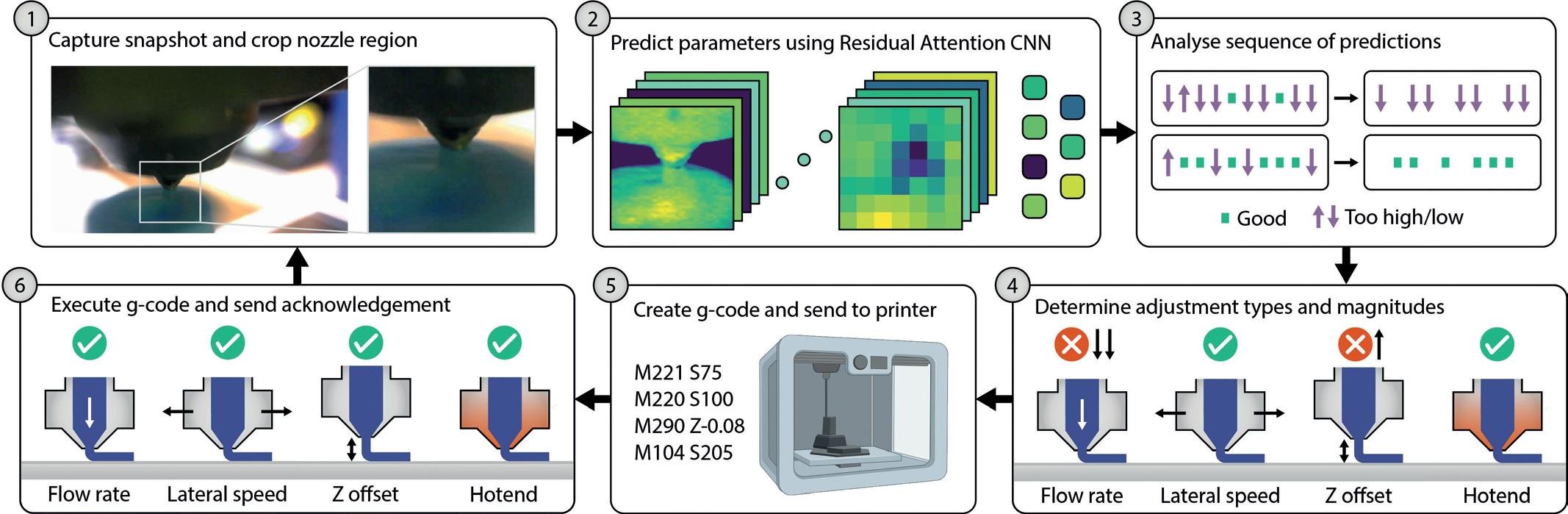
3 minute read
MACHINE LEARNING FOR CORRECTING AND PREVENTING AM ERRORS
MACHINE LEARNING FOR CORRECTING AND PREVENTING ADDITIVE MANUFACTURING ERRORS
WORDS: Douglas Brion, Sebastian Pattinson, Department of Engineering, University of Cambridge
While additive manufacturing processes provide unique opportunities, they are generally complex and slow. This leads to many ways for things to go wrong and makes it costly when they do. Typical errors range from small geometrical inaccuracies or mechanical weakness to complete build failures.
Usually, a skilled worker observes the process to prevent or correct errors. The worker needs to recognise an error, stop the print, remove the part, and make a judgement on how to adjust settings for a new part. Workers need training to carry out this task as well as time to gain experience when working with new printers or materials. Even so, a human cannot constantly watch multiple printers at the same time, especially not for very long prints. Nor can a human correct printer settings in real-time. And there are common errors, such as warping, where it is often unclear how to update settings to prevent future occurrences.
This has motivated a lot of work in integrating sensors into additive manufacturing systems to automatically detect errors. This has included acoustic, inertial and current sensors, but camerabased approaches are most widely adopted given their versatility and data-richness. Coupling cameras to traditional computer vision approaches has been successful in explicitly detecting specific errors in parts and 3D printing systems for which they have been calibrated. But it is very difficult to handcraft algorithms that work for different errors, parts, printers, materials and printing setups.
Machine learning, particularly deep learning, approaches have shown unprecedented performance in many applications including vision. This has led to several recent examples of applying machine learning to detecting errors in additive processes, but usually still only in a single part and for a single type of error. The greatest potential advantage of machine learning though, to enable robust error detection and correction that works across different parts, materials and printers, remains unexplored.
In work recently published open-access in Nature Communications, we made intelligent 3D printers that can rapidly detect and correct errors, even in new parts, materials and printing systems., via learning from other printers. We did this by developing a machine learning algorithm that can both detect and correct diverse errors in real-time, and can be easily added to new or existing machines to enhance their capabilities. The algorithm was trained by showing it approximately 950,000 images taken from a printheadmounted camera during the printing of 192 different parts. Every image was labelled with relevant printer settings, for example the temperature of the nozzle and the flow rate of material from the nozzle. The algorithm was also shown how far those settings were from appropriate values. This combination enabled the algorithm to learn not just when things go wrong, but also how to fix it when they do, just by looking at an image of the process.
During the training phase, the algorithm was only shown images from a single type of FFF 3D printer using PLA polymer. But the algorithm was able to correct errors in different polymers and even unfamiliar systems such as direct ink write ketchup and mayonnaise. This suggests that the algorithm is able to pick up features that are general to extrusion 3D printing.
A future system could significantly improve the productivity and reliability of additive manufacturing in general, leading to new markets including in safety-critical applications. We are commercialising this technology through Matta, a spin-out. We are especially interested in developing learning systems to prevent errors in high-value parts including in the aerospace, automotive and energy sectors. The loss in machine time, energy, material and from discovering an error during final inspection in such applications would amplify the benefits from our learning approach.
The potential for machine learning technologies in additive manufacturing is vast and we are only scratching the surface of what it is capable of. Given machine learning’s increasing power, accessibility and the need for better solutions to complex manufacturing challenges, machine learning is poised to play a key role in driving additive manufacturing to ever greater heights.