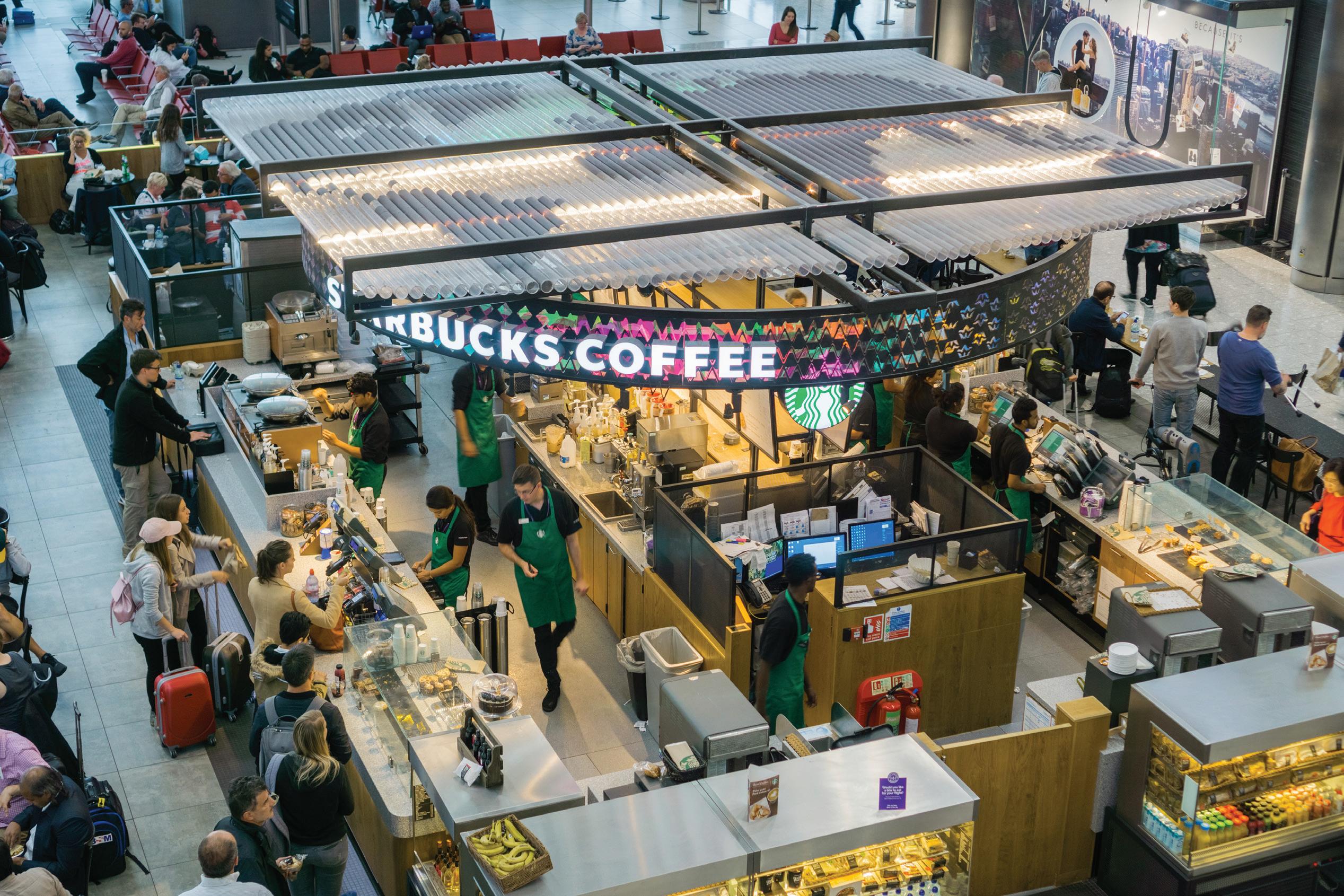
11 minute read
TERMINAL PROBLEM
NEIL ROBINSON
THANKS TO THE COVID-19 PANDEMIC, many of us might have forgotten how dizzyingly busy airports can be in normal times – and how easily late passengers can disrupt the delicate ecosystem of flight arrivals and departures. Can O.R. help solve the problem of missed connections and messed-up timetables?
Like creaking baggage carousels and glitzy duty-free shops, last calls for flights are a recognised element of an airport’s ambience. They bring comfort to those already safely ensconced at a departure gate. They provoke simmering resentment among those long since ready for take-off. They spark panic in those still desperately rummaging in their luggage at some distant passport control.
Crucially, they also indicate potential problems for managers. When last calls assume a genuine air of imminent finality – that is, when missing passengers are summoned by name – the chances are that behind the scenes, in whatever passes for an airport’s nerve centre, brows are becoming furrowed and timetables are in danger of unravelling.
This is because a breakdown in passenger flows, as they are known, is
© 2020, INFORMS. Reprinted with permission
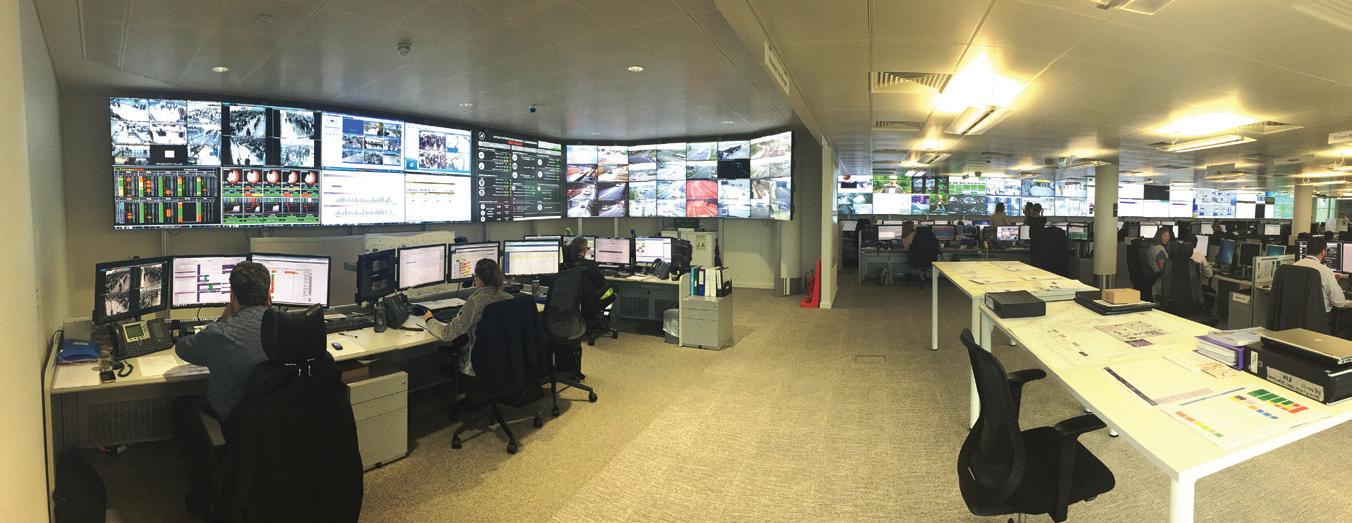
likely to lead to a delay; and a delay, in turn, could trigger ever-mounting disruption. By way of illustration, imagine that Mr John Smith is due to travel from London to New York and contrives to cause a hold-up by enjoying just one more swift gin and tonic at an ‘airside’ bar.
The consequences of this apparently insignificant act could be surprisingly far-reaching. Mr Smith’s own flight would be the obvious victim, but many others could also be affected as controllers battle to revise their priorities. It is even conceivable that what happens in London could have repercussions not only in the Big Apple but at every other destination somehow ensnared in the ensuing chaos.
Thus, one man’s sneaky G&T quickly metamorphoses into something resembling an international incident. While Mr Smith is gazing into the bottom of his tumbler and contemplating a brisk scuttle along the concourse, a web of interconnected complications is spreading all around him. This is not so much a domino effect or even a ripple effect: it is akin to a butterfly effect – a small change in initial conditions resulting in substantial differences subsequently.
One way of dealing with this issue would be to locate Mr Smith and drag him out of the bar with ample time to spare. Another approach – rather more scientific in its underpinnings and considerably more wide ranging in its impact – would be to apply the power of O.R.
GOING WITH THE FLOW Professor Bert De Reyck, director of the UCL School of Management at University College London, has an impressive track record of using O.R. to deliver improvements in transport and travel. For example, as previously featured in Impact, his work in the shipping sector – carried out with one of his former PhD students, Ioannis Fragkos, now an Associate Professor at Rotterdam School of Management – has helped the maritime industry keep pace with the demands of 21st-century global trade (see the Autumn 2016 issue).
In 2008 De Reyck and another of his former PhD students, Yael Grushka-Cockayne, now a Professor at the University of Virginia, developed a decision-making system to support the Single European Sky (SES) initiative, a scheme to integrate air traffic management across the continent. He devised a framework capable of accommodating the requirements of numerous stakeholders, allowing them to trade off objectives while taking into account both the need to act quickly and the issues associated with incomplete data.
Among the innovations to emerge from the SES were Airport Operations Centres (APOCs), the first of which was established at London’s Heathrow in 2014. An APOC brings together all key airport stakeholders in a single room, encouraging them to formulate and implement joint plans while maintaining their own respective areas of responsibility.
APOCs have since boosted collaborative operational decisionmaking through proximity and the sharing of information. Nonetheless, Eurocontrol – also known as the European Organisation for the Safety of Air Navigation – has been keen to see further progress, particularly with regard to moving beyond myopically designed legacy systems.
Commissioned to explore potential enhancements at Heathrow, De Reyck, Grushka-Cockayne and Xiaojia Guo – a third former PhD student of De Reyck and now an Assistant Professor at the University of Maryland – suggested a number of processes that might gain from the superior incorporation of advanced analytics. Of these, the management of passenger flows – especially in relation to ‘connecting’ or ‘transfer’ passengers – was deemed the most important. ‘Passengers navigating an airport often encounter delays, most notably at immigration and security’, says De Reyck. ‘Arrivals can be predicted to some extent, but there’s a lot of uncertainty around various factors – not least of which is that stakeholders have little knowledge of a passenger’s whereabouts within an airport, irrespective of whether a passenger is arriving, departing or connecting. So the task we set ourselves, in essence, was to track passengers in real time.’
CONNECTIONS AND COMPLEXITY As Europe’s busiest airport and a major international hub, Heathrow has a large proportion of connecting passengers: 32%. This would have equated to around 24 million people prior to the COVID-19 pandemic. Their transfers from one flight to another are more complex and involve more interaction than the routes taken by individuals who are merely arriving or departing.
As a member of Heathrow’s APOC team, a passenger flow manager (PFM) is chiefly reliant on volume forecasts, queuing time estimates and CCTV feeds to monitor passengers’ movements through terminals. A PFM also participates in two conference calls and two meetings each day with other APOC stakeholders.
Another vital role in smoothing connecting passengers’ journeys through the airport is played by a security flow manager (SFM). Using forecasts of passenger traffic and assessments of resource levels, an SFM aims to avoid the so-called queue breaches – incidents of passengers spending more time in a given security area than the Civil Aviation Authority recommends. Generally, an SFM is said to have presided over a breach if a passenger remains in a queue for longer than 15minutes.
Finally, taking into account worldwide air traffic and weather conditions, an aircraft flow manager (AFM) has the job of maintaining a continuous stream of planes. An AFM can request that airlines alter their schedules in light of developments and can also amend stand plans – the assignment of aircraft to gates.
‘From our discussions with the APOC team, it became clear that the PFM, the SFM and the AFM would all benefit from more timely and accurate predictions of passengers arriving at various points throughout the airport’, says De Reyck. ‘Such a predictive system would enable the PFM to resource immigration desks in advance of passenger surges, reducing the likelihood of queue breaches. It would enable the SFM to ensure the proper preparation and resourcing of security areas for incoming passengers. And it would enable the AFM to warn airlines when passengers might not make their connections, which would facilitate the offloading or expediting of passengers or the modifying of departure times.’
With these goals in mind, the researchers identified the key target variable for their study: the time
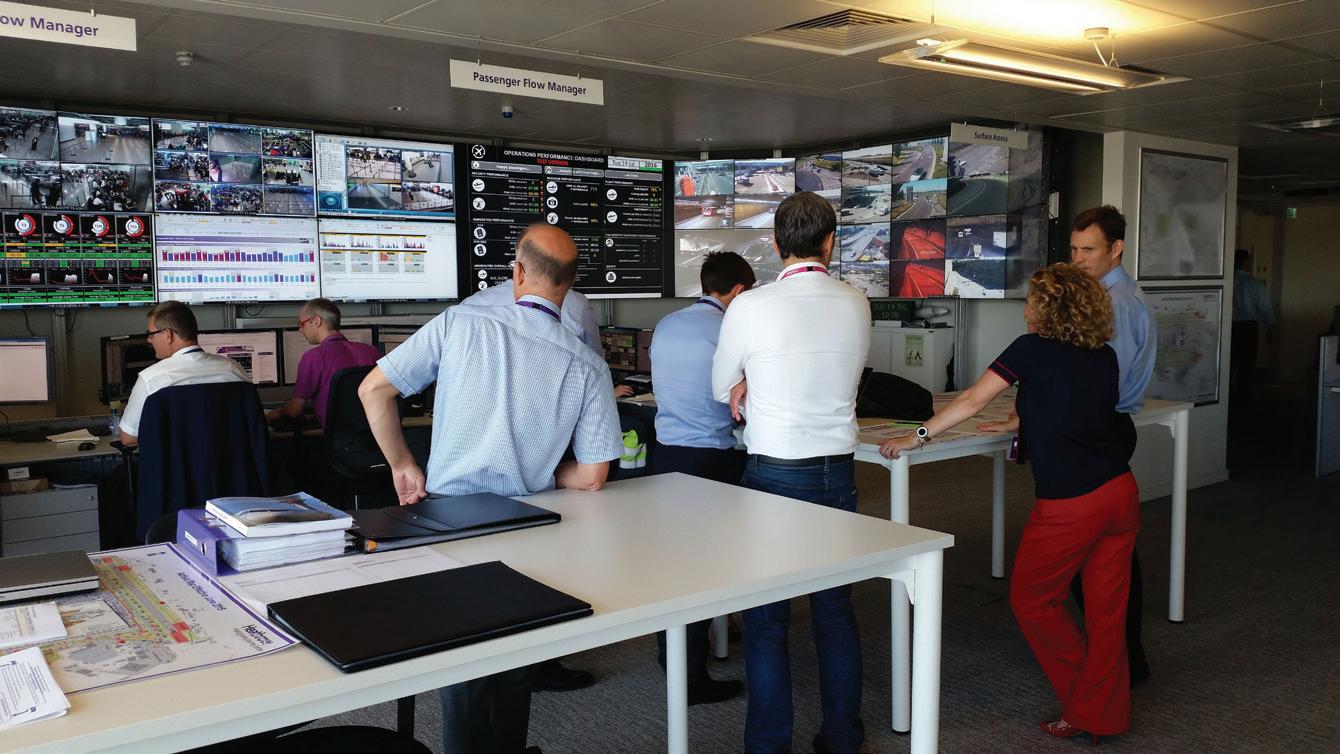
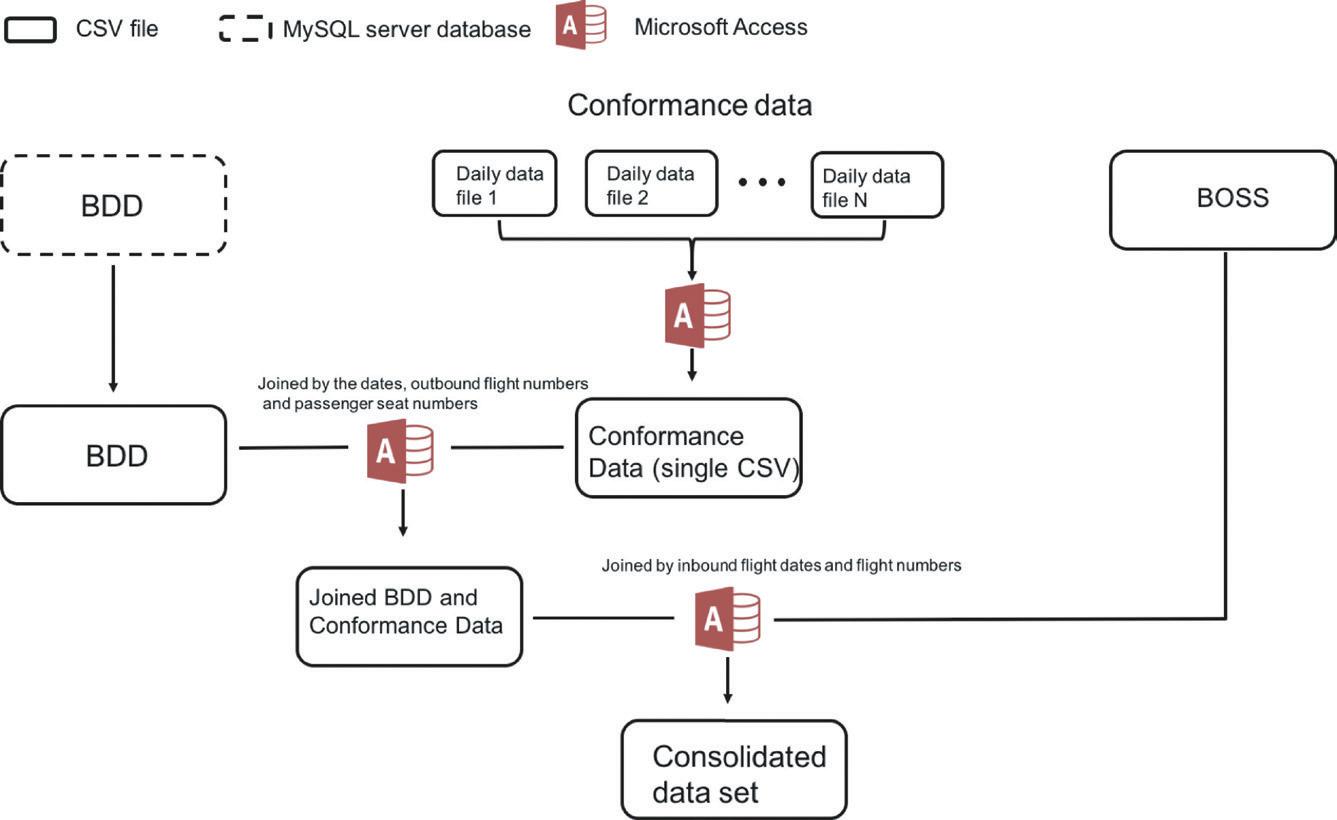
FIGURE 1 CONSOLIDATION AND MERGING OF KEY DATA Source: Guo et al. (2020) See ‘For Further Reading’. © 2020, INFORMS. Reprinted with permission
between a plane’s arrival at a gate and a passenger reaching the ‘conformance desk’, a checkpoint immediately before immigration and security. The conformance desk’s staff may deny boarding to any passenger deemed unlikely to catch a flight, but it can also be a scene of lengthy queues – itself leading to delays and missed connections.
FROM DATA TO DECISIONS The information needed for the prediction task at hand had to be consolidated from three sources: the business objective search system (BOSS), the baggage daily download (BDD) database and the conformance database. BOSS contains flight information, including arrival times and aircraft capacity; BDD details every piece of baggage belonging to passengers connecting through Heathrow; and the conformance database comprises boarding pass scans, as well as time stamps indicating when each passenger reaches the conformance desk (see Figure 1).
In seeking to train and validate their model, the researchers captured data for a whole year. Around 1% was found to be erroneous, leading to its removal. The final data set contained information related to almost 3,700,000 passengers with a mean connection time of just over half an hour.
The exercise revealed 30 variables, of which 11 were used as predictors in the final model. The remainder, which were either unavailable in real time or did not improve accuracy, were omitted. Drawing on Heathrow experts’ knowledge of the aviation sector and familiarity with connecting passengers’ journey, the researchers also constructed new variables as part of the ‘feature engineering’ necessary to build a truly effective machinelearning tool (see Figure 2).
‘Several iterations are often required when engineering novel variables and then training and validating a model’, says De Reyck. ‘We engaged in this process until we produced a model that could predict not only passengers’ connection times but also the distribution of passenger flows –
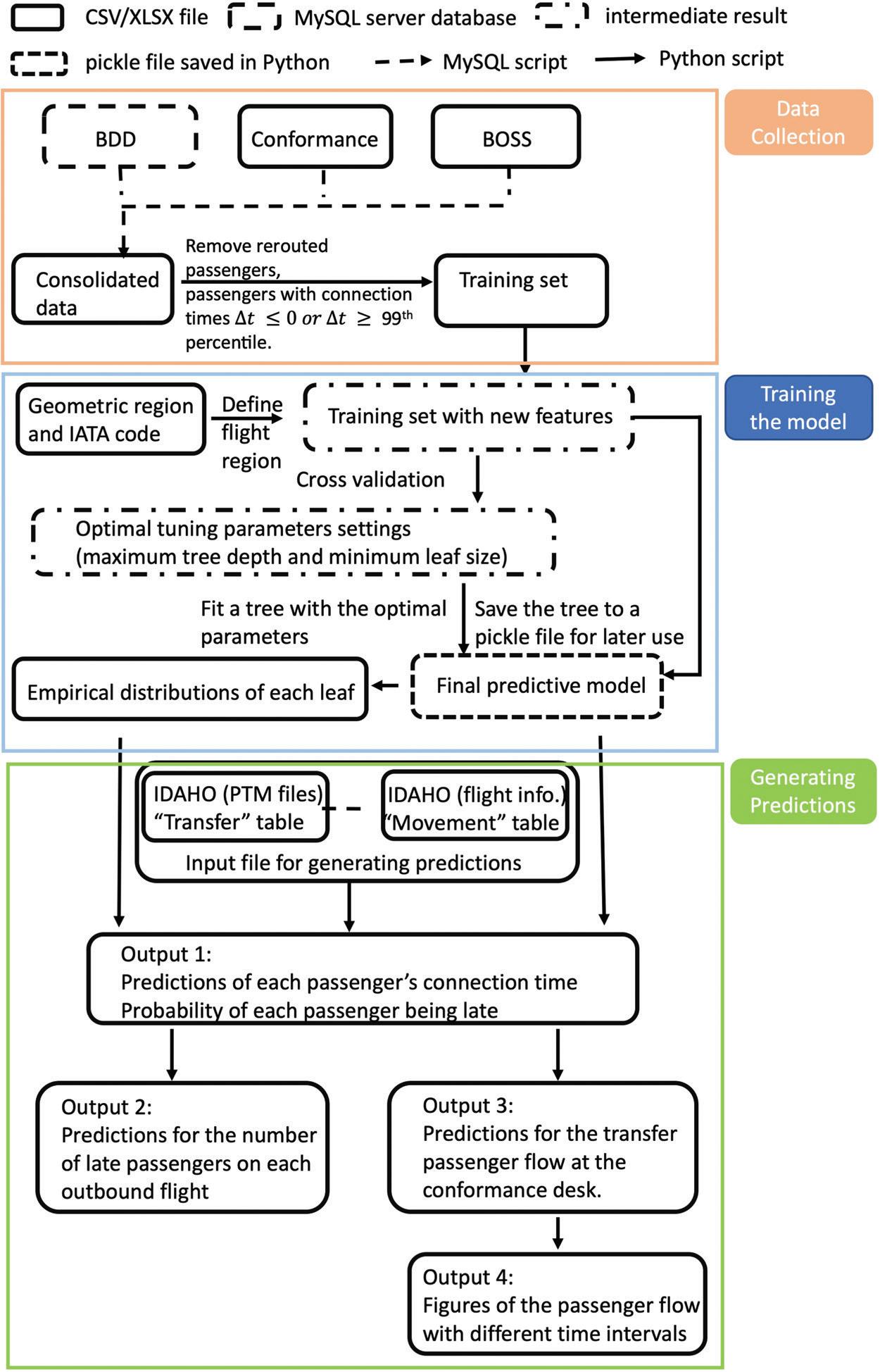
© 2020, INFORMS. Reprinted with permission
FIGURE 2 FROM DATA COLLECTION TO MODEL TRAINING TO PREDICTION GENERATION Source: Guo et al. (2020). See ‘For Further Reading’. Δt denotes the time between a plane’s arrival at a gate and a passenger reaching the conformance desk. The IDAHO system provides both flight-level and passenger-level information in real time. Flight-level information includes a flight’s scheduled and actual arrival times. Passenger-level information is extracted from PTMs (passenger transfer messages) sent by an airline and includes information about which passengers are known to be transferring to another flight. See the full journal paper for further details.
that is, the number of arrivals at the conformance desk – within specific time intervals. These two tasks are typically viewed as independent, but we used the first to calculate the second – an approach that yielded much better predictions to those traditionally achieved.’
we produced a model that could predict not only passengers’ connection times but also the distribution of passenger flows – that is, the number of arrivals at the conformance desk – within specific time intervals
The researchers eventually presented what they believed to be the most important features of their machinelearning model to Heathrow and APOC stakeholders. Tellingly, scientific rigour squared with professional experience and intuition. All concerned emphasised much the same factors. ‘This confirmed that we were very much on the right track’, says De Reyck.
The model underwent its first live trial following a further simulation study, running for an entire day. Additional testing and adaption in July and August 2017 confirmed its predictive power to be substantially greater than that of Heathrow’s legacy systems, and full implementation followed shortly afterwards.
GOING GLOBAL Now in use for over three years, the model not only provides more accurate and reliable forecasts: as expected, it also supports APOC decision-making more broadly. It assists managers’ understanding of the issues that influence connection times; it allows departures to be rescheduled in accordance with predictions, bringing more stability to airspace in general; and it guides the efficient deployment of resources.
Writing in 2019, Tom Garside, who was Heathrow’s head of integrated planning when the system was created, remarked that the model should benefit airports and airlines alike. Robert Graham, Eurocontrol’s head of airport research, has described the work carried out by De Reyck and his team as ‘groundbreaking’, ‘a reference’ and an example of ‘state-of-the-art thinking in machine learning’.
Little wonder, then, that operators around the world have taken an interest. They include Group ADP, which is responsible for France’s Charles de Gaulle, Orly and Le Bourget Airports, and managers at Singapore’s Changi Airport and Los Angeles International Airport in the USA.
Moreover, despite its huge impact on air travel, the advent of COVID-19 may have augmented rather than diminished the study’s relevance. Even before the pandemic struck, researchers at the Institute of Hygiene and Tropical Medicine in Lisbon, Portugal, began using the model to predict how passengers can spread infectious diseases – including while they are moving through airports.
‘To the best of our knowledge’, says De Reyck, ‘ours was the first system to use machine learning to model passengers flows in this setting. One of our hopes from the outset was that it could ultimately be used to support the development and implementation of other real-time, data-driven systems – and that’s precisely what has happened since.’
Countless passengers are likely to be grateful for this cutting-edge innovation when normality is finally restored to global travel. Persuading Mr Smith to abandon the bar in good time might yet prove another matter altogether, of course; but there are some problems that even O.R. cannot solve.
Neil Robinson is the managing editor of Bulletin Academic, a communications consultancy that specialises in helping academic research have the greatest economic, cultural or social impact.
FOR FURTHER READING
Guo, X., Y. Grushka-Cockayne and B. De Reyck (2020). London Heathrow Airport uses real-time analytics for improving operations. INFORMS Journal on Applied Analytics, 50: 325–339.