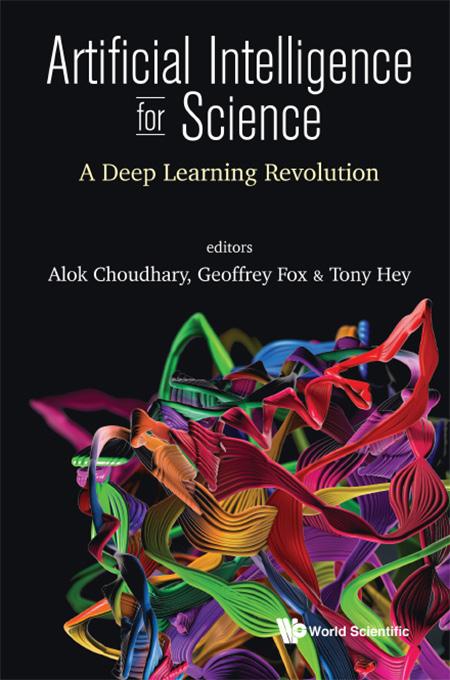
4 minute read
Artfcial Intelligence for Science
A Deep Learning Revoluton
Alok Choudhary
Advertisement
Northwestern University, USA
Geofrey Fox
University of Virginia, USA
Descripton
Tony Hey
Rutherford Appleton Laboratory, UK
This unique collecton introduces AI, Machine Learning (ML), and deep neural network technologies leading to scientfc discovery from the datasets generated both by supercomputer simulaton and by modern experimental facilites.
Huge uanttes o e perimental data come rom man sources telescopes, satellites, gene se uencers, accelerators, and electron microscopes, including internatonal acilites such as the Large Hadron Collider (LHC) at CE in Geneva and the E okamak in rance. hese sources generate man petab tes moving to e ab tes o data per ear. E tractng scientfc insights rom these data is a ma or challenge or scientsts, or whom the latest developments will be essental.
he tmel handbook benefts pro essionals, researchers, academics, and students in all felds o science and engineering as well as , L, and neural networks. urther, the vision evident in this book inspires all those who in uence or are in uenced b scientfc progress.
Editors
Dr Alok Choudhary is a pro essor, researcher and an entrepreneur. He is the Henr and sabel ever chaired Pro essor o Electrical and Computer Engineering, pro essor o Computer Science and also teaches at Kellogg School o anagement at orthwestern Universit . r lok Choudhar is also the ounder, chairman and chie scientst o 4C insights, a data science so ware compan . 4C was ac uired b edia cean in 2020.
Geofrey Fox received a Ph in heoretcal Ph sics rom Cambridge Universit , where he was Senior Wrangler. He is now a Pro essor in the iocomple it nsttute nitatve and Computer Science epartment at the Universit o irginia. He previousl held positons at Caltech, S racuse Universit , lorida State Universit , and ndiana Universit . a er being a postdoc at the nsttute or dvanced Stud at Princeton, Lawrence erkele Laborator , and Peterhouse College Cambridge. He has supervised the Ph o 75 students. He has an hinde o 85 with over 4 ,000 citatons. He received the High Per ormance Parallel and istributed Computng (HP C) chievement ward and the C EEE CS Ken Kenned ward or oundatonal contributons to parallel computng in 20 9. He is a ellow o PS (Ph sics) and C (Computng) and works on the interdisciplinar inter ace between computng and applicatons. He is currentl actve in the ndustr consortum LCommons LPer .
Tony Hey is a ellow o the o al cadem o Engineering, the ssociaton or Computng achiner , and the merican ssociaton or the dvancement o Science. t the Universit o Southampton in the UK, his parallel computng research group designed and built one o the frst distributed memor message passing computers using innovatve nmos transputers. He was later Head o the Electronics and Computer Science epartment at Southampton and also ean o Engineering. n 2005 he was awarded a C E or Services to Science a er leading the UK s eScience initatve.
February 2023
Imprint: World Scientfc Publishing Compan
Extent: 500pp
Type: eview olume
Main Subject: Computer Science
Sub-Subjects: rtfcial ntelligence achine Learning eural etworks etworking
Keywords: L eep ig eural etworks ig ata Scientfc pplicatons or Science
Readership: esearchers, pro essionals, academics, and graduate students in machine learning, neural networks and environmental science
• Contributng Authors:
AI for Science (Alok Choudhary, Geofrey Fox, and Tony Hey)
Overview of the Book
• Setng the Scene:
Data Driven Science in the Era of AI: From Paterns to Practce ( Alex Szalay)
AI in the Broader Context of Data Science (Rafael Alvarado and Philip Bourne)
AlphaFold — The End of the Protein Folding Problem or the Start of Something Bigger? (David T Jones and Janet M Thornton)
Applicatons of AI in Astronomy (S G Djorgovski, A A Mahabal, M J Graham, K Polsterer, and A Krone-Martns)
Machine Learning for Complex Instrument Design and Optmizaton (Barry C Barish, Jonathan Richardson, Evangelos Papalexakis, and Rutuja Gurav)
Artfcial Intelligence (AI) and Machine Learning (ML) at Experimental Facilites ( JA Sethian, JJ Donatelli, A Hexemer, MM Noack, DM Pelt, DM Ushizima, and PH Zwart)
The First Exascale Supercomputer: Acceleratng AI-for-Science and Beyond (Satoshi Matsuoka, Mohamed Wahib, Aleksandr Drozd, and Kento Sato)
Benchmarking for AI for Science (Jeyan Thiyagalingam, Mallikarjun Shankar, Geofrey Fox, and Tony He y)
• Exploring Applicaton Domains:
◊ Astronomy and Cosmology:
Radio Astronomy and the Square Kilometre Array ( Anna Scaife)
AI for Astronomy: The Rise of the Machines (Andy Connolly)
◊ Climate Change:
AI and Net Zero ( Alberto Arribas, Karin Strauss, Sharon Gillet, Amy Luers, Trevor Dhu, Lucas Joppa, Roy Zimmermann, and Vanessa Miller)
AI for Climate Science (Philip Ster)
◊ Energy:
Acceleratng Fusion Energy with AI (Steve Cowley, Dan Boyer, and Michael Churchill )
Artfcial Intelligence for a Resilient and Flexible Power Grid (Olufemi A Omitaomu, Jin Dong, and Teja Kurugant )
◊ Environmental Science:
AI and Machine Learning in Observing Earth from Space ( Jef Dozier)
Artfcial Intelligence in Plant and Agricultural Research (Sabina Leonelli and Hugh F Williamson)
◊ Health:
AI and Pathology: Steering Treatment and Predictng Outcomes (Rajarsi Gupta, Jakub Kaczmarzyk, Soma Kobayashi, Tahsin Kurc, and Joel Saltz)
The Role of Artfcial Intelligence in Epidemiological Modeling (Madhav V Marathe et al.)
◊ Life Sciences:
Big AI: Blending Big Data With Big Theory to Build Virtual Humans (Peter Coveney and Roger Highfeld )
A Roadmap for Defning Machine Learning Standards in the Life Sciences (Fots Psomopoulos, Carole Goble, Leyla Jael Castro, Jennifer Harrow, and Silvio C E Tosato)
◊ Materials Science and Engineering:
Artfcial Intelligence for Materials (Debra J Audus, Kamal Choudhary, Brian L DeCost, A Gilad Kusne, Francesca Tavazza, and James A Warren)
Artfcial Intelligence for Acceleratng Materials Discovery (Ankit Agrawal and Alok Choudhary)
◊ Partcle Physics:
Experimental Partcle Physics and Artfcial Intelligence (David Rousseau)
AI and Theoretcal Partcle Physics (Rajan Gupta, Tanmoy Bhatacharya, and Boram Yoon)
◊ The Ecosystem of AI for Science:
Schemaorg for Scientfc Data (Alasdair Gray, Leyla Castro, Nick Juty and Carole Goble)
AI-enabled HPC Workfows (Shantenu Jha, Vincent Pascuzzi, and Mateo Turilli )
AI for Scientfc Visualizaton (Han-Wei Shen and Chris Johnson)
Uncertainty Quantfcaton in AI for Science (Tanmoy Bhatacharya, Christna Garcia Cardona, and Jamal Mohd-Yusof )
AI for Next Generaton Global Network-Integrated Systems and Testbeds (Mariam Kiran and Harvey Newman)
AI for Optmal Experimental Design and Decision Making (Francis J Alexander, Kristofer-Roy Reyes, Lav R Varshney, and Byung-Jun Yoon)
FAIR: Making Data AI-Ready (Susanna-Assunta Sansone, Lee Harland, Philippe Rocca-Serra, and Mark Wilkinson)
• Perspectves on AI for Science:
Large Language Models for Science ( Austn Clyde, Arvind Ramanathan, and Rick Stevens)
AI for Autonomous Vehicles (Tom St John and Vijay Janapa Reddi )
The Automated AI-Drive Future of Scientfc Discovery (Ross King and Hector Zenil )
Towards Refecton Competencies in Intelligent Systems for Science (Yolanda Gil )
The Interface of Machine Learning and Causal Inference (Mohammad Taha Bahadori and David Heckerman)
• Endpiece: AI Tools and Concepts: Surve o eep Learning ethods ethods iscussed in Chapters