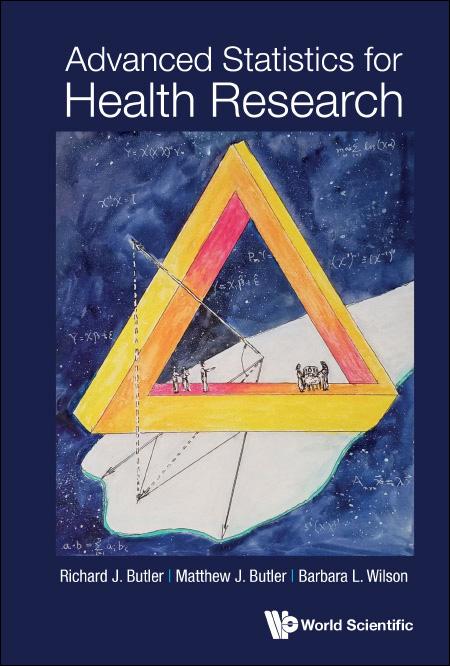
3 minute read
Advanced Statstcs for Health Research
Richard J Butler
Brigham Young University, USA & Southwestern University of Finance and
Advertisement
Economics, China
Mathew J Butler
Mathew J Butler
Key Features
Mathew J Butler University of Utah College of Nursing, USA
• Simple geometric fgures make causal in erence techni ues more intuitve
• Eas to understand empirical e amples are drawn rom a broad collecton o the health research literature
• S S, Stata, and codes make implementng regression models straigh orward
Descripton
Advanced Statstcs for Health Research provides a rigorous geometric understanding of models used in the analysis of health data, including linear and non-linear regression models, and supervised machine learning models. Models drawn rom the health literature include ordinar least s uares, two stage least s uares, probits, logits, Co regressions, duraton modeling, uantle regression and random orest regression. Causal in erence techni ues rom the health literature are presented including randomi aton, matching and propensit score matching, di erences in di erences, instrumental variables, regression discontnuit , and f ed e ects anal sis. Codes or the respectve statstcal techni ues presented are given or S , S S and .
Authors
Mathew J Butler, Economic Ph rom the Universit o Cali ornia erkele , has taught as a visitng and ad unct instructor or the righam oung Universit Economics epartment since 2007. He has also worked as a health economist or Universit o Utah Health. Currentl he works in the isk anagement ivision in he Church o esus Christ o La er da Saints. Prior to his positon in isk anagement, he worked in the Correlaton esearch ivision.
Richard J Butler, Economic Ph rom the Universit o Chicago, has taught at Cornell Universit , the Universit o innesota (C rthur Williams Pro essor o nsurance), and righam oung Universit ( artha ane Knowlton Cora Pro essor o Economics), and at Southwestern Universit o inance and Economics (China). His has received numerous awards or his research, including the Kulp Wright twice (200 or his solo authored te tbook in insurance, and again or contributng to another best book in insurance awarded in 20 5), the Kemper (best artcle in isk anagement eview), and the ehr (twice or best artcle standing the test o tme) awards. He was co editor o the ournal o isk and nsurance.
Barbara L Wilson, ursing Ph , Universit o ri ona, has spent man ears in the acute care se ng where she assumed various clinical and administratve roles, primaril in women s and newborn services. r Wilson s research interests are ocused on healthcare services, evaluatng the in uence o providers, hospitals, and the care deliver s stem on patent outcomes and on the use o Human actors Engineering (H E) in reducing clinical errors and promotng patent sa et .
February 2023
£85 | HARDCOVER 978 98 26 230 2
Imprint: World Scientfc Publishing Compan
Extent: 300pp
Type: onograph
Main Subject: Economics inance
Sub-Subjects: Health Economics iostatstcs General Li e Sciences
Politcal Science Polic Studies Public Polic Statstcs athematcal inance Economics
Keywords: ursing Ph sician
Healthcare Public Health egression rthogonal Pro ecton Geometric iew o Causal n erence 2SLS wo Stage Least S uares nstrumental ariables Probits Logits Proportonal Ha ards Co egressions uantle egression andom orest egression andomi aton atching Propensit Score i erences in i erences egression iscontnuit i ed E ects S S S esearch E amples
Empirical ule pplied Statstcs
Confdence ntervals
Readership: or health pro essionals, advanced undergraduate and graduate students who stud regressions, causal in erence, or supervised machine learning
• Getng Started with the Data: Introducton, Statstcs, Empirical Rule, and Histograms
Characterizing The Data: Scaterplots and Regressions
Multple Regression Contnued: Standardized Beta Coefcients, Picturing Regressions, Variance Infaton Factor
The Data Generatng Process (DGP) That Makes Regressions Important, and How to Do Regressions Badly Causal Estmates: Randomizaton, Matching, Instrumental Variables and Their Cousins
• Randomizaton and Causal Frameworks with Regressions: Studies with Randomized Treatments
Studies From Data Involving Matching and Propensity Score Matching
Studies with Instrumental Variable Estmaton (Two Stage Least Squares) Studies with Regression Discontnuity
Studies using Diference-In-Diferences with Propensity Score Matching
Studies with Panel Data Sets with Inital Randomizaton
Studies with Panel Data Sets with Fixed Efects and Lags
Studies with Panel Data Techniques using GMM Estmaton
• Beyond the Contnuous, Uncensored Regression Model: Discrete Outcomes, Duraton Modeling, Quantle and Random Forest Regressions: iscrete utcomes and a imum Likelihood Probits, Logits, ultnomial and rdered Logits
C Curves and the UC etrics, Count odels
Co egressions
Parametric uraton odels and iscrete uraton odels uantle egression
Selecton n Linear odels, L SS , and andom orest egression andom orest egression esidual nal sis and the egression Gini nde