microelectronics (MIEL), 2021, 275–279. DOI: 10.1109/MIEL52794.2021.9569094.
40. Sze V., Chen Y.H., Yang T.J., Emer J.S., Efficient processing of deep neural networks: A tutorial and survey. Proceedings of the IEEE, Vol. 105, No. 12, 2017, 2295–2329, DOI: .1109/JPROC.2017.2761740.
41. Werner S., Navaridas J., Luján M., A survey on design approaches to circumvent permanent faults in networkson-chip. „ACM Computing Surveys”, Vol. 48, No. 4, 2016, DOI:10.1145/2886781.
42. Yi C.H., Kwon K.H., Jeon J., Method of improved hardware redundancy for automotive system. 14th International Symposium on Communications and Information Technologies (ISCIT), 2014, 204–207, DOI: 10.1109/ISCIT.2014.7011901.
43. Zahid U., Gambardella G., Fraser N.J., Blott M., Vissers K., FAT: Training neural networks for reliable inference under hardware faults. 2020 IEEE International Test Conference (ITC), 2020, 1–10. DOI: 10.1109/ITC44778.2020.9325249.
44. Zhang J., Gu T., Basu K., Garg S., Analyzing and mitigating the impact of permanent faults on a systolic array based neural network accelerator. Proceedings of the IEEE VLSI Test Symposium, 2018, 1–6, DOI: 10.1109/VTS.2018.8368656.
45. Zhao K., Di S., Li S., Liang X., Zhai Y., Chen J., Ouyang K., Cappello F., Chen Z., Algorithm-Based Fault Tolerance for Convolutional Neural Networks. 2020, 1–13, DOI: 10.1109/TPDS.2020.3043449.
Streszczenie: Znaczący rozwój sztucznej inteligencji (SI) wpływa na wiele otaczających nas aplikacji, do tego stopnia, że SI jest obecnie coraz częściej wykorzystywana w aplikacjach okrytycznym znaczeniu dla bezpieczeństwa. Sztuczna inteligencja na brzegu sieci (Edge) jest rzeczywistością, co oznacza wykonywanie obliczeń na danych bliżej źródła danych, w przeciwieństwie do wykonywania ich wchmurze. Aplikacje o krytycznym znaczeniu dla bezpieczeństwa mają wysokie wymagania dotyczące niezawodności; dlatego ważne jest, aby modele SI działające na brzegu sieci (tj. sprzęt) spełniały wymagane standardy bezpieczeństwa. Z rozległej dziedziny sztucznej inteligencji, głębokie sieci neuronowe (DNN) są centralnym punktem tego badania, ponieważ nadal przynoszą znakomite wyniki wróżnych zastosowaniach, tj. medycznych, motoryzacyjnych, lotniczych, obronnych itp. Tradycyjne techniki niezawodności implementacji w przypadku DNN nie zawsze są praktyczne, ponieważ nie wykorzystują unikalnych cech DNN. Co więcej, istotne jest również zrozumienie docelowego sprzętu brzegowego, ponieważ wpływ usterek może być różny w układach ASIC i FPGA. Dlatego też wniniejszym przeglądzie najpierw zbadaliśmy wpływ usterek w układach ASIC i FPGA, a następnie staramy się zapewnić wgląd w ostatnie postępy poczynione w kierunku DNN odpornych na błędy. Omówiliśmy kilka czynników, które mogą wpływać na niezawodność sieci DNN. Ponadto rozszerzyliśmy tę dyskusję, aby rzucić światło na wiele najnowocześniejszych technik ograniczania błędów w sieciach DNN.
Rizwan Tariq Syed, MSc, Eng.
ORCID: 0000-0001-9232-734X
Markus Ulbricht, PhD, Eng.
ORCID: ORCID: 0000-0001-9230-640X
Krzysztof Piotrowski, PhD, Eng.
ORCID: 0000-0002-7231-6704
Prof. Milos Krstic, PhD, Eng.
ORCID: 0000-0003-0267-0203
Streszczenie: W pracy przedstawiono adaptacyjny algorytm sterowania strumieniem powietrza do zastosowania w bioreaktorze do kompostowania odpadów. Układ steruje strumieniami powietrza dostarczanego do sześciu kanałów bioreaktora. Bioreaktory tego typu charakteryzują się tym, że porowatość wsadu jest bardzo niejednorodna w całej objętości. Ponadto podczas trwania procesu następuje zagęszczanie się wsadu i niejednokrotnie powstają zatory dla przepływu gazu. Algorytm sterowania powinien uwzględniać powstawanie zatorów i podejmować próbę ich udrożnienia. W sytuacjach, w których udrożnienie jest niemożliwe, powinien zapewniać prawidłowy rozkład przepływu powietrza w pozostałych kanałach mimo występowania zatorów. Nadrzędnym celem algorytmu jest zapewnienie równych strumieni przepływu powietrza we wszystkich kanałach bez względu na różnice w oporach przepływu. Ponieważ w bioreaktorach obieg powietrza jest wymuszony za pomocą wentylatorów o stałej wydajności, algorytm powinien w sposób adaptacyjny określić maksymalną średnią wartość strumieni powietrza jaka jest możliwa do uzyskania w danych warunkach i tak dopasować układ regulacji, aby ją osiągnąć. W pracy zaproponowano rozwiązanie umożliwiające spełnienie tych wymagań.
Znaczna ilość odpadów w krajach rozwiniętych stanowią odpady organiczne z gospodarstw domowych. Są one bardzo zróżnicowane pod względem rodzaju oraz składu frakcyjnego oraz składu fizyko-chemicznego. Dużą część stanowią odpady biodegradowalne. Chcąc redukować ilość składowanych odpadów sortuje się je na kilku poziomach za pomocą selektywnej zbiórki odpadów, z których znaczna część jest poddawana recyklingowi. Mimo to odpady biodegradowalne stanowią znaczącą objętość w całości odpadów z gospodarstw domowych. Składowanie tego rodzaju odpadów wymaga posiadania składowisk o znacznych objętościach, z tego względu celowe jest poszukiwanie metod umożliwiających zmniejszenie objętości składowanych odpadów biodegradowalnych.
Kompostowanie odpadów jest metodą mająca na celu rozkład substancji organicznych poprzez zastosowanie procesów
tlenowych. Powoduje to zmniejszenie objętości cząstek biodegradowalnych oraz redukcję zawartości wody, potencjału górotwórczego i aktywności oddechowej odpadów. Kompostowanie odbywa się przy udziale licznych grup mikroorganizmów [1], którym należy zapewnić odpowiednie warunki takie jak ilość tlenu, wilgotność i temperaturę.
Proces kompostowania odbywa się w specjalnie zaprojektowanych bioreaktorach (rys. 1) z kontrolowanym systemem napowietrzania nawilżania i sterowania temperaturą [2]. Bioreaktor składa się z komory, w której dnie znajdują się kanały doprowadzające powietrze. Powietrze to natlenia wsad znajdujący się w komorze. W górnej części komory znajdują się kanały odprowadzające powietrze, które jest zawracane w obiegu zamkniętym. Jednocześnie na bieżąco jest kontrolowana zawartość tlenu w powietrzu obiegowym. Gdy zawartość tlenu maleje poniżej zadanej wartości, następuje częściowa wymiana powietrza. W przypadku nadmiernego wzrostu temperatury uruchamiany jest układ chłodzenia. Podobnie w przypadku obniżenia się wilgotności, wsad jest zraszany wodą.
Aby proces przebiegał prawidłowo w całej objętości bardzo istotne jest utrzymanie równomiernego przepływu powietrza we wszystkich kanałach zasilających umieszczonych w dnie komory. Ponieważ porowatość wsadu umieszczonego w komorze nie jest jednorodna co wymaga sterowania strumieniem powietrza dostarczanego do poszczególnych kanałów. Ponadto podczas trwania procesu następuje sukcesywne zagęszczanie wsadu. Powoduje to, że nie jest możliwe utrzymanie przepływu powietrza na stałym poziomie, lecz algorytm regulacji musi określić maksymalną średnią wartość strumieni powie-
trza, jaka jest możliwa do uzyskania w danych warunkach i tak dopasować układ regulacji, aby ją osiągnąć.
Wydajność wentylatora wymuszającego cyrkulację powietrza w bioreaktorze jest stała, zatem w celu zapewnienia równomiernego strumienia przepływu gazu we wszystkich kanałach doprowadzających powietrze, konieczne jest uwzględnienie zróżnicowanych oporów przepływu oraz możliwość powstania miejscowych zatorów. W przypadku wykrycia zatorów układ regulacji powinien podjąć próbę udrożnienia takiego zatoru, a gdy okaże się to nieskuteczne to pominąć ten kanał w procesie wyznaczania maksymalnej średniej wartości strumienia powietrza, jaka jest do uzyskania w danych warunkach dla drożnych kanałów. Aby spełnić powyższe założenia opracowano adaptacyjny algorytm sterowania [3]. Zaproponowany algorytm, który opisano w tej pracy.
Strumień powietrza jest zależny od kąta obrotu przepustnicy. Charakterystyka przepływu typowej przepustnicy jest nieliniowa (rys. 3) [5]. Stosunek Q/Qm oznacza proporcje aktualnego strumienia, jaki przepływa przez przepustnice do maksymalnego strumienia, jaki jest możliwy do uzyskania. Na potrzeby układu regulacji po uwzględnieniu zależności (1), układ przepustnica wraz z siłownikiem może być opisany funkcją czasu dla obiektu inercyjnego wyższego rzędu.
Kluczowym elementem mającym wpływ na równomierne napowietrzenie wsadu bioreaktora jest kolektor rozdzielczy doprowadzający powietrze do kanałów w dnie bioreaktora (rys. 2). W modelowym rozwiązaniu założono, że bioreaktor jest zasilany powietrzem za pomocą sześciu kanałów. Liczba kanałów jest uzależniona od konstrukcji komory bioreaktora, zwykle wynosi od 4 do 8. Przyjęta liczba kanałów jest wartością często stosowaną w praktyce [4]. Każdy z kanałów wyposażono w niezależną przepustnicę sterowana siłownikiem elektrycznym P1 –P6. Przed przepustnicą zamontowano kalorymetryczny czujniki przepływu gazu Q1 –Q6, które służą do pomiaru wartości mierzonego strumienia w poszczególnych kanałach.
Siłownik obrotowy stanowi obiekt całkujący, dla którego kąt obrotu opisuje liniowa funkcja zależna od czasu i prędkości obrotowej siłownika
= t (2)
Odczytując zmiany wartości kąta , jaki odpowiada min i max oraz uwzględniając zależność (2) określa się wartości czasu inercji Ti i opóźnienia T o
Przepustnice są sterowane za pomocą siłownika obrotowego o kącie obrotu 0–90°. Sterowanie siłownikiem odbywa się za pomocą sygnału elektrycznego umożliwiającego zamykanie/otwieranie lub zatrzymanie w obecnej pozycji. Kąt obrotu siłownika jest opisany liniową funkcją czasu:
90
m tt T 1 (1)
gdzie: T m – czas potrzebny do obrotu siłownika o 90°.
Kalorymetryczne czujniki przepływu powietrza również wprowadzają inercję i opóźnienie do układu regulacji. Poszczególne czasy oznaczono symbolami T co i T c
Każda przepustnica pracuje w układzie zamkniętym z regulatorem krokowym i odpowiednim czujnikiem przepływu. Na podstawie wartości czasów opóźnień oraz czasów inercji przepustnicy z siłownikiem oraz czujnika przepływu określa się czas pojedynczego kroku dla regulatora krokowego. Połączenie czujnika przepływu i przepustnicy w przybliżenie można traktować
Rys. 1. Bioreaktor do kompostowania odpadów Fig. 1. Waste composting bioreactor Rys. 2. Kolektor rozdzielający strumień powietrza Fig. 2. Collector separating the air stream Rys. 3. Charakterystyka przepływu przepustnicy Fig. 3. Characteristics of the throttle flowjako połączenie szeregowe dwóch obiektów inercyjnych z opóźnieniem o transmitancjach opisanych funkcjami:
k Gse sT (5)
1 o p sT p i
trza na podstawie wskazań z czujników przepływu. Wartość ta stanowi podstawę do podjęcia jakichkolwiek działań związanych z regulacja strumienia powietrza. Celem regulatora adaptacyjnego jest takie ustawienie przepustnic, aby uzyskać równomierny przepływ powietrza we wszystkich kanałach przy maksymalnej możliwej wartości średniej strumienia Qsr
6 1 1 6 sr i i QQ (10)
1 sTco c c c
k Gse sT (6)
gdzie: G p(s) – transmitancja przepustnicy, G c(s) – transmitancja czujnika przepływu.
Operatorowa funkcja przejścia dla obiektu regulacji po uwzględnieniu równań (5) i (6) przyjmuje postać:
k k GsGsGse sTsT (7)
11 sTToco p c pc ic
Funkcja przejścia stanowi podstawę do określenia parametrów regulatora krokowego zastosowanego w każdym z kanałów dostarczających powietrze do bioreaktora [6]. Minimalny czas pojedynczego kroku regulacji Tk (rys. 5), powinien być większy od sumy czasów inercji i opóźnienia.
Tk > Ti + T c + T o + T co (8)
Regulacja nastaw przepustnic odbywa się dla wszystkich kanałów ze stałym zadanym krokiem czasowym Tk i zadanym czasem działania przepustnic T a. Jeśli dla któregoś z kanałów wartość strumienia powietrza jest różna od średniej Qsr, to włącza się sygnał otwierania lub zamykania tych kanałów, w których odchyłka jest większa od wartości średniej. Gdy we wszystkich kanałach wartość strumienia mieści się w granicach odchyłki regulacji i żadna z przepustnic nie jest maksymalnie otwarta, regulator adaptacyjny w kolejnym kroku rozpoczyna się otwieranie wszystkich przepustnic celem zwiększenia średniego strumienia przepływu powietrza we wszystkich kanałach. W ten sposób dąży do uzyskania maksymalnej wartości średniej strumienia we wszystkich kanałach.
Rys. 5. Charakterystyka regulacji
Fig. 5. Regulation characteristics
Czas działania przepustnicy T a powinien być krótszy od czasu opóźnienia przepustnicy T co jednak ma on bezpośredni wpływ na wartość odchyłki regulacji być . Odchyłka ta zależy od prędkości obrotowej siłownika oraz maksymalnego strumienia powierza, jaki jest możliwy do uzyskania w danych warunkach. Zatem czas działania przepustnicy należy dobrać w taki sposób, aby uzyskać zadaną odchyłkę regulacji
maxmin a Q T (9)
max
Adaptacyjny algorytm przedstawiono na rysunku 6. Proces regulacji po uruchomieniu bioreaktora rozpoczyna się od ustawienia wszystkich przepustnic na całkowicie zamknięte. Następnie algorytm wylicza średnią wartość strumienia powie-
Rys. 6. Adaptacyjny algorytm regulacjiW sytuacji, gdy w którymś z kanałów powstaje zator i wartość strumienia powietrza jest poniżej zadanego minimum algorytm podejmuje próbę udrożnienia kanału. Polega to na całkowitym zamknięciu wszystkich pozostałych kanałów i odczekania zadanego czasu T z. Gdy po czasie T z w dalszym ciągu nie nastąpił wzrost strumienia powietrza w wybranym kanale powyżej Qmin pozostaje on całkowicie otwarty oraz oznaczony jako niedrożny Ni = 1. Wprowadzenie tabeli niedrożnych kanałów N ma na celu wyłączenie niedrożnych kanałów z dalszego procesu sterowania, aż do czasu jego udrożnienia. Ustawienie wartości Ni = 1 powoduje, że odczyt strumienia powietrza z tego kanału jest pomijany w obliczaniu kolejnych średnich wartości strumienia powietrza Qsr. Jeśli po pewnym czasie nastąpi udrożnienie kanału ponownie jest on włączony do układu regulacji.
cały czas trwania procesu kompostowania, który wynosił do 400 godzin. Czas stabilizowania się strumieni powietrza wynosił około 1 godziny, co w przypadku tak długich procesów jest czasem zadowalającym.
1. Manczarski P., Kompostowanie odpadów komunalnych, Referat na Forum Technologii Ochrony Środowiska, POLEKO 2007, Poznań, 21.11.2007
2. Anders D., Rząsa M.R., The possibility of composting animal waste products, “Environment Protection Engineering”, Vol. 33. No. 2, 2007, 7–15.
3. Dębowski A., Automatyka. Podstawy Teorii; PWN, Warszawa 2012.
4. Bidlingmaier W., Biological Waste Treatment Unit 5 Composting Techniques; A manuscript for students, Weimar 2016.
Przedstawiony algorytm został zaimplementowany w bioreaktorze do kompostowania odpadów i w zakresie 5 % odchyłki regulacji strumienia powietrza umożliwiał utrzymanie równomiernego przepływu w sześciokanałowej instalacji przez
5. Łudzień R., Wnęk M., Gil S., Problemy pomiarowe charakterystyki przepływowej przepustnicy motylkowe; „Instal”, Nr 9, 2022, 33–36, DOI: 10.36119/15.2022.9.3.
6. Dębowski A., Automatyka. Technika regulacji; PWN, Warszawa 2022.
Abstract: The paper presents an adaptive air stream control algorithm for use in a bioreactor for waste composting. The system controls the streams of air supplied to the six ducts of the bireactor. Bioreactors of this type are characterized by the fact that the porosity of the charge is very heterogeneous throughout the volume. In addition, during the process, the charge is compacted and blockages for the gas flow often occur. The control algorithm should take into account the formation of blockages and attempt to clear them. In situations where unblocking is impossible, it should ensure proper distribution of air flow in other ducts despite the presence of blockages. The overriding goal of this algorithm is to ensure equal air flow streams in all channels, regardless of differences in flow resistance. Since the air circulation in bioreactors is forced by fans with constant capacity, the algorithm should adaptively determine the maximum average value of air streams that is possible to obtain under given conditions and adjust the control system to achieve it. The paper proposes a solution to meet these requirements.
Keywords
ORCID: 0000-0002-3461-2131
Kwartalnik naukowotechniczny Pomiary
Automatyka Robotyka jest indeksowany w bazach
BAZTECH, Google Scholar
oraz INDEX COPERNICUS
w bazie naukowych
Wskazówki dla Autorów
Pomiary Automatyka Robotyka
naukowo-technicznym
Pomiary Automatyka
Robotyka.
Punktacja Ministerstwa
naukowe w kwartalniku
Pomiary Automatyka
Robotyka wynosi obecnie
naukowych i recenzowanych
naukowe – automatyka, elektrotechnika i elektronika.
Nie drukujemy komunikatów! realizacji idei Otwartej Nauki, Pomiary Automatyka Robotyka 1. wymieniowego Autora 2. jej powstanie Redakcja kwartalnika Pomiary Automatyka Robotyka 3.International Conference onDiagnostics of Processes and Systems, DPS 2022
Systems,
Control Engineering Practice Sensors
Archives of Control Sciences
International Journal of Applied Mathematics and Computer Science Polish Maritime Research Journal of Automation, Mobile Robotics and Intelligent Systems Pomiary Automatyka Robotyka Diagnostyka, Robotyka, Informatyka, Automatyka, Pomiary w Gospodarce Automation