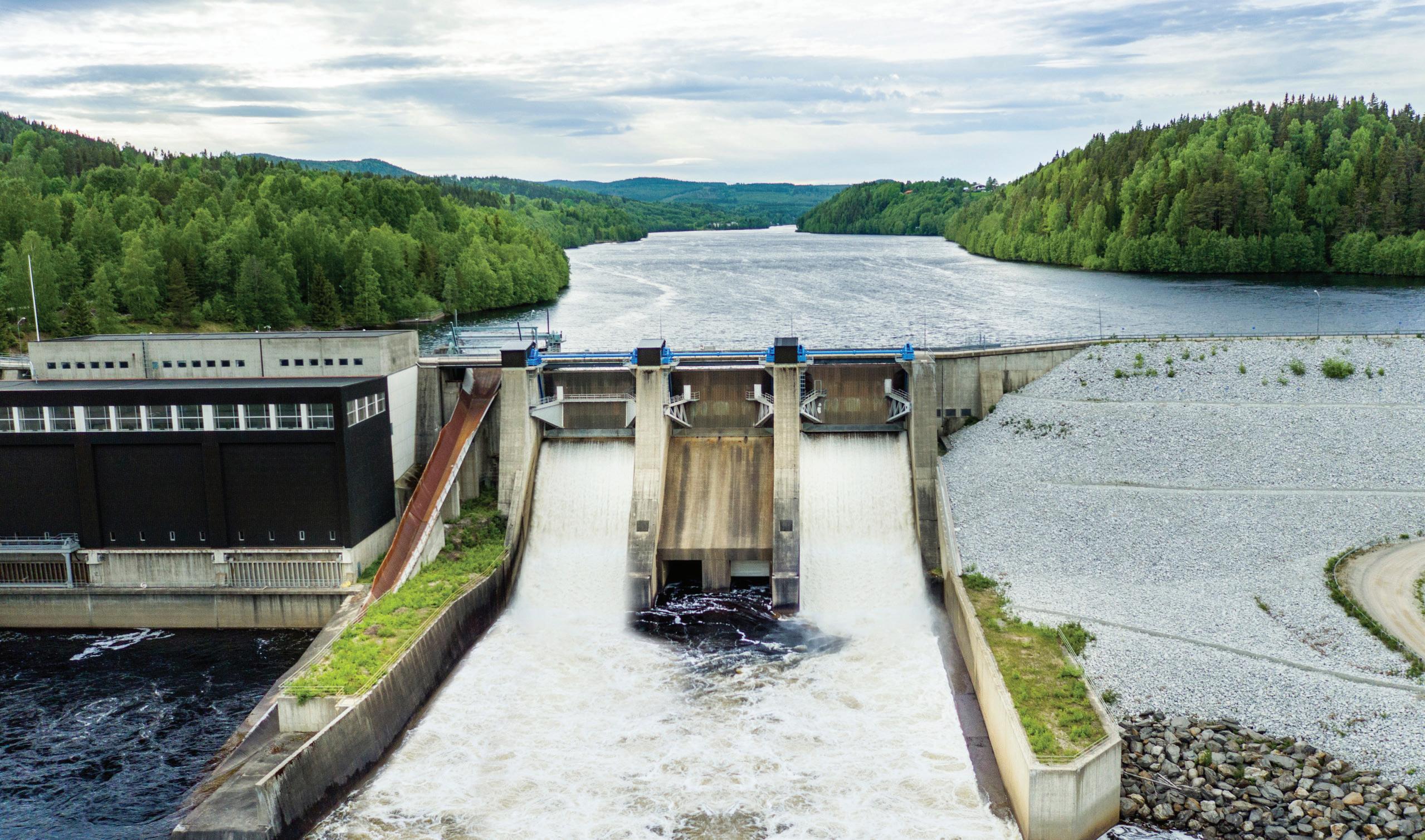
11 minute read
PORTFOLIO OPTIMISATION IN UNIPER
COLIN SILVESTER
UNIPER IS A GLOBAL ENERGY COMPANY that generates, trades, and markets energy on a large scale. Uniper also procures, stores, transports, and supplies energyrelated commodities such as natural gas, LNG, and coal. It is a major power producer in Western Europe and Russia with around 40 gigawatts of installed generating capacity with a portfolio mix of conventional and renewables assets.
In recent years, the power systems of Western Europe have been undergoing a transition towards high levels of renewable generation. Much of the new capacity is provided by wind and photovoltaics (PV). This has led to dramatic changes in how Uniper utilises its gas, hydro and coal assets within these markets. New renewable capacity reduces the amount of energy to be served by conventional generators but, as it is both variable and intermittent, conventional capacity is still required to meet demand at all times. Additionally, the reduction in conventional plant load factors reduces the supply of many ancillary services to the system operator.
The challenge of providing reliable power and grid support services whilst minimising costs and emissions means that mathematical optimisation
solutions are growing in importance within Uniper.
MARKET-BASED OPERATIONS Prior to the 21st century, most countries operated their electricity sectors through a single buyer model where a system operator would be responsible for determining the demand for electricity and then procuring generation to meet that demand. In many cases this single operator would fulfil all the roles of production, system operation and supply to customers.
Over the last two decades, market liberalisation has swept across Europe and many other countries around the world and electricity production is governed largely by market mechanisms. These changes mean that an electricity generation company has the freedom to decide if and how it operates each generating unit. The decision whether or not to run a generating unit in any particular period is based upon what is economically optimum and this changes from hour-to-hour.
A gas generator, for example, will have production costs that change from day-to-day as market prices for gas and carbon permits change on the commodities spot markets. Of course, energy companies will usually procure some or all of their gas requirements under longer term agreements and so will not need to buy gas on the volatile spot market. However, as contracted gas can be resold into the spot market, it is the spot market value that forms the basis of the determination of the economic optimum. The power produced is sold to the electricity market whose products have an hourly, or sub-hourly, basis.
Electricity generators are subject to physical constraints on starting up, the rate at which they can change load, minimum production levels, minimum on and off times and many other limitations. These mean that the economic optimum cannot be immediately calculated by a simple calculator or spreadsheet and in general they require complex optimisation algorithms. The reach of market solutions has also extended beyond the supply of electrical energy to include markets for a range of reserve and system support products to ensure both the quality and quantity of electricity supply. Electricity companies have to choose not only which generators to run and when, but also in which market channels they should be operating.
PORTFOLIO OPTIMISATION An important task for electricity companies is to carry out portfolio optimisation calculations. These have a number of roles across a range of timescales. In the short term, optimisation is required to understand which generators to run in each period. As Figure 1 shows, this is subject to a number of dynamic constraints that means generators cannot switch between maximum output and off instantaneously. As discussed above, each generator has a different threshold price for which generation is profitable and this is itself a function of the input prices of fuels and carbon obligations. This price is also affected by whether the unit must start up, as starting imposes extra costs that need to be recovered to make the running period profitable. Starting costs can be a function of the recent running history as a hot gas-fired generator requires less fuel to restart than a cold one. There are also constraints between generators as sometimes it will not be possible to start two generators at the same site simultaneously.
Beyond the short term, portfolio optimisation is required to support risk management activities, as a company will seek to strike contracts for future months and years for power sales and input fuels and carbon in order to give a more predictable financial outlook for investors. Portfolio optimisation also supports decisions about when to schedule major maintenance on plant, so as to minimise the financial impact of lost availability. Maintenance activities also can generate long term
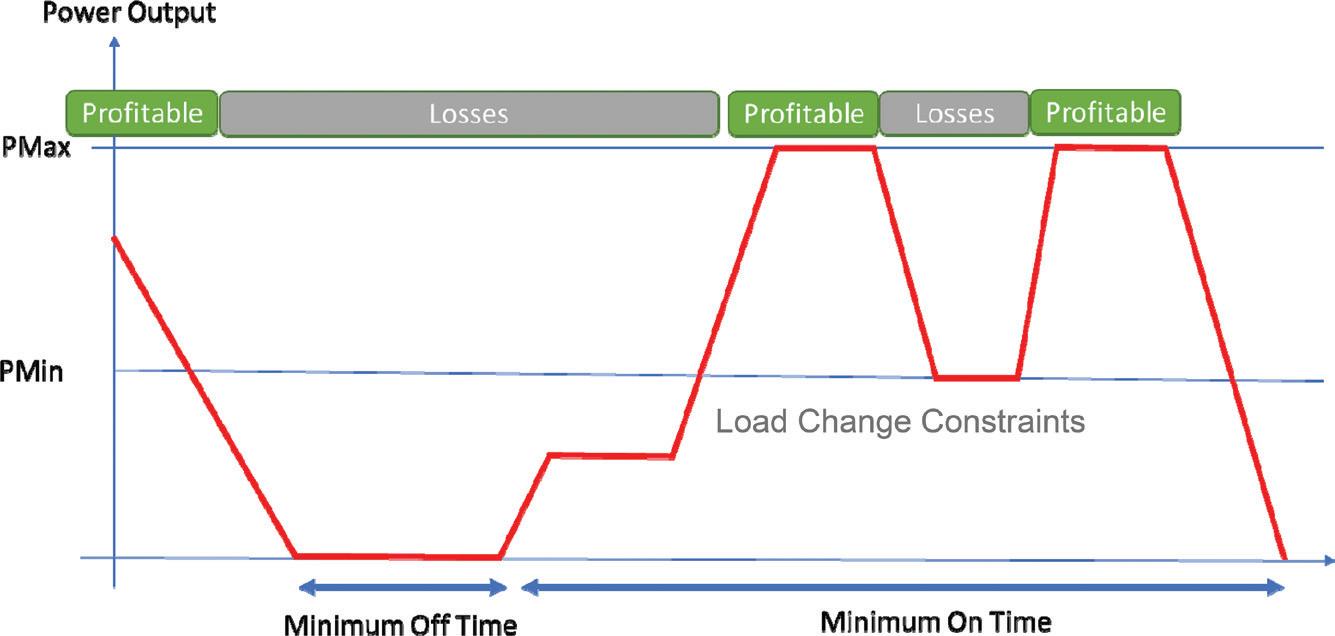
FIGURE 1 SAMPLE GENERATION PROFILE
constraints on generators as the number of starts or running hours available up to a planned maintenance may be limited. In an optimisation model, the long-term constraint can be included, and its shadow costs extracted and used as an input to the short-term optimisation process.
Uniper’s electricity assets are not limited to conventional gas, coal and oil-fired generators producing electricity alone. Uniper also plays an important role in the delivery of heat and steam to customers in Germany and the Netherlands. The generation of electricity from combustion processes naturally produces heat and so Combined Heat and Power (CHP) generation units are an attractive option to deliver this. In this case Uniper must optimise the overall delivery of customer demands for heat and steam but also the delivery of the power output either to the electricity market or an industrial customer.
This is an interesting area for optimisation modellers. Firstly, the CHP plant itself has flexibility in its mix of output of heat and power as shown by the Feasible Region in Figure 2. A CHP plant must produce a minimum amount of power before any heat can be delivered, but once this is reached then there is a high degree of flexibility in its ability to deliver heat and power. When power prices are attractive to the generator this is the best way of delivering the heat. However, in times of low, or even negative, power prices the minimum power production requirement can be costly to supply and so the optimal decision may be to run an efficient boiler instead - which can produce heat without power. The requirement to use boilers can be reduced by optimal use of a heat store. This allows the CHP to run when it is most economically attractive and produce excess heat which can be stored and used later instead of using a boiler.
HYDROELECTRIC POWER In Sweden and Germany, Uniper operates a large number of hydroelectric generators. Hydroelectric units are subject to many of the same constraints as thermal units but bring additional modelling challenges since it is essential to model reservoirs and water flows to get a realistic view of available production. Hydro units also have a strong coupling between assets as often a number of generators lie on the same river system. Production at one location results in additional water being delivered to reservoirs and generators downstream sometime later.
When modelling reservoirs, we take account of minimum and maximum surface level constraints. We also estimate inflows to each reservoir from precipitation and inflow from sources outside the scope of our optimisation such as smaller rivers and streams. We also need to take into account the flows from upstream that are a result of our optimiser’s decisions.
Hydro generation is modelled using non-linear relationships between water flow and power output and operation can be subject to flow limits, including some minimum flow limits where there may be an irrigation requirement.
The optimisation is complicated by the time taken for water to travel from one reservoir to the next and the fact that some of the reservoirs have little or no storage – meaning that the optimal production profile for the whole system can be quite different from an optimisation of each unit independently. Transfer times between reservoirs range from minutes to over twelve hours and some river systems can consist of over twenty reservoirs (each with one or several generators) spread over hundreds of kilometres. It is also the case that it may be optimal to spill water (i.e. flow more water than
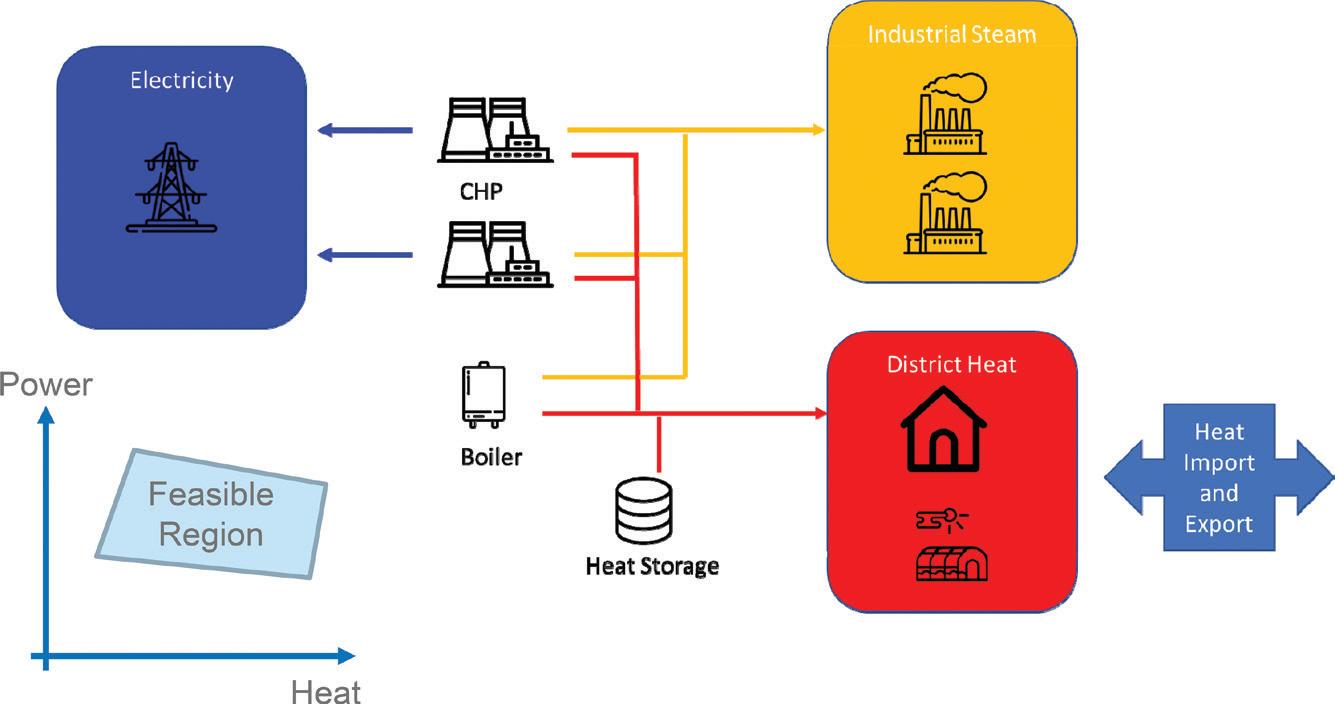
FIGURE 2 COMBINED HEAT AND POWER
is required to reach maximum output) as this can allow the water to be better used by generators downstream during high price periods.
Our goals in medium-term hydro portfolio optimisation are to forecast generator running patterns and sales of power and reserve products. In general, the hydro inflows have a seasonal pattern and the total amount of water available over the year is insufficient to allow continual production at full load. As the energy source of hydro units, i.e. water, has no purchase cost, generators can generate income by running at all periods where the price is greater than zero. However, this is suboptimal as the water will be exhausted at some point and the generators may then be unavailable to benefit from high power prices after this time. Therefore, we optimise production with the goal of capturing maximum value from limited available water. A key output of our portfolio optimisation models is the opportunity cost of water in our large reservoir storages which we call the Water Value. The water value is used in short term optimisation as a breakeven production cost which discourages the shortterm planning of production during lower price periods. Limited storage at each reservoir means that, once released from the large source reservoir, the water must pass through the entire system in a number of days – and our challenge is to do this in a way that creates the greatest value.
In Germany we also model pumped-storage hydroelectric power plants. These assets use grid electricity to pump water uphill during times of low prices and then release water through a hydro turbine at times of higher prices and so return a profit, provided that the difference between the prices overcomes the efficiency losses in the pumping process.
In addition, electricity generators can also provide reserve services. We optimise our mix of production across these products according to market prices. In the energy-only case we will normally produce at our maximum output whilst profitable (see Figure 3).
There are also products that can be delivered to supply reserve. Here we offer to the system operator the ability to turn up or turn down a running plant to help control the system. In this case the generator is offering capacity rather than delivering energy. To offer Down Reserve the generator must run at a higher level than PMin to be able to supply the down reserve and to supply Up Reserve the generator must run at a level lower than PMax. So, by choosing whether to offer reserve we will calculate whether the additional income from the reserve product plus the saved cost of production (from running at a lower level in the Up Reserve case) is greater than the lost energy income. In practice there are several reserve products traded for each period, some of which are symmetrical – meaning that generators must offer the same amount of Up Reserve as Down Reserve.
POWER PORTFOLIO OPTIMIZER Uniper has developed an optimisation application called Power Portfolio Optimizer which can carry out portfolio optimisation over planning horizons from days to as much as thirty years, taking into account power, fuel and carbon prices as well as opportunities to sell multiple
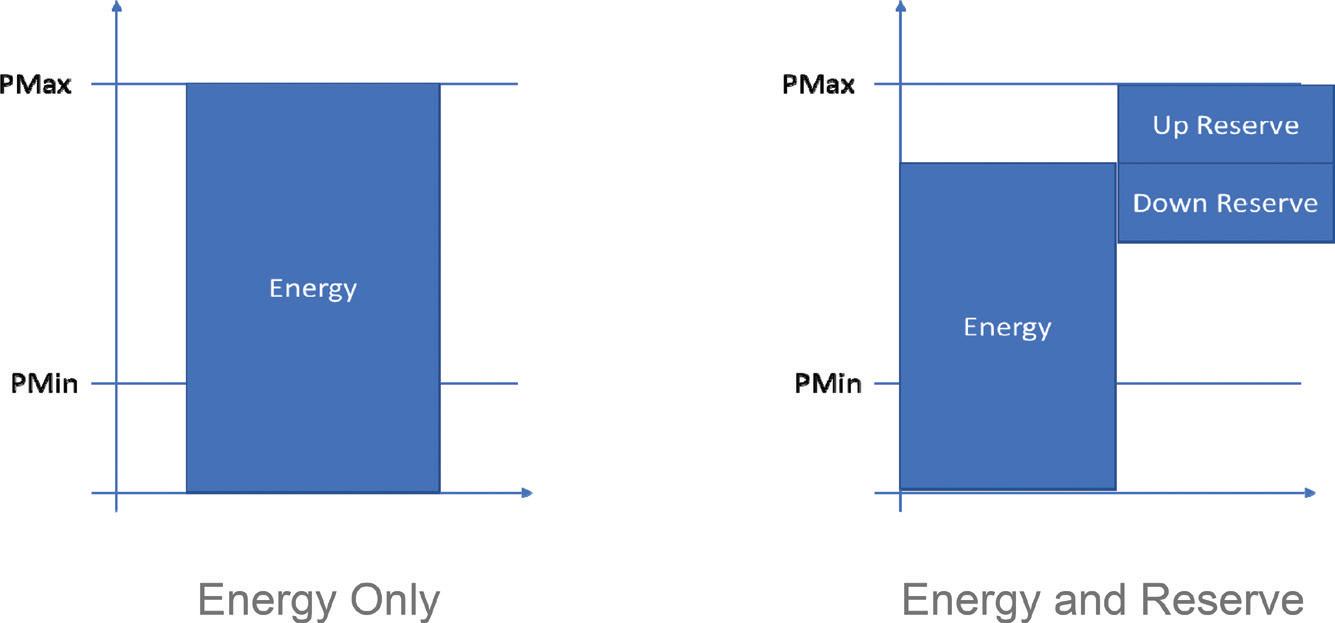
FIGURE 3 MARKET CHANNEL OPTIMISATION
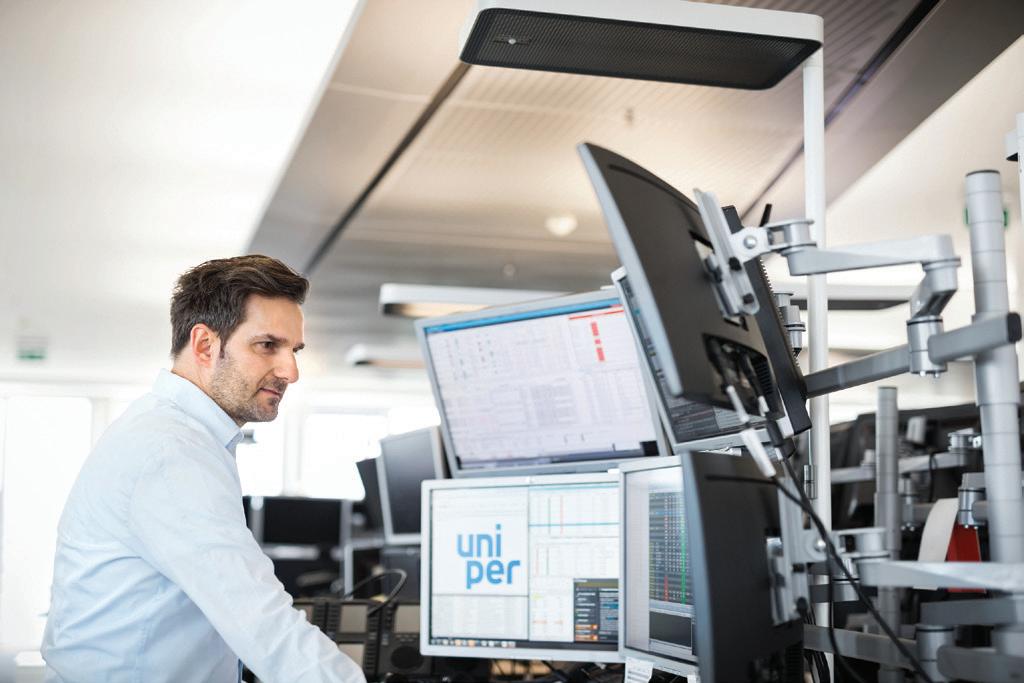
reserve products. Within a single system, based on Mixed Integer Linear Programming, Uniper carries out portfolio optimisation and asset valuation for all its European portfolios. The application also models multiple asset types and takes into account the special considerations for different assets discussed above. Power Portfolio Optimizer models Hydro and Heat Systems in addition to Gas, Coal, Biomass and Nuclear generation types.
Power Portfolio Optimizer has been used intensively for over five years for all of Uniper’s European portfolios on a daily basis and is a cornerstone of Uniper’s generation forecasting, power and fuel hedging and asset strategy activities. The application is embedded within many Uniper production systems but is also available to external customers through Uniper’s Enerlytics platform. Enerlytics offers many of Uniper’s Energy Services offerings to customers worldwide via web browsers, allowing Uniper to serve many external customers across the globe.
Maria Luisa Perez, Team lead - Asset Parameter Optimization: “Power Portfolio Optimizer makes an enormous difference to the way Uniper optimises a wide variety of market areas and technologies on a daily basis. It supports decisionmakers to extract the maximum value from our assets with a range of diverse sensitivity analyses (covering different market conditions and technical data). The key for the success of this application lies in an in-house development with optimisation and business experts working closely together - which is continuously enhanced to reflect the up to date requirements of the energy market.”
Colin Silvester is a Senior Modelling Specialist working at Uniper. He has over 20 years of experience in the energy sector. In recent years, Colin has focused on power and gas portfolio optimisation and price modelling. He has developed models that are used operationally within Uniper Global Commodities to optimise conventional, CHP and hydro assets across a wide range of timescales. Earlier experience included market modelling of liberalising markets, power-to-heat and power-to-gas evaluations and developing and running business simulation games. Colin holds a degree in Physics from Imperial College, London and is a Chartered Engineer.
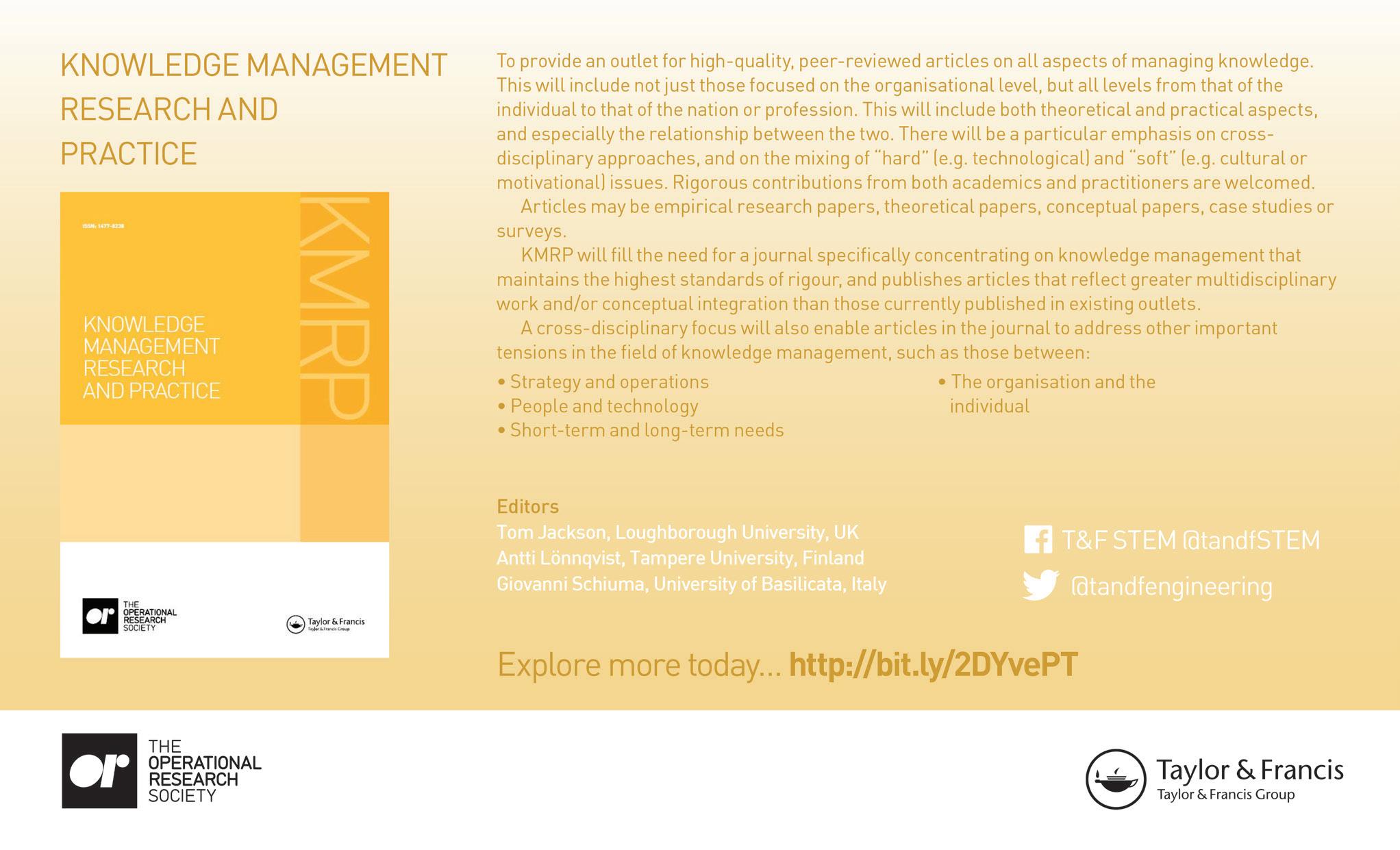