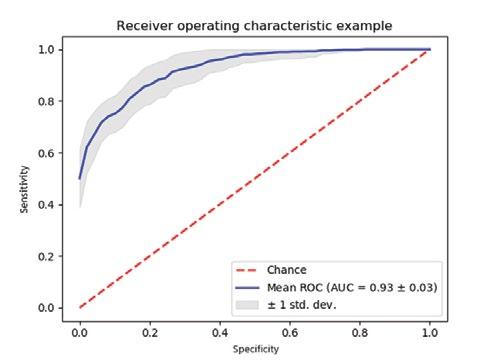
12 minute read
u Topological descriptor selection for a quantitative structure-activity relationship (QSAR) model to assess PAH mutagenicity
Topological descriptor selection for a quantitative structure-activity relationship (QSAR) model to assess PAH mutagenicity
Caitlin Sextona, Trevor Sleight, P.E.a,b, Carla Ng, Ph.D.b , Leanne Gilbertson, Ph.D.a
aGilbertson Lab Group, bNg Lab Group, Department of Civil and Environmental Engineering
Caitlin Sexton Caitlin Sexton is a junior chemical engineering student originally from Allentown, PA. Her interests include the various aspects of sustainability in application to the chemical industry. She aims to use her research experience in environmental hazards and data analysis as a guide in her post-grad career.
Trevor Sleight Trevor Sleight is a 3rd year Ph.D. student, co-advised by Dr. Ng and Dr. Gilbertson. His research interest include environmental health, data analysis and biodegradation.
Carla Ng, Ph.D. Carla Ng is an assistant professor of Civil & Environmental Engineering. Her group focuses on understanding and predicting the biological impacts of chemicals in the environment.
Leanne Gilbertson, Ph.D. Dr. Gilbertson is an Assistant Professor in the Department of Civil and Environmental Engineering at the University of Pittsburgh. Her research group at the University of Pittsburgh is currently engaged in projects aimed at informing sustainable design of emerging materials and technologies proposed for use in areas at the nexus of the environment and public health.
Significance Statement
This study aims to assess the mutagenicity, and therefore environmental hazard, of PAHs in soil using a computational QSAR model. PAH mutagenicity is difficult to assess due to the variety of metabolites which can result from many possible biodegradation pathways. Descriptors discussed in this study will be the basis of the QSAR model.
Category: Computational Research Abstract
Polycyclic aromatic hydrocarbons (PAHs) are an abundant byproduct of industrial and natural pyrogenic processes. PAHs tend to persist in soil, providing a rich nutrient source for degrading bacteria. The degradation process may lead to the formation of toxic metabolites, however, there is limited research examining the hazards of transformation products in soil. Currently, the Environmental Protection Agency (EPA) classifies 16 toxic PAHs as priority pollutants without addressing the harmful metabolites. This project aims to select descriptors for a quantitative structure-activity relationship (QSAR) model based upon a data set containing TA98 Ames test known mutagens and non-mutagens. A logistic regression model determined 20 significant descriptors representing the molecular features linked to mutagenicity classification. Of these 20 descriptors, the number of rings larger than 12 members containing oxygen, (nFG12HeteroRing), the average centered Broto-Moreau autocorrelation (AATSC6c), and the z-modified information content index (ZMIC4), had the most significant link to mutagenicity classification based upon assessment of the corresponding logistic regression coefficients. These descriptors highlight the molecular structures that contribute to mutagenicity of PAHs within biodegradation pathways.
1. Introduction
Polycyclic Aromatic Hydrocarbons (PAHs) contain at least two aromatic rings and often result as a byproduct of various natural and industrial processes, including forest fires, extraction and burning of fossil fuels, and plastic manufacturing. PAHs are commonly found in the atmosphere and soil of surrounding ecosystems due to these processes. However, PAHs in soil tend to be more persistent and are a possible source of carbon for degrading bacteria [1]. The Environmental Protection Agency (EPA) currently classifies 16 PAHs as priority pollutants [2]. There is increasing concern over the mutagenic properties of some PAHs in the human body. However, the degrading bacteria transform these parent PAHs into various metabolites via a large multitude of pathways, which may have different toxic properties from the parent PAHs, making it difficult to thoroughly assess the potential hazard in the laboratory setting.
Quantitative structure-activity relationships (QSARs) have proven to be a useful tool for characterizing the toxicity of large chemical datasets, including those from PAH biodegradation, because of their predictive power [3]. QSARs can classify the endpoint toxic potential of input data based on a foundation of empirical training data and relevant structural and electronic descriptors. This foundation provides reproducible predictive ability for input data containing chemically similar compounds.
The training dataset and chosen descriptors used to build a QSAR are crucial to its applicability [4]. There are currently a variety of powerful QSARs available to the public which can predict narcotic toxicity but lack the specificity to classify mutagenic metabolites of PAHs in soil.
One such example is the Ecosar module of the Estimation Programs Interface (EPI) Suite, provided by the U.S. EPA, designed to estimate the toxicity as LC50 for a variety of organisms [5]. This system relies heavily upon log KOW, the logarithm of the octanol-water coefficient, to classify the toxicity of molecules. However, mutagenicity of PAH metabolites is not linearly related to log KOW, so the accuracy of this QSAR is limited in predicting mutagenicity [6]. There are also QSARs available based on nitro-PAHs, however, PAH nitrogenation only occurs in the atmosphere, making these QSARs unsuitable for soil and water ecological toxicity assessment [7]. Due to the narrow applicability of current QSARs there is a gap in accurate data for PAHs related to the potential mechanisms of toxicity via biodegradation in soil [8].
The goal of this study is to identify the relevant structural and electronic descriptors based on available empirical training data to build a QSAR tailored to PAHs and their potential metabolites in soil and water environments. To do so, this study aims to identify the features of hydrocarbon PAHs which result in either mutagenic or non-mutagenic tendency. Overall, this QSAR aims to bridge the gap in current toxicity classifiers that lack the capability to accurately sort the metabolites that result from biodegradation.
2. Methods
2.1 Data Refining
The empirical training data set was composed of data from the TA98 Ames test strains, obtained from the Chemical Carcinogenesis Research Information System [9]. The Ames test is a common reverse mutation bioassay used to assess mutagenicity of a compound, a useful toxic endpoint due to its connection with genotoxicity and carcinogenicity [10]. The TA98 Ames test strain is commonly used for hydrocarbon mutagenicity assessment.
Prior to utilizing the data to identify relevant structural descriptors, the empirical data set was refined to remove any outliers that did not represent PAH degradation well. PAH degradation in soil typically follows a pathway involving oxygenation then a ring opening reaction. In order to best reflect compounds likely to occur in a natural environment, the dataset was limited to a molecular weight of less than 500 amu and fewer than 5 rings. The Ames test often uses enzymatic activation to represent an organism’s internal metabolism, however for this study, only data that did not use enzymatic activation, known as direct acting mutagens, was used in order to best represent a natural environment. 2.2 Descriptor Selection
The refined data set was then used to identify the relevant descriptors that may indicate a mutagen. Kohn-Sham density functional theory (DFT) calculations were performed in Gaussian 09 [11]. Neutral structures were optimized and confirmed to minima through the absence of imaginary frequencies. In order to simulate environmental conditions, calculations were performed at 20 °C with water as a solvent using the conductor-like polarizable continuum model (CPCM) [12]. The functional M06-2X [13] called M06 and M06-2X. The M06 functional is parametrized including both transition metals and nonmetals, whereas the M06-2X functional is a high-nonlocality functional with double the amount of nonlocal exchange (2X was used with basis set 6-311G(d,p) [14]. M06-2X includes dispersion correction in its formula and performs well with water-based solvation calculations. [15]. Gibbs free energy, Highest Occupied Molecular Orbital (HOMO) energy, Lowest Unoccupied Molecular Orbital (LUMO) energy, ionization potential, and electron affinity for each molecule in the data set was obtained. Ionization potential and electron affinity were performed at STP in the gas phase to agree with NIST.
Approximately 1495 topological descriptors were added via the PaDEL-Descriptor software [16]. Recursive feature elimination was used to select the most relevant descriptors. Variance inflation factor (<2.5) was then used to eliminate descriptors with close correlations, reducing the data set to the 20 most relevant descriptors. To then assess the accuracy of classification for the empirical data with these chosen descriptors, a receiver-operating characteristic (ROC) curve was generated using 10 iterations of 3-fold cross validation. On each iteration, two thirds of the data were used for training, and one third for testing. Each iteration the data was randomized to select different values for testing and training. Code used for this analysis is available on github: https://github.com/ngLabGroup/ ta98_mutagen_qsar.
3. Results
Using these descriptors, Figure 1 details the current Receiver Operating Characteristic (ROC) curve training assessment. With an area under the curve of 0.930, this suggests a high level of accurate classification of the testing data. The overall accuracy of this run was 0.876, precision was 0.659, sensitivity was 0.747, and the F1 was 0.700.
Figure 1: ROC curve representing 20 most relevant topological descriptors
These logistic regression coefficients of the descriptors indicate the relationship between the descriptor and mutagen classification, as shown in Figure 2. The strongest relationships, either positive or negative, are expressed in the logistic regression coefficients for descriptors relating the number of rings larger than 12 members containing oxygen (nFG12HeteroRing), the average centered Broto-Moreau autocorrelation (AATSC6c), and the z-modified information content index (ZMIC4), which have values of 1.85, -1.41, and -1.04 respectively.
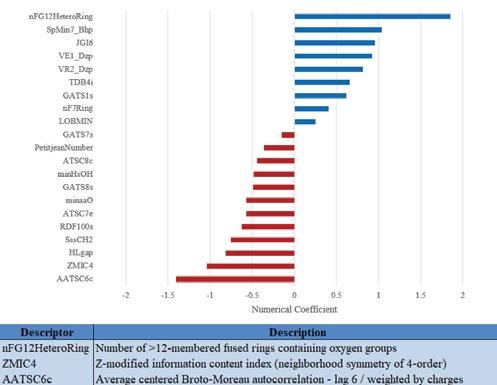
Figure 2: Coefficients of logistic regression for 20 most relevant topological descriptors and descriptions of the 3 strongest relationships for PAH mutagenicity classification
4. Discussion
Establishing this set of relevant descriptors to the training data set is the first step to building a QSAR able to assess novel data. These initial assessments of the empirical data have narrowed the descriptor data set to 20 descriptors from the original 1000. nFG12HeteroRing, AATSC6c, and ZMIC4 have the most significant effect on classification according to Figure 2. The strong positive regression coefficient for nFG12HeteroRing suggests that oxygen atoms within the ring are significant to the mechanism to reach mutagenic metabolites. The molecules with the highest nFG12HeteroRing count share epoxide rings as a common feature, agreeing with literature that epoxide rings in specific structural positions may influence mutagenicity [17]. AATSC6c relates charge to mutagenicity by assessing similar charges 6 atoms away which is in relation to oxygen groups for this study. The negative regression coefficient suggests that atoms with a large distance between oxygen groups are less likely to be mutagens. Finally, ZMIC4 refers to the symmetry and branching substituents of the molecule. The negative regression coefficient suggests that the number of branching substituents inversely relates to the mutagenicity. Overall, the influence of these descriptors in classification suggests that oxygen groups are a critical component in the mechanisms which drive mutagenicity. The accuracy value from the ROC curve suggests accurate overall classification of mutagens vs. non-mutagens for this QSAR model. Additionally, the sensitivity value from the ROC curve indicates that a number of mutagens are incorrectly classified as non-mutagens in the training data set. The dataset is unbalanced with about five times as many non-mutagens as mutagens. A threshold for classifying mutagens vs non mutagens was manually chosen to maximize the F1 score and the precision value for this analysis. Precision is another crucial measure to understanding how many structures are correctly classified based on the total number of mutagenic classifications. Future work should select this threshold mathematically based on the dataset and desired optimal performance of specific classifier metrics. Ideally, the precision and specificity values are maximized to ensure every mutagen is correctly classified.
5. Conclusions
One goal with this QSAR is to create a transparent computational model with easily interpretable results. 20 descriptors are simply too many factors to interpret individual effect, however, this study significantly narrows down which descriptors are most influential on mutagenicity classification. Additional methods of statistical elimination are necessary to both validate and refine the current results. Such steps include determining which descriptors overlap significantly in the classification of data. These next steps will aid the QSAR in providing a transparent and reliable prediction method of PAH metabolite mutagenicity, efficiently reducing the amount of lab work needed to experimentally determine the hazardous properties.
6. Acknowledgements
Mentorship was under Trevor Sleight, P.E, Dr. Carla Ng, and Dr. Leanne Gilbertson. Funding provided by the Swanson School of Engineering Summer Undergraduate Research Internship (SURI) program and the Office of the Provost. Special thanks for Dr. Ioannis Bourmpakis for assistance with the Gaussian calculations.
7. References
[1] H. I. Abdel-Shafy and M. S. M. Mansour, “A review on polycyclic aromatic hydrocarbons: Source, environmental impact, effect on human health and remediation,” Egypt. J. Pet. 25 (2016) 107–23. [2] U. S. EPA, “Priority Pollutant List.” [3] A. K. Debnath et al. “Quantitative structureactivity relationship investigation of the role of hydrophobicity in regulating mutagenicity in the Ames test: 2. Mutagenicity of aromatic and heteroaromatic nitro compounds in Salmonella typhimurium TA100,” Environ. Mol. Mutagen. 19 (1992) 53–70. [4] M. T. D. Cronin et al. “Use of QSARs in international decision-making frameworks to predict ecologic effects and environmental fate of chemical substances,” Environ. Health Perspect. 111 (2003) 1376–90. [5] O. US EPA, “EPI SuiteTM-Estimation Program Interface,” US EPA, 2015. https://www.epa.gov/tsca-screening-tools/ecological-structure-activity-relationships-ecosar-predictive-model (accessed Jun. 22, 2020). [6] D. Ghosal et al. “Current State of Knowledge in Microbial Degradation of Polycyclic Aromatic Hydrocarbons (PAHs): A Review,” Front. Microbiol. 7 (2016). [7] A. K. Debnath et al. “Structure-Activity Relationship of Mutagenic Aromatic and Heteroaromatic Nitro Compounds. Correlation with Molecular Orbital Energies and Hydrophobicity,” J. Med. Chem. 34 (1991) 786–97. [8] P. Gramatica et al. “Quantitative structure–activity relationship modeling of polycyclic aromatic hydrocarbon mutagenicity by classification methods based on holistic theoretical molecular descriptors,” Ecotoxicol. Environ. Saf. 66 (2007) 353–61. [9] National Library of Medicine, “Chemical Carcinogenesis Research Information System,” https://www.nlm. nih.gov/databases/download/ccris.html. [10] B. N. Ames et al. “An improved bacterial test system for the detection and classification of mutagens and carcinogens,” Proc. Natl. Acad. Sci. U. S. A. 70 (1973) 782–86. [11] Frisch, M. J. et al. “Gaussian 09,” Wallingford CT: Gaussian, Inc. 2013. [12] V. Barone and M. Cossi, “Quantum Calculation of Molecular Energies and Energy Gradients in Solution by a Conductor Solvent Model,” J. Phys. Chem. A. 102 (1998) 1995–2001. [13] Y. Zhao and D. G. Truhlar, “The M06 suite of density functionals for main group thermochemistry, thermochemical kinetics, noncovalent interactions, excited states, and transition elements: two new functionals and systematic testing of four M06-class functionals and 12 other functionals,” Theor. Chem. Acc. 120 (2008) 215–41.
[14] R. Krishnan et al. “Self-consistent molecular orbital methods. XX. A basis set for correlated wave functions,” J. Chem. Phys. 72 (1980) 650–54. [15] Z. L. Seeger and E. I. Izgorodina, “A Systematic Study of DFT Performance for Geometry Optimizations of Ionic Liquid Clusters,” J. Chem. Theory Comput. 16 (2020) 6735–53. [16] C. W. Yap, “PaDEL-descriptor: An open source software to calculate molecular descriptors and fingerprints,” J. Comput. Chem. 32 (2011) 1466–74. [17] A. W. Wood et al. “Inhibition of the mutagenicity of bay-region diol epoxides of polycyclic aromatic hydrocarbons by naturally occurring plant phenols: Exceptional activity of ellagic acid,” Proc. Natl. Acad. Sci. 79 (1982) 5513–17.