
25 minute read
PROVIDING REAL-TIME INFORMATION FOR URGENT CARE
NAV MUSTAFEE AND JOHN POWELL
A&E WAITING TIMES HAVE INCREASED SUBSTANTIALLY OVER RECENT YEARS. In the U.K., it is expected that 95% of patients should be assessed, treated, then either admitted, transferred (to a different provider) or discharged within four hours of presentation. This is commonly referred to as the four-hour standard. Nationally, NHS emergency departments have not met the standard in any year since 201314, and it has been missed in every month since July 2015, as reported by the King’s Fund (see http://bit.ly/NHS4hour). This standard also applies to Minor Injury Units (MIUs) and Urgent Care Centres (UCCs), which treat non-life-threatening conditions, and which, together with A&E departments, are part of the NHS Urgent Care Network. Here we explain how the NHSquicker platform was developed to help patients in need of urgent care to make more informed decisions about available healthcare choices, thereby not only reducing the wait time being experienced by the patients but also helping the NHS meet the four-hour standard.
MOTIVATION In 2015, while working on a project modelling the A&E department at Torbay Hospital, we found that in the Torbay Urgent Care Network, which in 2015 consisted of one A&E and seven MIU/UCC centres, all MIU/ UCCs met the four-hour standard, while A&E underperformed by nearly 20%. We concluded that research on the levelling of demand across the Network would offer an exciting new dimension to our existing A&E patient flow simulation work. A further motivation of our work was the 2013 Keogh Review of urgent and emergency care. It stated that patients with urgent but non-life-threatening needs should be treated outside of hospitals by services that deliver care in or as close to people’s homes as possible, in MIU/ UCCs, while those with more serious or life-threatening emergency needs are treated in centres (A&E departments) having the very best expertise and facilities specific to those needs. It was argued that increased localisation of the treatment of those with less serious needs will relieve pressure on the hospital-based emergency services, thus freeing up resources to cater for patients with more serious and life-threatening conditions such as severe chest pain, serious blood loss, choking and unconsciousness. The success of this partitioning policy is dependent on two related factors, namely the presentation of patients at the appropriate treatment facility and the capacity of the A&E, in particular, to cope with demand. Inevitably the capacity of A&E departments is finite, and it is highly desirable that patient demand be spread among the available facilities in a given region, so as to reduce waiting time and to shape demand, thus spreading the pressures on staff and facilities. it is highly desirable that patient demand be spread among the available facilities in a given region, so as to reduce waiting time and to shape demand
PARTNERSHIP BETWEEN THE UNIVERSITY AND THE NHS In response to these policies and requirements, we worked with several NHS Trusts in the South West of England to investigate how existing data, already being captured by NHS, could be used to relieve pressure on A&E departments. We founded the Health and Care IMPACT Network in 2016 through a collaboration between the University of Exeter Business School and Torbay and South Devon NHS Foundation Trust (Directorate of Strategy & Improvement). The purpose of the Network, Co-Chaired by Professor Nav Mustafee and Dr Andrew Fordyce from Torbay Hospital, is to improve delivery of health and care through applied research, knowledge dissemination and decision support.
The Network enabled us to bring together clinicians, managers and O.R./ data people from multiple NHS Trusts in the South West of England and to work towards agreeing on a format for A&E waiting time data. A common data standard was necessary as our objective was to develop a digital platform at a regional level (rather than a Trust-specific solution), and as the Trusts deploy various A&E patient flow systems (I.T. systems such as Symphony, PatientFirst and IMS Maxims), a consistent data format would ensure that the platform would receive data feeds from multiple systems. Further, the design and user interface of the NHSquicker app was co-developed with the NHS. Towards this, in June 2017, we organised the third IMPACT Network workshop on Urgent and Emergency Care in Exeter Business School. Susan Martin (Quality Lead at Torbay and South Devon NHS Foundation Trust) can be seen leading a session in Figure 1.
AN APP FOR URGENT CARE FOR THE SOUTHWEST The co-development work was instrumental in outlining the design of the architecture of the real-time platform and the app. We worked with an SME for the implementation work (funded through a grant). In a nutshell, the NHSquicker platform provides indirect suggestions (nudges) to support patients in need of urgent care to help them make more informed decisions about available healthcare choices. The platform comprises (a) a user-facing app (Android and iOS) that provides nudges taking into account the live waiting time from the urgent care centres and combining them with real-time travel time (retrieved through Google Maps APIs); (b) the platform backend that receives real-time feeds and allows for easy integration of new feeds; (c) a business intelligence dashboard designed for use in MIU/UCCs and A&E.
The NHSquicker app helps patients make informed decisions, for example,

FIGURE 1 THE CO-DEVELOPMENT WORKSHOP FOR NHSQUICKER
whether to visit a facility which may be nearer to them but with a long waiting time or travel to an alternative location that is further away but with a shorter waiting time. By transforming real-time wait time data to actionable insights and nudges, NHSquicker seeks to:
a) Encourage prospective patients to choose the appropriate type of treatment facility for their condition, so that only those with more serious needs present at the A&E. The aim of this is to reduce the overall demand on the A&E facilities by redirecting less serious cases to the more appropriate facilities of MIUs/
UCCs, thus reducing waiting times
at the A&E facilities. b) Shape demand at A&E facilities by encouraging patients needing such facilities to choose a destination with a lower waiting time.
NHSquicker aims to influence destination choices made by prospective patients so as to aid NHS frontline staff in their day-today operations, firstly by improving the appropriateness of centre choice and secondly by smoothing demand over inevitably stretched facilities, particularly those offering emergency treatment. What effect does (a) have on (b)? Patients do not have a direct role in managing the operations of an urgent care facility. However, the decisions they take have a bearing on its performance. For example, when confronted with the need for urgent treatment, the intended users have to make location decisions as to the place of treatment. If they are unaware of the availability of urgent care services appropriate to meet their needs close to where they are located, they will usually choose to go to A&E as they are confident they will be seen and have their needs met. This may lead to the overcrowding of A&E, while at the same time, MIU/UCCs that are located nearby may be operating under capacity; both cases will have operational implications.
NHSquicker (version 1) was launched in December 2017. Since then, the solution has worked uninterruptedly for almost three years. We have worked to expand its reach and to develop the real-time platform
further (e.g. version 2 was released in February 2020 and now includes integration with the NHS Directory of Services). At the time of writing (December 2020), NHSquicker receives real-time data on waiting times from 27 A&E departments and Urgent Care Centres (UCC) that are operated by seven NHS Trusts in Devon, Cornwall, Somerset and Bristol. The wait time data is not open-source data, but data that is available only to us through Trust-level contacts made possible through the Health and Care IMPACT Network which we developed.
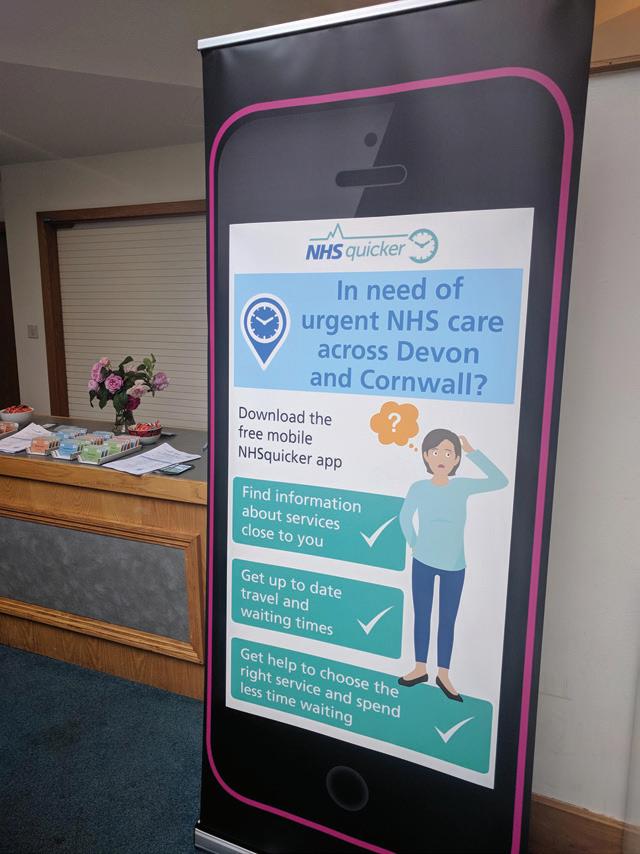
BENEFIT OF THE O.R. SOLUTION We outline three instances which demonstrate the efficacy of NHSquicker and the benefits being experienced by both patients and by the Trusts.
First, NHS Trusts in the South West have adopted this technology by interfacing their I.T. systems with NHSquicker. At launch (Dec 2017), only data feeds from Torbay & South Devon NHS Foundation Trust (TSDFT), Royal Devon & Exeter (RD&E) and Northern Devon Healthcare NHS Trust (NDFT) were integrated. As of December 2020, NHSquicker receives data from all four acute Trusts in the Devon STP (sustainability and transformation partnership), the Cornwall and the Isles of Scilly STP, one Trust from the Somerset STP and one from Bristol. Trusts invest in terms of their technical manpower to integrate our app with their services and for the operational upkeep. This demonstrates the benefits realised by Trusts in using the app. Nic Harrison, data analyst at NDFT, said: “We collect a huge amount of data in the NHS to help us to monitor and improve the care we provide. This project was about using information that is already available in a new way which helps to improve the experience of our services and helps us to make sure NHS resources are focused where they are most needed.”
Second, patients are finding the app useful. We know this because we developed an in-app questionnaire and integrated it with NHSquicker version 2 (launched Feb 2020). We received 543 responses between 24/03/2020 and 10/09/2020. The analysis of the questionnaire shows that ∼78% (424) of the users agreed that NHSquicker helped them decide where to go and a further 4% responded that NHSquicker provided helpful information at the point of use (in addition to real-time wait time and information from NHS Directory of Services on local services, the app also includes links for users to find information on conditions and treatment and a link to access NHS 111 Online - 111.nhs.uk).
Third, evidence from two early adopters of NHSquicker (TSDFT and NDFT) has shown that our solution is having the desired impact of signposting patients away from busy A&E department at peak times. For TSDFT, when analysing the data at peak time (between 11.00-18.00), our data analysis for the period Jan. 2016–Feb. 2019 has shown that there is a significant shift from the start of 2018 (when NHSquicker was first launched and we benefited from media publicity) in the pattern of attendances, with a reduction in A&E attendances and an increase in MIU attendances. The quarterly changes from the previous equivalent quarter confirm this. Our analysis of data for NDFT shows a similar pattern. Dr Nick Mathieu, consultant in emergency medicine and clinical director of the emergency department at TSDFT, said: “This app will give people the information they need so they can make informed decisions about where to go for treatment. We hope this will improve things for patients, as they may be able to receive the care they need more quickly and perhaps closer to home than they realise. NHS services across England are busier than ever and we hope NHSquicker will increase awareness of the different options for treating minor injuries and illnesses. We hope this will contribute to reducing pressure on emergency departments, so they can focus on the most urgent cases.”
In conclusion, it has been a rich experience working with the NHS and various other organisations like NHS Digital and Reactor15 (SME).
We have shown that local-level digital initiatives can be scaled-up to regional level solutions. We have demonstrated that unlocking existing data, captured in different A&E systems and stored in a multitude of databases, is possible through common standards and that it could also be accessible at real-time. We have shown how data from multiple sources could be joined (wait time data with travel data), and then transformed into a form that helps empower patient decision making.
ACKNOWLEDGEMENTS The work of this magnitude could only be realised through the shared vision of ‘making data work at both an individual (patient) level and at a more system (Trust) level’. We would like to specially acknowledge the contribution of Susan Martin, Dr Andrew Fordyce, Steve Judd (Torbay), Alison Harper, Surajeet Chakravarty and Todd Kaplan (Exeter), Alaric Moore (RD&E) and Nic Harrison (NHDT), Nick Metcalfe (SWAST), Paul Uren (Plymouth), Paul Brandwood (RCHT), Steve Read (Taunton & Somerset), Fran Draper (Bristol), Mike Saunders, Lee Wade and Mark Saunders (R15). We acknowledge funding that was received from the University of Exeter, ESRC Impact Acceleration Awards, Torbay Medical Research Fund and South West Academic Health Sciences Network.
Nav Mustafee is Professor of Analytics and Operations Management at University of Exeter Business School. He is an honorary researcher at Torbay & South Devon NHS Foundation Trust and is the founding co-chair of the Health and Care IMPACT Network. Nav has research interests in the use of interdisciplinary methods and techniques with computer simulation (hybrid modelling), simulation methodologies and hybrid simulation, and bibliometric analysis of knowledge domains. He is an Associate Editor for Simulation: The Transactions of the SCS, the Journal of Simulation and the Health Systems journal. He has an undergraduate degree from the University of Calcutta (India) and MSc and PhD degrees from Brunel University.
John Powell is Professor Emeritus at the University of Exeter. He is a Board Member for the Sustainable Leadership Foundation. His research interests are in systems modelling of strategic situations, scenario planning and modelling, knowledge in strategy and knowledge management. He was formerly the Head of School of the Stellenbosch University Business School, South Africa. He has a B.A. and an M.A. degree from the University of Cambridge and a PhD from Cranfield University.
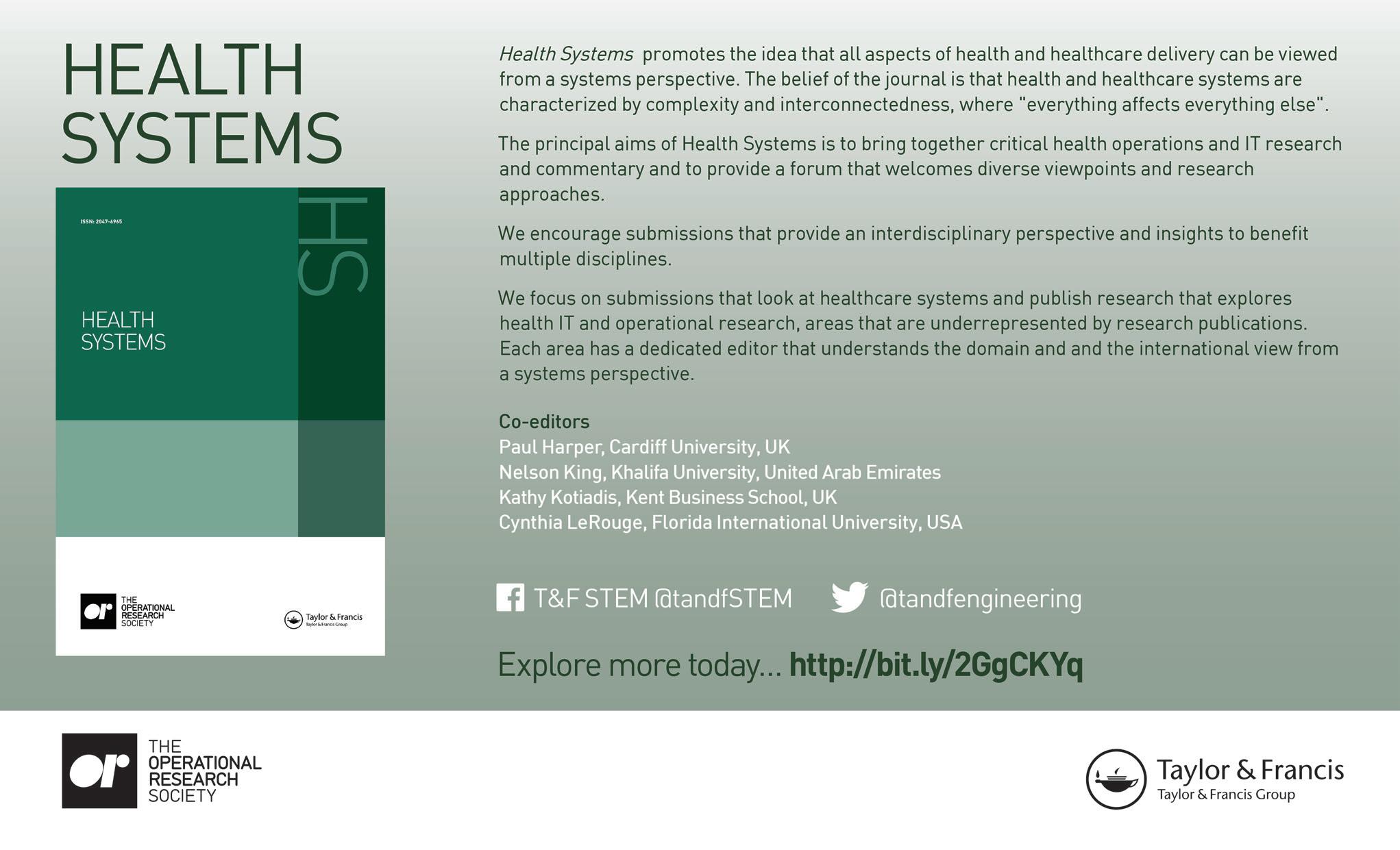
UNIVERSITIES MAKING AN IMPACT
EACH YEAR, STUDENTS ON MSC PROGRAMMES in analytical subjects at several UK universities spend their last few months undertaking a project, often for an organisation. These projects can make a significant impact. This issue features reports of projects recently carried out at two of our universities: Lancaster and Edinburgh. If you are interested in availing yourself of such an opportunity, please contact the Operational Research Society at email@theorsociety.com
TREATMENT PATHWAYS FOR PATIENTS WITH MULTIDRUG-RESISTANT TUBERCULOSIS
(Mengdi Jin, Lancaster University, MSc Business Analytics)
The Liverpool School of Tropical Medicine (LSTM) is a centre of excellence in research in the field of tropical medicine. It is the oldest institution of its kind in the world and has been at the forefront of tropical medicine since 1898. Mengdi’s project aimed to model decentralised treatment management strategies for multidrug-resistant tuberculosis (MDRTB) patients in Ethiopia. This project used data (patient and health system costs and frequency of patient assessment visits) from the STREAM Phase I trial* for which LSTM coordinated the Health Economics component.
During the project Mengdi required excellent communication skills to build high fidelity conceptual models of the MDR-TB treatment pathways. This process involved iterative discussions with her LSTM advisors to ensure the model captured the correct patient behaviour. She then translated the conceptual model into the discrete event simulation software WITNESS to run experiments comparing the cost to patients and to the health system of different treatment regimens and pathways. At the end of the project Mengdi delivered the simulation model to LSTM along with a user-friendly Excel interface which had the capability to set up and analyse the output from the model experiments.
Mengdi was selected for the project because of her strong performance within the Masters taught simulation module. Mengdi was supported through the process by supervisors from Lancaster University and LSTM but was encouraged to take ownership of her own project. From the start of the project Mengdi was very engaged and took time to learn about MDR-TB, a previously unknown area for her, before building her model. This attention to detail ultimately led to her presenting her model and findings to Liverpool’s Tuberculosis Research group (LIV-TB), which included a number of TB Clinicians and modellers, including some globally renowned experts. She received positive feedback from this presentation.
Ewan Tomeny and Laura Rosu supervised the project for LSTM. They said: ‘Mendy was a pleasure to work with. Over the course of her dissertation period she built a good model that well captured the essence of the conceptual problem, including several complexities of MDR-TB treatment. She familiarised herself well with the field of MDR-TB treatment pathways and developed a good foundational knowledge in the area. She was engaged in our project meetings, and between them, via MS Teams, always asking appropriate and insightful questions. We were particularly impressed with Mendy’s presentation given to Liverpool’s Tuberculosis Research group “LIV-TB”’.
Mendgi commented that she enjoyed working with LSTM to build a working model of a real- world process. She found joy in the project and in overcoming the challenges it presented. Going forward Mengdi, LSTM, and Lancaster University are continuing their working relationship to publish her work. This means additional model development and experimentation to gain further insights into the impact of decentralising treatment pathways for MDR-TB patients. The output of this work will be useful for decision makers, providing them with insight into the potential costs and consequences of implementing different MDR-TB treatment management pathways in Ethiopia, and potentially beyond. *STREAM Stage 1 was supported by the United States Agency for International Development through the TREAT TB Cooperative Agreement No. GHN-A-00-08-00004, with additional funding from the United Kingdom Medical Research Council and the United Kingdom Department for International Development under the MRC/DFID Concordat agreement.
ANALYSIS OF A VEHICLE ROUTING PROBLEM FOR FREIGHT DELIVERY
(Dung Tran, University of Edinburgh, MSc Business Analytics)

Optimizing logistics systems and reducing transportation and distribution costs serve as effective strategies for enterprises to enhance their competitiveness. Many companies have attempted to handle the logistics challenges by designing appropriate paths for their vehicles, which underlines the importance of routing and scheduling problems. However, their difficulty is the lack of a decision support system that can assist them in tackling the problem effectively.
That was very much the case for E. Tupling, a UK-based company that distributes heating systems and plumbing products to a group of scattered customers from the Midlands to Northern England every day. The Company also guarantees to delivery stocked products by trucks within 48hours of receiving a customer order. Business growth and customer expansion had presented a number of logistics and transportation challenges. The extra mileage involved through serving more customers has led to a considerable increase in delivery cost as well as an additional drop in profit margin.
Dung’s objective was to build models and algorithms for the Vehicle Routing Problem with Time Windows (VRPTW) in which appropriate tours are designed for multiple vehicles visiting customers during a specific time horizon, with the minimum total travel distance. In his project, the problem was analysed by two approaches: an exact algorithm that is associated with a classical mixed-integer programming (MIP) formulation and a Variable Neighbourhood Search (VNS) based heuristic. Dung also investigated the performance of multiple VNS algorithms that had been generated by modifying and utilising different elements of the general framework. All algorithms were first assessed using some benchmark instances and then applied to E. Tupling’s data set.
For the benchmark instances the MIP formulation could optimally solve some simple problems; however, the heuristic method offered better solutions with limited computational resources. The VNS proved successful for small and medium instances, and the solutions were better than, or competitive with, the best-known solutions from the literature for large instances with up to 100 customers.
In terms of the real-world application, experimental results indicated that the heuristic method was well suited for freight delivery scheduling on a daily basis. Compared to the actual schedule manually designed by E. Tupling, on a typical working day Dung’s solution reduced the total travel distance by approximately 14%. One key strength of the heuristic is that the approach generates suitable plans for truck scheduling in a timely fashion. In fact, the problem of E. Tupling with about 30 customers was solved within only 20seconds. The Company therefore will benefit from the new methodology in terms of time and financial savings.
Ross Duncanson, Commercial Director from E. Tupling, stated that Dung’s research had been an extremely useful piece of work: ‘We were proud to have been selected for a Business Analytics Dissertation Project by the University of Edinburgh Business School. One of our greatest challenges, as we grow, is the management of increased deliveries across a wider geographical area. This has been studied and thoroughly researched by Dung, and the analysis submitted to us was brilliantly insightful. We are hugely impressed by the suggested solutions and they will definitely influence our decision making in relation to transport and logistics going forward.’
The project has demonstrated the undeniable efficiency and effectiveness of Operational Research methods to assist enterprises in achieving their business goals in the best possible manner.
HEALTHCARE SYSTEMS: WHY SIMULATION OVERCOMES THE DESIGN BARRIERS
Terry Young
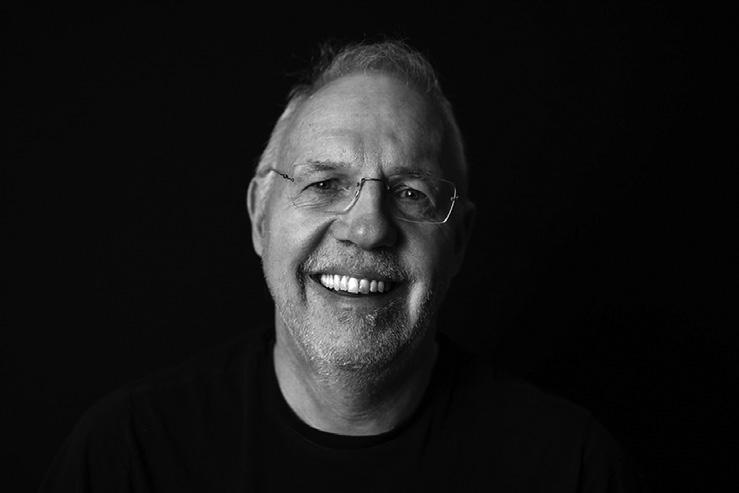
It is not difficult to find good news stories about health service improvement from modelling, or studies that led to faster service at lower cost. Some were included in the last issue of Impact in Paul Harper’s Healthy O.R. in Wales, describing his journey to close the gap between academic capability and healthcare’s needs. He notes such benefits as:
• An increasing appetite for modelling services by local
Health Boards; • Better organisational planning; • Efficiency gains worth £1.6m p.a. in one Emergency
Department.
Paul and I first collaborated in 2004. Ever since, we have each tried to fulfil our vision of putting health service design on a better footing. Here is where the quest took me.
I began my career in contract research with GEC, modelling the photonic components our research division was producing. Since hand-crafted laboratory prototypes were costly and far too small to probe accurately, computer modelling made sense. Fortunately, another GEC team modelled generators, and their world was millions of times bigger than mine: by swapping metres for microns, the graphics interfaces were quickly converted and we were soon up and running. Later, a paper on the topic won the 1989 IEE Premium, not due to novel modelling, but because it combined industrial graphics with leading-edge algorithms, and made routine design possible. Later, my appreciation of simulation was broadened as I encountered systems engineers who modelled to prototype their radar and avionics systems.
Moving in 2001 from industry, where models were ubiquitous, to academia, where modelling was a more boutique pursuit, was a shock. As in industry, however, working with those in different fields was still critical. After all, the glory of O.R. is breadth – not quite business, computing, engineering, manufacturing, or maths and certainly not quite social science, yet with roots in all.
THE CHALLENGE Paul has consistently demonstrated that models lead to better understanding of processes, as had many before him. However, the UK is not beating a path to his door to save £1.6m a year for every Emergency Department, despite urgent care having been a strategic challenge for most of this century. Why?
What would it take to convince senior health managers to model every time they design or modify a service?
In my first decade as an academic, this question led into unexpected territory: health economics. Again, I was privileged to work with some great thinkers – Martin Buxton, Alan Girling, and Richard Lilford among them – connecting business decisions to value-for money, where the UK’s National Institute for Health and Clinical Excellence, NICE, leads the world.
How does one convince hard-nosed senior managers, for whom simulation is not a natural design choice, to model in this way during design?
A collaboration between Brunel, Cambridge, Southampton and Ulster Universities in 2007–9 revealed that health O.R. lagged other sectors significantly when it came to implementation, so in 2010 a group of us set up the Cumberland Initiative to furnish the evidence and make the case for better and more robust modelling in health. Despite running Festivals of Evidence in 2014 and 2016, most of our findings were still by way of stories. It needed a different tack if we were to persuade service commissioners that each £ invested in simulation would generate or save £X later on. And we did not know what X might be.
So how much do you save or gain from every £ invested in modelling-based design?
GOOD NEWS Healthcare is unusual because epidemiologists have analysed what, in other sectors, would be termed the market. Furthermore, in health we can estimate both operational gains (e.g. greater efficiency) and health gains (where healthier individuals emerge from their care encounters).
In 2014, a collaboration with Sada Soorapanth started to unite a business view of Return on Investment (RoI) with the health economics of value for money. Our first paper, at WinterSim 2015 (see For Further Reading), analysed the financial case for improving a stroke service by modelling it. This laid the foundations: if you reach stroke patients faster you are likely to save on downstream treatment costs, so you can start to say what the modelling was worth.
However, patients who have recovered also enjoy better health – a benefit in addition to any downstream savings, so our second paper addressed the health benefits. This showed how to include health benefits in building an investment case (see For Further Reading).
In 2017–18 a sabbatical provided time to build a set of case studies from around the world to pin down modelling costs and quantify the benefits. It turned out that this was easier in the US, where the health services use modelling much more routinely. Two collaborations – one with Jim Wilkerson’s team at Memorial Health and one with David Morgareidge, led to evidence that the return can be anything from tens of times to many thousands of times the original investment in modelling. By adopting architectural and engineering perspectives rather than O.R., they are realising the same benefits. The first publication arising from these US studies has appeared in Health Systems (see For Further Reading).
Clearly, even this is not the end. For instance, we have yet to value insight, or to say what it is worth spending on a model that will convince the board to commission a new service. However, it is now possible in principle to answer the basic exam question.
NOT SUCH GOOD NEWS Perhaps the most compelling, but least popular, reason to model is the hidden cost of failure. Let’s close with the A$2bn (£1.2bn) Royal Adelaide Hospital (RAH), which opened in September 2017. My research took me to Adelaide before and after the opening, so I was interested in what was an imaginative and ambitious project.
An article in The Australian (May 15, 2018) not long after opening listed the travails of the RAH and highlighted the ramping of ambulances (queues at the hospital entrance), waits in the emergency department and general failures to sweep patients through the system smoothly and effectively. In a sad and costly development, see http://bit.ly/RAHinABCNews, administrators were appointed to run the hospital’s finances in November 2018.
The scale and scope of healthcare is now well beyond the experience or intuition of any group of people. Even when we start from scratch and design conscientiously, the options are beyond our ability to plan and deliver what we think will be needed by the time we have finished building. The nearest we can get to a crystal ball is a model of what our new facilities will look like and models of what the world around them will look like by then.
And now we can say how much it is worth spending, to stave off how much of a disaster.
With new health facilities being built all over the world, this is a reminder of how important it is to get every stage of a design fully proven ahead of opening. The health sector has tended to take a conservative view of design and migrates its designs slowly. To stay on top of burgeoning needs, however, better design processes are now needed. Simulation modelling is a proven tool for that very task.
SUMMARY Until now, the case for models in healthcare service design has relied largely on listing the benefits of good practice and citing examples of those benefits. Once we can estimate the RoI ahead of time, it is possible to say how much benefit to expect from a given amount of modelling – both operationally and in better health outcomes. Alternatively, given the improvements required, the calculation may
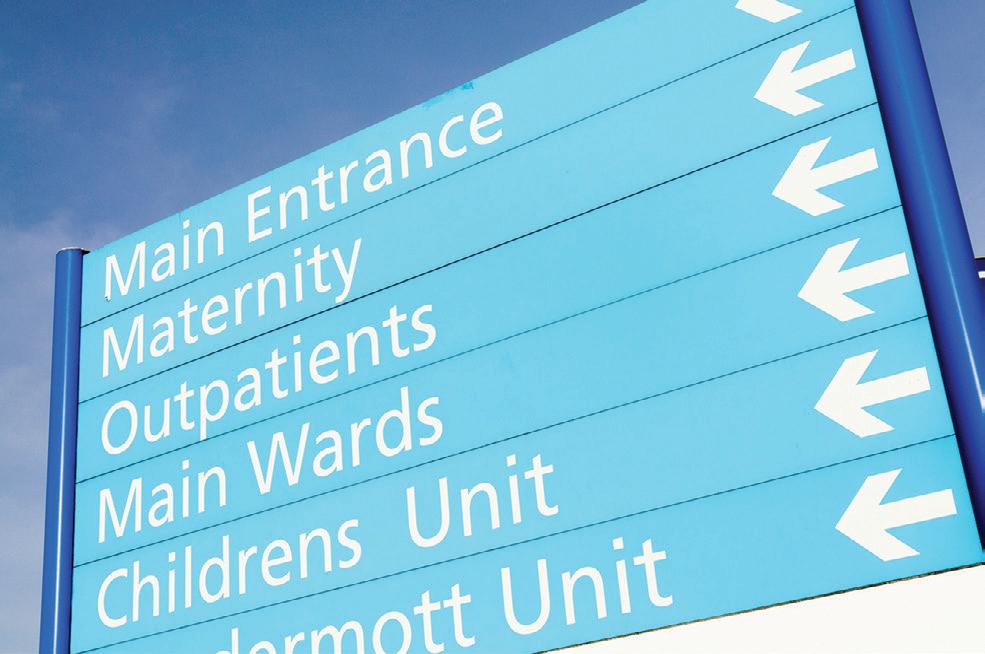
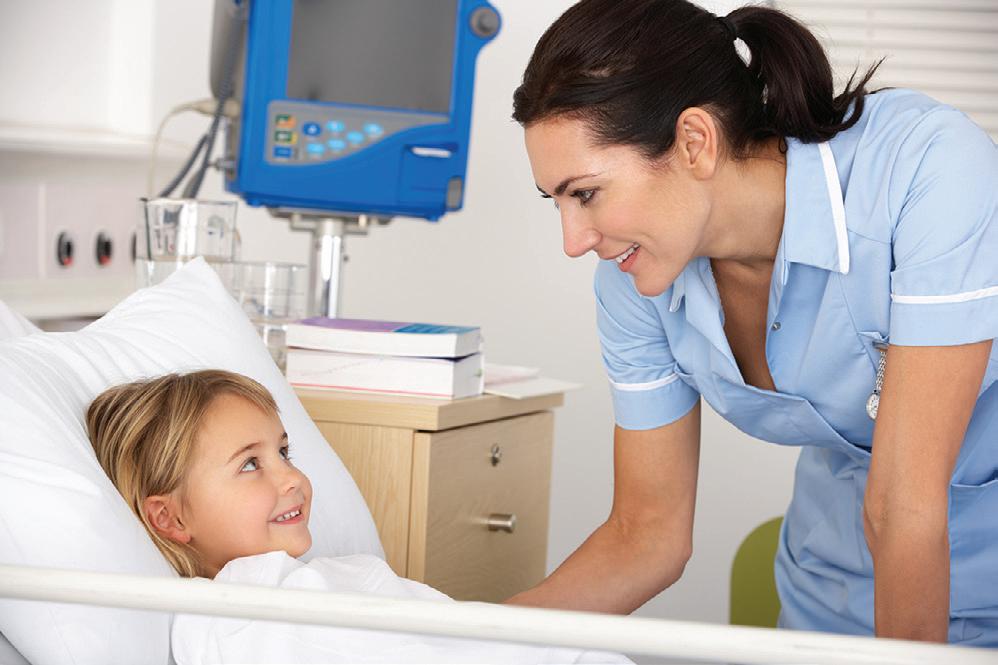
be reversed to work out how much modelling might appropriately be invested in solving the problem.
This is an important step for healthcare and also for the O.R. specialists seeking to engage with health services. Hopefully, they should find more of a welcome mat next time they go knocking.
For 16 years Terry Young designed devices and systems at GEC and Marconi and for 17 years was Professor of Healthcare Systems at Brunel where he built and led large multidisciplinary research teams in healthcare, while learning fun things about teaching and learning. He is Emeritus Professor at Brunel and Director of Datchet Consulting Ltd.
FOR FURTHER READING
Soorapanth, S. and T. Young (2015). Evaluating the financial impact of modeling and simulation in healthcare: Proposed framework with a case study. Proceedings of the 2015 Winter Simulation Conference (L. Yilmaz et al. eds.) pp. 1492–1502. Soorapanth, S. and T. Young (2019). Assessing the value of modelling and simulation in health care: An example based on increasing access to stroke treatment. Journal of the Operational Research Society, 70: 226–236. Young, T., S. Soorapanth, J. Wilkerson, L. Millburg, T. Roberts and D. Morgareidge (2020). The costs and value of modelling-based design in healthcare delivery: Five case studies from the US. Health Systems, 9: 253–262.
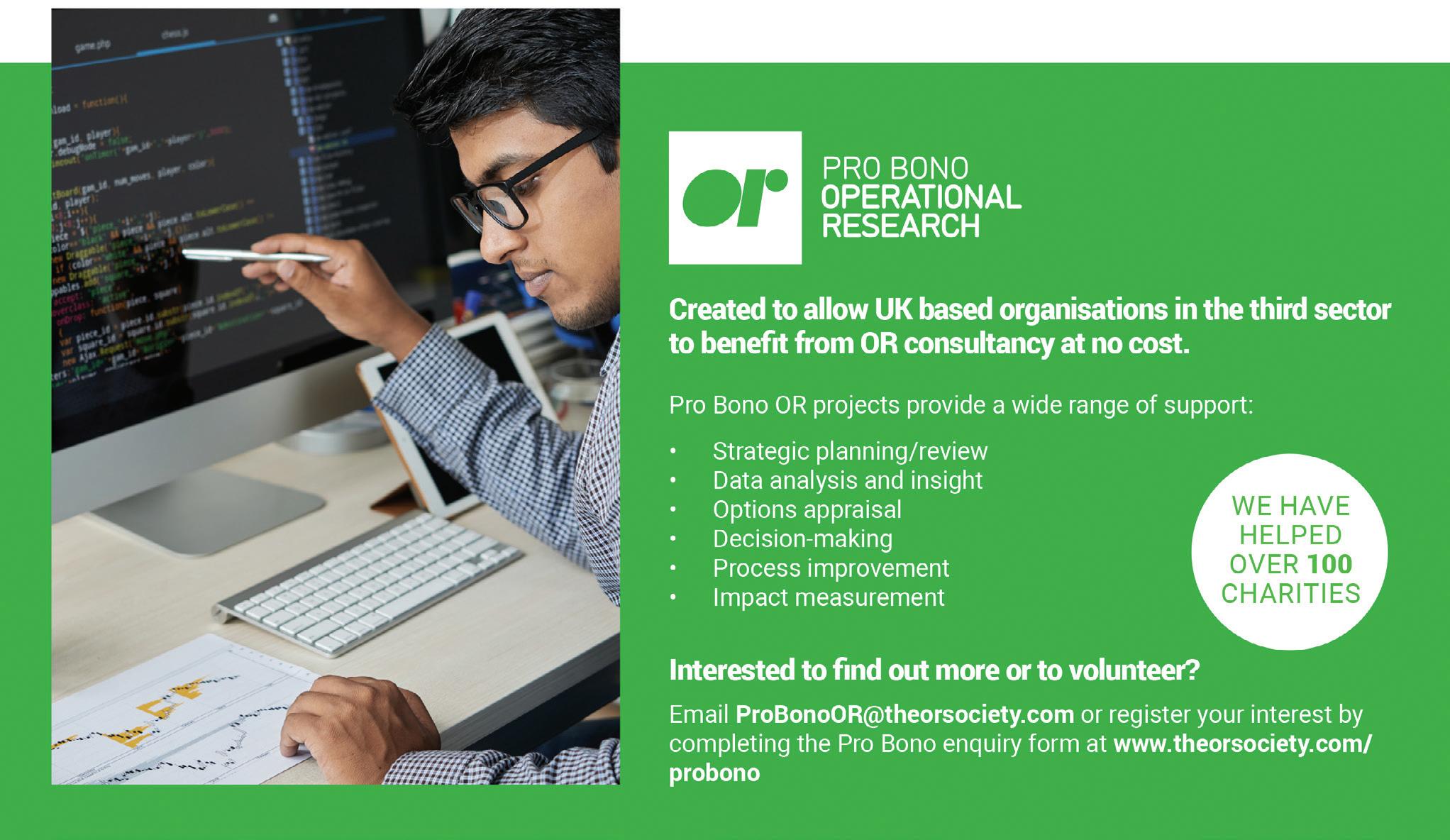