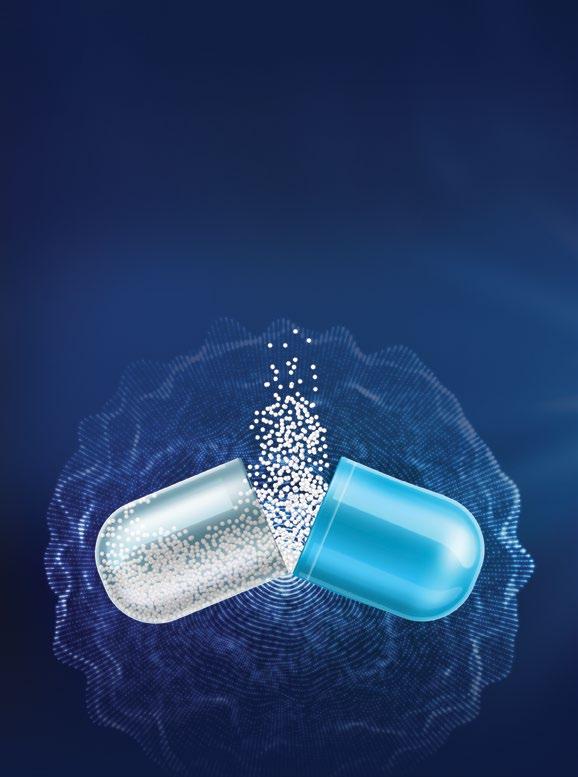




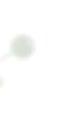

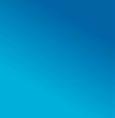
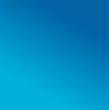

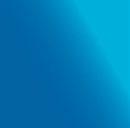




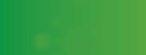


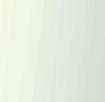
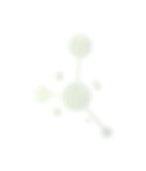
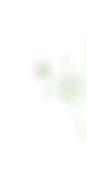
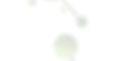

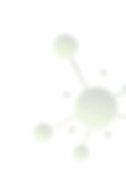
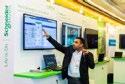

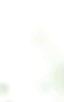

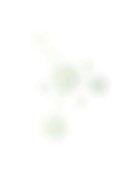


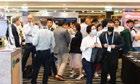
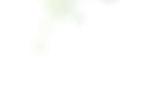
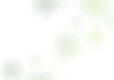





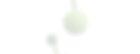
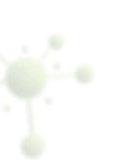

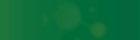




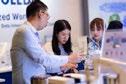
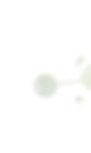




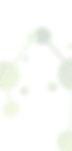
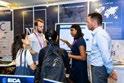
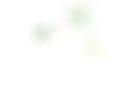

The pharmaceutical industry is on the verge of a technological revolution, catalysed by Artificial Intelligence (AI). The convergence of AI and pharma promises to reshape drug discovery, development, and delivery, ushering in an era of unprecedented innovation and efficiency. As AI evolves, its impact on the pharmaceutical sector is becoming increasingly profound, revolutionising every aspect from drug discovery to patient care.
AI's potential in the pharmaceutical sector is vast and multifaceted. Articles in this issue explore how AI-driven technologies are accelerating drug discovery processes, enhancing precision in clinical trials, and enabling personalised medicine. By harnessing vast amounts of data, AI is opening up new possibilities for understanding diseases, forecasting outcomes, and optimising treatments.
We also examine the challenges and opportunities that come with integrating AI into pharmaceutical operations. From regulatory considerations to ethical implications, the path forward requires careful navigation. Yet, the potential benefits are immense, promising not only greater efficiency and cost savings but also improved patient outcomes and a faster pace of innovation. Welcome to a new era in the pharmaceutical industry, where artificial intelligence is not merely an addition, but a pivotal game-changer.
I proudly introduce our new section ‘Pharma Pulse’ with the insights on various topics from more than one expert on each topic.I believe you will find it insightful. Please explore the same and send your valuable suggestions to prasanthi@ochre-media.com.
06 Artificial Strategy How to think strategically about AI
Brian Smith, Bocconi University, University of Hertfordshire and University College London
10 Addressing the Yearly $53B Problem for Pharma Companies using ‘Patient-on-a-chip’ Technology and Groundbreaking AI
Isaac Bentwich, CEO, Quris
13 Advancing Machine Learning and Artificial Intelligence in Pharmaceutical Manufacturing
Ajay Babu Pazhayattil, Pharmaceutical Expert
20 Predictive AI model for drug development
Manu Kumar Shetty, MBBD, MD, Maulana Azad Medical College
Prof Anubha Gupta, Department of ECE, Centre of Excellence in Healthcare, IIIT Delhi
24 Unlocking New Frontiers in Drug Discovery with Hybrid AI
Szczepan Baran, VMD, MS - Chief Science Officer VeriSIM Life
30 AI in Pharma Transforming Sample and Biomarker Data into a Force Amplifier and Decision Accelerator
Tobias Guennel, SVP, Product Innovation/Chief Architect, QuartzBio, part of Precision for Medicine
36 Predictive AI and ML Models in Drug Development
Rahul S Tade, Academic Researcher, Pharmaceutical Education and Research Institute
40 GenAI's Impact on Reshaping the Pharma Industry
Sameer Lal, SVP, Enterprise Medical Solutions, Indegene John Ward, Director, Ask GXP & ServBlock
Andree Bates, Expert, Artificial Intelligence (AI), Pharmaceuticals, and Strategic Implementation of AI
46 The AI Revolution in Pharma
Nirupama Parate, Marketing Specialist, Medicinal Products Division, Freyr Solutions
52 Artificial Intelligence Insights and outlook in precision and personalised medicine
Sumel Ashique, Mithun Bhowmick
Department of Pharmaceutical Sciences, Bengal College of Pharmaceutical Sciences & Research
56 Top Use Cases for Artificial Intelligence, Machine Learning, and Blockchain within Drug Development
Frank Leu, Founder and Managing Member, BioPharMatrix LLC
Alessio Piccoli
Lead, Sales and Business Development Activities
Europe Aragen Life Science
Andri Kusandri
Market Access and Government & Public Affairs
Director Merck Indonesia
Brian D Smith
Principal Advisor PragMedic
Gervasius Samosir Partner, YCP Solidiance, Indonesia
Hassan Mostafa Mohamed Chairman & Chief Executive Office ReyadaPro
Imelda Leslie Vargas
Regional Quality Assurance Director Zuellig Pharma
Neil J Campbell Chairman, CEO and Founder Celios Corporation, USA
Nicoleta Grecu
Director Pharmacovigilance Clinical Quality Assurance Clover Biopharmaceuticals
Nigel Cryer FRSC
Global Corporate Quality Audit Head, Sanofi Pasteur
Pramod Kashid
Senior Director, Clinical Trial Management Medpace
Quang Bui
Deputy Director at ANDA Vietnam Group Vietnam
Tamara Miller
Senior Vice President, Product Development Actinogen Medical Limited
Vivek Ahuja
Senior Vice President, Global Delivery Excellence Strategy & Growth Pharmacovigilance Quality and Regulatory Services
EDITOR
Prasanthi Sadhu
EDITORIAL TEAM
Grace Jones
Harry Callum
Rohith Nuguri
Swetha M
ART DIRECTOR
M Abdul Hannan
PRODUCT MANAGER
Jeff Kenney
SENIOR PRODUCT ASSOCIATES
Ben Johnson
David Nelson
John Milton
Peter Thomas
Sussane Vincent
PRODUCT ASSOCIATE
Veronica Wilson
CIRCULATION TEAM
Sam Smith
SUBSCRIPTIONS IN-CHARGE
Vijay Kumar Gaddam
HEAD-OPERATIONS
S V Nageswara Rao
www.pharmafocusasia.com | www.ochre-media.com
Ochre Media Private Limited Media Resource Centre,#9-1-129/1,201, 2nd Floor, Oxford Plaza, S.D Road, Secunderabad - 500003, Telangana, INDIA, Phone: +91 40 4961 4567, Fax: +91 40 4961 4555 Email: info@ochre-media.com © Ochre Media Private Limited. All rights reserved. No part of this publication may be reproduced, stored in a retrieval system or transmitted in any form or by any means, electronic, photocopying or otherwise, without prior permission of the publisher and copyright owner. Whilst every effort has been made to ensure the accuracy of the information in this publication, the publisher accepts no responsibility for errors or omissions. The products and services advertised are not endorsed by or connected with the publisher or its associates. The editorial opinions expressed in this publication are those of individual authors and not necessarily those of the publisher or of its associates.
Copies of Pharma Focus Asia can be purchased at the indicated cover prices. For bulk order reprints minimum order required is 500 copies, POA.
You can ensure optimal processes and even better medicines by prioritizing high quality, consistency and reliable GMP manufactured raw materials.
Our Benzalkonium Chloride is manufactured following cGMP guide ICH Q7 for APIs, the highest available quality standard in the industry.
Get peace of mind with:
• Full traceability
• High product purity
• Full pharmacopoeia compliance (PhEur, USP/NF, JP, ChP)
• Audit access
• Full regulatory documentation package
• Manufacturing under fully validated processes
• 30+ years of experience in cGMP manufacturing
Learn more about Benzalkonium Chloride at novonordiskpharmatech.com
Only two things are certain about AI. First, it will be possible to invest infinite resources into it. Second, success depends on investing wisely, not foolishly. Consequently, your challenge is to think strategically about AI investment and not merely tactically. This article tells you how to do that.
Brian Smith, Bocconi University, University of Hertfordshire and University College London
As our industry stands on the verge of a revolution enabled by artificial intelligence (AI), the future belongs to those who can see a world that doesn’t yet exist. But, as Neils Bohr said, it’s dangerous to make predictions, especially about the future. We can’t know, with any precision or in any detail, how AI will play out in the life sciences industry. But nor can we afford the luxury of ignoring AI or taking a “wait and see” approach. Today, every industry executive is asking what AI will mean for our sector, how to use it to gain strategic advantage and, equally, how to avoid the strategic
disadvantage by being left behind or placing the wrong bets.
Faced with this challenge, it is easy to become lost in the hype blizzard. This is true for any business but especially so for pharma, medtech and other life sciences sectors. In our industry, AI is only one change in the strategic environment among many others, from advanced therapies to the quest for health economic value. In this maelstrom of change, it is tempting to follow fashion but better to think independently. This article aims to help executives in our industry to rise above the short-term noise and think
strategically about how they can use AI to achieve their ultimate goals. It begins with identifying the three fundamental premises before identifying the four reflections that life science leaders can use to guide their firms into the future.
When we think about AI, we must begin by recognising that it is neither a homogenous nor static concept. AI is evolving rapidly and, like all new technologies, its youth is characterised by heterogeneity. At present, we can’t even agree about how to categorise different kinds of AI. At the very simplest, we talk of “weak” AI, like Siri and “strong” AI, which might in future approximate the cognitive abilities of the human brain, and “super” AI, which, if we ever achieve it, would surpass human capabilities. AI specialists bristle at this way of categorising by capability. IBM, for example, prefers to think of four different kinds of AI functionality, as shown in figure 1. This uncertainty and rapid evolution of AI means that, as we consider its impact, we must bear in mind what specific AI functionalities we’re thinking about. (Figure 1)
Although our industry is built on a continuous stream of new technological developments, most of them are narrow, specific technologies such as CRISPRCAS9 or, earlier, PCR. As impressive and important as these innovations are, their applications are mostly limited to biology and medicine. By contrast, AI falls into a different category, known as General Purpose Technology (GPT). This is a relatively select group of inventions that have the power to influence the whole economy, not just part of it. Historical examples of general purpose technologies include the steam engine, electricity and the internet.
Understanding that AI is a general purpose technology is useful because it allows us to draw two important parallels about how it will develop. First, like those earlier innovations, AI is likely to become pervasive. Much like electricity, it will not be restricted to industry niches. Second, it is not the core technology itself that will be important as much as its specialised applications,like electric motors, steam trains and the world wide web.
The general purpose nature of AI means that we can expect AI to become pervasive and ubiquitous, and, consequently, a strategic advantage will not arise simply from using AI. Instead, it is AI’s myriad specific applications that will become important, just as we saw with earlier GPTs.
As AI evolves into its many applications, it is reasonable to assume two things. First, there will be many more opportunities to spend money on AI applications than any company can afford. No company will be able to invest fully in every possible application of AI. Second, these many applications will vary not only in what they do but also in their relative costs, potential benefits and the probability of realising those benefits. So all companies, whether possessing huge or tiny AI investment resources, will find
it appropriate to invest heavily in some applications, less in others and perhaps not at all in some. In other words, every company will have choices to make about how much and where to invest in AI. Equally, that investment calculus will vary idiosyncratically between companies, so that the right pattern of choices will be different for each company. Consequently, in a world where AI is everywhere, strategic advantage will lie with those companies that make the set of AI resource allocation decisions that is most appropriate to their individual context. Conversely, strategic disadvantage will await those who make choices that may be right for others but don’t align with their own characteristic context. To put it another way, the source of competitive advantage will lie in a firm’s processes for strategic decision making.
These three premises interweave to make the job of a life science leader harder and more important. Harder because they require choices about something that is evolving and varied. Harder because they can’t copy the choices of other firms who don’t share exactly the same context. More important because, as AI becomes central to competitiveness, the gains from good strategic choices and the losses from poor ones become more salient. To rise to this hard and important challenge, our industry’s leaders might reflect on a sequence of four questions.
AI systems with no memory, designed to perform a very specific task.
Example: Netflix recommendations
AI that can understand thoughts and emotions, simulate relationships and personalise interactions
Example: Emotion AI is currently in development.
What value will AI create?
Although AI will change much, there is a strategic fundamental that it will not change. Strategic advantage will continue to flow from being significantly better at creating value for the customer (in our industry’s case, some combination of payer, patient and healthcare professionals) than our competitors. Equally, there will remain only three ways of creating value: lower costs, better products or more closely tailored products and services. This will remain as true in the AI age as it was when Porter enunciated these three “generic strategies” in the 1980s.
Central to Porter’s idea was that being significantly better at anything demands expensive, limited resources. Consequently, few firms can be significantly better than their best rivals at all three things at once. Instead, firms succeed by choosing to be research-based innovators or low-cost generics or “customer intimates”. We see obvious examples of all three choices in the global life sciences industry. We also see the failure of firms that avoid that choice.
It follows that industry leaders must be disciplined about how they intend to create value and their strategic decisions about where to invest in AI must mirror that strategy. A research-led innovator must focus its AI investment into research and development. At the same time, it will have to limit its AI investments in
AI that can recall past outcomes and monitor situations to decide on a course of action.
Example: Chat GPT
AI that has its own set of emotions, needs and beliefs as well as the ability to understand those of others.
Example: Currently theoretical
1: The four types of AI based on functionalities (Source: IBM)
manufacturing, supply chain and commercial to be merely adequate but no more. Cost leaders and customer intimates will have to make different choices that align to their different strategies.
Such strategic discipline won’t be as easy as it sounds. Every department will demand AI investment but effective industry leaders will resist the temptation to smear resources evenly across the entire value chain. They will decide what kind of superior value AI will help create—better products or better costs or better tailoring—and where AI will only maintain competitive parity. That decision will form a necessary framework for the set next of choices.
Porter’s generic strategies provide the first guide to alignment between AI investment and the rest of the firm’s focus. But it is only a rough guide because, within each of the three, there are many subsets of strategy. Within innovators, for example, these subsets include traditional blockbuster approaches, rare disease companies and advanced therapies supported by companion diagnostics. In my own research I've identified no less than 26 distinct business models in the life sciences industry.
This speciation of business models is important because whilst they share some capabilities, each is characterised by its own distinctive capabilities. For example, a primary care blockbuster model needs value demonstration capabilities in a way that a rare disease model does not. Similarly, some advanced therapy models in oncology require bioinformatics capabilities that are much less important to a branded generics model, which might rely on extracting insight from purchasing behaviours. The business-model-specific capabilities are also affected by the firm’s other strategic choices. For example, innovative firms differ in which research and development activities are in-house and which are outsourced, what technologies to pursue and what therapy areas to address. All of the choices influence
The challenge for our industry is to think strategically about AI. To do this, begin with the three fundamental premises in this article and then ask the four strategic questions every firm must answer.
what capabilities are most important to the firm’s competitiveness.
It follows that strategic investment in AI will have the most impact where it enhances those capabilities that are critical to, and characteristic of, the firm’s business model. To invest in outsourced activities or those capabilities that are not characteristic of the firm’s business model would risk under-resourcing the capabilities that most strongly influence its competitiveness. In practice, any life science business model requires a wide range of capabilities but, whilst all have to be at least adequate, only a few of them need to be distinctively superior (relative to the competitors) to ensure the success of the business model. Identifying these few would guide senior leaders to a more precise choice of AI investments. For instance, different innovator firms might allocate resources differently between AI for protein folding, for clinical trials data or for real-world value analysis, depending on exactly what their capabilities were critical to their business model’s effectiveness.
In essence then, appropriate decisions about AI depend on deciding what capabilities are the most important to the firm’s model. This again is difficult when no capability is unnecessary and every individual or department regards the capabilities they foster as the most important. But effective leaders will be able to separate essential but not distinguishing capabilities from those that are both essential and distinguishing. Having
used that categorisation to make specific AI investment decisions, they will be faced with the task of implementing them.
To adapt a Churchillian quote, the choice of what AI to invest in is not the end, or even the beginning of the end, but it is perhaps the end of the beginning. Industry executives must then make those decisions pay off. The difficulty of this is captured in what is known as the productivity paradox, observed during the 1980s’ introduction of information technology to business. At that time, productivity was seen to decline as firms computerised. As Nobel laureate Robert Solow observed in 1987: “You can see the computer age everywhere but in the productivity statistics”. As we now know, this phenomenon did not persist and, eventually, productivity rose rapidly. This pattern of investment leading to decreased productivity for a period, followed by rapidly increasing productivity, was christened the productivity J-curve by Erik Brynjolfsson and his colleagues at the Stanford Institute of AI.
The explanation of the J curve can be found in the parallels with earlier GPTs. The impact of GPTs doesn’t follow a linear path because they often require the adaptation of other parts of the economy to become fully effective, as we saw with the laying of railway lines, the installation of the electric grid and the development of fast internet connectivity. This leads to a short-term dip in economic productivity before the growth created by the innovation is felt. The length and depth of this dip depends on how well the economy or an organisation adapts around the GPT. If that adaptation involves simply replacing old methods with upgraded versions of the same methods and processes, productivity growth is less and longer delayed than if newer, better methods and processes are adopted. For example, the full benefits of both steam and electric power were not achieved by augmenting the existing water mills and small carriages but by
building large factories, with big, centralised power stations and long trains pulled by dedicated steam locomotives.
Applying the lessons of the J curve to the life sciences industry implies that its leaders reflect carefully on how to adapt their organisations to fully realise the benefits of AI. Minor adaptations of, for example, clinical trials to improve patient selection and recruitment, may produce a longer, deeper productivity dip than transforming trials with so called “digital twins”. In the same way, applying AI to analyse real world cost and outcome data may produce a shorter, shallower J shaped curve than using AI to improve existing health economic models based on limited trial data.
For life science leaders, the productivity paradox and the J shaped curve mean that their strategic decisions are not limited to AI and must include complementary capabilities, processes and activities that will enable a short, shallow J curve leading into a steep rise in productivity. These decisions will be hard to implement, especially in large companies with entrenched functional silos that regard information technology as a supporting, subordinate activity. But effective leaders will recognise capabilities and processes as cross-functional. They will make investments and changes across functional boundaries to achieve the goals of the business rather than the objectives of departments. Having done so, they might expect to successfully implement AI. But they will still need to avoid the Turing Trap.
Uniquely, the life sciences industry exists because of its implicit contract with society. Although rarely discussed, this arrangement has been in place for decades and has been enormously beneficial for both the industry and wider society. Under the unspoken terms of this social contract, society funds basic research and provides the highly educated personnel the industry requires. It also creates markets for its innovative but expensive products, through public and
subsidised private insurance systems. Importantly, it also creates temporary market exclusivity, through intellectual property rights and regulatory conditions, which enables necessary return on investment. In return for these accommodations, the industry undertakes to, and mostly does, provide innovative products that extend, improve and save lives and that, eventually, commoditise into a stream of good and inexpensive generic products. This arrangement also has valuable by-products of many wellpaid jobs and a strong contribution to the overall economy.
This social contract exists only with society’s agreement. It is vulnerable to how the industry is perceived by the electorate and how governments respond to those views. If, as we anticipate, AI has a large impact on the industry, then we would be naïve not to anticipate unintended consequences that might threaten this essential but fragile arrangement.
The most obvious risk is “Turing’s Trap”, another concept of Erik Brynjolfsson. Simplified and translated into the industry’s socially contracted context, the Turing Trap says that if AI is simply used to replace people, cut costs and improve profits and these benefits are not shared equitably between society and the industry, then society may decide the contract is no longer a fair one. If, for example, AI makes new medicines and technology better and cheaper to make but no less expensive to buy, society may begin to question the contract. And if AI reduces effective competition, allows exclusivity to be extended indefinitely and destroys skilled jobs, then society, through policy and legislation, may decide not to fulfil its part of the social contract. That would be an unintended consequence of huge, negative significance for both the industry and society.
Given the importance of the social contract to the industry, the Turing Trap is an existential threat not only to individual companies but to the industry. It will not be easy for profit-driven, shareholder owned, companies to balance the distribution of the benefits of AI. But
effective industry leaders will recognise that the long term survival of the industry requires them to avoid the trap and not place the social contract at risk. They will avoid AI’s unintended consequences by sharing its benefits with society.
Our industry is living in interesting times. As if the dramatic changes in its scientific and sociological environments were not challenging enough, its leaders and senior executives must anticipate and respond to the impact of AI. The industry’s media and consultants are keen to offer clear, simple answers. But as HL Mencken famously said, every complex problem has an answer that is clear, simple and wrong. The challenge for our industry is to avoid simplistic thinking and to think strategically about AI. To do this, it helps to begin with the three premises in this article. AI is evolving and heterogeneous. It will be pervasive in its applications. It will demand strategic choices. Those responsible for making strategic choices about AI will make better decisions if they reflect on the four questions in this article. Good choices will align AI investment with core strategy. They will enable the capabilities that most distinguish the firm’s business model. They will shape the J curve and limit the productivity paradox. They will avoid the Turing Trap. If they reflect this way, they will do what they must do regarding AI: they will think and then behave strategically.
and www.pragmedic.com
Quris pioneers a groundbreaking Bio-AI platform, merging advanced AI with innovative biology to revolutionise drug discovery. Partnering with major pharma companies like Merck, its patient-on-a-chip technology and real-time nano-sensing precisely predict drug safety, aligning with modern legislative changes and setting new standards in pharmaceutical innovation and clinical safety prediction.
Isaac Bentwich, CEO, QurisDrug development stands at a pivotal juncture, struggling with the challenges of slow progress and soaring costs. With an average investment exceeding US$2.6 billion and a timeline stretching over 12-15 years per drug, bringing new medicine to market is fraught with many financial and timely hurdles. However, at the heart of this challenge lies a fundamental dilemma:
the inability to predict which drug candidates will prove safe and effective for the human body.
The gap between preclinical testing and human trials remains a major hurdle in drug development. Notably, the high failure rate in clinical trials (around 92 per cent) suggests limitations in current methods, particularly considering the effectiveness of animal testing. This inefficiency can be costly, with estimates suggesting upwards of US$60 billion lost annually in failed oncology trials alone.
For decades, the pharmaceutical industry has heavily relied on animal testing as a means of predicting clinical outcomes. Yet, despite its widespread use, animal testing has proven to be an unreliable indicator of human response. Studies have revealed an alarming fact - nearly 89 per cent of drug candidates that successfully navigate animal testing fail to deliver the expected results in human clinical trials. This unfortunate reality emphasises the urgent need for innovative approaches to drug development.
Fortunately, recent regulatory changes have set the stage for a paradigm shift in drug development practices. The FDA Modernization Act 2.0, enacted in 2022, represent a landmark departure from the reliance on antiquated animal studies. Instead, the focus has shifted towards embracing modern, more effective methodologies that hold the promise of revolutionising drug development for the better good.
At the forefront of this revolution are several disruptive technologies that offer new approaches to tackling the clinical prediction challenge. Chief among them is machine learning (ML), a field that has witnessed remarkable advancements in recent years. ML algorithms have demonstrated remarkable capabilities in various facets of drug discovery and development, from identifying novel drug targets to predicting the safety profiles of candidate compounds.
With an average investment exceeding US$2.6 billion and a timeline stretching over 12-15 years per drug, bringing new medicine to market is fraught with many financial and timely hurdles.
One of the strengths of ML lies in its ability to analyse vast amounts of data and recognise complex patterns that may escape human perception. Supervised, unsupervised, and reinforcement learning algorithms are deployed to sift through massive datasets, extracting valuable insights that inform decision-making processes at every stage of drug development.
Traditionally, new drugs were first tested in animals. However, this doesn't always translate perfectly to how a drug will work in humans. Organoids and organ-on-chip technology are essentially miniaturised, simplified versions of human organs grown in a lab. These tiny organs offer a more realistic testing ground for potential drugs, allowing scientists to see how they might be absorbed, processed, and affect the body in a way that's closer to what happens in humans. This can help to identify potential problems earlier and avoid wasting time and resources on drugs that might not be effective or safe.
Stem cells are the body's master builders, with the potential to develop into many different cell types. This exciting field of science is leading to the development of patient-specific drug testing models. By
using stem cells from individual patients, scientists can create miniaturised versions of their organs. This allows for a more personalised approach to drug development, where researchers can see how a drug might affect a specific patient's unique biology. This holds promise for the future of personalised medicine, where treatments can be tailored to each individual's needs.
Imagine being able to watch a drug interact with cells in real-time. That's the potential of nano-sensing technologies. These tiny sensors can be used to monitor how cells respond to drug treatment, giving scientists a much clearer picture of how a drug is working and its potential effects. This real-time data can help researchers make better decisions about which drugs to pursue and how to optimise their effectiveness.
At the nexus of these disruptive technologies lies the Bio-AI safety prediction approach, a novel framework that integrates the power of machine learning with high-throughput experimentation on patient-on-chip platforms. This approach represents a paradigm shift in drug development, moving away from traditional animal testing models towards more accurate and personalised methodologies.
Central to the Bio-AI approach is the concept of high-throughput experimentation, enabled by patient-on-chip platforms that replicate the complexity of human physiology in vitro. These platforms allow researchers to conduct thousands of experiments simultaneously, generating vast amounts of data on drug responses across diverse patient populations.
ML algorithms are then deployed to analyse this data, identifying patterns and correlations that can predict drug safety and efficacy with unprecedented accuracy. By leveraging the power of ML, researchers can uncover subtle
relationships between drug properties and biological responses, leading to more informed decision-making in drug development.
Real-time sensing technologies further enhance the predictive capabilities of the Bio-AI approach by providing continuous monitoring of cellular responses to drug treatments. By capturing dynamic changes in metabolite levels and biomarker expression, these technologies offer valuable insights into drug mechanisms of action and potential adverse effects.
Stem cell genomic diversity plays a crucial role in the Bio-AI approach, allowing researchers to test drug responses across genetically diverse patient populations. By generating patient-specific stem cell models, researchers can simulate the variability observed in human populations, enabling more accurate drug safety and efficacy predictions.
The Bio-AI approach holds immense promise for revolutionising drug development, offering a more efficient, costeffective, and personalised alternative to traditional methodologies. By harnessing the power of machine learning, highthroughput experimentation, and realtime sensing, researchers can overcome the limitations of animal testing and accelerate the pace of drug discovery.
Moreover, the Bio-AI approach could potentially transform clinical trial design by enabling more precise patient stratification and selection. By leveraging patient-specific models, researchers can identify subpopulations that are most likely to benefit from a given treatment, thereby improving clinical trial outcomes and reducing the likelihood of trial failures.
The implications of the technology are substantial – it doesn't just potentially save drug developers considerable resources by mitigating the risk of a 90 per cent clinical trial failure rate, it also expands the realm of possible
treatments, benefiting patients with all sorts of conditions, including those suffering from rare diseases, which are often marginalised due to financial and trial limitations. Advanced Bio-AI platforms have proven the ability to simulate clinical trials and a real human body’s reaction to drugs to effectively predict drug toxicity by leveraging a patented organ-on-chip system through stemcell-derived tissue and AI. Bio-AI can better predict which drug candidates will safely work in humans—avoiding the tremendous risks and costs of failed clinical trials, ending the reliance on ineffective animal testing, and accelerating speed to market.
Despite its transformative potential, the widespread adoption of the Bio-AI approach faces several challenges. Foremost is the need for robust validation and standardisation of patient-onchip platforms and machine-learning algorithms. Ensuring the reliability and reproducibility of experimental results will be essential for building trust in the predictive capabilities of this approach.
Furthermore, integrating these technologies poses logistical and technical challenges that must be addressed. From optimising experimental protocols to developing interoperable data analysis pipelines, researchers must overcome numerous hurdles to realise the full potential of the Bio-AI approach.
However, with these challenges come unprecedented opportunities for innovation and collaboration. By bringing together experts from diverse fields, including biology, engineering, and computer science, researchers can leverage their collective expertise to overcome barriers and drive progress in drug development.
The Bio-AI approach represents a paradigm shift in drug development, offering a more efficient, predictive, and personalised alternative to tradi -
tional methodologies. By integrating disruptive technologies such as machine learning, high-throughput experimentation, and real-time sensing, researchers can overcome the limitations of animal testing and accelerate the pace of drug discovery.
Moreover, the Bio-AI approach holds promise for transforming clinical trial design, advancing our understanding of disease mechanisms, and improving patient outcomes. While challenges remain, the potential benefits of this approach are too significant to ignore, making it a compelling avenue for future research and innovation in drug development.
For more information about the Bio-AI technology, visit www.quris.ai
The article explores the transformative impact of artificial intelligence (AI) in pharmaceutical manufacturing, from process parameter monitoring to supply chain resilience. AI and machine learning drive efficiency, compliance, and innovation. The emphasis for today, however, needs to be on finding data science talent, developing learning tools, creating data collaboration platforms, management commitment, and readying the organisation to ensure a seamless adoption.
Ajay Babu Pazhayattil, Pharmaceutical ExpertThe pharmaceutical industry is undergoing a significant transformation by adapting machine learning (ML) and artificial intelligence (AI) technologies. This integration is poised to revolutionise various aspects of pharmaceutical manufacturing, from drug discovery to supply chain optimisa-
tion and regulatory compliance. ML and AI offer unprecedented opportunities to enhance operational efficiency and quality in pharmaceutical manufacturing. By analysing vast datasets and identifying patterns, these technologies accelerate response times, predict deviations, and aid in assuring process controls. ML and
AI algorithms support product quality, which is paramount in the pharmaceutical industry. As the industry embraces AI technologies, collaborative efforts, knowledge-sharing, and industry-wide standards are vital for driving innovation and propelling the industry toward a more efficient and competitive future. AI has become indispensable in the evolving landscape of pharmaceutical manufacturing.
Continued process verification (CPV) represents the third Stage in the pharmaceutical process validation life cycle. CPV's primary goal is to identify process variability, pinpoint areas for enhanced performance, decrease variability, and refine process controls. The implementation of CPV is a regulatory expectation and can provide benefits beyond compliance by improving production processes and ensuring the reliability of drug quality and supply. AI is being progressively utilised for the acquisition, storage, and surveillance of extensive manufacturing datasets, aiming to decipher process variability and its underlying causes. AI demonstrates the capacity to expedite response times to signals,
propose actionable steps, and streamline data presentation by highlighting pivotal factors that reveal the intrinsic correlation between outcomes and data. Additionally, AI enhances the precision of predicting deviations through advanced machine learning algorithms, conducts comprehensive root cause analyses, and upholds data integrity and compliance in predictions and root cause analyses. Nevertheless, successful implementation necessitates a strategic approach encompassing algorithm qualification and adherence to regulatory mandates. Compliance with regulatory standards is a critical aspect of pharmaceutical manufacturing. ML and AI solutions facilitate adherence to stringent regulations by automating documentation, monitoring processes, and identifying compliance risks. These technologies enable proactive risk management, reducing the likelihood of regulatory violations and associated penalties.
In accordance with the US FDA, AI models, specifically those employing machine learning, can be effectively utilised to expedite the identification of optimal processing parameters or facilitate the scaling-up of processes. This application aims to streamline development timelines and minimise resource wastage. Advanced process control (APC) can serve as a mechanism for dynamically regulating the manufacturing process to achieve desired outcomes. AI methods can play a pivotal role in the development of process controls capable of predicting the progression of a manufacturing process. This is achieved by integrating AI with real-time sensor data, allowing for proactive adjustments. The integration of AI in monitoring equipment enables the early detection of deviations from normal performance, triggering timely maintenance activities and reducing process downtime. AI methods can also prove valuable in monitoring product quality, encompassing packaging quality. Vision-based quality control, utilising AI-driven software to analyse images of packaging, labels, or glass vials, can
Embracing AI-driven innovations in pharmaceutical manufacturing is a strategic imperative for competitive advantage.
efficiently identify deviations from the specified attributes. AI can extend its utility to scrutinising consumer complaints and deviation reports, processing large volumes of textual data to identify patterns and cluster problem areas. These approaches aid in prioritising areas for continual improvement, contributing to a more comprehensive root cause identification process.
Efficient supply chain management is essential for ensuring the timely availability of raw materials, components, and finished products. ML and AI enable predictive demand forecasting, inventory optimisation, and dynamic routing, leading to streamlined supply chain operations, reduced inventory costs, and improved responsiveness to market demand fluctuations. Identifying emerging market trends and customer preferences is vital for strategic decisionmaking in pharmaceutical manufacturing. ML and AI algorithms analyse market data, social media trends, and consumer behaviour patterns to identify new market opportunities, optimise product portfolios, and tailor marketing strategies to specific customer segments. ML and AI technologies play a crucial role in optimising engineering processes, such as formulation design, process devel -
opment, and equipment optimisation. Through leveraging advanced modelling techniques and simulation tools, these technologies accelerate product development cycles, reduce experimentation costs, and facilitate the design of robust manufacturing processes. The AI-based USP Medicine Supply Map represents another advancement. By scrutinising an extensive dataset comprising over 250 million data points and insights from 40+ external sources, including FDA and non-US regulatory agencies, alongside USP's own proprietary data from more than 22,000 locations worldwide, the Medicine Supply Map provides a platform that enables the continuous evaluation of potential disruptions in the medicine supply chain. Such real-time insights can empower governments, regulatory bodies, manufacturers, and healthcare institutions to make informed decisions to enhance supply chain resilience.
The successful implementation of ML and AI in pharmaceutical manufacturing requires skilled professionals with expertise in data science, computer programming, and domain-specific knowledge. However, there is a shortage of qualified talent in the field, making talent acquisition a significant challenge for pharmaceutical companies. The rapid evolution of ML and AI technologies necessitates ongoing investment in talent development and education initiatives within the pharmaceutical industry. Strategic talent acquisition efforts, professional development programs, and collaborations with academic institutions are essential for cultivating a skilled workforce equipped to harness the full potential of these technologies. Additionally, specialised ML and AI educational initiatives tailored to the intricacies of pharmaceutical manufacturing can accelerate the adoption and integration of these technologies across the industry. Silos within organisations can impede the effective integration of ML and AI technologies across departments and functions. Overcoming
organisational barriers requires strong leadership, cross-functional collaboration, and a shared vision for leveraging technology to drive innovation and competitiveness. Senior management buy-in is essential for the successful adoption of ML and AI in pharmaceutical manufacturing. Executives must demonstrate a commitment to investing in technology infrastructure, talent development, and change management initiatives to realise the full potential of these technologies. Resistance to change is natural in any organisation undergoing technological transformation. Employees may be apprehensive about adopting new tools and methodologies, fearing job displacement or increased workload. Effective change management strategies, training programs, and communication efforts are essential to address these concerns and foster a culture of innovation and continuous improvement. (Figure: 01)
While ML and AI offer numerous benefits in pharmaceutical manufacturing, human oversight remains essential to ensure compliance with regulatory standards and patient safety. Regulatory agencies require the presence of trained professionals who can interpret and validate the outputs of ML and AI systems, mitigating the risks associated with algorithmic
biases and errors. Therefore, organisations must strike a balance between automation and human intervention to maintain the highest standards of product quality and regulatory compliance. As the adoption of these technologies proliferates, pharmaceutical companies will increasingly rely on data-driven decision-making, automation, and predictive analytics to drive regulatory compliance, efficiency and remain competitive in a rapidly evolving landscape. Furthermore, collaborative initiatives, knowledge-sharing platforms, and industry-wide standards for data interoperability will further facilitate innovation and accelerate technological adoption across the pharmaceutical ecosystem.
The future of pharmaceutical manufacturing is intricately linked to the continued advancements in ML and AI technologies. With the continuous advancements in technology and the evolving landscape of the pharmaceutical industry, the integration of ML and AI is not merely an option but a necessity for organisations aiming to remain at the forefront of innovation and competitive advantage. Stakeholders must commit to ongoing investment to harness the full potential of
these technologies and drive meaningful change across all facets of pharmaceutical manufacturing. In addition to enhancing operational efficiency and driving cost savings, ML and AI have the potential to revolutionise the way pharmaceutical companies interact with their customers. Using advanced analytics and predictive modelling techniques, organisations can gain deeper insights into patient behaviour and preferences, enabling targeted advancements in personalised healthcare. By means of embracing these technologies, fostering a culture of continuous improvement, and working together, the industry can harness the transformative power of these technologies to propel pharmaceutical manufacturing into a brighter and more thriving future.
References are available at www.pharmafocusasia.com
Dr. Ajay Pazhayattil is a seasoned pharmaceutical expert with a wealth of experience in industrial pharmacy and executive management. He has a long history of driving innovation and value creation, resulting in the successful launch of numerous products for the brand, generic and CDMO pharmaceutical organisations. Dr. Pazhayattil is respected in the industry for his dedication to implementing data-driven, regulatory-compliant approaches that enhance the quality and effectiveness of pharmaceutical formulations. He is actively involved with various industry organisations such as AAPS, PDA, RAPS, ISPE and is a speaker at industry events, sharing his expertise and insights with his peers. In addition to his professional accomplishments, Dr. Pazhayattil has made significant contributions to the field through his published journal articles and textbooks, further solidifying his reputation as a thought leader in the pharmaceutical industry.
With the inherent complexity of the immune system, immune response, therapeutic interaction and clinical outcome, tools that provide additional functions to simultaneously assess target distribution and functional markers within a broader global immune landscape can expand and accelerate drug discovery and development.
Jennifer Ellis, Lifesciences WriterThe biology of disease can be confounding. While some single-source diseases can be accurately predicted and have a clear onset and progression path, many of the most common illnesses are incredibly complex. Take cancer. We have multiple treatments for it, but with its dynamic tumor growth and constant evolution, we have yet to grasp what it is that can stop it.
Methods to unravel this complexity produce such vast amounts of data to truly cover all aspects of a disease – how it is initiated, molecular pathways involved in progression, our immune response, cell types involved in resolution – that every step involves its own processes, each cascading from the next, that are always changing depending on our response and the microenvironment they are part of.
This is particularly important in efforts to develop safe and effective medicines. Understanding how a disease progresses can provide potential mechanisms of action that can then be the target of a new drug. Deciphering this information using the latest technologies in cytometry empowers users to ask broader questions and leverage more quantitative analysis that can accelerate studies, moving from sample to results faster.
The immune system is integral to our health and plays a key role in therapeutic success. Yet, because of variations in immune systems across the human population, individuals can respond very differently
The 37 immune cell subsets identified using the Maxpar Direct Immune Profiling Assay and Maxpar Pathsetter™ software
to the same treatment. A deeper understanding of immune cell activity in relation to treatment efficacy and safety can help us predict positive response from those who get no benefit from a medicine, ultimately enabling the development of better, more targeted treatments.
How can we thoroughly and systemically delve into these processes?
Immune profiling offers a snapshot of a person’s immune health status. Applying this approach longitudinally helps us gain a more comprehensive view of disease progression and immune response. It is an invaluable technique to assess the immune system, especially to
• discover biomarkers to predict responders to therapy;
• monitor onset of disease or response to treatment;
• gain a deeper understanding of the immune system and its role in multiple disease states.
The more we can get from immune profiling about the action of a novel therapeutic, the more likely we are to be able to determine its success.
Even though technologies have advanced to enable the generation of more data, initiation of new programs and investment in updating or fully replacing protocols
can be time-consuming. However, once implemented, these newer technologies can have a far greater impact and significantly improve not only the drug development process but also how and what we can learn from the underlying biology we can newly observe.
For example, conventional flow cytometry is a very low-parameter approach, with the ability to detect just 2–10 markers at a time, and the higher parameter you go, the more tedious and complex the assays become. Since the technology is based on fluorescent markers, there is a significant challenge in balancing co-expression, signal overlap and fluorophore brightness, all of which influence the number of parameters that can be measured. Depending on the level of protein expression targeted by each antibody, the fluorophore type must be matched to a protein with an expression level such that no fluorophore will be too bright to cover other signals or so dim as to go undetected. In an experimental or clinical/translational setting, expression of key markers such as for checkpoint or signaling molecules can be quite unpredictable, adding to the complexity of panel design. These issues also necessitate additional validation and staining controls, adding more steps and tubes of sample for every experiment. As one would surmise,
the more fluorophores used, the more antibody management needed and the more difficult it is to build or modify larger panels. While several challenges must be managed when using conventional and spectral flow cytometry for immune profiling, mass cytometry empowers scientists to overcome interfering factors such as workflow standardisation, preparation and storage of cells for later analysis, autofluorescence, sample heterogeneity, data quality and post-processing requirements.
An increased need to discern cell phenotype and function simultaneously in a multiplexed fashion necessitates these types of improvements in speed and resolution of single-cell technologies. Suspension-based cytometry, refined to meet the required levels of sensitivity and reproducibility for adequate measurement, can be used to monitor the immune system in assessing efficacy of different treatments and gauge success of novel immunotherapies.
As a more advanced approach to immune profiling, mass cytometry, the basis of CyTOF® instruments, immediately offers a paradigm shift in what you can do and how much you can learn from one sample. The technology uses pure metal-tagged antibodies and mass spectrometry to resolve highly multiplexed protein markers (both surface and intracellular) and
reveals systems-level biology at single-cell resolution. This powerful yet simple single-detector system is built with the capability to use 135 channels and a straightforward signal path from ionisation to detection that results in negligible background and minimal signal overlap. The ability to match metals to antibodies without worrying about color combinations allows addition or removal of markers with ease. What these features translate to is the ability to quickly build larger panels and a high degree of inter-site reproducibility and intermediate precision, minimising variability from different technicians, instruments or day-to-day operations. The use of high-parameter tools allows a look at everything at once, making the most efficient use of limited samples and providing an unbiased view of underlying biology. Whether you want to see cell type by surface markers or activation status by intracellular markers, CyTOF panels empower bigger questions to be asked and solutions realised. This equates to true high-parameter analysis, demonstrated in over 2,000 publications and more than 200 clinical trials.
The ability to ask broader questions using CyTOF technology is due to its inherently high-parameter capabilities. For example, non-targeted effects can stop a novel drug candidate from moving forward. The capability to extensively screen for non-targeted
Distinct signal detection seen with CyTOF technology results in cleaner data with no need for additional manipulation
effects offers insight into efficacy data and enables further analysis, such as translational modeling and simulation, to determine if a target is direct or is being taken up by non-targeted cell populations.
More quantitative analysis facilitates receptor quantification and receptor occupancy assays to assess binding of therapeutics to their targets and advise dosing decisions, while retaining the ability to deeply phenotype complex biological systems. This helps better inform a project and generates additional information that empowers teams to expand the questions that they can ask.
Multiplexing outputs and simultaneously detecting stimulation states of cells within different immune subpopulations broaden data generation and accelerate a lab’s ability to do more and deliver therapeutics faster.
For example, cell barcoding using CyTOF technology enables multiplexing, improves workflows and enhances data quality. Barcoded samples are stained together in a single tube, eliminating staining variability between samples and reducing antibody and reagent use. Acquisition time is faster, requiring only a single run of the multiplexed sample. This allows for higher sample throughput in scaled-up experiments and improved data consistency for large studies.
CyTOF workflows and instrumentation also allow for the standardisation of immune profiling assays with more automated sample acquisition to help reduce error and variability. They also offer the ability to prepare, stain and store samples for batch analysis at a later date or for shipping to a central facility. The fact that more than 50 markers can be confidently identified in a single experiment without having to run validation or staining controls enables a deeper dive into cell types and their functions while maximising small sample volumes. Additionally, the Maxpar® Direct™ Immune Profiling Assay™, a singletube assay measuring 37 cell populations from a 30-marker antibody panel, improves standardisation of protocols since sample (human whole blood or PBMC) is added directly to the tube and no assayspecific optimisation is required.
CyTOF technology provides impressively high resolution of cytometric profiles, empowering basic research and practical biomarker-driven clinical/ translational research to optimise and accelerate drug development. CyTOF instruments are proven valuable additions to the drug development pipeline, aiding in the creation of a framework to integrate knowledge and build a foundation on which drug development and treatment strategies can rely, ultimately leading to effective medicines and potential cures.
the University of Washington,
on the genetics of cancer. She is an experienced life sciences writer, marketing and business development professional having held positions in cancer research, diagnostic testing, life sciences sales and marketing and science writing for several institutions and biotech companies. Currently, she specialises in content development and strategy for cytometry, imaging and genomics applications, as a Staff Scientific Writer at Standard BioTools.
www.standardbio.com
Incorporating AI predictive models into drug development demands thoughtful consideration. Challenges encompass navigating complex biology and acquiring ample human data. Computational methods, GAN models and transfer learning, address knowledge gaps. The use of XAI enhances decision transparency. Addressing challenges is pivotal for its adoption in predicting drug efficacy and safety.
Manu Kumar Shetty, MBBD, MD, Maulana Azad Medical College Prof Anubha Gupta, Department of ECE, Centre of Excellence in Healthcare, IIIT Delhi.
The integration of artificial intelligence (AI) predictive models in drug discovery and development demands a thoughtful examination. AI models, particularly large Language Models, have undoubtedly proven their effectiveness in certain tasks. However, expecting the same levels of accuracy and output in drug discovery and development field is unrealistic. AI application in drug development requires careful consideration and a comprehensive understanding to avoid futile efforts. The underlying reason lies is the fact that LLM models were trained using extensive human-generated data, a luxury not easily replaceable in the complex biology field of drug development. To harness the power of AI in this sector, a substantial amount of real and intricate biological data must be generated.
In this context, the initial step involves comprehensively identifying all factors influencing the outcome related to drug development. Even if we may not under-
stand the either positive or negative influences or their direct or indirect effects on outcome, recognising these factors is crucial. Secondly, animal model generated data can not be useful in developing AI models and predicting the drug efficacy in humans. AI models developed using animal data would generate outcomes that will have the same level of attrition rate in drug success Therefore, it is essential to gather a sufficient amount of human data to develop accurate AI models . Third, the development of an explainable AI (XAI) model is necessary. This XAI model should provide clarity on how and why the AI model reaches its decisions. By doing so, we can analyse established features and their correlation with the XAI decision, gaining insights into the intricate nature of biological processes.
Currently, tackling these challenges is important before applying AI. This is complicated by limited understanding of complex biology and limited resources.
However, by employing computational methods and leveraging AI, we can address a significant portion of these issues with the right approach. The primary challenge involves comprehending all factors and relationships influencing outcomes in drug development. In these conditions, fully trained AI models using animal data directly cant be used for humans, but these models can be applied to generate the data of common biology concepts and relationships. After transfer learning is applied to develop generative AI models. Considering the complexities of biology, from DNA and RNA sequences to amino acid structures and the impact of external and internal factors on protein structures, computational methods such as sequence analysis models and generative adversarial network (GAN) models prove valuable. These models help fill gaps in our understanding. Once we understand that, it is possible to generate the extra data using generative AI models. Finally, by using transfer learning and XAI models, more reliable AI models can be developed.
Effective decision-making in the implementation of AI involves the thoughtful selection of appropriate AI models at various stages of drug discovery and development. This encompasses the prediction of key drug characteristics. The integration of AI and data science has uncovered valuable applications in the execution of clinical trials, with numerous industries seamlessly incorporating these cutting-edge technologies.
Hospitals that embrace electronic health records (EHRs) contribute significantly to the pool of high-quality healthcare data. Such data not only supports the swift adoption of AI in clinical trials but also plays a pivotal role in advancing medical research. Notably, advancements in speech-to-text technology and other language models have empowered healthcare professionals by saving time in managing electronic health records. The transformation of doctor-patient conversations into electronic health records not only streamlines the process but also alleviates the stress burden on healthcare workers. This EHRs data proves instrumental in various aspects, including patient cohort selection, expediting subject recruitment, facilitating the coordination of multi-center trials, and predicting early outcomes. Despite these advancements, challenges persist in other phases of drug development, enhancing drug efficacy, optimising drug pharmacokinetics, and predicting offtarget side effects.
The pharmacokinetics of a drug, a critical determinant of both its efficacy and safety, is intricately influenced by factors such as lipophilicity, solubility, permeability, molecular structure, metabolising enzymes, blood pH, and in-vivo physiochemical reactions. Developing a Multi-level AI model that incorporates all these factors is essential to derive a holistic understanding of the four key elements of drug pharmacokinetics: absorption, distribution, metabolism, and excretion. Currently, pharmacokinetic parameters are derived from in-vitro and animal studies. However, AI models built solely on these data may lack accuracy, given the higher complexity of human biology compared to in-vitro or animal conditions. Achieving a more precise AI model requires a significant infusion of human data that reflects the nuanced pharmacokinetics of drugs in humans. Furthermore, the early stages of drug design benefit from the adoption of AI for achieving desired pharmacokinetics. Utilising concepts such as structure-activity relationships and other
in-vivo factors specific to human biology enhances the accuracy and effectiveness of AI models in predicting and optimising pharmacokinetics profiles during the drug development process.
Drug Efficacy: Despite a drug exhibiting effectiveness in animal models, the attrition rate during phases 2 and 3 trials remains high due to a lack of drug efficacy. Relying mainly on animal studies for efficacy results is not entirely trustworthy. Various factors influence drug efficacy, such as target and drug features—these include the drug's affinity to the target, whether the binding is reversible or irreversible, and the conditions of drug binding in-vivo. Implementing the aforementioned solutions is essential for the development of a reliable AI model to predict drug efficacy.
Ensuring Drug Safety and Pharmacovigilance: Anticipating offtarget side effects prior to the marketing of a drug requires a comprehensive understanding of its affinity to various targets. AI models play a crucial role in facilitating this prediction by taking into account factors beyond the mere protein and drug chemical structures. The intricate nature of protein structures, influenced by both physiological and pathological conditions, demands an advanced AI model capable of navigating the intricacies of the body's biology and physiochemical reactions. Post release of a drug in the market, monitoring side effects becomes a key aspect of pharmacovigilance, and AI proves instrumental in this sector as well. Unstructured data is efficiently transformed into structured data using AI methodologies. Leveraging EHR significantly expedites the pharmacovigilance process, enhancing its efficiency and effectiveness. The integration of AI not only streamlines the monitoring of side effects but also brings agility to the overall pharmacovigilance workflow.
The dynamic landscape of AI in drug development continues to evolve, promising innovative solutions while simultaneously necessitating thoughtful consideration of the associated challenges. Adopting AI in
drug development involves recognising factors that influence the drug efficacy, safety, PK, And addressing challenges in understanding complex biology, and utilising computational methods. Overcoming the limitations of animal data necessitates gathering sufficient human data for accurate AI models. GAN models and transfer learning contribute to filling knowledge gaps between human and animal data, while XAI models enhance the reliability of AI models by providing transparency into decision-making processes. This approach enables a more informed and comprehensive application of AI in navigating the intricacies of drug development.
in patient care, drug discovery, and teaching medical professionals. Proficient in AI/ML, he has contributed to notable projects, including ECG-based AI prediction model and risk stratification AI model.
China’s pharmaceutical market is forecast to expand from CNY1.5trn(USD213.5bn) in 2023 to CNY4.8trn (USD676.4bn) by 2032.
• Far-reaching healthcare reforms continue to improve acces to and demand for medical services in China, boosting sales of innovative medicines
• The positive prospects in view of the progressive healthcare reforms will incentivise multinational drugmakers to invest in China
• The Chinese pharma market presents many advantages, including its size, growing incomes and notable government commitment to health sector development
Be one of the 3,500+ exhibiting companies and do business with over 75,000 pharma professionals
• Meet your current clients and source new buyers & suppliers in 1 location
• Enter the 2nd largest and rapidly growing pharma market in the world
• Educate yourself about the latest industry trends & developments, and get up-to-speed with your competition
• Network, learn and do business during the 6th edition of China Pharma Week. Your opportunity to maximise your business objectives during your stay in Shanghai
59% Manager, Project Manager, Department Head
17% President, Chairman, Director, General Manager
81% of visitors will continue to attend CPHI & PMEC China
88% visitors will recommend CPHI & PMEC China to co-workers & industry partner
“At CPHI
we are
to get good manufacturers with good price & quality!”
Yogesh Salunkhe, Purchase Manager
“As an annual event for the pharmaceutical industry, CPHI & PMEC China offers exhibitors an excellent platform full of networking, learning and trading opportunities. The outstanding service and various well-organized promotional activities led to an increased level of our company’s brand awareness.”
APTAR (CHINA) INVESTMENT CO., LTD
In the realm of pharmaceuticals, the quest for new therapies is a testament to humanity's relentless pursuit of healing and health. Yet, this journey is riddled with challenges that span the complexity of biological systems to the rigours of regulatory approval. Historically, the drug development process has been a painstaking marathon, fraught with inefficiencies and a daunting attrition rate. Enter the age of artificial intelligence (AI) and machine learning (ML)— technologies poised to revolutionise how we discover, test, and bring new drugs to market. This integration promises to accelerate the pace at which lifesaving drugs reach those in need, offering a beacon of hope in the complex landscape of pharmaceutical research.
Szczepan Baran, Chief Scientific Officer, VeriSIM LifeNavigating the drug development pathway is akin to embarking on an expedition through uncharted territories. Each phase, from discovery through to clinical
trials, is a step into the unknown, with the potential for both breakthroughs and setbacks. The traditional approach, while meticulous and grounded in decades of scientific inquiry, often encounters significant obstacles. High failure rates, particularly in transitioning from animal
models to human trials, highlight a critical need for innovation. The reliance on these models, despite their contributions to our understanding of disease mechanisms, underscores a pressing gap in our ability to predict human responses accurately.
The preclinical phase of drug development is pivotal, serving as the foundation upon which the safety and efficacy of new compounds are assessed. However, this stage is hampered by models that, though sophisticated, cannot fully replicate the intricacies of human biology. The challenge is multifaceted: on one hand, there's the biological complexity of human diseases, which can vary significantly from the conditions simulated in animal models. On the other, there's the diversity of human physiology, making it difficult to predict how a broad population will respond to a new therapy. This gap not only hampers the efficiency of drug development but also poses significant ethical and financial considerations, driving the search for more predictive and human-relevant models.
The integration of AI and ML into drug development heralds a shift from conventional methodologies to a more dynamic, predictive approach. These technologies have the potential to transform every stage of the drug development process. From identifying novel drug targets using AI-driven analysis of genetic data to optimising clinical trial design through predictive modelling, the possibilities are
vast. Regulatory bodies are beginning to recognise this potential, with the U.S. Food and Drug Administration (FDA) and the European Medicines Agency (EMA) exploring frameworks to evaluate and incorporate AI/ML technologies. The FDA has notably seen a surge in regulatory submissions incorporating AI and ML, a testament to the escalating integration of these technologies across the spectrum of drug development activities. This includes drug discovery and repurposing, clinical trial design, dose optimisation, and postmarketing surveillance, spanning a diverse range of therapeutic areas. The uptick highlights the extensive role of AI and ML in tackling the complexities of healthcare challenges, underpinning their significance in modern pharmaceutical research. In response to this growing reliance, the FDA is proactively enhancing its capabilities to assess and manage AI/ML applications within drug development. A key component of this strategy involves the publication of a discussion paper titled "Using Artificial Intelligence & Machine Learning in the Development of Drug and Biological Products," aimed at fostering a dialogue with the community through comments and feedback. The agency's efforts are focused on promoting responsible innovation through multi-disciplinary collaboration, the assurance of data quality, stringent cybersecurity measures, and the maintenance of independence between training and testing datasets. Parallel to the FDA's initiatives, the EMA has introduced a reflection paper on the incorporation of AI across the medicinal product lifecycle. This document seeks to establish scientific principles crucial for the regulatory evaluation of AI and ML technologies in medicine development and usage. It reflects the EMA's dedication to comprehensively grasp and integrate AI/ML advancements into regulatory structures, thereby ensuring the safety and effectiveness of the drug development process. These efforts by regulatory agencies underscore a proactive approach to harnessing the potential
Moreover, this integrated approach surpasses "AI alone" methodologies, which, despite their capability to process and analyse vast datasets, may not fully capture the dynamic and stochastic nature of biological interactions.
Harnessing the complementary strengths of both predictions and simulations allows for adeptly compensating for the limitations of each approach by leveraging the broad, pattern-identifying capabilities of AI with the depth of insight provided by knowledge-based simulations. It exemplifies how predictive analytics can identify potential leads and highlight areas of concern, while simulations can delve into the "how" and "why" behind these predictions, offering a comprehensive understanding that neither approach could achieve alone. This integrated strategy exemplifies a sophisticated symbiosis between AI's predictive analytics and the detailed, causal explorations facilitated by simulations, presenting a comprehensive and nuanced view of drug efficacy and safety profiles.
of AI and ML while ensuring patient safety and the integrity of clinical trial results. By providing guidance and establishing frameworks for the use of these technologies, the FDA and EMA are paving the way for a new era in drug development, characterised by increased efficiency, precision, and predictability.
Hybrid AI, in the context of combining simulation and predictions, stands as a transformative approach in preclinical research and development (R&D), enriching the drug discovery process with a depth of analysis unattainable through traditional or AI-alone methods. This approach leverages the predictive power of artificial intelligence to forecast outcomes and interactions within biological systems, while simultaneously utilising simulation techniques to model these complex processes in a controlled, virtual environment. This dual-methodology enables a comprehensive exploration of the pharmacokinetic and pharmacodynamic profiles of potential drug candidates, facilitating the identification of optimal molecular structures and the prediction of potential adverse effects before they reach the clinical trial phase.
Contrastingly, traditional experimental methods, while foundational to our understanding of biological systems, often require extensive time and resources, with a high risk of failure due to unforeseen toxicological effects or inefficacy in later stages of development. Hybrid AI surmounts these challenges by providing a scaffold for rapid hypothesis generation and testing through simulations, substantially narrowing the scope of physical experiments to the most promising candidates. Moreover, this integrated approach surpasses "AI alone" methodologies, which, despite their capability to process and analyse vast datasets, may not fully capture the dynamic and stochastic nature of biological interactions without the contextual grounding provided by simulation models.
Therefore, by fusing the forwardlooking analytics of AI with the detailed, process-oriented insights of simulation, Hybrid AI presents a synergistic model that not only complements but significantly enhances traditional drug discovery and development methods. This combination promises to accelerate the pace of innovation, reduce R&D costs, and improve the predictability of drug efficacy and safety profiles, marking a leap forward in our approach to meeting complex pharmaceutical challenges.
Hybrid AI has demonstrated its potential in evaluating the suitability of combination therapies for certain leukaemia types. The concept of "suitability" encompasses crucial aspects such as safety, efficacy, and dose-exposure relationships. To quantitatively assess the likelihood of success for these therapies, a multimetric index was employed. This involved using predictive AI models to gauge the overall toxicity probability of the combinations based on compound toxicities. Additionally, AI models assessing various pharmacological characteristics of the drug molecules informed physiologically-based simulations, illustrating the time-dependent drug exposure across different organs of interest.
For each component of the combination therapy, mechanistic simulations delineated the tumour growth trajectory, offering a precise visualisation of therapeutic impact over time. Importantly, given that drugs within a combination therapy can exhibit synergistic effects, AI models dedicated to predicting drug synergism were applied. These models helped quantify deviations from the merely additive effects of the drugs, offering a nuanced understanding of their interactive potential.
This comprehensive approach resulted in the calculation of multimetric index scores for more than 80 combination therapies in mice. Subsequent in vivo testing of a select subset of these combinations revealed a striking correlation of over 90 per cent between mice survivability and the computed multimetric index scores. Such findings underscore the efficacy of hybrid AI methods, showcasing their instrumental role in advancing the precision and reliability of combination therapy assessments for leukaemia treatment.
Another example of the benefits of hybrid AI methodologies involves comprehensive assessment of a drug’s toxicity via the inclusion of its metabolites and their toxicity and organ exposure considera-
tions. In pharmaceutical development and safety assessment, the significance of evaluating not only the primary drug candidate but also its metabolites cannot be overstated. This comprehensive approach is vital for accurately quantifying the potential toxicity of a drug in clinical settings. The metabolites of a drug, formed through various physiological processes, can significantly contribute to the overall toxicity profile of the drug system, especially if they display slow clearance.
A hybrid AI approach is well-suited for assessing the overall toxicity of a drug under development. Predictive models that incorporate physiological metabolic knowledge helps in determining the metabolites of a drug candidate directly from its chemical structure or SMILES string. These can be combined with predictive models for toxicity of metabolites and simulations of the drug and its metabolites that quantify their organ exposure to provide a comprehensive toxicity assessment of a drug candidate. Hybrid AI was utilised in one example to assess the wholistic toxicity potential of several drug candidates by incorporating predictions (metabolites, drug and metabolite toxicities, and associated DDI risks) with simulations (organ-specific exposure of the drugs and their metabolites) to determine that the inclusion of metabolites in the hybrid AI analysis can increase the likelihood of a drug candidate by several folds.
As we embrace the integration of hybrid AI into drug development, we stand on the cusp of a new era—a time when the discovery and delivery of therapies are not only faster but also more precise. This approach not only enhances the predictability of drug candidates' success but also bridges the translational gap, signalling the dawn of a new era in drug discovery. The complexity of biological systems and the high failure rates of drug candidates in clinical trials underscore
the urgent need for this innovative approach. Hybrid AI methodologies, as demonstrated in the provided case studies, offer the potential to de-risk the drug development process by accurately predicting human responses, tailoring therapies to individual needs, and significantly cutting down the time and costs associated with market introduction. This integrated strategy not only confronts the uncertainties intrinsic to drug development but also establishes a new standard for developing safer, more effective therapies. Ultimately, by leveraging the predictive power of AI and the innovative capabilities of ML, we are set to fulfil the Life Sciences industry's mission of enhancing patient care and treatment outcomes, paving the way for a more efficient and effective paradigm in drug discovery and development.
and veterinarian
He is passionate about transforming the delivery of humane medicines to patients through digital technologies and data enablement while pushing the scientific envelope and reimagining patient engagement.
Szczepan currently serves as the Chief Scientific Officer at VeriSIM Life, which leverages AI to enhance drug discovery and development through a virtual drug development platform. He leads regulatory engagement and strategy, oversees research and development, and manages scientific functions, including both basic and applied research projects. Additionally, he develops new processes, technologies, and products.
11–13 September 2024 | Marina Bay Sands, Singapore
Covering over 15,000 square metres, the two synergistic exhibitions will showcase more than 1,000 exhibitors from 50 countries and regions, and 18 national pavilions and country groups.
This year’s iteration features highlights such as the Start-Up Park, Community Care Pavilion and industry-specific conferences and seminars.
The 15th edition of MEDICAL FAIR ASIA and 6th edition of MEDICAL MANUFACTURING ASIA, the region’s leading specialist trade fairs for the medical, healthcare, and medical technology (MedTech) sectors return to Marina Bay Sands from 11 to 13 September. As the largest exhibitions of their kind in Southeast Asia serving the full value chain and end-to-end needs of the medical and healthcare sectors, the two exhibitions will present products and solutions covering diagnostics, wearable technology, connected healthcare solutions, rehabilitation and therapy equipment, 3D printing
technology, MedTech components, micro and nanotechnology, and more. The comprehensive range of companies on show makes MEDICAL FAIR ASIA and MEDICAL MANUFACTURING ASIA the ideal destination for medical and healthcare buyers looking to meet their sourcing and procurement objectives.
MEDICAL FAIR ASIA 2024 comes at a time when Southeast Asia's healthcare market is experiencing substantial growth. According to Bain & Company, the Asia-Pacific region is set to account for more than 20% of global healthcare spending by 2030. The region continues to be one of the fastest-growing globally, driven by increasing consumer engagement and technological advancements. On the domestic front, Singapore's healthcare investments are intensifying. By 2030, nearly one million Singaporeans will be over the age of 65, significantly increasing the demand for elderly healthcare services. This demographic shift will drive Singapore’s annual healthcare expenditure to more than S$66 billion according to industry experts.
Globally, the digital healthcare market is also expanding rapidly. The Southeast Asia digital health market is projected to reach US$6.07 billion by 2024,
while telehealth, a significant component of digital healthcare, has seen substantial adoption, with its usage stabilising post-pandemic and becoming a new normal in the region according to McKinsey & Company). The global HealthTech boom continues to open new opportunities, with innovations enhancing service delivery and healthcare outcomes across Southeast Asia.
MEDICAL FAIR ASIA and MEDICAL MANUFACTURING ASIA 2024 is set against this backdrop of robust growth and innovation, making it both pivotal events for industry professionals to explore new opportunities, forge connections, source the latest innovations, and stay abreast of the latest trends in healthcare.
MEDICAL FAIR ASIA will also see some 18 national pavilions and country groups, with new representation this year from Belgium, Greece, Puerto Rico, and Qatar. The return of signature zones such as the Start-Up Park
and Community Care Pavilion, and shed a spotlight on pandemic management solutions, alongside thought leadership content at concurrent conferences and seminars.
A key feature at the exhibition for the past three editions, the Start-Up Park is an ideal platform for innovative companies with ready-to-market solutions to meet relevant buyers and partners, industry influencers and potential investors. Innovations on show include Artificial Intelligence (AI) and Big Data solutions, wearable technologies and innovative healthcare software. Notable start-ups include Doinglab Inc, Fcode Labs/Progress Magic, Talos Corp, and Platforce Limited.
Another key feature alongside the Start-Up Park, the Start-Up Podium will include a special line-up and series of fireside chats and panel discussions with high-level speakers on topics such as:
• Revolutionising Healthcare: Achieving the 3Es and 3As - Efficiency, Effectiveness Affordability, Accessibility, and Availability
• Digital Biomarkers and Remote Patient Monitoring
• Voice Assistants and Natural Language Processing in Healthcare
• Digital Therapeutics and Prescription Digital Health
• Remote Healthcare -Healthcare Gamification for Behavior Change
Community Care Pavilion
The Community Care Pavilion will showcase products and solutions designed for the aging population, focusing on preventive care and chronic disease management. This includes a comprehensive range of geriatric medicine, rehabilitative equipment, mobility products utilising robotic technology, assistive technology, smart fabrics, and wearable technology.
A comprehensive, full-day live programme covering cutting-edge technologies and the latest advances in wearable technologies, where attendees can explore key topics in health, sport and many more with insights from global experts across the spectrum of wearable technology.
(Continuing Medical Education) points
Returning once again is the MEDICAL FAIR ASIA MEDICINE+SPORTS CONFERENCE which will see top international sports medicine and sports science experts, physiotherapists and healthcare professionals, sports techies, and professional athletes exchanging insights on innovative approaches in prevention, training, regeneration and rehabilitation.
Paradigm Shifts in Healthcare Symposium
Returning for its third run, this year’s symposium will be held over two half-days focusing on topics relating to community healthcare.
As the MedTech industry continues rethinking and adapting to healthcare needs of the future, MEDICAL MANUFACTURING ASIA is well-timed as Singapore continues to strengthen its foothold and prime position as a high-value MedTech manufacturing base for the region. Jointly organised by Messe Düsseldorf Asia and long-time partner Singapore Precision Engineering & Technology Association (SPETA), MEDICAL MANUFACTURING ASIA is a specialist trade fair focused on manufacturing, and this year welcomes over 100 MedTech companies from over 15 countries and regions, including national and group pavilions from Singapore, Austria, Japan, and the United States.
Visitor registration for MEDICAL FAIR ASIA 2024 and MEDICAL MANUFACTURING ASIA 2024 is now open, go online to register now!
www.medicalfair-asia.com
www.medmanufacturing-asia.com
Drug development teams make data-driven decisions, but these decisions can be limited by disparate data sources, siloed technologies, and fragmented insights. Deploying generative artificial intelligence in drug development can empower users to access and interact with sample and biomarker data in new ways and extract insights at the speed of decisioning.
1. Could you provide insights into how artificial intelligence (AI) is currently being employed to analyse sample and biomarker data in clinical research?
AI is currently being employed in various ways throughout clinical research. Some examples include identification of drug targets, patient recruitment, and synthesisation of information from scientific literature. AI and machine learning algorithms can help researchers sift through vast amounts of clinical trial data before a clinical
trial begins, which can help determine the appropriate patient population for their study. One of its applications in clinical trial research and design is the amplification of data management and insight generation to support patient selection strategies, drive clinical trial efficiency and democratise knowledge across scientific teams, which is a focus area of QuartzBio’s AI-powered Biomarker Intelligence Platform to support critical workflows and decisions that must be made throughout the precision medicine lifecycle.
2. How do these AI applications enhance the efficiency and accuracy of sample and biomarker data analysis?
One of the core challenges faced by the life science industry and more specifically by drug development teams is a disconnected data and technology ecosystem, resulting in fragmented insights from sample and biomarker data assets. Instead of spending time on high-value work, teams are left manually navigating between various files or spreadsheets to analyse this critical data. QuartzBio’s AI-enabled Data Management and Business Intelligence tools can address this challenge by streamlining and automating data ingestion, quality control, and standardisation. This process establishes a high-quality data foundation, which subsequently enables insights through conversational and prescriptive AI capabilities. These insights are easily consumable and highlight operational and scientific trends within a sponsor’s data asset.
3. In what ways does AI serve as a force amplifier in transforming sample and biomarker data into actionable insights?
AI serves as a force amplifier in transforming sample and biomarker data into actionable insights in several ways:
Efficient Data Management: By leveraging natural language processing and workflow automation, AI significantly reduces the time spent on low-
value data management activities, such as locating, organising, processing, and mapping sample and biomarker data. This efficiency allows scientific, operations, and data teams to allocate more time to high-value tasks such as insight generation, biomarker-guided decision-making, and collaboration. Teams are therefore more productive and are able to extract the maximum value out of precious clinical trial samples and data..
Instant Insight Generation: AI dramatically shortens the time from question to answer. Machine learning algorithms, combined with natural language understanding, can quickly sift through vast amounts of data, providing rapid responses to users, even users without
specific data expertise. This combination of user accessibility and speed is particularly beneficial for time-sensitive decisions in clinical program management, which can lead to improved patient and business outcomes.
Through pattern recognition and machine learning, AI has the unique ability to uncover trends in sample or biomarker data that were previously hidden or unnoticed. These insights, in the context of a connected ecosystem where biomarker and sample data are linked, can directly inform clinical programs. Example outcomes include using these insights to guide patient / dose selection or identify new therapeutic targets.
4. How does the integration of AI technologies accelerate decisionmaking processes in clinical research?
The integration of AI technologies significantly accelerates decision-making processes in clinical research in several ways:
Shortening the Question-to-Insight
Lifecycle: Clinical trials generate millions of data points. AI can process and analyse large volumes of clinical, sample, and biomarker data, rapidly transforming raw information into actionable insights informed by business rules.
Enabling Complex Queries:
Generative AI technologies allow for iterative data exploration. This means that, as initial questions are answered, researchers can pivot to more complex or nuanced questions. Extracting more comprehensive, detailed insights in this way empowers teams to make more informed decisions, faster.
Broadening Use Cases: AI technologies operating on interconnected clinical programme data assets allow researchers to explore a broader range of use cases. By linking different data sets, AI can uncover correlations and patterns that might be missed in more traditional, siloed approaches to data analysis.
Augmenting Human Expertise:
The most successful applications of AI to clinical research combine AI-enabled processes with human scientific and operational expertise. AI can process data and recognise patterns, but human experts are still needed to provide context, interpret results, and make final decisions. This combination of AI and human life science domain-specific expertise leads to more accurate and effective decision-making in clinical research.
In conclusion, the integration of AI technologies in clinical research not only accelerates decision-making processes but also enhances the depth and breadth of insights obtained, ultimately leading to more effective and efficient research outcomes.
As the market becomes more saturated with AI-powered products or capabilities with their own domain-specific benefits, it’s important that these technologies integrate with or work in conjunction with other technologies, making them easier to implement into existing environments to support specific initiatives.
involves rigorous data cleaning, validation, and preprocessing procedures to ensure that the data fed into the AI models is accurate and reliable.
Model Validation: AI models need to be validated to ensure their accuracy and reliability. This can be achieved by implementing a robust validation framework. Incorporating a “Human-in-theLoop” approach, where human experts review and verify the AI’s outputs, can provide an additional layer of checks and balances. This not only helps in catching errors but also in refining the AI models over time.
6.What opportunities do you foresee in the future for further leveraging AI in this domain?
5. What challenges do you encounter in implementing AI for analysing sample and biomarker data, and how do you overcome them?
Implementing AI for analysing sample and biomarker data presents several challenges, but with the right strategies, these can be effectively managed:
Data Privacy and Security: Protecting the privacy and security of clinical, sample and biomarker data is crucial for ethical and regulatory compliance. The risk of data breaches can be mitigated by avoiding the use of third-party APIs, which may have unknown security vulnerabilities. Instead, using trusted and secure hosted-platforms can help ensure that data remains confidential and secure.
Data Quality: The principle of “garbage in, garbage out” is particularly relevant in AI. Poor quality or inaccurate data can lead to misleading or incorrect results. This challenge can be addressed by building a strong foundation of high-quality, consistent sample and biomarker data. This
As the market becomes more saturated with AI-powered products or capabilities with their own domain-specific benefits, it’s going to be important that these technologies can integrate with or work in conjunction with other similar technologies to meet the needs of the market. In this domain, there won’t be one solution that can solve every challenge, so the more user-friendly and interconnective these technologies can be, the easier they will be to implement into existing environments to support specific initiatives.
7. Are there any ethical or regulatory considerations that need to be addressed when utilising AI in sample and biomarker data analysis?
Yes, there are several ethical and regulatory considerations that need to be addressed when utilising AI in sample and biomarker data analysis:
Data Privacy and Security: AI technologies often require access to large volumes of data, which may include sensitive patient information. It’s crucial to ensure that AI frameworks are integrated into secure data workflows that comply with privacy regulations and support secure data storage and processing to prevent unauthorised access.
Regulatory Guidance: Various regulatory agencies have provided guidance on leveraging AI throughout the drug development lifecycle. It’s important to stay updated with these guidelines and ensure that the use of AI aligns with them.
GxP Compliance: While AI can enhance many aspects of clinical research, it’s essential to follow existing processes to support best GxP practices. These practices ensure that the products support their intended use and adhere to quality processes during the Software Development Lifecycle.
Risk-Based Approach: Given the inherent challenges posed by new technologies to existing paradigms, a risk-based approach should be applied to support GxP requirements where needed to ensure data privacy, data security and model performance and accuracy. This involves identifying potential risks associated with the use of AI, assessing their impact, and implementing strategies to mitigate them.
Human-in-the-Loop Approach: Despite the capabilities of AI, human oversight is still crucial. A human-inthe-loop approach allows for checks and balances, ensuring that the AI system’s outputs are accurate and reliable. This approach combines the strengths of AI with human judgement and expertise.
In conclusion, while AI holds great promise for enhancing clinical research, it’s essential to navigate its use carefully, considering the ethical and regulatory aspects to ensure its benefits are realised responsibly and safely.
8.What do you envision as the future trajectory of AI applications in transforming sample and biomarker data analysis in clinical research?
The future of AI in the domain of sample and biomarker data analysis holds immense potential and opportunities:
Revolutionising Drug R&D: AI is poised to change drug research and
development in novel ways, making the process more efficient and effective, which can help automate processes that were once manual and time-consuming and ultimately accelerate R&D workflows.
Optimisation of Learning
Capabilities: There is an expected focus on the optimisation of AI’s learning capabilities that empower output and outcomes. This means delivering more value, speed, and accuracy in data analysis and interpretation. This also drives the importance of domainspecific learning capabilities that can continue to learn and adapt in a specialised environment for the most accurate and optimised output.
Evolution of Capabilities and Processes: AI will help capabilities and processes evolve to become more predictive or prescriptive in nature. This evolution will enable more proactive and informed decision-making in the field. Rather than just pointing in the right direction to solve a challenge, AI-enabled technology can flag discrepancies or issues and recommend options or actions to solve them. This feature enhances the problem-solving capacity in the domain and helps users work smarter, not harder.
In conclusion, the future of AI in this domain is promising, with the potential to significantly enhance the efficiency and effectiveness of sample and biomarker data analysis coupled with shortening time-to-insight significantly.
9. How do you see AI continuing to shape the landscape of clinical research in the years to come?
AI is expected to continue shaping the landscape of clinical research by disrupting practices across the R&D lifecycle, impacting clinical and translational research as well as trial planning and management, leading to continual adoption by pharma and biotech innovators and leaders. As adoption of AI throughout clinical research continues to grow,
we expect to see these capabilities lend themselves to key growth areas that can drive the most impact. At QuartzBio, we see the importance of AI to fuel and support precision medicine efforts across the drug development lifecycle and the need for purpose-built technology that can support R&D teams in this work.
10. Any other comments?
AI is not a new concept; it’s been around for a while and has been used to support core research activities, especially around drug target identification, validation, and screening. Recent advances in computational capacity coupled with the emergence of transformer-based AI models have enabled AI to surpass current applications and really broaden its reach and use cases in the drug R&D space.
Tobi has over 12 years of experience leading informatics, data science and software development operations, driving innovation and development of technology solutions across a variety of data-centric spaces ranging from development of complex biomarker signatures for use in patient selection, to deployment of enterprise software solutions geared towards pharmaceutical and biotechnology companies. As SVP of Product Innovation and Chief Architect at QuartzBio, Tobi leads QuartzBio’s Product Innovation organisation while continuing to drive the technology vision for QuartzBio's next phase of development and growth.
PMMI Business Intelligence surveyed pharmaceutical manufacturers in late 2023 and early 2024 to track trends since the 2022 report on Pharmaceutical Manufacturing. Amid projected growth, the industry faces opportunities and challenges in meeting marketplace demands.
Top Machine Types by Share of the Pharmaceutical Market (total
Artificial Intelligence (AI), especially deep learning, is revolutionising drug development by harnessing big data and Machine Learning (ML) algorithms like artificial neural networks. Despite initial scepticism, AI's role in expediting and economising the drug discovery process is gaining momentum. This review explores AI's transformative potential in refining pharmaceutical development, promising a future of innovation and efficiency.
Rahul S Tade, Academic Researcher, Pharmaceutical Education and Research InstituteIn the intricate realm of drug development, where innovation stands as the cornerstone of human well-being, the journey from discovery to market is a labyrinthine path fraught with challenges. With costs averaging around US$2.6 billion and a timeframe spanning over 12 years, the pharmaceutical industry has long sought avenues to streamline this arduous process. Enter AI, particularly its deep-learning (DL) component, heralding a new era in computational drug discovery.
The convergence of classified big data, robust computing capabilities, and cloud storage has catalysed the integration of AI into pharmaceutical research. Machine Learning (ML) algorithms, notably DL methods like artificial neural networks (ANNs), have gained prominence for their ability to extract complex patterns from vast datasets and discern nonlinear relationships, thus surpassing traditional human-engineered molecular descriptors.
Despite initial hesitations, AI's role in drug discovery is steadily gaining acceptance, promising to revolutionise medicinal chemistry. DL-powered methods have begun tackling pivotal challenges in drug development, leveraging advancements such as "message-passing paradigms" and "hybrid de novo design." As we delve deeper, the synergy between AI and experimental knowledge holds the key to expediting and economising the quest for novel therapeutics.
The integration of AI and ML in drug discovery offers several advantages. Firstly, these technologies enable the analysis of large datasets, including biological,
chemical, and clinical data, facilitating the identification of novel drug targets and compounds with therapeutic potential. By leveraging DL methods, researchers can uncover hidden patterns and relationships within these datasets, accelerating the identification of promising candidates for further investigation. Moreover, AI-driven approaches facilitate the optimisation of lead compounds, enhancing their efficacy and safety profiles. Through iterative modelling and simulation, researchers can predict the pharmacokinetic and pharmacodynamic properties of potential drugs, enabling informed decision-making early in the development process. This not only reduces the likelihood of late-stage failures but also minimises the need for costly and time-consuming experimental validation.
Furthermore, AI and ML techniques contribute to the design of novel drug molecules through de novo synthesis or virtual screening of compound libraries. By generating virtual chemical libraries and employing predictive models, researchers can prioritise compounds with the highest likelihood of success, thereby streamlining the lead identification process.
In addition to accelerating drug discovery, AI and ML play a crucial role in personalised medicine. By analysing patient data, including genomic information and clinical outcomes, researchers can identify biomarkers associated with disease susceptibility, progression, and treatment response. This enables the development of targeted therapies tailored to individual patients, improving efficacy and minimising adverse effects. Despite the promising potential of AI and ML in drug discovery, several challenges remain. One major hurdle is the quality and availability of data, particularly in areas such as rare diseases or unexplored therapeutic targets. Additionally, the interpretability of AI models poses a significant concern, as understanding the rationale behind model predictions is essential for regulatory approval and clinical adoption.
To address these challenges, efforts are underway to promote data sharing
and collaboration within the scientific community. Initiatives such as openaccess databases and collaborative research platforms facilitate the sharing of datasets and methodologies, enabling researchers to leverage collective knowledge and resources. Moreover, advancements in model interpretability techniques, such as attention mechanisms and feature importance analysis, are improving our ability to understand and trust AI-driven predictions. By elucidating the underlying rationale behind model decisions, these techniques enhance transparency and facilitate regulatory approval and clinical translation.
Recent advancements in ML-based strategies in drug development have significantly accelerated the pace and efficiency of discovering new therapeutics (Figure 1). Some notable examples include:
1.Generative Models: Generative models, such as Generative Adversarial
Networks (GANs) and Variational Autoencoders (VAEs), have emerged as powerful tools for generating novel molecular structures with desired properties. These models can aid in the exploration of chemical space and the discovery of lead compounds for drug development.
2.Graph Neural Networks (GNNs): GNNs have gained traction for their ability to model molecular structures as graphs and capture intricate relationships between atoms and functional groups. These models excel in tasks such as molecular property prediction, molecular similarity assessment, and drug-target interaction prediction.
3.Reinforcement Learning (RL): RL algorithms have been applied to optimise drug discovery processes, such as molecular generation and lead optimisation. By iteratively learning from feedback, RL agents can design molecules with desired properties while navigating the vast space of chemical compounds efficiently.
4.Transfer Learning: Transfer learning techniques, borrowed from computer vision and natural language processing, have been adapted to drug discovery tasks. Pre-trained models can be fine-tuned on small molecular datasets to improve predictive performance, especially in scenarios with limited labelled data.
5.Deep Cheminformatics: Deep learning architectures tailored for cheminformatics tasks, such as molecular property prediction and compound optimisation, have demonstrated remarkable performance improvements over traditional methods. Models like graph convolutional networks (GCNs) and attentionbased mechanisms have revolutionised the field by capturing hierarchical and spatial dependencies in molecular data.
6.Explainable AI (XAI): With the increasing complexity of ML models, there's a growing need for interpretability in drug discovery. XAI techniques aim to provide insights into model predictions and decision-making processes, enhancing trust and facilitating knowledge transfer between computational and medicinal chemists. (Figure 1)
AI and ML play a crucial role in predicting pharmacokinetic parameters, aiding in the fight against animal cruelty. Pharmacokinetic studies encompass absorption, distribution, metabolism, and excretion, along with pharmacody-
namic effects. These studies necessitate numerous calculations, and even slight errors or missing data can have significant consequences. AI facilitates swift and accurate computations, reducing the likelihood of errors while ensuring costeffectiveness. By transforming complex data into comprehensible graphs, AI aids in identifying underlying issues efficiently. Moreover, AI minimises the need for animal experimentation by simulating various conditions such as enzyme activity, disease states, and dosing variations across different species. Consequently, fewer animals are required for clinical trials, contributing to the reduction of animal cruelty (Figure 2).
The integration of AI and Machine Learning (ML) in drug delivery technologies is transforming pharmaceutical research and healthcare practices. By harnessing AI's capabilities in data analysis, pattern recognition, and optimisation, researchers and healthcare professionals can develop targeted, personalised, and adaptive therapies. AI-based methods offer advantages over traditional experimental approaches by predicting pharmacokinetic parameters, simulating drug distribution and clearance, and optimising dosage and administration routes. Computational pharmaceutics, driven by AI and big data, streamlines drug delivery processes, enhances regula-
tory compliance, and reduces risks. This revolution promises to accelerate drug development, improve patient outcomes, and propel the pharmaceutical industry into its next evolutionary stage.
References are available at www.pharmafocusasia.com
Rahul S Tade is an academic researcher at a pharmaceutical education and research institute. He is currently focusing on novel therapeutic strategies in the treatment of triple negative breast cancer (TNBC). His research area includes advanced nanomaterials in the design of nanotheranostics. He recently received a start-up research grant for his research. He has published more than 40 research and review articles. He is exploring the application of AI and ML in pharmaceutical research.
Let the true “Digital Transformation” be the base of all your marketing campaigns
Highly accountable marketing campaigns. Every dollar counts. Digitally powered marketing campaigns may be cheaper than you thought... Ask us how?
Use the webinar as a platform to launch new products and services
Grow your audience with increased reach, impact and user-friendliness
Rise above geographical boundaries
Generate new business
Gain the strong web presence differentiating yourself from competitors
Connect and engage with your target audience
Give more exposure to industry specific people
Increase your brand profile and share your capabilities with leading industry professionals
Email : advertise@pharmafocusasia.com
Website : www.pharmafocusasia.com
SVP, Enterprise Medical Solutions, Indegene.
Director, Ask GXP & ServBlock
In an interview, Sameer Lal discusses the impact of GenAI in reshaping the pharma industry. Exploring GenAI's transformative potential, emerging trends, and regulatory challenges, this discussion offers valuable insights for pharmaceutical executives navigating the evolving landscape of AI in drug development and commercialisation. It also sheds light on the potential impact of GenAI adoption on the pharma workforce.
The integration of Generative AI (GenAI) technologies in the pharmaceutical industry represents a significant paradigm shift, promising to enhance drug discovery, streamline manufacturing processes, and optimise knowledge management. We discuss the methodology of implementing GenAI tools, such as automated document generation, AI-driven simulation models for clinical trials, and adaptive learning systems for workforce training.
Expert, Artificial Intelligence (AI), Pharmaceuticals, and Strategic Implementation of AI
Generative AI (GenAI) holds tremendous promise for automating drug design, speeding up clinical trials with synthetic data and digital twins, automating many aspects of regulatory and medical affairs and personalising sales and marketing. Nevertheless, fully realising these advancements while managing associated risks demands meticulous planning from both industry and regulators.
How is AI and especially generative AI (GenAI) starting to transform the pharma industry? What advantages does it offer over traditional methods, and what are the limitations?
SAMEER: From my vantage point, over the past year, the initial hype as well as the wariness around GenAI has started to settle into cautious optimism. Within the confines of their secure platforms, pharma enterprises have been experimenting with use cases right from R&D to medical affairs to commercial functions. GenAI specifically is allowing a
fundamental re-think on three broad fronts: summarising and generating insights from vast amounts of data; generation of content; and healthcare professional (HPC) engagement. Each of these in turn is being used by pharma to serve any (or a mix) of these objectives—efficiency, effectiveness, or strengthening competitive edge.
Having said that, in the absence of clear regulations, data security and privacy concerns, clarity over copyrights as well as overall risk-averseness of the industry implies that scaling up from these experiments will take some time to come to fruition.
JOHN WARD: The introduction of GenAI in pharmaceutical manufacturing is shifting the landscape for knowledge workers. By automating routine documentation, data entry, and monitoring tasks, workers are freed up to focus on more strategic and creative tasks such as process optimisation and innovation. This shift not only enhances productivity but also improves job satisfaction by allowing workers to engage in more meaningful and rewarding work.
Generative AI models possess the potential to revolutionise drug discovery, research and development, streamline clinical trials, improve patient care, and enhance numerous aspects of regulatory affairs, medical affairs and personalise sales and marketing at scale. Examples of gen AI applications in Pharma include:
• Creating New Drugs/Drug Discovery: Generative AI can make new molecules that are specifically designed to deal with certain diseases or health problems. The molecules can be made to work better and be safer. They can also be created so they can be absorbed, distributed, metabolised, and excreted more effectively in the body.
• Improving Drug Candidates/Lead Optimisation: Generative AI can help with this by making new molecules that are similar to the drug candidate but have better properties. These new molecules can then be made and tested to see how well they work and how safe they are.
• Finding New Uses for Drugs/Drug Repurposing: Another use for Generative AI is to find new uses for drugs that already exist. Generative AI can do this by making new molecules that are similar to existing drugs but work differently. These new molecules can then be tested to see if they could be used to treat other diseases.
• Creating Personalised Medicines: Generative AI can help create these personalised drugs by making new molecules that are specifically designed for certain groups of patients. It can help make treatments work better and have fewer side-effects by making new molecules that are specifically tailored to individual patients.
• Clinical Trials: Generative AI can make clinical trials more efficient by figuring out which groups of patients are
likely to respond well to a certain treatment. For example, generative AI programmes are being trained to find genetic markers that can predict if a patient will respond well to a certain drug. It can also speed up trials by finding the patients using AI, and leveraging Gen AI-generated synthetic data and digital twins reducing the need for as many humans in the trials.
• Regulatory: Automated document sorting, text recognition, automated filling of standard regulatory forms, automatically classify and tag various types of documents, ensuring that every submission is complete and correct, collating daily guidance, regulations, news and Predict compliance changes
• Medical Affairs: Intelligent literature monitoring, track and respond to large volumes of medical queries, Automated medico-legal reviews, automated SLRs, automated literature monitoring
• Sales and Marketing: Create content and assist with
personalised interactions (Websites, social media, and messaging ), and create personalised messages (emails and marketing materials), precisely target the right physicians, identify patients who will stop adhering in advance and much more.
The advantages over traditional methods are the ability to analyse extensive datasets rapidly, formulate predictions, and generate new data and insights making them indispensable tools for researchers, clinicians, and pharmaceutical teams in general. In particular, some of the Generative AI models being employed for this are Generative Adversarial Networks (GAN), Recurrent neural networks, Variational auto-encoders, Deep Reinforcement learning and Transformer models.
What emerging trends do you foresee in the intersection of GenAI and pharma? How might advancements in AI technologies reshape the industry in the coming years?
SAMEER: We are seeing some excellent results come through in all the 3 areas that I mentioned earlier. Here are some specific examples:
• Summarisation and generation of insights: A clear use-case is in reviewing and analysing vast amounts of information that gets presented at conferences like ASCO. GenAI allows the generation of quick summaries from large articles and actionable insights to be curated from there
• Content generation is likely to face the most change in the coming years. From “writing” of the first draft of various regulatory and safety documents, creation of plain language summaries, translation from one language to another, and transcreation from one format to another (Word to infographic) all have been successfully demonstrated. With Sora, the creation of video from text is now child’s play and does not need a full-service agency
• Customer engagement is already turning on its head with conversational AI implemented in chatbots, speech-to-text conversion in call centres, as well as hyper-personalisation possibilities that hitherto were not even possible
The pharma industry which has always been at the forefront of innovation will now need to assess their use of the vast data that at its disposal to create small LLMs for their own use, reengineer process flows, and rethink traditional roles and job descriptions to get the most value from this change.
Management: GenAI excels in organising and processing large volumes of data, transforming how knowledge is managed on the shop floor. It can analyse historical data to identify trends and patterns, suggest process improvements, and even predict maintenance needs before breakdowns occur. This proactive approach to maintenance and operations not only enhances efficiency but also extends the lifespan of manufacturing equipment.
ANDREE BATES: The industry has the potential to be streamlined with Gen AI taking on more roles within identifying new molecules, speeding up clinical trials, and increasing efficiency throughout most functions. With the advent of whole-body digital twins, we are likely to see pharma stop
SAMEER heads the Enterprise Medical Solutions business at Indegene. He has 25 years of experience in the pharmaceutical industry and technology-led healthcare solutions provider sector. Prior to joining Indegene, Inc., he was associated with GlaxoSmithKline Pharmaceuticals Limited, Ranbaxy Laboratories Limited and Sudler & Hennessey Private Limited. He holds a bachelor of science degree and a bachelor of science (technology) degree, both from University of Mumbai and master of management studies degree from the Narsee Monjee Institute of Management Studies, Mumbai
doing manufacturing and each patient will have personalised dosages of medicines as determined by their digital twin. Each pharmacy will have a 3D printer and print the drug for the patient. 3D printers that already print biologics exist so this is not as far-fetched as you may think!
SAMEER: I anticipate that the traditional timelines for a drug to reach from lab to market are likely to get shortened as GenAI makes its impact felt through the drug development value chain. Starting from the lab through high-velocity screening of drug candidates to clinical trials where patient recruitment can be upended to generating regulatory documents for submission to auto-generate personalised content for rep/MSL interaction with HCP—each stage will be impacted with GenAI.
Generation and Management: GenAI tools can automate the creation, retrieval, and storage of documents. They ensure that the latest versions of documents are easily accessible, reducing the time workers spend searching for information. This capability is crucial in highly regulated environments where compliance and up-to-date documentation are paramount.
ANDREE BATES: Gen AI will reshape clinical trials in many ways such as faster patient enrolment, synthetic data control arms and digital twin studies, automated data analysis and automated CSR writing. Regulatory submissions will be largely automated and the time from bench to market will be sped up by between 60-70%.
How is the adoption of GenAI affecting the pharma workforce? What new skill sets are required, and how can companies facilitate reskilling and upskilling initiatives?
SAMEER: I do not anticipate the role of humans to ever disappear once GenAI starts getting implemented at a scale that will justify the prom-
WARD serves as the Director of Ask GXP and ServBlock, with a robust background spanning over 14 years in pharmaceutical operations and advanced technology applications. At Ask GXP, John leads the adoption of Generative AI (GenAI) technologies, focusing on transforming processes across pharmaceutical manufacturing and significantly enhancing knowledge management systems. His leadership ensures the strategic integration of GenAI to streamline document generation, automate compliance tracking, and optimise data-driven decision-making processes.
In his role at ServBlock, John harnesses blockchain technology to safeguard data integrity and security within pharmaceutical supply chains, ensuring transparency and reliability.
ise. But the roles will definitely evolve. Let me give you a specific example. Take the case of medical writers; with the use of Gen AI, writers will become subject matter experts (SMEs) and reviewers. While the machine will be able to generate a first draft that is 70 per cent accurate, it will never be able to provide the narrative or tell a story that only a human is capable of. In addition, to guard against implicit bias as well as hallucination, a higher degree of data fact check will be needed.
But there is no doubt that a human + machine combination can be a truly formidable partnership in this field. New skills will be needed that we had never contemplated. Prompt engineering is now a legitimate skill set that is being taught in colleges, just like data scientists did not exist 5 years ago!
JOHN WARD: The shift towards GenAI necessitates a transformation in the skill sets required on the shop floor. The focus moves towards skills in digital literacy, AI management, and data analysis. To support this transition, companies must invest in comprehensive training and development programs that not only focus on technical skills but also emphasise critical thinking and problem-solving in a technologically advanced environment. Learning new skills such as prompt engineering will be crucial for the workforce.
ANDREE BATES: In light of these changes, the emergence of new high-skilled roles will be more significant, with a focus on skills in managing AI systems. People need to be able to understand what is possible and how to manage AI. There will be more focus on human skills such as strategy, communication and others so having a mix of an understanding of how to manage AI and the softer human skills will provide an advantage.
SAMEER: This is a clear opportunity area, for sure. While the Joe Biden administration did release an executive order in late 2023 to establish new standards for AI safety and security, the reality is limited progress has been made around guardrails to drive safe, secure, and trustworthy development of AI. Currently, companies are self-regulating and most of the work has been done on internal-facing documents. In the absence of clear guidelines on copyrights, privacy, security, etc., each company is making its own internal guidelines based on its risk appetite and understanding of the space. This clearly is non-sustainable and needs to be corrected so that clear governance can be established before things get out of hand.
JOHN WARD: As GenAI becomes more integrated into pharmaceutical manufacturing, it's crucial to navigate the associated regulatory and ethical challenges. Ensuring data privacy, securing informed consent for data use, and maintaining data integrity are paramount. Companies must establish clear protocols and work closely with regulatory bodies to ensure their AI solutions comply with all legal and ethical standards.
The future of GenAI in pharmaceutical manufacturing promises significant advancements in productivity, efficiency, and innovation. By embracing these technologies, companies can not only streamline operations but also foster a more dynamic and innovative workplace. The key to successful integration lies in balancing technology with human insight and ensuring ethical use of AI.
John WardANDREE BATES: As AI systems assume increasingly autonomous roles in drug design and discovery, regulatory bodies will confront novel challenges in safeguarding the safety of algorithmically generated therapies. Conventional drug approval protocols hinge upon rigorous clinical trials and full transparency throughout the developmental journey. Nevertheless, the extensive utilisation of vast datasets manipulated in intricate, less intuitive manners by generative AI models may curtail the extent of traceability and comprehensibility. Regulators must thus devise fresh benchmarks to authenticate the safety and effectiveness of AI-derived molecules and research propositions intended for human application, leveraging the existing evidence. Moreover, it’s necessary to devise regulations governing AI systems themselves and ensure adequate oversight when models are updated or retrained over time.
use of GenAI in pharma, particularly regarding privacy, consent, and data security?
SAMEER: There are myriad dimensions of risks and ethical considerations for the responsible use of GenAI. It starts with copyrights as these LLMs have been developed on public sources
of information that in turn have been generated from protected sources. Privacy concerns are justified if we are not able to protect against the release of personal information or data (even if inadvertently). The output data is dependent on the quality of the prompt that is the input. Inherent bias can creep in. The machine could “hallucinate” and cite references that do not exist putting a high burden on data fact-checking. These are just a few!
JOHN WARD: The ethical deployment of Generative AI (GenAI) in the pharmaceutical industry is critically important, particularly concerning the issues of privacy, consent, and data
DR. BATES Has spent over 20 years working specifically in pharma Artificial Intelligence. She brings blended expertise in Artificial Intelligence (AI), Pharmaceuticals, and Strategic Implementation of AI. Dr. Bates has led AI projects for numerous pharmaceutical in clinical trials market access, regulatory, medical affairs, and sales and marketing. (https://www.linkedin.com/in/dr-andree-bates)
security. As we integrate these technologies at AskGXP, we are acutely aware of the responsibilities that come with handling sensitive data and the potential impacts on patients and consumers.
ANDREE BATES: As generative models advance, the establishment of scientifically and legally endorsed protocols for certifying AI-generated pharmaceuticals is anticipated to emerge as a paramount obstacle. Confronting this challenge is imperative to maximise patient access to the transformative potential offered by AI-enhanced drug discovery endeavours.
What advice would you give to pharmaceutical executives and decision-makers looking to leverage GenAI effectively in their organisations?
SAMEER: Cautious optimism, the theme that I started this interview with, is possibly the most practical way to approach the shifts in the landscape that executives and decision-makers are being seized with. Controlled experiments within the confines of their secure environments will allow them to form their own view of what is possible or not, and more importantly the best way to achieve what is possible. Keeping an open mind and preparing their teams for change will allow the scepticism and nervousness to be gradually replaced with an embrace of the possibilities that lie ahead of us!
JOHN WARD: To leverage GenAI effectively, executives should focus on building a robust digital infrastructure and fostering a culture of innovation and agility. This includes setting clear goals for AI integration, ensuring transparency in AI-driven decisions, and maintaining open channels of communication with all stakeholders. Additionally, executives should prioritise ongoing training and development to keep pace with technological advancements.
ANDREE BATES: With an eye on the future, pharmaceutical companies that prioritise enhancing their AI capabilities alongside implementing rigorous governance and oversight mechanisms are positioned to spearhead the industry into an era driven by artificial intelligence. This strategic approach promises to catalyse groundbreaking medical advancements for the global benefit of patients.
The pharmaceutical industry stands at a crossroads, grappling with escalating costs, stagnant pipelines, and the everpresent need for personalised medicine.
Artificial intelligence (AI) emerges as a beacon, offering a transformative toolkit to revolutionise drug discovery, development, and clinical trials. This editorial delves into the multifaceted realm of AI in Pharma, dissecting its current landscape, challenges, and profound implications for drug discovery, patient care, and healthcare equity. Through an exploration of AI’s role in expediting drug development processes, tailoring treatment modalities through personalised medicine, and addressing healthcare disparities, this editorial navigates the intricate intersection of technology and healthcare with precision.
Nirupama Parate, Marketing Specialist, Medicinal Products Division, Freyr Solutionshe pharmaceutical industry faces rising costs, stagnant innovation, and growing demand for personalised medicine, hindering patient access to crucial treatments. However, Artificial intelligence (AI) offers a promising solution, potentially revolutionising drug discovery, development, and clinical trials.
Despite its vital role in healthcare, the pharmaceutical sector struggles with complex challenges. Research & development are expensive and time-consuming, with low success rates and intricate regulations. AI, specifically machine learning (ML), presents a powerful opportunity to address these issues.
By analysing vast datasets, AI and ML can facilitate faster, more precise, and more accessible processes throughout the pharmaceutical pipeline. This includes generating innovative drug candidates, optimising clinical trials, and personalising medicine. These advancements have the potential to unlock significant market growth and improve patient access to lifesaving treatments.
The integration of AI in the pharmaceutical industry is rapidly evolving, transforming various aspects of drug development and healthcare delivery. Here are some highlights:
Exponential Growth: Investment in AI-powered drug discovery startups has skyrocketed, demonstrating the industry's growing confidence and recognition of AI's potential.
Strategic Partnerships: Leading pharmaceutical companies are actively forging partnerships with AI-driven biotech
firms and technology vendors to leverage their expertise and accelerate innovation.
Global Expansion: AI integration in Pharma is not confined to specific regions. There's a growing presence of AI-powered startups and initiatives across the globe, fostering international collaboration and knowledge sharing.
Drug Discovery and Development:
Target Identification: AI algorithms analyse vast datasets to identify potential drug targets associated with specific diseases
Lead Generation and Optimisation: AI helps design and optimise new drug candidates by simulating their interactions with biological systems
Repurposing Existing Drugs: AI can identify new applications for existing drugs, potentially accelerating the development process for new indications.
Clinical Trials:
Patient Selection: AI can analyse patient data to identify individuals most likely to respond to a specific drug, leading to more efficient trials
Virtual Trials and Simulations: AI-powered simulations can model potential drug effects, reducing the need for some traditional clinical trial phases and accelerating the process.
Real-Time Monitoring and Risk Assessment: AI can monitor patient data during trials in real-time, allowing for early detection of potential safety issues.
Manufacturing and Supply Chains:
Predictive Maintenance: AI can predict equipment failures, preventing costly downtime and ensuring consistent production quality.
Process Optimisation: AI can analyse production data to identify inefficiencies and optimise manufacturing processes for increased efficiency and cost savings.
Personalised Drug Delivery: AI-powered systems can personalise drug delivery methods and dosage regimens based on individual patient needs.
While AI in pharma presents numerous challenges and risks, it also offers unprecedented opportunities for innovation, improvement, and transformation across the drug development lifecycle. By addressing regulatory hurdles, ethical considerations, and technical limitations, pharmaceutical companies can harness the full potential of AI to revolutionise drug discovery, personalised medicine, and healthcare delivery, ultimately improving patient outcomes and advancing public health.
Regulatory Ambiguity: The current regulatory frameworks may lack the necessary provisions to effectively evaluate and authorise AI-driven processes in drug discovery and development. Establishing precise and adaptable regulations is imperative to promote innovation while upholding standards of safety and efficacy.
Data Confidentiality and Security: Safeguarding patient data ethically and securely is of utmost importance. Implementing robust data governance protocols and stringent security measures is essential to prevent misuse and safeguard patient privacy.
Algorithmic Bias: AI algorithms have the potential to perpetuate biases inherent in their training data, leading to unfair and discriminatory outcomes. Addressing bias through diverse datasets and conscientious development practices is critical.
Transparency and Explainability: A comprehensive understanding of how AI algorithms reach their conclusions is essential for instilling trust and ensuring responsible deployment. Developing interpretable and transparent AI models is indispensable for fostering transparency and accountability. (Table:1)
Data quality and accessibility are crucial for robust AI models.
Incomplete, biased, or poor-quality data can lead to inaccurate predictions and suboptimal outcomes.
Explainable AI models are essential for building trust, regulatory approval, and clinical adoption. Lack of model interpretability hinders understanding the rationale behind AI-generated recommendations.
Adversarial attacks, data perturbations, and domain shifts can compromise AI models.
Rigorous testing and validation are necessary to ensure robustness against potential threats.
AI is a tool, not a replacement for human expertise and judgment.
Overdependence can lead to overlooking crucial aspects and undermining human decision-making.
AI automation may disrupt employment in specific industry segments.
Reskilling and upskilling strategies are vital to address ethical concerns and workforce adaptation needs.
Significant investment and expertise are required for robust AI infrastructure.
Data quality, technical constraints, and ongoing maintenance pose persistent challenges.
Opportunities for innovation and improvements
Enhanced Drug Discovery: AI expedites the discovery of novel drug candidates through predictive modelling, virtual screening, and structure-based drug design methods. Integration of AI into high-throughput screening assays and phenotypic profiling platforms facilitates swift identification of lead compounds with therapeutic promise.
Precision Medicine: AI personalises treatment through genomic, proteomic, and clinical data analysis. Predictive modelling and machine learning empower: (Figure:1)
Biomarker identification
Patient stratification
Pinpointing key indicators of disease and treatment response. Grouping individuals with similar characteristics for targeted therapies.
Treatment response prediction
Anticipating individual responses to different treatments.
These capabilities pave the way for precision medicine, tailoring treatments to each patient's unique needs.
Real-world Evidence Generation: AI leverages real-world data (electronic health records, wearables, patient reports) to gain insights into disease progression, treatment effectiveness, and patient outcomes. This real-world evidence complements clinical trial data, offering valuable insights into drug safety, effectiveness, and comparative performance in real-world settings.
Patient Engagement and Empowerment: AI-powered technologies like chatbots, virtual assistants, and remote monitoring solutions improve patient engagement, education, and selfmanagement. These tools Deliver personalised health insights, enable proactive interventions, and provide support resources. Through this collaborative approach, AI fosters better patientprovider partnerships, leading to improved health outcomes and satisfaction.
The drug discovery and development continuum entails a series of intricate phases. Historically, these stages have been labour-intensive, expensive, fraught with risk, and governed by stringent regulations. (Figure:2)
Current drug discovery methods face substantial limitations. Random screening, despite massive compound testing, yields few relevant results. Rational drug design, while utilising biotarget structures, often misses intricate biological interactions. Combinatorial chemistry, while generating vast compound libraries, produces numerous irrelevant options.
1 2 3 4 5
AI and ML address these challenges through data-driven approaches. They facilitate novel drug discovery, streamline testing, and enable:
Adaptive clinical trials: Advanced algorithms adapt trial designs dynamically
Synthetic controls: These controls enhance trial efficiency and reduce costs
Real-world evidence integration: Real-world data informs decision-making.
Furthermore, AI and ML revolutionise drug marketing and post-launch monitoring through:
Predictive modelling: This allows for targeted marketing and resource allocation
Reinforcement learning: This optimises post-launch activities based on real-world feedback.
AI and ML integration marks a transformative shift in pharmaceuticals, offering unprecedented efficiency, precision, and effectiveness in drug discovery and development.
AI and ML are revolutionising drug discovery, a pivotal phase in the drug development lifecycle. This phase encompasses multifaceted processes like target identification, lead generation, lead optimisation, and preclinical assessments, all essential for selecting viable candidates for clinical trials. AI and ML introduce unprecedented efficiency, accuracy, and velocity to these stages through data-centric approaches.
Target Identification:
AI and ML employ advanced techniques to identify potential drug targets and signalling pathways associated with disease mechanisms. Analysing extensive datasets across genomics, proteomics, metabolomics, and transcriptomics, AI algorithms pinpoint therapeutic targets with remarkable precision. For example, some companies utilise deep learning to model the molecular impacts of genetic variations, offering insights into their effects on gene expression, splicing, translation, and protein structure. These capabilities are invaluable for identifying targets relevant to genetic disorders and precision medicine applications.
Lead Generation:
The integration of AI in lead generation is reshaping the conventional drug discovery paradigm. Using deep learning, some entities predict the binding affinities of small molecules to specific proteins based on their three-dimensional structures. These predictions significantly enhance the generation of promising drug leads across diverse disease areas.
Lead Optimisation:
AI and ML extend beyond target identification to provide predictive models for optimising drug candidate properties. Employing a combination of knowledge graphs, machine learning models, and reasoning algorithms, companies refine drug leads based on criteria such as efficacy, safety, and pharmacokinetics. This holistic approach elevates the quality and diversity of candidates progressing to clinical trials.
Preclinical Testing:
AI plays a significant role in preclinical testing as well. Certain pharmaceutical companies leverage computer vision and deep learning techniques to interpret high-throughput cellular imagery. This enables comprehensive analysis of the phenotypic effects of drug candidates on various cell types, facilitating robust evaluations of efficacy and toxicity in preclinical settings.
Clinical trials are a core component of drug development, evaluating new medications in humans for safety and efficacy. They follow a structured progression: (Figure:3)
Strict protocols, regulations, and ethical oversight are paramount throughout all clinical trial phases. Innovative approaches rooted in Artificial Intelligence (AI) and Machine Learning (ML) offer avenues to enhance and streamline the clinical trial process. Leveraging adaptive designs, synthetic controls, and real-world evidence methodologies, AI and ML applications manifest in several key areas:
Adaptive Designs: AI and ML enable clinical trial designs to adapt to incoming data, adjusting parameters such as sample size and randomisation in a statistically robust manner. Platforms utilising causal machine learning optimise adaptive trial designs, tailoring treatments precisely.
Synthetic Controls: AI and ML create synthetic control groups mirroring actual cohorts using historical or external data. Employing deep learning, platforms generate synthetic patient data, reducing sample size and trial duration while enhancing result reliability.
Real-World Evidence: AI and ML augment clinical trial data with information from electronic health records, wearable devices, and social media. Automation via natural language processing and machine learning yields timely and comprehensive evidence for trials.
As the trajectory of AI takes shape, the potential for enhancing patient care and propelling personalised medicine into new frontiers appears boundless. However, it is imperative to confront ethical considerations encompassing data privacy, algorithmic bias, and equitable access to AI-driven health -
Focuses on safety, assessing safe dosage ranges in a small number of participants.
Evaluates efficacy and side effects in a larger group of patients with the target condition.
Compares the new treatment against standard care or placebo, confirming effectiveness and safety in a much larger and diverse patient population.
Post-marketing monitoring detects long-term effects and potential interactions in realworld use.
care solutions. Through responsible and ethical utilisation of AI capabilities, we can forge a future where comprehensive, efficient, and individualised healthcare is accessible to all.
AI-driven patient monitoring and diagnosis:
Real-time Surveillance: AI algorithms exhibit the capability to scrutinise physiological data derived from wearable gadgets or electronic health records (EHRs), facilitating the early detection of potential health anomalies, and enabling timely interventions for enhanced patient outcomes.
Automated Image Interpretation: AI-enabled tools excel in scrutinising medical images like X-rays and MRIs, surpassing conventional methods in accuracy and efficiency, thereby expediting diagnoses with heightened precision.
Chatbots and Virtual Assistants: AI-driven chatbots and virtual assistants extend patients round-the-clock access to information, offering responses to basic inquiries and facilitating appointment scheduling, alleviating healthcare provider workload while augmenting patient contentment.
Tailored treatment plans and precision medicine:
Personalised Treatment Strategies: AI utilises individual patient data, including genetic profiles, medical histories, and lifestyle attributes, to predict responses to various treatments, enabling tailored treatment plans that enhance efficacy and minimise side effects.
This technology, capable of creating new data points based on existing data, can be used to generate novel drug candidates with desired properties, accelerating the discovery process.
This collaborative learning approach allows training Al models on distributed datasets without compromising patient data privacy, fostering collaboration while addressing ethical concerns
Drug-drug interaction prognosis: AI scrutiny of a patient's medication records enables the anticipation of potential drug interactions, furnishing healthcare practitioners with informed decision-making insights concerning treatment regimens and mitigating associated risks.
Dosage optimisation: AI analysis of patient data coupled with clinical trial findings allows for the customisation of medication dosages, delivering optimal therapeutic effects while mitigating the likelihood of adverse reactions.
Risk prediction: AI-driven analysis of extensive patient datasets facilitates the identification of individuals predisposed to specific ailments, thus enabling early interventions and preventive measures.
Personalised health recommendations: AI scrutinises an individual's health metrics and lifestyle facets to furnish personalised suggestions regarding dietary patterns, exercise routines, and preventative screenings, empowering individuals to adopt a proactive stance toward their well-being.
Population health management: AI delves into data spanning entire demographics to unveil trends and patterns, empowering healthcare systems to allocate resources judiciously and target interventions toward the most susceptible segments of the populace.
This powerful computing paradigm has the potential to simulate complex biological processes and molecular interactions, leading to more rational drug design and personalised medicine approaches.
With XAI techniques, researchers can gain insights into how Al models arrive at their predictions, building trust and transparency in Al-powered drug development processes.
Al will play an even more pivotal role in identifying novel drug targets, optimising drug design, and predicting potential safety and efficacy, leading to a faster and more efficient drug development pipeline.
By analysing individual patient data, Al will enable the creation of personalised treatment plans, tailoring medications and dosages to individual needs, and maximising therapeutic benefits.
Al will facilitate virtual trials, streamline patient selection, and enable real-time monitoring, reducing costs and accelerating the clinical trial process while ensuring patient safety.
Al will optimise production processes, predict equipment failures, and personalise drug delivery methods, leading to increased efficiency, reduced costs, and improved drug accessibility.
The pharmaceutical industry stands at a pivotal juncture, with the transformative potential of Artificial Intelligence (AI) poised to revolutionise various facets of drug development, clinical trials, and healthcare delivery. As stakeholders navigate the challenges and opportunities inherent in this dynamic landscape, Freyr's Freya emerges as a valuable tool offering intuitive access to regulatory information. Empowering researchers, developers, and regulatory teams, Freya enables them to easily navigate complex regulations, prioritise innovation, and stay informed and up to date. Looking ahead, the future of AI in Pharma holds promise for improved healthcare outcomes and global health advancement. By embracing innovation responsibly, fostering collaboration, and prioritising ethical considerations, we can harness AI's power to create a future where everyone has access to safe, effective, and personalised healthcare solutions. Freya serves as a testament to AI's potential, facilitating the pharmaceutical industry's navigation of regulatory complexities and unlocking AI's full benefits for the benefit of patients worldwide.
References are available at www.pharmafocusasia.com
Nirupama Parate, an accomplished Marketing Specialist within Freyr Solutions' Medicinal Products Division, brings a unique blend of expertise in pharmaceutical technology and a passion for innovation to the forefront. Armed with an MBA in Pharmaceutical Tech, she possesses extensive experience spanning various sectors of the pharmaceutical and medical devices industries.
Her professional journey encompasses roles in Regulatory Affairs, pharmaceutical marketing and communications, and innovation in pharma business. With a firm belief in going above and beyond, Nirupama is committed to infusing every task with a personal touch, ensuring excellence in every endeavour.
The intersection of Artificial Intelligence (AI) and personalised medicine is transforming the landscape of pharmaceutical systems by improving the precision of drug delivery. Precision medicine, which focuses on patient phenotypes with diminished treatment responses, is significantly enhanced by AI's advanced computational models. This integration provides physicians with valuable tools for rapid and improved diagnosis and disease management. In the current landscape, AI is effectively addressing challenges associated with both genomic and nongenomic determinants. It aids in collecting information on disease symptoms, clinical history, and lifestyle factors, thereby facilitating personalised diagnosis and prognostication.
Sumel Ashique, Mithun Bhowmick Department of Pharmaceutical Sciences, Bengal College of Pharmaceutical Sciences & ResearchThe healthcare industry is undergoing a significant shift towards the adoption of precision medicine. Recent advancements in biomedical research have led to an increased reliance on big data and precision medicine. This has created a substantial demand for the integration of machine learning (ML) and
systems genomics algorithms, working in conjunction with multiomics data, to assess potential phenotype-genotype associations. Differences in responses to specific medications or treatment strategies within a population can be attributed to variant genetic and expression profiles. Computational biology has progressed
significantly, enabling the analysis and functional annotation of extensive biological datasets. AI in biology plays a crucial role in identifying patterns between functional annotations, allowing for the construction of a functional hologram from diverse data types. AI has shown promise in augmenting the capabilities of healthcare specialists, offering support in tasks ranging from diagnostics to treatment planning. The use of AI can help mitigate human limitations, such as fatigue and inattention, and address the risks associated with human error in medical decision-making. By leveraging machine learning algorithms and advanced data analysis, AI systems can process vast amounts of medical data quickly and accurately, leading to improved diagnostic accuracy and personalised treatment options. Successful adoption of AI in healthcare relies on three key principles: data and security, analytics and insights, and shared expertise. The importance
of data and security lies in establishing full transparency and trust in how AI systems are trained and the information used for their training. As humans and AI increasingly collaborate, trust in the output of these systems becomes essential. It's worth noting that AI is not the sole data-driven field influencing healthcare; precision medicine, with a history of over a decade, holds an equal or even greater impact on the trajectory of healthcare. (Figure 1)
The integration of AI and precision medicine is playing a pivotal role in addressing complex challenges within personalised healthcare. A notable application involves genomic considerations in tailoring therapy plans. Patients harbouring pharmacogenomically actionable variants may necessitate adjusted prescription or dosing. The groundbreaking aspect lies in genome-informed prescribing, showcasing the scalability of precision medicine. The real-time effectiveness of recommendations relies on the development of machine-learning algorithms capable of predicting which patients may require
medication based on genomic information. This early use case exemplifies the synergy between AI and precision medicine, where AI techniques prove invaluable for efficient and high-throughput genome interpretation. The key to personalising medications and dosages is preemptive genotyping, ensuring the availability of genomic information when needed. Some advantages provides by AI in Precision medicine are
(i) AI analyses large datasets, including genomic information, clinical records, and other relevant data, to identify patterns and correlations. This enables the development of personalised treatment plans tailored to the unique genetic and molecular characteristics of each patient.
(ii) AI accelerates drug discovery by analysing vast datasets to identify potential drug candidates and predict their efficacy. This speeds up the drug development process, reduces costs, and increases the likelihood of finding targeted therapies for specific genetic or molecular profiles.
(iii) AI is instrumental in analysing genomic data to identify genetic variations associated with diseases. This infor-
mation can be used to understand the genetic basis of diseases, predict susceptibility, and design targeted therapies.
AI and Personalised medicine
Artificial Intelligence (AI) plays a significant role in advancing personalised medicine, a medical approach that tailors healthcare decisions and interventions to individual patient characteristics. Here are some ways in which AI is being utilised in personalised medicine:
1. Data Analysis and Integration
Genomic Data Analysis: AI is used to analyse vast amounts of genomic data to identify genetic variations associated with diseases or drug responses.
Integration of Multi-Omics Data: AI can integrate data from various sources, such as genomics, proteomics, and metabolomics, to provide a comprehensive understanding of an individual's health.
2. Disease Prediction and Diagnosis
Machine Learning Models: AI algorithms can predict disease risk by analysing patient data, family history, and genetic information.
Image Analysis: AI is employed for image-based diagnosis, such as interpreting medical imaging data like MRI, CT scans, and pathology slides to aid in early disease detection.
3. Drug Discovery and Development
Target Identification: AI helps identify potential drug targets by analysing biological data and understanding disease pathways.
Drug Repurposing: AI can identify
existing drugs that may be repurposed for new therapeutic uses, accelerating drug development.
4. Treatment Personalisation
Predicting Treatment Response: AI models analyse patient data to predict how an individual will respond to specific treatments, helping clinicians choose the most effective therapies.
Dosing Optimisation: AI can assist in determining the optimal dosage of
medications based on an individual's characteristics, improving treatment outcomes.
5. Clinical Trial Optimisation
Patient Recruitment: AI helps identify suitable candidates for clinical trials based on specific criteria, speeding up the recruitment process.
Trial Design: AI optimises clinical trial designs, ensuring more efficient and targeted research.
Figure 2: Role of AI in précised and personalised medicine Pandya et al., 2021; This article is an open access article distributed under the terms and conditions of the Creative Commons Attribution (CC BY) license (https://creativecommons.org/licenses/by/4.0/).
Wearable Devices: AI analyses data from wearable devices to monitor patients in real-time, providing continuous feedback to healthcare providers about an individual's health status.
Remote Patient Monitoring: AI facilitates remote monitoring of patients, enhancing personalised care and reducing the need for frequent hospital visits.
7. Privacy and Security
Secure Data Handling: AI is employed to ensure the secure handling and analysis of sensitive patient data, addressing privacy concerns associated with personalised medicine.
8. Patient Engagement
Personalised Treatment Plans: AI assists in creating personalised treatment plans, helping patients better understand and engage in their healthcare decisions.
Health Risk Communication: AI tools can communicate health risks and potential interventions in a way that is easily understandable to patients. (Figure 2)
Challenges in using AI in precision & personalised medicine
Efforts to integrate AI into precision medicine for tasks like disease diagnosis, risk prediction, and treatment response have shown promise in experimental settings. However, the true impact of AI on healthcare is not fully realised. The success of translating an AI system into a practical application depends not only on accuracy but also on its ability to function reliably, safely, and in a generalisable manner. There are concerns that AI models trained on biased data may perpetuate and exacerbate existing biases, leading to unfavourable decisions for certain demographic groups. Additionally, the performance and clinical efficacy of AI models can be influenced by environmental factors and specific workflows in real-world deployment. Ensuring the quality and standardisation of health data is crucial to the effective and responsible applica-
tion of AI in healthcare. In the realm of personalised medicine, the integration of diverse data sources, including electronic health records (EHRs), genomic data, and lifestyle information, presents a formidable challenge. This difficulty arises from the diverse formats, structures, and varying quality of these datasets. To ensure the reliability of AI predictions, it becomes imperative to standardise and clean these datasets thoroughly. Moreover, the ethical dimensions associated with employing AI in personalised medicine raise pertinent concerns. Issues such as obtaining informed consent, ensuring transparency, and mitigating potential biases in algorithms must be carefully addressed. Ensuring trust in personalised medicine applications requires transparent communication with patients about data utilisation and a dedicated effort to identify and rectify biases in AI algorithms. The intricate nature of personalised medicine, driven by complex algorithms and models, may not neatly align with current regulatory frameworks. The process of obtaining regulatory approval for AI-driven personalised medicine applications is often arduous and time-consuming. Ongoing efforts are underway to establish standards for the validation and approval of AI algorithms in this evolving field.
Artificial intelligence has the potential capability to analyse large amounts of population data, learn from several patterns, and can make accurate predictions which enhance personalised medicine in the healthcare system. The application of AI in personalised and precision medicine offered a great future where healthcare is more efficient, safe, and mainly tailored to the distinctive needs of each individual. The effective application of AI in health and medicine offers cost effectiveness and also value-adding augmentation of human capabilities. The error free accurate prediction of AI in the health system has improved the value of life in a greater way among much of the population.
Conflict of Interest
None
Funding Not applicable
Keywords:
Artificial Intelligence, precision drug delivery, personalised medicine
References are available at www.pharmafocusasia.com
Sumel Ashique has been working as an assistant professor in Bengal College of Pharmaceutical Sciences & Research, Durgapur 713212, West Bengal, India. He has 3.5 years of teaching experience. He has achieved 50+ publications of International and national accredited reputed journals (Scopus, UGC). He has knowledge in drug delivery, nanotechnology and targeted treatment strategy.
Prof. (Dr.) Mithun Bhowmick (M.Pharm, Ph.D.) is working as Professor and Principal in Bengal College of Pharmaceutical Sciences & Research, Durgapur (WB). He has received many prestigious awards. He has participated and presented many research papers in various International & National conferences. He has published more than 50 research & review papers in National and International journals. He has many Australian, German and Indian Patents, Design Patents and Copyrights in his credits. He is a reviewer of many pharmaceutical Journals.
When artificial intelligence (AI), machine learning (ML), and blockchain are used cohesively and correctly, they could ameliorate the existing complexity, inefficiency, lengthy, and costly drug discovery to market. Here we will address a few executive-level questions within the context of these rapidly growing disruptive techs in drug development.
Frank Leu, Founder and Managing Member, BioPharMatrix LLCThe drug development process, from discovery to market, is an overly complex, inefficient, lengthy, and costly journey that involves multiple phases of research, development, and regulatory reviews. This process consists of four major steps, with each step having significant internal and external inefficiencies that could potentially be best addressed using disruptive techs. The costliest, while not revenue generating, and most effort-intensive in validating a drug’s usefulness are the first two: discovery to preclinical testing (1-6 years) and clinical trials (6 to 7 years). Discovery involves first the identification of potential targets and compounds. High-throughput screening, arti ficial intelligence (AI), machine learning (ML), and blockchain, collectively referred to as the “disruptive techs” and meth ods can accelerate this phase and move to the preclinical examination of the candidate via tests on cell cultures and animals to assess the safety
and biological activity. This phase is critical as it determines whether a compound is safe for human testing while demonstrating efficacy in at least two animal models.
The next step would be clinical trials, commonly conducted in three major phases:
Phase I: Test a drug on a small group of people (20-100) to evaluate its safety, determine a safe dosage range, and identify side effects.
Phase II: The drug is given to a larger group of people (100-300) to see if it is effective and to evaluate its
Conducted on large groups of people (1,000-3,000) to confirm its effectiveness, monitor side effects, compare it to commonly used treatments, and collect information that will allow the drug to be used safely.
Once the candidate is deemed to be safe enough and effective by the developer for its intended disease indication, it moves on to the third step, which is to seek regulatory review by submitting a New Drug Application (NDA) or Biologics License Application (BLA) with the intent to seek approval to market by the Food and Drug Administration (FDA) and its similar agencies around the world. The final and seemingly perpetual step is post-market surveillance, as the drug of interest continues for ongoing monitoring for any adverse effects that may not have been detected
Decentralised, immutable, and trustless scientific and medical data architecture using blockchain serves as the foundation for the best use of Artificial Intelligence in drug development.
What is the cost estimated for a drug from discovery to market without fullscale implementation of disruptive technologies, such as AI, ML, and blockchain?
The cost of developing a new prescription medicine that gains marketing approval is estimated to be over US$2.5 billion, according to a study by the Tufts Center for the Study of Drug Development. This figure includes the cost of most drug candidates that fail during development (the cost of failure is factored into the overall cost of successful drug development). It's important to note, however, that this is an estimated average and can vary widely depending on the drug, the disease it targets, the complexity of clinical trials, and the regulatory path it follows. The estimated cost of US$2.5 billion encompasses discovery, preclinical development, clinical trials, and the regulatory submission process. It also includes opportunity costs (the cost of capital) and the costs associated with research and development activities that do not lead to a successful new medicine. As one can imagine, the
rampant inefficiencies in the current drug developmental process can be minimised or eliminated by using disruptive techs.
In general, how could the implementation of disruptive technologies such as AI, ML, and blockchain enhance the process of
Many major factors often affect the timeline and cost during a legacy drug development process. Adopting AI, ML, and blockchain could potentially reduce significant amounts of time and cost by facilitating the identification of promising drug candidates more efficiently and enhancing the design of clinical trials. These disruptive techs can be used to directly tackle novel drug mechanisms and to properly reduce the complexity of the process. Next, the same techs could also be used to comply with an ever-changing regulatory environment, which would then reduce the duration and cost of the clinical trials. Ultimately, they can improve clinical trial outcomes and reduce the need for any unnecessary additional clinical trials.
Blockchain ensures the integrity and immutability of research data, clinical trial results, and manufacturing records, preventing data tampering, and enhancing trust; accomplished through the improvement of data integrity and immutability. Through the application of AI and ML drug companies could quickly and intelligently analyse vast datasets to identify potential drug candidates much faster than traditional
methods, significantly reducing the time and cost of the discovery phase. With good blockchain-encapsulated data blocks, this high-confidence information can be used by AI and ML to predict how drugs will interact with specific targets in the body, helping scientists understand the potential efficacy and safety of a drug before it goes to clinical trials, which would be a much-improved understanding of drug-target interaction. Additionally, by implementing the fitting AI algorithms in their drug design, pharma companies can derive novel drug molecules with desired properties and predict their synthesis paths, streamlining the drug design process by leaning toward in silico. Furthermore, ML models can be deployed here and used to analyse historical data to predict the outcomes of clinical trials, identify the most promising drug candidates, and optimise trial designs to improve drug candidates’ success rates.
Disruptive techs enable secure and transparent sharing of research data and intellectual property among multiple relevant stakeholders, fostering real-time collaboration while protecting proprietary information on a need-to-know basis. Can blockchain improve the management of intellectual property in drug development? Yes, it provides a secure and transparent platform to register and manage intellectual property rights, facilitating licensing and collaboration. Furthermore, algorithmic approaches such as natural language processing (NLP) can be used by AI and ML to extract relevant data and information from collaborators’ databases and documentation, public scientific literature, and pre-clinical and clinical
reports, automating and expediting the research process and identifying new drug candidate opportunities.
Blockchain can first facilitate patient consent and data sharing by securely managing and simultaneously verifying consent, this allows stakeholders, such as sponsors, hospitals, CROs, and patients to control their data during clinical trials. This is accomplished by providing stakeholders with complete control down to the individual level (i.e. patient), with enhanced transparency, trust, and engagement during clinical trials. Subsequently, the Al algorithms and ML models can be used to analyse historical and real-time collected clinical data, identifying and designing the most promising drug candidates and optimising the best trial design fitting to determine candidate drugs’ efficacy accurately.
Blockchain could be used to capture and facilitate patient consent and personal/medical data during a clinical trial, hence allowing the managing and sharing of data in a secure and verifiable manner. This allows patients to have control over their data and provide direct consent to participate in any clinical trial. AI and ML could then be applied to analyse patients’ genetic data and other biomarkers to develop personalised drug regimens with precision, ensuring higher efficacy with fewer side effects, possibly to achieve the best outcome in a personalised manner.
Blockchain can ensure that clinical trial and medical data are captured and stored according to ethical guidelines, with patient consent recorded in realtime in a trustless (meaning with the highest trust) manner with immutability, thus forging best ethical clinical testing practices. This enables downstream processing of the clinical data to be organised for regulatory compliance. Essentially, blockchain can securely store and manage regulatory submissions and communications with high fidelity, ensuring traceability and thus compliance with the best regulatory standards. Equipped with these highfidelity data compiled using blockchain, AI and ML then can be deployed to best analyse personalised clinical data with precision to identify disease markers, track disease progression, and evaluate potential drug efficacy. Simultaneously, adverse drug reactions and interactions are also closely monitored, ultimately enhancing drug safety for patients.
How could disruptive techs help to maintain the integrity
of the management of the supply chain and optimise the
Blockchain's traceability features can verify the authenticity of drugs at every step of the supply chain, which can significantly reduce the prevalence of counterfeit drugs. This is accomplished by providing a transparent and secure way to track the production, distribution, and delivery of pharmaceuticals. Subsequently, AI and ML
could then be used to optimise drug manufacturing processes by predicting and controlling the parameters required for best drug manufacturing processes to ensure quality, reduce waste, and lower costs via implementing on-demand production.
Despite the apparent usefulness of disruptive techs, the challenge that remains is the proper and consensus integration of blockchain techs throughout drug development. Technical complexity, scalability, regulatory acceptance, and the need for industry-wide standardisation are significant challenges that must be addressed before these techs can become useful. Human resistance, the fear of being replaced and the cost of replacing legacy systems also create a chasm in their adoption. However, the cost of not immediately devising a plan to adopt will be devastating for late adopters. For example, once the blockchain, AI, and ML plans are readying to integrate, not only will
they cut tremendous costs to facilitate drug development, but also add tremendous value to reliably analyse market data, patent information, and competitive landscape to predict the commercial viability of drug candidates.
In conclusion, a major bottleneck for adopting disruptive techs in an organisation is the actual commitment to bringing drug development into the future. The decision to adopt is not “if”, rather it is only a matter of “when”. When Steve Jobs saw the graphic interface at Xerox, he knew that technology would best serve the future of personal computing. But his executives told him, “We can’t do that. It would blow up the company.” Jobs replied, “Better we should blow it up than someone else.” When your entire business model is challenged and under great duress in the face of AI, ML, and blockchain revolution, would you be willing to blow up your legacy system to survive and out compete
your competitors? If you have any doubts, the history of technological disruption has provided valuable lessons.
Frank Leu is the Founder and managing member of BioPharMatrix LLC, providing intelligence in the areas of innovative processes and platforms for biotech, pharmaceutical, and life sciences. Frank has combined around a hundred speaking presentations and publications on disruptive technologies and processes in drug development.
Year of Publication: 29 February 2024
No of Pages: 320
Book Description: AI and Machine Learning Impacts in Intelligent Supply Chain is a groundbreaking book that offers a comprehensive solution to the challenges posed by the Industry 4.0 revolution. This book is your indispensable guide to navigating the intricate world of supply chain digital transformation using innovative technologies. It provides real-world examples and insights that illustrate how AI and ML are the keys to solving complex supply chain problems, from inventory management to route optimisation and beyond. Whether you are an academic scholar seeking to delve into the impact of AI and ML on supply chain management or a business leader striving to gain a competitive edge, this book is tailored to meet your needs. It bridges the gap between theory and practice, offering a roadmap to success in the era of intelligent supply chains.